High-Quality Sleep Mitigates ABCA7-Related Generalization Deficits in Healthy Older African Americans
Abstract
Background:
Both sleep deficiencies and Alzheimer’s disease (AD) disproportionately affect older African Americans. Genetic susceptibility to AD further compounds risk for cognitive decline in this population. Aside from APOE ɛ4, ABCA7 rs115550680 is the strongest genetic locus associated with late-onset AD in African Americans. While sleep and ABCA7 rs115550680 independently influence late-life cognitive outcomes, we know too little about the interplay between these two factors on cognitive function.
Objective:
We investigated the interaction between sleep and ABCA7 rs115550680 on hippocampal-dependent cognitive function in older African Americans.
Methods:
One-hundred fourteen cognitively healthy older African Americans were genotyped for ABCA7 risk (n = 57 carriers of risk “G” allele; n = 57 non-carriers), responded to lifestyle questionnaires, and completed a cognitive battery. Sleep was assessed via a self-reported rating of sleep quality (poor, average, good). Covariates included age and years of education.
Results:
Using ANCOVA, we found that carriers of the risk genotype who reported poor or average sleep quality demonstrated significantly poorer generalization of prior learning—a cognitive marker of AD—compared to their non-risk counterparts. Conversely, there was no genotype-related difference in generalization performance in individuals who reported good sleep quality.
Conclusion:
These results indicate that sleep quality may be neuroprotective against genetic risk for AD. Future studies employing more rigorous methodology should investigate the mechanistic role of sleep neurophysiology in the pathogenesis and progression of AD associated with ABCA7. There is also need for the continued development of non-invasive sleep interventions tailored to racial groups with specific AD genetic risk profiles.
INTRODUCTION
Sleep is a vital physiological process that is associated with a number of health benefits including metabolic and hormonal equilibrium [1]; enhanced adaptive immunological function [2]; synaptic homeostasis [3]; glymphatic clearance [4]; and improved memory encoding and consolidation [5, 6]. Sleep deficiencies include poor sleep quality, irregular timing of sleep, and extreme (too long or short) sleep duration [7]. From a public health perspective, the prevalence of sleep deficiencies has increased in recent decades [8, 9]. Furthermore, sleep deficiencies contribute to cognitive dysfunction, cognitive decline and Alzheimer’s disease (AD) development [10, 11]. Fortunately, sleep is potentially a modifiable risk factor for cognitive decline and dementia [10].
On the other hand, genetic susceptibility to AD, while not modifiable by individual behaviors, may interact with lifestyle factors such as sleep, to influence cognitive function and AD development [12–14]. The APOE ɛ4 allele, the most well-known genetic risk factor for AD, appears to interact with sleep quality on AD risk [15]. Lim and colleagues [15] found that better sleep consolidation (i.e., less fragmented sleep and hence, better sleep quality) attenuated the deleterious effects of the APOE ɛ4 allele on incident AD risk in a prospective longitudinal cohort study of predominantly white older adults. As such, genetic susceptibility loci may influence the sleep-AD relationship.
ABCA7, or adenosine triphosphate (ATP)-binding cassette member 7, is also a risk locus associated with late-onset AD [16]. In brief, ABCA7 is involved in amyloid precursor protein transport, cellular Aβ clearance, and lipid metabolism [17–19], three processes implicated in late-onset AD. Although originally identified in European samples as an AD risk factor [20, 21], several genome-wide association studies detected that ABCA7 single-nucleotide polymorphism (SNP) rs115550680 confers a higher risk among African Americans than most other genetic loci identified in non-Hispanic whites [22, 23]. In particular, ABCA7 rs115550680 is associated with about a 75% increase in risk for African Americans [24]. Similarly, a 44-base pair frameshift deletion in ABCA7 rs115550680 was associated with AD in African Americans [25]. This base-pair deletion is relatively rare in non-Hispanic whites as compared to people of African ancestry [25]. Overall, accumulating evidence demonstrates that ABCA7 rs115550680 confers greater AD risk among African Americans as compared to European Americans [22, 23, 25]. Furthermore, we have shown in a previous study that older African American carriers of the ABCA7 rs115550680 high-risk “G” allele demonstrate poorer generalization of prior learning [26], a cognitive marker of prodromal AD [27].
This is particularly alarming given that African Americans are among the most vulnerable demographic groups to both sleep deficiences and AD. For example, African Americans have a higher prevalence and severity of sleep disordered breathing and report lower sleep satisfaction, more daytime sleepiness, and more difficulty falling asleep as compared to white Americans [28, 29]. Further, African Americans show a higher incidence of shorter sleep duration as compared to white Americans [9]. In parallel, African Americans also bear a disproportionate burden of AD as compared to the general population, with two to three times the overall risk for dementia [30]. While the reasons for these sleep and cognitive health disparities are not fully understood, common candidate factors include exposures across the lifespan such as educational opportunity differences and disparate socio-environmental circumstances [7, 30, 31].
Given the links between sleep and cognition, widening sleep health disparities and an individual’s genetic predisposition to AD may exacerbate cognitive health disparities. Although these factors independently influence cognitive health in late life, we know too little about the interplay between sleep and AD genetic risk on cognitive function within populations at elevated risk for both cognitive and sleep disorders, such as African Americans.
Purpose
The purpose of the present study was to investigate the interaction between sleep and ABCA7 rs115550680 on cognitive function in older African Americans. We assessed sleep via a self-reported rating of sleep quality, a dimension of sleep deficiencies [7]. Because sleep is implicated in memory consolidation, previous sleep-cognition research has primarily focused on hippocampal function. The current study focused on a cognitive outcome also subserved by the hippocampus, generalization, the ability to apply previously learned rules to new contexts [27]. Developed from non-verbal conditioning paradigms, this task is usedful among populations with a broader distribution of education quality and quantity, including older, urban-residing, underrepresented populations in biomedical research [32]. Our previous studies have shown that generalization is a sensitive marker of prodromal AD and AD risk, long before explicit memory consolidation deficits arise [27, 33–35].
MATERIALS AND METHODS
Participants in this study were selected from a larger parent study, Pathways to Healthy Aging in African Americans, focused on understanding links between genetics, cognition, health, and lifestyle variations in older African Americans residing in Greater Newark, NJ. Community engagement and recruitment methods are described in more detail elsewhere [36, 37].
Individuals were eligible to participate if they identified as African American or Black and were at least 60 years old. Initial screening took place over the phone. Candidates were excluded if they self-reported a diagnosis of mild cognitive impairment (MCI) or dementia; were taking medication typically prescribed for cognitive impairment; and/or performed within abnormal ranges on standardized neuropsychological assessments (described below). Participants scoring 24±1 points on the Mini-Mental State Examination (MMSE) were flagged for review to characterize if their cognitive functioning fell within the normal or abnormal range; participants scoring 22 and under on the MMSE were not eligible. Race-appropriate norms were used to convert standardized neuropsychological test raw scores to age and education-adjusted scaled scores [38, 39]. Individuals who scored 1.5 standard deviations below age and education-adjusted normal were excluded. Other exclusion criteria were: presence of sleep disorders, color blindness, psychiatric disorders, neurological disorders, and significant cerebrovascular or cardiovascular diseases. All participants were fluent English speakers and completed written informed consent prior to participation. Ethical approval was granted by the Rutgers University institutional review board and experiments were done in accord with the Helsinki Declaration of 1975.
Participants
At the time of data extraction from the larger parent study (N = 353), participants were selected for analysis in a case– control matched design. Of the parent sample, 57 individuals were ABCA7 rs115550680 risk “G” allele carriers. Using sleep quality, age, and education as predictors of group membership (0 = non-risk, 1 = at-risk) and a matching tolerance set to 0.10, we propensity-score matched these 57 at-risk individuals with 57 individuals who were homozygous for the non-risk “A” allele [40]. Hence, individuals with the ABCA7 rs115550680 AG (n = 53) or GG (n = 4) genotype constituted the risk group (the GG genotype was not considered a separate risk group), while individuals with the AA genotype were the non-risk group. See Table 1 for demographic information by genotype group for the analytic sample.
Table 1
Demographics and neuropsychological assessments for carriers of the ABCA7 rs115550680 non-risk (AA) and risk (AG/GG) genotype within the sleep quality groups
Non-Risk (AA) Genotype | Risk (AG/GG) Genotype | |||||
Sleep Quality | Poor | Average | Good | Poor | Average | Good |
Sample Size | 13 (3 males) | 29 (7 males) | 15 (4 males) | 13 (0 males) | 29 (6 males) | 15 (3 males) |
Age | 67 (9.44) | 69 (7.52) | 67 (5.08) | 67 (10.03) | 70 (7.05) | 66 (6.72) |
Education (y) | 13.77 (1.60) | 13.55 (1.74) | 13.67 (2.03) | 13.81 (1.95) | 13.09 (1.98) | 13.67 (1.99) |
MMSE | 27.69 (1.44) | 28.24 (1.57) | 27.20 (2.18) | 27.46 (1.81) | 27.59 (2.19) | 28.13 (1.46) |
RAVLT - Delayed | 6.92 (3.49) | 7.45 (3.30) | 6.93 (2.49) | 6.00 (2.97) | 6.24 (3.03) | 6.93 (4.06) |
NAART | 34.15 (14.95) | 38.93 (11.75) | 34.74 (12.00) | 36.92 (11.64) | 39.62 (10.05) | 36.53 (10.77) |
WAIS-IV Digit Span | 24.23 (4.55) | 22.41 (3.99) | 24.53 (3.38) | 22.00 (3.61) | 22.10 (3.98) | 22.20 (4.43) |
Data are presented as mean (standard deviation). MMSE, Mini-Mental State Examination; RAVLT – Delayed, Rey Auditory Verbal Learning Test Delayed Recall; NAART, North American Adult Reading Test; WAIS-IV, Wechsler Adult Intelligence Scale – IV edition. There were no statistically significant differences between non-risk and risk genotype groups on age or education, ps > 0.05, indicating propensity-score matching success.
Standardized neuropsychological assessments
To assess cognition, participants attended a lab visit to undergo a two-hour in-person battery of paper-and-pencil neuropsychological tests. The neuropsychological battery consisted of the MMSE (broad assay of cognitive impairment) [41], Rey Auditory Verbal Learning Test (RAVLT) Delayed Recall (episodic memory) [42], North American Adult Reading Test (NAART35) (literacy) [43], and Wechsler Adult Intelligence Scale (WAIS-IV) Digit Span (attention) [44].
Sleep quality
Sleep quality was self-reported using a single item, ‘Rate your usual sleep quality’ with response choices on a Likert scale from 1 = ‘very poor’ to 5 = ‘very good’. This single-item of self-reported sleep quality has been used in other published cross-sectional analyses derived from the larger Pathways to Healthy Aging in African Americans cohort [45]. We collapsed ‘very poor’ and ‘very good’ sleep quality due to low frequencies in these categories. As such, the recoded sleep quality variable comprised three categories of sleep quality: 0 = ‘very poor and poor sleep quality’; 1 = ‘satisfactory sleep quality’; 2 = ‘good and very good sleep quality.’
Behavioral paradigm: Concurrent discrimination and generalization task
We have previously described the concurrent discrimination and generalization task in more detail [27, 45]. Derived from prior neurocomputational network models of the entorhinal cortex and hippocampal– brain regions disrupted in the earliest stages of preclinical AD– this task indexes the ability to generalize prior learning to new situations and contexts.
In brief, participants learn through a trial-and-error acquisition phase, a series of discriminations based on visual features (i.e., shape or color). In the generalization phase, participants are confronted with new stimuli to test whether they can apply past learning to new situations (see Fig. 1). The outcome measure was the number of generalization errors, adjusting for acquisition phase errors; higher scores indicate worse performance.
Fig. 1
An example of the concurrent discrimination and generalization task. On each trial of initial learning (acquisition), the discrimination pair is presented and if the participant responds correctly, the chosen object is raised to reveal a smiley face icon underneath. During test phase (generalization), events are similar to the acquisition phase, but the objects are changed so that the relevant feature remains the same, but the irrelevant feature is novel. Here (A) is an example of a trial where the relevant feature is shape, but not color, while (B) is an example of a trial where the relevant feature is color, but not shape.
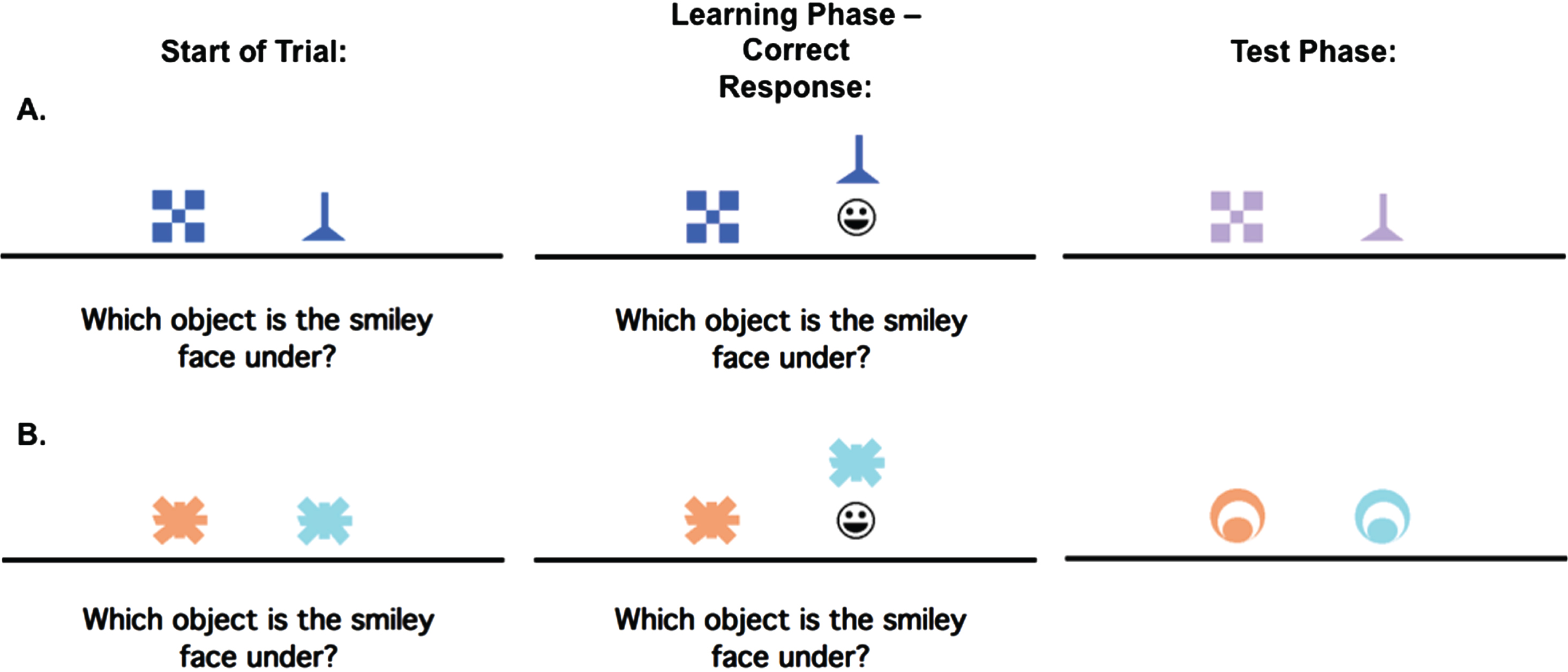
Genetic data collection and processing
Saliva samples for genotyping were collected using Oragene kits during the neuropsychological testing visit. ABCA7 rs115550680 genotyping was conducted via quantitative polymerase chain reaction on an Eppendorf Mastercycler thermal cycler, using a TaqMan Custom Genotyping assay.
Statistical analyses
To examine the main effects of ABCA7 genotype (risk versus non-risk) on cognition and the moderating role of sleep quality (poor, average, good), a univariate analysis of covariance (ANCOVA) was conducted, with ABCA7 genotype and sleep quality as fixed factors and age and years of education as covariates. Any significant interactions were explored further with simple main effects analysis (with Bonferroni correction for multiple comparisons) as described in the Results section below. All assumptions for ANCOVA were checked and met prior to analyses. All statistical analyses were performed using IBM SPSS® Statistics for Mac, version 28.0.1.1 (IBM Corp., Armonk, NY, USA).
RESULTS
All participants underwent a battery of standardized neuropsychological assessments and were included in our analyses only if they were within normal age and education adjusted norm limits (Table 1). No main effects or interactions were observed for any of these standardized measures of cognitive functioning (MMSE, RAVLT, NAART and Digit Span). There was no bivariate relationship between sleep quality and ABCA7, χ2 (2)= 1.48, p = 0.48.
On the concurrent discrimination and generalization task, all participants reached the criterion of 16 consecutive correct responses on the acquisition phase, indicating that they successfully learned the task. There was no significant sleep quality×ABCA7 genotype interaction nor were there any main effects of sleep quality or ABCA7 genotype observed for acquisition errors, indicating that all groups learned the task equally well. Among the covariates, both age (F(1, 106) = 12.73, p = 0.001) and education (F(1, 106) = 4.53, p = 0.036) were significant predictors of acquisition errors.
When analyzing performance on the generalization phase, acquisition errors were entered as an additional covariate in the ANCOVA. For generalization phase errors, there was a main effect of ABCA7 genotype (F(1, 105) = 13.62, p = 0.001), and a significant effect of acquisition errors (F(1, 105) = 14.8, p = 0.001). Figure 2 shows the effect of ABCA7 genotype on the concurrent discrimination and generalization task, adjusted for covariates. While there was no difference in learning (acquisition errors) between the two groups, the risk group (M = 19.76, SE = 1.54) made significantly more generalization errors compared to the non-risk group (M = 11.69, SE = 1.55).
Fig. 2
Performance (total errors) on the concurrent discrimination and generalization task based on ABCA7 genotype. While there were no group differences on the initial learning (acquisition phase), carriers of the AG/GG risk genotype made significantly more errors during generalization.
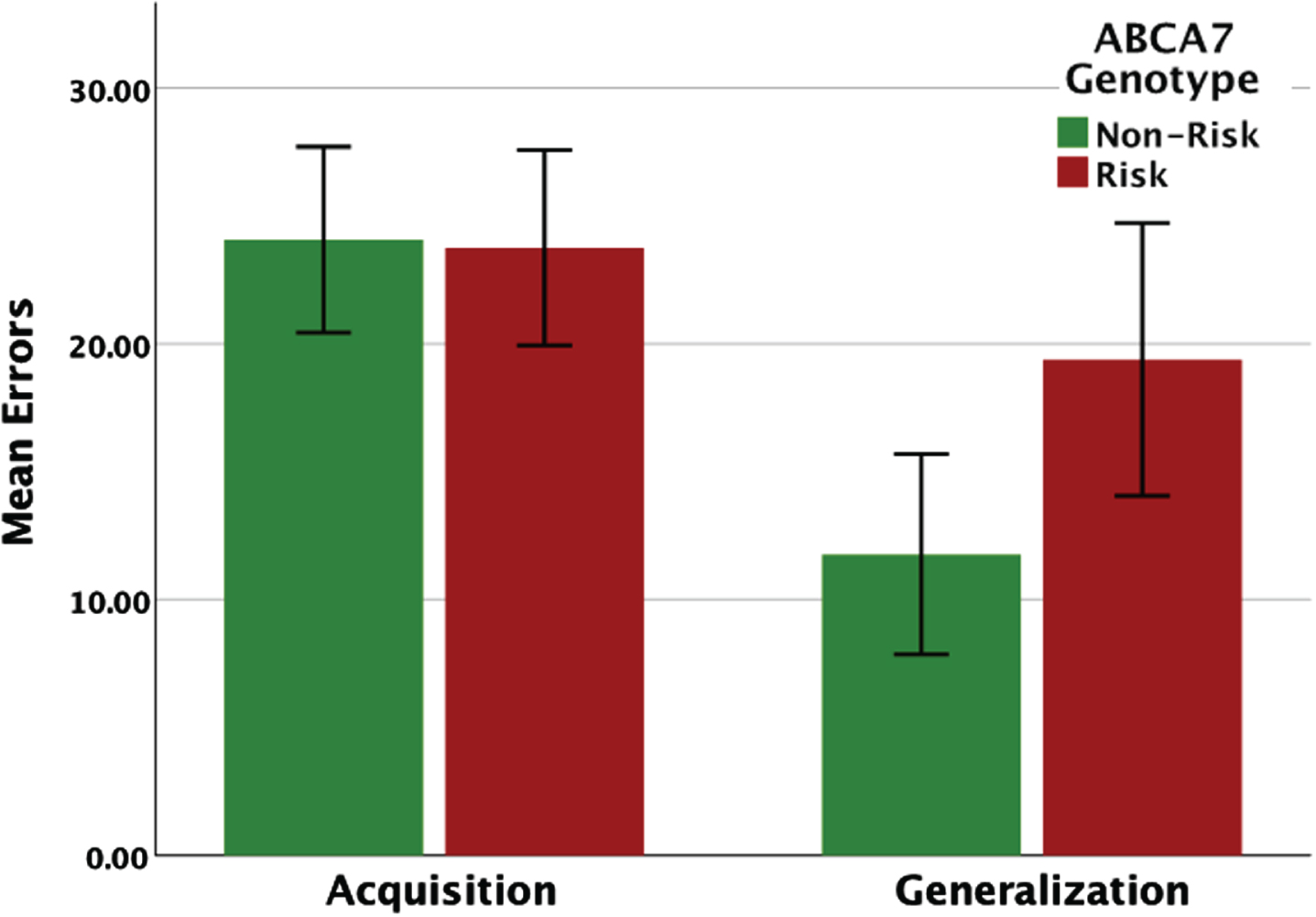
Furthermore, for generalization errors, there was a significant ABCA7 genotype x sleep quality interaction (F(2, 105) = 3.45, p = 0.035). To understand the exact nature of the interaction, a follow-up simple effects analysis was conducted (Fig. 3). The ABCA7 risk group made significantly more generalization errors compared to the non-risk group if their sleep quality was poor (F(1, 105) = 12.53, p = 0.001) or average (F(1, 105) = 9.20, p = 0.003). However, for individuals with good sleep quality, there was no difference in generalization based on ABCA7 genotype (F(1, 105) = 0.001, p = 0.995). Additionally, an examination of the effect of sleep quality at each level of ABCA7 genotype (risk and non-risk) showed that for the risk group, individuals with poor and average sleep quality made significantly more generalization errors compared to those with good sleep quality (F(2, 105) = 5.97, p = 0.004). For the non-risk group, there was no difference in generalization performance based on sleep quality (F(2, 105) = 0.059, p = 0.943). Hence, sleep quality moderates the association between ABCA7 genotype and generalization performance, such that, good sleep quality mitigates ABCA7-related impairments in generalization. Results were largely unchanged after controlling for gender (see Supplementary Table 1).
Fig. 3
Carriers of the ABCA7 risk genotype made significantly more generalization errors compared to the non-risk individuals if they reported poor or average sleep quality, while for individuals with good sleep quality there was no difference in generalization based on ABCA7 genotype.
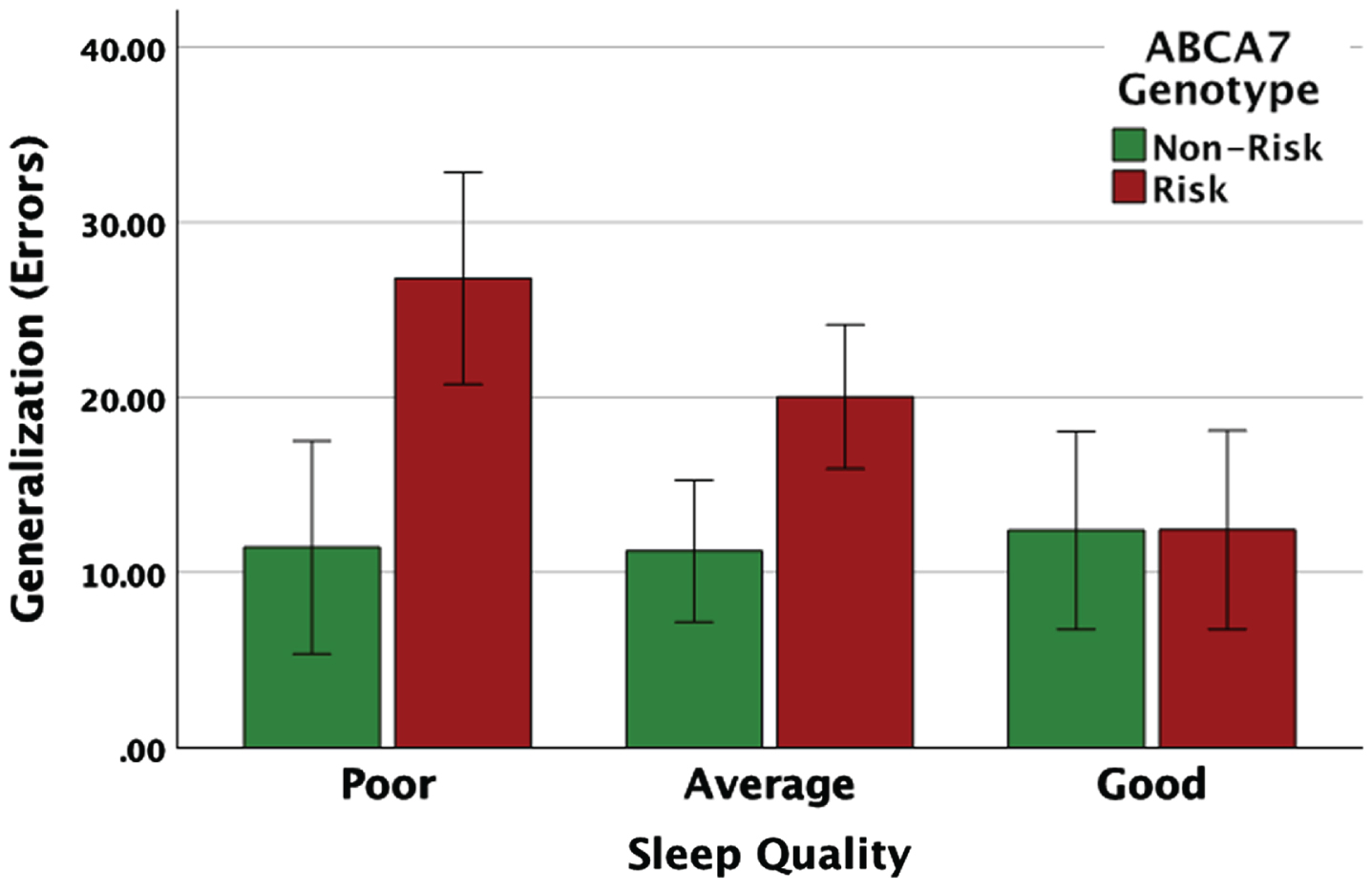
DISCUSSION
We observed that self-reported sleep quality moderates generalization deficits associated with the ABCA7 risk genotype. Carriers of the risk genotype who reported poor or average sleep quality made significantly more generalization errors compared to their non-risk counterparts. Conversely, there was no genotype-related difference in generalization performance in individuals who reported good sleep quality.
Consistent with previous findings [26], we observed an overall ABCA7 rs115550680 related impairment in generalization (Fig. 2). While both groups solved the task equally well during the acquisition phase, the risk group performed significantly worse during the generalization phase of initial learning compared to the non-risk group, confirming that there is a specific generalization deficit associated with the ABCA7 risk genotype. We further extend these findings by demonstrating that self-reported sleep quality moderates this association, such that the deleterious effects of ABCA7 risk genotype are observed in individuals with poor or average sleep quality, but not in those with good sleep quality. The results of the present study, therefore, indicate that sleep quality may be neuroprotective against ABCA7 rs115550680 genetic risk for AD, and thus should be a candidate target for future intervention studies focused on reducing AD risk.
ABCA7 dysregulation influences the properties of neurons and microglia, and these alterations likely facilitate amyloid precursor protein processing and suppression of cellular Aβ clearance respectively, contributing to AD development [46]. Increasing evidence also supports sleep deficiency as a marker for AD pathology [47–49] and future risk of cognitive decline [50–54]. In a healthy older adult population, both subjective and objective measures of sleep disturbances and sleep deprivation were associated with cognitive impairments, in part via an Aβ mechanism [55, 56]. As such, a common pathway through which sleep may interact with ABCA7 to impact cognition is likely related to Aβ burden, although emerging work suggests inflammation mechanisms involving microglia activation impairs sleep expression and sleep-dependent memory functions [57].
Two physiological mechanisms could explain the neuroprotective role of sleep in the pathways directly linking ABCA7 to Aβ. First, sleep, particularly slow wave sleep (SWS), augments the action of the glymphatic system in clearing Aβ from the brain when compared with wakefulness state [58, 59]. Second, neurometabolic activity is reduced in SWS as compared with wakefulness and, consequently, sleep disruption could lead to increase neurometabolic activity resulting in Aβ increases [60, 61]. Conversely, enhanced sleep through treatment decreased Aβ plaque deposition in mice models [62]. Similarly in humans, continuous positive airway pressure treatment in adults with obstructive sleep apnea increased slow wave activity; and greater increases of slow wave activity were associated with greater decreases in Aβ burden [63]. Both processes suggest a possible beneficial role of good quality and efficient sleep in ameliorating the increased Aβ deposition (via increased production and suppressed clearance) caused by ABCA7 dysregulation.
Recent electroencephalographic (EEG) studies have confirmed SWS disruption as the mechanistic pathway through which Aβ pathology may contribute to cognitive decline and, conversely, the eventual useful role of SWS in facilitating Aβ reduction [55]. Further, disrupted SWS was found to specifically impact the hippocampal-neocortical pathway [64, 65], resulting in impairment of hippocampal-dependent cognitive function [64–68]. This is consistent with our finding of a neuroprotective effect of sleep quality on generalization following rule learning, a measure known to be sensitive to hippocampal function [27]. Equivalent effects were not observed on any standardized neuropsychological tests: RAVLT delayed recall, MMSE, and WAIS-IV Digit Span (see Supplementary Tables 2–4, respectively). The current study demonstrates that this task may be sensitive to variations sleep quality. Other studies involving involving cognitively healthy older African American samples with varied educational opportunity differences and socio-environmental exposures show that generalization is sensitive to aerobic fitness and body mass index variations [45, 69]. As such, the concurrent discrimination and generalization behavioral paradigm may be an especially useful tool for assessing the efficacy of modifying risk factors for AD, particularly among individuals underrepresented in biomedical research and in the earliest phases of prodromal AD when individuals are otherwise cognitively healthy and at ceiling on performance on standardized neuropsychological tests.
Although the association of ABCA7 risk variants with the development of late-onset AD has been confirmed worldwide [22, 24, 70–72], the effect size of the ABCA7 locus on the relative odds of being diagnosed with AD is significantly higher in African Americans [24]. Additionally, while the ABCA7 SNP (rs115550680) examined in the present study has an effect size comparable with that of APOE ɛ4 [24], interestingly, the APOE ɛ4 allele is less predictive of AD development among those with African ancestry [73, 74]. Furthermore, ABCA7 rs115550680 is monomorphic in those of European ancestry who do not show the risk variant. A related study in a cohort of predominantly non-Hispanic whites found that higher levels of wrist actigraphy-measured consolidated sleep, and thus better sleep quality, attenuated the effect of APOE genotype on incident AD and cognitive decline over six years [15]. However, it remains an open question as to whether or not the results observed in our present study can be replicated across races with other genetic loci for AD, including APOE. In particular, it is not clear if all genetic risk for AD (such as from APOE) can be ameliorated by improved sleep quality or if this sleep/genetic interaction is specific to this SNP of ABCA7. Answering that question will have important future consequences for low-cost lifestyle-based approaches in support of personalized medicine.
This study is not without limitations. Given the matching methods were based on characteristics of the sample (e.g., age, education), generalizability of the results may be limited. Our study used a single self-report item for sleep quality. Therefore, the multidimensional features of sleep [75] may not have been captured, such as the presence of sleep disorders, global disruptions of sleep duration, efficiency, and overall quality, or local disruptions in sleep microstructure quantified using topographical EEG approaches. Although this approach is not ideal, other studies also used single self-reported sleep measures [45, 76– 78] and have identified linkages with other lifestyle factors and cognition. For example, a recent study found that sleep quality, measured via a self-reported single-item, partially explained the relationship between physical activity and cognitive function in community-dwelling middle-aged and older adults, even after controlling for depressive symptoms [77]. Similarly, in a study that used the same self-reported sleep quality measure as the present study, aerobic fitness and the interaction between fitness and sleep quality were independently related to generalization of prior learning among older African Americans, controlling for depressive symptoms, age, education, gender, and body mass index [45]. Finally, important possible confounders such as cognitive activities, occupation, and socioeconomic status were unavailable at the time of data extraction. Nevertheless, in the present study, we have identified an important interaction between an understudied AD risk gene and sleep quality that will guide future mechanistic studies. Future research should incorporate objectively measured sleep metrics via actigraphy and polysomnography including sleep staging and architectural features of sleep (e.g., SWS). More detailed and validated self-report sleep questionnaires targeting sleep disorder symptoms and more comprehensive assessments of sleep duration, timing and regularity, and quality may provide further insights.
Conclusion
Overall, these results add to an emerging picture of differing effects of genetic susceptibility on AD-related neuropathology based on sleep: while the ABCA7 rs115550680 risk genotype is associated with cognitive impairments and an increased likelihood of AD and other dementias, these detriments may be alleviated in those with sufficient, high-quality sleep. These findings are consistent with those observed with APOE genotypes, highlighting the possibility that sleep is a candidate general factor supporting cognitive reserve and/or resilience. Moreover, they invite further investigation on the role of the different aspects of sleep neurophysiology in the pathogenesis and progression of AD, as well as the continued development of non-invasive intervention strategies based on sleep restoration and enhancement. Finally, the linkage between good sleep quality and reduced impact of the ABCA7 rs115550680 risk variant specific to those of African ancestry may provide us with insights and tools toward reducing racial health disparities in AD.
ACKNOWLEDGMENTS
We are indebted to the thousands of community members who have participated in our brain health events since 2006, and from over 500 community members who have enrolled, to date, as VIPs (Very Important Participants) in our Pathways to Healthy Aging in African Americans study.
FUNDING
This work was supported by grants to M.A.G. from the National Institutes of Health-National Institute on Aging (grant number 1R01AG053961), Office of Minority Health at HHS (grant number MH-STT-15-001) and the NJ Department of Health’s Office of Minority and Multicultural Health (grant number OMMH21HDP002), and by support from the Chancellor’s and Provost’s offices at Rutgers University-Newark.
CONFLICT OF INTEREST
The authors have no conflicts of interest to report.
DATA AVAILABILITY
The data supporting the findings of this study are available on request without undue reservation from the corresponding author.
SUPPLEMENTARY MATERIAL
[1] The supplementary material is available in the electronic version of this article: https://dx.doi.org/10.3233/JAD-230043.
REFERENCES
[1] | Sharma S , Kavuru M ((2010) ) Sleep and metabolism: An overview. Int J Endocrinol 2010: , 270832. |
[2] | Besedovsky L , Lange T , Born J ((2012) ) Sleep and immune function. Pflugers Arch 463: , 121–137. |
[3] | Bellesi M , Riedner BA , Garcia-Molina GN , Cirelli C , Tononi G ((2014) ) Enhancement of sleep slow waves: Underlying mechanisms and practical consequences. Front Syst Neurosci 8: , 208. |
[4] | Xie L , Kang H , Xu Q , Chen MJ , Liao Y , Thiyagarajan M , O’Donnell J , Christensen DJ , Nicholson C , Iliff JJ ((2013) ) Sleep drives metabolite clearance from the adult brain. Science 342: , 373–377. |
[5] | Stickgold R ((2005) ) Sleep-dependent memory consolidation. Nature 437: , 1272–1278. |
[6] | Walker MP , Stickgold R ((2004) ) Sleep-dependent learning and memory consolidation. Neuron 44: , 121–133. |
[7] | Jackson CL , Walker JR , Brown MK , Das R , Jones NL ((2020) ) A workshop report on the causes and consequences of sleep health disparities. Sleep 43: , zsaa037. |
[8] | Caraballo C , Mahajan S , Valero-Elizondo J , Massey D , Lu Y , Roy B , Riley C , Annapureddy AR , Murugiah K , Elumn J , Nasir K , Nunez-Smith M , Forman HP , Jackson CL , Herrin J , Krumholz HM ((2022) ) Evaluation of temporal trends in racial and ethnic disparities in sleep duration among US adults, 2004-2018. JAMA Netw Open 5: , e226385. |
[9] | Sheehan CM , Frochen SE , Walsemann KM , Ailshire JA ((2019) ) Are US adults reporting less sleep? Findings from sleep duration trends in the National Health Interview Survey, 2004–2017. Sleep 42: , zsy221. |
[10] | Baumgart M , Snyder HM , Carrillo MC , Fazio S , Kim H , Johns H ((2015) ) Summary of the evidence on modifiable risk factors for cognitive decline and dementia: A population-based perspective. Alzheimers Dement 11: , 718–726. |
[11] | Yaffe K , Falvey CM , Hoang T ((2014) ) Connections between sleep and cognition in older adults. Lancet Neurol 13: , 1017–1028. |
[12] | Leng Y , Ackley SF , Glymour MM , Yaffe K , Brenowitz WD ((2021) ) Genetic risk of Alzheimer’s disease and sleep duration in non-demented elders. Ann Neurol 89: , 177–181. |
[13] | Palpatzis E , Bass N , Jones R , Mukadam N ((2022) ) Longitudinal association of apolipoprotein E and sleep with incident dementia. Alzheimers Dement 18: , 888–898. |
[14] | Yuan S , Ma W , Yang R , Xu F , Han D , Huang T , Peng Mi , Xu A , Lyu J ((2022) ) Sleep duration, genetic susceptibility, and Alzheimer’s disease: A longitudinal UK Biobank-based study. BMC Geriatr 22: , 638. |
[15] | Lim ASP , Yu L , Kowgier M , Schneider JA , Buchman AS , Bennett DA ((2013) ) Modification of the relationship of the apolipoprotein E ɛ4 allele to the risk of Alzheimer disease and neurofibrillary tangle density by sleep. JAMA Neurol 70: , 1544–1551. |
[16] | Tosto G , Reitz C ((2013) ) Genome-wide association studies in Alzheimer’s disease: A review. Curr Neurol Neurosci Rep 13: , 381. |
[17] | Chan SL , Kim WS , Kwok JB , Hill AF , Cappai R , Rye K , Garner B ((2008) ) ATP-binding cassette transporter A7 regulates processing of amyloid precursor protein. J Neurochem 106: , 793–804. |
[18] | Lyssenko NN , Praticò D ((2021) ) ABCA7 and the altered lipidostasis hypothesis of Alzheimer’s disease. Alzheimers Dement 17: , 164–174. |
[19] | Tanaka N , Abe-Dohmae S , Iwamoto N , Yokoyama S ((2011) ) Roles of ATP-binding cassette transporter A7 in cholesterol homeostasis and host defense system. J Atheroscler Thromb 18: , 274–281. |
[20] | Cuyvers E , De Roeck A , Van den Bossche T , Van Cauwenberghe C , Bettens K , Vermeulen S , Mattheijssens M , Peeters K , Engelborghs S , Vandenbulcke M ((2015) ) Mutations in ABCA7 in a Belgian cohort of Alzheimer’s disease patients: A targeted resequencing study. Lancet Neurol 14: , 814–822. |
[21] | Steinberg S , Stefansson H , Jonsson T , Johannsdottir H , Ingason A , Helgason H , Sulem P , Magnusson OT , Gudjonsson SA , Unnsteinsdottir U ((2015) ) Loss-of-function variants in ABCA7 confer risk of Alzheimer’s disease. Nat Genet 47: , 445–447. |
[22] | Hollingworth P , Harold D , Sims R , Gerrish A , Lambert J-C , Carrasquillo MM , Abraham R , Hamshere ML , Pahwa JS , Moskvina V ((2011) ) Common variants at ABCA7, MS4A6A/MS4A4E, EPHA1, CD33 and CD2AP are associated with Alzheimer’s disease. Nat Genet 43: , 429. |
[23] | Reitz C , Mayeux R ((2014) ) Genetics of Alzheimer’s disease in Caribbean Hispanic and African American populations. Biol Psychiatry 75: , 534–541. |
[24] | Reitz C , Jun G , Naj A , Rajbhandary R , Vardarajan BN , Wang L-S , Valladares O , Lin C-F , Larson EB , Graff-Radford NR ((2013) ) Variants in the ATP-binding cassette transporter (ABCA7), apolipoprotein E ɛ4, and the risk of late-onset Alzheimer disease in African Americans. JAMA 309: , 1483–1492. |
[25] | Cukier HN , Kunkle BW , Vardarajan BN , Rolati S , Hamilton-Nelson KL , Kohli MA , Whitehead PL , Dombroski BA , Van Booven D , Lang R ((2016) ) ABCA7 frameshift deletion associated with Alzheimer disease in African Americans. Neurol Genet 2: , e79. |
[26] | Sinha N , Reagh ZM , Tustison NJ , Berg CN , Shaw A , Myers CE , Hill D , Yassa MA , Gluck MA ((2019) ) ABCA7 risk variant in healthy older African Americans is associated with a functionally isolated entorhinal cortex mediating deficient generalization of prior discrimination training. Hippocampus 29: , 527. |
[27] | Myers CE , Kluger A , Golomb J , Ferris S , de Leon MJ , Schnirman G , Gluck MA ((2002) ) Hippocampal atrophy disrupts transfer generalization in nondemented elderly. J Geriatr Psychiatry Neurol 15: , 82–90. |
[28] | Ancoli-Israel S , Klauber MR , Stepnowsky C , Estline E , Chinn A , Fell R ((1995) ) Sleep-disordered breathing in African-American elderly. Am J Respir Crit Care Med 152: , 1946–1949. |
[29] | Ruiter ME , DeCoster J , Jacobs L , Lichstein KL ((2010) ) Sleep disorders in African Americans and Caucasian Americans: A meta-analysis. Behav Sleep Med 8: , 246–259. |
[30] | Barnes LL , Bennett DA ((2014) ) Alzheimer’s disease in African Americans: Risk factors and challenges for the future. Health Affairs 33: , 580–586. |
[31] | Laposky AD , Van Cauter E , Diez-Roux AV ((2016) ) Reducing health disparities: The role of sleep deficiency and sleep disorders. Sleep Med 18: , 3–6. |
[32] | Rentz DM , Parra Rodriguez MA , Amariglio R , Stern Y , Sperling R , Ferris S ((2013) ) Promising developments in neuropsychological approaches for the detection of preclinical Alzheimer’s disease: A selective review. Alzheimers Res Ther 5: , 58. |
[33] | Montgomery KS , Edwards G III , Levites Y , Kumar A , Myers CE , Gluck MA , Setlow B , Bizon JL ((2016) ) Deficits in hippocampal-dependent transfer generalization learning accompany synaptic dysfunction in a mouse model of amyloidosis. Hippocampus 26: , 455–471. |
[34] | Myers CE , Kluger A , Golomb J , Gluck MA , Ferris S ((2008) ) Learning and generalization tasks predict short-term cognitive outcome in non-demented elderly. J Geriatr Psychiatry Neurol 21: , 93–103. |
[35] | Petok JR , Myers CE , Pa J , Hobel Z , Wharton DM , Medina LD , Casado M , Coppola G , Gluck MA ((2018) ) Impairment of memory generalization in preclinical autosomal dominant Alzheimer’s disease mutation carriers. Neurobiol Aging 65: , 149–157. |
[36] | Esiaka D , Yarborough CC , Fausto BA , Gluck MA ((2022) ) A mini-review of strategies for recruiting older African Americans to Alzheimer’s disease research.. Community Health Equity Res Policy. doi: 10.1177/0272684X221118493. |
[37] | Gluck MA , Shaw A , Hill D ((2018) ) Recruiting older African Americans to brain health and aging research through community engagement. Generations 42: , 78–82. |
[38] | Ferman TJ , Lucas JA , Ivnik RJ , Smith GE , Willis FB , Petersen RC , Graff-Radford NR ((2005) ) Mayo’s Older African American normative studies: Auditory verbal learning test norms for African American elders. Clin Neuropsychol 19: , 214–228. |
[39] | Wechsler D ((2008) ) WAIS-IV: Wechsler adult intelligence scale. Pearson, San Antonio, TX. |
[40] | Eeren HV , Spreeuwenberg MD , Bartak A , de Rooij M , Busschbach JJ ((2015) ) Estimating subgroup effects using the propensity score method: A practical application in outcomes research. Med Care 53: , 366–373. |
[41] | Folstein MF , Folstein SE , McHugh PR ((1975) ) “Mini-mental state”: A practical method for grading the cognitive state of patients for the clinician. J Psychiatr Res 12: , 189–198. |
[42] | Schmidt M ((1996) ) Rey auditory verbal learning test: A handbook, Western Psychological Services, Los Angeles, CA. |
[43] | Uttl B ((2002) ) North American Adult Reading Test: Age norms, reliability, and validity. J Clin Exp Neuropsychol 24: , 1123–1137. |
[44] | Wechsler D ((2008) ) WAIS-IV: Administration and scoring manual, The Psychologial Corporation, New York, NY. |
[45] | Fausto BA , Gluck MA ((2022) ) Low body mass and high-quality sleep maximize the ability of aerobic fitness to promote improved cognitive function in older African Americans. Ethn Health 27: , 909–928. |
[46] | Aikawa T , Holm M-L , Kanekiyo T ((2018) ) ABCA7 and pathogenic pathways of Alzheimer’s disease. Brain Sci 8: , 27. |
[47] | Carvalho DZ , Louis EK St. , Knopman DS , Boeve BF , Lowe VJ , Roberts RO , Mielke MM , Przybelski SA , Machulda MM , Petersen RC ((2018) ) Association of excessive daytime sleepiness with longitudinal β-amyloid accumulation in elderly persons without dementia. JAMA Neurol 75: , 672–680. |
[48] | Ju Y-ES , McLeland JS , Toedebusch CD , Xiong C , Fagan AM , Duntley SP , Morris JC , Holtzman DM ((2013) ) Sleep quality and preclinical Alzheimer disease. JAMA Neurol 70: , 587–593. |
[49] | Spira AP , Gamaldo AA , An Y , Wu MN , Simonsick EM , Bilgel M , Zhou Y , Wong DF , Ferrucci L , Resnick SM ((2013) ) Self-reported sleep and β-amyloid deposition in community-dwelling older adults. JAMA Neurol 70: , 1537–1543. |
[50] | Ancoli-Israel S , Palmer BW , Cooke JR , Corey-Bloom J , Fiorentino L , Natarajan L , Liu L , Ayalon L , He F , Loredo JS ((2008) ) Cognitive effects of treating obstructive sleep apnea in Alzheimer’s disease: A randomized controlled study. J Am Geriatr Soci 56: , 2076–2081. |
[51] | Cooke JR , Ayalon L , Palmer BW , Loredo JS , Corey-Bloom J , Natarajan L , Liu L , Ancoli-Israel S ((2009) ) Sustained use of CPAP slows deterioration of cognition, sleep, and mood in patients with Alzheimer’s disease and obstructive sleep apnea: A preliminary study. J Clin Sleep Med 5: , 305–309. |
[52] | Leng Y , Blackwell T , Stone KL , Hoang TD , Redline S , Yaffe K ((2016) ) Periodic limb movements in sleep are associated with greater cognitive decline in older men without dementia. Sleep 39: , 1807–1810. |
[53] | Tworoger SS , Lee S , Schernhammer ES , Grodstein F ((2006) ) The association of self-reported sleep duration, difficulty sleeping, and snoring with cognitive function in older women. Alzheimer Dis Assoc Disord 20: , 41–48. |
[54] | Yaffe K , Laffan AM , Harrison SL , Redline S , Spira AP , Ensrud KE , Ancoli-Israel S , Stone KL ((2011) ) Sleep-disordered breathing, hypoxia, and risk of mild cognitive impairment and dementia in older women. JAMA 306: , 613–619. |
[55] | Cordone S , Annarumma L , Rossini PM , De Gennaro L ((2019) ) Sleep and β-amyloid deposition in Alzheimer disease: Insights on mechanisms and possible innovative treatments. Front Pharmacol 10: , 695. |
[56] | Lucey BP , Bateman RJ ((2014) ) Amyloid-β diurnal pattern: Possible role of sleep in Alzheimer’s disease pathogenesis. Neurobiol Aging 35: , S29–S34. |
[57] | Mander BA , Dave A , Lui KK , Sprecher KE , Berisha D , Chappel-Farley MG , Chen IY , Riedner BA , Heston M , Suridjan I ((2022) ) Inflammation, tau pathology, and synaptic integrity associated with sleep spindles and memory prior to β-amyloid positivity. Sleep 45: , zsac 135. |
[58] | Benveniste H ((2018) ) The brain’s waste-removal system. In Cerebrum: The Dana Forum on Brain Science, Dana Foundation. |
[59] | Stowell RD , Sipe GO , Dawes RP , Batchelor HN , Lordy KA , Whitelaw BS , Stoessel MB , Bidlack JM , Brown E , Sur M , Majewska AK ((2019) ) Noradrenergic signaling in the wakeful state inhibits microglial surveillance and synaptic plasticity in the mouse visual cortex. Nat Neurosci 22: , 1782–1792. |
[60] | Vyazovskiy VV , Olcese U , Lazimy YM , Faraguna U , Esser SK , Williams JC , Cirelli C , Tononi G ((2009) ) Cortical firing and sleep homeostasis. Neuron 63: , 865–878. |
[61] | Ju Y-ES , Lucey BP , Holtzman DM ((2014) ) Sleep and Alzheimer disease pathology—a bidirectional relationship. Nat Rev Neurol 10: , 115–119. |
[62] | Kang J-E , Lim MM , Bateman RJ , Lee JJ , Smyth LP , Cirrito JR , Fujiki N , Nishino S , Holtzman DM ((2009) ) Amyloid-β dynamics are regulated by orexin and the sleep-wake cycle. Science 326: , 1005–1007. |
[63] | Ju Y-ES , Zangrilli MA , Finn MB , Fagan AM , Holtzman DM ((2019) ) Obstructive sleep apnea treatment, slow wave activity, and amyloid-β. Ann Neurol 85: , 291–295. |
[64] | Mander BA , Rao V , Lu B , Saletin JM , Lindquist JR , Ancoli-Israel S , Jagust W , Walker MP ((2013) ) Prefrontal atrophy, disrupted NREM slow waves and impaired hippocampal-dependent memory in aging. Nat Neurosci 16: , 357–364. |
[65] | Mander BA , Marks SM , Vogel JW , Rao V , Lu B , Saletin JM , Ancoli-Israel S , Jagust WJ , Walker MP ((2015) ) β-amyloid disrupts human NREM slow waves and related hippocampus-dependent memory consolidation. Nat Neurosci 18: , 1051–1057. |
[66] | Fogel S , Vien C , Karni A , Benali H , Carrier J , Doyon J ((2017) ) Sleep spindles: A physiological marker of age-related changes in gray matter in brain regions supporting motor skill memory consolidation. Neurobiol Aging 49: , 154–164. |
[67] | Mander BA , Santhanam S , Saletin JM , Walker MP ((2011) ) Wake deterioration and sleep restoration of human learning. Curr Biol 21: , R183–R184. |
[68] | Ngo H-VV , Martinetz T , Born J , Mölle M ((2013) ) Auditory closed-loop stimulation of the sleep slow oscillation enhances memory. Neuron 78: , 545–553. |
[69] | Berg CN , Sinha N , Gluck MA ((2019) ) ABCA7 risk genotype diminishes the neuroprotective value of aerobic fitness in healthy older African Americans. Front Aging Neurosci 11: , 73. |
[70] | Beecham GW , Hamilton K , Naj AC , Martin ER , Huentelman M , Myers AJ , Corneveaux JJ , Hardy J , Vonsattel J-P , Younkin G , Bennett DA , Jager PLD , Larson EB , Crane PK , Kamboh MI , Kofler JK , Mash DC , Duque L , Gilbert JR , Gwirtsman H , Buxbaum JD , Kramer P , Dickson DW , Farrer LA , Frosch MP , Ghetti B , Haines JL , Hyman BT , Kukull WA , Mayeux RP , Pericak-Vance MA , Schneider JA , Trojanowski JQ , Reiman EM , Alzheimer’s Disease Genetics Consortium (ADGC); Schellenberg GD , Montine TJ ((2014) ) Genome-wide association meta-analysis of neuropathologic features of Alzheimer’s disease and related dementias. PLoS Genet 10: , e1004606. |
[71] | Kamboh MI , Demirci FY , Wang X , Minster RL , Carrasquillo MM , Pankratz VS , Younkin SG , Saykin AJ , for the Alzheimer’s Disease Neuroimaging Initiative, Jun G , Baldwin C , Logue MW , Buros J , Farrer L , Pericak-Vance MA , Haines JL , Sweet RA , Ganguli M , Feingold E , DeKosky ST , Lopez OL , Barmada MM ((2012) ) Genome-wide association study of Alzheimer’s disease. Transl Psychiatry 2: , e117. |
[72] | Liu C-C , Kanekiyo T , Xu H , Bu G ((2013) ) Apolipoprotein E and Alzheimer disease: Risk, mechanisms and therapy. Nat Rev Neurol 9: , 106. |
[73] | Rajabli F , Feliciano BE , Celis K , Hamilton-Nelson KL , Whitehead PL , Adams LD , Bussies PL , Manrique CP , Rodriguez A , Rodriguez V ((2018) ) Ancestral origin of ApoE ɛ4 Alzheimer disease risk in Puerto Rican and African American populations. PLoS Genet 14: , e1007791. |
[74] | Hendrie HC , Murrell J , Baiyewu O , Lane KA , Purnell C , Ogunniyi A , Unverzagt FW , Hall K , Callahan CM , Saykin AJ ((2014) ) APOE ɛ4 and the risk for Alzheimer disease and cognitive decline in African Americans and Yoruba. Int Psychogeriatr 26: , 977–985. |
[75] | National Center on Sleep Disorders Research ((2011) ) National institutes of health sleep disorders research plan. National Institutes of Health, Bethesda, MD. |
[76] | Gamaldo AA , Allaire JC , Whitfield KE ((2008) ) The relationship between reported problems falling asleep and cognition among African American elderly. Res Aging 30: , 752–767. |
[77] | Cheval B , Maltagliati S , Sieber S , Cullati S , Zou L , Ihle A , Kramer AF , Yu Q , Sander D , Boisgontier MP ((2022) ) Better subjective sleep quality partly explains the association between self-reported physical activity and better cognitive function. J Alzheimers Dis 87: , 919–931. |
[78] | Atroszko PA , Bagińska P , Mokosińska M , Sawicki A , Atroszko B ((2015) ) Validity and reliability of single-item self-report measures of general quality of life, general health and sleep quality. Comparative European Research 2015, pp. 207–211. |