White Matter Hyperintensity Volume and Amyloid-PET Synergistically Impact Memory Independent of Tau-PET in Older Adults Without Dementia
Abstract
Background:
Alzheimer’s disease (AD) and cerebrovascular disease are common, co-existing pathologies in older adults. Whether the effects of cerebrovascular disease and AD biomarkers on cognition are additive or synergistic remains unclear.
Objective:
To examine whether white matter hyperintensity (WMH) volume moderates the independent association between each AD biomarker and cognition.
Methods:
In 586 older adults without dementia, linear regressions tested the interaction between amyloid-β (Aβ) positron emission tomography (PET) and WMH volume on cognition, independent of tau-PET. We also tested the interaction between tau-PET and WMH volume on cognition, independent of Aβ-PET.
Results:
Adjusting for tau-PET, the quadratic effect of WMH interacted with Aβ-PET to impact memory. There was no interaction between either the linear or quadratic effect of WMH and Aβ-PET on executive function. There was no interaction between WMH volume and tau-PET on either cognitive measure.
Conclusion:
Results suggest that cerebrovascular lesions act synergistically with Aβ to affect memory, independent of tau, highlighting the importance of incorporating vascular pathology into biomarker assessment of AD.
INTRODUCTION
Alzheimer’s disease (AD) pathology is defined by the presence of amyloid-β (Aβ) plaques and tau tangles in the brain [1]. While both Aβ and tau have been associated with cognition in pathology studies [2] and in vivo positron emission tomography (PET) imaging studies [3, 4], neurofibrillary tau tangles have been most robustly correlated with cognition in a regionally-dependent manner [5]. In contrast, the correlation with Aβ is weak, and Aβ pathology is not uncommon in older adults without cognitive impairment [6]. It is not yet fully understood to what degree other factors may exacerbate the effects of AD pathologies on concurrent cognition.
Postmortem examination shows that Aβ plaques and tau tangles commonly co-occur with non-AD neuropathology, such as cerebrovascular disease [7]. Neuroimaging markers of small vessel cerebrovascular disease, such as white matter (WMH) volume, have been found to be elevated in participants with autosomal-dominant AD prior to symptom onset [8] as well as cognitively healthy participants with AD-like biomarkers [9]. Given the weak association between Aβ and cognition, these findings have led to growing interest in disentangling the role of AD and cerebrovascular pathologies on cognitive status and functioning. In particular, whether cerebrovascular disease may exacerbate the effect of AD biomarkers is highly debated, and several studies have tested for synergistic AD-cerebrovascular biomarker interactions with mixed findings. In examining whether there may be an interaction between cerebrovascular disease and tau on cognition, findings are limited, with one study reporting that cerebrovascular disease and CSF p-tau did not interact to affect cognition in young onset dementia [10]. The interaction between cerebrovascular disease and Aβ on cognition has been more extensively explored, finding that in subcortical vascular mild cognitive impairment (MCI) [11], cognitive impairment without dementia [12], and among cognitively unimpaired [13], the effect of one pathology biomarker on cognition was greater when the other was elevated; similar effects have been seen when looking at longitudinal cognitive decline in subcortical vascular dementia [14] and individuals with and without cognitive impairment [15]. However, other studies have found that cerebrovascular disease assessed via WMH and/or other magnetic resonance imaging (MRI) biomarkers do not act synergistically with PET or cerebrospinal fluid (CSF) Aβ biomarkers to impact cognition among cognitively unimpaired [16–19], subjective cognitive decline [20], young onset dementia [10], and subcortical vascular cognitive impairment [21], nor that they interact to impact cognitive decline [22] or likelihood of dementia [23, 24] in samples encompassing those with and without cognitive impairment. Instead, most of these studies suggest that cerebrovascular injury and Aβ contribute to cognition additively and independently.
Few studies have accounted for the effects of tau when assessing for synergistic effects of Aβ and cerebrovascular disease, and vice versa. In those that have, synergistic effects were not consistently found [25, 26]. Given these limited and mixed results, the interplay between Aβ, tau, and cerebrovascular disease on clinical outcomes has yet to be fully elucidated, and researchers have called for further characterization and inclusion of cerebrovascular biomarkers into analyses of aging and AD [27]. To our knowledge, there have been a lack of studies that have investigated whether WMH volume moderates the independent association between each AD biomarker and domain-specific cognition. Therefore, our study will examine the value of incorporating cerebrovascular biomarker information to Aβ and tau biomarker assessment of AD by testing 1) the tau-independent interaction between WMH volume and Aβ on cognition, and 2) the Aβ-independent interaction between WMH volume and tau on cognition in a sample of older adults without dementia.
METHODS
The ADNI dataset
Data used in the preparation of this article were obtained from the Alzheimer’s Disease Neuroimaging Initiative (ADNI) database (http://adni.loni.usc.edu). The ADNI was launched in 2003 as a public-private partnership, led by Principal Investigator Michael W. Weiner, MD. The primary goal of ADNI has been to test whether serial MRI, PET, other biological markers, and clinical and neuropsychological assessment can be combined to measure the progression of MCI and early AD. For up-to-date information, see http://www.adni-info.org. ADNI was approved by Institutional Review Boards of participating institutions. Informed written consent was obtained from all participants. Treatment of participants during this study was in accordance with the ethical standards set forth by the Helsinki Declaration.
Participants
As previously described [28], ADNI enrolls participants that are 55–90 years old, fluent in English or Spanish with at least 6 years of education, have a Geriatric Depression Scale score of less than 6, and have a modified Hachinski Ischemic Scale score of less than 5 at enrollment. The present study included 586 older adults with an actuarial classification of either MCI or cognitively unimpaired (CU) [29, 30] and who had Aβ-PET, tau-PET, WMH volume, clinical and cognitive data available concurrently. Concurrent data was matched by ADNI visit (e.g., screening or baseline, month 12, etc.).
Aβ and tau-PET imaging
Both Aβ and tau burden were assessed using PET imaging. Aβ-PET was assessed using [18F]AV45 (n = 360) or [18F]FBB (n = 226), while tau-PET was assessed using [18F]AV1451. Details on ADNI PET acquisition and preprocessing are available online (http://www.adni.loni.usc.edu). For both Aβ-PET tracers, a cortical composite was calculated from bilateral frontal, anterior/posterior cingulate, lateral parietal, and lateral temporal regions [31]. For tau-PET, a bilateral composite was calculated from regions representing Braak III/IV stage pathology, including the bilateral parahippocampal gyrus, fusiform gyrus, lingual gyrus, amygdala, middle temporal gyrus, caudal anterior cingulate gyrus, rostral anterior cingulate gyrus, posterior cingulate gyrus, isthmus cingulate gyrus, insula, inferior temporal gyrus, and temporal pole [32]. This region was selected to obtain a reliable estimate of mild-moderate stage tau pathology due to risk of contamination by off-target binding in earlier Braak stages [32]. Standardized uptake value ratios (SUVR) were calculated by dividing the composite values by the values in the whole cerebellum (Aβ-PET) or inferior cerebellar gray matter (tau-PET) [31–33]. To allow for inclusion of both [18F]AV45 and [18F]FBB as comparable continuous values in our analysis, SUVRs were transformed into centiloid (CL) values using the following equations: CL=(157.15 X SUVRFBB) –151.87 and CL=(188.22 X SUVRAV45) –189.16) [34]. Tau-PET values were partial volume corrected via the geometric transform method [35]. As the tau-PET variable was not distributed normally, statistical analyses were performed on both a raw and natural-log transformed tau-PET variable. As the results were qualitatively unchanged using both variables, the results using raw tau-PET data are reported. Furthermore, as there was an outlier on Aβ-PET (240.4 CL), statistical analyses were performed with and without this participant. As the results were qualitatively unchanged when excluding this outlier, the results including this datapoint arereported.
White matter hyperintensity and hippocampal volume data
All participants underwent whole-brain MRI scanning on 3-Tesla scanners. For each participant, an anatomical T1-weighted scan and a T2-weighted fluid attenuated inversion recovery (FLAIR) scan were acquired. All ADNI MRI acquisition sites passed rigorous scanner validation tests, and scan protocols were optimized across sites and manufacturers (GE, Philips, Siemens) [36].
WMH volumes were obtained from data collected in ADNI2 and ADNI3. WMHs were detected on FLAIR images using a method that has been previously described [37]. This method includes 1) linear co-registration of 3D T1-weighted image to the FLAIR image, 2) removal of non-brain elements from the FLAIR image using 3D T1-weighted brain mask, 3) image intensity normalization of the FLAIR image, 4) non-linear warping of 3D T1-weighted brain image to a minimal deformation template [38], 5) nonlinear deformation of the FLAIR volume to the atlas using the registration parameters for the 3D T1-weighted volume, 6) application of Bayesian segmentation to both 3D T1-weighted and the FLAIR volumes, 7) creation of four-tissue segmentation volume, 8) reverse transformation of three-tissue segmented volume into 3D T1-weighted native space, 9) reverse transformation of WMH segmented volume into FLAIR native space, 10) reverse transformation of four tissue segmented volume into 3D T1-weighted native space, 11) output of these volumes into the directory from which the program is launched. WMH volumes were segmented using 3D T1-weighted and T2-weighted fluid attenuated inversion recovery (FLAIR) MRI sequences. Further details on acquisition and segmentation are available online (http://www.adni.loni.usc.edu). Because WMH volume may vary as a function of total brain volume (TBV), total WMH volume was divided by TBV, consistent with previous research [39]. The TBV measure included both cerebrum and infratentorial regions. This TBV-normalized WMH volume variable was then natural log-transformed to reduce skew. The natural log-transformed, TBV-normalized WMH volume variable was utilized in all analyses.
As previously described [40], hippocampal volume was derived from a standard atlas based diffeomorphic approach [41] with label refinement modifications. Harmonized hippocampal masks developed via the European Alzheimer’s Disease Consortium (EADC) and ADNI Working Group on the Harmonized Protocol for Manual Hippocampal Segmentation were utilized with the following steps: 1) pre-processing with extraction of intracranial cavity, non-uniformity correction, and tissue classification [42]; 2) atlas registration of EADC-ADNI hippocampal masks [43]; 3) atlas fusion utilizing Multi-Atlas Label Fusion [44]; and 4) intensity-based label refinement. Hippocampal volume was divided by TBV prior to further analysis as consisted with prior research [45], in order to account for variation in hippocampal volume due to variation in brain volume.
Clinical and cognitive data
All participants had demographic, cardiovascular risk, and apolipoprotein E (APOE) genotyping data. Demographic data included age, sex, and education. Cardiovascular risk was assessed using pulse pressure (systolic blood pressure – diastolic blood pressure) as a proxy for arterial stiffness [46]. APOE ɛ4 frequency was determined by the number of ɛ4 alleles (0, 1, 2). Cognitive status (MCI or CU) was determined using actuarial neuropsychological criteria as described previously [29, 30]. Briefly, among participants without dementia per ADNI criteria [28], age-, sex-, and education-adjusted Z-scores were calculated using normative data from a ‘robust normal’ control group of participants who remained cognitively normal throughout their participation in ADNI. If participants demonstrated impairment on two scores in one cognitive domain or on one score across all three cognitive domains, they were classified as MCI (n = 151). Participants that did not demonstrate these impairments were classified as cognitively unimpaired (n = 435).
All participants completed neuropsychological testing which included assessment of memory and executive domains. The ADNI composite score for memory (ADNI_MEM) was derived from the Rey Auditory Verbal Learning Test, ADAS-Cog word list, Logical Memory, and Mini-Mental State Exam [47]. The ADNI composite score for executive function (ADNI_EF) was derived from Category Fluency, Weschler Adult Intelligence Scale-Revised Digit Symbol Substitution, Digit Span backwards, Clock Drawing, and Trails A and B [47]. As such, the ADNI_EF composite is a broad measure of executive function, including measurement of processing speed (Trails A) [48]. Further detail on the development of ADNI_MEM and ADNI_EF is available online (http://www.adni.loni.usc.edu). While all participants had data available for either ADNI_MEM or ADNI_EF (NTotal = 586), not all participants had data available for both measures (NADNI _ MEM = 584; NADNI _ EF = 582). Participants with missing data were not included in the analyses of the corresponding cognitive outcome variable.
Statistical analysis
Four general linear models were employed. The first set of models tested the interaction between Aβ-PET CL and both the linear and quadratic effects of global WMH volume on executive function and memory, while controlling for Braak III/IV tau-PET SUVR. A quadratic effect of global WMH was included due to literature which has suggested that negative effects of WMH on cognition may only occur at greater levels of WMH, such that there may be a possible threshold effect [49, 50]. The second set of models tested the interaction between Braak III/IV tau-PET SUVR and both the linear and quadratic effects of global WMH on executive function and memory, while controlling for Aβ-PET CL. All models additionally controlled for age, sex (recorded as male or female), education, pulse pressure, APOE ɛ4 frequency, and the main effects of the WMH and PET variables. Results were considered statistically significant at p < 0.05. All continuous variables were z-scored for each analysis. Each model was assessed for influential values using the deleted fit statistic, and models were tested with and without cases which exceeded an absolute value of
All analyses were performed using the Statistical Package for the Social Sciences (SPSS) version 27 (SPSS IBM, New York, USA), Stata/SE 13.0 and R version 4.0.2.
RESULTS
Demographics
Descriptive information of demographics, clinical, and imaging characteristics are reported in Table 1. The mean age of the sample was 73.4 years, and 53.9% of the sample was female. The sample was predominantly white (91.1%) and highly educated, with a mean education of 16.6 years.
Table 1
Participant demographic, clinical and imaging characteristics
Total [n = 586] | |
Age, y | 73.4±7.34 |
Sex (% Female) | 53.9% |
Education, y | 16.6±2.44 |
Pulse Pressure, mmHG | 58.8±14.76 |
Race | |
American Indian or Alaskan Native (%) | 0.3% |
Asian (%) | 1.2% |
Native Hawaiian or Other Pacific Islander (%) | 0% |
Black or African American (%) | 5.5% |
White (%) | 91.1% |
More than one race (%) | 1.7% |
Unknown (%) | 0.2% |
Ethnicity | |
Hispanic or Latino | 4.9% |
Not Hispanic or Latino | 94.5% |
Unknown | 0.5% |
APOE ɛ4 | |
0 alleles (%) | 64.0% |
1 allele (%) | 30.7% |
2 alleles (%) | 5.3% |
MCI (%) | 25.8% |
ADNI_MEM | 0.810±0.674 [n = 584] |
ADNI_EF | 0.905±0.904 [n = 582] |
Aβ Centiloids | 26.7±37.5 |
Braak III/IV Tau-PET SUVR | 1.49±0.261 |
WMH Volume, cm3 | 4.98±9.65 |
All continuous numerical data are presented as mean±SD [subgroup with data available]. WMH volume was normalized by total brain volume and natural log-transformed prior to further analysis. MCI indicates whether patients were considered to have mild cognitive impairment as opposed to being cognitively unimpaired. Race indicates patients’ self-reported race, while ethnicity indicates whether patients reported being of Hispanic or Latino ethnicity. MCI, mild cognitive impairment; PET, positron emission tomography; SUVR, standardized uptake value ratio; WMH, white matter hyperintensity.
Correlations between model predictors
Significant bivariate correlations were found among several predictor variables. A correlation panel for all predictors is available in Supplementary Table 1).
WMH volume by Aβ-PET centiloids interaction on executive function
Independent of Braak III/IV tau-PET SUVR, there were no significant interactions between centiloids and either the linear or quadratic main effect of WMH volume on executive function (βstds≤|0.071|, ps≥0.068) (Table 2, Fig. 1B). Despite this interaction being non-significant, examination of simple slopes (SS) demonstrated that centiloids were not significantly associated with executive function at average and lower (-1 SD) levels of WMH volume (SSs≤|0.065|, ps≥0.201), but that centiloids were associated with executive function at higher (+1 SD) levels of WMH (SS=-0.12, p = 0.019) (Fig. 1B). In this model, Braak III/IV tau-PET was significantly associated with executive function (βstd=-0.225, p < 0.001). Results remained qualitatively similar when adding hippocampal volume to the model (Supplementary Table 2).
Fig. 1
The effects of Aβ centiloids on cognition at varying levels of white matter hyperintensity burden. A) Aβ burden does not interact with either the linear or quadratic effect of white matter hyperintensity volume to effect executive function. B) Aβ burden interacts with the quadratic effect of white matter hyperintensity volume to effect memory, independent of tau-PET.
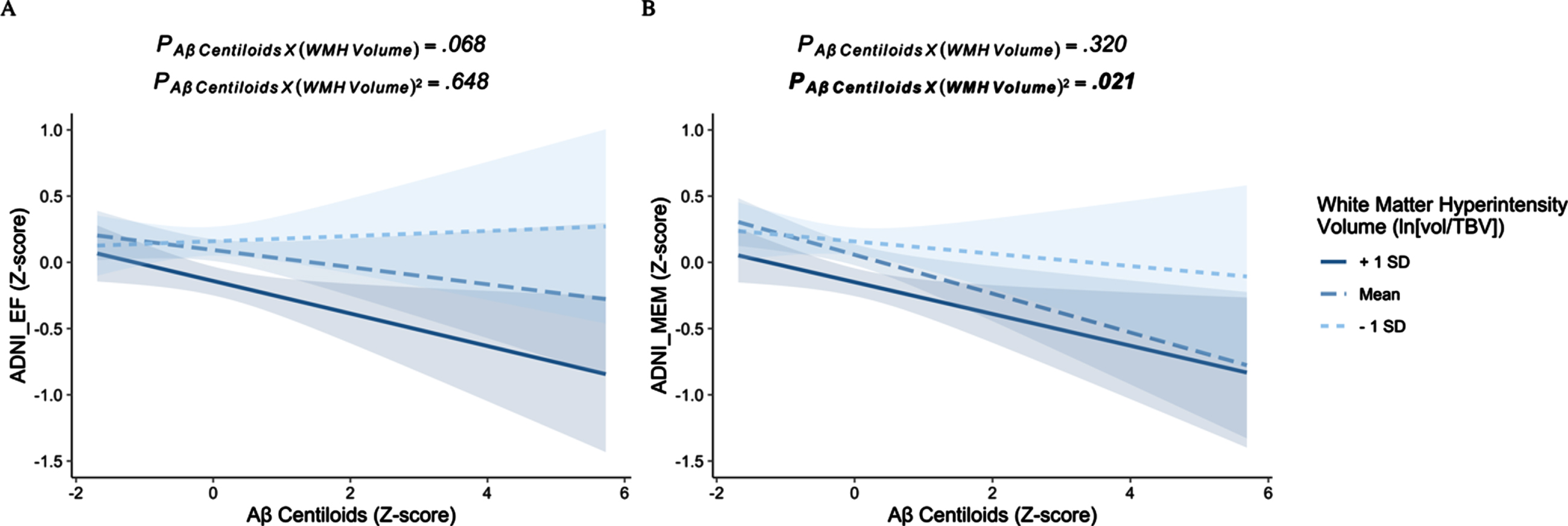
Table 2
Model parameters of the Aβ centiloids by WMH volume interactions
Executive Function | Memory | ||||||
[n = 581*] | [n = 584] | ||||||
Estimate | SE | p | Estimate | SE | p | ||
(Intercept) | 0.011 | 0.064 | 0.865 | –0.260 | 0.062 | <0.001 | |
Age | –0.242 | 0.043 | <0.001 | –0.091 | 0.042 | 0.031 | |
Sex | |||||||
Male | – | – | – | – | – | – | |
Female | 0.183 | 0.073 | 0.012 | 0.640 | 0.070 | <0.001 | |
Education | 0.211 | 0.036 | <0.001 | 0.240 | 0.034 | <0.001 | |
APOE ɛ4 | |||||||
0 alleles | – | – | – | – | – | – | |
1 allele | 0.044 | 0.082 | 0.596 | –0.006 | 0.079 | 0.938 | |
2 alleles | –0.573 | 0.167 | 0.001 | –0.482 | 0.160 | 0.003 | |
Pulse pressure | –0.020 | 0.037 | 0.588 | –0.016 | 0.036 | 0.657 | |
Braak III/IV tau– PET SUVR | –0.225 | 0.040 | <0.001 | –0.263 | 0.039 | <0.001 | |
Aβ Centiloids | –0.065 | 0.051 | 0.201 | –0.147 | 0.049 | 0.003 | |
WMH volume (linear) | –0.150 | 0.043 | <0.001 | –0.154 | 0.041 | <0.001 | |
WMH volume (quadratic) | –0.084 | 0.024 | <0.001 | –0.053 | 0.023 | 0.019 | |
Aβ Centiloids by WMH volume (linear) | –0.071 | 0.039 | 0.068 | –0.037 | 0.037 | 0.320 | |
Aβ Centiloids by WMH volume (quadratic) | 0.013 | 0.029 | 0.648 | 0.063 | 0.027 | 0.021 |
*When assessing for influential values using the deleted fit statistic (see Methods), the results of this model were unduly driven by a single participant. Therefore, this participant was excluded from this model. PET, positron emission tomography; SUVR, standardized uptake value ratio; WMH, white matter hyperintensity.
WMH volume by Aβ-PET centiloids interaction on memory
Independent of Braak III/IV tau-PET SUVR, there was a significant interaction between centiloids and the quadratic effect of WMH volume on memory (βstd=0.063, p = 0.021) (Table 2). Examination of simple slopes suggested that greater Aβ burden was associated with poorer memory at both average (SS=-0.147, p = 0.003) and higher levels of WMH burden (+1 SD; SS = -0.120, p = 0.018), but not at low levels of WMH burden (-1 SD; SS = -0.047, p = 0.440) (Fig. 1B). Notably, while effects at both higher and average levels of WMH burden were statistically significant, the magnitude of effect was found to be greater at the average level of WMH burden compared to higher level of WMH burden. In this model, Braak III/IV tau-PET was significantly associated with memory (βstd=-0.263, p < 0.001). Results remained qualitatively similar when adding hippocampal volume to the model (Supplementary Table 2).
WMH volume by tau-PET SUVR interaction on executive function
Independent of centiloids, there were no significant interactions between Braak III/IV tau-PET and either the linear or quadratic main effect of WMH volume on executive function (βstds≤|0.026|, ps≥0.561) (Table 3). Tau-PET was significantly associated with executive function at both higher (+1 SD), average, and lower (-1 SD) levels of WMH volume (SSs≤-0.193, ps≤0.004) (Fig. 2A). Centiloids were not significantly associated with executive function in this model (βstd=-0.065, p = 0.125). Results remained qualitatively similar when adding hippocampal volume to the model (Supplementary Table 3).
Fig. 2
The effects of tau-PET on cognition at varying levels of white matter hyperintensity burden. There is no amyloid-independent interaction between tau-PET and either the linear or quadratic effects of white matter hyperintensity volume on either (A) executive function or (B) memory.
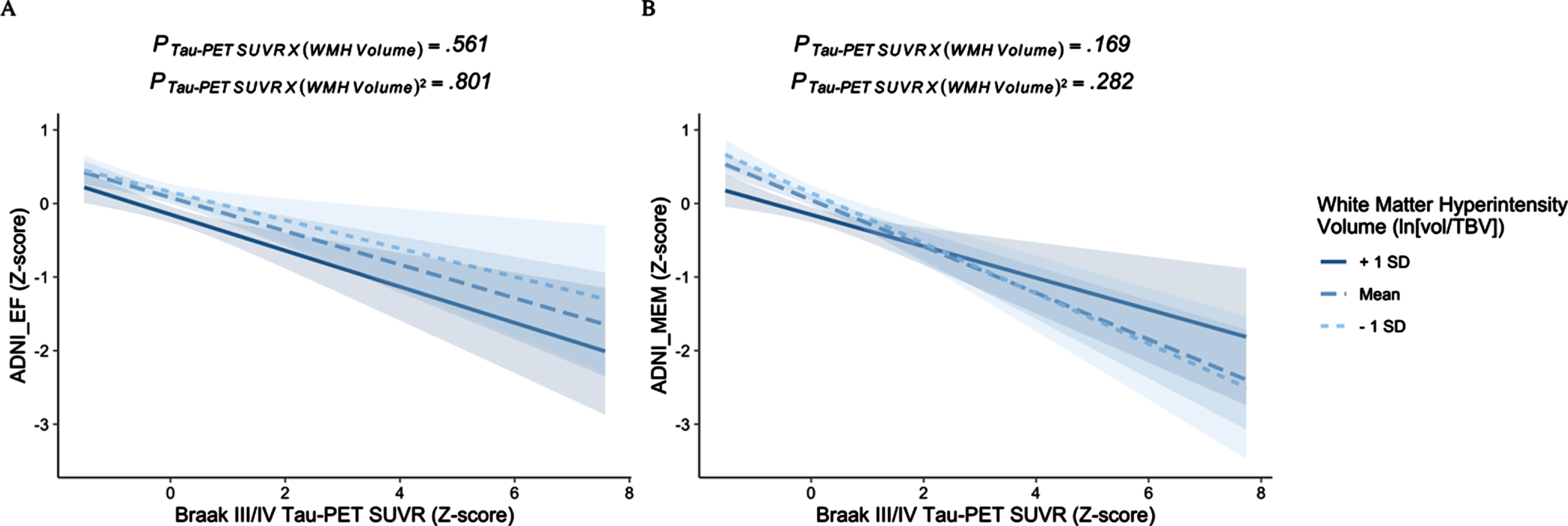
Table 3
Model parameters of the tau-PET by WMH volume interactions
Executive Function | Memory [n = 583*] | ||||||
[n = 582] | |||||||
Estimate | SE | p | Estimate | SE | p | ||
(Intercept) | –0.012 | 0.064 | 0.849 | –0.270 | 0.062 | <0.001 | |
Age | –0.246 | 0.044 | <0.001 | –0.083 | 0.042 | 0.048 | |
Sex | |||||||
Male | – | – | – | – | – | – | |
Female | 0.198 | 0.073 | 0.007 | 0.639 | 0.070 | <0.001 | |
Education | 0.210 | 0.036 | <0.001 | 0.233 | 0.034 | <0.001 | |
APOE ɛ4 | |||||||
0 alleles | – | – | – | – | – | – | |
1 allele | 0.046 | 0.083 | 0.579 | –0.009 | 0.079 | 0.910 | |
2 alleles | –0.515 | 0.166 | 0.002 | –0.435 | 0.159 | 0.006 | |
Pulse pressure | –0.019 | 0.037 | 0.607 | –0.034 | 0.035 | 0.336 | |
Aβ Centiloids | –0.065 | 0.043 | 0.125 | –0.091 | 0.041 | 0.026 | |
Braak III/IV tau– PET SUVR | –0.228 | 0.047 | <0.001 | –0.316 | 0.044 | <0.001 | |
WMH volume (linear) | –0.154 | 0.043 | <0.001 | –0.148 | 0.041 | <0.001 | |
WMH volume (quadratic) | –0.077 | 0.025 | 0.002 | –0.053 | 0.024 | 0.025 | |
Tau– PET by WMH volume (linear) | –0.026 | 0.045 | 0.561 | 0.064 | 0.046 | 0.169 | |
Tau-PET by WMH volume (quadratic) | 0.009 | 0.035 | 0.801 | 0.038 | 0.035 | 0.282 |
*When assessing for influential values using the deleted fit statistic (see Methods), the results of this model were unduly driven by a single participant. Therefore, this participant was excluded from this model. PET, positron emission tomography; SUVR, standardized uptake value ratio; WMH, white matter hyperintensity.
WMH volume by tau-PET SUVR interaction on memory
Independent of centiloids, there were no significant interactions between Braak III/IV tau-PET and either the linear or quadratic main effect of WMH volume on memory (βstds≤|0.064|, ps≥0.169) (Table 3). Tau-PET was significantly associated with memory at both higher (+1 SD), average, and lower (-1 SD) levels of WMH volume (SSs≤-0.215, ps < 0.001) (Fig. 2B). Centiloids were also significantly associated with memory in this model (βstd=-0.091, p = 0.026). Results remained qualitatively similar when adding hippocampal volume to the model (Supplementary Table 3).
DISCUSSION
In this cross-sectional study of older adults without dementia, Aβ interacted with the quadratic effect of WMH burden to influence memory independent of tau. Aβ was associated with memory at average-to-high levels of WMH burden, but not at lower levels of WMH burden. In contrast, Aβ did not interact with either the linear or quadratic effect of WMH burden to influence executive function independent of tau. Furthermore, tau did not interact with either the linear or quadratic effect of WMH burden to influence cognition independent of amyloid.
These findings suggest that Aβ and cerebrovascular pathologies interact synergistically to influence cognition. These results are consistent with previous findings which support a synergism between these pathologies on concurrent cognition, cognitive decline, and cognitive status [11–15]. However, not all studies have supported this synergistic effect [10, 16–18, 20–23]. Results of one study even suggested a possible antagonistic effect such that among individuals with a major vascular cognitive disorder, Aβ positivity was associated with better cognition, while Aβ status did not affect cognition among individuals with a mild vascular cognitive disorder. However, this pattern of results may be related to differences in location and type (e.g., infarcts versus WMH) of cerebrovascular disease biomarker between the Aβ-positive and -negative groups [52]. Importantly, these previous studies did not account for tau pathology, which is known to have a robust effect on cognition. Indeed, the main effect of tau on cognition was stronger than the interaction between Aβ and WMH volume on cognition. Still, the synergistic effect reported in the present study was found to be significant regardless of tau-PET level. While a prior study that accounted for CSF p-tau found no interaction between CSF Aβ and WMH on progression to MCI among cognitively unimpaired participants [26], another study found a tau-PET-independent interaction between Aβ-PET and clinical group (subcortical vascular cognitive impairment versus AD-related cognitive impairment) on cognitive decline [25]. Such variability in findings of synergistic or additive effects between Aβ and cerebrovascular disease may be dependent on whether tau burden is accounted for, the clinical characteristics of the sample, and the operationalization of cerebrovascular disease and Aβ burden. Biomarker modality is likely another contributor to mixed findings, and future studies should seek to compare synergistic associations using both CSF and PET markers in a given sample.
Importantly, our findings differed by cognitive domain. Aβ interacted with the quadratic effect of WMH volume to affect memory, but Aβ did not interact with either the linear or quadratic effect of WMH volume to affect executive function. These results suggest that the degree to which WMH volume affects the relationship between Aβ and memory is further dependent on level of WMH severity. In turn, the effect of Aβ on memory was not significant at low levels of WMH burden but was significant and strongest at a mid-level of WMH burden, and significant but weaker at higher levels of WMH burden. This suggests that the synergistic effect of the two pathologies plateaus at high levels of WMH. Prior work examining the interactive effects of cerebrovascular disease and Aβ on specific cognitive domains has resulted in mixed findings, with some studies showing a synergistic influence on executive function and attention [13], semantic word fluency decline [14], and visuospatial function [11]. Other studies have found no synergistic effect on domain-specific cognition or cognitive decline [10, 16, 18–22], including memory, and even an antagonistic effect on attention and executive function [52]. Given this variability in findings by domain, future studies should seek to better elucidate interactive effects of cerebrovascular disease and Aβ on distinct cognitive domains both cross-sectionally and longitudinally, including considering potential quadratic effects of WMH volume, which, to our knowledge, has not been reported previously.
The mechanisms by which the interaction between Aβ and cerebrovascular disease affect memory independent of tau remains unclear. Synergistic effects between WMH and Aβ have been associated with reduced hippocampal volume in older adults without dementia [53], suggesting that neurodegeneration is a potential mechanism by which cognition may be affected. However, our sensitivity analyses including hippocampal volume as an additional predictor demonstrated that this did not account for the synergistic effects in our analyses. Effects of WMH on memory may also be a result of white matter tract disruption and functional network alterations [54, 55], though it is unclear to what degree Aβ may further potentiate those effects. Future work should seek to design and test mediation models to better understand what mechanisms drive the effects of interaction of amyloid and cerebrovascular disease on cognition. Additionally, cerebrovascular lesions are thought to result from injury due to vascular risk factors. Studies of vascular risk have reported results consistent with a synergistic relationship with Aβ, including synergistic effects on longitudinal cognitive decline and both cross-sectional and longitudinal atrophy in cognitively normal participants [56–58]. These studies suggest that vascular risk may interact with Aβ through mechanisms other than WMH which could include lesions not captured by conventional MRI, such as microinfarcts or blood-brain barrier dysfunction. However, in our study, the synergistic effects between WMH and Aβ on memory were discovered while adjusting for vascular risk measured by pulse pressure, suggesting that this cannot be fully accounted for by arterial stiffening. It will be important for future studies to examine the differential effects of cerebrovascular disease and vascular risk on cognition, and their interaction with AD biomarkers. Overall, these results are supportive of moving on from the Aβ hypothesis given that Aβ alone does not appear to sufficiently account for AD-related cognitive impairment.
Finally, our study found no interaction between WMH volume and tau-PET on cognition, such that the effect of tau on cognition was significant at all levels of WMH volume. These results are consistent with previous investigations which found no interaction between WMH volume and CSF p-tau on either cognition in young onset dementia [10] or risk of cognitively normal older adults progressing to MCI [26], suggesting that the effects of cerebrovascular disease and tau are likely additive rather than synergistic. However, another study has reported differing results, finding that tau does interact with cerebrovascular disease to impact clinical outcomes independent of Aβ [25]. Furthermore, an interaction between tau and vascular risk on 1-year memory performance has also been reported [59]. Ultimately, these results further highlight the need for future research disentangling cerebrovascular disease and vascular risk, and how they may interact with AD pathology.
This study had several limitations. First, this sample consisted of mostly white, highly educated individuals, thereby having a substantial lack of racial and ethnic diversity. Future research is needed to improve generalizability of these results by including participants with greater diversity in race, ethnicity, and educational attainment. Similarly, given the inclusion/exclusion criteria of ADNI [28], this sample had low vascular risk burden overall, and future research should seek to investigate the role that vascular risk burden may play in the relationships between AD and cerebrovascular biomarkers on cognition. Furthermore, the present study examined total WMH volume only. However, prior research has suggested that particular regions of WMH may be most robustly associated with AD [60], and it is worth investigating interactive effects of AD biomarkers with regional WMH volume. Finally, WMH volume may reflect pathologies other than small vessel disease [61], including some degree of Wallerian degeneration as a result of AD pathology [62]. Relatedly, WMH does not necessarily capture other measures of small vessel disease, including microbleeds, lacunes, and other features that are not currently readily assessed via MRI [61]. Therefore, future research should seek to disentangle the pathologies represented by WMH and their associations with cognition, as well as investigate additional measures of small vessel and other cerebrovascular disease.
This study had several strengths. We utilized a relatively large sample with multimodal imaging data available across both cognitively unimpaired and MCI participants. Additionally, the use of continuous measures of imaging biomarker data allowed for modeling of quadratic effects of WMH and is generally thought to increase statistical power. Finally, a strength of our study was the examination of both memory and executive function. To the best of our knowledge, this is the first study to investigate whether WMH volume moderates the independent association between each AD biomarker and multidomain cognition. Still, future work should seek to examine these associations in relation to additional and more precise cognitive domains, including domains such as processing speed, visuospatial function, and language.
These findings highlight the value of incorporating vascular pathology into the biomarker assessment of AD. Given both the frequency of mixed brain pathologies [7] and the fact that many cohort studies and clinical trials tend to exclude mixed AD and cerebrovascular pathologies, future research should seek to better understand their combined effects. Furthermore, these findings suggest that prevention of cerebrovascular injury may attenuate the negative effects of Aβ on cognition. Both vascular and AD pathologies should be considered in the development of future treatment targets, including modifiable risk factors of cerebrovascular disease.
ACKNOWLEDGMENTS
The authors have no acknowledgments to report.
FUNDING
This work was supported by the U.S. Department of Veterans Affairs Clinical Sciences Research and Development Service [Career Development Award-2 1IK2CX001865 to K.R.T. and Merit Award 1I01CX001842 to K.J.B], NIH grants [R01 AG063782 to K.J.B., R03 AG070435 to K.R.T., R01 AG049810 to M.W.B.], and the Alzheimer’s Association [AARF-17-528918 to K.R.T., AARG-18-566254 to K.J.B.].
Data collection and sharing for this project was funded by the Alzheimer’s Disease Neuroimaging Initiative (ADNI) [National Institutes of Health Grant U01 AG024904] and DOD ADNI [Department of Defense award number W81XWH-12-2-0012]. ADNI is funded by the National Institute on Aging, the National Institute of Biomedical Imaging and Bioengineering, and through generous contributions from the following: AbbVie, Alzheimer’s Association; Alzheimer’s Drug Discovery Foundation; Araclon Biotech; BioClinica, Inc.; Biogen; Bristol-Myers Squibb Company; CereSpir, Inc.; Cogstate; Eisai Inc.; Elan Pharmaceuticals, Inc.; Eli Lilly and Company; EuroImmun; F. Hoffmann-La Roche Ltd and its affiliated company Genentech, Inc.; Fujirebio; GE Healthcare; IXICO Ltd.; Janssen Alzheimer Immunotherapy Research & Development, LLC.; Johnson & Johnson Pharmaceutical Research & Development LLC.; Lumosity; Lundbeck; Merck & Co., Inc.; Meso Scale Diagnostics, LLC.; NeuroRx Research; Neurotrack Technologies; Novartis Pharmaceuticals Corporation; Pfizer Inc.; Piramal Imaging; Servier; Takeda Pharmaceutical Company; and Transition Therapeutics. The Canadian Institutes of Health Research is providing funds to support ADNI clinical sites in Canada. Private sector contributions are facilitated by the Foundation for the National Institutes of Health (http://www.fnih.org). The grantee organization is the Northern California Institute for Research and Education, and the study is coordinated by the Alzheimer’s Therapeutic Research Institute at the University of Southern California. ADNI data are disseminated by the Laboratory for Neuro Imaging at the University of Southern California.
CONFLICT OF INTEREST
Dr. Bondi is a paid consultant for Eisai, Novartis and Roche Pharmaceuticals and receives royalties from Oxford University Press.
Dr. Thomas, Dr. Nation, Dr. Bondi, and Dr. Bangen are Editorial Board members of the Journal of Alzheimer’s Disease and were not involved in peer reviewing the present paper.
No other authors have competing interests to declare.
DATA AVAILABILITY
Publicly available datasets were analyzed in this study. This data can be found here: http://adni.loni.usc.edu/.
SUPPLEMENTARY MATERIAL
[1] The supplementary material is available in the electronic version of this article: https://dx.doi.org/10.3233/JAD-221209.
REFERENCES
[1] | McKhann , Drachman D , Folstein M , Katzman R , Price D , Stadlan EM ((1984) ) Clinical diagnosis of Alzheimer’s disease: Report of the NINCDS-ADRDA Work Group under the auspices of Department of Health and Human Services Task Force on Alzheimer’s Disease. Neurology 34: , 939–944. |
[2] | Nelson PT , Alafuzoff I , Bigio EH , Bouras C , Braak H , Cairns NJ , Castellani RJ , Crain BJ , Davies P , Tredici KD , Duyckaerts C , Frosch MP , Haroutunian V , Hof PR , Hulette CM , Hyman BT , Iwatsubo T , Jellinger KA , Jicha GA , Kövari E , Kukull WA , Leverenz JB , Love S , Mackenzie IR , Mann DM , Masliah E , McKee AC , Montine TJ , Morris JC , Schneider JA , Sonnen JA , Thal DR , Trojanowski JQ , Troncoso JC , Wisniewski T , Woltjer RL , Beach TG ((2012) ) Correlation of Alzheimer disease neuropathologic changes with cognitive status: A review of the literature. J Neuropathol Exp Neurol 71: , 362–381. |
[3] | Brier MR , Gordon B , Friedrichsen K , McCarthy J , Stern A , Christensen J , Owen C , Aldea P , Su Y , Hassenstab J , Cairns NJ , Holtzman DM , Fagan AM , Morris JC , Benzinger TLS , Ances BM ((2016) ) Tau and Aβ imaging, CSF measures, and cognition in Alzheimer’s disease. Sci Transl Med 8: , 338ra66. |
[4] | Bejanin A , Schonhaut DR , La Joie R , Kramer JH , Baker SL , Sosa N , Ayakta N , Cantwell A , Janabi M , Lauriola M , O’Neil JP , Gorno-Tempini ML , Miller ZA , Rosen HJ , Miller BL , Jagust WJ , Rabinovici GD ((2017) ) Tau pathology and neurodegeneration contribute to cognitive impairment in Alzheimer’s disease. Brain 140: , 3286–3300. |
[5] | Ossenkoppele R , Schonhaut DR , Schöll M , Lockhart SN , Ayakta N , Baker SL , O’Neil JP , Janabi M , Lazaris A , Cantwell A , Vogel J , Santos M , Miller ZA , Bettcher BM , Vossel KA , Kramer JH , Gorno-Tempini ML , Miller BL , Jagust WJ , Rabinovici GD ((2016) ) Tau PET patterns mirror clinical and neuroanatomical variability in Alzheimer’s disease. Brain 139: , 1551–1567. |
[6] | Jansen WJ , Ossenkoppele R , Knol DL , Tijms BM , Scheltens P , Verhey FRJ , Visser PJ , Amyloid Biomarker Study Group, Aalten P , Aarsland D , Alcolea D , Alexander M , Almdahl IS , Arnold SE , Baldeiras I , Barthel H , van Berckel BNM , Bibeau K , Blennow K , Brooks DJ , van Buchem MA , Camus V , Cavedo E , Chen K , Chetelat G , Cohen AD , Drzezga A , Engelborghs S , Fagan AM , Fladby T , Fleisher AS , van der Flier WM , Ford L , Förster S , Fortea J , Foskett N , Frederiksen KS , Freund-Levi Y , Frisoni GB , Froelich L , Gabryelewicz T , Gill KD , Gkatzima O , Gómez-Tortosa E , Gordon MF , Grimmer T , Hampel H , Hausner L , Hellwig S , Herukka S-K , Hildebrandt H , Ishihara L , Ivanoiu A , Jagust WJ , Johannsen P , Kandimalla R , Kapaki E , Klimkowicz-Mrowiec A , Klunk WE , Köhler S , Koglin N , Kornhuber J , Kramberger MG , Van Laere K , Landau SM , Lee DY , de Leon M , Lisetti V , Lleó A , Madsen K , Maier W , Marcusson J , Mattsson N , deMendonça A , Meulenbroek O , Meyer PT , Mintun MA , Mok V , Molinuevo JL , Møllergård HM , Morris JC , Mroczko B , Van der Mussele S , Na DL , Newberg A , Nordberg A , Nordlund A , Novak GP , Paraskevas GP , Parnetti L , Perera G , Peters O , Popp J , Prabhakar S , Rabinovici GD , Ramakers IHGB , Rami L , Resende de Oliveira C , Rinne JO , Rodrigue KM , Rodríguez-Rodríguez E , Roe CM , Rot U , Rowe CC , Rüther E , Sabri O , Sanchez-Juan P , Santana I , Sarazin M , Schröder J , Schütte C , Seo SW , Soetewey F , Soininen H , Spiru L , Struyfs H , Teunissen CE , Tsolaki M , Vandenberghe R , Verbeek MM , Villemagne VL , Vos SJB , van Waalwijk van Doorn LJC , Waldemar G , Wallin A , Wallin ÅK , Wiltfang J , Wolk DA , Zboch M , Zetterberg H ((2015) ) Prevalence of cerebral amyloid pathology in persons withoutdementia: A meta-analysis. JAMA 313: , 1924–1938. |
[7] | Schneider JA , Arvanitakis Z , Bang W , Bennett DA ((2007) ) Mixed brain pathologies account for most dementia cases in community-dwelling older persons. Neurology 69: , 2197–2204. |
[8] | Lee S , Viqar F , Zimmerman ME , Narkhede A , Tosto G , Benzinger TLS , Marcus DS , Fagan AM , Goate A , Fox NC , Cairns NJ , Holtzman DM , Buckles V , Ghetti B , McDade E , Martins RN , Saykin AJ , Masters CL , Ringman JM , Ryan NS , Förster S , Laske C , Schofield PR , Sperling RA , Salloway S , Correia S , Jack CJr. , Weiner M , Bateman RJ , Morris JC , Mayeux R , Brickman AM , Dominantly Inherited Alzheimer Network ((2016) ) White matter hyperintensities are a core feature of Alzheimer’s disease: Evidence from the dominantly inherited Alzheimer network. Ann Neurol 79: , 929–939. |
[9] | Calvin CM , de Boer C , Raymont V , Gallacher J , Koychev I , The European Prevention of Alzheimer’s Dementia (EPAD) Consortium ((2020) ) Prediction of Alzheimer’s disease biomarker status defined by the ‘ATN framework’ among cognitively healthy individuals: Results from the EPAD longitudinal cohort study. Alzheimers Res Ther 12: , 143. |
[10] | Yatawara C , Ng KP , Cristine Guevarra A , Wong B , Yong T , Kandiah N ((2020) ) Small vessel disease and associations with cerebrospinal fluid amyloid, tau, and neurodegeneration (ATN) biomarkers and cognition in young onset dementia. J Alzheimers Dis 77: , 1305–1314. |
[11] | Lee MJ , Seo SW , Na DL , Kim C , Park JH , Kim GH , Kim CH , Noh Y , Cho H , Kim HJ , Yoon CW , Ye BS , Chin J , Jeon S , Lee J-M , Choe YS , Lee K-H , Kim JS , Kim ST , Lee J-H , Ewers M , Werring DJ , Weiner MW ((2014) ) Synergistic effects of ischemia and β-amyloid burden on cognitive decline in patients with subcortical vascular mild cognitive impairment. JAMA Psychiatry 71: , 412–422. |
[12] | Saridin FN , Hilal S , Villaraza SG , Reilhac A , Gyanwali B , Tanaka T , Stephenson MC , Ng SL , Vrooman H , van der Flier WM , Chen CLH ((2020) ) Brain amyloid β, cerebral small vessel disease, and cognition: A memory clinic study. Neurology 95: , e2845–e2853. |
[13] | Dupont PS , Bocti C , Joannette M , Lavallée MM , Nikelski J , Vallet GT , Chertkow H , Joubert S ((2020) ) Amyloid burden and white matter hyperintensities mediate age-related cognitive differences. Neurobiol Aging 86: , 16–26. |
[14] | Ye BS , Seo SW , Kim J-H , Kim GH , Cho H , Noh Y , Kim HJ , Yoon CW , Woo S , Kim SH , Park HK , Kim ST , Choe YS , Lee KH , Kim JS , Oh SJ , Kim C , Weiner M , Lee J-H , Na DL ((2015) ) Effects of amyloid and vascular markers on cognitive decline in subcortical vascular dementia. Neurology 85: , 1687–1693. |
[15] | Yassi N , Hilal S , Xia Y , Lim YY , Watson R , Kuijf H , Fowler C , Yates P , Maruff P , Martins R , Ames D , Chen C , Rowe CC , Villemagne VL , Salvado O , Desmond PM , Masters CL ((2020) ) Influence of comorbidity of cerebrovascular disease and amyloid-β on Alzheimer’s disease. J Alzheimers Dis 73: , 897–907. |
[16] | Marchant NL , Reed BR , DeCarli CS , Madison CM , Weiner MW , Chui HC , Jagust WJ ((2012) ) Cerebrovascular disease, beta-amyloid, and cognition in aging. Neurobiol Aging 33: , 1006.e25–1006.e36. |
[17] | Vemuri P , Lesnick TG , Przybelski SA , Knopman DS , Preboske GM , Kantarci K , Raman MR , Machulda MM , Mielke MM , Lowe VJ , Senjem ML , Gunter JL , Rocca WA , Roberts RO , Petersen RC , Jack CR ((2015) ) Vascular and amyloid pathologies are independent predictors of cognitive decline in normal elderly. Brain 138: , 761–771. |
[18] | Garnier-Crussard A , Bougacha S , Wirth M , André C , Delarue M , Landeau B , Mézenge F , Kuhn E , Gonneaud J , Chocat A , Quillard A , Ferrand-Devouge E , de La Sayette V , Vivien D , Krolak-Salmon P , Chételat G ((2020) ) White matter hyperintensities across the adult lifespan: Relation to age, Aβ load, and cognition. Alzheimers Res Ther 12: , 127. |
[19] | Nebes RD , Snitz BE , Cohen AD , Aizenstein HJ , Saxton JA , Halligan EM , Mathis CA , Price JC , Kamboh MI , Weissfeld LA , Klunk WE ((2013) ) Cognitive aging in persons with minimal amyloid-β and white matter hyperintensities. Neuropsychologia 51: , 2202–2209. |
[20] | Ortega G , Espinosa A , Alegret M , Monté-Rubio Gc , Sotolongo-Grau O , Sanabria A , Tartari Jp , Rodríguez-Gómez O , Marquié M , Vivas A , Gómez-Chiari M , Alarcón-Martín E , Pérez-Cordón A , Roberto N , Hernández I , Rosende-Roca M , Vargas L , Mauleón A , Abdelnour C , Esteban De Antonio E , López-Cuevas R , Alonso-Lana S , Moreno-Grau S , de Rojas I , Orellana A , Montrreal L , Tárraga L , Ruiz A , Boada M , Valero S , FACEHBI group , Aguilera N , Alonso-Lana S , Berthier M , Buendia M , Bullich S , Campos F , Cañabate P , Cañada L , Cuevas C , Diego S , Gailhajenet A , García P , Giménez J , Gismondi R , Guitart M , Ibarria M , Lafuente A , Lomeña F , Masip E , Martín E , Martínez J , Moreno M , Niñerola A , Nogales AB , Núñez L , Páez A , Pancho A , Pelejà E , Pérez-Grijalba V , Perissinotti A , Pesini P , Preckler S , Roé-Vellvé N , Romero J , Ramis MI , Sarasa M , Tejero MA , Torres M ((2021) ) Combination of white matter hyperintensities and Aβ burden is related to cognitive composites domain scores in subjective cognitive decline: TheFACEHBI cohort. Alzheimers Res Ther 13: , 141. |
[21] | Park J-H , Seo SW , Kim C , Kim SH , Kim GH , Kim ST , Jeon S , Lee JM , Oh SJ , Kim JS , Choe YS , Lee K-H , Shin JS , Kim CH , Noh Y , Cho H , Yoon CW , Kim HJ , Ye BS , Ewers M , Weiner MW , Lee J-H , Werring DJ , Na DL ((2014) ) Effects of cerebrovascular disease and amyloid beta burden on cognition in subjects with subcortical vascular cognitive impairment. Neurobiol Aging 35: , 254–260. |
[22] | Bos I , Verhey FR , Ramakers IHGB , Jacobs HIL , Soininen H , Freund-Levi Y , Hampel H , Tsolaki M , Wallin ÅK , van Buchem MA , Oleksik A , Verbeek MM , Olde Rikkert M , van der Flier WM , Scheltens P , Aalten P , Visser PJ , Vos SJB ((2017) ) Cerebrovascular and amyloid pathology in predementia stages: The relationship with neurodegeneration and cognitive decline. Alzheimers Res Ther 9: , 101. |
[23] | Gordon BA , Najmi S , Hsu P , Roe CM , Morris JC , Benzinger TLS ((2015) ) The effects of white matter hyperintensities and amyloid deposition on Alzheimer dementia. Neuroimage Clin 8: , 246–252. |
[24] | Provenzano FA , Muraskin J , Tosto G , Narkhede A , Wasserman BT , Griffith EY , Guzman VA , Meier IB , Zimmerman ME , Brickman AM , Alzheimer’s Disease Neuroimaging Initiative ((2013) ) White matter hyperintensities and cerebral amyloidosis: Necessary and sufficient for clinical expression of Alzheimer disease? JAMA Neurol 70: , 455–461. |
[25] | Jang H , Kim HJ , Choe YS , Kim S-J , Park S , Kim Y , Kim KW , Lyoo CH , Cho H , Ryu YH , Choi JY , DeCarli C , Na DL , Seo SW , Alzheimer’s Disease Neuroimaging Initiative ((2020) ) The impact of amyloid-β or tau on cognitive change in the presence of severe cerebrovascular disease. J Alzheimers Dis 78: , 573–585. |
[26] | Soldan A , Pettigrew C , Zhu Y , Wang M-C , Moghekar A , Gottesman RF , Singh B , Martinez O , Fletcher E , DeCarli C , Albert M , BIOCARD Research Team ((2020) ) White matter hyperintensities and CSF Alzheimer disease biomarkers in preclinical Alzheimer disease. Neurology 94: , e950–e960. |
[27] | Sweeney MD , Montagne A , Sagare AP , Nation DA , Schneider LS , Chui HC , Harrington MG , Pa J , Law M , Wang DJJ , Jacobs RE , Doubal FN , Ramirez J , Black SE , Nedergaard M , Benveniste H , Dichgans M , Iadecola C , Love S , Bath PM , Markus HS , Salman RA , Allan SM , Quinn TJ , Kalaria RN , Werring DJ , Carare RO , Touyz RM , Williams SCR , Moskowitz MA , Katusic ZS , Lutz SE , Lazarov O , Minshall RD , Rehman J , Davis TP , Wellington CL , González HM , Yuan C , Lockhart SN , Hughes TM , Chen CLH , Sachdev P , O’Brien JT , Skoog I , Pantoni L , Gustafson DR , Biessels GJ , Wallin A , Smith EE , Mok V , Wong A , Passmore P , Barkof F , Muller M , Breteler MMB , Román GC , Hamel E , Seshadri S , Gottesman RF , van Buchem MA , Arvanitakis Z , Schneider JA , Drewes LR , Hachinski V , Finch CE , Toga AW , Wardlaw JM , Zlokovic BV ((2019) ) Vascular dysfunction-The disregarded partner of Alzheimer’s disease. Alzheimers Dement 15: , 158–167. |
[28] | Petersen RC , Aisen PS , Beckett LA , Donohue MC , Gamst AC , Harvey DJ , Jack CR , Jagust WJ , Shaw LM , Toga AW , Trojanowski JQ , Weiner MW ((2010) ) Alzheimer’s Disease Neuroimaging Initiative (ADNI): Clinical characterization. Neurology 74: , 201–209. |
[29] | Bondi MW , Edmonds EC , Jak AJ , Clark LR , Delano-Wood L , McDonald CR , Nation DA , Libon DJ , Au R , Galasko D , Salmon DP ((2014) ) Neuropsychological criteria for mild cognitive impairment improves diagnostic precision, biomarker associations, and progression rates. J Alzheimers Dis 42: , 275–289. |
[30] | Weigand AJ , Thomas KR , Bangen KJ , Eglit GML , Delano-Wood L , Gilbert PE , Brickman AM , Bondi MW , Alzheimer’s Disease Neuroimaging Initiative ((2021) ) APOE interacts with tau PET to influence memory independently of amyloid PET in older adults without dementia. Alzheimers Dement 17: , 61–69. |
[31] | Landau S, Murphy A, Lee J, Ward T, Jagust W. Florbetaben (FBB) processing methods. https://adni.bitbucket.io/reference/docs/UCBERKELEYFBB/UCBerkeley_FBB_Methods_11.15.2021.pdf. |
[32] | Landau S, Ward TJ, Murphy A, Jagust W (2021) Flortaucipir (AV-1451) processing methods. https://adni.bitbucket.io/reference/docs/UCBERKELEYAV1451/UCBERKELEY_AV1451_Methods_2021-01-14.pdf.pdf. |
[33] | Landau S, Murphy A, Ward T, Jagust W (2021) Florbetapir (AV45)processing methods. https://adni.bitbucket.io/reference/docs/UCBERKELEYAV45/UCBERKELEY_AV45_Methods_11.15.2021.pdf. |
[34] | Royse SK , Cohen AD , Snitz BE , Rosano C ((2021) ) Differences in Alzheimer’s disease and related dementias pathology among African American and Hispanic women: A qualitative literature review of biomarker studies. Front Syst Neurosci 15: , 685957. |
[35] | Baker SL , Maass A , Jagust WJ ((2017) ) Considerations and code for partial volume correcting [18F]-AV-1451 tau PET data. Data Brief 15: , 648–657. |
[36] | Jack CR Jr. , Bernstein MA , Fox NC , Thompson P , Alexander G , Harvey D , Borowski B , Britson PJ , L. Whitwell J , Ward C , Dale AM , Felmlee JP , Gunter JL , Hill DLG , Killiany R , Schuff N , Fox-Bosetti S , Lin C , Studholme C , DeCarli CS , Krueger G , Ward HA , Metzger GJ , Scott KT , Mallozzi R , Blezek D , Levy J , Debbins JP , Fleisher AS , Albert M , Green R , Bartzokis G , Glover G , Mugler J , Weiner MW ((2008) ) The Alzheimer’s disease neuroimaging initiative (ADNI): MRI methods. J Magn Reson Imaging 27: , 685–691. |
[37] | Maillard P , Lu H , Arfanakis K , Gold BT , Bauer CE , Zachariou V , Stables L , Wang DJJ , Jann K , Seshadri S , Duering M , Hillmer LJ , Rosenberg GA , Snoussi H , Sepehrband F , Habes M , Singh B , Kramer JH , Corriveau RA , Singh H , Schwab K , Helmer KG , Greenberg SM , Caprihan A , DeCarli C , Satizabal CL , MarkVCID Consortium ((2022) ) Instrumental validation of free water, peak-width of skeletonized mean diffusivity, and white matter hyperintensities: MarkVCID neuroimaging kits. Alzheimers Dement (Amst) 14: , e12261. |
[38] | Kochunov P , Lancaster JL , Thompson P , Woods R , Mazziotta J , Hardies J , Fox P ((2001) ) Regional spatial normalization: Toward an optimal target. J Comput Assist Tomogr 25: , 805–816. |
[39] | Lin C , Huang C-M , Fan Y-T , Liu H-L , Chen Y-L , Aizenstein HJ , Lee TM-C , Lee S-H ((2020) ) Cognitive reserve moderates effects of white matter hyperintensity on depressive symptoms and cognitive function in late-life depression. Front Psychiatry 11: , 249. |
[40] | Bangen KJ , Preis SR , Delano-Wood L , Wolf PA , Libon DJ , Bondi MW , Au R , DeCarli C , Brickman AM ((2018) ) Baseline white matter hyperintensities and hippocampal volume are associated with conversion from normal cognition to mild cognitive impairment in the Framingham Offspring Study. Alzheimer Dis Assoc Disord 32: , 50–56. |
[41] | Vercauteren T , Pennec X , Perchant A , Ayache N ((2007) ) Non-parametric diffeomorphic image registration with the demons algorithm. In Medical Image Computing and Computer-Assisted Intervention – MICCAI 2007, AyacheN, OurselinS, MaederA, eds. Springer, Berlin, Heidelberg, pp. 319–326. |
[42] | Fletcher E , Singh B , Harvey D , Carmichael O , DeCarli C ((2012) ) Adaptive image segmentation for robust measurement of longitudinal brain tissue change. In 2012 Annual International Conference of the IEEE Engineering in Medicine and Biology Society, pp.5319–5322. |
[43] | Boccardi M , Bocchetta M , Ganzola R , Robitaille N , Redolfi A , Duchesne S , Jack CR Jr. , Frisoni GB , EADC-ADNI Working Group on The Harmonized Protocol for Manual Hippocampal Segmentation and for the Alzheimer’s Disease Neuroimaging Initiative, Bartzokis G , Csernansky JG , de Leon MJ , deToledo-Morrell L , Killiany RJ , Lehéricy S , Malykhin N , Pantel J , Pruessner JC , Soininen H , Watson C ((2015) ) Operationalizing protocol differences for EADC-ADNI manual hippocampal segmentation. Alzheimers Dement 11: , 184–194. |
[44] | Wang H , Suh JW , Das SR , Pluta JB , Craige C , Yushkevich PA ((2013) ) Multi-atlas segmentation with joint label fusion. IEEE Trans Pattern Anal Mach Intell 35: , 611–623. |
[45] | Knoops AJG , Gerritsen L , van der Graaf Y , Mali WPTM , Geerlings MI ((2012) ) Loss of entorhinal cortex and hippocampal volumes compared to whole brain volume in normal aging: The SMART-Medea study. Psychiatry Res Neuroimaging 203: , 31–37. |
[46] | Nation DA , Delano-Wood L , Bangen KJ , Wierenga CE , Jak AJ , Hansen LA , Galasko DR , Salmon DP , Bondi MW ((2012) ) Antemortem pulse pressure elevation predicts cerebrovascular disease in autopsy-confirmed Alzheimer’s disease. J Alzheimers Dis 30: , 595–603. |
[47] | Gibbons LE , Carle AC , Mackin RS , Harvey D , Mukherjee S , Insel P , Curtis SM , Gross A , Jones RN , Mungas D , Weiner M , Crane PK , Alzheimer’s Disease Neuroimaging Initiative ((2020) ) Compositemeasures of executive function and memory: ADNI_EF and ADNI_Mem. https://adni.bitbucket.io/reference/docs/UWNPSYCHSUM/ADNI_Methods_UWNPSYCHSUM.pdf. |
[48] | Gibbons LE , Carle AC , Mackin RS , Harvey D , Mukherjee S , Insel P , Curtis SM , Mungas D , Crane PK , for the Alzheimer’s Disease Neuroimaging Initiative ((2012) ) A composite score for executive functioning, validated in Alzheimer’s Disease Neuroimaging Initiative (ADNI) participants with baseline mild cognitive impairment. Brain Imaging Behav 6: , 517–527. |
[49] | Wright CB , Festa JR , Paik MC , Schmiedigen A , Brown TR , Yoshita M , DeCarli C , Sacco R , Stern Y ((2008) ) White matter hyperintensities and subclinical infarction. Stroke 39: , 800–805. |
[50] | Boone KB , Miller BL , Lesser IM , Mehringer CM , Hill-Gutierrez E , Goldberg MA , Berman NG ((1992) ) Neuropsychological correlates of white-matter lesions in healthy elderly subjects: A threshold effect. Arch Neurol 49: , 549–554. |
[51] | Belsley DA , Kuh E , Welsch RE ((1980) ) Regression Diagnostics: Identifying Influential Data and Sources of Collinearity, John Wiley & Sons, Inc. |
[52] | Leijenaar JF , Groot C , Sudre CH , Bergeron D , Leeuwis AE , Cardoso MJ , Carrasco FP , Laforce R , Barkhof F , van der Flier WM , Scheltens P , Prins ND , Ossenkoppele R ((2020) ) Comorbid amyloid-β pathology affects clinical and imaging features in VCD. Alzheimers Dement 16: , 354–364. |
[53] | Freeze WM , Jacobs HIL , Gronenschild EH , Jansen JFA , Burgmans S , Aalten P , Clerx L , Vos SJ , van Buchem MA , Barkhof F , van der Flier WM , Verbeek MM , Rikkert MO , Backes WH , Verhey FR , LeARN project ((2017) ) White matter hyperintensities potentiate hippocampal volume reduction in non-demented older individuals with abnormal amyloid-β. J Alzheimers Dis 55: , 333–342. |
[54] | Smith EE , Salat DH , Jeng J , McCreary CR , Fischl B , Schmahmann JD , Dickerson BC , Viswanathan A , Albert MS , Blacker D , Greenberg SM ((2011) ) Correlations between MRI white matter lesion location and executive function and episodic memory. Neurology 76: , 1492–1499. |
[55] | Reijmer YD , Schultz AP , Leemans A , O’Sullivan MJ , Gurol ME , Sperling R , Greenberg SM , Viswanathan A , Hedden T ((2015) ) Decoupling of structural and functional brain connectivity in older adults with white matter hyperintensities. Neuroimage 117: , 222–229. |
[56] | Rabin JS , Schultz AP , Hedden T , Viswanathan A , Marshall GA , Kilpatrick E , Klein H , Buckley RF , Yang H-S , Properzi M , Rao V , Kirn DR , Papp KV , Rentz DM , Johnson KA , Sperling RA , Chhatwal JP ((2018) ) Interactive associations of vascular risk and β-amyloid burden with cognitive decline in clinically normal elderly individuals: Findings from the Harvard Aging Brain Study. JAMA Neurol 75: , 1124–1131. |
[57] | Rabin JS , Pruzin J , Scott M , Yang H-S , Hampton O , Hsieh S , Schultz AP , Buckley RF , Hedden T , Rentz D , Johnson KA , Sperling RA , Chhatwal JP ((2022) ) Association of β-amyloid and vascular risk on longitudinal patterns of brain atrophy. Neurology 99: , e270–e280. |
[58] | Villeneuve S , Reed BR , Madison CM , Wirth M , Marchant NL , Kriger S , Mack WJ , Sanossian N , DeCarli C , Chui HC , Weiner MW , Jagust WJ ((2014) ) Vascular risk and Aβ interact to reduce cortical thickness in AD vulnerable brain regions. Neurology 83: , 40–47. |
[59] | Weigand AJ , Macomber AJ , Walker KS , Edwards L , Thomas KR , Bangen KJ , Nation DA , Bondi MW , Alzheimer’s Disease Neuroimaging Initiative ((2022) ) Interactive effects of pulse pressure and tau imaging on longitudinal cognition. J Alzheimers Dis 89: , 633–640. |
[60] | Brickman AM , Provenzano FA , Muraskin J , Manly JJ , Blum S , Apa Z , Stern Y , Brown TR , Luchsinger JA , Mayeux R ((2012) ) Regional white matter hyperintensity volume, not hippocampal atrophy, predicts incident Alzheimer disease in the community. Arch Neurol 69: , 1621–1627. |
[61] | Gouw AA , Seewann A , van der Flier WM , Barkhof F , Rozemuller AM , Scheltens P , Geurts JJG ((2011) ) Heterogeneity of small vessel disease: A systematic review of MRI and histopathology correlations. J Neurol Neurosurg Psychiatry 82: , 126–135. |
[62] | McAleese KE , Walker L , Graham S , Moya ELJ , Johnson M , Erskine D , Colloby SJ , Dey M , Martin-Ruiz C , Taylor J-P , Thomas AJ , McKeith IG , De Carli C , Attems J ((2017) ) Parietal white matter lesions in Alzheimer’s disease are associated with cortical neurodegenerative pathology, but not with small vessel disease. Acta Neuropathol (Berl) 134: , 459–473. |