Hypermethylation at CREBBP Is Associated with Cognitive Impairment in a Mexican American Cohort
Abstract
Background:
The aging Mexican American (MA) population is the fastest growing ethnic minority group in the US. MAs have a unique metabolic-related risk for Alzheimer’s disease (AD) and mild cognitive impairment (MCI), compared to non-Hispanic whites (NHW). This risk for cognitive impairment (CI) is multifactorial involving genetics, environmental, and lifestyle factors. Changes in environment and lifestyle can alter patterns and even possibly reverse derangement of DNA methylation (a form of epigenetic regulation).
Objective:
We sought to identify ethnicity-specific DNA methylation profiles that may be associated with CI in MAs and NHWs.
Methods:
DNA obtained from peripheral blood of 551 participants from the Texas Alzheimer’s Research and Care Consortium was typed on the Illumina Infinium® MethylationEPIC chip array, which assesses over 850K CpG genomic sites. Within each ethnic group (N = 299 MAs, N = 252 NHWs), participants were stratified by cognitive status (control versus CI). Beta values, representing relative degree of methylation, were normalized using the Beta MIxture Quantile dilation method and assessed for differential methylation using the Chip Analysis Methylation Pipeline (ChAMP), limma and cate packages in R.
Results:
Two differentially methylated sites were significant: cg13135255 (MAs) and cg27002303 (NHWs) based on an FDR p < 0.05. Three suggestive sites obtained were cg01887506 (MAs) and cg10607142 and cg13529380 (NHWs). Most methylation sites were hypermethylated in CI compared to controls, except cg13529380 which was hypomethylated.
Conclusion:
The strongest association with CI was at cg13135255 (FDR-adjusted p = 0.029 in MAs), within the CREBBP gene. Moving forward, identifying additional ethnicity-specific methylation sites may be useful to discern CI risk in MAs.
INTRODUCTION
Alzheimer’s disease (AD) is currently the 7th leading cause of mortality in the United States (US), though its prevalence is expected to triple over the coming decades as the Baby Boom generation continues to age [1]. Demographic shifts in the US population, however, will precipitate uneven effects affecting health outcomes across racial and ethnic groups [1]. For example, Mexican Americans (MA) who are the largest and fastest growing aging minority group in the US have a unique predisposition for cognitive impairment (e.g., AD and mild cognitive impairment (MCI)) [2–4]. Existing protein biomarker panels for predicting AD risk in MAs include various metabolic factors such as FABP and GLP-1 [5], suggesting that MAs may suffer from a distinct metabolic-related form of AD. In contrast, non-Hispanic whites (NHW) have predominantly inflammation-based AD associated protein biomarkers [5]. Further, MAs are often diagnosed with more severe forms of AD at a younger age relative to NHWs [3, 6]. Despite this, MAs are still likely to live 3 years longer (on average) compared to NHW counterparts— a phenomenon referred to as the “Hispanic Paradox” [7, 8]. The frequency and effect of the Apolipoprotein E (APOE) ɛ4 allele [3, 4], known to confer 3- to 12-fold risk for AD in NHWs [9], is much lower in MAs [3]. Though poorly understood, exploring the intersection of genetics and environmental/lifestyle factors via epigenetic studies may help elucidate the root cause of these differences in AD etiology and presentation.
The multifactorial risk for cognitive impairment (CI) involves both genetics and epigenetics which is influenced by environmental and lifestyle factors [4, 10, 11]. The genetic risk for AD has been estimated to be 58% (based on twin studies) [12]. While over two dozen single nucleotide polymorphisms (SNPs) have been associated with AD risk [13, 14], including ɛ4 allele of the APOE gene [15], this heritability fails to explain the heterogeneity of AD onset and presentation across individuals and racial/ethnic groups, a term referred to as ‘missing heritability’ [13, 16]. Twin studies have shown that monozygotic twins subjected to different environments experience different disease outcomes despite sharing the same genetic risk for disease [12]. This suggests that genetics, environmental, and lifestyle factors play important roles in conferring risk for AD. Examples of lifestyle factors influencing risk for CI are smoking and inactivity [17, 18]. Some factors however can decrease CI risk, such as education. Education has been proven to be a protective factor against dementia as having no education doubles the risk of developing dementia [19]. As such, investigations of the interplay between genetics and environment are pivotal for understanding AD etiology.
Epigenetic factors regulate gene function through chemical modification of DNA (most often via methylation) that does not alter the sequence [20]. DNA methylation occurs through the addition of a methyl group to the cytosine base of DNA [21]. This process is catalyzed by DNA methyltransferases, and typically occurs at CpG dinucleotides where cytosine and guanine bases are consecutively paired together [21]. Degree of CpG methylation at sites involved in gene regulation are modifiable [20] and potentially reversible [22] depending on environmental factors such as diet, exercise, and lifestyle [20]. Environmental factors can impact the rate of biological aging [23]. The human aging process can be quantified epigenetically through a collective number of CpG sites that have varying degrees of methylation such as hyper- (increased) or hypo- (decreased) methylation [23, 24]. Certain patterns of methylation, however, have been associated with age-related diseases such as AD [25, 26]. The rate of epigenetic aging can also differ across ethnic groups depending on burden of co-morbidities and lifestyle factors [27].
In this study we sought to identify differentially methylated positions of the genome that may be associated with CI (diagnosed as AD/MCI) in MAs and NHWs. We hypothesized there would be differentially methylated genomic sites or regions associated with CI in both groups (MAs and NHWs) but that methylation profiles would differ across ethnic groups. Differential DNA methylation may explain differences in racial and ethnic susceptibility for CI since environmental factors influencing methylation levels can vary from one ethnic group to another. Understanding how DNA methylation might impact age associated diseases/phenotypes such as AD and MCI among varying ethnic groups could inform future race- and ethnicity-specific risk assessments. Targeted risk assessments may also aid the development of more-efficacious interventions/therapeutics for deterring/delaying onset of AD in the future.
METHODS
Dataset and study design
Participants were selected from the Texas Alzheimer’s Research and Care Consortium (TARCC), a collaborative effort aimed at understanding the etiology, pathophysiology, treatment, and prevention of AD. Specifically, TARCC aims to bridge health disparities by incorporating minority populations in Texas such as the Hispanic community, in particular MAs, into research. Peripheral blood samples and accompanying demographic, clinical, and cognitive data from 600 individuals (300 NHWs and 300 MAs) were provided by TARCC. Participants were stratified into two groups based on cognitive status: those with CI (diagnosed as either AD or MCI) and normal controls. All participants were ≥50 years old and matched based on age and sex. Following removal of duplicates and participants with missing data, the final cohort (n = 551) consisted of 252 NHW and 299 MA participants (Table 1). This study was approved by the North Texas Regional Institutional Review Board # 1330309-1. Informed consent was obtained by TARCC from all participants.
Table 1
Demographic table of the TARCC cohort
TARCC Demographic Table | |||
Non-Hispanic Whites n = 252 | |||
NC | CI (AD/MCI) | p | |
n | 143 | 109 | |
Sex (F/M) | 72/71 | 51/58 | |
Age (y) | 71.1±7.4 | 72.1±4.5 | |
Education (y) | 16.0±2.6 | 14.9±2.7 | <0.01 |
MMSE | 29.2±1.0 | 23.9±5.1 | <0.01 |
CDR Sum | 0.0±0.1 | 3.8±3.2 | <0.01 |
Mexican Americans n = 299 | |||
NC | CI (AD/MCI) | p | |
n | 177 | 122 | |
Sex (F/M) | 101/76 | 65/57 | |
Age (y) | 67.6±5.6 | 70.7±7.8 | <0.01 |
Education (y) | 11.0±4.4 | 9.1±5.3 | <0.01 |
MMSE | 28.1±2.0 | 23.6±5.0 | <0.01 |
CDR Sum | 0.0±0.1 | 2.4±3.0 | <0.01 |
Standard two-sample t-tests assuming unequal variances were used to evaluate any significant difference between normal controls (NC) and participants with cognitive impairment (CI) for age (in years), education level (in years), Mini-Mental State Exam (MMSE), and Clinical Dementia Rating (CDR) sum.
Cognitive function diagnosis
Participants were determined cognitively normal or diagnosed with AD/MCI based on a consensus by a review committee consisting of healthcare professionals such as a neuropsychologist and clinician [28]. Each participant underwent a battery of neurocognitive tests (SF1) such as Clinical Dementia Rating (CDR) and Mini-Mental State Exam (MMSE) to determine cognitive status. An English or Spanish version of assessments was provided to participants depending on their native language in order to obtain a fair assessment of cognitive status. Individuals with other neurological diseases or conditions such as Parkinson’s disease or severe depression-related cognitive dysfunction, were excluded.
DNA extraction, genotyping, and methylation analysis
DNA from the buffy coat of peripheral blood was extracted using the MagBind ® Blood and Tissue DNA HDQ Kit (Omega Bio-tek, Norcross, GA) and a Microlab STAR liquid handling system. DNA extracts were quantified using the Qubit ® dsRNA BR Assay kit (Thermofisher, Waltham, MA). All blood samples were handled according to standard regulations and protocols, IBC/p/NP-2018-2. DNA samples (200 ng with a concentration of ≥10 ng/μl) were genotyped using the Infinium® HTS Global Screening Array v.2 (Illumina, San Diego, CA). Extracts below 10 ng/μL were concentrated using Microcon® DNA Fast Flow Filters (Sigma-Aldrich, Milwaukee, WI). The >10 ng/μL genotyping criterion was also applied for methylation typing to select suitable samples. DNA samples were bisulfite converted using the EZ DNA MethylationTM kit (Zymo Research, Irvine, CA) and subsequently processed on the Infinium ® MethylationEPIC BeadChip array (Illumina) which assesses the methylation status of over 850,000 CpG sites. Although spike ins were not included, control probes within the EPIC array were present to ensure efficient bisulfite conversion. According to the Illumina Infinium HD Assay Methylation Protocol Guide (Document # 15019519 v01), the Infinium I probe and Infinium II probes have bisulfite conversion controls, each that emit varying high signals depending on whether the bisulfite conversion was successful or not. Technical replicates per batch of EPIC BeadChips were run and all had an R2 value of ≥0.98. Signal intensity data (IDAT) files containing raw data obtained from the array [29] were uploaded and assessed in R studio. Beta values from these IDAT files representing levels of methylation detected by array probes at respective CpG sites (whereby 0 is an unmethylated site and 1 is a fully methylated site) were analyzed [30].
Methylation data and statistical analysis
Any differences between cognitively impaired participants and normal controls were assessed using the standard two-sample t-test assuming unequal variances. The analysis found significant differences (p-values<0.05) between cognitively impaired participants and normal controls for education, MMSE, and CDR sum among both the MA and NHW groups. The education level among cognitively impaired participants was significantly lower than normal controls in both MAs and NHWs. Education has been proven to be a protective factor against dementia [19] and might confer some protective effect against CI in normal controls here too. As expected, MMSE scores were significantly lower and CDR sum rating significantly higher, among cognitively impaired participants compared to normal controls in both MAs and NHWs. Age was deemed significantly different between cases and controls in the NHW group only. Beta values obtained from raw IDAT files were analyzed using the ChAMP Bioconductor package in R [31–34]. Data was normalized using the BMIQ method [35], batch effect correction was undertaken using the ComBat function [36, 37]. Covariates contributing to significant variation in results were visualized using singular value decomposition (SVD) plots within ChAMP [38].
Covariates that were adjusted for include age, sex, education level (in years), APOE ɛ4 allele status (present or not), participant recruitment site, PCA eigenvector values 1 & 2 and the minfi package generated white blood cell type proportions (CD8 T-cells, CD4 T-cells, natural killer cells, B-cells, monocytes, and neutrophils) [32]. The recruitment site was selected as a covariate since TARCC is a collaborative research effort with seven sites used to recruit participants. Genetic ancestry was accounted for by using PCA eigenvector values 1 & 2 derived from linkage disequilibrium pruned SNP data. These eigenvector values were generated using smartPCA for population stratification and thereby to verify self-reported ethnicity. The first two eigenvectors of the genetic relatedness matrix were used as covariates since they accounted for the most genetic variation in the dataset.
The covariates displaying the strongest significant association with CI in the SVD plots were chosen for further downstream analysis using the cate R package [39]. The cate R package was used to compare methylation at CpG sites for significance between the cognitively impaired and controls while controlling for potential unmeasured confounders and incorporating associated covariates using SVD plots. Covariates selected for adjustment using cate in MAs were sample group, age, sex, CD8 T-cells, CD4 T-cells, NK cells, B-cells, monocytes, neutrophils, EV1, EV2, and recruitment site (SF2c). In NHWs the covariates were sample group, sex, CD8 T-cells, CD4 T-cells, NK cells, B-cells, monocytes, neutrophils, EV2, and recruitment site (SF3c). Limma was used to convert beta values to M-values as M-values are better suited for statistical analysis of differential methylation from microarray based data [30, 40, 41]. The lambda value (genomic inflation factor) of p-values were calculated using QCEWAS [42]. False discovery rate (FDR) approach was used to adjust for multiple testing. An FDR-adjusted p-value <0.05 was used to determine significantly differentially methylated CpG sites between those cognitively impaired versus controls.
RESULTS
The cate package was used to adjust for confounders and qqman package was used to check for genomic inflation (SF4) in both MAs and NHWs [43]. Q-Q plots of p-values (Fig. 1) generated thereafter displayed little signs genomic inflation.
Fig. 1
Q-Q plots obtained from p-values after adjusting for confounders using cate. A) p-values from the Mexican American (MA) cohort. B) p-values from the non-Hispanic white (NHW) cohort. Most of the p-values observed for each of the CpG sites investigated fall within the expected range of methylation differences between cognitively impaired individuals and normal controls however the outlier data points at the end of the graph suggested those CpG sites are differentially methylated between these groups.
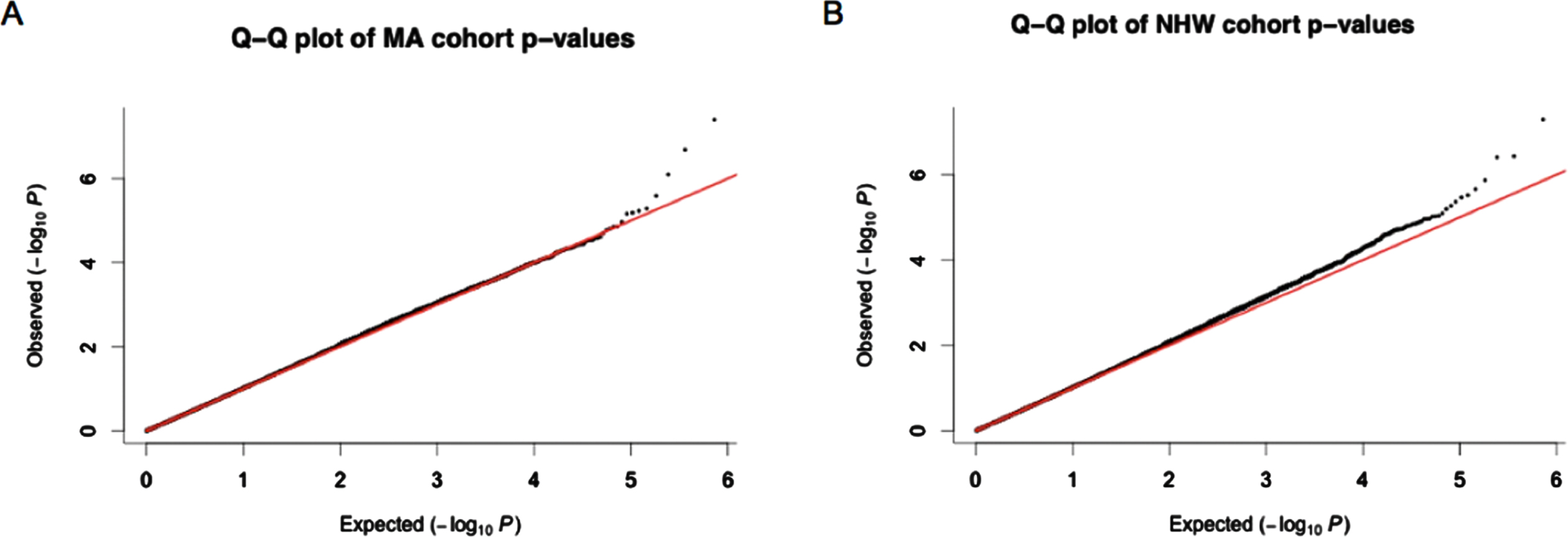
Two CpG sites in MAs (cg13135255 and cg01887506) and three in NHWs (cg27002303, cg10607142, and cg13529380) displayed differential methylation between cognitively impaired participants and normal controls (Table 2). Based on an FDR-adjusted p-value threshold of 0.05, two significant differentially methylated CpG sites were obtained: cg13135255 in MAs and cg27002303 in NHWs. Three CI-associated suggestive sites obtained, based on an FDR-adjusted p-value threshold of 0.1, were cg01887506 (MAs) and cg10607142 and cg13529380 (NHWs). Methylation in those cognitively impaired versus normal controls tend to be slightly elevated (hypermethylation) at all the CpG sites reported, except for cg13529380 in NHWs which shows a slight decrease (hypomethylation) in methylation instead (Fig. 2).
Fig. 2
Average beta values at significant and suggestive CpG sites in cognitively impaired and normal controls among (A) Mexican Americans and (B) non-Hispanic whites. The y-axis displays the beta values between 0 and 1 reflecting level of methylation at the CpG site, where 0 represents an unmethylated site and 1 is a fully methylated site [30].
![Average beta values at significant and suggestive CpG sites in cognitively impaired and normal controls among (A) Mexican Americans and (B) non-Hispanic whites. The y-axis displays the beta values between 0 and 1 reflecting level of methylation at the CpG site, where 0 represents an unmethylated site and 1 is a fully methylated site [30].](https://content.iospress.com:443/media/jad/2023/92-4/jad-92-4-jad221031/jad-92-jad221031-g002.jpg)
Table 2
Differentially methylated CpG sites obtained among Mexican Americans and non-Hispanic whites from the TARCC cohort with the associated gene and FDR adjusted p-values
Differentially methylated CpG sites associated with cognitive impairment in a TARCC cohort | ||||||
Mexican Americans | Non-Hispanic whites | |||||
CpG site | Gene/Loci | p | CpG site | Gene/Loci | p | |
Significant CpG sites (FDR p < 0.05) | cg13135255 | CREBBP | 0.029 | cg27002303 | LOC100188947 | 0.037 |
Suggestive CpG sites (FDR p < 0.1) | cg01887506 | EPHA4 | 0.074 | cg10607142 | ADAMTS14 | 0.095 |
cg13529380 | –/– | 0.095 |
DISCUSSION
Despite well-established pathological features of AD, there are number of remaining questions and challenges related to understanding AD pathogenesis. It remains relatively unknown how late onset AD pathology begins and diagnosis of AD is often not confirmed until an autopsy [44]. AD related changes in the brain are detectable prior to diagnosis, despite a lack of functional or psychiatric change [1, 45, 46]. Identifying molecular factors associated with AD, within easily accessible tissue such as peripheral blood, could help identify individuals at risk for AD early. Methylation-based studies can provide necessary insight into these issues since AD risk is also influenced by environmental factors.
In this study, five differentially methylated sites in peripheral blood were ascertained to be associated with CI, either significantly (FDR p < 0.05) or suggestively (FDR p < 0.1). These were CpG sites cg13135255 (FDR-adjusted p = 0.029) and cg01887506 (FDR-adjusted p = 0.074) in MAs, as well as cg27002303 (FDR-adjusted p = 0.037), cg10607142 (FDR-adjusted p = 0.095) and cg13529380 (FDR-adjusted p = 0.095) in NHWs. Altogether, three CpG sites (cg01887506, cg10607142, and cg13529380) were identified as suggestive based on an FDR p-value threshold of 0.1.
Notably, out of the five CI-associated CpG sites, two hits fell below the traditional FDR threshold of 0.05: cg13135255 in MAs (FDR-adjusted p = 0.029) and cg27002303 (FDR-adjusted p = 0.037) in NHWs. The strongest association was observed for the CpG site cg13135255 (FDR-adjusted p = 0.029) in MAs, which is situated within the intron of the CREB binding protein (CREBBP) gene. CREBBP is a histone acetyltransferase that regulates acetylation impacting downstream gene transcription (Fig. 3) [47]. It has an important role in forming memory and mutations in this gene can cause cognitive dysfunction (e.g., Rubinstein-Taybi syndrome) [47]. Regulation of CREBBP through an upstream non-coding RNA and a mi-RNA causes hyperacetylation at CREB1, GATA2, NFKB1 and FOXA1 genes; all of which have been implicated in AD associated with exposure to toxic metals [48]. A genetic variant in this gene has also been associated with episodic memory loss in healthy aging individuals [49]. The cg13135255 site has also been previously associated with post-traumatic stress disorder severity following social adversity and stress in a predominantly female African American cohort [50]. CREBBP plays an indirect role in some proinflammatory pathways through co-activation of transcription factors such as CREB and NF-kappaB. AD associated protein biomarkers are predominantly non-inflammation based in MAs and there are no studies yet proving direct association between CREBBP and CI-based inflammation. Therefore, it is unsurprising that this hit was significant in MAs and not in NHWs within this TARCC cohort [5]. Hypermethylation within CREBBP at a global level in peripheral blood is associated with CI in MAs, in this study.
Fig. 3
Loci of differentially methylated significant or suggestive CpG sites among Mexican American and non-Hispanic white TARCC participants (site of gene/loci derived from UCSC genome browser [47]).
![Loci of differentially methylated significant or suggestive CpG sites among Mexican American and non-Hispanic white TARCC participants (site of gene/loci derived from UCSC genome browser [47]).](https://content.iospress.com:443/media/jad/2023/92-4/jad-92-4-jad221031/jad-92-jad221031-g003.jpg)
The cg27002303 site (NHWs) is located within the LOC100188947 loci, which is a non-coding RNA (Fig. 3) [47]. A SNP variant within this loci has been associated with increased ADHD risk among children in a family-based study set in Montreal [52]. This CpG site has been reported in Han et al. (2021) which examined fetal malnutrition and its impact on pineal development, by observing patterns of melatonin production and assessing DNA methylation levels in a Chinese cohort. Network analysis found this CpG site involved in processes such as nervous system development and synapse assembly among others [53]. Further information regarding its function or role in influencing gene expression is yet to be elucidated. Both highly significant sites (cg13135255 and cg27002303) among MAs and NHWs respectively have been associated with either cognitive function or development, therefore environmental factors influencing methylation at these sites could possibly contribute to AD pathogenesis collectively alongside other AD risk associated CpG sites.
In addition to the aforementioned significant CpG sites with FDR-adjusted p < 0.05, the other suggestive hits (FDR-adjusted p < 0.1) associated with CI were CpG sites cg01887506 (MAs), cg10607142 (NHWs), and cg13529380 (NHWs). The site cg01887506 is situated within the EPHA4 gene which is most expressed in the brain hippocampus and plays an important role in the formation of the dendritic spines within the hippocampus (Fig. 3) [47, 54]. EPHA4 was found to regulate amyloid-β production [55]. A decrease in EPHA4 expression has been associated with an increase in BACE1 expression resulting in increased levels of amyloid-β [55]. One study in a cohort from the Netherlands found protein levels of the gene in AD and control participants to be the same, however distribution of the protein in the hippocampus differed among cases and controls whereby it was found alongside plaques in AD patients [54]. Of the five CpG sites reported in this study, cg01887506 is the only CpG site that is already associated with AD pathogenesis. Hypermethylation at EPHA4 globally in peripheral blood might either reflect methylation changes in the hippocampus as it’s highly expressed there or possibly indirectly influence methylation in the hippocampus via the blood-brain barrier.
The site cg10607142 (NHWs) is within the ADAMTS14 gene (Fig. 3) [47]. The A Disintegrin and Metalloproteinase with Thrombospondin Motifs (ADAMTS) genes play important roles in processes such as angiogenesis and inflammation and has been associated with AD [56]. The ADAMTS14 gene, however, is normally involved in producing collagen and a polymorphism within is associated with osteoarthritis [57]. The ADAMTS14 gene associated with this CpG site has the least established association with CI out of the genes reported in this study.
Whether the five reported CpG sites confer early CI risk or are modulated as a result of CI presence is yet to be determined. A longitudinal study may be best suited to identify whether methylation patterns at these CpG sites are a cause or consequence of CI. The results of this study demonstrate, however, that the methylation landscape associated with cognitive impairment is vastly different between MAs and NHWs. Further comparative analysis between cognitively impaired NHWs and MAs displayed hyperinflation on Q-Q plots even after adjusting for confounders using cate, which can be explained due to population stratification [58] since the groups compared differ genetically (SF5). Similar hyperinflation was observed comparing normal control NHWs and MAs (SF5).
Methylation associated with AD has previously been established in various studies, such as with the APOE region and AD [59, 60] and a collectively higher risk from 71 methylation sites in the genome than already established single allele risk variants [25, 61]. Many studies report a mixture of either hypermethylated or hypomethylated regions or sites that are associated with CI and can vary depending on the ethnic population in focus. DNA methylation levels can be specific to certain populations such as increased global methylation in peripheral blood from AD Caucasians with poor cognitive performance [26] and altered methylation at 48 CpG sites within the APOE region associated with aging and age-related cognitive decline in a healthy African American population [61]. In Caucasian based population studies, many of the CI associated alteration of methylation are at genes involved in inflammatory pathways [25, 62], like the Religious Order Study or the Memory and Aging Project study that found AD associated methylation in brain tissue within 5 genes involved in lipid metabolism, tau tangles and the inflammatory pathway (ABCA7, BIN1, HLA-DRB5, SLC24A4, and SORL1) [63]. Japanese population-based studies have also found similar associations between cognitive impairment and methylation levels in genes within inflammatory pathways, such as reduced TREM1 methylation at three CpG islands in peripheral leukocytes of AD patients [64].
In other ethnic populations, differentially methylated genes have been reported in pathways that are not associated with inflammation in patients with cognitive decline. In Chinese populations, heavily methylated CpG islands in the KLOTH gene promotor was associated with MCI [65] and heavy methylation of BDNF which has neuroprotective functions was associated with AD [66]. A study evaluating methylation levels at 48 CpG sites within the APOE region in peripheral blood from African Americans found lack of methylation in 8 CpG islands across the APOE, PVRL2, and TOMM40 genes to be significantly associated with delayed recall cognitive function [61]. Studies in Mexican Americans also show association between cognitive decline and non-inflammatory pathways that lean more towards metabolic processes [5, 67, 68].
A previous study conducted by our group discovered 10 CpG sites and 4 regions differentially methylated associating with MCI in 90 MA participants from the Health and Aging Brain among Latino Elders (HABLE) cohort [69]. Notably, some of the previously identified genes associated with CI (SEPT9, CCNY, and KLHL29) were replicated here (based on unadjusted nominal p < 0.005). However, the individual CpG sites vary between studies. The CpG sites were cg09029294 in SEPT9 (NHWs), cg16922732 and cg16015468 in SEPT9 (MAs), cg02524725, cg11958675, and cg20772106 in CCNY (MAs), and finally cg10755016 in KLHL29 (MAs) (Table 3).
Table 3
Significant CpG sites (nominal p-value <0.005) at replicated genes from previous study [69]
List of replicated genes from HABLE study within the TARCC cohort | ||
Mexican Americans | ||
Gene | CpG site | Nominal p |
CCNY | cg02524725 | 0.001 |
cg11958675 | 0.005 | |
cg20772106 | 0.003 | |
KLHL29 | cg10755016 | 0.003 |
SEPT9 | cg16015468 | 0.001 |
cg16922732 | 0.005 | |
Non-Hispanic Whites | ||
Gene | CpG site | Nominal p-value |
SEPT9 | cg09029294 | 0.005 |
Though informative, this study did have some limitations. The focus of this study was to identify differential methylation associated with CI; however, next steps could involve adjusting for type 2 diabetes comorbidity as its presence is known to increase the risk of developing AD [1, 70]. Methylation studies based on peripheral blood provide insight into global methylation levels that might influence CI risk; however, methylation signatures in peripheral blood may not accurately reflect epigenetic changes in brain tissue. Despite this, there are a plethora of studies showing correlation between methylation changes in the blood and cognitive changes in the brain as discussed earlier. Given this correlation, peripheral epigenetic markers such as DNA methylation may be useful for predicting ethnicity-specific risk for AD. Early signs of cognitive dysfunction can be indistinguishable between MCI and AD patients in the general population, and often MCI patients develop AD later in life [1, 71]. A biomarker that indicates risk at preclinical stage for progression to AD (e.g., MCI) would have great utility since there is still no cure or method of slowing neuronal degeneration [1]. Methylation based biomarkers could potentially be more useful since methylation can be reversed depending on lifestyle choices and changes.
ACKNOWLEDGMENTS
The research team thanks the Texas Alzheimer’s Research and Care Consortium team of investigators.
FUNDING
This research project was supported (in part) by funding provided to the Texas Alzheimer’s Research and Care Consortium by the Darrell K Royal Texas Alzheimer’s Initiative, directed by the Texas Council on Alzheimer’s Disease and Related Disorders.
This work was also supported by the Office of Vice President for Research and Innovation, the Institute for Healthy Aging, and National Institutes of Health/National Institute on Aging (T32 AG020494) (2018 and 2020) including half predoctoral international fellowship through the Neurobiology of Aging and Alzheimer’s Disease (NBAAD) Training Program 2021-2022 and R01AG070862 to RCB.
CONFLICT OF INTEREST
The authors have no conflict of interest to report.
DATA AVAILABILITY
The data supporting the findings of this study are available on request from the corresponding author. The data are not publicly available due to privacy or ethical restrictions.
SUPPLEMENTARY MATERIAL
[1] The supplementary material is available in the electronic version of this article: https://dx.doi.org/10.3233/JAD-221031.
REFERENCES
[1] | ((2022) ) 2022 Alzheimer’s disease facts and figures. Alzheimers Dement 18: , 700–789. |
[2] | Vega IE , Cabrera LY , Wygant CM , Velez-Ortiz D , Counts SE ((2017) ) Alzheimer’s disease in the Latino community: Intersection of genetics and social determinants of health. J Alzheimers Dis 58: , 979–992. |
[3] | Novak K , Riggs JA ((2004) ) Hispanics/Latinos and 562 Alzheimer’s disease, Alzheimer’s Association. |
[4] | O’Bryant SE , Johnson L , Balldin V , Edwards M , Barber R , Williams B , Devous M , Cushings B , Knebl J , Hall J ((2013) ) Characterization of Mexican Americans with mild cognitive impairment and Alzheimer’s disease. J Alzheimers Dis 33: , 373–379. |
[5] | O’Bryant SE , Xiao G , Edwards M , Devous M , Gupta VB , Martins R , Zhang F , Barber R , Texas Alzheimer’s Research and Care Consortium (TARCC) ((2013) ) Biomarkers of Alzheimer’s disease among Mexican Americans. J Alzheimers Dis 34: , 841–849. |
[6] | O’Bryant SE , Humphreys JD , Schiffer RB , Sutker PB ((2007) ) Presentation of Mexican Americans to a memory disorder clinic. J Psychopathol Behav Assess 29: , 137–140. |
[7] | Santos OA , Pedraza O , Lucas JA , Duara R , Greig-Custo MT , Hanna Al-Shaikh FS , Liesinger AM , Bieniek KF , Hinkle KM , Lesser ER , Crook JE , Carrasquillo MM , Ross OA , Ertekin-Taner N , Graff-Radford NR , Dickson DW , Murray ME ((2019) ) Ethnoracial differences in Alzheimer’s disease from the FLorida Autopsied Multi-Ethnic (FLAME) cohort. Alzheimers Dement 15: , 635–643. |
[8] | Arias E ((2010) ) United States life tables by Hispanic origin, DIANE Publishing. |
[9] | Verghese PB , Castellano JM , Holtzman DM ((2011) ) Apolipoprotein E in Alzheimer’s disease and other neurological disorders. Lancet Neurol 10: , 241–252. |
[10] | Tanzi RE ((2012) ) The genetics of Alzheimer disease. Cold Spring Harbor Persp Med 2: , a006296. |
[11] | Shao Y , Shaw M , Todd K , Khrestian M , D’Aleo G , Barnard PJ , Zahratka J , Pillai J , Yu C-E , Keene CD , Leverenz JB , Bekris LM ((2018) ) DNA methylation of TOMM40-APOE-APOC2 in Alzheimer’s disease. J Hum Genet 63: , 459–471. |
[12] | Gatz M , Reynolds CA , Fratiglioni L , Johansson B , Mortimer JA , Berg S , Fiske A , Pedersen NL ((2006) ) Role of genes and environments for explaining Alzheimer disease. Arch Gen Psychiatry 63: , 168–174. |
[13] | Ridge PG , Mukherjee S , Crane PK , Kauwe JS , Alzheimer’s Disease Genetics Consortium ((2013) ) Alzheimer’s disease: Analyzing the missing heritability. PloS One 8: , e79771. |
[14] | Kunkle BW , Grenier-Boley B , Sims R , Bis JC , Damotte V , Naj AC , Boland A , Vronskaya M , Van Der Lee SJ , Amlie-Wolf A , et al. ((2019) ) Genetic meta-analysis of diagnosed Alzheimer’s disease identifies new risk loci and implicates Aβ, tau, immunity and lipid processing. Nat Genet 51: , 414–430. |
[15] | Saunders AM , Strittmatter WJ , Schmechel D , George-Hyslop PS , Pericak-Vance MA , Joo S , Rosi B , Gusella J , Crapper-MacLachlan D , Alberts M , et al. ((1993) ) Association of apolipoprotein E allele ɛ4 with late-onset familial and sporadic Alzheimer’s disease. Neurology 43: , 1467–1467. |
[16] | Bertram L , Lill CM , Tanzi RE ((2010) ) The genetics of Alzheimer disease: Back to the future. Neuron 68: , 270–281. |
[17] | Fiorito G , Polidoro S , Dugué PA , Kivimaki M , Ponzi E , Matullo G , Guarrera S , Assumma MB , Georgiadis P , Kyrtopoulos SA , Krogh V , Palli D , Panico S , Sacerdote C , Tumino R , Chadeau-Hyam M , Stringhini S , Severi G , Hodge AM , Giles GG , Marioni R , Karlsson Linnér R , O’Halloran AM , Kenny RA , Layte R , Baglietto L , Robinson O , McCrory C , Milne RL , Vineis P ((2017) ) Social adversity and epigenetic aging: A multi-cohort study on socioeconomic differences in peripheral blood DNA methylation. Sci Rep 7: , 16266. |
[18] | Corley J , Cox SR , Harris SE , Hernandez MV , Maniega SM , Bastin ME , Wardlaw JM , Starr JM , Marioni RE , Deary IJ ((2019) ) Epigenetic signatures of smoking associate with cognitive function, brain structure, and mental and physical health outcomes in the Lothian Birth Cohort 1936. Transl Psychiatry 9: , 248. |
[19] | Katzman R ((1993) ) Education and the prevalence of dementia and Alzheimer’s disease. Neurology 43: , 13. |
[20] | Qazi TJ , Quan Z , Mir A , Qing H ((2018) ) Epigenetics in Alzheimer’s disease: Perspective of DNA methylation. Mol Neurobiol 55: , 1026–1044. |
[21] | Moore LD , Le T , Fan G ((2013) ) DNA methylation and its basic function. Neuropsychopharmacology 38: , 23–38. |
[22] | Jeltsch A , Jurkowska RZ ((2014) ) New concepts in DNA methylation. Trends Biochem Sci 39: , 310–318. |
[23] | Hannum G , Guinney J , Zhao L , Zhang L , Hughes G , Sadda S , Klotzle B , Bibikova M , Fan J-B , Gao Y , Deconde R , Chen M , Rajapakse I , Friend S , Ideker T , Zhang K ((2013) ) Genome-wide methylation profiles reveal quantitative views of human aging rates. Mol Cell 49: , 359–367. |
[24] | Horvath S ((2013) ) DNA methylation age of human tissues and cell types. Genome Biol 14: , 3156. |
[25] | De Jager PL , Srivastava G , Lunnon K , Burgess J , Schalkwyk LC , Yu L , Eaton ML , Keenan BT , Ernst J , McCabe C , Tang A , Raj T , Replogle J , Brodeur W , Gabriel S , Chai HS , Younkin C , Younkin SG , Zou F , Szyf M , Epstein CB , Schneider JA , Bernstein BE , Meissner A , Ertekin-Taner N , Chibnik LB , Kellis M , Mill J , Bennett DA ((2014) ) Alzheimer’s disease: Early alterations in brain DNA methylation at ANK1, BIN1, RHBDF2 and other loci. Nat Neurosci 17: , 1156–1163. |
[26] | Di Francesco A , Arosio B , Falconi A , Micioni Di Bonaventura MV , Karimi M , Mari D , Casati M , Maccarrone M , D’Addario C ((2015) ) Global changes in DNA methylation in Alzheimer’s disease peripheral blood mononuclear cells. Brain Behav Immun 45: , 139–144. |
[27] | Levine ME , Lu AT , Quach A , Chen BH , Assimes TL , Bandinelli S , Hou L , Baccarelli AA , Stewart JD , Li Y ((2018) ) An epigenetic biomarker of aging for lifespan and healthspan. Aging (Albany NY) 10: , 573. |
[28] | O’Bryant SE , Waring SC , Cullum CM , Hall J , Lacritz L , Massman PJ , Lupo PJ , Reisch JS , Doody R , Texas Alzheimer’s Research Consortium ((2008) ) Staging dementia using Clinical Dementia Rating Scale Sum of Boxes scores: A Texas Alzheimer’s Research Consortium study. Arch Neurol 65: , 1091–1095. |
[29] | Assenov Y , Müller F , Lutsik P , Walter J , Lengauer T , Bock C ((2014) ) Comprehensive analysis of DNA methylation data with RnBeads. Nat Methods 11: , 1138–1140. |
[30] | Du P , Zhang X , Huang C-C , Jafari N , Kibbe WA , Hou L , Lin SM ((2010) ) Comparison of Beta-value and M-value methods for quantifying methylation levels by microarray analysis. BMC Bioinformatics 11: , 587. |
[31] | Morris TJ , Butcher LM , Feber A , Teschendorff AE , Chakravarthy AR , Wojdacz TK , Beck S ((2014) ) ChAMP: 450k chip analysis methylation pipeline. Bioinformatics 30: , 428–430. |
[32] | Aryee MJ , Jaffe AE , Corrada-Bravo H , Ladd-Acosta C , Feinberg AP , Hansen KD , Irizarry RA ((2014) ) Minfi: A flexible and comprehensive Bioconductor package for the analysis of Infinium DNA methylation microarrays. Bioinformatics 30: , 1363–1369. |
[33] | Fortin J-P , Triche TJ Jr , Hansen KD ((2017) ) Preprocessing, normalization and integration of the Illumina HumanMethylationEPIC array with minfi. Bioinformatics 33: , 558–560. |
[34] | Zhou W , Laird PW , Shen H ((2017) ) Comprehensive characterization, annotation and innovative use of Infinium DNA methylation BeadChip probes. Nucleic Acids Res 45: , e22–e22. |
[35] | Teschendorff AE , Marabita F , Lechner M , Bartlett T , Tegner J , Gomez-Cabrero D , Beck S ((2013) ) A beta-mixture quantile normalization method for correcting probe design bias in Illumina Infinium 450 k DNA methylation data. Bioinformatics 29: , 189–196. |
[36] | Johnson WE , Li C , Rabinovic A ((2007) ) Adjusting batch effects in microarray expression data using empirical Bayes methods. Biostatistics 8: , 118–127. |
[37] | Leek J , Johnson W , Parker H , Fertig E , Jaffe A , Storey J , Zhang Y , Torres L ((2017) ) sva: Surrogate Variable Analysis. R package version 3.24.4. |
[38] | Teschendorff AE , Menon U , Gentry-Maharaj A , Ramus SJ , Gayther SA , Apostolidou S , Jones A , Lechner M , Beck S , Jacobs IJ ((2009) ) An epigenetic signature in peripheral blood predicts active ovarian cancer. PloS One 4: , e8274. |
[39] | Wang J , Zhao Q ((2015) ) Comprehensive R Archive Network (CRAN). |
[40] | Wettenhall JM , Smyth GK ((2004) ) limmaGUI: A graphical user interface for linear modeling of microarray data. Bioinformatics 20: , 3705–3706. |
[41] | Smyth GK ((2004) ) Linear models and empirical bayes methods for assessing differential expression in microarray experiments. Stat Appl Genet Mol Biol 3: , Article3. |
[42] | Van der Most PJ , Küpers LK , Snieder H , Nolte I ((2017) ) QCEWAS: Automated quality control of results of epigenome-wide association studies. Bioinformatics 33: , 1243–1245. |
[43] | Turner SD ((2014) ) qqman: An R package for visualizing GWAS results using QQ and manhattan plots. Biorxiv 005165. |
[44] | Gauthreaux K , Bonnett TA , Besser LM , Brenowitz WD , Teylan M , Mock C , Chen YC , Chan KCG , Keene CD , Zhou XH , Kukull WA ((2020) ) Concordance of clinical Alzheimer diagnosis and neuropathological features at autopsy. J Neuropathol Exp Neurol 79: , 465–473. |
[45] | Watson GS , Craft S ((2004) ) Modulation of memory by insulin and glucose: Neuropsychological observations in Alzheimer’s disease. Eur J Pharmacol 490: , 97–113. |
[46] | Henstridge CM , Hyman BT , Spires-Jones TL ((2019) ) Beyond the neuron– cellular interactions early in Alzheimer disease pathogenesis. Nat Rev Neurosci 20: , 94–108. |
[47] | Kent WJ , Sugnet CW , Furey TS , Roskin KM , Pringle TH , Zahler AM , Haussler D ((2002) ) The human genome browser at UCSC. Genome Res 12: , 996–1006. |
[48] | Gupta R , Kumar P ((2022) ) Integrative analysis of OIP5-AS1/miR-129-5p/CREBBP axis as a potential therapeutic candidate in the pathogenesis of metal toxicity-induced Alzheimer’s disease. Gene Rep 26: , 101442. |
[49] | Barral S , Reitz C , Small SA , Mayeux R ((2014) ) Genetic variants in a ‘cAMP element binding protein’(CREB)-dependent histone acetylation pathway influence memory performance in cognitively healthy elderly individuals. Neurobiol Aging 35: , 2881. e2887-2881.e2810 |
[50] | Wani AH , Aiello AE , Kim GS , Xue F , Martin CL , Ratanatharathorn A , Qu A , Koenen K , Galea S , Wildman DE , Uddin M ((2021) ) The impact of psychopathology, social adversity and stress-relevant DNA methylation on prospective risk for post-traumatic stress: A machine learning approach. J Affect Disord 282: , 894–905. |
[51] | Barnes P , Adcock I , Ito K ((2005) ) Histone acetylation and deacetylation: Importance in inflammatory lung diseases. Eur Respir J 25: , 552–563. |
[52] | Thakur GA , Sengupta SM , Grizenko N , Choudhry Z , Joober R ((2012) ) Family-based association study of ADHD and genes increasing the risk for smoking behaviours. Arch Dis Child 97: , 1027–1033. |
[53] | Han T , Jiang W , Wu H , Wei W , Lu J , Lu H , Xu J , Gu W , Guo X , Wang Y , Ruan J , Li Y , Wang Y , Jiang X , Zhao S , Li Y , Sun C ((2021) ) Fetal malnutrition is associated with impairment of endogenous melatonin synthesis in pineal via hypermethylation of promoters of protein kinase C alpha and cAMP response element-binding. J Pineal Res 71: , e12764. |
[54] | Rosenberger AF , Rozemuller AJ , van der Flier WM , Scheltens P , van der Vies SM , Hoozemans JJ ((2014) ) Altered distribution of the EphA4 kinase in hippocampal brain tissue of patients with Alzheimer’s disease correlates with pathology. Acta Neuropathol Commun 2: , 79. |
[55] | Tamura K , Chiu YW , Shiohara A , Hori Y , Tomita T ((2020) ) EphA4 regulates Aβ production via BACE1 expression in neurons. FASEB J 34: , 16383–16396. |
[56] | Gurses MS , Ural MN , Gulec MA , Akyol O , Akyol S ((2016) ) Pathophysiological function of ADAMTS enzymes on molecular mechanism of Alzheimer’s disease. Aging Dis 7: , 479. |
[57] | Rodriguez-Lopez J , Pombo-Suarez M , Loughlin J , Tsezou A , Blanco FJ , Meulenbelt I , Slagboom PE , Valdes AM , Spector TD , Gomez-Reino JJ , Gonzalez A ((2009) ) Association of a nsSNP in ADAMTS14 to some osteoarthritis phenotypes. Osteoarthritis Cartilage 17: , 321–327. |
[58] | Alghamdi J , Padmanabhan S ((2014) ) Fundamentals of complex trait genetics and association studies. In Handbook of Pharmacogenomics and Stratified Medicine, Padmanabhan S, ed. Academic Press, San Diego, pp. 235–257. |
[59] | Foraker J , Millard SP , Leong L , Thomson Z , Chen S , Keene CD , Bekris LM , Yu C-E ((2015) ) The APOE gene is differentially methylated in Alzheimer’s disease. J Alzheimers Dis 48: , 745–755. |
[60] | Marioni RE , Shah S , McRae AF , Chen BH , Colicino E , Harris SE , Gibson J , Henders AK , Redmond P , Cox SR , Pattie A , Corley J , Murphy L , Martin NG , Montgomery GW , Feinberg AP , Fallin MD , Multhaup ML , Jaffe AE , Joehanes R , Schwartz J , Just AC , Lunetta KL , Murabito JM , Starr JM , Horvath S , Baccarelli AA , Levy D , Visscher PM , Wray NR , Deary IJ ((2015) ) DNA methylation age of blood predicts all-cause mortality in later life. Genome Biol 16: , 25. |
[61] | Liu J , Zhao W , Ware EB , Turner ST , Mosley TH , Smith JA ((2018) ) DNA methylation in the APOE genomic region is associated with cognitive function in African Americans. BMC Med Genomics 11: , 43. |
[62] | Cho SH , Chen JA , Sayed F , Ward ME , Gao F , Nguyen TA , Krabbe G , Sohn PD , Lo I , Minami S , Devidze N , Zhou Y , Coppola G , Gan L ((2015) ) SIRT1 deficiency in microglia contributes to cognitive decline in aging and neurodegeneration via epigenetic regulation of IL-1β . J Neurosci 35: , 807–818. |
[63] | Yu L , Chibnik LB , Srivastava GP , Pochet N , Yang J , Xu J , Kozubek J , Obholzer N , Leurgans SE , Schneider JA ((2015) ) Association of brain DNA methylation in SORL1, ABCA7, HLA-DRB5, SLC24A4, and BIN1 with pathological diagnosis of Alzheimer disease. JAMA Neurol 72: , 15–24. |
[64] | Ozaki Y , Yoshino Y , Yamazaki K , Sao T , Mori Y , Ochi S , Yoshida T , Mori T , Iga J-I , Ueno S-I ((2017) ) DNA methylation changes at TREM2 intron 1 and TREM2 mRNA expression in patients with Alzheimer’s disease. J Psychiatr Res 92: , 74–80. |
[65] | Luo M , Zhou X , Ji H , Ma W , Liu G , Dai D , Li J , Chang L , Xu L , Jiang L , Duan S , Wang Q ((2015) ) Population difference in the associations of KLOTH promoter methylation with mild cognitive impairment in Xinjiang Uygur and Han populations. PloS One 10: , e0132156. |
[66] | Chang L , Wang Y , Ji H , Dai D , Xu X , Jiang D , Hong Q , Ye H , Zhang X , Zhou X , Liu Y , Li J , Chen Z , Li Y , Zhou D , Zhuo R , Zhang Y , Yin H , Mao C , Duan S , Wang Q ((2014) ) Elevation of peripheral BDNF promoter methylation links to the risk of Alzheimer’s disease. PloS One 9: , e110773. |
[67] | O’Bryant SE , Xiao G , Barber R , Reisch J , Doody R , Fairchild T , Adams P , Waring S , Diaz-Arrastia R; Texas Alzheimer’s Research Consortium ((2010) ) A serum protein-based algorithm for the detection of Alzheimer disease. Arch Neurol 67: , 1077–1081. |
[68] | O’Bryant SE , Xiao G , Zhang F , Edwards M , German DC , Yin X , Como T , Reisch J , Huebinger RM , Graff-Radford N , Dickson D , Barber R , Hall J , O’Suilleabhain P , Grammas P ((2014) ) Validation of a serum screen for Alzheimer’s disease across assay platforms, species, and tissues. J Alzheimers Dis 42: , 1325–1335. |
[69] | Pathak GA , Silzer TK , Sun J , Zhou Z , Daniel AA , Johnson L , O’Bryant S , Phillips NR , Barber RC ((2019) ) Genome-wide methylation of mild cognitive impairment in Mexican Americans highlights genes involved in synaptic transport, Alzheimer’s disease-precursor phenotypes, and metabolic morbidities. J Alzheimers Dis 72: , 733–749. |
[70] | Ott A , Stolk R , Van Harskamp F , Pols H , Hofman A , Breteler M ((1999) ) Diabetes mellitus and the risk of dementia: The Rotterdam Study. Neurology 53: , 1937–1942. |
[71] | Malek-Ahmadi M ((2016) ) Reversion from mild cognitive impairment to normal cognition. Alzheimer Dis Assoc Disord 30: , 324–330. |