From Association to Intervention: The Alzheimer’s Disease-Associated Processes and Targets (ADAPT) Ontology
Abstract
Background:
Many putative causes and risk factors have been associated with outcomes in Alzheimer’s disease (AD) but all attempts at disease-modifying treatment have failed to be clinically significant. Efforts to address this “association—intervention” mismatch have tended to focus on the novel design of interventions.
Objective:
Here, we instead deal with the notion of association in depth. We introduce the concept of disease-associated process (DAP) as a flexible concept that can unite different areas of study of AD from genetics to epidemiology to identify disease-modifying targets.
Methods:
We sort DAPs using three properties: specificity for AD, frequency in patients, and pathogenic intensity for dementia before using a literature review to apply these properties in three ways. Firstly, we describe and visualize known DAPs. Secondly, we exemplify qualitative specificity analysis with the DAPs of tau protein pathology and autophagy to reveal their differential implication in AD. Finally, we use DAP properties to define the terms “risk factor,” “cause,” and “biomarker.”
Results:
We show how DAPs fit into our collaborative disease ontology, the Alzheimer’s Disease-Associated Processes and Targets (ADAPT) ontology. We argue that our theoretical system can serve as a democratic research forum, offering a more biologically adequate view of dementia than reductionist models.
Conclusion:
The ADAPT ontology is a tool that could help to ground debates around priority setting using objective criteria for the identifying of targets in AD. Further efforts are needed to address issues of how biomedical research into AD is prioritized and funded.
INTRODUCTION
Prognosis has not changed in the 115 years since Alois Alzheimer first described Alzheimer’s disease (AD) in 1906 as a clinical amnestic and behavioral syndrome with hallmark neuropathological features (now known as “amyloid-β” and “tau” proteins).
Since that time, there has been a long-standing historical debate about whether the proteins used to define AD also cause cognitive decline. Amyloid and tau removal have been the dominant therapeutic strategy against AD since the 2000 s, but there is still no fully-validated treatment, despite the FDA’s recent and controversial accelerated approval of the amyloid-removing aducanumab and the recent publication of “moderately less decline on measures of cognition” in patients treated with lecanemab versus placebo [1]. Beyond the targeting of AD neuropathology, the other major biomedical approach to dementia is risk reduction through action against lifestyle risk factors including inadequate education and poor physical, mental, and social health across the lifetime [2].
Nevertheless, amyloid removal has been the dominant therapeutic since the 2000 s when the first antibodies were tested for use in humans [3]. It is based on the amyloid cascade hypothesis (ACH), which posits that the brain amyloid-β used to define the disease also plays a major causative role [4]. Given the number of failed anti-amyloid trials for AD, we argue that there is an ethical imperative to move beyond the dominance of the amyloid-lowering therapeutic strategy within a high-risk/high-reward economic model that leaves a therapeutic void with disastrous consequences for patients [5].
Herrup [6] underlines the limits of the anti-amyloid and reductionist approaches towards AD. Arguing that amyloid-β deposition is not the sole disease-associated process (DAP, our term which we define herein) for AD, Herrup proposes a multi-factorial approach which brings together all the known DAPs. He uses preclinical and clinical data to attempt to demonstrate “that a simple linear pathway tracing disease progression from amyloid-β to AD is inadequate as a formal hypothesis” and instead offers “a number of alternative ways of viewing the disease”. His alternative to the ACH is to embrace “the list of disease-causing options... [recognising no formal] guidance as to how to focus our quest to understand and treat AD” [6]. He summarizes: “AD can be viewed as a disease of amyloid . . . [and] “a long list of disease-causing options . . . The answer to the question of which option shall we choose is. . .choose them all” [6].
We aim to continue Herrup’s important initiative at a time when no single DAP fully explains the totality of AD-related dementia, inviting a pluralistic approach. However, his theoretical and therapeutic scheme lacks hierarchization, for his “choose them all” strategy is a political call to action that does not offer priority to any DAP. Furthermore, his analysis concerns only the molecular level (from genes to cellular pathways), thus overlooking a whole portion of the literature, such as population epidemiology, and is also challenging to apply to clinical research.
In this article, in order to build on Herrup, we propose a systemic approach that offers a holistic theoretical framework of AD by adding new property-based dimensions to represent DAPs for AD according to different levels of specificity, while allowing for the existence of DAPs at biological levels other than the molecular level. We offer definitions of causes, risk factors, and markers for AD based on our DAP properties and suggest future directions for research. Our framework is termed the Alzheimer’s Disease-Associated Processes and Targets (ADAPT) Ontology.
DEFINING DAP PROPERTIES
A DAP is a process defined as being “associated with” onset and prognosis. This vague definition, which we shall improve upon, is precisely so because the biomedical literature is replete with an ever-growing number of empirical and review articles referring to various processes “associated with” AD. In behavioral neuroscience, Krakauer et al. [7] argue that the existence of “filler terms” such as contributes to, is involved with, participates in, is associated with, mediates suggests “the lack of an explicit conceptual framework for the mapping between circuit and behavior.... [which] just fills in for it”. These verbs are often used instead of correlates with (for example when a biological process is modulated coordinately with disease stages), which bears the comparative advantage of having a formal statistical definition. Moreover, such terms often tacitly imply a form of causation and are used to justify the necessity to target the given DAP to therapeutic ends.
Therefore, to more accurately describe the relationship between a given DAP and disease according to explicit, quantifiable criteria, we identified three separate primary properties of DAPs by which they are defined as “associated.” DAPs derive their association with AD because of their specificity for AD, and/or frequency of appearance in AD patients, and/or pathogenic intensity with respect to dementia. For reasons we will clarify in these definitions, these properties should be understood separately, since it is not because a DAP lacks association in one of the dimensions that it does not occupy a worthy place within a holistic scheme of AD theory and therapeutics.
Specificity
This scale sorts DAPs according to an inverse function of the probability of their association with other pathologies, reflecting the extent to which a change in the DAP leads to AD and not to other pathologies. Controversies around the definition of AD suggest the improbability of a broadly-defined DAP (such as “amyloid deposition”) being exclusively involved in AD, versus healthy aging or other pathologies. Furthermore, comorbidities are the rule in the elderly brain, not the exception. Nevertheless, some DAPs are more specific to AD than to other diseases, even if this specificity is not absolute. We use the concept of 7 levels of specificity with some example DAPs to illustrate our hierarchy (Table 1 and Fig. 1).
Table 1
Attributing DAP specificity values from 1 (Fixed) to 7 (Individual mutations)
Level | Description | Value |
Fixed | Chronological age and chromosomal sex. | 1 |
Lifestyle (Social determinants and health behaviors) | Generic DAPs linked to social determinants and lifestyle habits (education, environmental stimulation, physical exercise, nutrition, etc.). | 2 |
Pathological | DAPs associated with various pathologies affecting the whole body (obesity, autophagy dysfunction, herpes infection). | 3 |
Neurodegenerative disorder | DAPs for neurodegenerative pathologies (tau aggregation, excitotoxicity, neuroinflammation). | 4 |
Alzheimer’s disease | DAPs defining AD (amyloid-β deposition, Tau hyperphosphorylation). | 5 |
Alzheimer’s disease (sub)type | DAPs differentiating AD subtypes (e.g., amyloid-β, Tau strains). | 6 |
AD-determining | DAPs (e.g., ADAD mutations) | 7 |
The least specific DAPs for AD are chronological aging and chromosomal sex—determined risk factors for many chronic pathologies—whereas the most specific DAPs are specific aggregates of amyloid-β (senile plaques) and tau (tangles), these neuropathological hallmarks being part of this disease’s definition. Individual-level specificity accounts for the existence of heritable forms of familial AD. It must be stressed here that the “value” attributed to specificity here is a code to facilitate communication around classification with the computer in our disease ontology, and should not be understood as a measure of “importance.”.
Fig. 1
A schematic representation of our hypothetical model of disease associated processes (DAPs) for Alzheimer’s disease (AD) sorted by level of specificity. The schema allows for the understanding of why a specific patient or patient population may develop AD due to the converging pathological burden of several DAPs at different specificity levels. Fixed DAPs cannot be changed (age, sex chromosomes). DAPs of social determinants and lifestyle, such as smoking and alcohol use, are the next least specific DAPs. Pathological DAPs are those which clinicians use to define different diseases; DAPs involved in neurodegenerative disease contribute to cognitive decline in AD, but for non-specific reasons, e.g., cerebrovascular impairments. Amyloid-β accumulation and tau hyperphosphorylation are used to define AD-type impairments as per the literature. AD-determining DAPs are restrained to deterministic mutations affecting amyloid-β metabolism in familial autosomal dominant AD (ADAD). Aβ, amyloid-β; fAD, familial (autosomal dominant) AD (aka. ADAD).
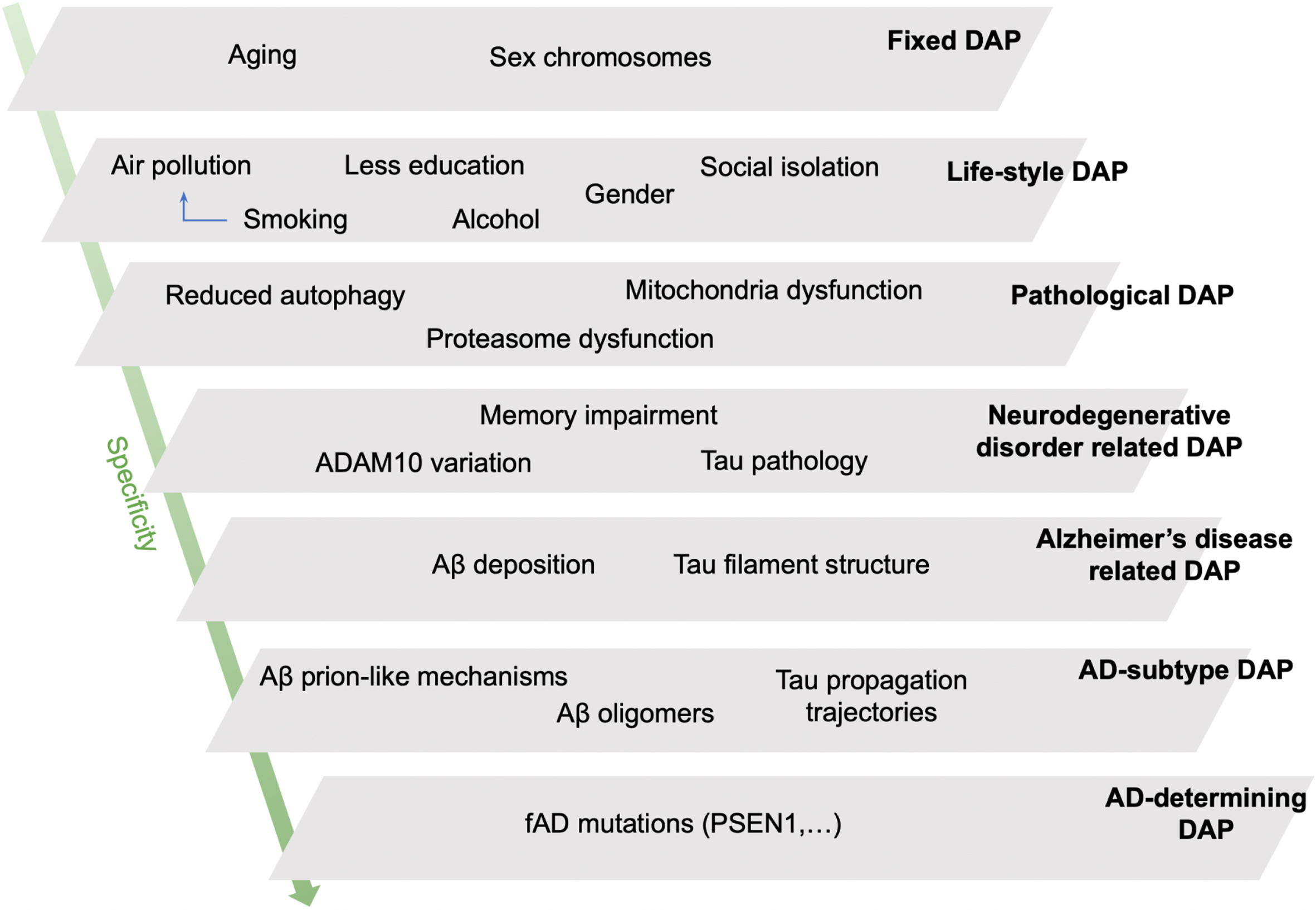
Specificity offers three advantages. Firstly, it establishes a first hierarchy of the DAPs, beyond the molecular level (Fig. 1). Secondly, it is based on accessible biomedical knowledge. Finally, this scale is sufficiently detailed to be able to specify sub-processes of broad DAPs (referred to as sub-DAPs henceforth). With this in mind, we perform a qualitative analysis of tau-related pathology and autophagy dysfunction (Fig. 2). Tau protein dysfunction has a relatively high level of specificity for AD without being entirely specific, as it is also found in tauopathies such as progressive supranuclear palsy for example (Fig. 2A). Autophagy is an evolutionary-conserved, ubiquitous, cellular pathway allowing for the degradation of cellular components in lysosomes, and is our second example of specificity analysis (Fig. 2B). These examples show how DAPs at the same biological “level” can be more or less specific for AD, and how DAPs identified at different levels are interrelated within a single coherent framework (e.g., lifestyle “diet” affecting “autophagy” and its various sub-DAPs).
Fig. 2
Multi-level specificity analyses for tau-related pathology and autophagy. Arrows connect sub-parts (higher specificity) to part (lower specificity). a) Tau aggregation is a specific instance of protein aggregation, observed in many pathologies [8]. “Tau” is neurodegenerative-level. Tau filaments are composed of different isoforms: AD filaments contain all six isoforms expressed in the human brain, while progressive supranuclear palsy filaments contain only four-repeat isoforms [9]. Recent structural studies using cryo-electron microscopy have characterized tau filaments with unique structures associated with disease [10–12]. Although a single conformer is described per disease for now, converging evidence suggests that tau strains could be unique to single patients or subsets [13–15]. Likewise, the profile of post-translational modifications (PTMs) may be specific to AD and to certain subgroups of AD patients [16]. Tau seeding activity is different between subtypes of AD patients (slow and fast progressers) [13], and four distinct trajectories of tau deposition have been identified in AD [17]. b) Autophagy is influenced by ageing and nutritional status [18], and autophagy dysfunction is observed in many pathologies, including AD [19, 20]. Genetic alteration of murine autophagy alters neuronal proteostasis, resembling neurodegenerative conditions [21, 22]. Mammals exhibit three coordinate and partially compensatory forms of autophagy, which differ in lysosomal cargo delivery mechanisms: macroautophagy, chaperone-mediated autophagy (CMA), and microautophagy. Because of more abundant literature on them, we consider only macroautophagy and CMA. There are signatures of autophagy impairments in AD and other diseases at different levels [19, 23, 24]. Specificity increases between pathways (macroautophagy/CMA) and steps within a given pathway (orange text).
![Multi-level specificity analyses for tau-related pathology and autophagy. Arrows connect sub-parts (higher specificity) to part (lower specificity). a) Tau aggregation is a specific instance of protein aggregation, observed in many pathologies [8]. “Tau” is neurodegenerative-level. Tau filaments are composed of different isoforms: AD filaments contain all six isoforms expressed in the human brain, while progressive supranuclear palsy filaments contain only four-repeat isoforms [9]. Recent structural studies using cryo-electron microscopy have characterized tau filaments with unique structures associated with disease [10–12]. Although a single conformer is described per disease for now, converging evidence suggests that tau strains could be unique to single patients or subsets [13–15]. Likewise, the profile of post-translational modifications (PTMs) may be specific to AD and to certain subgroups of AD patients [16]. Tau seeding activity is different between subtypes of AD patients (slow and fast progressers) [13], and four distinct trajectories of tau deposition have been identified in AD [17]. b) Autophagy is influenced by ageing and nutritional status [18], and autophagy dysfunction is observed in many pathologies, including AD [19, 20]. Genetic alteration of murine autophagy alters neuronal proteostasis, resembling neurodegenerative conditions [21, 22]. Mammals exhibit three coordinate and partially compensatory forms of autophagy, which differ in lysosomal cargo delivery mechanisms: macroautophagy, chaperone-mediated autophagy (CMA), and microautophagy. Because of more abundant literature on them, we consider only macroautophagy and CMA. There are signatures of autophagy impairments in AD and other diseases at different levels [19, 23, 24]. Specificity increases between pathways (macroautophagy/CMA) and steps within a given pathway (orange text).](https://content.iospress.com:443/media/jad/2023/94-s1/jad-94-s1-jad221004/jad-94-jad221004-g002.jpg)
Frequency
What we define as frequency is the percentage of AD patients with the DAP versus healthy controls or other conditions. Thus, by definition, the appearance of amyloid-β in senile plaques and tau in neurofibrillary tangles is observed in 100% of cases, given that these DAPs define the disease. However, Apolipoprotein E (APOE) haplotype, a gene coding for a protein involved in cholesterol metabolism, demonstrates the separability of frequency and specificity. The presence of 1 or 2 copies of the epsilon 4 “ɛ4” (APOE4) allele is a risk factor for various neurodegenerative diseases and is observed in over >50% of AD patients, making it three folds more common among AD patients than it is among the non-AD population, but it is possible to have sporadic AD without it, i.e., its frequency is not at 100%.
This second dimension makes it possible to establish a hierarchy of DAPs independent of specificity. However, working out the details of the hierarchy of DAPs according to this scale is more difficult to apply than for specificity, since it is based on knowledge derived from technology-dependent large-scale cohort analysis which remains to be undertaken for the vast majority of DAPs (though see below).
Pathogenic intensity
Pathogenic intensity is a reflection of the extent to which a change in the DAP is associated with a worsening of AD prognosis, and how much therapeutic impact would be likely to be obtained from targeting the DAP. Figure 3 offers a visual representation of the 3 DAP properties frequency, specificity, and pathogenic intensity.
Fig. 3
Visualizing specificity, frequency, and pathogenic intensity. Genetic examples confirm the intuition some DAPs are associated with greater pathogenicity in AD. a) Genome-wide association studies demonstrating the variable pathogenic impact of single nucleotide polymorphisms (SNPs) for sporadic AD, such as ADAM10 involved in lipid metabolism. Yet these SNPs in sporadic AD are not deterministic. b) In contrast, deterministic AD mutations in familial AD (e.g., PSEN1) are nearly 100% penetrant, but they are exceedingly rare. c) The non-genetic factor of smoking provides a lifestyle DAP probably representing a small but significant contribution to the dementia burden at the population level. d) Visualizing the differential implication of DAPs along three dimensions to show typical DAPs of lifestyle DAP (smoking), SNP (ADAM10), and infrequent deterministic DAPs (PSEN1 mutation). Table 1 of Livingston et al. [2] offers population-level intensity scores for dementia.
![Visualizing specificity, frequency, and pathogenic intensity. Genetic examples confirm the intuition some DAPs are associated with greater pathogenicity in AD. a) Genome-wide association studies demonstrating the variable pathogenic impact of single nucleotide polymorphisms (SNPs) for sporadic AD, such as ADAM10 involved in lipid metabolism. Yet these SNPs in sporadic AD are not deterministic. b) In contrast, deterministic AD mutations in familial AD (e.g., PSEN1) are nearly 100% penetrant, but they are exceedingly rare. c) The non-genetic factor of smoking provides a lifestyle DAP probably representing a small but significant contribution to the dementia burden at the population level. d) Visualizing the differential implication of DAPs along three dimensions to show typical DAPs of lifestyle DAP (smoking), SNP (ADAM10), and infrequent deterministic DAPs (PSEN1 mutation). Table 1 of Livingston et al. [2] offers population-level intensity scores for dementia.](https://content.iospress.com:443/media/jad/2023/94-s1/jad-94-s1-jad221004/jad-94-jad221004-g003.jpg)
Pathogenic intensity is independent of frequency and specificity. Nevertheless, while the prioritization of DAPs by pathogenic intensity is conceptually consistent, as in the case of frequency, the current possibility of assigning a value to them is very limited, since it requires an estimate of the impact of DAPs at the population- and patient-level; meta-analyses of genome-wide association studies and lifestyle risk factors provide the best opportunity of quantifying pathogenicity scores for AD [2]. However, homogenizing different data types into a coherent scheme of AD risk remains a large conceptual and empirical obstacle, since there are currently no algorithms available to translate statistical conclusions into a risk factor for an individual AD patient. This is particularly difficult with ADAD patients with deterministic amyloidogenic mutations who represent a very small percentage of total AD cases. For example, observational data suggest that physical activity may be even more protective in ADAD patients than in sporadic AD patients [25], inviting further comparative studies.
ILLUSTRATING THE REASONING BEHIND THE MODEL
Inspired by Herrup’s important community-wide call to “choose them all” when studying DAPs for AD, we have sought to offer properties of DAPs so as to avoid putting them all on the same footing.
To illustrate our reasoning in another medical condition, we apply DAP properties to understand bone fracture, i.e., a partial or complete break in the continuity of a bone. Explaining instances of bone fracture requires appeals to different types of association. Highly specific DAPs for bone fractures include very rare mutations in cartilage-associated protein (CRTAP), prolyl 3-hydroxylase 1 (P3H1), and Peptidylprolyl Isomerase B (PPIB), which cause a condition known as osteogenesis imperfecta [26], or brittle bone disease. Intense DAPs for bone fractures are different kinds of falls [27]. Falls are not specific to bone fractures, as they lead to tissue damage that may produce phenotypes other than fractures. Finally, for an example of a frequent DAP in bone fractures, low bone mineral density is an example [28]. These examples show that plotting association along different dimensions is fruitful and complementary.
We offer a putative lexicon for terms used frequently in AD research articles derived from the primary properties that we have identified for DAPs (specificity, frequency, and intensity): cause, risk factor, and markers (Table 2).
Table 2
A putative AD lexicon founded upon DAP properties: Risk factor, cause, and marker
Definition | DAP property must satisfy the following minimal criteria in AD patients: | ||
Specificity for AD | High frequency in AD patients | Pathogenic Intensity | |
Risk factor | Low to intermediate | Not for all patients | Low to intermediate |
Cause | High | Not for all patients | High |
Marker | High | Yes for most patients | None |
Risk factor: any DAP associated with AD onset and prognosis. A cause: a specific risk factor, i.e., be associated via both pathogenic intensity and specificity. ADAD mutations are low-frequency causes. A marker: both specific and high frequency in AD, in order to discriminate AD from non-AD states. However, markers themselves need not be associated with pathogenic intensity.
THE ADAPT ONTOLOGY
The three DAP properties described—specificity, frequency, and pathogenic intensity—can be used to design an “ontology” of AD, where DAPs can be defined according to logical relationships in a common framework to be completed with quantitative knowledge of pathogenic intensity and frequency in AD patients.
Briefly, in computer science, ontologies are resources that formalize concepts in relation to each other and produce controlled (natural language) vocabulary, thus reducing ambiguity for humans and computers. Today, there are many biomedical ontologies (bio-ontologies) (https://bioportal.bioontology.org). Once centralized, they are implicitly linked by “mapping” between common terms.
We have designed ADAPT aimed at classifying DAPs according to our 3 properties for AD. This ontology is subdivided into 2 main types of classes: Identified disease-associated process and classified disease-associated process. Identified disease-associated process (iDAP) is the branch of ontology in which the known DAPs for AD are classified according to their traditional biological identification. It is subdivided into 6 subclasses: molecular DAP, physiological DAP, environmental DAP, gene-related DAP, pathological DAP, and other DAP.
Classified disease-associated process (cDAP) is the branch of ontology that contains our three properties as the paradigm of hierarchization. It is therefore divided into 3 sub-classes: disease-associated process by specificity, disease-associated process by frequency, and disease-associated process by pathogenic intensity. They are themselves subdivided into subclasses representative of the scale of the property (by specificity: lifestyle DAP, pathogenic-type DAP, neurodegenerative DAP, AD DAP, AD-subtype DAP, and AD-determining DAP; as illustrated in Fig. 1 and Table 1). All of these subclasses aim to automatically integrate the DAPs previously listed in the branch iDAPs by computed inference, resulting in an ontological model that is functional, coherent, and flexible. While other bio-ontologies also focus on AD (Alzheimer’s Disease Ontology (ADO) and Common Alzheimer’s Disease Research Ontology (CADRO)), they aim to compile AD entities in order to index projects or data. ADAPT focuses on DAPs and aims to represent them according to their impact on AD.
ADAPT is best understood as a continuation of Herrup’s community-wide call to “choose them all,” i.e., not to reduce the scope of useful therapeutic targets in AD to one pathway, but we also provide three criteria to focus efforts and reduce ambiguity. It should therefore contribute to conceptual and therapeutic improvements. However, the long-term value of the model provided here will depend on concerted field-wide efforts to foster the convergence of efforts.
Concerning conceptual improvements, long-standing debates about the role of DAPs in AD etiology should be resolved with the use of precise language about exactly which DAPs and sub-DAPs are under investigation in experimental investigation. One necessary condition for such debate will be data precision. The AD literature is a heterogeneous entity, a reflection of the different profiles working within it with different methods, theories, and objectives. Improving signal-to-noise within the literature requires an end-to-end data quality approach. Thus, as data quantity increases exponentially, in order to not lose sight of a coherent, quantified and hierarchized picture of the relationships between DAPs and AD, the community should aim to improve the labelling of published results. This is made possible thanks to the existence of Medical Subject Headings (MeSH) terms in PubMed, offering a semantic framework which can be adapted to DAPs in order to improve their description of how they affect AD prognosis according to our criteria of specificity, frequency, and pathogenic intensity.
Those relationships between DAPs and AD etiology should be quantified and hierarchized, so as to improve precision. Where MeSH terms are not precise enough for molecular processes, the terminology of gene ontology (GO) consortium for genes and gene products can be used [29]. While there are and certainly will be DAPs and sub-DAPs in AD which do not belong to either MeSH or GO terminology, existing resources with controlled vocabulary such as ADO should be used as much as possible so as to avoid ambiguity in the literature. Thus, ADAPT has adopted and encourages the use of pre-existing terms. As community-wide efforts to improve homogenization of conclusions within the literature improve, the automatic inclusion of standardized results (via MeSH/GO/ADO) into a quantifiable, hierarchized scheme like our ADAPT ontology, should be promoted.
However, the comparisons with other ontologies are limited, since ADAPT has been designed to play a very specific role to order DAPs to therapeutic ends following Herrup [6] and should be assessed according to how satisfyingly it helps to achieve this end. Other ontologies are useful for annotations and researching information, whereas ADAPT is to be used for profiling DAPs as a function of their specificity, frequency, or pathogenic intensity, thus adapting to the scientific literature.
While we have defined and highlighted specificity, there is arguably no more specific treatment for AD than anti-amyloid strategies. However, the last 20 years of unsuccessful anti-amyloid clinical trials have shown how difficult elaborating a specific treatment for AD is. Furthermore, for other indications, such as cardiovascular disease, non-specific treatments (e.g., statins) have indeed shown that important public health gains can be made by treatments against a treatment despite them lacking specificity.
Thus, every DAP property is important when choosing therapeutic targets for AD, and when considering treatments, further or “secondary” DAP properties could be elaborated, which though they do not define DAPs, may instead qualify their suitability in a therapeutic scheme. Figure 2b shows how DAPs with varying specificity interact (e.g., diet and autophagy). This could allow for non-reductive discussion of how knowledge from different approaches to treatment (e.g., lifestyle interventions) can be both explored now for their disease-reducing potential, and also used to inspire pharmacology-based neurological approaches against specific DAPs thanks to study of mechanisms. But importantly, their therapeutic value at the community level should not be reduced to the reductionist strategy, since specificity should not be the only criterion used in developing treatments.
Finally, the notion of specificity can also be further analyzed. Given that AD is defined as a clinico-pathological entity, it follows that the notion of specificity can also be separated along these two dimensions where the separability of clinical and pathological specificity becomes more apparent for highly specific DAPs. For example, amyloid and tau are highly pathologically specific for AD; while ADAD mutations suggest amyloid’s high pathological specificity for AD, clinically speaking, however, tau pathology has better topographical clinical specificity for sporadic AD than amyloid-β depositions [30].
The suppleness of the term “associated” in “disease-associated process” also allows clinical manifestations of AD themselves to be considered as DAPs with different specificities, frequencies, and correlation with cognitive decline. For instance, hippocampal-type amnestic syndrome is more specific than subjective cognitive decline and is a better predictor of future cognitive decline in AD [31]. Future studies with comparisons of different symptoms of AD at different stages of the disease are required in order to clarify their respective weight as DAPs. Intra-cohort normalization of cognitive assessment might serve this endeavor. Clinical DAPs can also be correlated with their biological, structural, or functional underpinnings in order to promote useful discussion about DAPs and their interactions [32]. Such discussion could be useful in the design and evaluation of clinical trials targeting specific DAPs and measuring specific clinical outcomes.
CONCLUSION
In the spirit of adaptation to the major problem of AD, we offer ADAPT as a collaborative platform for vital communication in the heterogeneous AD research community. We hope that it can offer objective criteria to ground fruitful discussion and strategy around priority-setting, thus speeding up therapeutic efforts against AD and avoiding injustices against defenders of different theories [33].
ACKNOWLEDGMENTS
Thanks to Drs. Stéphane Epelbaum and Fabrizio de Falliani for help in developing the manuscript. An earlier version of the ADAPT ontology (“AD-DROP”) appeared as a Poster presentation at the Alzheimer’s Association International Congress 2021 and can be found here: https://alz-journals.onlinelibrary.wiley.com/doi/full/10.1002/alz.051653.
FUNDING
This work was written mostly during Timothy Daly’s PhD thesis, funded for the years 2017–2020 by a Sorbonne Université doctoral contract and for the years 2019–2021 by a doctoral bursary from the Fondation Médéric Alzheimer. There is no other funding to report.
CONFLICT OF INTEREST
Timothy Daly is an Associate Editor of the Journal of Alzheimer’s Disease but was not involved in the peer-review process nor had access to any information regarding its peer-review. The authors have no other conflicts of interest to report.
DATA AVAILABILITY
The ADAPT ontology is available at https://zenodo.org/record/4740141#.YJaJ3i3pMUs. All the secondary data in this manuscript are derived from previously published literature available in the References section.
REFERENCES
[1] | van Dyck CH , Swanson CJ , Aisen P , Bateman RJ , Chen C , Gee M , Kanekiyo M , Li D , Reyderman L , Cohen S , Froelich L , Katayama S , Sabbagh M , Vellas B , Watson D , Dhadda S , Irizarry M , Kramer LD , Iwatsubo T ((2022) ) Lecanemab in early Alzheimer’s disease. N Engl J Med 388: , 9–21. |
[2] | Livingston G , Huntley J , Sommerlad A , Ames D , Ballard C , Banerjee S , Brayne C , Burns A , Cohen-Mansfield J , Cooper C , Costafreda SG , Dias A , Fox N , Gitlin LN , Howard R , Kales HC , Kivimäki M , Larson EB , Ogunniyi A , Orgeta V , Ritchie K , Rockwood K , Sampson EL , Samus Q , Schneider LS , Selbæk G , Teri L , Mukadam N ((2020) ) Dementiaprevention, intervention, and care: 2020 report of the LancetCommission. Lancet 396: , 413–446. |
[3] | Liu PP , Xie Y , Meng XY , Kang JS ((2019) ) History and progress of hypotheses and clinical trials for Alzheimer’s disease. Signal Transduct Target Ther 4: , 29. |
[4] | Hardy JA , Higgins GA ((1992) ) Alzheimer’s disease: The amyloid cascade hypothesis. Science 256: , 184–185. |
[5] | Daly T , Herrup K , Espay AJ (2022) An ethical argument for ending human trials of amyloid-lowering therapies in Alzheimer’s disease. AJOB Neurosci. doi: 10.1080/21507740.2022.2129858. |
[6] | Herrup K ((2015) ) The case for rejecting the amyloid cascade hypothesis. Nat Neurosci 18: , 794–799. |
[7] | Krakauer JW , Ghazanfar AA , Gomez-Marin A , MacIver MA , Poeppel D ((2017) ) Neuroscience needs behavior: Correcting a reductionist bias. Neuron 93: , 480–490. |
[8] | Aguzzi A , O’Connor T ((2010) ) Protein aggregation diseases: Pathogenicity and therapeutic perspectives. Nat Rev Drug Discov 9: , 237–248. |
[9] | Spillantini MG , Goedert M ((2013) ) Tau pathology and neurodegeneration. Lancet Neurol 12: , 609–622. |
[10] | Fitzpatrick AWP , Falcon B , He S , Murzin AG , Murshudov G , Garringer HJ , Crowther RA , Ghetti B , Goedert M , Scheres SHW ((2017) ) Cryo-EM structures of tau filaments from Alzheimer’s disease. Nature 547: , 185–190. |
[11] | Falcon B , Zhang W , Murzin AG , Murshudov G , Garringer HJ , Vidal R , Crowther RA , Ghetti B , Scheres SHW , Goedert M ((2018) ) Structures of filaments from Pick’s disease reveal a novel tau protein fold. Nature 561: , 137–140. |
[12] | Zhang W , Tarutani A , Newell KL , Murzin AG , Matsubara T , Falcon B , Vidal R , Garringer HJ , Shi Y , Ikeuchi T , Murayama S , Ghetti B , Hasegawa M , Goedert M , Scheres SHW ((2020) ) Novel tau filament fold in corticobasal degeneration. Nature 580: , 283–287. |
[13] | Dujardin S , Commins C , Lathuiliere A , Beerepoot P , Fernandes AR , Kamath TV , De Los Santos MB , Klickstein N , Corjuc DL , Corjuc BT , Dooley PM , Viode A , Oakley DH , Moore BD , Mullin K , Jean-Gilles D , Clark R , Atchison K , Moore R , Chibnik LB , Tanzi RE , Frosch MP , Serrano-Pozo A , Elwood F , Steen JA , Kennedy ME , Hyman BT ((2020) ) Tau molecular diversity contributes to clinical heterogeneity in Alzheimer’s disease. Nat Med 26: , 1256–1263. |
[14] | Kaufman SK , Sanders DW , Thomas TL , Ruchinskas AJ , Vaquer-Alicea J , Sharma AM , Miller TM , Diamond MI ((2016) ) Tau prion strains dictate patterns of cell pathology, progression rate, and regional vulnerability in vivo. Neuron 92: , 796–812. |
[15] | Sanders DW , Kaufman SK , DeVos SL , Sharma AM , Mirbaha H , Li A , Barker SJ , Foley AC , Thorpe JR , Serpell LC , Miller TM , Grinberg LT , Seeley WW , Diamond MI ((2014) ) Distinct tau prion strains propagate in cells and mice and define different tauopathies. Neuron 82: , 1271–1288. |
[16] | Wesseling H , Mair W , Kumar M , Schlaffner CN , Tang S , Beerepoot P , Fatou B , Guise AJ , Cheng L , Takeda S , Muntel J , Rotunno MS , Dujardin S , Davies P , Kosik KS , Miller BL , Berretta S , Hedreen JC , Grinberg LT , Seeley WW , Hyman BT , Steen H , Steen JA ((2020) ) Tau PTM profiles identify patient heterogeneity and stages of Alzheimer’s disease. Cell 183: , 1699–1713.e13. |
[17] | Vogel JW , Young AL , Oxtoby NP , Smith R , Ossenkoppele R , Strandberg OT , La Joie R , Aksman LM , Grothe MJ , Iturria-Medina Y ; Alzheimer’s Disease Neuroimaging Initiative, Pontecorvo MJ , Devous MD , Rabinovici GD , Alexander DC , Lyoo CH , Evans AC , Hansson O ((2021) ) Four distinct trajectories of tau deposition identified in Alzheimer’s disease. Nat Med 27: , 871–881. |
[18] | Kaushik S , Cuervo AM ((2015) ) Proteostasis and aging. Nat Med 21: , 1406–1415. |
[19] | Scrivo A , Bourdenx M , Pampliega O , Cuervo AM ((2018) ) Selective autophagy as a potential therapeutic target for neurodegenerative disorders. Lancet Neurol 17: , 802–815. |
[20] | Menzies FM , Fleming A , Rubinsztein DC ((2015) ) Compromised autophagy and neurodegenerative diseases. Nat Rev Neurosci 16: , 345–357. |
[21] | Hara T , Nakamura K , Matsui M , Yamamoto A , Nakahara Y , Suzuki-Migishima R , Yokoyama M , Mishima K , Saito I , Okano H , Mizushima N ((2006) ) Suppression of basal autophagy in neural cells causes neurodegenerative disease in mice. Nature 441: , 885–889. |
[22] | Komatsu M , Waguri S , Chiba T , Murata S , Iwata J , Tanida I , Ueno T , Koike M , Uchiyama Y , Kominami E , Tanaka K ((2006) ) Loss of autophagy in the central nervous system causes neurodegeneration in mice. Nature 441: , 880–884. |
[23] | Menzies FM , Fleming A , Caricasole A , Bento CF , Andrews SP , Ashkenazi A , Füllgrabe J , Jackson A , Jimenez Sanchez M , Karabiyik C , Licitra F , Lopez Ramirez A , Pavel M , Puri C , Renna M , Ricketts T , Schlotawa L , Vicinanza M , Won H , Zhu Y , Skidmore J , Rubinsztein DC ((2017) ) Autophagy and neurodegeneration: Pathogenic mechanisms and therapeutic opportunities. Neuron 93: , 1015–1034. |
[24] | Caballero B , Bourdenx M , Luengo E , Diaz A , Sohn PD , Chen X , Wang C , Juste YR , Wegmann S , Patel B , Young ZT , Kuo SY , Rodriguez-Navarro JA , Shao H , Lopez MG , Karch CM , Goate AM , Gestwicki JE , Hyman BT , Gan L , Cuervo AM ((2021) ) Acetylated tau inhibits chaperone-mediated autophagy and promotes tau pathology propagation in mice. Nat Commun 12: , 2238. |
[25] | Müller S , Preische O , Sohrabi HR , Gräber S , Jucker M , Ringman JM , Martins RN , McDade E , Schofield PR , Ghetti B , Rossor M , Fox NN , Graff-Radford NR , Levin J , Danek A , Vöglein J , Salloway S , Xiong C , Benzinger T , Buckles V , Masters CL , Sperling R , Bateman RJ , Morris JC , Laske C ; Dominantly InheritedAlzheimer Network (DIAN) ((2018) ) Relationship between physicalactivity, cognition, and Alzheimer pathology in autosomal dominantAlzheimer’s disease. Alzheimers Dement 14: , 1427–1437. |
[26] | van Dijk FS , Nesbitt IM , Zwikstra EH , Nikkels PG , Piersma SR , Fratantoni SA , Jimenez CR , Huizer M , Morsman AC , Cobben JM , van Roij MH , Elting MW , Verbeke JI , Wijnaendts LC , Shaw NJ , Högler W , McKeown C , Sistermans EA , Dalton A , Meijers-Heijboer H , Pals G ((2009) ) PPIB mutations cause severe osteogenesis imperfecta. Am J Hum Genet 85: , 521–527. |
[27] | Luukinen H , Herala M , Koski K , Honkanen R , Laippala P , Kivelä SL ((2000) ) Fracture risk associated with a fall according to type of fall among the elderly. Osteoporos Int 11: , 631–634. |
[28] | Li HL , Shen Y , Tan LH , Fu SB , Dai RC , Yuan LQ , Sheng ZF , Xie ZJ , Wu XP , Liao EY , Tang XL , Wu XY ((2021) ) Relationship between bone mineral density and fragility fracture risk: A case-control study in Changsha, China. BMC Musculoskelet Disord 22: , 728. |
[29] | Gene Ontology Consortium ((2019) ) The Gene Ontology Resource: 20 years and still GOing strong. Nucleic Acids Res 47(D1): , D330–D338. |
[30] | La Joie R , Visani AV , Lesman-Segev OH , Baker SL , Edwards L , Iaccarino L , Soleimani-Meigooni DN , Mellinger T , Janabi M , Miller ZA , Perry DC , Pham J , Strom A , Gorno-Tempini ML , Rosen HJ , Miller BL , Jagust WJ , Rabinovici GD ((2021) ) Association of APOE4 and clinical variability in Alzheimer disease with the pattern of tau- and amyloid-PET. Neurology 96: , e650–e661. |
[31] | Di Stefano F , Epelbaum S , Coley N , Cantet C , Ousset PJ , Hampel H , Bakardjian H , Lista S , Vellas B , Dubois B , Andrieu S ; GuidAge study group ((2015) ) Prediction of Alzheimer’s disease dementia: Data from the GuidAge Prevention Trial. J Alzheimers Dis 48: , 793–804. |
[32] | Toschi N , Lista S , Baldacci F , Cavedo E , Zetterberg H , Blennow K , Kilimann I , Teipel SJ , Melo Dos Santos A , Epelbaum S , Lamari F , Genthon R , Habert MO , Dubois B , Floris R , Garaci F , Vergallo A , Hampel H ; INSIGHT-preAD study group; Alzheimer Precision Medicine Initiative (APMI) ((2019) ) Biomarker-guided clustering of Alzheimer’s disease clinical syndromes. Neurobiol Aging 83: , 42–53. |
[33] | Daly T , Houot M , Barberousse A , Petit A , Epelbaum S ((2021) ) A proposal to make biomedical research into Alzheimer’s disease more democratic following an international survey with researchers. J Alzheimers Dis Rep 5: , 637–645. |