Impact of Computerized Cognitive Training on Default Mode Network Connectivity in Subjects at Risk for Alzheimer’s Disease: A 78-week Randomized Controlled Trial
Abstract
Background:
Mild cognitive impairment (MCI) represents a high risk group for Alzheimer’s disease (AD). Computerized Cognitive Games Training (CCT) is an investigational strategy to improve targeted functions in MCI through the modulation of cognitive networks.
Objective:
The goal of this study was to examine the effect of CCT versus a non-targeted active brain exercise on functional cognitive networks.
Methods:
107 patients with MCI were randomized to CCT or web-based crossword puzzles. Resting-state functional MRI (fMRI) was obtained at baseline and 18 months to evaluate differences in fMRI measured within- and between-network functional connectivity (FC) of the default mode network (DMN) and other large-scale brain networks: the executive control, salience, and sensorimotor networks.
Results:
There were no differences between crosswords and games in the primary outcome, within-network DMN FC across all subjects. However, secondary analyses suggest differential effects on between-network connectivity involving the DMN and SLN, and within-network connectivity of the DMN in subjects with late MCI. Paradoxically, in both cases, there was a decrease in FC for games and an increase for the crosswords control (p < 0.05), accompanied by lesser cognitive decline in the crosswords group.
Conclusion:
Results do not support a differential impact on within-network DMN FC between games and crossword puzzle interventions. However, crossword puzzles might result in cognitively beneficial remodeling between the DMN and other networks in more severely impaired MCI subjects, parallel to the observed clinical benefits.
INTRODUCTION
Mild cognitive impairment (MCI) is a common condition in older adults characterized by mild cognitive deficits with largely intact functions of daily living. It is considered a transitional stage between healthy aging and dementia and represents a high-risk group for Alzheimer’s disease (AD) [1, 2]. MCI can be divided into early and late stages, depending on the extent of episodic memory impairment. Late MCI (LMCI) subjects represent individuals with higher degrees of cognitive and functional impairment than early MCI (EMCI) subjects, and their rate of progression is faster [3]. There is growing evidence that a cognitively active lifestyle may reduce rates of dementia. A systematic review of 22 population-based studies found that mental activities may reduce overall incident dementia risk by 46% over a median 7-year period [4].
Computerized Cognitive Training (CCT) provides a novel strategy to improve cognitive performance in MCI by supporting a more cognitively active lifestyle. CCT involves computerized cognitive exercises that target specific abilities, for example, memory, executive function, language and attention, to improve cognitive functioning. This effect is durable and likely mediated by neuroplasticity in large-scale brain networks. CCT has been used successfully to improve cognitive functioning in individuals with MCI. A recent meta-analysis examining CCT in MCI subjects showed overall moderate efficacy in improving cognitive outcomes [5].
Currently, the brain mechanisms underlying the observed effects of CCT remain unknown. Previous studies of CCT using functional imaging to understand how large-scale networks are modified have been conducted mainly in healthy older adults [6–9]. These studies suggest remodeling of the default mode network (DMN) as a possible mechanism. The DMN is a resting-state functional network of several highly interconnected cortical hubs, including the posteromedial parietal, anteromedial frontal, and inferolateral parietal cortices, which has been implicated in the progression of MCI to AD [10]. Our group has previously shown that impaired deactivation and functional connectivity (FC) in the DMN may be a significant predictor in MCI subjects of poor memory and transition to dementia over a 2–3 year follow-up period [10, 11]. It is unclear, however, whether CCT might normalize connectivity within the DMN and/or between the DMN and other cognitive networks in these subjects, and whether such changes might correlate with cognitive improvement.
Here we report the first randomized controlled trial in subjects with EMCI and LMCI examining the effects of home-based CCT on resting-state connectivity within the DMN as well as within and between other functional networks modulated by CCT effects. The primary outcome cognitive findings from this trial are being reported separately [12]. Our hypothesis was that CCT would normalize changes in the DMN from MCI, compared to a crossword puzzle control condition, and that the resulting network changes would correlate with a slowing of cognitive decline or with cognitive improvement.
MATERIALS AND METHODS
A detailed description of trial design features and study intervention of this two-site study has been published elsewhere [12–14]. The prior articles dealt with the primary and secondary neuropsychological testing outcomes as a result of CCT, whereas we report on the within- and between-network connectivity of the DMN and other functional networks influenced by the CCT intervention. The study was of a 78-week duration, in which 107 participants were randomized into CCT (games) or crossword puzzle training arms, with individuals stratified by EMCI versus LMCI, age, and site. The training was administered by Lumosity, a web-based platform. Participants in both training arms underwent an intensive training period of four 30-min sessions per week during the first 12 weeks, followed by booster sessions at scheduled time points. Subjects were scheduled to come to the clinic for five scheduled visits (Weeks 0, 12, 32, 52, and 78) to complete further training as well as neuropsychological testing. Enrolled individuals were within the age ranges of 55–95 years, expressed cognitive concerns, e.g., naming/language, and scored≥23 on the Folstein Mini-Mental State Examination (MMSE). EMCI and LMCI categorization was determined by years of education combined with a score between 0 and 11 on the Wechsler Memory Scale-III (WMS-III) Logical Memory Story A, per the Alzheimer’s Disease Neuroimaging Initiative (ADNI) diagnostic criteria. Notable exclusions included a history of major psychiatric or neurological illness, dementia diagnosis, contraindication to magnetic resonance imaging (MRI) scan, lack of English-speaking ability, regular engagement with digital brain training or crossword puzzles, and diagnosis of Major Depressive Disorder.
Image acquisition
At baseline and end-study, subjects at both sites were imaged with high-resolution T1-weighted IR-prepped 3D-SPGR, T2 FLAIR, and GE-EPI resting-state functional MRI (fMRI) scans. Supplementary Table 1 lists the scanners and scan parameters at both sites. For the resting-state fMRI sequence, participants were instructed to remain still with their eyes open and fixated on a white cross-hair displayed on a black background. Following shimming and calibration scans, a single 10-minute resting-state fMRI scan was acquired. Imaging procedures were the same at both baseline and endpoint.
fMRI quality control
fMRI was evaluated using three quality control (QC) criteria to assess for excessive motion, signal change and focal image distortion, based on established criteria [15, 16]. Motion was evaluated on the basis of orthogonal translation and rotation plots allowing no single movement translation > 3 mm or rotation > 3 degrees. Global and brain mean signal time course could not exceed 3% of baseline. fMRI source images were to be free of focal distortion by visual inspection. Subjects needed to pass all QC criteria on both baseline and 78 week fMRI scans to be included in the final analysis.
Image preprocessing
Structural magnetic resonance images were preprocessed using standard steps. Bias correction, skull stripping, tissue segmentation, and spatial normalization were performed using voxel-based morphometry [17] in SPM12 [18] where the above steps were integrated into one model. Modulated normalized parameters were used to segment the images into gray matter (GM), white matter (WM), and cerebrospinal fluid (CSF) probabilistic maps. Images were resliced to 3×3×3 mm3 with 4th degree B-spline for interpolation and smoothed with a Gaussian kernel of full-width half maximum (FWHM) of 8×8×8 mm3.
Functional images were slice time corrected with the first slice as the reference frame. Images were motion-corrected with the first timepoint with rigid body translation and rotation. Subjects with greater than 0.3 mm mean framewise displacement (FD) were excluded from further analysis. Time points of motion greater than 0.2 mm were noted and were included as motion outlier regressors. Co-registration of functional images onto structural images was performed in multiple steps to obtain higher registration accuracy. As the first timepoint volume of fMRI contains higher image contrast, it was used to register the fMRI scans to structural images, followed by the registration of other fMRI timepoints. After co-registration, all images were visually inspected for registration accuracy and then the first five fMRI volumes were discarded to ensure steady-state longitudinal magnetization. Linear trends were removed to account for signal drift and the six motion parameters and nuisance signals (average WM and CSF) were regressed out from the fMRI time series. To reduce nonneuronal contribution to BOLD fluctuations, bandpass temporal filtering was performed in the low-frequency range from 0.01 to 0.1 Hz in line with previous resting fMRI studies. Finally, the images were spatially normalized to the MNI space with a voxel size of 3×3×3 mm3 with 4th degree B-spline for interpolation and smoothed with a Gaussian kernel of FWHM of 8×8×8 mm3.
Image analysis
Our analysis was focused on the DMN, because of its known association with cognitive decline in MCI [19]. We also performed exploratory analyses in two other cognitive networks: the executive control network (ECN) and the salience network (SLN). The ECN and SLN were investigated because they have been implicated in previous CCT studies in healthy older adults [33–35]. The sensorimotor network (SMN) was selected to serve as an internal control network [20, 21]. As changes in MCI can be associated with both within- and between-network FC, we examined both measures and they are further explained below.
Within-network functional connectivity
Within-network FC was evaluated using a priori regions of interest (ROI). The ROIs for each network were picked from Atlas55+ [22], a brain functional atlas of resting-state networks derived from fMRI data of more than 500 subjects in their late adulthood. For each network of interest, the primary hub locations were found using the peak t-value within each cluster of the network. The hub locations for the DMN and other networks are presented in Fig. 2. For each hub location a 6 mm radius sphere was constructed and the average timecourse of all voxels within the sphere was calculated. Pearson’s Correlations between the averaged time courses of all hubs of a network were calculated and the within-network FC was defined as the average of all between-hub correlations.
Between-network functional network connectivity
To account for potential connectivity changes that might occur between the DMN and other networks, a secondary group independent component analysis (ICA) was performed to identify the resting state functional networks using GIFT v3.0 [23] software on MATLAB R2021a. Group ICA was applied to fMRI data of all subjects obtained at both time points to decompose the fMRI data into twenty networks. The InfoMax algorithm was applied to identify the networks. Functional networks derived through ICA were visually inspected and labeled by comparing them against the ICA network atlas software [24], Atlas55+ and using the brain regions defined by the Harvard Oxford MNI atlas [25]. The functional networks derived through our ICA decomposition are presented in Fig. 3. The Pearson’s correlation between the timecourse of the networks was used to compute between-network FC.
Statistical analyses
Summary statistics were obtained and compared across the games and crossword groups using a Wilcoxon’s rank sum test for continuous variables and Fisher’s exact test for categorical variables. Changes in DMN within-network FC over the course of training were evaluated for games versus the crossword control condition using a linear model. The model was then stratified by EMCI versus LMCI. Changes in FC were evaluated both without and with adjusting for motion, measured by FD, and baseline FC. Changes within other networks, as well as between the DMN and other networks were also evaluated on an exploratory basis. For any FC metrics showing differences between conditions in previous analyses, Pearson correlation of change in FC with change in cognition, measured by ADAS-Cog11 score, was performed. Statistical significance was considered at the alpha < 0.05 level. As the analysis was exploratory, except for the primary outcome of DMN within-network FC, we did not adjust for multiple comparisons.
RESULTS
Participants
Between Nov 2017 and January 2020, 107 of 168 eligible participants were randomized (Fig. 1, Table 1) and the last participant completed the study in September 2021. Mean age was 71.2 years (SD 8.8), and 42% were male and 58% female. Dropout rates did not differ between games (9/51) and crosswords (7/56), p = 0.59. Among LMCI participants, dropout rate was marginally greater for games (6/30 = 20%) than crosswords (5/33 = 15.2%). Cognitive and functional outcomes in the trial have been reported elsewhere [12]. Among the 107 subjects who were randomized, 20 additional subjects were excluded based on failed fMRI QC and preprocessing criteria (12/51 for games, 8/56 for crosswords), leaving a total of 87 subjects (39 for games and 48 for crosswords) with both baseline and 78 week fMRI data. Participants assigned to games and crosswords did not differ significantly (p < 0.05) in demographic measures (Table 1).
Fig. 1
Flow diagram for participants. Note, “Underwent fMRI scanning” indicates that subjects were scanned at least at baseline, though all may not have completed the week 78 scan.
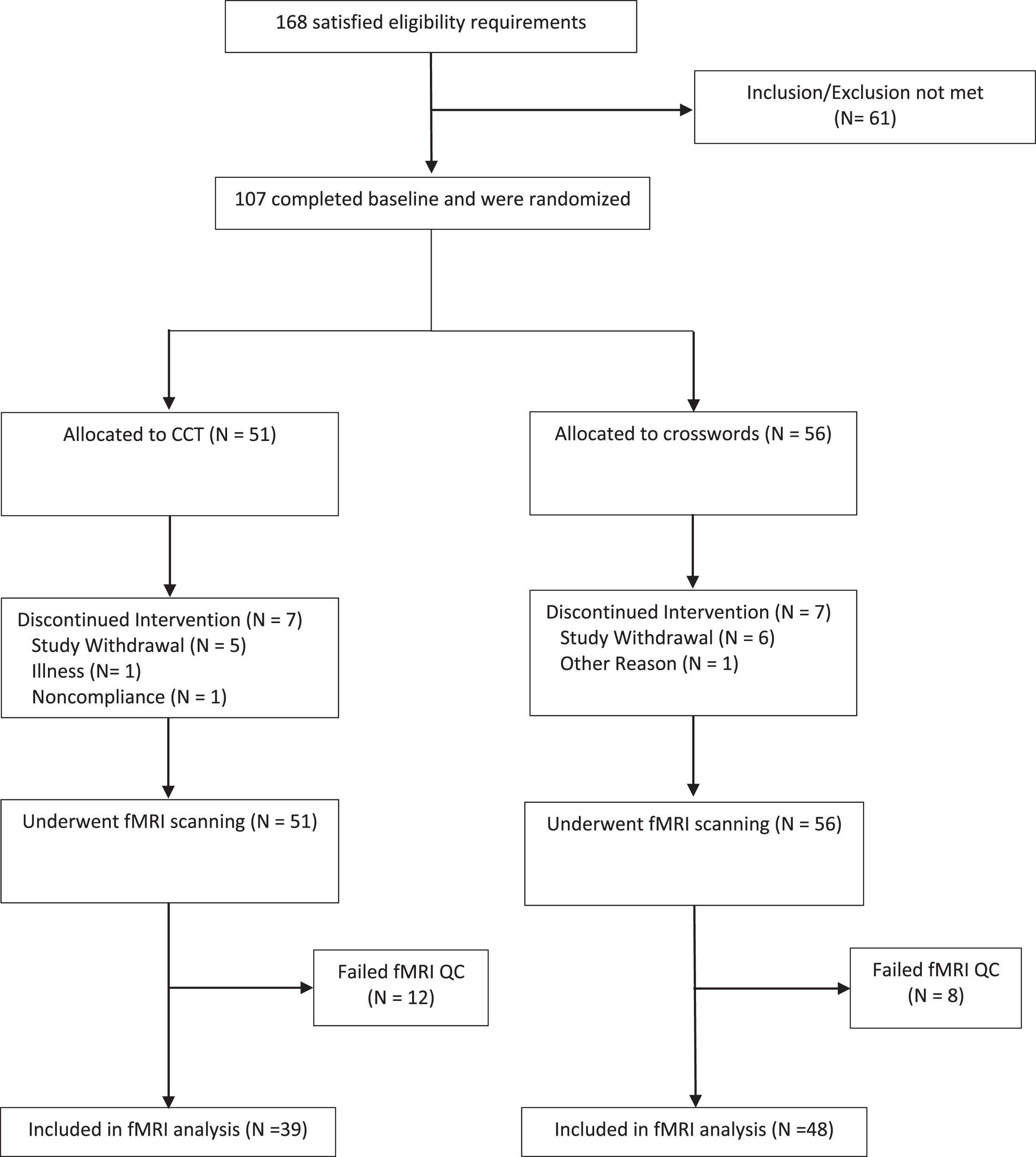
Fig. 2
Hub locations of default mode (red), executive control (blue), salience (green), and sensorimotor (yellow) networks, as defined by Atlas55+.
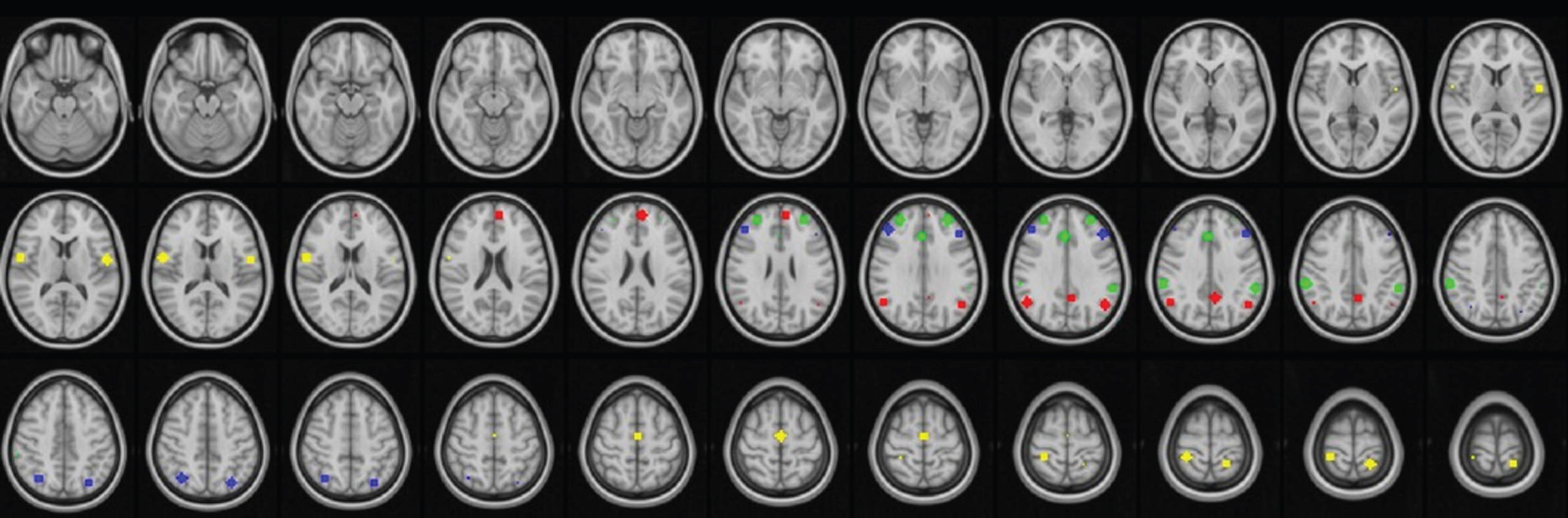
Fig. 3
Functional networks derived through independent component analysis.
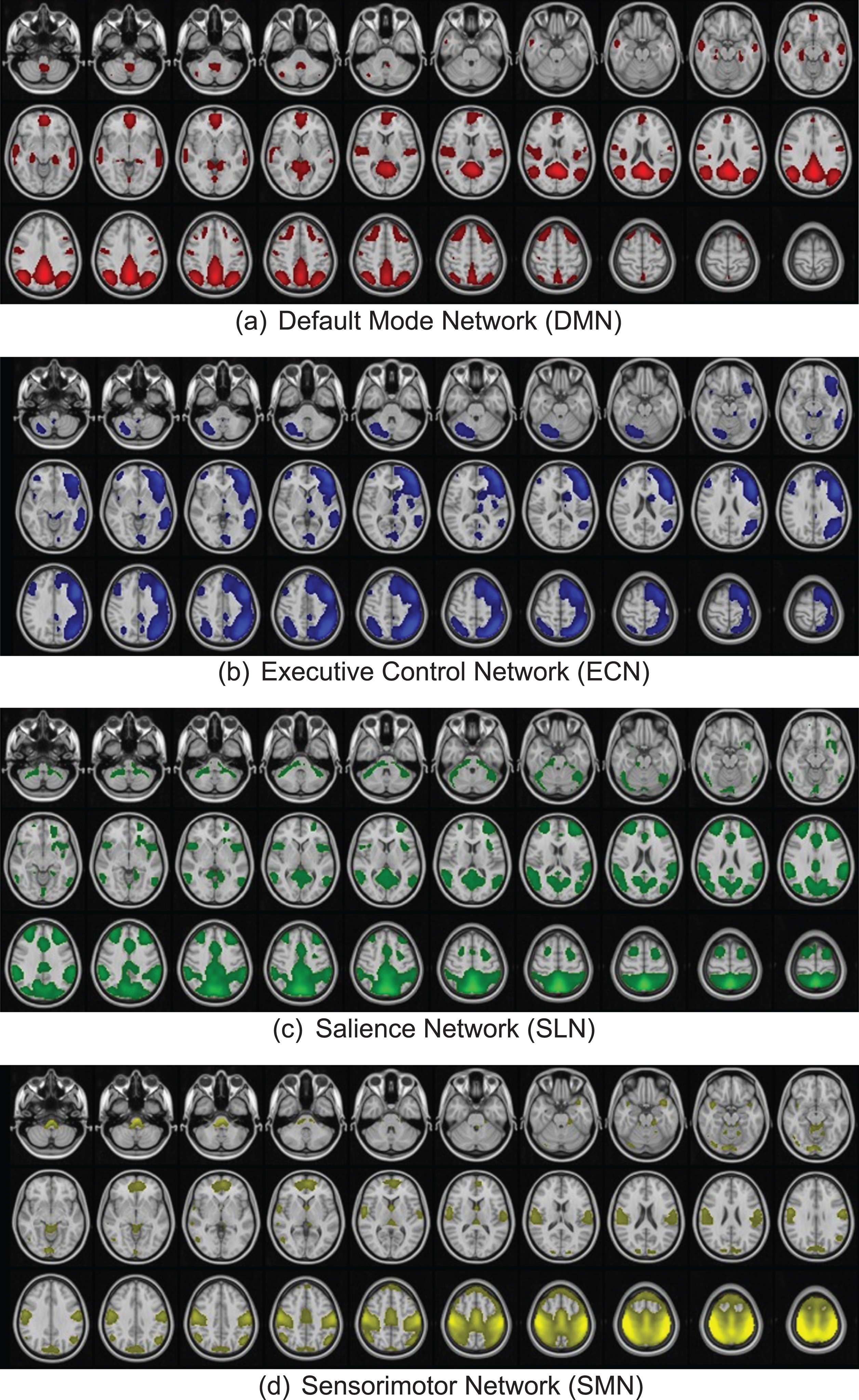
Table 1
Baseline Characteristics of the fMRI Study Sample (mean and SD)
Variable | Games | Crosswords | p |
Sample size | N=39 | N=48 | . |
Male (%) | 11/39 (28.2) | 26/48 (54.2) | 0.015 |
Age | 69.28±8.10 | 70.81±8.69 | 0.402 |
Educational level | 16.74±3.03 | 17.06±3.19 | 0.637 |
EMCI (%) | 17/39 (43.6) | 21/48 (43.8) | 0.988 |
APOE ɛ4 + genotype (%) | 21/39 (53.8) | 18/48 (37.5) | 0.127 |
MMSE | 27.33±1.68 | 27.02±1.69 | 0.392 |
ADAS-Cog | 8.77±3.37 | 9.04±3.00 | 0.691 |
Frame Displacement (FD) | 0.06±0.05 | 0.07±0.03 | 0.597 |
Default Mode Network (DMN) Connectivity | 0.49±0.16 | 0.44±0.13 | 0.156 |
Executive Control Network (ECN) Connectivity | 0.31±0.17 | 0.33±0.14 | 0.531 |
Salience Network (SLN) Connectivity | 0.34±0.12 | 0.28±0.12 | 0.020 |
DMN-ECN Between Network Connectivity | 0.12±0.22 | 0.09±0.23 | 0.512 |
DMN-SLN Between Network Connectivity | 0.03±0.25 | 0.11±0.23 | 0.139 |
ECN-SLN Between Network Connectivity | 0.06±0.25 | 0.08±0.21 | 0.584 |
Global Connectivity | 0.16±0.06 | 0.17±0.05 | 0.640 |
EMCI, early mild cognitive impairment; APOE, apolipoprotein E gene; MMSE, Mini-Mental State Examination; ADAS-Cog, Alzheimer’s Disease Assessment Scale-Cognitive subscale.
Within-network connectivity outcomes
There was no statistically significant (p < 0.05) treatment-related difference in the change from baseline in within-network connectivity involving the DMN or other networks across the entire study group. In subgroup analysis (Table 2), however, there was a statistically significant difference between the two treatments in DMN within-network connectivity in the LMCI group (p = 0.034), but not the EMCI group (p = 0.447). For the LMCI group, within network FC in the DMN decreased over the course of the trial in the games condition (–0.039±0.023), but increased in the crossword control group (0.027±0.020). This difference was statistically significant (p = 0.034). The exploratory analysis in the LMCI subgroup failed to demonstrate significant differences between the interventions in any of the other networks. For the EMCI subgroup, no significant differences between interventions were demonstrated in the DMN or other networks included in the exploratory analysis. The games and crosswords groups did not differ significantly (p < 0.05) in baseline within-network connectivity measures, with the exception of the SLN network (Table 1). This was covaried for in the analysis along with all other baseline connectivity metrics.
Table 2
Change from baseline in within-network connectivity (78 weeks – baseline)
EMCI (adjusted for change in FD and baseline value) | LMCI (adjusted for change in FD and baseline value) | |||||||
Game | Crosswords | Diff | p | Game | Crosswords | Diff | p | |
DMN_change | 0.025 (0.025) | –0.002 (0.024) | 0.027 (0.035) | 0.447 | –0.039 (0.023) | 0.027 (0.020) | –0.066 (0.031) | 0.034 |
ECN_change | 0.039 (0.029) | –0.014 (0.027) | 0.053 (0.040) | 0.186 | –0.029 (0.025) | –0.008 (0.023) | –0.021 (0.034) | 0.544 |
SLN_change | –0.056 (0.026) | –0.012 (0.023) | –0.044 (0.036) | 0.220 | 0.001 (0.022) | –0.008 (0.020) | 0.008 (0.030) | 0.779 |
SMN_change | –0.011 (0.029) | 0.059 (0.027) | –0.070 (0.039) | 0.073 | –0.027 (0.026) | –0.012 (0.022) | –0.016 (0.034) | 0.644 |
Between-network connectivity outcomes
Between baseline and 78 weeks, there was a decrease in between-network FC in involving the DMN and all other networks in the games group, whereas the crosswords group showed increased connectivity between these networks. This dissociation only reached statistical significance between the DMN and SLN, however (Fig. 4, Table 3). The games and crosswords groups did not differ significantly (p < 0.05) in baseline between-network connectivity measures (Table 1).
Fig. 4
Between-network functional connectivity change from baseline to 78-weeks (78-weeks – baseline). The games group showed a decrease in connectivity between the DMN and all other networks (ECN, SLN, SMN), whereas the crosswords group showed increased connectivity between the DMN and these networks. Values in the figure are unadjusted for change in FD and baseline FC values. For adjusted values, see Table 3.
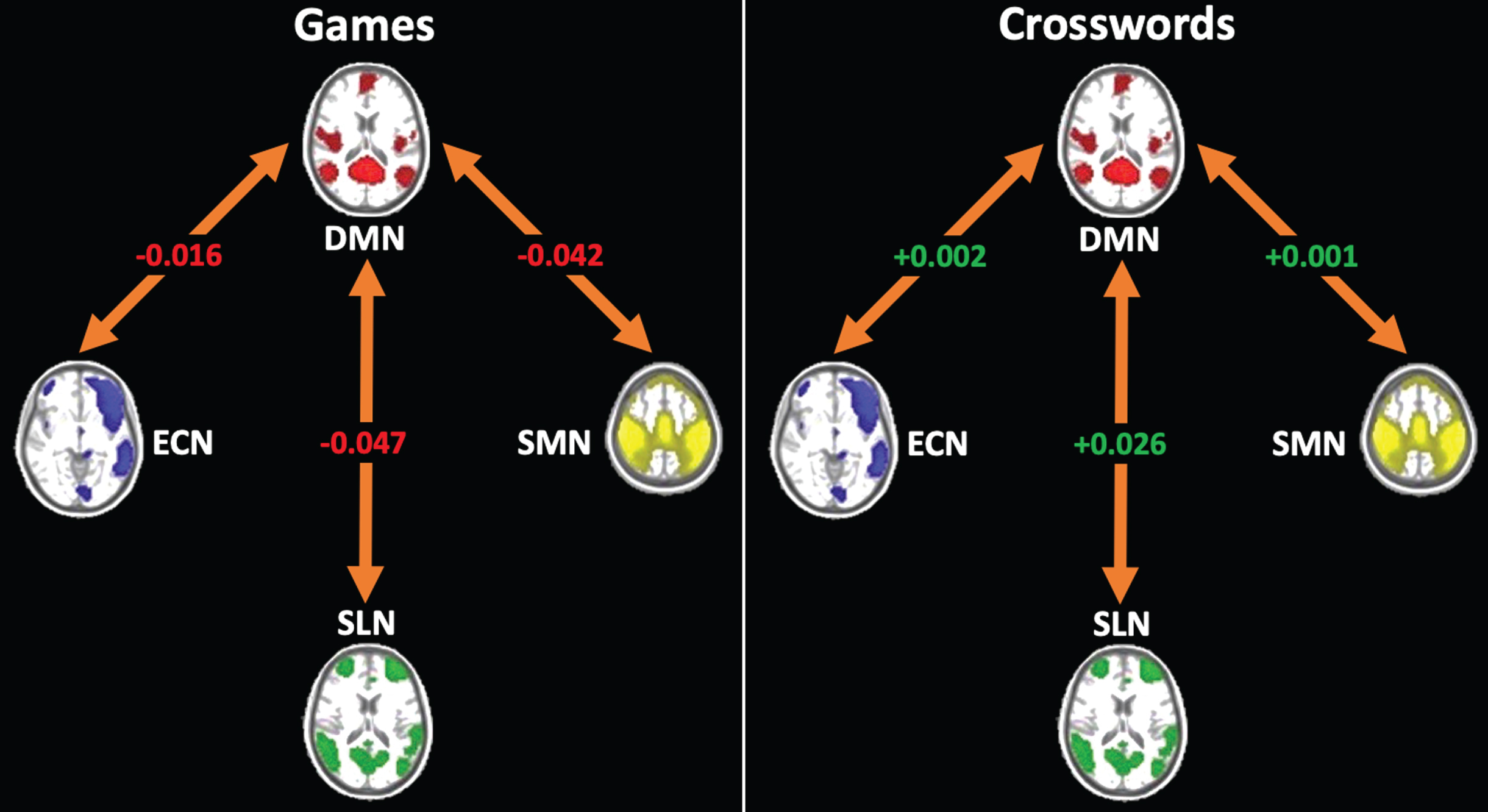
Table 3
Change from Baseline in Between-Network Connectivity (78 weeks – baseline)
Adjusted for change in FD and baseline value | ||||
Games | Crosswords | Diff | p | |
DMNECN_change | –0.012 (0.034) | –0.001 (0.030) | –0.011 (0.046) | 0.805 |
DMNSLN_change | –0.061 (0.036) | 0.036 (0.032) | –0.097 (0.048) | 0.045 |
DMNSMN_change | –0.043 (0.034) | 0.001 (0.030) | –0.044 (0.045) | 0.335 |
Correlation of change of DMN connectivity with change in cognition
There was no significant correlation in between- or within-network DMN changes and cognitive changes (measured by the ADAS-Cog) in either MCI subgroup, or both groups combined, for either the games or crossword conditions (p = NS).
DISCUSSION
We report results from the first randomized controlled trial in MCI subjects examining the effects of CCT versus an active control on within- and between-network connectivity in the DMN, and how these effects correlate with cognitive change. Across all subjects, we detected no treatment-related differences in within-network connectivity involving the DMN or other networks. The subgroup analysis, however, suggests that games and crosswords have different effects on the within-network connectivity of the DMN in subjects with LMCI. Specifically, DMN within-network FC increased with the crossword control intervention but decreased with games. Similarly, across all subjects, between-network connectivity involving the DMN and SLN increased with the crossword control intervention but decreased with games. Because the primary outcome of this trial was within-network DMN FC, we did not adjust p-values for multiple comparisons; therefore, these secondary findings should be viewed as preliminary. Though no direct correlation between change in DMN FC and cognitive outcomes was demonstrated, these exploratory findings nevertheless support the overall trial findings in which the primary cognitive outcome measure, Alzheimer’s Disease Assessment Scale-Cognitive Subscale (ADAS-Cog) 11, showed a small, but significant, decline for games compared to improvement for crosswords within the LMCI group as well as across all subjects [12]. However, within the EMCI group, there was no difference between crosswords and games in any cognitive or functional outcomes [12].
The reason for improvements in both cognitive measures and DMN FC with crosswords, as opposed to games, is unknown. One possible reason is a slightly higher proportion of ApoE4 carriers in the games group (Table 1) which could have biased the group toward faster decline. A second reason might be the active control condition. The use of an active control group (crossword puzzle solving) was designed to account for engagement of subjects without targeting any particular cognitive domain. Crossword puzzles, however, might nonspecifically engage multiple specialized cognitive networks at a low level, nevertheless synergistically, resulting in cognitively beneficial remodeling of the DMN. In subjects with LMCI, this may exceed that of more domain-specific types of training characteristic of CCT games. Indeed, previous studies support the investigation of crossword puzzles as a cognitive enhancing strategy in a clinical trial [26, 27]. Crossword puzzles require the use of several cognitive abilities: retrieval memory, verbal knowledge, language skills, attention, processing speed, and executive function. Simultaneous engagement of these abilities may have durable cognitive benefits [26]. Another potential reason for these findings is differences in engagement between the interventions. High levels of participation, in the absence of target engagement, have been reported in failed CCT trials in other conditions such as the schizophrenia eCaesar trial. It is possible that subjects were more compliant and engaged with the crossword intervention due to its familiarity. Although dwell time was monitored in all subjects to assure that they were spending 30 min in the training sessions, we did not analyze their actual levels of engagement. Indeed, crossword puzzles are considered a cognitive leisure activity, and decreased perceived stress levels of the activity may increase compliance [28].
Comparison of our study with prior studies is difficult for several reasons, including differences in the type of MCI studied, nature of both the CCT and control interventions, post-invention intervals of evaluation, and fMRI analysis methodology. Accordingly, studies looking at the effects of CCT on FC within the DMN in MCI subjects have shown mixed results [29–32, 37]. Of the randomized controlled trials, one notable trial of 26 weeks duration in 100 MCI subjects, included multidomain CCT for 3 h per week. Results revealed a decrease in DMN FC in the training groups. Specifically, the posterior cingulate cortex, the principal hub of the DMN, showed significant decreases in resting-state FC with the superior frontal lobe and anterior cingulate cortex hubs [29]. These decreases did not correlate with changes in ADAS-Cog scores, however. It should also be noted that our findings may be specific to the type of CCT we employed in our study and may not necessarily generalize to other types of CCT which engage the brain differently.
The strengths of the current work include the randomized controlled design with an active control condition, crossword puzzles, across two centers, stratified evenly by both EMCI and LMCI subjects. Although no differential effects of the interventions on the DMN or related networks were demonstrated across the entire study population, we did not test against a no-intervention condition. The purpose of this study was to assess whether specific domain training is superior to non-specific training on a complex task that simultaneously involves multiple domains. Secondly, the resting-state FC component of this study was conducted with a priori regions for the DMN, SN and ECNs and the metric for within-network connectivity was obtained by taking an average across connections between all major hubs of the networks, rather than testing separate connections within and between the networks individually. This distinguishes this study from those prior with similar designs. Thus, this study is unique in several aspects. First, it was the first of its kind to use pre-defined metrics of within- and between-network connectivity rather than voxelwise clusters or arbitrary hub-to-hub connections in an exploratory fashion. This hypothesis-driven, rather than hypothesis-generating, approach is not common to fMRI studies and this may explain why many previous findings are neither convergent nor reproducible. Indeed, though this hypothesis-driven study was negative on its primary outcome measure, within-network DMN FC, across all subjects, the findings in LMCI suggest that further work may confirm treatment-related network alterations in this more severely affected subgroup. Second, this was the first study of its kind to separate EMCI, many of whom do not convert to dementia and revert to normal cognition on testing, from LMCI, an established at-risk condition for AD. Third, this study included a high percentage of minority subjects, up to 30%, including African Americans, adding to the generalizability the results in a community setting.
We acknowledge that the beneficial effects of crosswords on cognition found in the overall trial [12] were not reflected in the primary outcome fMRI measure across the entire sample. This could be due to timing of maximal effects of the intervention. It may be that maximal effects of crosswords interventions on the DMN and related networks occur at the end of the more intensive phase of training, and dissipated over the 18-month duration of the trial, given that subjects were only scanned at entry and exit. Another potential cause could be technical factors. For example, it is possible that fMRI does not have sufficient fidelity in its present state to serve as a sensitive biomarker of subtle clinical changes, particularly in less impaired subjects, such as those with EMCI, while still being able to detect the changes in LMCI. Despite significant efforts to harmonize MRI protocols across institutions and scanners before the trial (Supplementary Table 1), as well as covarying for frame displacement in the analysis, software upgrades and other differences among scanners may have contributed to bias and loss of precision in fMRI metrics. Indeed, emerging plasma biomarkers of AD pathological hallmarks may offer more efficacy in the realm of surrogate outcomes of cognitive decline [36].
In conclusion, this randomized controlled fMRI study in MCI subjects revealed no treatment-related differences between crosswords and games in the primary outcome measure, within-network DMN FC. However, between network and subgroup analysis suggest differential effects on between network connectivity involving the DMN and SLN, and within-network connectivity of the DMN in subjects with LMCI. Crossword puzzles might nonspecifically, but synergistically, engage multiple specialized cognitive networks at a low level, resulting in cognitively beneficial remodeling between the DMN and other networks, and within the DMN in subjects at higher risk of dementia. These exploratory findings need to be confirmed in a hypothesis-driven manner.
ACKNOWLEDGMENTS
We thank all the participants and their informants in the trial. We thank the study staff for their assistance in data collection and conduct of the study. We thank Dr. William McDonald of Emory University, Dr. Anand Kumar of University of Illinois, and Dr. Stephen Rapp of Wake Forest University for their valuable oversight as members of the Data Safety and Monitoring Board. We thank Lumos Labs for providing the computerized platform for the interventions in this trial.
This work is supported by National Institute on Aging NIH grant number 1R01AG052440. Lumos Labs provided the gaming platform at no cost and were not involved in the final design, analyses, or drafting of the manuscript.
Authors’ disclosures available online (https://www.j-alz.com/manuscript-disclosures/22-0946r1).
SUPPLEMENTARY MATERIAL
[1] The supplementary material is available in the electronic version of this article: https://dx.doi.org/10.3233/JAD-220946.
REFERENCES
[1] | Petersen RC ((2004) ) Mild cognitive impairment as a diagnostic entry. J Intern Med 256: , 183–194. |
[2] | Grundman M , Petersen RC , Ferris SH , Thomas RG , Aisen PS , Bennett DA , Foster NL , Jack J , Clifford R , Galasko DR , Doody R , Kaye J , Sano M , Mohs R , Gauthier S , Kim HT , Jin S , Schultz AN , Schafer K , Mulnard R ((2004) ) Mild cognitive impairment can be distinguished from Alzheimer’s disease and normal aging for clinical trials. Arch Neurol 61: , 59–66. |
[3] | Aisen PS , Petersen RC , Donohue MC , Gamst A , Thomas RG , Walter S , Trojanowski JQ , Shaw LM , Jack CR Jr , Jagust W , Toga AW , Saykin AJ , Morris JC , Green RC , Weiner MW , Alzheimer’s Disease Neuroimaging Initiative ((2010) ) Clinical core of the Alzheimer’s Disease Neuroimaging Initiative: Progress and plans. Alzheimers Dement 6: , 239–246. |
[4] | Valenzuela MJ , Sachdev P ((2006) ) Brain reserve and dementia: A systematic review. Psychol Med 36: , 441–454. |
[5] | Hill NTM , Mowszowski L , Naismith SL , Chadwick VL , Valenzuela M , Lampit A ((2017) ) Computerized cognitive training in older adults with mild cognitive impairment or dementia: A systematic review and meta-analysis. Am J Psychiatry 174: , 329–340. |
[6] | Brehmer Y , Rieckmann A , Bellander M , Westerberg H , Fischer H , Backman L ((2011) ) Neural correlates of training-related working-memory gains in old age. Neuroimage 58: , 1110–1120. |
[7] | Heinzel S , Lorenz RC , Pelz P , Heinz A , Walter H , Kathmann N , Rapp MA , Stelzel C ((2016) ) Neural correlates of training and transfer effects in working memory in older adults. Neuroimage 134: , 236–249. |
[8] | Belleville S , Mellah S , De Boysson C , Demonet J , Bier B ((2014) ) The pattern and loci of training-induced brain changes in healthy older adults are predicted by the nature of the intervention. PloS One 9: , 102710. |
[9] | Kim H , Chey J , Lee S ((2017) ) Effects of multicomponent training of cognitive control on cognitive function and brain activation in older adults. Neurosci Res 124: , 8–15. |
[10] | Petrella JR , Sheldon FC , Prince SE , Calhoun VD , Doraiswamy PM ((2011) ) Default mode network connectivity in stable vs progressive mild cognitive impairment. Neurology 76: , 511–517. |
[11] | Petrella JR , Prince SE , Wang L , Hellegers C , Doraiswamy PM ((2007) ) Prognostic value of posteromedial cortex deactivation in mild cognitive impairment. PloS One 2: , 1104. |
[12] | Devanand DP , Goldberg TE , Qian M , Rushia SN , Sneed JR , Andrews HF , Nino I , Phillips J , Pence ST , Linares AR , Hellegers CA , Michael AM , Kerner NA , Petrella JR , Doraiswamy PM ((2022) ) Computerized games versus crosswords training in mild cognitive impairment. NEJM Evid, doi: 10.1056/EVIDoa2200121 |
[13] | D’Antonio J , Simon-Pearson L , Goldberg T , Sneed JR , Rushia SN , Kerner NA , Andrews AM , Hellegers CA , Tolbert S , Perea E , Petrella JR , Doraiswamy PM ((2019) ) Cognitive training and neuroplasticity in mild cognitive impairment (COG-IT): Protocol for a two-site, blinded, randomised, controlled treatment trial. BMJ Open 9: , e028536. |
[14] | Dai W , Lopez OL , Carmichael OT , Becker JT , Kuller LH , Gach HM ((2009) ) Mild cognitive impairment and Alzheimer disease: Patterns of altered cerebral blood flow at MR imaging. Radiology 250: , 856–866. |
[15] | Satterthwaite TD , Elliott MA , Gerraty RT , Ruparel K , Loughead J , Calkins ME , Eickhoff SB , Hakonarson H , Gur RC , Gur RE , Wolf DH ((2013) ) An improved framework for confound regression and filtering for control of motion artifact in the preprocessing of resting-state functional connectivity data. Neuroimage 64: , 240–256. |
[16] | Shirer WR , Jiang H , Price CM , Shirer WR , Jiang H , Price CM , Ng B , Greicius MD ((2015) ) Optimization of rs-fMRI pre-processing for enhanced signal-noise separation, test-retest reliability, and group discrimination. Neuroimage 117: , 67–79. |
[17] | Ashburner J , Friston KJ ((2000) ) Voxel-based morphometry—the methods. Neuroimage 11: , 805–821. |
[18] | Ashburner J , Barnes G , Chen C-C ((2021) ), SPM12 Manual 2: , 525. |
[19] | Hedden T , Van Dijk KR , Becker JA , Mehta A , Sperling RA , Johnson KA , Buckner RL ((2009) ) Disruption of functional connectivity in clinically normal older adults harboring amyloid burden. J Neurosci 29: , 12686–12694. |
[20] | Chand GB , Wu J , Hajjar I , Qiu D ((2017) ) Interactions of the salience network and its subsystems with the default-mode and the central-executive networks in normal aging and mild cognitive impairment. Brain Connect 7: , 401–412. |
[21] | Chen H , Li Y , Liu Q , Shi Q , Wang J , Shen H , Chen X , Ma J , Ai L , Zhang YM ((2019) ) Abnormal interactions of the salience network, central executive network, and default-mode network in patients with different cognitive impairment loads caused by leukoaraiosis. Front Neural Circuits 13: , 42. |
[22] | Doucet GE , Labache L , Thompson PM , Joliot M , Frangou S , Alzheimer’s Disease Neuroimaging Initiative ((2021) ) Atlas55+: Brain functional atlas of resting-state networks for late adulthood. Cereb Cortex 31: , 1719–1731. |
[23] | Calhoun VD , Adali T , Pearlson GD , Pekar JJ ((2002) ) A method for making group inferences from functional MRI data using independent component analysis. Hum Brain Mapp 16: , 140–151. |
[24] | Laird AR , Fox PM , Eickhoff SB , Turner JA , Ray KL , McKay DR , Glahn DC , Beckmann CF , Smith SM , Fox PT ((2011) ) Behavioral interpretations of intrinsic connectivity networks. J Cogn Neurosci 23: , 4022–4037. |
[25] | Desikan RS , Ségonne F , Fischl B , Quinn BT , Dickerson BC , Blacker D , Buckner RL , Dale AM , Maguire RP , Hyman BT , Albert MS , Killiany RJ ((2006) ) An automated labeling system for subdividing the human cerebral cortex on MRI scans into gyral based regions of interest. Neuroimage 31: , 968–980. |
[26] | Pillai JA , Hall CB , Dickson DW , Buschke H , Lipton RB , Verghese J ((2011) ) Association of crossword puzzle participation with memory decline in persons who develop dementia. J Int Neuropsychol Soc 17: , 1006–1013. |
[27] | Pressler SJ , Jung M , Gradus-Pizlo I , Titler MG , Smith DG , Gao S , Lake KR , Burney H , Clark DG , Wierenga KL , Dorsey SG , Giordani B ((2022) ) Randomized controlled trial of a cognitive intervention to improve memory in heart failure. J Card Fail 28: , 519–530. |
[28] | Lizuka A , Suzuki H , Ogawa S , Kobayashi-Cuya KE , Kobayashi M , Takebayashi T , Fujiwara Y ((2019) ) Can cognitive leisure activity prevent cognitive decline in older adults? A systematic review of intervention studies. Geriatr Gerontol Int 19: , 469–482. |
[29] | Suo C , Singh MF , Gates N , Wen W , Sachdev P , Brodaty H , Saigal N , Wilson GC , Meiklejohn J , Singh N , Baune BT , Baker M , Foroughi N , Wang Y , Mavros Y , Lampit A , Leung I , Valenzuela MJ ((2016) ) Therapeutically relevant structural and functional mechanisms triggered by physical and cognitive exercise. Mol Psychiatry 21: , 1633–1642. |
[30] | Barban F , Mancini M , Cercignani M , Adriano F , Perri R , Annicchiarico R , Carlesimo GA , Ricci C , Lombardi MG , Teodonno V , Serra L , Giulietti G , Fadda L , Federici A , Caltagirone C , Bozzali M ((2017) ) A pilot study on brain plasticity of functional connectivity modulated by cognitive training in mild Alzheimer’s disease and mild cognitive impairment. Brain Sci 7: , 50. |
[31] | Kim S , Park E , Cha H , Jung J , Jung T , Chang Y ((2020) ) Effects of cognitive training in mild cognitive impairment measured by resting state functional imaging. Behav Sci 10: , 175. |
[32] | Tang Y , Xing Y , Zhu Z , He Y , Li F , Yang J , Liu Q , Li F , Teipel SJ , Zhao G , Jia J ((2019) ) The effects of 7-week cognitive training in patients with vascular cognitive impairment, no dementia (the Cog-VACCINE study): A randomized controlled trial. . Alzheimers Dement 15: , 605–614. |
[33] | Ten Brinke LF , Davis JC , Barha CK , Liu-Ambrose T ((2017) ) Effects of computerized cognitive training on neuroimaging outcomes in older adults: A systematic review. BMC Geriatr 17: , 139. |
[34] | Lampit A , Hallock H , Suo C , Naismith SL , Valenzuela M. ((2015) ) Cognitive training-induced short-term functional and long-term structural plastic change is related to gains in global cognition in healthy older adults: A pilot study. Front Aging Neurosci 7: , 14. |
[35] | Cao W , Cao X , Hou C , Li T , Cheng Y , Jiang L , Luo C , Li C , Yao D ((2016) ) Effects of cognitive training on resting-state functional connectivity of default mode, salience, and central executive networks. Neurosci 8: , 70. |
[36] | Cullen NC , Leuzy A , Janelidze S , Palmqvist S , Svenningsson AL , Stomrud E , Dage JL , Mattsson-Carlgren N , Hansson O ((2021) ) Plasma biomarkers of Alzheimer’s Disease improve prediction of cognitive decline in cognitively unimpaired elderly populations. Nat Commun 12: , 3555. |
[37] | Hampstead BM , Stringer AY , Iordan AD , Ploutz-Snyder R , Sathian K ((2022) ) Toward rational use of cognitive training in those with mild cognitive impairment. Alzheimers Dement, doi: 10.1002/alz.12718. |