A Precision Medicine Approach to Treating Alzheimer’s Disease Using Rosiglitazone Therapy: A Biomarker Analysis of the REFLECT Trials
Abstract
Background:
The REFLECT trials were conducted to examine the treatment of mild-to-moderate Alzheimer’s disease utilizing a peroxisome proliferator-activated receptor gamma agonist.
Objective:
To generate a predictive biomarker indicative of positive treatment response using samples from the previously conducted REFLECT trials.
Methods:
Data were analyzed on 360 participants spanning multiple negative REFLECT trials, which included treatment with rosiglitazone and rosiglitazone XR. Support vector machine analyses were conducted to generate a predictive biomarker profile.
Results:
A pre-defined 6-protein predictive biomarker (IL6, IL10, CRP, TNFα, FABP-3, and PPY) correctly classified treatment response with 100%accuracy across study arms for REFLECT Phase II trial (AVA100193) and multiple Phase III trials (AVA105640, AV102672, and AVA102670). When the data was combined across all rosiglitazone trial arms, a global RSG-predictive biomarker with the same 6-protein predictive biomarker was able to accurately classify 98%of treatment responders.
Conclusion:
A predictive biomarker comprising of metabolic and inflammatory markers was highly accurate in identifying those patients most likely to experience positive treatment response across the REFLECT trials. This study provides additional proof-of-concept that a predictive biomarker can be utilized to help with screening and predicting treatment response, which holds tremendous benefit for clinical trials.
INTRODUCTION
Alzheimer’s disease (AD) is the most common neurodegenerative dementia. More than 5.7 million Americans suffer from this devastating disease [1]. Every 65 seconds, an American develops AD, which is the 5th leading cause of death for those over the age of 65 [2]. AD has an annual healthcare cost similar to that of cardiovascular disease (CVD) and more than that of cancer [3]. While death rates due to CVD and cancer have declined in recent decades, death rates due to AD have steadily increased [1] likely due to ineffective therapies. It is our hypothesis that AD is a heterogeneous condition and, therefore, a paradigm shift is required to identify specific subpopulations for targeted—precision medicine [4] —interventions [5–7]. In fact, the complexity of AD may be the very key to addressing this devastating disease.
In fact, biomarker guided therapies in oncology have resulted in drastically improved patient outcomes [4, 8]. A precision medicine approach has been proposed for numerous diseases [9–11], including neurological diseases such as multiple sclerosis [12, 13] and AD [5, 6, 14]. Despite the proposed use of precision medicine for AD, few studies to date have provided direct empirical support. By leveraging previously conducted clinical trial biorepositories, it is possible to provide proof-of-concept data for the precision medicine approach in AD [14, 15]. In fact, we have previously utilized stored samples to demonstrate the utility of a precision medicine approach to treating AD using NSAIDs therapy [14].
The FDA defines a “Predictive Biomarker” as “a biomarker used to identify individuals who are more likely than similar patients without the biomarker to experience a favorable or unfavorable effect from a specific intervention or exposure [16].” It is our view that the failure of clinical trials targeting AD is due to the fact that “most medical treatments are designed for the ‘average patient’; as a one-size-fits-all approach” [4]. This approach does not consider the substantial biological heterogeneity among patients [5, 6]. As seen in Fig. 1, we hypothesize that there are multiple subgroups of patients within the larger AD patient population. Therefore, if “Treatment A” was appropriate and effective in only 20%of the population (or 1 subgroup), the clinical trial was doomed to fail as 80%of the patients selected were inappro-priate. However, targeting that specific 20%of pat-ients based on his/her biological dysfunction driving his/her dementia, optimal treatment outcomes can be seen that may have been impossible to find due to the trial design itself [14]. Predictive biomarkers can be used to only enroll the specific group of patients most likely to benefit from the trial-specific intervention.
Fig. 1
Precision medicine approach to trial enrollment with predictive biomarkers.
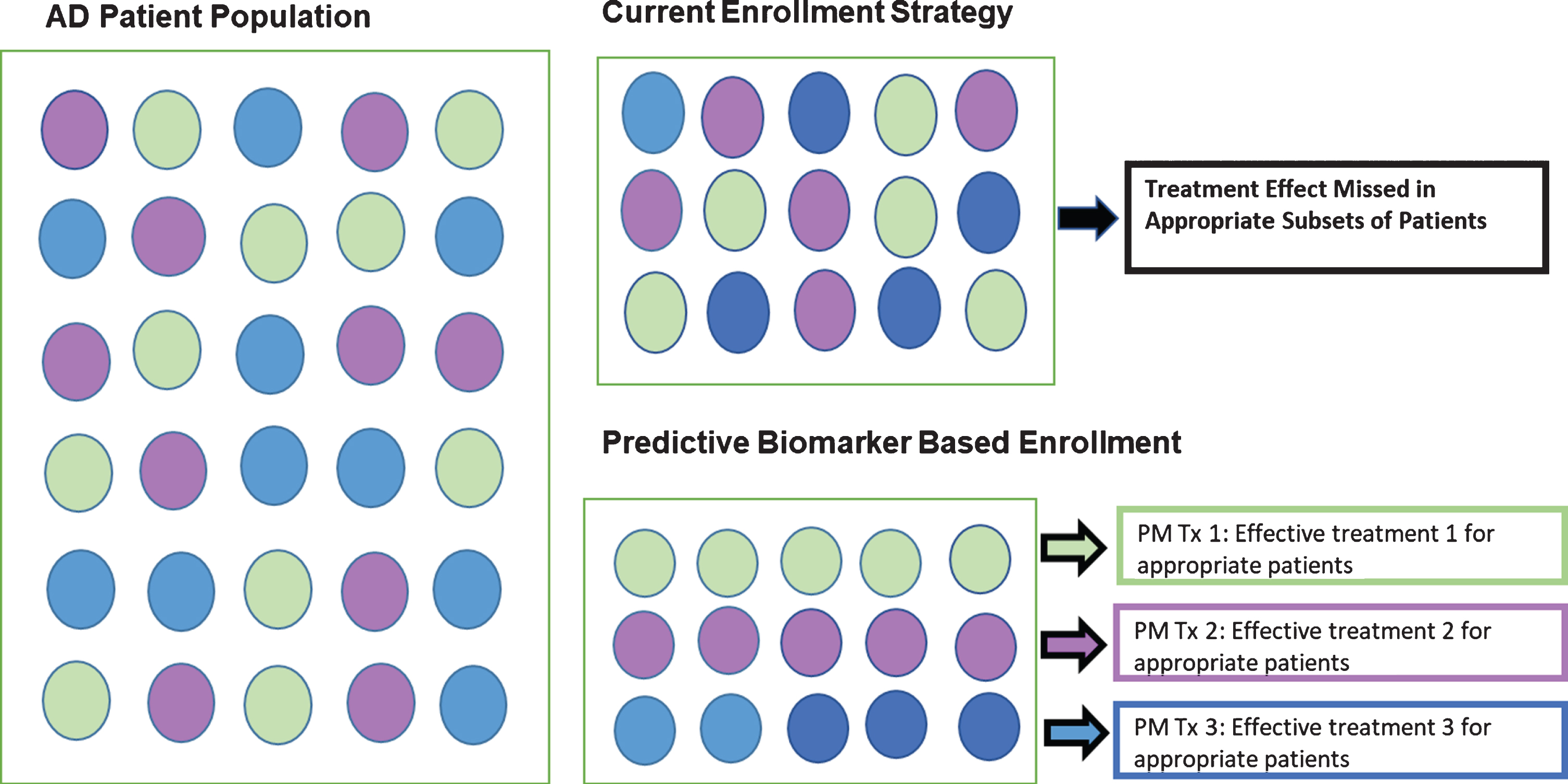
There is substantial literature linking diabetes and AD. In clinic samples, AD cases have been shown to have higher blood glucose levels [17], and diabetics with AD have been found to have increased rates of decline [18] as well as significantly greater cortical atrophy than non-diabetic AD cases [19]. Among epidemiological studies, the increased risk for AD and cognitive dysfunction among those with diabetes has been shown in the Rotterdam Study [20, 21], the Canadian Study of Health and Aging [22], Framingham Heart Study [23], the Washington Heights Inwood Columbia Aging Project (WHICAP) [24], the Honolulu-Asia Aging Study [25], the Religious Orders Study [26] and the Sacramento Area Latino Study on Aging (SALSA) [27]. Based on these findings, numerous clinical trials have been undertaken to treat or prevent AD with anti-diabetic medications.
Peroxisome proliferator-activated receptor gamma (PPARγ) agonist are widely used for treatment of diabetes. PPARγ agonists such as rosiglitazone modulate many cellular processes, including several ass-ociated with AD through its reduction of tau and amyloid pathology and inhibition of inflammation [28–30]. Rosiglitazone was examined in multiple trials (Phase II and Phase III) as a potential treatment for mild-to-moderate AD in the REFLECT trials [31, 32], but these clinical trials did not meet clinical endpoints. However, we hypothesize that the one-size-fits-all approach to the clinical trial design masked the therapeutic benefit experienced by a subset of patients. Therefore, we tested our previously generated methods [14] to create a predictive biomarker that identifies those specific AD patients that benefited from rosiglitazone therapy in the REFLECT trials.
METHODS
Participants and methods for REFLECT trials [31, 32]
The current study includes samples and data from multiple trials of rosiglitazone therapy in AD incl-uding a Phase IIb (NCT00334568) study of 2 mg, 4 mg, and 8 mg. Three REFLECT trials included multiple studies of 2 mg or 8 mg rosiglitazone XR as a potential therapy for mild-to-moderate AD. REFLECT-1 (AVA105640; NCT00428090) was a 24-week, double-blind, double-dummy, randomized, parallel-group Phase III study. REFLECT-5 (AVA102677; NCT00550420) open-label extension of REFLECT-1, REFLECT-2 (Study AVA10267, NCT00348309) and REFLECT-3 (study AVA102670; NCT00348140), was a 52-week, randomized, doub-le-blind, placebo-controlled, parallel-group study of rosiglitazone XR as an adjunctive therapy to ongoing acetylcholinesterase inhibitor (AChEI) treatment for 48 weeks. Participants who completed either study could then enroll into the open-label extension REFLECT-4 study for longer-term treatment. The sample size randomized per trial were as follows: REFLECT-1 n = 581, REFLECT-2 n = 1,496, and REFLECT-3 n = 1,485. The samples and data from these trials were provided to the ADCS for academic research use and utilized for the purposes of this study. All clinical trials were conducted under IRB approved protocols and all patients or informants provided written informed consent. Due to funding limitations, only subsets of samples were assayed from each of the trials.
Participant screening criteria for the REFLECT trials
Inclusion criteria
Age 50–90 years with a diagnosis of mild-to-moderate AD according to NINDS-ADRDA criteria [33], Mini-Mental Status Examination (MMSE) score between 10–26 at screening and at least 6-months of ongoing donepezil or other approved AChEI therapy with stable dosing for at least 2 months prior to enrollment.
Exclusion criteria
Vascular dementia diagnosis; history or evidence of another cause of dementia; history of seizures; history of congestive heart failure; significant psychiatric illness that in the opinion of the investigator would interfere with the study; participants with controlled behavioral symptoms on stable doses of atypical antipsychotics, SSRIs, or anxiolytics were allowed; participants with untreated active major depressive disorder were excluded; type 1 and type 2 diabetes treated with insulin/PPARγ agonists/insulin secretagogues and agents with incretin effects were excluded; subjects with type 2 diabetes controlled by diet or exercise or metformin were allowed to enter the study if HbA1c < 8.5%at screening.
Proteomic assays
All blood biomarker assays were conducted in the Institute for Translational Research (ITR) Biomarker Core.
Sample preparation
Preparation of samples for proteomic assay was conducted using the Hamilton Robotics StarPlus system, which facilitates substantially improved quality of assays, increased QA/QC monitoring, as well as increased proteomic capacity in the laboratory. Any re-aliquoting was conducted via the Hamilton easyBlood robotic system.
Sample assay
Plasma samples were assayed via multi-plex bio-marker assay platform using electrochemiluminescence (ECL). All plasma samples were assayed for targeted markers of our proinflammatory endophenotype and metabolic endophenotype: C-reactive protein (CRP), interleukin (IL)-6, IL-10, tumor necrosis factor alpha (TNFα), fatty acid binding protein (FABP)-3, and pancreatic polypeptide (PPY). Additional markers were assayed as part of the ITR Biomarker Core standard panel. The ITR laboratory has assayed over n > 20,000 samples on these markers using this system. This set of six proteins was selected from our larger 21-protein panel that has been shown to be highly accurate in detecting AD; inter- and intra-assay variability has been excellent [34–36]. Average CVs (>3000 samples) for these assays are all < 10%with the majority being ≤5%.
Statistical analyses
The predictive biomarker profile was generated using support vector machine (SVM) analyses. SVM is based on the concept of decision planes that define decision boundaries and serves primarily as a classifier method by performing classification tasks through constructing hyperplanes in a multidimensional space that can separate cases of different class labels. SVM has the capacity to simultaneously take into account a large volume of data in order to generate an overall profile (e.g., over and under-expression of select proteins) that most accurately classifies multiple outcomes rather than only binary outcomes. As with all learning machine methods, a primary concern is for overfitting the data. In order to avoid this problem we: 1) restricted the number of proteins included in the predictive model to a total of six pre-specified inflammatory and metabolic markers each with a substantial literature linking them with AD and cognitive decline from our previously established larger blood-based profile [20, 37] and our previously published predictive biomarker [14]; 2) built the predictive biomarker based on responders versus non-responders (i.e., only 2 groups). Treatment responder was defined as an MMSE score that was stable or improved over trial duration whereas non-responder was defined as any decline in MMSE scores over the clinical trial duration. The goal of responder was to identify those who experience clinically meaningful outcomes rather than slowed decline. The purpose of this approach was to have a predictive biomarker that could selectively identify only those most likely to respond while all others would be ruled out; 3) conducted internal five-fold cross-validation within the sample with the SVM analyses. The SVM analyses were conducted with the e1071 package (v1.6–8) in R (v3.4.2). In order to build an SVM model to predict treatment response, the radial basis function kernel was used together with five-fold cross-validation, cost = 100 and gamma = 0.001. The original data was randomly partitioned into 5 equal sized subsamples. A single subsample was retained as a testing set while the rem-aining 4 subsamples were used as training sets. For each model, we ran the cross-validation randomly five times. The W weights of SVM in Libsvm when RBF kernel is used can be calculated by w = coef’* SVs. Then the decision values are calculated according to w’*x. And subsequently, the labels are predi-cted according to sign(w’*x + b) where b is some threshold. If the label is positive, it belongs to the positive class, if it is negative it belongs to the negative class. The absolute value of SVM weight W can be used to determine the importance of each feature. The closer to zero that the absolute W is, the less useful the corresponding feature is for separating the data. The higher the absolute W is, the more important the corresponding feature is for the SVM classifier.
Additionally, to avoid influence of outliers, all outliers beyond the fifth quintile were set at the fifth quintile. Finally, due to instability of assays at extremely low levels, any assay values below the standard curve were set at the least detectable limit for the assay. These approaches restricted any influence of outliers in any direction. SVM does not assume normality and, therefore, raw data were utilized. The analyses were restricted to rosiglitazone arms across trials as the goal was specifically to identify a predictive biomarker of treatment response. The SVM models were first generated by trial x arm and then by dosage combined across trials, where possible.
Of note, SVM was selected over other power classification algorithms, such as Random Forest, because of the objective for the classification tasks proposed in this study. SVM has been shown to perform better on specific datasets such as imaging and microarray data [38]. Therefore, SVM was the ideal choice for our protein microarray dataset, particularly as there was not mixture of numerical and categorical features for binary classification problems. Additionally, SVM was also the better choice for our data given that outliers were removed and missing values imputed prior to analysis. Lastly, SVM was the ideal classification algorithm choice for datasets with small sample sizes such as ours.
RESULTS
A total of 534 samples were assayed as part of this proof-of-concept study. Table 1 provide the descriptive statistics of the study population by clinical trial. First, the predictive biomarker was examined using our pre-specified inflammatory and metabolic markers, which included IL-6, IL-10, CRP, TNFα, FABP-3, and PPY. These markers were used to predict treatment response versus non-response (based on change in MMSE score) within each clinical trial.
Table 1
All patients randomized and patients who are responders and non-responders
S | Total | Responder | Non-responder |
All patients | |||
No. randomized | 534 | 251 | 283 |
Age percentiles | |||
50 | 73 | 73 | 73 |
25, 75 | 67, 78 | 67.0, 78.5 | 67.5, 78.0 |
0, 100 | 50, 90 | 50, 90 | 50, 90 |
Gender,% | |||
Female | 60.7 | 64.9 | 56.9 |
Male | 39.3 | 35.1 | 43.1 |
Total | Responder | Non-responder | |
2 mg RSG XR | |||
No. randomized | 99 | 34 | 65 |
Age percentiles | |||
50 | 73 | 74 | 73 |
25, 75 | 67.0, 78.5 | 67.0, 79.8 | 67, 78 |
0, 100 | 51, 89 | 55, 87 | 51, 89 |
Gender,% | |||
Female | 53.5 | 67.6 | 46.2 |
Male | 46.5 | 32.4 | 53.8 |
Total | Responder | Non-responder | |
8 mg RSG XR | |||
No. randomized | 116 | 54 | 62 |
Age percentiles | |||
50 | 74 | 75 | 73 |
25, 75 | 67.8, 78.2 | 68.2, 79.0 | 65.2, 78.0 |
0, 100 | 50, 86 | 50, 86 | 51, 85 |
Gender,% | |||
Female | 55.2 | 53.7 | 56.5 |
Male | 44.8 | 46.3 | 43.5 |
Total | Responder | Non-responder | |
Donepezil (10 mg) | |||
No. randomized | 21 | 15 | 6 |
Age percentiles | |||
50 | 74 | 74 | 77.5 |
25, 75 | 70, 80 | 70.5, 79.0 | 67.2, 81.8 |
0,100 | 59, 84 | 59, 84 | 62, 84 |
Gender,% | |||
Female | 66.7 | 66.7 | 66.7 |
Male | 33.3 | 33.3 | 33.3 |
Total | Responder | Non-responder | |
Placebo | |||
No. randomized | 153 | 63 | 90 |
Age percentiles | |||
50 | 74 | 73 | 75 |
25, 75 | 68, 79 | 67, 80 | 69.0, 78.8 |
0, 100 | 50, 90 | 52, 90 | 50, 90 |
Gender,% | |||
Female | 69.9 | 74.6 | 66.7 |
Male | 30.1 | 25.4 | 33.3 |
Total | Responder | Non-responder | |
RSG 2 mg | |||
No. randomized | 50 | 31 | 19 |
Age percentiles | |||
50 | 72 | 72 | 72 |
25, 75 | 67, 75 | 67.0, 75.5 | 68.5, 74.5 |
0, 100 | 50, 83 | 54, 83 | 50, 81 |
Gender,% | |||
Female | 64 | 64.5 | 63.2 |
Male | 36 | 35.5 | 36.8 |
Total | Responder | Non-responder | |
RSG 4 mg | |||
No. randomized | 52 | 28 | 24 |
Age percentiles | |||
50 | 72.5 | 70 | 73.5 |
25, 75 | 59.8, 76.2 | 58.8, 75.2 | 62.2, 77.5 |
0, 100 | 52, 83 | 52, 82 | 53, 83 |
Gender,% | |||
Female | 51.9 | 57.1 | 45.8 |
Male | 48.1 | 42.9 | 54.2 |
Total | Responder | Non-responder | |
RSG 8 mg | |||
No. randomized | 43 | 26 | 17 |
Age percentiles | |||
50 | 72 | 72 | 71 |
25, 75 | 66.0, 75.5 | 65.5, 75.0 | 68, 77 |
0, 100 | 53, 84 | 54, 83 | 53, 84 |
Gender,% | |||
Female | 62.8 | 69.2 | 52.9 |
Male | 37.2 | 30.8 | 47.1 |
In the Phase II trial (AVA100193), there was a total of 31 responders and 19 non-responders in the 2 mg arm, 28 responders and 24 non-responders in the 4 mg arm, and 26 responders and 17 non-responders in the 8 mg arm. Using our 6-protein algorithm, 100%of patients were correctly classified across study arms (Fig. 2).
Fig. 2
Predictive biomarker accuracy in predicting treatment response in the Phase 2 trial.
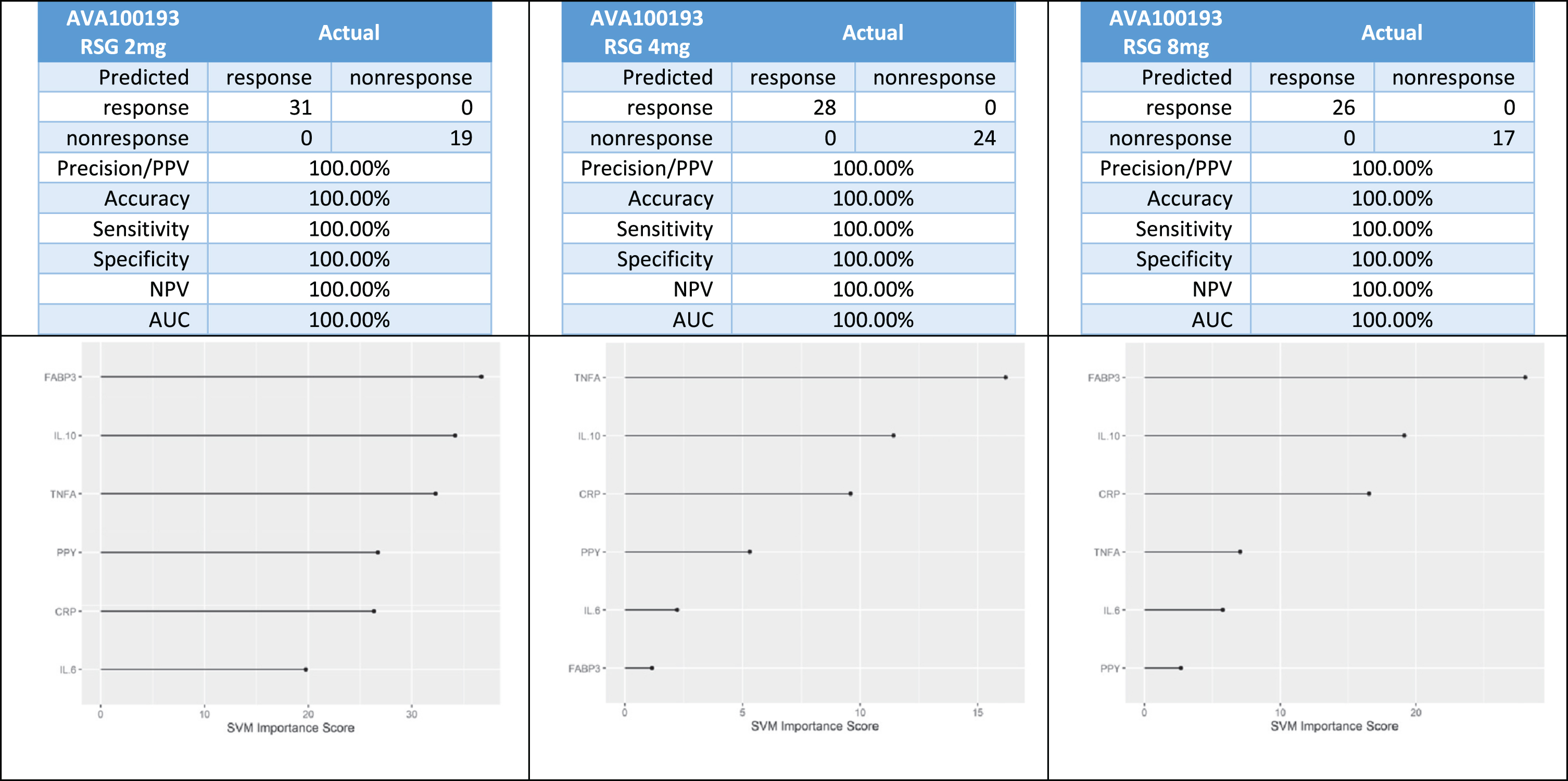
In the Phase III trial (AVA105640), there was 20 responders and 25 non-responders in the 2 mg XR group and 22 responders and 23 non-responders in the 8 mg XR group. Using the 6-protein predictive biomarker algorithm, 100%of the patients were correctly classified as responder or non-responder (Fig. 3).
Fig. 3
Predictive biomarker accuracy in identifying responders versus non-responders in the Phase 3 trial AV105640.
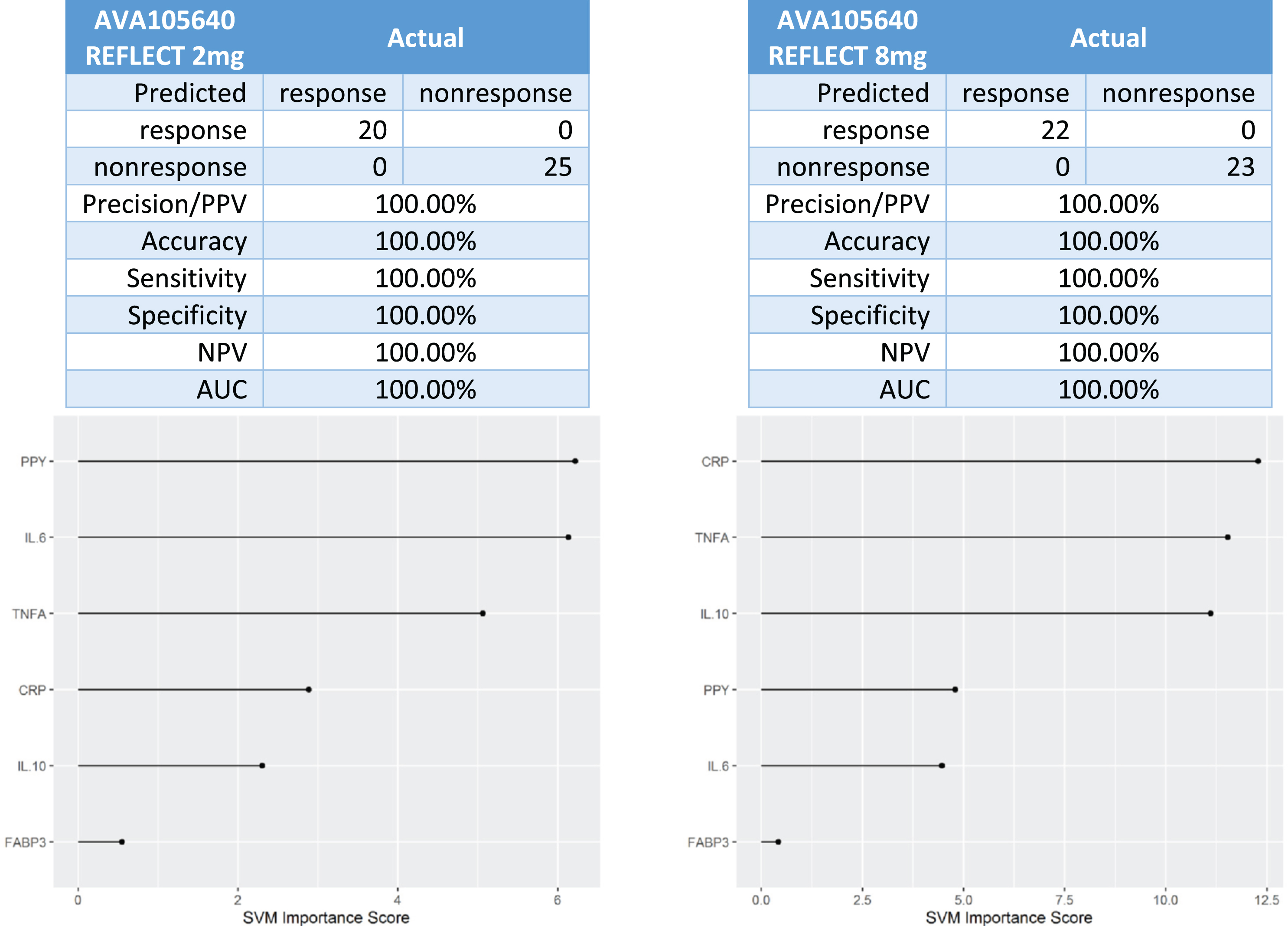
In the Phase III trial (AV102672), there was 7 responders and 17 non-responders in the 2 mg XR arm and 12 responders and 17 non-responders in the 8 mg XR arm. The 6-protein predictive biomarker algorithm was 100%accurate in identifying responders versus non-responders (Fig. 4).
Fig. 4
Predictive biomarker accuracy in identifying responders versus non-responders in the Phase 3 trial AV102672.
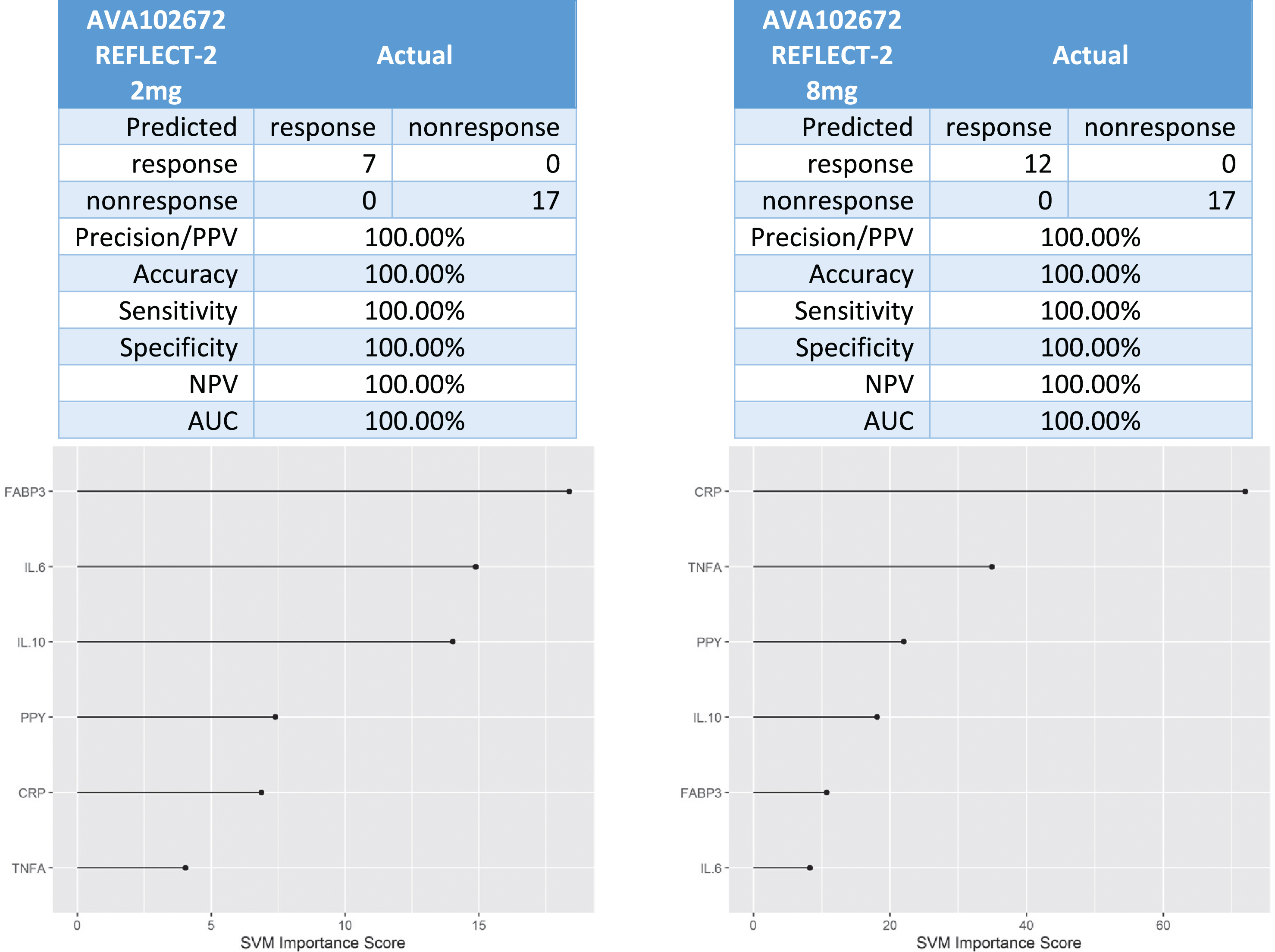
In the Phase III trial (AVA102670), there were 7 responders and 23 non-responders in the 2 mg XR arm and 20 responders and 22 non-responders in the 8 mg XR arm. The 6-protein predictive biomarker algorithm was 100%accurate in identifying responder versus non-responder (Fig. 5).
Fig. 5
Predictive biomarker accuracy in identifying responders versus non-responders in the Phase 3 trial AV102670.
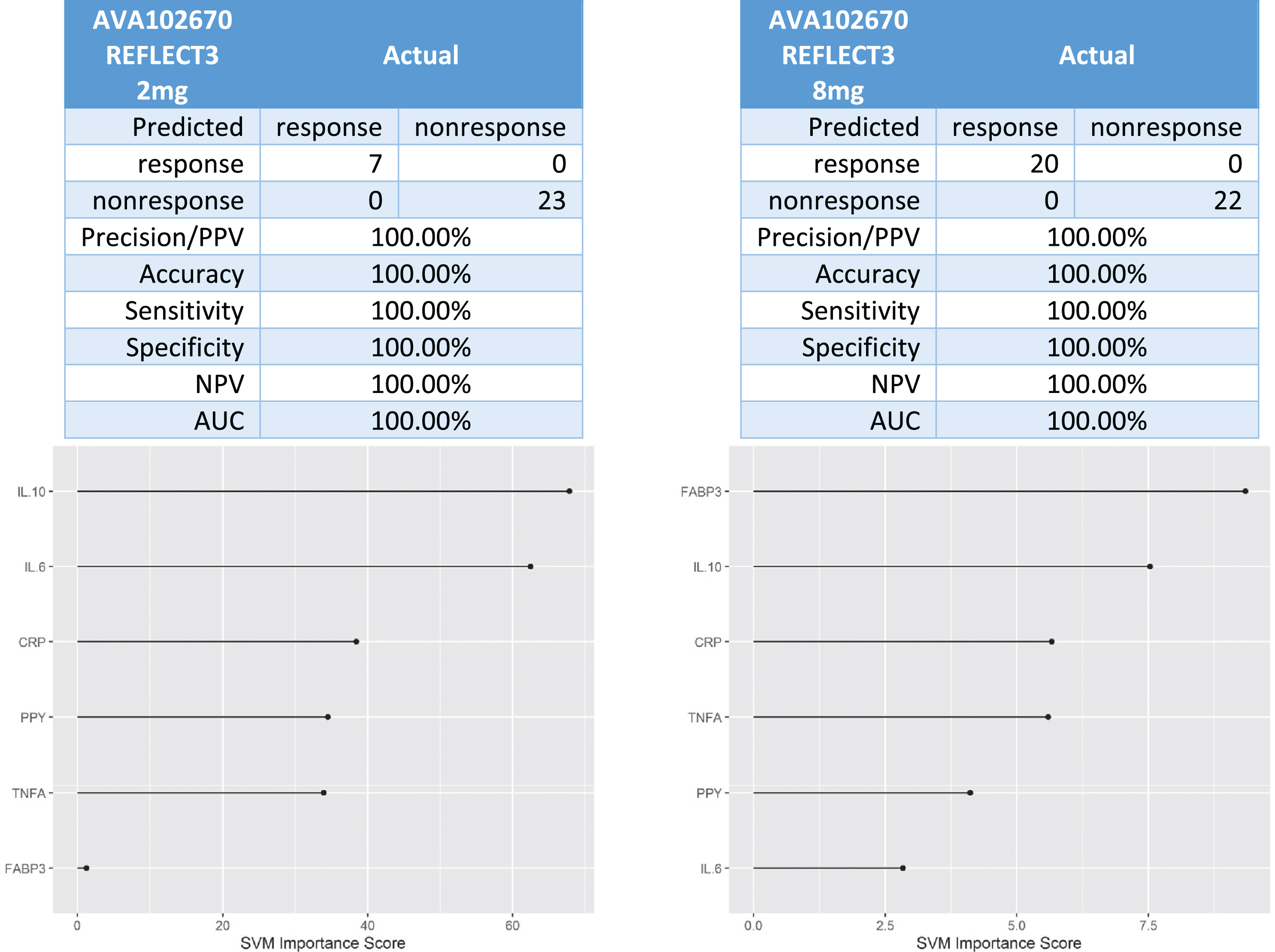
Next, data was combined across the 2 mg XR and 8 mg XR arms across trials. There were 34 responders and 65 non-responders in the 2 mg XR arm and 54 responders and 62 non-responders in the 8 mg XR arm. When the data was combined across these arms, the 6-protein predictive biomarker algorithm was again 100%accurate in identifying responders versus non-responders to rosiglitazone (Fig. 6).
Fig. 6
Predictive biomarker accuracy in identifying responders versus non-responders in across 2mg XR and 8mg XR arms across trials.
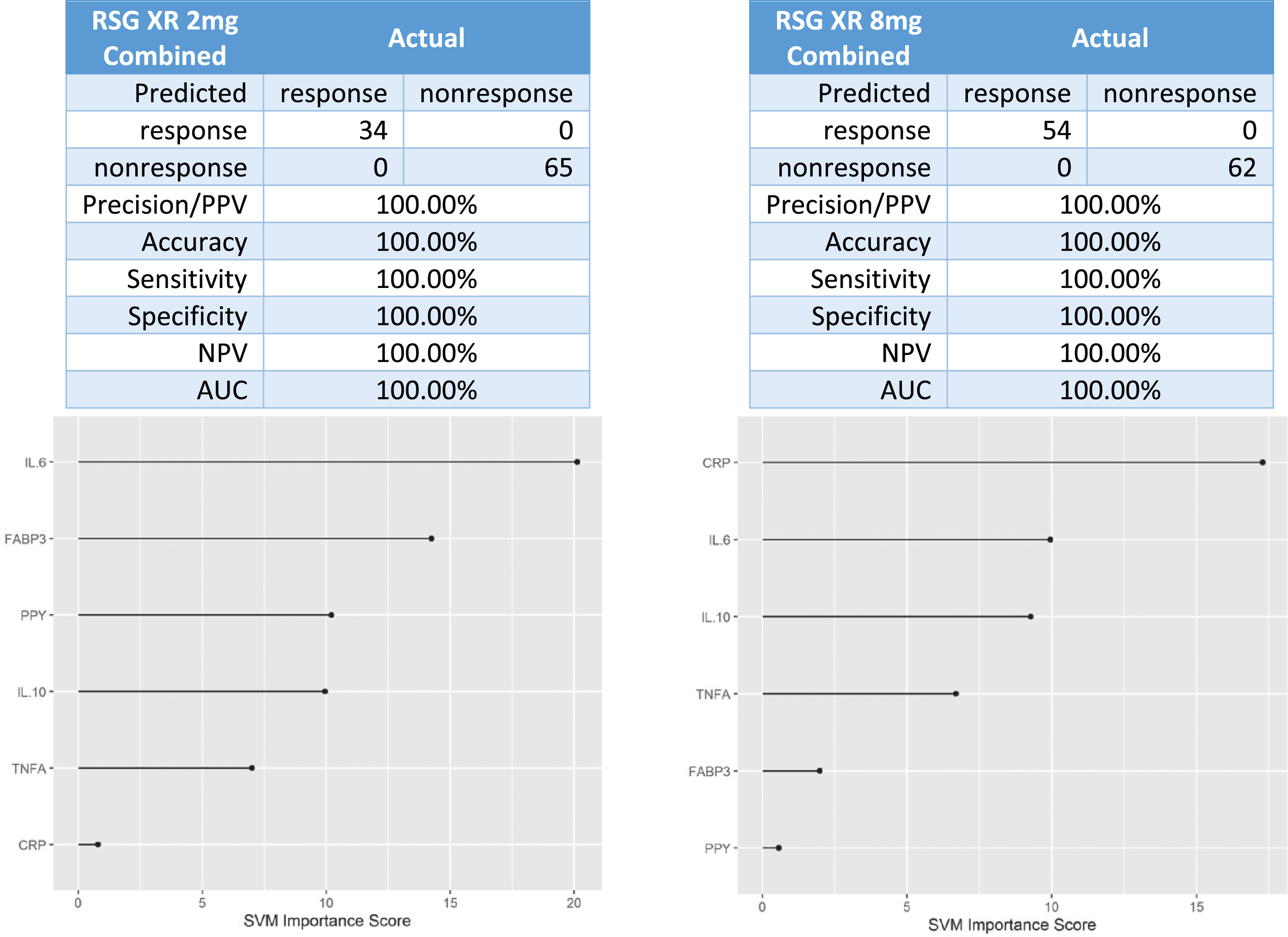
Finally, data was combined across all rosiglitazone therapy arms to determine if a global RSG-predictive biomarker could be generated. When combined acr-oss arms and trials, there were 173 responders and 187 non-responders. The 6-protein predictive biomarker was 98%accurate overall with 98%of treatment responders accurately classified (Fig. 7).
Fig. 7
Predictive accuracy in identifying responders versus non-responders dosages.
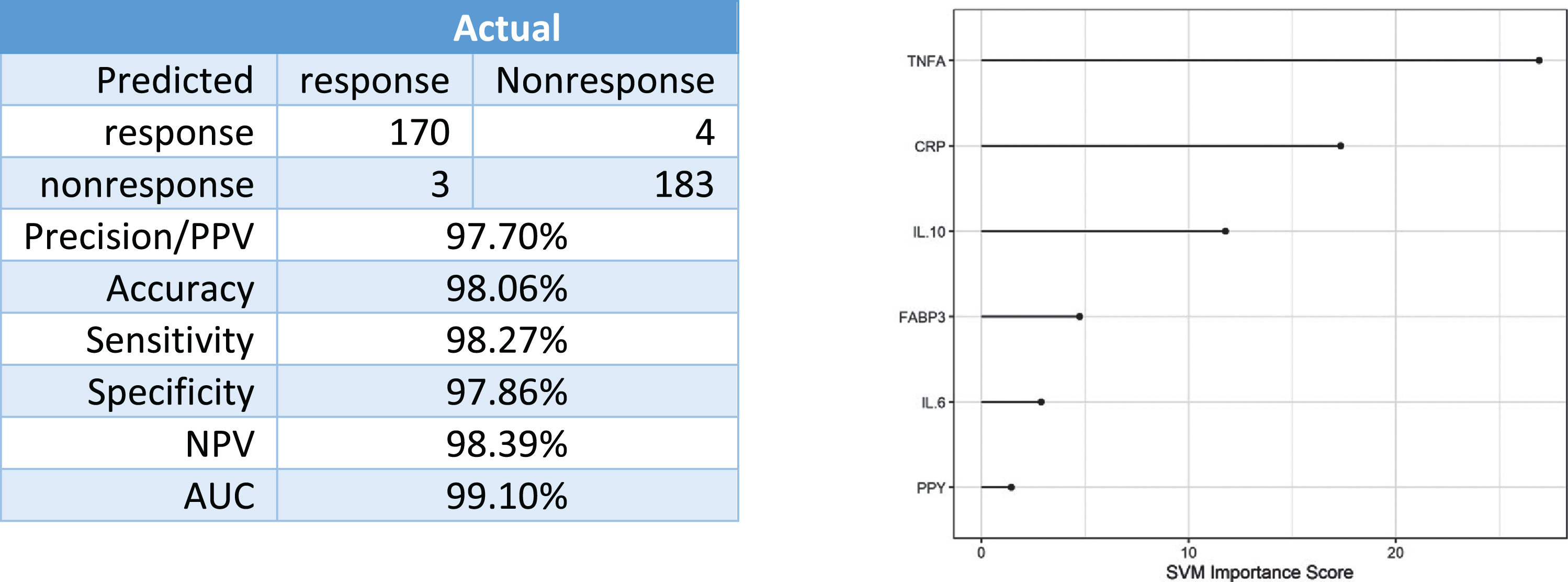
DISCUSSION
The current data suggests that a 6-protein algorithm consisting of markers covering inflammatory and metabolic pathways can be utilized to generate a predictive biomarker that can be used to identify those AD patients most likely to benefit from 2 mg, 4 mg, and 8 mg rosiglitazone therapy. Therefore, the current findings offer additional proof-of-concept support for a precision medicine approach to targeted treatment among specific subsets of patients suffering from AD. In fact, when combined with our prior work, we have now demonstrated two subgroups of patients that could be screened from the population of AD patients and enrolled into targeted therapeutic trials for optimal benefit from NSAID [14] or rosiglitazone therapies (Fig. 2).
As was shown in our prior work [14], a targeted panel of markers can be utilized to generate a predictive biomarker for the identification of specific subsets of AD patients who would most likely benefit from specified therapies. Previously, we analyzed data from the ADCS NSAID trial [39], a multicenter, randomized, double-blind, placebo-controlled parallel group trial with 1-year exposure to study medications. Individuals who met enrollment criteria with a diagnosis of probable AD in this trial were randomized to rofecoxib (25 mg once daily), naproxen (220 mg twice-daily), or placebo. In our study, the inflammatory-specific predictive biomarker was 97%accurate in identifying treatment response to naproxen and 98%accurate in identifying treatment response to rofecoxib.
In this study, we examined a specific a priori def-ined set of metabolic and inflammatory markers. Rosiglitazone has well-documented anti-inflamm-atory and neuroprotective qualities. In fact, rosiglitazone has been shown to modulate the inflammatory markers in our proinflammatory endophenotype [40–42]. Therefore, we hypothesized that combining both metabolic and inflammatory markers into the predictive biomarker would yield optimal success. Additional markers were assayed for further refinement; however, such markers did not need to be considered given the overall accuracy of the profile. Our team will continue to conduct supplementary analyses to determine if there is an optimal set of proteins to include in the RSG-predictive biomarker. Of note, our recent work has expanded the metabolic marker panel to include GLP-1, insulin, peptide YY, and glucagon with our data showing that these markers are predictive of a MRI-based marker of “neurodegeneration” from the AT(N) research framework [43], but only among Mexican Americans (data under review for publication). Combined, this data suggests that interventions targeting the metabolic pathway (such as rosiglitazone) may need to be ethnically-tailored as vascular factors were more predictive of N among non-Hispanic whites.
Ours is not the first study to identify potential biomarkers related to treatment response to rosiglitazone among AD patients. Akuffo and colleagues [44] examined plasma samples from 41 patients enrolled in the Phase IIb study of rosiglitazone. Protein expression was related directly to improvement in cognitive test performance on the ADAS-Cog. In that study, A2M, complement C1 inhibitor, complement factor H and apolipoprotein E expression showed significant correlations with ADAS-Cog scores at the higher doses (4 mg and 8 mg) of rosiglitazone. Given the well-established, but poorly understood, link between diabetes and AD, there remains a strong interest in the possible utility of diabetes medication in the treatment of AD.
There are limitations to the current study. First, this is a retrospective study of previously conducted clinical trials. A prospective study that enrolled new patients based on the predictive biomarker still needs to be conducted to fully validate the use of rosiglitazone in these specific patients. Second, while this work spanned multiple clinical trials, the sample size remained small. Additional work will be undertaken to assay the remaining samples from the REFLECT trials to (a) further validate these findings and (b) determine if change in biomarkers over the course of treatment can be used as a surrogate outcome. Further research is ongoing to better characterize the metabolic and inflammatory endophenotypes, which have now been shown as predictive biomarkers, among Mexican Americans and non-Hispanic whites in the HABLE study [45] in order to refine and prepare for prospective application in novel clinical trials. The addition of genetic and/or neuroimaging biomarkers may aid in the precision medicine approach and will be investigated in future work. In our prior work, a subset of these markers predicted treatment response to NSAID therapy among AD cases; however, additional work is needed to determine if this approach as well as these markers will predict treatment response to additional interventions or if accuracy varies by disease stage. If this approach is further validated, the pre-analytic processing factors associated with each of these markers and the predictive biomarker will be examined; however, this is not possible with the current data given the long-term storage nature of these samples. A final limitation to this study is the fact that the trial population was selected based on clinical criteria and not on biomarker confirmation. Overall, these findings provide further support for our proposed precision medicine approach to AD.
There are multiple substantial benefits of the precision medicine approach for enrolling patients using predictive biomarkers into novel trials as outlined here. First, by enrolling only those patients most likely to benefit, the effect size of the trial increases and, therefore, the sample size decreases substantially. Second, by screening for multiple subgroups in the AD patient population, multiple trials can be enrolled simultaneously (Fig. 2) thereby reducing cost and patient burden. Finally, the predictive biomarker approach increases likelihood of success of trials and, therefore, can expedite novel therapeutic interventions to market thereby providing patients novel treatments sooner and companies extended patent life. The current data supports the possibility of a precision-medicine model for AD and, therefore, it is our stance that the precision medicine model needs to be further investigated both using existing biorepository samples as well as in new prospective clinical trials.
ACKNOWLEDGMENTS
Research reported here was supported by the National Institute on Aging of the National Institutes of Health under Award Numbers R01AG018440, P30AG062429, R01AG051848. The content is solely the responsibility of the authors and does not necessarily represent the official views of the National Institutes of Health.
Authors’ disclosures available online (https://www.j-alz.com/manuscript-disclosures/20-1610r1).
REFERENCES
[1] | Alzheimer’s Association ((2020) ) 2020 Alzheimer’s disease facts and figures. Alzheimers Dement 16: , 391–460. |
[2] | Alzheimer’s Association ((2018) ) 2018 Alzheimer’s disease facts and figures. Alzheimers Dement 14: , 367–429. |
[3] | Hurd MD , Martorell P , Delavande A , Mullen KJ , Langa KM ((2013) ) Monetary costs of dementia in the United States. N Engl J Med 368: , 1326–1334. |
[4] | Precision Medicine, FDA, https://www.fda.gov/medical-devices/vitro-diagnostics/precision-medicine Last updated September 27, 2018, Accessed on March 12, 2021. |
[5] | Hampel H , O’Bryant SE , Durrleman S , Younesi E , Rojkova K , Escott-Price V , Corvol JC , Broich K , Dubois B , Lista S ((2017) ) A Precision Medicine Initiative for Alzheimer’s disease: The road ahead to biomarker-guided integrative disease modeling. Climacteric 20: , 107–118. |
[6] | Hampel H , O’Bryant SE , Castrillo JI , Ritchie C , Rojkova K , Broich K , Benda N , Nisticò R , Frank RA , Dubois B , Escott-Price V , Lista S ((2016) ) PRECISION MEDICINE - The Golden Gate for detection, treatment and prevention of Alzheimer’s disease. J Prev Alzheimers Dis 3: , 243–259. |
[7] | Hampel H , O’Bryant SE , Molinuevo JL , Zetterberg H , Masters CL , Lista S , Kiddle SJ , Batrla R , Blennow K ((2018) ) Blood-based biomarkers for Alzheimer disease: Mapping the road to the clinic. Nat Rev Neurol 14: , 639–652. |
[8] | Jorgensen JT ((2015) ) Clinical application of companion diagnostics. Trends Mol Med 21: , 405–407. |
[9] | Cresci S , Depta JP , Lenzini PA , Li AY , Lanfear DE , Province MA , Spertus JA , Bach RG ((2014) ) Cytochrome p450 gene variants, race, and mortality among clopidogrel-treated patients after acute myocardial infarction. Circ Cardiovasc Genet 7: , 277–286. |
[10] | Currie G , Delles C ((2016) ) Use of biomarkers in the evaluation and treatment of hypertensive patients. Curr Hypertens Rep 18: , 54. |
[11] | Muraro A , Lemanske RF , Hellings PW , Akdis CA , Bieber T , Casale TB , Jutel M , Ong PY , Poulsen LK , Schmid-Grendelmeier P , Simon HU , Seys SF , Agache I ((2016) ) Precision medicine in patients with allergic diseases: Airway diseases and atopic dermatitis - PRACTALL document of the European Academy of Allergy and Clinical Immunology and the American Academy of Allergy, Asthma & Immunology. J Allergy Clin Immunol 137: , 1347–1358. |
[12] | Comabella M , Sastre-Garriga J , Montalban X ((2016) ) Precision medicine in multiple sclerosis: Biomarkers for diagnosis, prognosis, and treatment response. Curr Opin Neurol 29: , 254–262. |
[13] | Poutiainen P , Jaronen M , Quintana FJ , Brownell AL ((2016) ) Precision medicine in multiple sclerosis: Future of PET imaging of inflammation and reactive astrocytes. Front Mol Neurosci 9: , 85. |
[14] | O’Bryant SE , Zhang F , Johnson LA , Hall J , Edwards M , Grammas P , Oh E , Lyketsos CG , Rissman RA ((2018) ) A precision medicine model for targeted NSAID therapy in Alzheimer’s disease. J Alzheimers Dis 66: , 97–104. |
[15] | Tajik P , Zafarmand MH , Zwinderman AH , Mol BW , Bossuyt PM ((2018) ) Development and evaluating multimarker models for guiding treatment decisions. BMC Med Inform Decis Mak 18: , 52. |
[16] | FDA-NIH Biomarker Working Group (2016) BEST (Biomarkers, EndpointS, and other Tools) Resource Glossary Available from: https://www.ncbi.nlm.nih.gov/books/NBK326791, Accessed on March 12, 2021. |
[17] | Razay G , Wilcock GK ((1994) ) Hyperinsulinaemia and Alzheimer’s disease. Age Ageing 23: , 396–399. |
[18] | Bhargava D , Weiner MF , Hynan LS , Diaz-Arrastia R , Lipton AM ((2006) ) Vascular disease and risk factors, rate of progression, and survival in Alzheimer’s disease. J Geriatr Psychiatry Neurol 19: , 78–82. |
[19] | Biessels GJ , De Leeuw FE , Lindeboom J , Barkhof F , Scheltens P ((2006) ) Increased cortical atrophy in patients with Alzheimer’s disease and type 2 diabetes mellitus. J Neurol Neurosurg Psychiatry 77: , 304–307. |
[20] | Ott A , Stolk RP , Hofman A , Van Harskamp F , Grobbee DE , Breteler MMB ((1996) ) Association of diabetes mellitus and dementia: The Rotterdam Study. Diabetologia 39: , 1392–1397. |
[21] | Ott A , Stolk RP , Van Harskamp F , Pols HAP , Hofman A , Breteler MMB ((1999) ) Diabetes mellitus and the risk of dementia: The Rotterdam Study. Neurology 53: , 1937–1942. |
[22] | Lindsay J , Laurin D , Verreault R , Hébert R , Helliwell B , Hill GB , McDowell I ((2002) ) Risk factors for Alzheimer’s disease: A prospective analysis from the Canadian Study of Health and Aging. Am J Epidemiol 156: , 445–453. |
[23] | Elias MF , Elias PK , Sullivan LM , Wolf PA , D’Agostino RB ((2005) ) Obesity, diabetes and cognitive deficit: The Framingham Heart Study. Neurobiol Aging 26: , 11–16. |
[24] | Luchsinger JA , Tang MX , Stern Y , Shea S , Mayeux R ((2001) ) Diabetes mellitus and risk of Alzheimer’s disease and dementia with stroke in a multiethnic cohort. Am J Epidemiol 154: , 635–641. |
[25] | Peila R , Rodriguez BL , Launer LJ ((2002) ) Type 2 diabetes, APOE gene, and the risk for dementia and related pathologies: The Honolulu-Asia Aging Study. Diabetes 51: , 1256–1262. |
[26] | Arvanitakis Z , Wilson RS , Bienias JL , Evans DA , Bennett DA ((2004) ) Diabetes mellitus and risk of Alzheimer disease and decline in cognitive function. Arch Neurol 61: , 661–666. |
[27] | Haan MN , Mungas DM , Gonzalez HM , Ortiz TA , Acharya A , Jagust WJ ((2003) ) Prevalence of dementia in older Latinos: The influence of type 2 diabetes mellitus, stroke and genetic factors. J Am Geriatr Soc 51: , 169–177. |
[28] | Govindarajulu M , Pinky PD , Bloemer J , Ghanei N , Suppiramaniam V , Amin R ((2018) ) Signaling mechanisms of selective PPAR γ modulators in Alzheimer’s disease. PPAR Res 2018: , 2010675. |
[29] | Khan MA , Alam Q , Haque A , Ashafaq M , Khan MJ , Ashraf GM , Ahmad M ((2018) ) Current progress on peroxisome proliferator-activated receptor gamma agonist as an emerging therapeutic approach for the treatment of Alzheimer’s disease: An update. Curr Neuropharmacol 17: , 232–246. |
[30] | Lin CH , Nicol CJB , Cheng YC , Chen SJ , Yen CH , Huang RN , Chiang MC ((2018) ) Rosiglitazone rescues human neural stem cells from amyloid-beta induced ER stress via PPARγ dependent signaling. Exp Cell Res 370: , 312–321. |
[31] | Gold M , Alderton C , Zvartau-Hind M , Egginton S , Saunders AM , Irizarry M , Craft S , Landreth G , Linnamägi Ü , Sawchak S ((2010) ) Rosiglitazone monotherapy in mild-to-moderate Alzheimer’s disease: Results from a randomized, double-blind, placebo-controlled phase III study. Dement Geriatr Cogn Disord 30: , 131–146. |
[32] | Harrington C , Sawchak S , Chiang C , Davies J , Donovan C , Saunders M.A , Irizarry M , Jeter B , Zvartau-Hind M , van Dyck H.C , Gold M ((2011) ) Rosiglitazone does not improve cognition or global function when used as adjunctive therapy to AChE inhibitors in mild-to-moderate Alzheimer’s disease: Two phase 3 studies. Curr Alzheimer Res 8: , 592–606. |
[33] | McKhann G , Drachman D , Folstein M , Katzman R , Price D , Stadlan EM ((1984) ) Clinical diagnosis of Alzheimer’s disease: Report of the NINCDS-ADRDA work group*; under the auspices of Department of Health and Human Services Task Force on Alzheimer’s disease. Neurology 34: , 939–944. |
[34] | O’Bryant SE , Ferman TJ , Zhang F , Hall J , Pedraza O , Wszolek ZK , Como T , Julovich D , Mattevada S , Johnson LA , Edwards M , Graff-Radford NR ((2019) ) A proteomic signature for dementia with Lewy bodies. Alzheimers Dement (Amst) 11: , 270–276. |
[35] | O’Bryant SE , Edwards M , Zhang F , Johnson LA , Hall J , Kuras Y , Scherzer CR ((2019) ) Potential two-step proteomic signature for Parkinson’s disease: Pilot analysis in the Harvard Biomarkers Study. Alzheimers Dement (Amst) 11: , 374–382. |
[36] | O’Bryant SE , Edwards M , Johnson L , Hall J , Villarreal AE , Britton GB , Quiceno M , Cullum CM , Graff-Radford NR ((2016) ) A blood screening test for Alzheimer’s disease. Alzheimers Dement (Amst) 3: , 83–90. |
[37] | Li Q , Xiao Y , Lu G , Xie D , Zhai Y , Zhang J , Li J , Gao X ((2019) ) Inhibition of perivascular mast cell activation is involved in the atheroprotective effect of rosiglitazone in apolipoprotein E–deficient mice. Biochem Biophys Res Commun 519: , 261–266. |
[38] | Statnikov A , Wang L , Aliferis CF ((2008) ) A comprehensive comparison of random forests and support vector machines for microarray-based cancer classification. BMC Bioinformatics 9: , 319. |
[39] | Aisen PS , Schafer KA , Grundman M , Pfeiffer E , Sano M , Davis KL , Farlow MR , Jin S , Thomas RG , Thal LJ ((2003) ) Effects of rofecoxib or naproxen vs placebo on Alzheimer disease progression: A randomized controlled trial. J Am Med Assoc 289: , 2819–2826. |
[40] | Liu H , Rose ME , Culver S , Ma X , Dixon CE , Graham SH ((2016) ) Rosiglitazone attenuates inflammation and CA3 neuronal loss following traumatic brain injury in rats. Biochem Biophys Res Commun 472: , 648–655. |
[41] | Wang TD , Chen WJ , Cheng WC , Lin JW , Chen MF , Lee YT ((2006) ) Relation of improvement in endothelium-dependent flow-mediated vasodilation after rosiglitazone to changes in asymmetric dimethylarginine, endothelin-1, and C-reactive protein in nondiabetic patients with the metabolic syndrome. Am J Cardiol 98: , 1057–1062. |
[42] | Fidan E , Onder Ersoz H , Yilmaz M , Yilmaz H , Kocak M , Karahan C , Erem C ((2011) ) The effects of rosiglitazone and metformin on inflammation and endothelial dysfunction in patients with type 2 diabetes mellitus. Acta Diabetol 48: , 297–302. |
[43] | Jack CR Jr. , Bennett DA , Blennow K , Carrillo MC , Dunn B , Haeberlein SB , Holtzman DM , Jagust W , Jessen F , Karlawish J , Liu E , Molinuevo JL , Montine T , Phelps C , Rankin KP , Rowe CC , Scheltens P , Siemers E , Snyder HM , Sperling R ((2018) ) NIA-AA Research Framework: Toward a biological definition of Alzheimer’s disease. Alzheimers Dement 14: , 535–562. |
[44] | Akuffo EL , Davis JB , Fox SM , Gloger IS , Hosford D , Kinsey EE , Jones NA , Nock CM , Roses AD , Saunders AM , Skehel JM , Smith MA , Cutler P ((2008) ) The discovery and early validation of novel plasma biomarkers in mild-to-moderate Alzheimer’s disease patients responding to treatment with rosiglitazone. Biomarkers 13: , 618–636. |
[45] | Institute for Translational Research, https://www.unthsc.edu/translational-research/, Accessed on March 12, 2021. |