Procedural Learning Improves Cognition in Multiple Sclerosis
Abstract
Background:
Multiple sclerosis (MS) is considered a neurodegenerative disease and an inflammatory demyelinating neuropathology in young population. Procedural memory has been poorly investigated in MS.
Objective:
We assessed whether the MS group was able to develop a motor-cognitive skill, using a procedural task (PLSC) developed in our laboratory, applying a manual and serial reaction time (RT) paradigm to semantic categorization.
Methods:
We evaluated 26 MS patients and 26 socio-demographic matched control participants using the PLSC task.
Results:
Using non-parametric statistical analyses, we observed a significant improvement of semantic categorization RTs with practice (p = 0.002), even with new verbal material to categorize in MS patients (p = 0.006), despite their motor and executive moderate deficits. This same profile of semantic procedural learning in MS was observed in previous studies carried out with Alzheimer’s and Parkinson’s diseases. Moreover, the visual-motor RTs remained stable or slightly improved over the five blocks in both groups, as well as in the AD groups of previous studies. The MS group showed longer visual-motor reaction times than those of the control group (p < 0.042), except in motor initiation aspect (p = 0.064). Both groups showed no significant differences for any type of error. Additionally, disability level and cognitive performances were not associated with the ratio of semantic procedural learning.
Conclusion:
The present results support the notion that MS patients may be capable of acquiring semantic skill, despite their motor disabilities and executive troubles. This work also addresses the possibilities to improve motor-cognitive skill RTs in neurodegenerative diseases.
INTRODUCTION
Multiple sclerosis (MS) is considered a neurodegenerative disease and a chronic inflammatory-demyelinating of the central nervous system in young populations, affecting white matter, predominantly in the form of demyelinated plaques or lesions, as well as grey matter playing an important role in MS symptomatology and progression [1–3].
MS is a disabling neuropathology such as neurodegenerative diseases like Alzheimer’s (AD) or Parkinson’s (PD) diseases, presenting behavioral, cognitive, sensory, and/or motor disturbances, which diminish the quality of life of patients [3, 4]. Cognitive dysfunction affects 40% to 70% of patients with MS and has a detrimental effect on quality of life that is independent of their physical disability. Cognitive deficits have been reported in all stages of the disease. In addition, the presence of cognitive dysfunction early in the disease predicts a faster rate of disease progression [5, 6]. Cognitive dysfunction is mainly focused on executive functions such as sustained and divided attention, conceptual reasoning, verbal fluency, speed of information processing, and working memory, as well as episodic memory and visual-spatial abilities dysfunctions [1, 7, 8].
Concerning the declarative memory characterized by an explicit recall of information, most studies have found an altered working memory in MS. However, there are controversial results about the episodic memory, but results show more learning deficits with visual material than with verbal material [7–9]. In contrast, procedural memory is defined as the knowledge of a know-how, which is acquired progressively through the repetition of the task. It permits us to acquire motor or cognitive skills automatically and progressively by practice, without awareness of what is being learned [10]. A variety of motor and/or cognitive tasks have been used to assess procedural learning (Mirror reading task, Prism adaptation task, Serial reaction time tasks, Tower of Hanoi, etc.). The evaluation of procedural learning is carried out without the subject being aware of it and acquisition is judged without the knowledge of the subject. Its acquisition can be measured by a reduction of reaction times (RTs) and errors through the practice of procedural task. Converging evidence from animal research and human brain-imaging studies has attributed to an important role for the frontal striatal circuits and cerebellum in procedural learning (for a review, see [11, 12]).
In this context, procedural learning is an essential part of our life which allows us, for instance, to learn driving a car/bicycle, cook, or play a video game, and it can improve the life quality of patients with neurodegenerative diseases such as AD, PD, and MS. The motor-cognitive procedural memory in AD and PD patient groups has been much studied, but few studies have been done in MS. Mostly studies showed contradictory results about procedural capacities in AD [13–16] and in PD [17–19]. In contrast, procedural memory has been poorly investigated in MS, though a few recent studies have evaluated only motor procedural learning and the results are also inconclusive (see [8–12, 20–23]). While some authors have reported that MS patients are preserved in motor learning, independent of severity of disability [21], others have found impaired motor learning even at early phases of the disease [22, 23]. These discrepancies between studies in several neurodegenerative diseases have been interpreted considering differences in methodological variations of the tasks, the processing nature of each procedural task, etc. However, semantic procedural memory has been assessed in AD and PD patients [14, 24] using the same methodology and the nature of a procedural learning task of semantic categorization (PLSC task) developed in our laboratory [25]. These studies observed they were able to acquire semantic skill despite cognitive and motor troubles. However, no studies have evaluated semantic procedural learning in MS patients.
This goal of the present work was to determine whether MS patients were able to develop a semantic skill using the PLSC task, which employs a serial RT paradigm. We hypothesize that, although MS pathology may affect performance speed and motor-cognitive capacities, MS patients can progressively improve procedural skill RTs of the semantic categorization through the repetition of the PLSC task. In addition, we will try to understand motor-cognitive procedural learning in neurodegenerative diseases.
MATERIALS AND METHODS
Subjects
A total of 52 subjects participated in this study: 26 MS patients (6 female and 20 male) and 26 healthy volunteers (7 female and 19 male).
Patients with MS were selected in the study from the Department of Neurology at the Poitiers University Hospital, Poitiers, France. All MS patients were diagnosed by a trained neurologist, using McDonald diagnostic criteria for MS [26]. Patients presenting upper limb impairment, not corrected visual acuity or visual field deficits, a personal history of alcohol or drug abuse, cardiovascular illness, head trauma, major psychiatric disorders or other neurological disorders, or systemic illnesses were excluded from the study. The MS group was composed of 15 MS patients with a relapsing–remitting course, 10 patients with a secondary progressive course, and 3 patients with a primary progressive course. Disease duration ranged from 9 to 19 years, with an average (±SD) of 10.1 years (±7.12). Clinical disability was quantified using the Expanded Disability Status Scale (EDSS) score [27]. The MS group was characterized by a mean score of 4.8 (±1.36) at the EDSS. The MS group had a mean age (±SD) of 42.24 years old (±9.53) and a mean educational level (±SD) of 9.29 years (±1.34). The research was conducted during Methylprednisolone treatment at the hospital.
Twenty-six healthy volunteers matched for age, gender, and educational level served as the control group. The control group had an age mean (±SD) of 43.1 years (±6.47) and a mean educational level (±SD) of 8.94 years (±1.21). The controls were screened, and none was found to have any neurological disorder, psychiatric illness, head injury, or alcohol or drug abuse.
Both subjects’ groups had no global cognitive deterioration assessed by the Mini-Mental State Examination (MMSE) [28] and Rapid Evaluation of Cognitive Function (RECF) [29]. They have no depressive disorders, as evaluated with the Zerssen Rating Scale [30]. All participants were right-handed [31], native French speakers, and used their dominant hand to perform the experimental protocol. The demographic characteristics of both groups are shown in Table 1.
Table 1
Means (M) and standard deviations (SD) of several demographic characteristics and clinical data of multiple sclerosis (MS) and control groups
MS Group M (SD) | Control group M (SD) | pa | |
Gender: | |||
Men | 6 (23.07%) | 7 (26.92%) | 0.99 NS |
Women | 20 (76.93%) | 19 (73.08%) | |
Age | 42.24 (9.53) | 43.1 (6.47) | 0.92 NS |
Education, y | 9.29 (1.34) | 8.94 (1.21) | 0.84 NS |
Expanded Disability Status Scale | 4.8 (1.36) | ||
Years of illness | 10.1 (7.12) |
ap values referred to comparison between control and MS groups. NS, not significant (p > 0.05).
Neuropsychological assessment
A comprehensive battery of neuropsychological tests was administered to all participants in two sessions per week and with the same test order to evaluate the most frequent cognitive difficulties in MS, and the most relevant for our study aim (e.g., verbal fluency, speed of information processing, verbal and visual explicit memory, sustained and divided attention, etc.).
Thus, general mental state was evaluated with MMSE [28] and RECF [29] tests, involving a rapid assessment on cognitive functions.
Visual attention abilities and psychomotor speed were assessed with the Wechsler Adult Intelligence Scale-Revised (WAISI-R) Digit Symbol-coding test [32], as well as the Trail-Making Test part A [33]. In addition, we included the WAIS–R Block design [32] and the Rey complex figure copying test [34] to measure visual-spatial and constructive abilities.
Declarative memory was explored using the French version of a verbal memory test designed by Grober and Buschke [35], consisting of a word list learning and free/cued recall, as well as the Rey complex figure memory test [34] to explore the visual material memory.
Frontal functions were assessed with the Frontal Battery of Luria [36], as well as the naming task with interference of Stroop Test [37] to essentially explore the ability to inhibit cognitive interference, the WAIS–R Similarities Subtest [32], assessing conceptual reasoning, and the Trail-Making Test part B [33], which is a task switching. In addition, we also used the verbal fluency tasks as the Category Fluency task [38] (fruits as items), Phonemic Fluency [39] (items beginning with letter /p/) and Alternate Categorical Fluencies [39] (to name examples from two different categories alternately; e.g., animal and furniture).
Furthermore, we added the WAIS–R Vocabulary [32] and the DO80 verbal naming test [40], which assesses pictures naming to evaluate language abilities.
Finally, the neuropsychological assessment was completed by the mood state examination of the participants, using Zerssen Rating Scale [30].
Ethics
Written, informed consent was obtained from all the included patients. The study was approved by The Regional Committee for Research Ethics, CHU Poitiers.
Experimental task
Procedural learning of semantic categorization was evaluated by the PLSC task, which used a manual and serial reaction time paradigm based on Knopman and Nissen’s 41 similar procedure, but for semantic categorization. The Knopman and Nissen’s paradigm consisted of manual and serial visual reaction time task with an embedded visual repeating sequence. We modified it and constructed a verbal task in order to obtain evidence concerning the procedural learning of semantic categorization [25, 42].
The PLSC task was administered on a DELL 3255X PC computer. Subjects sat up from 50 cm facing a video screen. Four semantic categories of manufactured objects were shown permanently on the four quadrants of the computer screen: kitchen utensils, musical instruments, clothes, and school objects. The positions of the semantic categories corresponded to the spatial arrangement of four Lafayette instrument keys: one key in the center of the instrument (CK) and four peripheral keys corresponding to the spatial position of the semantic categories (PK). A word appeared in the center of the screen and had to be assigned to one of the four semantic categories. Participants were asked to classify each word in one of the four semantic categories. They were instructed to press the CK continuously to make a word appear in the center of the video screen. When they pushed the CK a warning sign appeared and after 500 ms, one word appeared and remained in the place of the warning sign. When the subjects were sure of the word’s semantic category, they released the CK and pressed the PK that corresponded to the spatial position of the semantic category selected. They then immediately released the PK and pressed the CK, and so forth, to make another word appear.
Verbal material used in the PLSC task
Five blocks of trials were administered all together, each consisting of 40 verbal stimuli, 10 stimuli for each semantic category. In the first four blocks, 40 stimuli (A list) were repeated randomly. The fifth block differed from the first four in that, 40 novel stimuli (B list) were presented. The beginning and end of each repetition were not marked. Stimulus-set items were selected from four semantic categories of kitchen articles, musical instruments, types of clothing, and school objects. No categories overlapped semantically. We chose the same two lists of verbal stimuli that were used previously by our research group [see 25, 42] and were matched (word by word from each list) as a function of grammatical class (Brulex) [43], categorical frequency [44], word length (Brulex) [43], and form frequency (Brulex) [43]. Both lists were counterbalanced among subjects.
Data analysis
Data were analyzed using the Statistical Package for the Social Sciences software (version 15.0). In this study an α level of 0.05 was selected for statistical significance.
To check whether there were significant differences between the two groups as a function of age, we used Student’s t-test; in the case of educational level and gender, we used χ2 tests.
Concerning the data from PLSC task, response accuracy and RTs were recorded for each block (B) of stimuli by software [45] on the DELL 3255 PC computer. Four different measurements of RT in milliseconds were obtained:
• RT1: Interval between the appearance of a word and the time of release of the CK. This was designated the “RT of semantic categorization and motor initiation”.
• RT2: Interval between the release of the CK and the time of pressing one of the four PK. This was called the “RT of visual-spatial search”.
• RT3: Interval between pressing the selected PK and the time of releasing this PK. This was called “RT of motor initiation”.
• RT4: Interval between release of the PK and the time of pressing the CK. This was called the “RT of motor movement”.
Considering the software involved in the PLCS task and the instructions given to the subjects for completing this task, RT1 minus RT3 (RT1-RT3) is the most interesting reaction time, reflecting “semantic categorization”. RT1 was the reaction time required by the subject to categorize a word appearing on the screen and then release his/her finger from the CK. It was necessary to remove the motor initiation reaction time aspect (RT3) from RT1 and hence RT1-RT3 reflected only the “semantic categorization RT”.
In addition, the software also recorded three kinds of errors:
• E1: Release of the CK before a word appeared in the middle of screen.
• E2: Consecutive presses and releases of the CK.
• E3: Error in semantic categorization.
Our prediction was that if the MS group was able to acquire a procedural learning of semantic categorization using this PLSC task, three results within the “semantic categorization RT” (RT1–RT3) would be recorded, as observed in previous studies [14, 24, 25, 42].
(1) A decrease in RT1–RT3 across B1 to B4, in which the repeating list was given. The ratio reflecting the improvement in the semantic categorization of the same verbal material was: (B1 minus B4 of RT1–RT3)×100/B1 of RT1–RT3 (% B1–B4 RT1–RT3). However, this RT reduction could also be induced by simple perceptual-motor learning. Therefore, it was necessary to record the following two results in order to observe the procedural learning of semantic categorization:
(2) RT1–RT3 in B5 (B5 with list B of new items), which should be slower than B4 (block with list A of items which were repeated four times), because the acquired semantic procedural skill should continue to improve performance with new words. It was defined operationally as the RT1–RT3 difference between B5 and B4 (B5–B4 RT1–RT3).
(3) Accordingly, RT1–RT3 in B5 (B5) should be faster than in B1 because the semantic procedural skill could not completely compensate for the novelty of the verbal material. It was defined operationally as the RT1–RT3 difference between B1 and B5 (B1–B5 RT1–RT3).
In general, the ratio reflecting the procedural learning of semantic categorization is measured by the following formula: (B1 minus B5 RT1–RT3)×100/B1 RT1–RT3 (% B1–B5 RT1–RT3).
Incorrect responses were not included in the analyses of RTs. The RTs were measured for each block of trials using the individual medians of RT. Moreover, the PLSC task does not allow answers to be corrected and does not provide feedback. The reaction times were measured for each block of trials using the individual medians of RT. For non-parametric statistical analyses, the Friedman and Wilcoxon tests were used to compare the medians of RT between blocks, which afforded confirmatory evidence of the procedural learning of semantic categorization. The Friedman test was also used to compare the results of two subject groups, in particular the results concerning the RT1–RT3 differences between block 1 and block 4 (B1–B4 RT1–RT3) and the ratio reflecting the procedural learning of semantic categorization (% B1–B5 RT1–RT3).
RESULTS
Demographic and neuropsychological characteristics
Concerning the demographic characteristics of the MS and control groups, there were no significant differences in age (p = 0.99), gender (p = 0.92), or educational level (p = 0.84) distributions between the groups. The means and standard deviations of the different demographic characteristics of the MS and control groups are shown in Table 1.
In relation to the battery of neuropsychological tests administered to both groups, the means and standard deviations of the neuropsychological tests are shown in Table 2. We observed that there were no significant differences between the groups for the general mental state evaluated by the MMSE [28] (p = 0.068) and RECF [29] (p = 0.061) tests.
Table 2
Means (M) and standard deviations (SD) of cognitive assessment tests in multiple sclerosis (MS) and control groups
Assessment Tests | MS Group M (SD) | Control Group M (SD) | pa |
Mental state | |||
MMSE | 27.86 (2.2) | 28.32 (1.51) | 0.068 |
RECF | 47.34 (2.37) | 48.5 (1.71) | 0.061 |
Visual attention abilities and psychomotor speed | |||
Digit Symbol-Coding (WAIS-R*) | 6.91 (2.29) | 12.65 (2.1) | 0.003** |
Trail Making: part A | 64.87 (38) | 33.2 (4.5) | 0.001** |
Visual-spatial and constructive abilities | |||
Block design (WAI-R*) | 8.72 (2.64) | 10.23 (1.36) | 0.074 |
Rey complex figure test: | |||
copy accuracy | 32.14 (3.49) | 34.10 (2.41) | 0.064 |
copy time | 5.27 (1.62) | 3.92 (5.94) | 0.074 |
Memory capacities | |||
Rey complex figure test: | |||
delayed recall | 19.83 (7.11) | 24.29 (2.93) | 0.059 |
Free/cued recall test (Grober and Buschke): | |||
immediate recall | 14.54 (1.9) | 15.42 (0.64) | 0.078 |
short free recall | 25 (10.56) | 32.28 (4.02) | 0.038** |
short delay cued recall | 10.96 (6.24) | 11.67 (2.25) | 0.08 |
total recognition | 15.45 (1.29) | 15.88 (0.53) | 0.093 |
false semantic recognition | 0.1 (0.61) | 0.27 (0.31) | 0.069 |
false non-semantic recognition | 0.2 (0.57) | 0.3 (0.59) | 0.084 |
long delay free recall | 8.91 (3.14) | 13.02 (1.41) | 0.014** |
long delay cued recall | 4.02 (2.43) | 2.92 (0.31) | 0.091 |
Frontal functions | |||
Luria’s Frontal Battery | 14.64 (8.27) | 6.42 (4.81) | 0.011** |
Stroop test: naming task with interference | 0.26 (8.57) | 2.13 (2.84) | 0.096** |
Trail Making: part B | 124.36 (29) | 77.4 (6.6) | 0.024** |
Similarities (WAIS-R*) | 10.31 (3.9) | 15.14 (1.98) | 0.042** |
Alternate categorical fluency | 13.32 (5.14) | 19.86 (2.62) | 0.012** |
Language abilities | |||
Category fluency | 17.67 (5.95) | 29.07 (2.26) | 0.009** |
Phonemic fluency | 10.62 (5.52) | 14.64 (1.51) | 0.030** |
Vocabulary (WAIS-R*) | 9.24 (2.36) | 10.18 (0.84) | 0.077 |
DO80 | 78.96 (2.54) | 79.44 (1.23) | 0.086 |
Mood state | |||
Zerssen Rating Scale | 9.97 (4.39) | 10.58 (5.12) | 0.056 |
ap values referred to comparison between control and MS groups. *Age-adjusted score. **Significant difference (p < 0.05).
Visual attention abilities and psychomotor speed assessed by the WAISI-R Digit Symbol-coding test [32] and the Trail-Making Test part A [33] were significantly different between subject groups (p = 0.003 and 0.001, respectively). But both subject groups showed no significant differences in visual-spatial and constructive abilities assessed by the WAIS–R Block design [32] (p = 0.074) and the Rey complex figure copying test [34] (p = 0.064).
Concerning the memory capacities evaluated by Grober and Buschke verbal memory [35] and the Rey complex figure memory tests [34], analysis did not reveal significant differences between the subject groups (p = 0.059), except in free recall items of Grober and Buschke verbal memory test [35] (p = 0.08).
Besides, we found significant differences between the two subject groups concerning the frontal functions assessed by Frontal Battery of Luria [36] (p = 0.011), naming task with interference of Stroop Test [37] (p = 0.096), the WAIS–R Similarities Subtest [32] (p = 0.042), and the Trail-Making Test part B [33] (p = 0.024). In addition, the MS group showed significant lower results than those of control group in all verbal fluency tasks (the Category Fluency [38]; p = 0.01), Phonemic Fluency [39]; p = 0.03), and Alternate Categorical Fluency [39]; p = 0.01). But there were not significant differences in others tests of language abilities such as the WAIS–R Vocabulary [32] (p = 0.077) and the DO80 verbal naming test [40] (p = 0.086).
For the non-cognitive tests, there were no significant differences between the groups according to the Zerssen Rating Scale [30]; the MS and control groups did not have depressive disorders (mean±SD: 9.97±4.39 and 10.98±4.67, respectively; p = 0.052).
Experimental protocol performances
We studied the procedural learning of semantic categorization in 26 MS patients. In the beginning of PLSC task (B1), the MS group showed slightly longer RT1–RT3 medians than the control group without reaching significance (p > 0.05). And, the MS patients produced comparable errors of semantic categorization (E3) compared to those of control group (p > 0.08) in B1.
As seen in Tables 3 and 4 as well as in Figs. 1 and 2, we recorded the three results in RT1–RT3, which we had hypothesized reflect procedural learning of semantic categorization in both groups (see section “Data analysis” above):
(1) The MS group showed a significant reduction in RT1–RT3 medians over the first four blocks (920 ms in B1 versus 608 ms in B4; p = 0.002), as occurred with the control group (792 ms in B1 versus 520 ms in B4; p = 0.0001). However, no significant group effect was observed (p = 0.24). The ratio of improvement in the semantic categorization of the same verbal material (% B1–B4) was significantly high in the MS group (31.36%; p = 0.0001) and in the control group (30.1%; p = 0.0001). However, there was no significant group effect (p = 0.82). We failed to observe any significant correlation between (% B1–B4) and all neuropsychological tests (p > 0.06) or EDSS score (r = –0.3; p > 0.48).
(2) All participants had RT1–RT3 medians in B5 that were significantly slower than in B4 (MS group: 800 ms in B5 versus 608 ms in B4; p = 0.04 and control group: 643 ms in B5 versus 520 ms in B4; p = 0.01). The RT1–RT3 difference between B5 and B4 (B5–B4 RT1–RT3) had a median of 144 (p = 0.006) for the MS group and 121.25 (p = 0.004) for the control group. However, no significant group effect was observed (p = 0.31).
(3) In the MS and control groups, the RT1–RT3 in B5 was significantly faster than in B1 (MS group: 800 ms in B5 versus 920 ms in B1; p = 0.0003 and control group: 643 ms in B5 versus 792 ms in B1; p = 0.0001). However, there was no significant group effect (p = 0.78).
Table 3
Reaction time (RT) means, standard deviations (SD) and medians in each block of multiple sclerosis (MS) and control groups
MS group | Control group | ||||||||||
B1 | B2 | B3 | B4 | B5 | B1 | B2 | B3 | B4 | B5 | ||
RT1 | mean | 1235.95 | 991.25 | 861.13 | 857.51 | 1070.2 | 1034.24 | 831.85 | 761.68 | 726.45 | 858.96 |
SD | 475.55 | 388.66 | 325.96 | 346.1 | 435.4 | 233.01 | 193.2 | 148.97 | 143.61 | 179.13 | |
median | 1083.25 | 815 | 782.25 | 771 | 961 | 1028.75 | 783.5 | 733 | 686.5 | 837 | |
RT2 | mean | 952.55 | 855.52 | 919.13 | 771.3 | 774.02 | 652.54 | 580.1 | 514.65 | 519.1 | 476.8 |
SD | 709.89 | 700.14 | 909.23 | 500.84 | 547.15 | 466.32 | 395.14 | 312.14 | 323.64 | 251.1 | |
median | 641 | 628.5 | 590.25 | 585 | 563 | 487.75 | 469.5 | 451 | 432.5 | 422.25 | |
RT3 | mean | 210.06 | 201.2 | 168.56 | 158.24 | 191.6 | 232.9 | 218.2 | 214.76 | 188.16 | 208.8 |
SD | 136.14 | 130.12 | 107.27 | 88.5 | 122.59 | 233.99 | 117.96 | 124.9 | 104.8 | 125.97 | |
median | 214 | 169.25 | 143.75 | 154 | 162 | 210 | 197.5 | 186 | 165 | 176.5 | |
RT4 | mean | 744.1 | 662.21 | 644.1 | 601.19 | 586.1 | 531.94 | 485.25 | 454.64 | 442.14 | 433 |
SD | 360.2 | 284.8 | 281.84 | 224.65 | 229.32 | 232 | 203.28 | 175.5 | 162.11 | 161.8 | |
median | 557 | 573.25 | 549.5 | 526 | 501 | 469 | 435.5 | 403 | 400 | 395 | |
RT1–RT3 | mean | 1027.89 | 791.2 | 692.14 | 699.88 | 880.19 | 797.24 | 616.26 | 546.4 | 536.7 | 649.47 |
SD | 525.94 | 451.1 | 379.17 | 390.47 | 412.75 | 261.25 | 208.5 | 183.75 | 169.75 | 225.75 | |
median | 920 | 610.5 | 560.25 | 608 | 800 | 792 | 578.5 | 524.75 | 520 | 643 |
Table 4
RT1–RT3 differences and performance ratios between blocks of multiple sclerosis (MS) and control groups
RT1–RT3 | B1–B4 RT | B5–B4 RT | B1–B5 RT | % B1–B4 RT | % B1–B5 RT | |
MS group | median | 244.25 | 144 | 97.75 | 31.36 | 13.78 |
mean | 328.01 | 180.31 | 147.70 | 31.91 | 14.36 | |
SD | 221.4 | 232.3 | 235.64 | 14.72 | 22.9 | |
pa | 0.0001 | 0.001 | 0.005 | 0.0001 | 0.01 | |
Control group | median | 223.3 | 121.25 | 104.12 | 30.1 | 15.9 |
mean | 260.54 | 112.77 | 147.77 | 32.68 | 18.53 | |
SD | 225.1 | 168.4 | 266.1 | 22.2 | 29.8 | |
pa | 0.0001 | 0.0001 | 0.0001 | 0.0001 | 0.0001 | |
pb | 0.24* | 0.31* | 0.78* | 0.82* | 0.5* |
ap referred to RT differences between blocks in each subjects group. bp values referred to comparison between control and MS groups. *Statistically non-significant group effect (p > 0.1).
Fig.1
Median reaction times (RT) in milliseconds in each block of the MS group. RT1, The “RT of semantic categorization and motor initiation”; RT2, The “RT of visual-spatial search”; RT3, The “RT of motor initiation”; RT4, The “RT of motor movement”; RT1–RT3, The “RT of semantic categorization”: RT1 minus RT3.
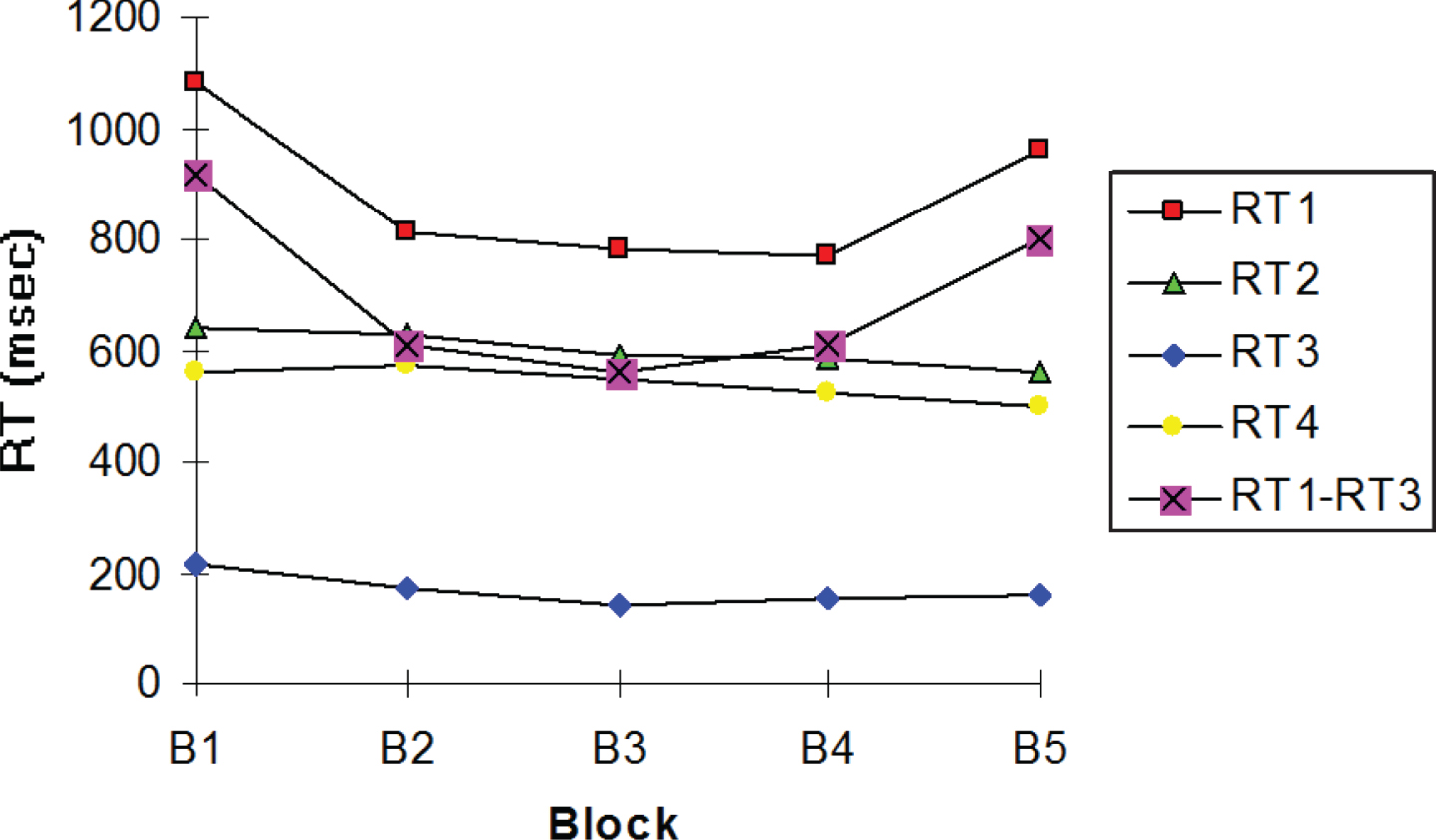
Fig.2
The “Reaction time of semantic categorization” (RT1–RT3) median in milliseconds (ms) in each block for the MS and control groups.
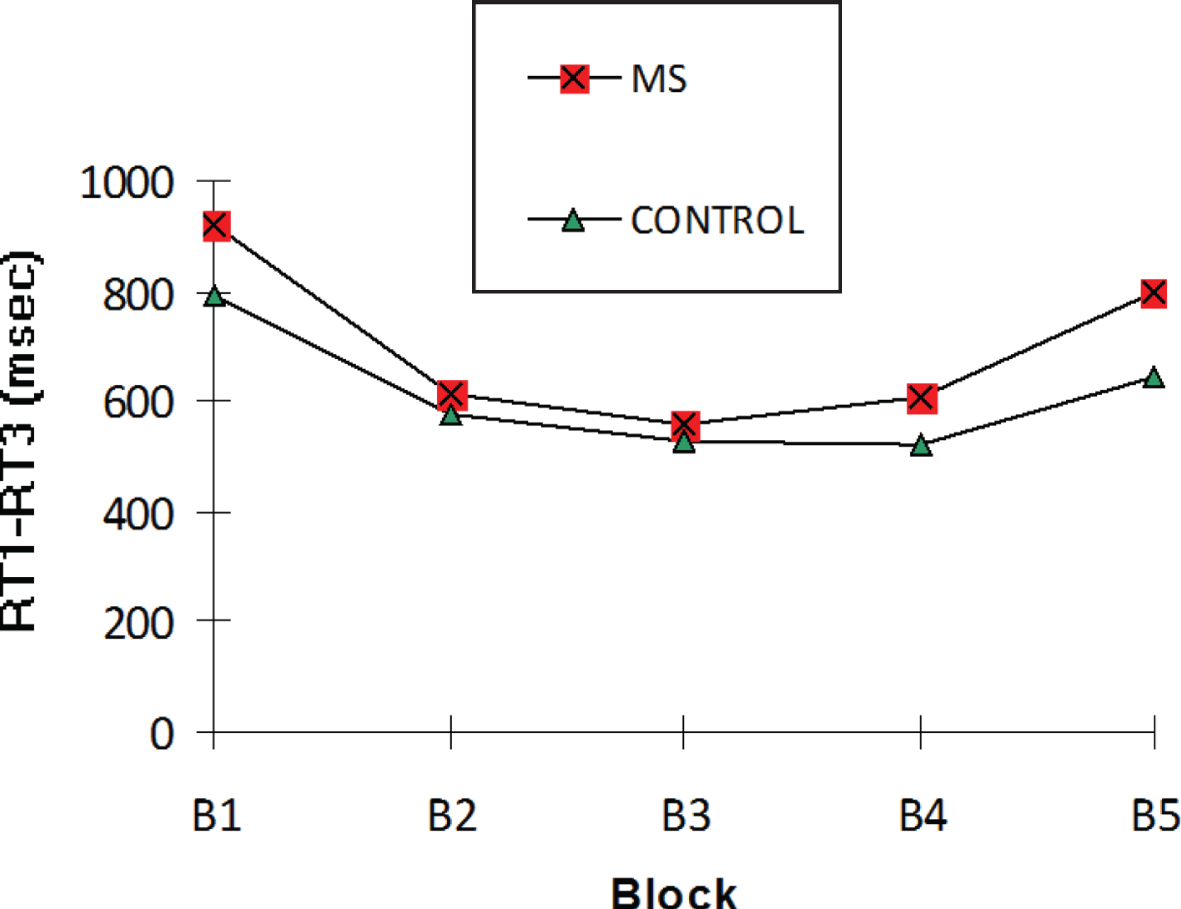
The global ratio reflecting the procedural learning of semantic categorization (% B1–B5 RT1–RT3) was 13.78% (p = 0.001) in the MS group versus 15.9% (p = 0.0001) in the control group. However, there was no significant group effect (p = 0.5). Besides, we failed to observe any significant correlation between the % B1–B5 RT1–RT3 and each neuropsychological test (p > 0.094) or EDSS score (r = –2.3; p > 0.54).
Moreover, we observed how RT of the three visuo-motor aspects of our PLSC task changed over the five blocks: RT2, RT3, and RT4. Those visual-motor RTs of our PLSC task were relatively similar over the five blocks in all participants, but they were significantly longer in MS group than those of the control group in each block (RT2: p = 0.042 and RT4: p = 0.039), except the RT3 (p = 0.064).
Concerning the errors during the PLSC task produced for both groups, Table 5 displays the means of three types of errors as a function of blocks and block differences (B1–B4 and B1–B5). E1 and E2 accuracy were similar in both subject groups and there were no significant group effects in the B1–B4 and B1–B5 differences for E1 (p = 0.26 and p = 0.56, respectively) and for E2 (p = 0.22 and p = 0.18, respectively). The most interesting type of error in our PLSC task was one of semantic categorization (E3). The MS patients scored slightly higher E3 means over the five blocks in comparison with the normal subjects, without reaching significance (p > 0.05). Furthermore, there were no significant group effects in B1–B4 E3 (p = 0.14) and in B1–B5 E3 (p = 0.1).
Table 5
Error mean and standard deviation (SD) in each block and in block differences of the multiple sclerosis (MS) and control groups
B1 | B2 | B3 | B4 | B5 | B1–B4 E | B1–B5 E | |||
MS Group | Mean | 0.06 | 0.37 | 0.4 | 0.17 | 0.27 | –0.11 | –0.21 | |
E1 | SD | 0.23 | 1.01 | 2.81 | 0.37 | 0.73 | 0.46 | 0.79 | |
Control Group | Mean | 0.05 | 0.09 | 0.12 | 0.13 | 0.12 | –0.07 | –0.1 | |
SD | 0.31 | 0.32 | 0.44 | 0.58 | 0.44 | 0.6 | 0.5 | ||
pa | 0.26* | 0.56* | |||||||
MS Group | Mean | 3.1 | 3.26 | 2.51 | 3.38 | 3.47 | –0.28 | –0.37 | |
E2 | SD | 2.86 | 2.93 | 2.69 | 2.98 | 3.06 | 3.92 | 3.84 | |
Control Group | Mean | 1.77 | 1.38 | 2.2 | 1.75 | 2.22 | 0.02 | –0.45 | |
SD | 2.54 | 2.37 | 2.1 | 3.31 | 3.27 | 3.5 | 3.27 | ||
pa | 0.22* | 0.18* | |||||||
MS Group | Mean | 2.24 | 2.23 | 1.9 | 1.87 | 1.8 | 0.37 | 0.44 | |
E3 | SD | 1.81 | 1.47 | 1.41 | 1.57 | 1.44 | 1.11 | 1.89 | |
Control group | Mean | 1.51 | 1.55 | 1.57 | 1.53 | 1.77 | –0.02 | –0.26 | |
SD | 1.44 | 1.43 | 1.44 | 1.39 | 1.69 | 0.88 | 1.88 | ||
pa | 0.14* | 0.11* |
ap values referred to comparison between control and MS groups. *Statistically non-significant group effect (p > 0.1).
DISCUSSION
The aim of this study was to determine whether a MS group with motor disabilities and cognitive difficulties could develop the procedural learning of semantic categorization using the PLSC task [25] which would be reflected in improvements in semantic categorization RTs, even with new verbal material.
Despite MS patients showed moderate level of disability, executive dysfunction, and psychomotor speed troubles, the results suggest that MS patients acquired semantic categorization skill by practice/repetition. In the MS and control groups we observed a significant improvement in semantic performance with the same verbal material across the first four blocks as a result of repetition, and this improvement in semantic performance was able to facilitate the semantic categorization of new words significantly more quickly. Additionally, the ratio reflecting the procedural learning of semantic categorization was similar in both groups. Besides, in both groups there were no significant B1–B4 and B1–B5 differences for any type of error in each subject group, but MS patients slightly produced more errors. Thus, the MS subjects had a moderate disability assessed by EDSS, but they were able to access semantic memory faster and faster in a procedural manner efficiently. The MS and control groups produced similar profiles of procedural learning of semantic categorization.
This same profile of semantic procedural learning using the PLSC task in MS was observed in previous studies carried out with others neurodegenerative diseases as AD [14] and PD [24], as well as normal people [25]. Concerning previous studies with the PLSC, the AD group was also able to acquire semantic skill without awareness, simply by repeated exposure, although their semantic accuracy will not reach normal levels. And the non-demented PD group could also develop comparable semantic skill to those of the control group, despite their motor impairments and executive deficits. However, we have not found any study about cognitive procedural learning with verbal stimuli in MS, but a few studies have assessed motor procedural learning, using serial RT tasks [21, 23]. They observed controversial results in MS patients at different disease stages.
Improved performances with the PCLS task demand both motor system ‘feed-forward’ adaptations and reinforcement of a specific cognitive procedural memory over successive blocks [46]. Several studies have indicated that the basal ganglia and its cortical connections play an important role in procedural learning [47, 48]. The cortical–subcortical systems (particularly cortical-cerebellar and cortical-striatal circuits) that are implicated in procedural learning are affected by neurodegenerative diseases such as PD and MS [17]. However, MS, AD [14], and PD patients [24] were able to develop that motor-cognitive skill, using the same PCLS task. It seems that subcortical structures, which are damaged in MS and PD, as well as the cortical-subcortical posterior atrophy in AD are not necessary to produce impairments of the semantic procedural learning. In this sense, learning of new skills could demand a qualitatively different type of motor-cognitive functional adaptation in patients relative to healthy controls. Previous imaging studies [48] showed that brain activation patterns associated with simple tasks in MS patients resemble those engaged in healthy controls for executing complex tasks. Further functional studies are needed to elucidate mechanisms of skill learning in MS. In addition, results from other studies in neurodegenerative diseases have shown that patients may be successful at accomplishing some cognitive skill learning tasks only through the use of alternate brain areas compared to those activated by healthy control subjects, in order to compensate their basal ganglia and hippocampus activity deficits [19, 49]. In our work, it is possible that our MS patients were using their declarative memory capacities to perform the PCLS task in order to compensate for impaired cortical–subcortical systems, although we failed to observe any significant associations between any of the neuropsychological tests, even the executive tests, and the ratio of the procedural learning of semantic categorization in the MS group. It is well established that the cognitive changes brought about by MS include executive deficits [1, 7, 9], like our MS group. We observed similar results concerning the cognitive troubles and no correlation between cognitive tests and semantic procedural learning similar cognitive troubles in the MS group than those of the PD group [50, 51] from our previous study [24]. In both studies, the semantic skill seems also independent of executive performance. Besides, it seems that cortical and subcortical structures, which are damaged in neurodegenerative diseases such as PD, AD, and MS, are not necessary to produce impairments of motor-cognitive skills assessed by the same PCLS task and methodology. Other studies have also implicated the cerebellum and frontal subcortical circuits, which are involved in MS pathology [11, 12], as components of the neural network that supports the acquisition of new skills [52]. However, the specific contributions of each of these structures seem to vary depending on the motor or cognitive processes underlying the specific task [53, 54]. Further studies are needed to elucidate the implication of cerebellum structure and largest cortico-subcortical circuits on motor-cognitive skills.
This PLSC task also involves other learning processes such as motor or visual-perceptual learnings. We observed that the figure pattern of semantic RT was different from those of the visual-motor RTs for both groups. In the MS and control groups, the visual-motor RTs remained stable or slightly improved over the five blocks because of visual-motor training. Several previous studies have shown similar results [21, 23, 55], using others motor and visual-motor procedural tasks. Nevertheless, the MS group was slower than control group in visual-motor RTs, except in RT3. RT3 assessed the motor initiation aspect and was similar to that of control group. This result suggests that MS did not affect the initiation of controlled motor movement, but it affected the other visual-motor aspects of the PLSC task, which have been associated primarily with white matter atrophy [56]. In addition, it suggests that performances across the blocks of the PLSC task were not affected by fatigue in MS patients. However, several studies observed an important fatigue effect using RT tasks [57, 58] or cognitive tasks in MS groups [59, 60]. A previous study using the same PCLS in neurodegenerative diseases as AD [14] also found the visual and motor performance improvements more moderate over the five blocks than for semantic categorization RTs. However, the study in PD [24] observed fluctuations in visual and motor performances, but a significant semantic skill learning.
While disability and brain lesion load could be reflected in the level of task-related motor performance, they both did not predict the rate of semantic skill. Thus, we suggest that the procedural learning of semantic categorization can be developed independently of visual-motor deficits. Besides, we did not find any relation between disability assessed by EDSS and rate of semantic skill. In this sense, Tomassini et al. [21] observed the same results, using motor skill task independently of disability level. But a recent study has found impairment of motor skill learning in MS patients with minimal disability [23]. Possible limitations of this study can be related to the small simple size with a similar disability level, which reduced the ability to detect potential statistically significant differences in performance changes between both groups, as well as to examine the effect of various disabilities levels of semantic skill. Besides, we must consider that the EDSS is a widely used scale for quantifying MS disability, but it is biased toward the impairment of lower limbs [61]. We suggest that limitations in EDSS scoring may have contributed to our findings and the application of a more specific measure of upper limb disability might have revealed some relationship with the performances of the PCLS task.
Moreover, it is necessary to consider a limitation in our work, to the future studies, concerning not having performed a follow-up evaluation to test the persistence and the generalization of the semantic procedural learning effect in participants, as well as to examine deeper the possibilities of the PLSC task for the cognitive stimulation of semantic knowledge in patients. It will also be interesting to use an ecological semantic or language task to have evidence about long-term effects in an ecological communication context.
In conclusion, the present results support the notion that MS patients may be capable of acquiring semantic skill using a motor-cognitive procedural task, despite their motor disabilities and cognitive difficulties. These outcome measures could improve motor-cognitive RTs of the daily life activities such as video games, communicative abilities, use of mobile/technological applications, etc. in patients with neurodegenerative diseases.
ACKNOWLEDGMENTS
Support for this research was provided by “la CAIXA” bank.
Authors’ disclosures available online (https://www.j-alz.com/manuscript-disclosures/19-1083r2).
REFERENCES
[1] | Thompson AJ , Baranzini SE , Geurts J , Hemmer B , Ciccarelli O ((2018) ) Multiple sclerosis. Lancet 391: , 1622–1636. |
[2] | Bø L ((2009) ) The histopathology of grey matter demyelination in multiple sclerosis. Acta Neurol Scand 189: , 51–57. |
[3] | Chaudhuri A ((2013) ) Multiple sclerosis is primarily a neurodegenerative disease. J Neural Transm 120: , 1463–1466. |
[4] | Doshi A , Chataway J ((2016) ) Multiple sclerosis, a treatable disease. Clin Med (Lond) 16: , s53–s59. |
[5] | Deloire M , Ruet A , Hamel D , Bonnet M , Brochet B ((2010) ) Early cognitive impairment in multiple sclerosis predicts disability outcome several years later. Mult Scler 16: , 581–587. |
[6] | Chiaravalloti ND , DeLuca J ((2008) ) Cognitive impairment in multiple sclerosis. Lancet Neurol 7: , 1139–1151. |
[7] | Korakas N , Tsolaki M ((2016) ) Cognitive impairment in multiple sclerosis: A review of neuropsychological assessments. . Cogn Behav Neurol 29: , 55–67. |
[8] | Langdon DW ((2011) ) Cognition in multiple sclerosis. Curr Opin Neurol 24: , 244–249. |
[9] | Koutsouraki E , Kalatha T , Grosi E , Koukoulidis T , Michmizos D ((2019) ) Cognitive decline in multiple sclerosis patients. Hell J Nucl Med 22: , 75–81. |
[10] | Squire LR ((2004) ) Memory systems of the brain: A brief history and currenterspective. Neurobiol Learn Mem 82: , 171–177. |
[11] | Reber PJ ((2013) ) The neural basis of implicit learning and memory: A review of neuropsychological and neuroimaging research. Neuropsychologia 51: , 2026–2042. |
[12] | Carpenter KL , Wills AJ , Benattayallah A , Milton F ((2016) ) A comparison of the neural correlates that underlie rule-based and information-integration category learning. Hum Brain Mapp 37: , 3557–3574. |
[13] | Chauvel G , Maquestiaux F , Gemonet E , Hartley A , Didierjean A , Masters R , Dieudonné B , Verny M , Bier N , Joubert S ((2018) ) Intact procedural knowledge in patients with Alzheimer’s disease: Evidence from golf putting. J Mot Behav 50: , 268–274. |
[14] | Arroyo-Anlló EM , Ingrand P , Gil R ((2012) ) Improvement of semantic categorization through procedural learning in Alzheimer’s disease. J Alzheimers Dis 29: , 1–9. |
[15] | Merbah S , Salmon E , Meulemans T ((2011) ) Impaired acquisition of a mirror-reading skill in Alzheimer’s disease. Cortex 47: , 157–165. |
[16] | Dick MB , Hsieh S , Bricker J , Dick-Muelhlke C ((2003) ) Facilitating acquisition of a continuous motor task in healthy older adults and patients with Alzheimer’s disease. Neuropsychology 17: , 202–212. |
[17] | Smith JG , McDowall J ((2011) ) Dissociating sequence learning performance in Parkinson’s disease: Visuomotor sequence acquisition and pattern judgment on a serial reaction time task. Acta Neurobiol Exp 71: , 359–380. |
[18] | Wilkinson L , Jahanshahi M ((2007) ) The striatum and probabilistic implicit sequence learning. Brain Res 1137: , 117–130. |
[19] | Moody TD , Bookheimer SY , Vanek Z , Knowlton BJ ((2004) ) An implicit learning task activates medial temporal lobe in patients with Parkinson’s disease. Behav Neurosci 118: , 438–442. |
[20] | Tablerion JM , Wood TA , Hsieh KL , Bishnoi A , Sun R , Hernandez M , An R , Sosnoff JJ ((2019) ) Motor learning in people with multiple sclerosis: A systematic review and meta-analysis. Arch Phys Med Rehabil 19: , 31312–31317. |
[21] | Tomassini V , Johansen-Berg H , Leonardi L , Paixao L , Jbabdi S , Palace J , Pozzilli C , Matthews PM ((2011) ) Preservation of motor skill learning in patients with multiple sclerosis. Mult Scler 17: , 103–115. |
[22] | Marié RM , Defer GL ((2001) ) Memory and executive functions in multiple sclerosis: Preliminary finding with a cognitive battery. Rev Neurol (Paris) 157: , 402–408. |
[23] | Tacchino A , Bove M , Roccatagliata L , Mancardi GL , Uccelli A , Bonzano L ((2014) ) Selective impairments of motor sequence learning in multiple sclerosis patients with minimal disability. Brain Res 2: , 91–98. |
[24] | Arroyo-Anlló EM , Ingrand P , Neau JP , Gil R ((2015) ) Procedural learning of semantic categorization in Parkinson’s disease. J Alzheimers Dis 45: , 205–216. |
[25] | Arroyo-Anlló EM , Ingrand P , Gil R ((2007) ) Procedural learning of semantic categorization: A study of 110 non-disabled individuals. Disabil Rehabil Assist Technol 2: , 366–374. |
[26] | McDonald WI , Compston A , Edan G , Goodkin D , Hartung HP , Lublin FD , McFarland HF , Paty DW , Polman CH , Reingold SC , Sandberg-Wollheim M , Sibley W , Thompson A , van den Noort S , Weinshenker BY , Wolinsky JS ((2001) ) Recommended diagnostic criteria for multiple sclerosis: Guidelines from the international panel on the diagnosis of multiple sclerosis. Ann Neurol 50: , 121–127. |
[27] | Kurtzke JF ((1983) ) Rating neurologic impairment in multiple sclerosis: An expanded disability status scale (EDSS). Neurology 33: , 1444–1452. |
[28] | Folstein MF , Folstein SE , McHugh PR ((1975) ) “Mini-Mental State”: A practical method for grading the cognitive state of patients for the clinician. J Psychiatr Res 12: , 189–198. |
[29] | Gil R , Toullat G , Pluchon C , Micheneau D , Cariou B , Rivault L , Sicot I , Boissonnot L , Neau JP ((1986) ) Une méthode d’évaluation rapide des fonctions cognitives (ERFC). Son application à la démence sénile de type Alzheimer. Sem Hop Paris 62: , 2127–2133. |
[30] | Heimann H , Bobon-Schrod H , Schmocker AM , Bobon DP ((1975) ) Self-rating of mood using a list of adjectives, Zerssen’s befindlichkeits Skala. Encephale 1: , 165–183. |
[31] | Salmaso D , Logoni AM ((1985) ) Problems in the assessment of hand preference. Cortex 21: , 533–549. |
[32] | Wechsler D ((1991) ) Manuel de l’chelle clinique de mémoire de Wechsler - révisée (WMS - R). Editions du Centre de Psychologie Appliquée, Paris. |
[33] | Reitan RM ((1958) ) Validity of the trail making test as an indicator of organic brain damage. Percept Mot Skills 8: , 271–276. |
[34] | Rey A ((1959) ) Manuel de la figure complexe de Rey, Editions du CPA, Paris. |
[35] | Grober E , Buschke H ((1987) ) Genuine memory deficits in dementia. Dev Neuropsychol 3: , 13–36. |
[36] | Malloy PF , Webster JS , Russel W ((1985) ) Tests of Luria’s frontal lobe syndromes. Int J Neuropsychol 7: , 88–95. |
[37] | Golden CJ ((1978) ) Stroop color and word test. A manual for clinical and experimental uses, Stoelting Company, Chicago, IL, USA. |
[38] | Mattis S ((1973) ) Dementia Rating Scale (Professional Manual).. Psychological Assessment Resources, Odessa, FL. |
[39] | Habib M , Joanette Y , Puel M ((1991) ) Déemences et syndromes démentiels. Approche neuropsychologique. Masson, Paris. |
[40] | Deloche G , Metz-Lutz MN , Kremin H , Hannequin D ((1986) ) Test de dénomination orale de 80 images du réseau de recherche Clinique. INSERM, Paris. |
[41] | Knopman DS , Nissen MJ ((1987) ) Implicit learning in patients with probable Alzheimer’s disease. Neurology 37: , 784–788. |
[42] | Arroyo-Anlló EM , Gil R , Esperet E , Pluchon C , Neau JP , Perea V ((1996) ) Apprentissage procédural de la catégorisation sémantique: Étude d’une population d’aphasiques. Rev Neuropsychol 3: , 309–327. |
[43] | Content A , Mousty PH , Radeau M ((1990) ) Une base de données lexicales informatisée pour le français écrit et parlé (BRULEX). Annee Psychol 90: , 551–566. |
[44] | Libon DJ , Bogdanoff B , Cloud BS , Skalina S , Giovannetti T , Gitlin HL ((1998) ) Declarative and procedural learning, quantitative measures of hippocampus, and subcortical white alterations in Alzheimer’s disease and ischemic vascular dementia. J Clin Exp Neuropsychol 20: , 30–41. |
[45] | Gabard J , Gil R , Arroyo-Anlló EM ((1996) ) Procédure informatique de la tâche procédurale sémantique, CHU, Poitiers. |
[46] | Grafton ST , Schmitt P , Van Horn J , Diedrichsen J ((2008) ) Neural substrates of visuomotor learning based on improved feedback control and prediction. Neuroimage 39: , 1383–1395. |
[47] | Rocca MA , Absinta M , Valsasina P , Ciccarelli O , Marino S , Rovira A , Gass A , Wegner C , Enzinger C , Korteweg T , Sormani MP , Mancini L , Thompson AJ , De Stefano N , Montalban X , Hirsch J , Kappos L , Ropele S , Palace J , Barkhof F , Matthews PM , Filippi M ((2009) ) Abnormal connectivity of the sensorimotor network in patients with MS: A multicenter fMRI study. Hum Brain Mapp 30: , 2412–2425. |
[48] | Wegner C , Filippi M , Korteweg T , Beckmann C , Ciccarelli O , De Stefano N , Enzinger C , Fazekas F , Agosta F , Gass A , Hirsch J , Johansen-Berg H , Kappos L , Barkhof F , Polman C , Mancini L , Manfredonia F , Marino S , Miller DH , Montalban X , Palace J , Rocca M , Ropele S , Rovira A , Smith S , Thompson A , Thornton J , Yousry T , Matthews PM ((2008) ) Relating functional changes during hand movement to clinical parameters in patients with multiple sclerosis in a multi-centre fMRI study. Eur J Neurol 15: , 113–122. |
[49] | Dagher A , Owen AM , Boecker H , Brooks DJ ((2001) ) The role of the striatum and hippocampus in planning: A PET activation study in Parkinson’s disease. Brain 124: , 1020–1032. |
[50] | Laforce RJ , Doyon J ((2001) ) Distinct contribution of the striatum and cerebellum to motor learning. Brain Cogn 45: , 189–211. |
[51] | Shin JC , Ivry RB ((2003) ) Spatial and temporal sequence learning in patients with Parkinson’s disease or cerebellar lesions. J Cogn Neurosci 15: , 1232–1243. |
[52] | Willingham DB ((1998) ) A neuropsychological theory of motor skill learning. Psychol Rev 105: , 558–584. |
[53] | Fincham JM , Carter CS , van Veen V , Stenger VA , Anderson JR ((2002) ) Neural mechanisms of planning: A computational analysis using event-related fMRI. Proc Natl Acad Sci U S A 99: , 3346–3351. |
[54] | Seger CA , Cincotta CM ((2005) ) The roles of the caudate nucleus in human classification learning. J Neurosci 25: , 2941–2951. |
[55] | Mancini L , Ciccarelli O , Manfredonia F , Thornton JS , Agosta F , Barkhof F , Beckmann C , De Stefano N , Enzinger C , Fazekas F , Filippi M , Gass A , Hirsch JG , Johansen-Berg H , Kappos L , Korteweg T , Manson SC , Marino S , Matthews PM , Montalban X , Palace J , Polman C , Rocca M , Ropele S , Rovira A , Wegner C , Friston K , Thompson A , Yousry T ((2009) ) Short-term adaptation to a simple motor task: A physiological process preserved in multiple sclerosis. Neuroimage 45: , 500–511. |
[56] | Covey TJ , Zivadinov R , Shucard JL , Shucard DW ((2011) ) Information processing speed, neural efficiency,and working memory performance in multiple sclerosis: Differential relationships with structural magnetic resonance imaging. J Clin Exp Neuropsychol 33: , 1129–1145. |
[57] | Jennekens-Schinkel A , Sanders EA , Lanser JB , Van der Velde EA ((1988) ) Reaction time in ambulant multiple sclerosis patients. Part. I. Influence of prolonged cognitive effort. J Neurol Sci 85: , 173–186. |
[58] | Cohen RA , Fisher M ((1989) ) Amantadine treatment of fatigue associated with multiple sclerosis. Arch Neurol 46: , 676–680. |
[59] | Bol Y , Duits AA , Hupperts RM , Verlinden I , Verhey FR ((2010) ) The impact of fatigue on cognitive functioning in patients with multiple sclerosis. Clin Rehabil 24: , 854–862. |
[60] | Hanken K , Eling P , Hildebrandt H ((2015) ) Is there a cognitive signature for MS-related fatigue? Mult Scler 21: , 376–381. |
[61] | Hobart J , Freeman J , Thompson A ((2000) ) Kurtzke scales revisited: The application of psychometric methods to clinical intuition. Brain 123: , 1027–1040. |