Dissecting Alzheimer’s Disease Risk in Asian American Elders: A Classification and Regression Tree Approach
Abstract
Background:
Alzheimer’s disease (AD) poses a growing public health challenge, particularly with an aging population. While extensive research has explored the relationships between AD, socio-demographic factors, and cardiovascular risk factors, a notable gap exists in understanding these connections within the Asian American elderly population.
Objective:
This study aims to address this gap by employing the Classification and Regression Tree (CART) approach to investigate the intricate interplay of socio-demographic variables, cardiovascular risk factors, sleep patterns, prior antidepressant use, and AD among Asian American elders.
Methods:
Data from the 2017 Uniform Data Set, provided by the National Alzheimer’s Coordinating Center, were analyzed, focusing on a sample of Asian American elders (n = 4,343). The analysis utilized the Classification and Regression Tree (CART) approach.
Results:
CART analysis identified critical factors, including levels of independence, specific age thresholds (73.5 and 84.5 years), apnea, antidepressant use, and body mass index, as significantly associated with AD risk.
Conclusions:
These findings have far-reaching implications for future research, particularly in examining the roles of gender, cultural nuances, socio-demographic factors, and cardiovascular risk elements in AD within the Asian American elderly population. Such insights can inform tailored interventions, improved healthcare access, and culturally sensitive policies to address the complex challenges posed by AD in this community.
INTRODUCTION
Alzheimer’s disease (AD) represents a distinctive form of dementia characterized by progressive memory loss and a decline in communication skills and responsiveness [1]. The escalating prevalence of AD has thrust it into the forefront of scientific inquiry, warranting concerted research efforts. The CDC highlights a concerning trajectory, revealing that approximately 5.8 million Americans were living with AD in 2020, with projections indicating a tripling of this number to nearly 14 million individuals by 2060 [1]. Of even more significant concern, this surge is particularly pronounced among minority older populations, with a focus on understanding AD within the context of Asian American older adults. Wong and colleagues [2] pointed to the under-researched nature of this demographic, their limited exposure to information about AD, and the dearth of culturally tailored services to cater to their needs. However, despite the rising prevalence, the challenge of achieving accurate and timely AD diagnoses persists. This critical gap can hinder the implementation of timely interventions that are most effective during the initial stages of the disease. An investigation into the factors influencing this diagnostic delay reveals pronounced disparities [3]. Recent studies highlight that Asian American older adults experience a higher AD burden than other racial groups [4]. This disparity is multifaceted, rooted in various socio-cultural and healthcare access factors.
As awareness of healthcare disparities grows, there is a need for additional research to understand the extent of this issue thoroughly. AD and race are interconnected, making examining specific variables related to this association and assessing their statistical significance essential [4].
A significant research gap becomes apparent within the aim of the current study, which seeks to explore the intricate association between socio-demographic variables, cardiovascular risk factors, antidepressant use, apnea, and AD among Asian American elders. While existing literature acknowledges the rising AD prevalence and disparities across racial groups, there is a distinct dearth of comprehensive investigations explicitly delving into the Asian American community. Predominantly centered on AD prevalence and risk factors among diverse populations, studies often amalgamate various ethnic groups into broader categories. This amalgamation overlooks unique socio-cultural, genetic, and environmental factors contributing to AD manifestation among Asian American older adults [4, 5]. Consequently, the current research body may need more granularity to comprehend the nuanced relationships between socio-demographic variables, cardiovascular risk factors, and AD within this racial group.
This study employs an advanced analytical technique, the CART approach, to fill this knowledge gap. It aims to untangle the intricate interplay between socio-demographic variables, cardiovascular risk factors, obstructive sleep apnea, prior antidepressant usage, and AD within the Asian American elderly population. By identifying crucial predictors and their intricate relationships, this research aspires to provide valuable insights. These insights inform the development of customized interventions, the shaping of policies, and the enhancement of healthcare strategies. Ultimately, this holistic approach aims to reduce the burden of AD within the often-underserved Asian American elderly community, aligning with the objectives of previous research [4, 5].
The aim of the study is to explore the intricate association between socio-demographic variables, cardiovascular risk factors, obstructive sleep apnea, prior antidepressant usage, and AD among Asian American elders. The study employs the CART approach to analyze these associations and provide insights that can inform the development of customized interventions, policy shaping, and the enhancement of healthcare strategies. This holistic approach aims to reduce the burden of AD within the often-underserved Asian American elderly community. The study’s objectives include identifying crucial predictors and their intricate relationships, thereby offering valuable insights for tailored interventions and culturally sensitive policy development to address the challenges posed by AD in this community.
Socio-demographic characteristics and AD
According to a previous study, a compelling gender-related disparity emerges, with women constituting more than half of the diagnosed AD cases [6]. Intriguingly, this discrepancy tends to materialize in older age groups, as AD rates for men and women remain comparable until age 79, after which women’s rates exhibit elevation. Nevertheless, much of the literature delving into AD and race predominantly focuses on elucidating specific biological and neurological underpinnings contributing to the divergence between genders.
A prevailing consensus within existing literature emphasizes AD as an age-associated cognitive disorder. With the worldwide growth of the elderly population, there has been a marked rise in cognitive disorders, including AD [7–9]. Future projections suggest a continued increase in AD cases, attributed to the global rise in life expectancy [7, 9, 10]. Data supports this projection, indicating that approximately 6.5 million Americans aged 65 and older were diagnosed with AD, with an expected growth in the number of older adults diagnosed with AD and related cognitive impairments [11–13]. Given the significant correlation between age and AD, it is crucial to conduct a detailed examination of age-related factors when studying AD. Age remains a pivotal factor intricately linked to AD, underscoring the need for its thorough examination.
The role of education as a demographic variable of interest garners substantial attention in the literature. Educational attainment emerges as a potential protective factor against AD and cognitive impairment, capable of mitigating the risk of its development or aiding in managing its symptoms [14–17]. The findings illustrate a consistent protective effect of education against the risk of developing AD, with a dose-dependent relationship prevailing across genders and age groups [16, 17]. Similarly, in a few studies, it was underscored that education may play a pivotal role in bolstering brain reserve and fortifying cognitive coping strategies, not just in healthy individuals but also in patients diagnosed with AD [18–20]. Notably, Asian Americans exhibit higher education levels, potentially attributed to immigration trends and the “model minority” stereotype, reinforcing the importance of studying this group in the context of AD [21].
The independence level constitutes another salient dimension to explore in AD and its trajectory. AD’s impact on functional capacity underscores its significance in assessing health status. Encouraging patients’ independence is vital, as observed in a study that demonstrated varying levels of dependence among AD-diagnosed or dementia-diagnosed patients [22–24]. In a few studies, assistive technologies can foster independence and autonomy, alleviating caregiver burden [25, 26]. Within the Asian American context, familial caregiving norms and “familial obligation” emphasize preserving independence, aligning with culturally embedded practices.
Cardiovascular risk factors and AD
AD has established intricate connections with cardiovascular risk factors, including smoking history, body mass index (BMI), and stroke history. While the impact of smoking history on AD remains debated, it is noteworthy that smoking is a globally pervasive habit recognized for its propensity to induce neurobiological and neurocognitive abnormalities. Such anomalies, including hippocampal volume loss and cognitive deficits, align with AD risk factors [8, 26, 27]. Strikingly prevalent worldwide, smoking’s associations with AD warrant scrutiny.
Asian Americans, constituting 7.0% of American smokers, are a demographic with notable smoking prevalence [14]. Further examination reveals a gender dichotomy, with 12.0% of Asian men smoking compared to 2.6% of Asian women [14]. This distinctive pattern underscores the importance of dissecting smoking’s influence on AD within the Asian American context, where gender and cultural nuances may interplay.
Similarly, BMI is a salient factor shaping AD risk. There is a correlation between BMI decline and heightened AD risk in later life [29–31]. One study attributed this relationship to compromised repair mechanisms leading to tissue dysfunction and subsequent weight loss [29]. However, the extent of this phenomenon within the Asian American population remains inadequately explored, signifying a research gap necessitating attention.
The association between stroke history and AD adds another layer of complexity. Stroke occurrences resulting from halted blood flow disrupt the oxygen supply to neurons, a phenomenon implicated in AD neuropathological alterations like brain atrophy and protein accumulation [16]. Astonishingly, Asian Americans confront unique challenges concerning stroke care, with disparities in outcomes and limited research attributed to barriers in care, education, and research [17]. This underscores the critical need to address stroke-related comorbidities within Asian American populations, where attention and intervention can potentially reshapeoutcomes.
Antidepressants and AD
The association between antidepressant use and AD has been examined in several studies. A group of researchers conducted a case-control study comparing the prevalence of depressive symptoms and antidepressant use in Chinese American and white subjects with and without cognitive impairment [18]. They found that Chinese American subjects with cognitive impairment were less likely to receive treatment for depression compared to white subjects with cognitive impairment, indicating potential disparities in antidepressant use in the context of cognitive impairment.
In another study, a complex case of progressive supranuclear palsy (PSP) accompanied by depression and anxiety, was presented. The patient showed extrapyramidal symptoms and major depression, leading to impaired movement [19]. Antidepressant therapy was initiated, resulting in a reduction of depressive symptoms and somatic complaints. While this study focused on PSP, it highlights the potential effectiveness of antidepressant treatment in improving emotional and cognitive symptoms in neurodegenerative diseases, including those with features of dementia.
One study investigated the relationship between depression and incident cognitive impairment progression across ethnic and racial groups [20]. They found that depression independently predicted a faster progression to incident cognitive impairment, with Hispanics and Asian participants having a higher progression hazard than non-Hispanic whites. While this study primarily focused on the relationship between depression and cognitive impairment, it indirectly suggests that the use of antidepressants, which is a common treatment for depression, may have implications for the risk of cognitive impairment.
Apnea and AD
Obstructive sleep apnea (OSA) has gained attention in recent research due to its potential association with AD. OSA is characterized by repeated episodes of interrupted breathing during sleep, leading to intermittent drops in oxygen levels and disrupted sleep patterns. Guay and colleagues conducted a systematic review and meta-analysis of cohort studies to investigate the association between sleep apnea and different etiologies of dementia, including AD [21]. The meta-analysis revealed that patients with sleep apnea had an increased risk of developing neurocognitive disorders, including AD, as indicated by hazard ratios. This finding suggests that sleep apnea may be a modifiable risk factor for dementia, particularly AD.
In a prospective cohort study, the focus was on patients with severe OSA requiring surgical treatment [22]. They measured AD biomarkers in the plasma of OSA patients and evaluated cognitive function and sleepiness. The study found significantly higher levels of AD biomarkers, including Ab40, t-tau, and p-tau, in OSA patients compared to healthy controls. However, after surgical intervention for OSA, plasma AD biomarker levels decreased significantly after uvulopalatopharyngoplasty. Moreover, cognitive function and sleepiness improved following surgery and were correlated with changes in AD biomarkers.
These findings collectively suggest that there may be a link between OSA and AD. OSA could contribute to increased AD biomarkers and potentially impact cognitive function. The studies highlight the importance of addressing OSA as a potential modifiable risk factor in AD prevention and management.
However, it is important to note that while these studies provide valuable insights, more research is needed to fully understand the complex relationship between OSA and AD, especially considering different populations and potential confounding variables. Additionally, the literature does not currently include specific studies on Asian Americans, indicating a need to address this gap in knowledge within diverse populations.
The literature review provides a comprehensive overview of factors associated with AD and reveals critical gaps in knowledge. Existing research highlights gender-related disparities, emphasizing that women constitute most AD cases, particularly in older age groups. Age is recognized as a significant factor, with AD closely associated with increasing age. Educational attainment, a potential protective factor, garners attention, especially in the context of Asian Americans with higher education levels. Independence level is a salient dimension for assessing AD’s impact on functional capacity. At the same time, cardiovascular risk factors, including smoking history, BMI, and stroke history, exhibit complex relationships with AD. Antidepressant use in AD and the potential link between OSA and AD are also explored, with some preliminary evidence suggesting associations.
Several research gaps emerge from the literature review. These gaps include a lack of focused studies on Asian American elderly adults, limited exploration of the nuanced relationship between apnea and AD, a need to understand smoking patterns and their gender disparities within this demographic, inadequate investigation into the role of BMI in AD among Asian Americans, insufficient attention to stroke-related comorbidities in this population, and gaps in understanding the implications of antidepressant use in AD. Furthermore, while there is emerging interest in the association between OSA and AD, especially in diverse populations, more research is needed to comprehensively understand this relationship and its potential modification in different ethnic groups.
To address these recognized gaps, this study leverages advanced analytical techniques, such as the CART approach, to examine the intricate interactions between socio-demographic variables, cardiovascular risk factors, sleep behavior, past antidepressant history, and AD, specifically within the Asian American elderly population. By narrowing the focus to this unique demographic, the research aims to provide insights into disparities and risk factors relevant to Asian American elderly adults. The study’s nuanced exploration of the role of apnea in AD, the analysis of unique smoking patterns and gender disparities, the investigation of BMI’s relationship with AD in this population, and the emphasis on stroke-related comorbidities align with the identified gaps in the literature. Additionally, the study contributes to the emerging field of OSA and AD research, shedding light on this relationship and its potential implications for diverse populations.
MATERIALS AND METHODS
Study sample
We used the 2017 Uniform Data Set (UDS) from the National Alzheimer’s Coordinating Center (NACC; naccdata.org). The NACC data includes clinical data from participants across 39 past and present AD research centers in the United States. All contributing AD research centers were required to obtain approvals from their respective institutional regulatory boards and informed consent from their participants before submitting data to the NACC.
For the current study, the sample consisted of 4343 Asian American elders. The distribution of gender in the sample was approximately balanced, with a slightly higher percentage of females (58.2%) compared to males (41.8%). The mean age of the participants was consistent with the older adult population (Mean = 73.61) with a relatively large standard deviation (10.38) indicating some variability in the participants’ age. The average years of education in the sample were 16.29 years, suggesting a relatively well-educated cohort. Regarding the level of independence, 3031 participants (69.8%) were classified as independent, while 1288 participants (29.8%) were classified as dependent.
Measures
In our study, the dependent variable is AD status, determined by the following response values: 0=“No,” 1 = “Yes,” and 8=“No cognitive impairment.” However, for our analysis, we treated response 8, “No cognitive impairment,” as “No,” the same as the category represented by 0=“No.” A diagnosis of AD was confirmed by a consensus team or physician using the results of a structured clinical history, neuropsychology testing, and validated assessments of symptoms and function. Dementia of the AD type was diagnosed according to the criteria set by the National Institute of Neurological and Communicative Disorders and Stroke and the Alzheimer’s Disease and Related Disorders Association prior to 2015. Post-2015, the diagnostic criteria from the National Institute on Aging and the Alzheimer’s Association were applied. Participants were diagnosed with AD if it was the primary or contributing cause of cognitive impairment, which included participants who had probable or possible dementia of the AD type.
Socio-demographic characteristics include gender (1 = “Male” and 2 = “Female”), age (in years), education (in years), and independence in activities of daily living. In this study, the age variable represents the age at first assessment for all participants, both cases and controls, due to the unavailability of uniform data on the exact age of diagnosis of AD for the cases. This approach provides a standardized baseline for comparison across the dataset. However, it is acknowledged as a limitation compared to using age at diagnosis for cases and age at last visit for controls. Participants’ level of independence was assessed using the following response options: 1 = “Able to live independently,” 2 = “Requires some assistance with complex activities,” 3 = “Requires some assistance with basic activities,” and 4 = “Completely dependent.” In the analysis, we grouped categories 2, 3, and 4 as “Dependent life,” while category 1 was designated as “Independent life.”
Cardiovascular risk factors include smoking history, BMI, and stroke history. Smoking history was quantified in terms of years, spanning from 0 to 87. BMI values ranged from 10 to 100. Regarding stroke history, the response categories were as follows: 0 = “Absent,” 1 = “Recent/Active,” and 2 = “Remote/Inactive.” For analytical purposes, categories 1 and 2 were combined into a single category labeled “Yes.”
Regarding the use of antidepressants, participants had the following response options: 0 = “No,” 1 = “Yes.” As for apnea, the response categories were as follows: 0 = “Absent,” 1 = “Recent/Active,” 2 = “Remote/Inactive.” For analytical purposes, response categories 2 and 3 were combined into a single category labeled “Yes.”
Data analysis
Chi-square tests for categorical variables: Initially, we employed chi-square tests to assess the associations between each categorical predictor variable and the binary outcome variable, denoting AD status (0 = No, 1 = Yes). This step was crucial for discerning any significant relationships or dependencies among these variables.
Independent t-tests for continuous variables: For continuous variables such as age and BMI, we utilized independent t-tests. This approach enabled us to identify statistically significant differences in these variables between individuals with and without AD.
Multivariate analysis using CART: Subsequently, we embarked on a more advanced multivariate analysis using the CART algorithm. In choosing the CART, we aimed to employ a tool that aligns with the specific research objectives of our study. CART is a robust and interpretable machine learning technique that identifies complex, non-linear relationships within data. It is well-suited for our exploration of AD susceptibility in the context of Asian American older adults. We acknowledge that various statistical and machine learning approaches are available for data analysis, and the nature of the research question and dataset should guide the selection of a particular method. In our case, the CART method was chosen due to its ability to reveal intricate interactions among predictors and its suitability for creating easily interpretable decision trees. While we recognize the value of other approaches, adopting CART was motivated by our aim to uncover vulnerable subgroups and provide insights tailored to our study population. Our study employed Minitab 23 for CART analysis, focusing on AD in Asian American elders. Minitab, known for its robust data analysis capabilities, allowed us to approach the analysis with methodological rigor. We maintained equal prior probabilities for all classes and used the Gini index to split nodes. The minimum misclassification cost determined the optimal tree, validated through 10-fold cross-validation. Our analysis utilized 4,343 rows, identifying eight important predictors out of nine, leading to seven terminal nodes. We emphasized transparency and accuracy by providing detailed performance metrics like log-likelihood, AUC, lift, misclassification cost, and a confusion matrix highlighting key rates for training and test datasets.
Handling of collinearity in CART analysis: Although collinear variables can distort results in linear models, CART independently evaluates each predictor’s contribution to the model’s predictive power at every split. This unique approach allows CART to navigate collinear relationships among variables [32–34]. However, we conducted a multicollinearity analysis using traditional regression to provide additional assurance and transparency. The results of this analysis indicate that multicollinearity is not a significant concern in our dataset, as variance inflation factor (VIF) values for all predictor variables were well within acceptable limits (typically VIF < 10). Therefore, collinearity did not unduly influence our CART analysis results. This inherent handling of collinearity underscores the robustness of CART in analyzing complex datasets where collinearity is common.
Computational aspects of the study: Our study utilized Minitab 23 software, run on a computing system equipped with Intel i9, 16GB RAM, and operating on Windows 10. The CART algorithm, while robust, is known for its computational efficiency, especially in handling medium-sized datasets like ours. The algorithm’s complexity is primarily influenced by the number of predicting variables and the size of the dataset. Our analysis involved nine predicting variables and a dataset comprising 4,343 rows, which falls within the manageable range for CART analysis in Minitab. One of the computational challenges faced was ensuring efficient handling of the dataset without compromising the system’s performance. To mitigate this, we optimized the data by removing irrelevant variables and streamlined the analysis process by focusing on key predictors. No significant software limitations impacted the analysis.
RESULTS
Bivariate analysis
Table 1 provides compelling evidence of significant associations between AD and various sociodemographic variables. Notably, gender exhibited a significant association (χ2 = 7.48, p < 0.01), suggesting that gender may play a role in influencing susceptibility to AD. Similarly, age emerged as a crucial factor, showing a significant correlation (χ2=–19.88, p < 0.001), emphasizing the pivotal role of age in the prevalence of AD. As an indicator of cognitive enrichment, years of education showed a robust relationship with AD (t = 9.04, p < 0.001), underscoring its potential as a protective factor against the disease. Independence level, reflecting functional autonomy, demonstrated a profound linkage with AD (χ2 = 1,223.75, p < 0.001). This result aligns with the understanding that AD significantly affects functional capacity, making this variable essential in assessing disease progression. Years of smoking, a potentially modifiable behavior, displayed a notable relationship with AD (t=–2.52, p < 0.001). This connection highlights the intricate interplay between lifestyle choices and the risk of developing AD. Conversely, BMI exhibited a significant inverse association with AD (t=–3.33, p < 0.001), suggesting that body composition may play a role in influencing disease susceptibility. Furthermore, there is a significant association between antidepressant use and AD (χ2 = 170.1, p < 0.001). However, no significant association was found between AD and stroke history or apnea. This unexpected finding sheds light on the complex nature of AD’s multifactorial etiology, hinting at distinct pathways contributing to its development.
Table 1
Characteristics of demographic and independent variables
Variables | No AD | Yes AD | χ2 or t-value |
Sex | |||
Male | 1,151 (40.4) | 666 (44.7) | 7.48** |
Female | 1,701 (59.6) | 825 (55.3) | |
Total | 2,852 (100) | 1,491 (100) | |
Age | 71.44 (9.88) | 77.76 (10.06) | –19.88*** |
Years of education | 16.61 (2.99) | 15.67 (3.67) | 9.04*** |
Independence level | |||
Independence | 2,494 (87.7) | 537 (36.4) | 1,223.75*** |
Dependence | 349 (12.3) | 939 (63.6%) | |
Total | 2,843 (100) | 1,476 (100) | |
Years of smoking | 4.93 (10.98) | 6.14 (13.28) | –2.52*** |
BMI | 24.11 (3.75) | 23.30 (3.54) | 5.98*** |
Antidepressant | |||
Yes | 315 (11.3) | 399 (26.9) | 170.1*** |
No | 2,474 (88.7) | 1,082 (73.1) | |
Apnea | |||
Yes | 46 (13.7) | 22 (14.5) | 0.05 |
No | 289 (86.3) | 130 (85.5) | |
Stroke history | |||
Yes | 1,213 (95.8) | 748 (94.6) | 1.71 |
No | 53 (4.2) | 43 (5.4%) | |
Total | 1,266 (100) | 791 (100) |
AD, Alzheimer’s disease; BMI, body mass index. *p≤0.05; **p≤0.01; ***p≤0.001.
We employed a significance level (alpha) of 0.05 for our statistical tests in this study. This choice of alpha represents a standard threshold for statistical significance in hypothesis testing and is widely accepted in social science research. We considered p-values less than 0.05 statistically significant, indicating strong evidence against the null hypothesis.
Decision tree results
Our study employed Minitab 23 for CART analysis and emphasized accuracy and transparency by detailing comprehensive performance metrics. The average log-likelihood values were observed at 0.4855 for the training set and 0.4921 for the test set, suggesting that the model provides a reasonably good fit to the data, with a marginally better performance on the training set. The area under the ROC curve (AUC) was 0.8031 in the training set and 0.7912 in the testing set, indicating a strong ability of the model to distinguish between cases with and without AD. These values reflect a good discriminative capacity, essential in medical or diagnostic models. Furthermore, our model demonstrated a true positive rate (sensitivity) of 71.9% in training and 69.3% in testing, implying that it correctly identified approximately 70% of AD cases. The true negative rate (specificity) stood at 81.9% in training and 82.6% in testing, indicating a robust capability for identifying individuals without AD. These metrics collectively suggest that our CART model achieves a good balance between sensitivity and specificity, making it effective in predicting AD among Asian American elders. The slight variation between the training and testing metrics implies that the model generalizes well to new data, minimizing the risk of overfitting. Overall, the performance of our model is indicative of its practical utility in a clinical setting, offering valuable insights for the early detection and managementof AD.
The CART model, aimed at identifying key factors associated with AD in Asian American elders, is represented in Fig. 1. The model’s root node indicates 34.3% of respondents with AD and 65.7% do not. This root node led to seven levels of node splits, illustrating the decision-making process of the model. The initial split of all respondents was based on their level of independence in life. Of those participants who reported that they were able to live independently, 18% (n = 547) reported having AD, whereas among those participants who reported living dependently, 72.7% (n = 944) reported having AD. This is the first and most significant factor in relation to AD among Asian American elderly people.
Fig. 1
Classification and Regression Tree of Alzheimer’s Disease among Asian Americans. rINDEPEND, level of independence; NACCAGE, subject’s age; APNEA, sleep apnea history; NACCADEP, use of antidepressant; NACCBMI, body mass index.
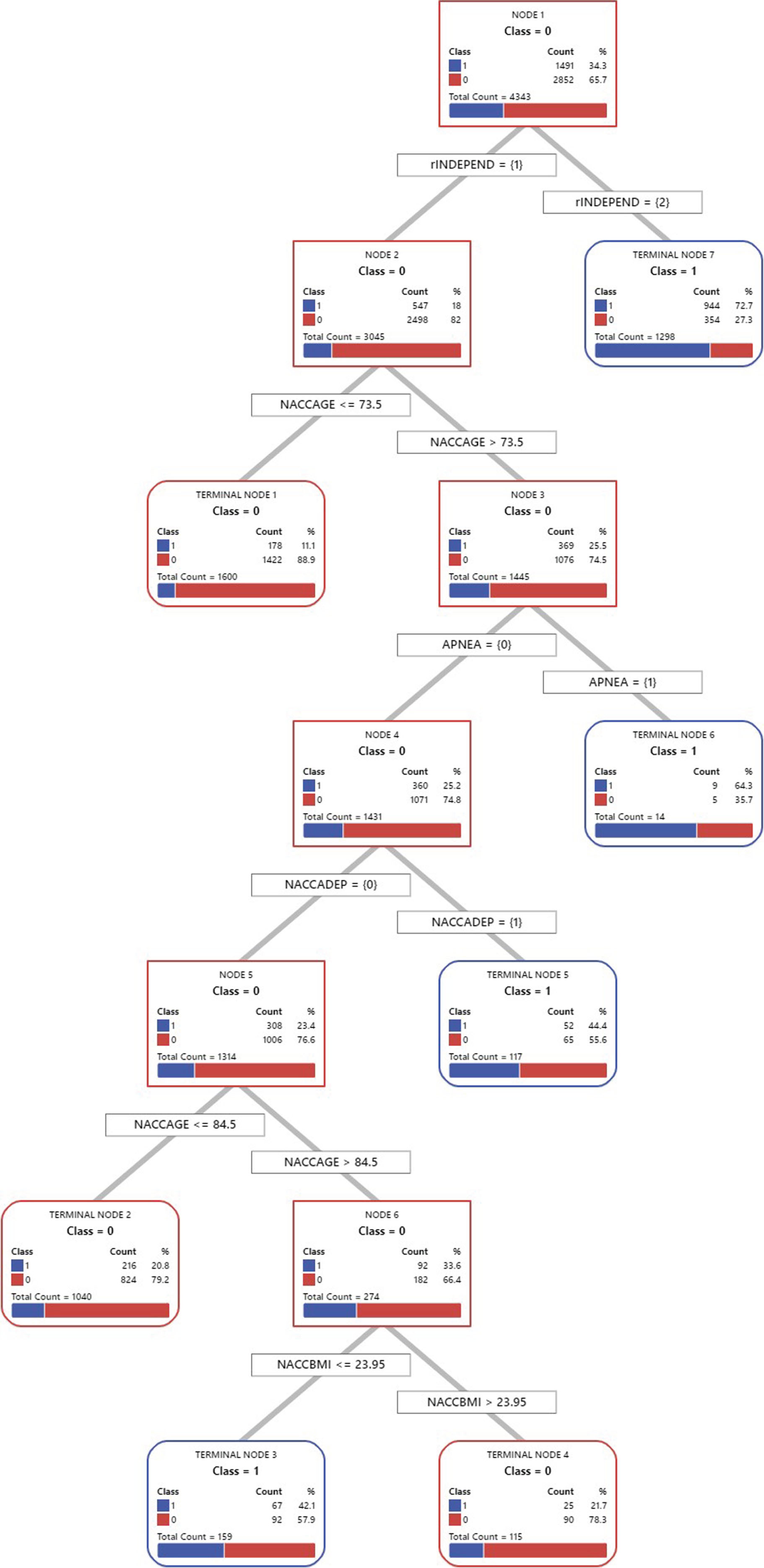
The second split was based on age. Among participants who reported living independently and whose age was less than or equal to 73.5 years, 11.1% (n = 178) reported having AD, while of those participants who reported that their age was greater than 73.5, 25.5% (n = 369) reported having AD, which branched down to the right. These results indicate that 73.5 years is a kind of yardstick related to AD. It is important to note that while 73.5 years serves as a useful reference point, the choice of cutoffs in CART analysis is data-driven and intended to identify meaningful subdivisions in the data. In our case, this age threshold effectively distinguished two groups with significantly different AD risks, facilitating a more precise understanding of age-related AD susceptibility within the Asian American elderly population.
The branch to the right was based on apnea. Of those respondents reporting without apnea, 25.2% (n = 360) reported having AD, which branched down to the left. However, in the case of respondents who reported having apnea, 64.3% (n = 9) reported having AD. These results suggest that apnea is an important factor in relation to AD among Asian American elderly people.
The fourth split was divided based on the use of antidepressants. Of those participants who reported using an antidepressant, 44.4% (n = 52) reported having AD, while among those respondents reporting not using an antidepressant, 23.4% (n = 308) reported having AD, which branched to the left. These results indicate that the use of an antidepressant is related to AD.
The fifth split was based on the age of 84.5 years. Of those respondents whose age was less than or equal to 84.5 years, 20.8% (n = 216) reported having AD, whereas among the participants whose age was greater than 84.5 years, 33.6% (n = 92) reported having AD, which branched to the right. Based on these results, the age of 84.5 years is another touchstone related to AD.
The final split was divided based on the BMI of 23.95. Among the participants whose BMI was less than or equal to 23.95, 42.1% (n = 67) reported having AD, while of those respondents whose BMI was greater than 23.95, 21.7% (n = 25) reported having AD. The two branches resulted in the terminal nodes. These results indicate that BMI is a significant factor related to AD.
Figure 1 also illuminates the distinct vulnerability profiles for AD within our study population. It is evident that various combinations of predictors contribute to varying levels of susceptibility to AD. The most vulnerable group, identified in Terminal Node 7, comprises individuals living dependently, among whom a striking 72.7% reported AD. Following closely, the second vulnerable group, situated in Terminal Node 6, consists of those living independently, aged over 73.5, and affected by apnea, with 64.3% reporting AD. Notably, the third vulnerable group, found in Terminal Node 5, encompasses individuals living independently, aged over 73.5, without apnea, but with a history of antidepressant use, with 44.4% reporting AD. Lastly, the fourth vulnerable group, residing in Terminal Node 3, encompasses those living independently, aged between 73.5 and 84.5, without apnea, without a history of antidepressant use, and with a BMI less than 23.95, among whom 42.1% reported AD.
Lastly, the CART reports “variable importance scores” to determine variable selection. The variable with a score of 100 is indicated as the most influential independent variable for predicting AD, followed by other variables based on their relative importance. Based on these variable importance scores, level of independence was the most influential variable (100%), followed by age (34%), the use of an antidepressant (16.1%), years of education (4.8%), BMI (3.7%), apnea (3.5%), and sex (1%) (see Table 2).
Table 2
Relative Variable Importance
Variables | Relative Importance (%) |
Level of Independence | 100 |
Age | 34 |
Use of Antidepressant | 16.1 |
Years of Education | 4.8 |
Body Mass Index | 3.7 |
Apnea | 3.5 |
Sex | 1.0 |
Years of Smoking | 0 |
DISCUSSION
This study comprehensively analyzed factors associated with AD among Asian American elderly adults, employing a CART model. The investigation unveiled several key factors significantly linked to the likelihood of AD development in this population.
First and foremost, the level of independence in daily life emerged as the most influential factor concerning AD risk. Participants reporting living dependently faced a substantially higher AD risk, with a prevalence rate of 72.7%, in stark contrast to a significantly lower rate of 18% among those living independently (see Fig. 1). These findings show the intricate interplay between functional independence and AD. While AD is traditionally characterized by cognitive decline [1], our results hint at the level of independence as a potential precursor or marker of the disease [22].
Age proved to be another pivotal factor. An age threshold of 73.5 years was identified, and participants older than this threshold were more likely to report AD (25.5%) compared to their younger counterparts (11.1%). Subsequently, another age-related threshold emerged at 84.5 years, with participants older than this threshold exhibiting a higher risk of AD (33.6%) compared to those younger (20.8%).
The presence of apnea was found to be a crucial AD risk factor. Participants without apnea had a lower likelihood of AD (25.2%), while those with apnea faced a substantially higher risk (64.3%). Additionally, the use of antidepressants was associated with a higher risk of AD, with 44.4% of users reporting the condition, compared to 23.4% among non-users. These findings emphasize the multifaceted nature of AD risk factors.
Moreover, this study focuses on the often-underrepresented demographic of Asian American elderly adults, offering unique insights into potential disparities and risk factors relevant to this group. One distinctive finding is the nuanced exploration of apnea’s role in AD among Asian Americans. While traditional bivariate analysis failed to reveal a significant association, a more sophisticated CART analysis identified apnea as a crucial AD risk factor, shedding light on the complex relationship between the two.
The study also highlights unique smoking patterns among Asian Americans, particularly gender disparities. Notably, 12.0% of Asian men smoke compared to only 2.6% of Asian women, challenging conventional understandings of smoking’s influence on AD. This underscores the importance of conducting gender-specific analyses within this demographic.
Furthermore, the investigation into the association between BMI and AD risk adds complexity to existing literature. Unlike some previous studies highlighting the role of BMI in AD risk, our study did not provide conclusive evidence of this association within the Asian American population. This suggests that the relationship between BMI and AD may not be uniform across all demographic groups, emphasizing the need for further research.
The study’s attention to stroke-related comorbidities within Asian American populations offers a unique perspective. This emphasis highlights the critical need to address stroke-related issues within this population, potentially reshaping outcomes, and care strategies.
Furthermore, the exploration of antidepressant uses in the context of AD builds on existing research, emphasizing the potential role of antidepressant treatment in managing emotional and cognitive symptoms among Asian Americans with neurodegenerative diseases. This underscores the importance of considering mental health interventions and treatments in the context of AD within this demographic, highlighting the need for comprehensive care approaches addressing cognitive and emotional well-being.
Finally, the results depicted in Fig. 1 offer valuable insights into the complex landscape of AD vulnerability within our study population of Asian American older adults. It is abundantly clear that the risk of AD is not solely determined by individual factors but rather by intricate interactions among predictors. These findings highlight the need for a more nuanced understanding of AD risk assessment and underscore the importance of considering multifaceted relationships among predictors in this demographic. The most vulnerable group, as identified in Terminal Node 7, consists of individuals living dependently, with an alarming 72.7% reporting AD. This underscores the critical role of independence in daily life as a primary marker of AD susceptibility. However, the interactions between independence and other factors must be considered. The second vulnerable group in Terminal Node 6 includes individuals living independently, aged over 73.5, and affected by apnea, with 64.3% reporting AD. This finding emphasizes the significance of considering age and sleep-related factors when assessing AD risk. The third vulnerable group, found in Terminal Node 5, comprises individuals living independently, aged over 73.5, without apnea, but with a history of antidepressant use, with 44.4% reporting AD. This highlights the potential impact of mental health treatments in the context of AD risk, warranting further investigation into the interplay between antidepressant use and other risk factors. The fourth vulnerable group, residing in Terminal Node 3, encompasses those living independently, aged between 73.5 and 84.5, without apnea, without a history of antidepressant use, and with a lower BMI, among whom 42.1% reported AD. This suggests that the intricate interplay of factors can substantially elevate AD susceptibility, even within a seemingly lower-risk group. These findings emphasize the need for a holistic approach to AD risk assessment within the Asian American elderly population. Recognizing the interactions among predictors can enhance risk stratification, guide early detection efforts, and inform tailored interventions to mitigate the impact of AD in this community.
Our study’s findings on AD among Asian American elders align with recent research emphasizing the critical role of socio-demographic factors and health conditions in AD risk. Consistently with Wong et al. [2], we observe limited exposure to AD information within this community, underscoring the need for culturally tailored healthcare services. Our results also resonate with Keohane et al. [3], highlighting disparities in AD diagnoses across different racial groups. However, our study uniquely identifies specific age thresholds, apnea, antidepressant use, and BMI as key factors linked to AD risk in Asian Americans, expanding the current understanding within this demographic. Contrasting with some existing studies, our findings did not strongly correlate stroke history with AD, suggesting the variability of AD’s risk factors across different ethnic populations. This points to the need for further research on the intricate relationships between these variables, specifically within the Asian American older population.
Future directions and application in practice and policy
The insights from our CART model present several avenues for future research and practical application in the medical field. One significant direction involves the integration of our model into clinical decision-support systems. By incorporating our predictive model, healthcare providers can more accurately identify individuals at higher risk of AD, particularly within the Asian American elderly population. This could facilitate early intervention strategies, tailored patient education, and targeted screening programs. Additionally, future research could refine the model by including a broader range of variables, such as genetic markers or environmental factors, to enhance its predictive accuracy.
Another promising direction is using our model as a tool for personalized medicine. By understanding the specific risk factors and their interactions in predicting AD, clinicians can develop more customized care plans that consider the unique risk profiles of individual patients. This individualized approach could lead to better patient outcomes and more efficient use of healthcare resources.
The findings of our study also have significant implications for healthcare policy, especially in addressing the needs of the aging Asian American population. Policymakers can use the insights from our model to develop targeted health promotion campaigns and preventive strategies aimed at reducing the prevalence of AD in this demographic. Moreover, our study highlights the need for policies that support the integration of advanced predictive analytics in healthcare settings, which can lead to more proactive and preventive healthcare strategies.
Furthermore, the study underscores the importance of considering cultural and demographic factors in healthcare policy formulation. Policies that support research into specific minority groups, like Asian American elders, can lead to more equitable healthcare outcomes and address disparities in healthcare access and treatment. By tailoring healthcare policies to the needs of diverse populations, policymakers can ensure more inclusive and effective healthcare systems.
Study limitations
While this study contributes valuable insights into the associations between socio-demographic variables, cardiovascular risk factors, and AD among Asian American older adults, several limitations should be considered when interpreting the findings.
First, the cross-sectional design employed in this study still needs to be revised to establish causal relationships between predictor variables and AD, even though the CART analysis provided a more nuanced understanding of the factors influencing AD. The inability to track changes over time restricts our knowledge of the temporal sequence of events and hinders the establishment of causality. Longitudinal data would be more appropriate for exploring the dynamic relationships identified by the CART analysis and confirming the potential predictors of AD development among Asian American older adults.
Second, we identified a limitation regarding the use of age data. Specifically, the age variable in our analysis denotes the age at first assessment for all participants, rather than the age at AD diagnosis for cases or the age at the last visit for controls. This approach was necessitated due to the lack of uniformly available data on the exact age of AD diagnosis for our cases. While using the age at first assessment ensures a consistent and standardized comparison across the dataset, it may not fully capture the nuances that the age of diagnosis or last visit age could provide. We acknowledge this as a limitation of our study, as it might affect the interpretation and generalizability of our findings. Future research could benefit from incorporating these specific age metrics to yield different insights into the progression and risk factors associated with AD.
Third, despite the CART analysis, this study still relies on self-reported measures, including smoking history and stroke history. Self-reported data might introduce recall bias, as participants could underreport or misreport their behaviors or medical history due to memory lapses or social desirability. This limitation might affect the accuracy of the associations observed between these variables and AD. The potential bias in reporting could continue to contribute to discrepancies between the reported and actual prevalence of risk factors.
Fourth, while the CART analysis offered a more sophisticated examination of the data, it did not eliminate the possibility of omitted or unmeasured confounding variables that might have influenced the observed associations between predictor variables and AD. Factors such as socioeconomic status, genetic predisposition, and environmental exposures, which were not included in the CART analysis, could still confound the relationships. The inability to account for all possible confounders, even with CART analysis, might limit the accuracy of our estimates of the associations.
Fifth, we employed Minitab for statistical analysis, valuing its strengths in handling complex datasets and its user-friendly interface. While Minitab is proficient in many statistical procedures, it’s important to note its limitations in advanced machine learning tasks, particularly in hyperparameter optimization for tree-based models. Hyperparameter optimization is pivotal in refining machine learning models, ensuring they are robust and accurately tuned to the dataset. This process is critical in preventing overfitting, especially with tree-based models inherently prone to this issue if not carefully calibrated. The inability to perform hyperparameter optimization in Minitab means that our models were built with default or manually selected parameters, which might not represent the optimal settings. This limitation raises concerns about the potential overfitting of our models, which could impact the generalizability and validity of our findings. While we took measures to mitigate this risk through careful model selection and validation techniques, the absence of a formal optimization process might limit the precision of our predictive modeling. We acknowledge this as a constraint of our study and suggest that future research, aiming to build upon our findings, consider utilizing more specialized machine learning software capable of extensive hyperparameter tuning. This would enhance the model’s accuracy and reliability, providing a more robust foundation for understanding and addressing the complexities of AD in Asian American elders.
Finally, the observed relationships between predictor variables and AD, as the CART analysis reveals, do not necessarily imply causality. For instance, while the CART analysis identified independence level as a significant factor, it is still plausible that AD progression itself could lead to a decline in independence, and the CART analysis does not address causality directly. The complex bidirectional relationship between AD and specific predictors, such as independence level, continues to warrant caution in interpreting the direction of causality, emphasizing the need for further longitudinal research.
AUTHOR CONTRIBUTIONS
Sung Seek Moon (Conceptualization; Data curation; Formal analysis; Methodology; Supervision; Validation; Writing – original draft); Lindsey Anderson (Conceptualization; Data curation; Writing – review & editing); Jinyu Lee (Conceptualization; Data curation; Resources; Visualization; Writing – review & editing); Jinwon Lee (Data curation; Software; Visualization; Writing – review & editing); Youngkwang Moon (Conceptualization; Data curation; Formal analysis; Resources; Writing – review & editing).
ACKNOWLEDGMENTS
The NACC database is funded by NIA/NIH Grant U24 AG072122. NACC data are contributed by the NIA-funded ADRCs: P30 AG062429 (PI James Brewer, MD, PhD), P30 AG066468 (PI Oscar Lopez, MD), P30 AG062421 (PI Bradley Hyman, MD, PhD), P30 AG066509 (PI Thomas Grabowski, MD), P30 AG066514 (PI Mary Sano, PhD), P30 AG066530 (PI Helena Chui, MD), P30 AG066507 (PI Marilyn Albert, PhD), P30 AG066444 (PI John Morris, MD), P30 AG066518 (PI Jeffrey Kaye, MD), P30 AG066512 (PI Thomas Wisniewski, MD), P30 AG066462 (PI Scott Small, MD), P30 AG072979 (PI David Wolk, MD), P30 AG072972 (PI Charles DeCarli, MD), P30 AG072976 (PI Andrew Saykin, PsyD), P30 AG072975 (PI David Bennett, MD), P30 AG072978 (PI Neil Kowall, MD), P30 AG072977 (PI Robert Vassar, PhD), P30 AG066519 (PI Frank LaFerla, PhD), P30 AG062677 (PI Ronald Petersen, MD, PhD), P30 AG079280 (PI Eric Reiman, MD), P30 AG062422 (PI Gil Rabinovici, MD), P30 AG066511 (PI Allan Levey, MD, PhD), P30 AG072946 (PI Linda Van Eldik, PhD), P30 AG062715 (PI Sanjay Asthana, MD, FRCP), P30 AG072973 (PI Russell Swerdlow, MD), P30 AG066506 (PI Todd Golde, MD, PhD), P30 AG066508 (PI Stephen Strittmatter, MD, PhD), P30 AG066515 (PI Victor Henderson, MD, MS), P30 AG072947 (PI Suzanne Craft, PhD), P30 AG072931 (PI Henry Paulson, MD, PhD), P30 AG066546 (PI Sudha Seshadri, MD), P20 AG068024 (PI Erik Roberson, MD, PhD), P20 AG068053 (PI Justin Miller, PhD), P20 AG068077 (PI Gary Rosenberg, MD), P20 AG068082 (PI Angela Jefferson, PhD), P30 AG072958 (PI Heather Whitson, MD), P30 AG072959 (PI James Leverenz, MD).
FUNDING
The authors have no funding to report.
CONFLICT OF INTEREST
The authors have no conflict of interest to report.
DATA AVAILABILITY
The research was conducted using data from the 2017 Uniform Data Set (UDS), provided by the National Alzheimer’s Coordinating Center (NACC). This comprehensive dataset is publicly available and can be accessed through the NACC’s website at naccdata.org.
REFERENCES
[1] | Centers for Disease Control and Prevention. Alzheimer’s disease Accessed August 18, 2023, from https://www.cdc.gov/aging/aginginfo/alzheimers.htm |
[2] | Wong JJ , Min D , Kranick J , Ushasri H , Trinh-Shevrin C , Kwon S ((2022) ) Exploring community knowledge, attitudes and perceptions of Alzheimer’s disease/Alzheimer’s disease-related dementias and healthy ageing in Asian American, Native Hawaiian and Pacific Islanders. Health Soc Care Community 30: , 5946–5958. |
[3] | Miles A ((2017) ) The psychological implications of diagnostic delay in colorectal cancer patients. In Timely diagnosis of symptomatic cancer: Colorectal cancer, Olsson L, ed. Springer, pp. 103–119. |
[4] | Keohane LM , Nikpay S , Braun K , Cheng A , Stevenson DG , Buntin MB , Yu D , Blot WJ , Lipworth L ((2022) ) Association of race and income with incident diagnosis of Alzheimer’s disease and related dementias among black and white older adults. J Appl Gerontol 42: , 898–908. |
[5] | Fitten LJ , Ortiz F , Pontón MO ((2001) ) Frequency of Alzheimer’s disease and other dementias in a community outreach sample of Hispanics. J Am Geriatr Soc 49: , 1301–1308. |
[6] | Avila-Rieger JF ((2023) ) Intersectionality between sex/gender and race/ethnicity on cognitive trajectories and the development of Alzheimer’s disease. Dissertation Abstracts International: Section B: The Sciences and Engineering, Vol. 84, Issue 7-B. Retrieved from ProQuest Information & Learning. (Accession No. xri:pqdiss:13858556) |
[7] | Terry AV , Callahan PM , Hall BJ , Webster SJ ((2011) ) Alzheimer’s disease and age-related memory decline (preclinical). Biochem Behav 99: , 190–210. |
[8] | Durazzo TC , Mattsson N , Weiner MW ((2014) ) Smoking and increased Alzheimer’s disease risk: A review of potential mechanisms. Alzheimers Dement 10: , 122–145. |
[9] | Sengoku R ((2019) ) Aging and Alzheimer’s disease pathology. Alzheimers Dement 40: , 22–29. |
[10] | Chambers-Richards T , Chireh B , D’Arcy C ((2022) ) Trends in prevalence of self-reports of Alzheimer’s disease/dementia among non-institutionalized individuals 45+in Canada, 1994–2014. J Public Health Res 11: , 22799036221135221. |
[11] | Gillis C , Montenigro PH , Nejati M , Maserejian NN ((2022) ) Estimating prevalence of early Alzheimer’s disease in the United States, accounting for racial and ethnic diversity. Alzheimers Dement 19: , 1841–1848. |
[12] | ((2022) ) 2022 Alzheimer’s disease facts and figures. Alzheimers Dement 18: , 700–789. |
[13] | Lee TW , Chung J , Song KJ , Ji Y ((2023) ) Prevalence and associated factors of cognitive frailty in community-dwelling older adults: Results from the Korean longitudinal study of aging. Int J Older People Nurs 18: , e12576. |
[14] | Livingston G , Huntley J , Sommerlad A , Ames D , Ballard C , Banerjee S , Brayne C , Burns A , Cohen-Mansfield J , Cooper C , Costafreda SG , Dias A , Fox NC , Gitlin LN , Howard R , Kales HC , Kivimäki M , Larson EB , Ogunniyi A , Orgeta V , Ritchie K , Rockwood K , Sampson EL , Samus QM , Schneider LS , Selbæk G , Teri L , Mukadam N ((2020) ) Dementia prevention, intervention, and care: 2020 report of the Lancet Commission. Lancet 396: , 413–446. |
[15] | Sugden K , Moffitt TE , Arpawong TE , Arseneault L , Belsky DW , Corcoran DL , Crimmins EM , Hannon E , Houts R , Mill J , Poulton R , Ramrakha S , Wertz J , Williams B , Caspi A ((2023) ) Cross-national and cross-generational evidence that educational attainment may slow the pace of aging in European-Descent individuals. J Gerontol B Psychol Sci Soc Sci 78: , 1375–1385. |
[16] | Sando SB , Melquist S , Cannon A , Hutton M , Sletvold O , Saltvedt I , White LR , Lydersen S , Aasly J ((2008) ) APOE ɛ4 lowers age at onset and is a high risk factor for Alzheimer’s disease; A case control study from central Norway. BMC Neurol 8: , 9. |
[17] | Sando SB , Melquist S , Cannon A , Hutton M , Sletvold O , Saltvedt I , White LR , Lydersen S , Aasly J ((2008) ) Risk-reducing effect of education in Alzheimer’s disease. Int J Geriatr Psychiatry 23: , 1156–1162. |
[18] | Liu Y , Julkunen V , Paajanen T , Westman E , Wahlund L , Aitken AP , Sobów T , Mecocci P , Tsolaki M , Vellas B , Muehlboeck S , Spenger C , Lovestone S , Simmons A , Soininen H ((2012) ) Education increases reserve against Alzheimer’s disease—evidence from structural MRI analysis. Neuroradiology 54: , 929–938. |
[19] | Zhu W , Li X , Li X , Wang H , Li M , Gao Z , Wu X , Tian Y , Zhou S , Wang K , Yu Y ((2021) ) The protective impact of education on brain structure and function in Alzheimer’s disease. BMC Neurol 21: , 423. |
[20] | Roe CM , Xiong C , Miller JP , Morris JC ((2006) ) P1-171: Education and preclinical Alzheimer’s disease without dementia. Alzheimers Dement 2: , S146. |
[21] | Meyer OL , Eng CW , Ko MJ , Chan M , Ngo U , Gilsanz P , Glymour MM , Mayeda ER , Mungas DM , Whitmer RA ((2020) ) Generation and age of immigration on later life cognitive performance in KHANDLE. Int Psychogeriatr 35: , 17–28. |
[22] | Kahle-Wrobleski K , Andrews JS , Belger M , Ye W , Gauthier S , Stern Y , Rentz DM ((2016) ) O5-07-04: Dependence levels as interim clinical milestones along the continuum of Alzheimer’s disease (AD): 18-Month Results from the Geras Observational Study. Alzheimers Dement 12: , 394–396. |
[23] | Vidoni ED , Honea RA , Burns JM ((2010) ) Neural correlates of impaired functional independence in early Alzheimer’s disease. J Alzheimers Dis 19: , 517–527. |
[24] | Yates L , Csipke E , Moniz-Cook E , Leung P , Walton H , Charlesworth G , Spector A , Hogervorst E , Mountain G , Orrell M ((2019) ) The development of the Promoting Independence in Dementia (PRIDE) intervention to enhance independence in dementia. Clin Interv Aging 14: , 1615–1630. |
[25] | Czarnuch S , Ricciardelli R , Mihailidis A ((2016) ) Predicting the role of assistive technologies in the lives of people with dementia using objective care recipient factors. BMC Geriatr 16: , 143. |
[26] | Han SS , White K , Cisek E ((2022) ) A feasibility study of individuals living at home with Alzheimer’s disease and related dementias: Utilization of visual mapping assistive technology to enhance quality of life and reduce caregiver burden. Clin Interv Aging 17: , 1885–1892. |
[27] | Anstey KJ , Von Sanden CC , Salim A , O’Kearney R ((2007) ) Smoking as a risk factor for dementia and cognitive decline: A meta-analysis of prospective studies. Am J Epidemiol 166: , 367–378. |
[28] | American Lung Association ((2023) ) Tobacco use in racial and ethnic populations. Accessed on August 25, 2023, from https://www.lung.org/quit-smoking/smoking-facts/impact-of-tobacco-use/tobacco-use-racial-and-ethnic |
[29] | Ukraintseva SV , Duan H , Arbeev KG , Fang F , Bagley O , Kulminski AM , Akushevich I , Yashin AI ((2018) ) P3-102: Dynamic relationships between APOE4, decline in BMI and Alzheimer’s disease. Alzheimers Dement 14: , 1106. |
[30] | Moody JN , Valerio KE , Hasselbach AN , Prieto S , Logue M , Hayes SL , Hayes JP , Alzheimer’s Disease Neuroimaging Initiative ((2021) ) Body mass index and polygenic risk for Alzheimer’s disease predict conversion to Alzheimer’s disease. J Gerontol A Biol Sci Med Sci 76: , 1415–1422. |
[31] | Mathys J , Gholam-Rezaee M , Henry H , Von Gunten A , Popp J ((2017) ) Decreasing body mass index is associated with cerebrospinal fluid markers of Alzheimer’s pathology in MCI and mild dementia. Exp Gerontol 100: , 45–53. |
[32] | James G , Witten D , Hastie T , Tibshirani R ((2013) ) An Introduction to Statistical Learning. Springer Texts in Statistics. |
[33] | Loh W ((2011) ) Classification and regression trees. Wiley Interdiscip Rev Data Min Knowl Discov 1: , 14–23. |
[34] | Kühn M , Johnson K ((2013) ) Applied Predictive Modeling. Springer. |