Validity and Reliability Study of Online Cognitive Tracking Software (BEYNEX)
Abstract
Background:
Detecting cognitive impairment such as Alzheimer’s disease early and tracking it over time is essential for individuals at risk of cognitive decline.
Objective:
This research aimed to validate the Beynex app’s gamified assessment tests and the Beynex Performance Index (BPI) score, which monitor cognitive performance across seven categories, considering age and education data.
Methods:
Beynex test cut-off scores of participants (n = 91) were derived from the optimization function and compared to the Montreal Cognitive Assessment (MoCA) test. Validation and reliability analyses were carried out with data collected from an additional 214 participants.
Results:
Beynex categorization scores showed a moderate agreement with MoCA ratings (weighted Cohen’s Kappa = 0.48; 95% CI: 0.38–0.60). Calculated Cronbach’s Alpha indicates good internal consistency. Test-retest reliability analysis using a linear regression line fitted to results yielded R∧2 of 0.65 with a 95% CI: 0.58, 0.71.
Discussion:
Beynex’s ability to reliably detect and track cognitive impairment could significantly impact public health, early intervention strategies and improve patient outcomes.
INTRODUCTION
Currently, more than 55 million people have dementia worldwide, over 60% of whom live in low-and middle-income countries. It is estimated that global spending on dementia increased by 4.5% annually from 2000 to 2019, reaching $263 billion attributable to dementia in 2019 [1]. Individuals with cognitive disorders frequently avoid visiting the doctor, and it is common that they must be taken by another individual only after these dysfunctions seriously start to affect their daily lives. Currently, it is estimated that 75% of people with dementia globally are not diagnosed, and this rate is likely even higher in some countries [2]. On the other hand, several individuals worry about dementia unnecessarily due to their forgetfulness. In a review on the determination of communication patterns in patients applying to memory clinics, the multidimensional evaluation of patients complaining of forgetfulness is emphasized. Whether the patient attends with a companion, how they participate, give autobiographical history, demonstrate working memory, and make qualitative observations during routine cognitive testing are all useful in building a diagnostic picture [3]. This diagnostic picture is then validated by impartial data such as neuropsychological tests.
Despite recent advances in the identification of mild cognitive impairment (MCI)-related biomarkers, the neuropsychological assessment remains a critical component of evaluation to ensure that cognitive function correlates with biomarker abnormalities to assist in detecting and tracking the progression of MCI to early Alzheimer’s disease (AD) [4]. However, these assessments are not designed for longitudinal tracking of patients in short time intervals. They are designed to be administered by trained professionals to support a diagnosis with high specificity and sensitivity with both the patient and the administrator being present. Unfortunately, given the prevalence of the disease and the number of people seeking professional help, for both addressing this public health concern from a preventive point of view and adapting how the disease is managed, a valid, reliable, accessible, engaging, and affordable digital cognitive screening instruments for clinical use is in urgent demand and rapid cognitive screening of societies is now possible with online applications that have developed rapidly in recent years [5]. Studies have shown that these online applications can be used in other neurological diseases that require cognitive monitoring, such as stroke, in addition to dementia [6]. Significant progress has been made in the field of digital cognitive testing in recent years. There are now digital cognitive test alternatives to Montreal Cognitive Assessment (MoCA) that still require a trained professional to administer such as CNS Vital Signs [7], NIH toolbox for assessment of neurological and behavioral function [8], CogState [9], BrainCheck [10], and many other such tests. These tests, while being digital, still cannot offer a solution on how to address the undiagnosed population and patients that do not seek professional help on time. Other alternatives such as Cognivue [11] are self-administered but still require either a costly device or presence of the patient in the clinic. And finally, tools such as MemTrax [12] and Boston Cognitive Assessment [13] have been developed to assess patients online with a self-administered digital assessment test. Especially, the MemTrax test, has shown in various studies that it can be relied upon to screen for cognitive impairment and other conditions [5, 12]. To address the concerns of healthy individuals and detect early signs of cognitive decline in patients that may lead to AD, it can be said that accurate, self-administered online longitudinal cognitive tracking will be invaluable in the future.
AD care requires cognitive tracking of cognitive performance at the earliest point of concern and monitoring disease progression and treatment when appropriate. Traditionally, a broad range of separate tests is combined into a neurocognitive test battery to measure the full spectrum of cognitive functioning. However, combining enough of these traditional tests into a complete test set can lead to a long time-consuming evaluation. As a result, clinical cognitive monitoring takes a lot of time and causes high costs. Therefore, online cognitive tracking applications are quite feasible. However, these applications should be shown to be valid by clinical tests, and cut-off values should be determined.
With the contribution of today’s digital technology, many online applications have started to be used in the topics of cognitive stimulation, training, and rehabilitation. Research results of programs targeting training and rehabilitation are discussed in various review articles [14, 15]. Most studies reported that users do not need to be technologically skilled to successfully complete or benefit from training. Overall, findings are comparable to or better than reviews of more traditional, paper-and-pencil cognitive training approaches suggesting that computerized training is an effective, less labor-intensive alternative [12]. However, those skeptical of the benefits of cognitive training or rehabilitation are right to argue that positive data are insufficient. However, in our opinion, online cognitive applications can provide longitudinal tracking information to the person by constantly comparing the performance of the users with those of their age groups and similar education levels and by monitoring their daily life activities. As it moves away from the average cognitive values, it may recommend that the person applies to the clinic. With today’s developing artificial intelligence (AI) technology, it can determine the life factors that affect a person’s cognitive level and produce unique messages for that person [16]. In this study, we aim to demonstrate that the Beynex gamified assessment test can be self-administered by its users and provide longitudinally data on users’ cognitive performance in various parameters in line with the current standard of pen and paper based neuropsychological tests.
METHODS
Beynex is an application created by neurologists, psychologists, and computer engineers. The first version was released in January 2020. The application, which can be downloaded in Android and IOS environments, works on tablets and smartphones. It monitors people with cognitive concerns in 7 different categories (speed, flexible thinking, language, memory, visual perception problem-solving, and attention). Each day, the users are prompted to take one of the gamified exercises that tests these parameters in one category to eliminate learning bias and 3 times to standardize the results. Each test lasts about 4 to 6 minutes. Thus, a full cognitive survey of each user is completed every 7 days. Beynex instantly evaluates the user’s cognitive performance in each category, and presents it in a single score, Beynex Performance Index (BPI).
(1)
φn: average in n’th category
BPI is a summary of your cognitive level, with respect to the cognitive performance that a person in a population is supposed to have compared to the average.
As for the 7 categories, users are scored based on their accuracy and/or their speed in completing the gamified assessments described below:
Speed: A circle appears on the screen at random intervals, users must then and only then click on them before they disappear.
Flexible thinking: Users are given 2 color names with different font colors; they must answer correctly or incorrectly depending on whether or not the meaning of the first color name is the same as the second color’s written font color.
Language: An image is given to users. They must then write its name.
Memory: Users are given a grid of unlit squares which then light up randomly. Once the grid is once again unlit, they must replicate the lit squares.
Visual perception: Users are given objects with location descriptions and are asked to select if the statements are correct or incorrect.
Problem-solving: There are multiple jars with different colored items. Users must collect all the same-colored items in the same jar.
Attention: A number is shown on-screen. After it disappears the users must rewrite it backwards.
Additionally, Beynex provides a way to track daily activities such as time spent reading or watching TV. There are nearly 20 free exercise games for people who want to exercise more. However, the performance scores of additional games do not affect cognitive follow-up parameters.
This study was performed, reviewed, and approved by the ethical committee of the Maltepe University Medical Faculty in Istanbul, Turkey (2023/900/31). All participants voluntarily provided signed informed consent.
Cut-off score determination
The study to determine the Beynex cutoff scores included 91 adults aged 49 to 83 years at risk of age-related cognitive decline or dementia (Table 1). The subjects of our study comprised both individuals who believed their cognitive health to be good and those who had memory problems. The subjects of our study were selected among the volunteers in social gathering places. They complete both the MoCA (reference standard) and the Beynex tests. Exclusion criteria included the presence of motor or visual disabilities and the inability to provide informed consent.
Table 1
Description of participants’ basic attributes, BEYNEX, and MoCA results belong to the cut-off score determination study
MoCA | BPI | Age | |||||||||
count | mean | Std | min/max | mean | std | min/max | mean | std | min/max | ||
Female | Bachelor’s | 13 | 26.31 | 3.15 | [20, 30] | 62.55 | 10.26 | [46.3, 87.08] | 61.85 | 8.36 | [54, 80] |
High School | 27 | 23.59 | 3.46 | [16, 29] | 52.32 | 14.95 | [29.7, 84.05] | 62.48 | 9.00 | [51, 83] | |
Literate | 2 | 19.50 | 6.36 | [15, 24] | 34.16 | 11.76 | [25.85, 42.47] | 72.50 | 3.54 | [70, 75] | |
Master’s | 3 | 27.67 | 2.08 | [26, 30] | 69.27 | 5.40 | [63.51, 74.23] | 57.33 | 7.37 | [49, 63] | |
Middle School | 2 | 20.00 | 4.24 | [17, 23] | 57.13 | 15.44 | [46.21, 68.05] | 60.00 | 5.66 | [56, 64] | |
Primary School | 4 | 23.25 | 2.22 | [20, 25] | 50.89 | 14.27 | [39.56, 71.02] | 57.00 | 7.79 | [51, 68] | |
Male | Bachelor’s | 17 | 25.41 | 2.76 | [21, 30] | 68.28 | 14.25 | [37.2, 89.67] | 61.71 | 7.56 | [51, 78] |
Doctorate | 3 | 26.00 | 2.65 | [24, 29] | 72.32 | 19.72 | [55.2, 93.89] | 62.33 | 12.70 | [55, 77] | |
High School | 12 | 26.17 | 2.55 | [21, 30] | 63.80 | 9.11 | [51.46, 74.61] | 59.17 | 6.07 | [51, 70] | |
Master’s | 2 | 24.50 | 3.54 | [22, 27] | 60.05 | 18.18 | [47.2, 72.91] | 68.00 | 19.80 | [54, 82] | |
Middle School | 2 | 25.50 | 3.54 | [23, 28] | 56.68 | 32.89 | [33.43, 79.93] | 70.50 | 6.36 | [66, 75] | |
Primary School | 4 | 20.00 | 2.45 | [17, 23] | 39.20 | 9.81 | [28.24, 51.93] | 66.00 | 7.79 | [55, 72] |
The MoCA is an 8-item questionnaire with scores ranging from 0 to 30, and it is designed to quickly assess executive functions, visuospatial abilities, short-term and working memory, attention, language, and orientation [17]. Participants were grouped according to their MoCA score (>24 = unimpaired, 24–20 = mildly impaired, and <20 = impaired) as given in a recent study [18].
The cut-off values for Beynex scores corresponding to the MoCA classifications of unimpaired, intermediate (mildly impaired), and impaired were established. The optimization function defined as the multiplication of negative percent agreement (NPA) and positive percent agreement (PPA) between Beynex and MoCA scores is maximized to find the cut-off values.
(2)
(3)
The inaccuracy and error bias of our findings were calculated to assess the performance of the determined cut-offs.
(4)
ACC, Accuracy; FN, False negative; FP, False positive; TN, True negative; TP, True positive.
Clinical validation study
The validation study included another 214 participants, ranging in age from 49 to 83 (Table 2). These individuals were identified as being at risk for age-related cognitive decline or dementia. Both those who see themselves as cognitively healthy and those who experience forgetfulness were included in our study. The exclusion criteria are the same as the cut-off determination study.
Table 2
Description of participants’ basic attributes, BEYNEX, and MoCA results belong to the clinical validation study
MoCA | BPI | Age | |||||||||
count | mean | std | min/max | mean | std | min/max | mean | std | min/max | ||
Female | Bachelor’s | 35 | 25.54 | 2.80 | [20, 30] | 62.84 | 13.43 | [34.49, 83.53] | 60.63 | 7.05 | [51, 77] |
Doctorate | 1 | 27.00 | – | [27, 27] | 78.69 | – | [78.69, 78.69] | 52.00 | – | [52, 52] | |
High School | 46 | 24.35 | 3.49 | [17, 29] | 56.86 | 13.40 | [30.6, 81.89] | 61.72 | 7.80 | [50, 81] | |
Literate | 1 | 19.00 | – | [19, 19] | 38.56 | – | [38.56, 38.56] | 62.00 | – | [62, 62] | |
Master’s | 6 | 26.33 | 2.80 | [23, 30] | 64.74 | 7.71 | [50.47, 73.49] | 57.17 | 4.54 | [53, 64] | |
Middle School | 13 | 22.69 | 3.15 | [15, 26] | 47.36 | 12.51 | [34.22, 76.12] | 62.69 | 9.47 | [52, 77] | |
Primary School | 19 | 22.95 | 3.36 | [17, 29] | 46.02 | 9.43 | [31.65, 61.88] | 62.68 | 9.45 | [50, 80] | |
Male | Bachelor’s | 35 | 25.71 | 3.14 | [17, 30] | 64.35 | 13.45 | [41.29, 86.65] | 62.49 | 8.04 | [53, 81] |
Doctorate | 5 | 27.80 | 1.79 | [26, 30] | 69.62 | 7.28 | [60.01, 77.33] | 59.60 | 5.46 | [54, 67] | |
High School | 20 | 24.60 | 3.25 | [18, 30] | 63.65 | 12.81 | [42.28, 93.32] | 62.30 | 7.57 | [51, 77] | |
Literate | 1 | 24.00 | – | [24, 24] | 46.04 | – | [46.04, 46.04] | 74.00 | – | [74, 74] | |
Master’s | 12 | 27.00 | 2.30 | [22, 29] | 70.11 | 9.49 | [52.46, 83.72] | 58.92 | 6.58 | [51, 71] | |
Middle School | 11 | 22.45 | 2.62 | [18, 27] | 57.38 | 18.90 | [29.01, 87.42] | 65.27 | 9.21 | [55, 81] | |
Primary School | 9 | 23.56 | 4.13 | [16, 30] | 48.29 | 13.48 | [27.36, 69.53] | 65.56 | 8.03 | [55, 82] |
The analysis was conducted with the validation group and NPA, PPA, and error bias as given in Equations 2–4 were calculated. Additionally, the retest reliability of Beynex was assessed by finding the best fit line by linear regression and calculating the coefficient of determination between two consecutive BPI scores of subjects that are at least 2 weeks apart. Cronbach’s alpha was also calculated to further test the consistency and stability of BPI scores [19]. Lastly, to measure the inter-rater reliability of the Beynex and MoCA tests, Cohen’s kappa was determined [20].
RESULTS
Cut-off score determination
The results of 91 subjects who took the MoCA and the Beynex tests are used to determine the cut-off values. The categorical distribution of the participants based on their test scores is given in the scatterplot can be seen in Fig. 1. The cut-offs for BPI scores were determined by maximizing the optimization function as mentioned in the method section. Two cut-offs were found to be 49.0 and 55.6 and the categories defined by scores of both MoCA and BPI are given in Table 3. According to the MoCA scores, 51.6% of participants had no impairments (>24), 39.6% had mild impairments (24–20), and 8.8% had severe impairments (<20). Of the 91 subjects 55.0% had no impairments, 17.6% had mild impairments, and 27.5% had severe impairments after applying the cut-offs that have been determined for BPI scores.
Fig. 1
Scatterplot of MoCA and BPI scores of participants for cut-off score determination study. The blue linear regression line, represented by the equation y = (0.14±0.02)×BPI + (16.29±1.17), has an R∧2 of 0.38. Green lines correspond to lower cut-offs of the tests whereas yellow ones represent upper cut-offs. NPA = 0.78 and PPA = 0.88 for the lower cut-offs while NPA = 0.83 and PPA = 0.75 for the upper cut-offs.
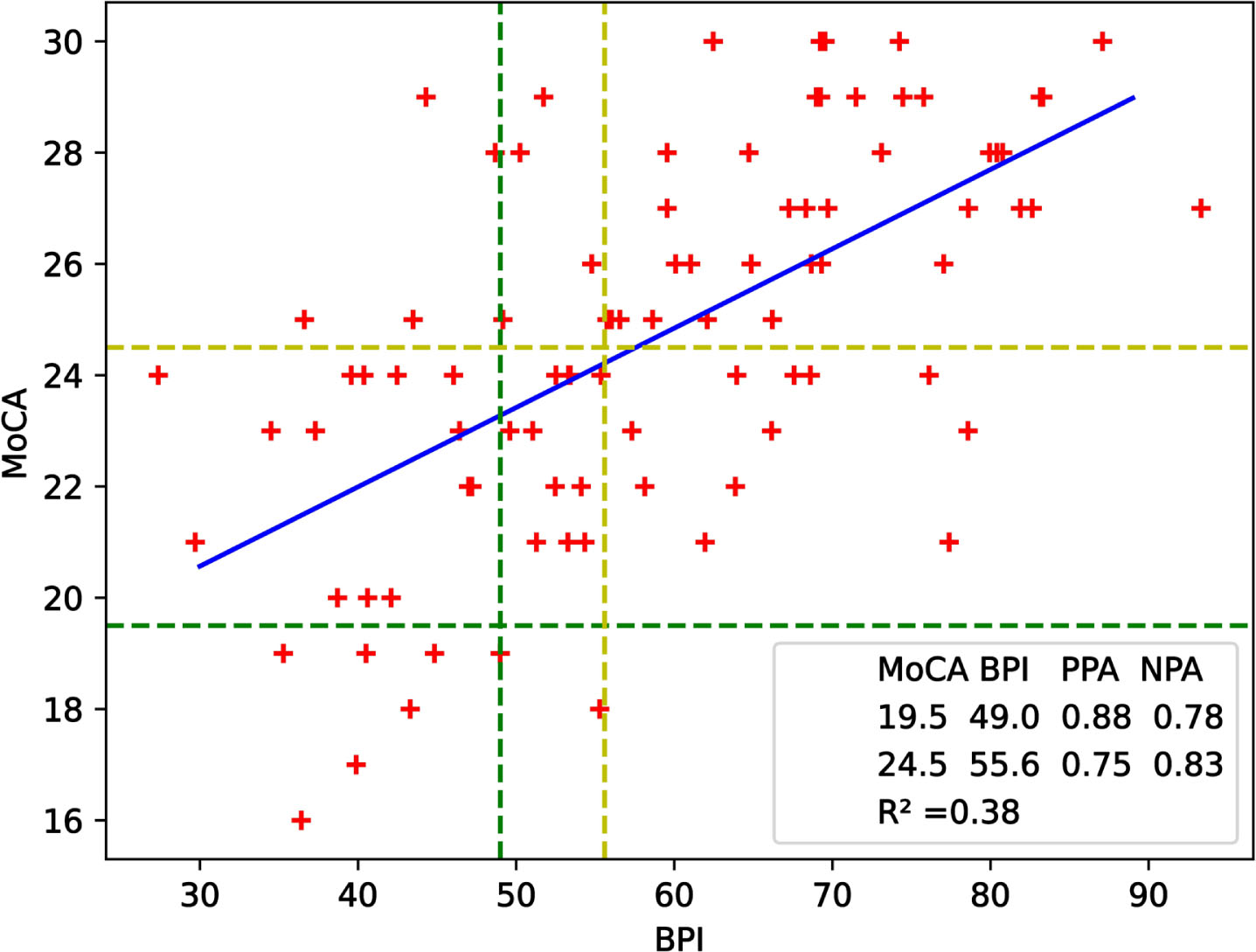
Table 3
Descriptive categories and their corresponding MoCA and BPI cut-offs
Cut-off Scores | ||
MoCA | BPI | |
Impaired | <20.0 | <49.0 |
Mildly Impaired | 20.0–24.0 | 49.0–55.6 |
Unimpaired | >24.0 | >55.6 |
Percentage agreements for the lower cut-offs that maximized the optimization function are NPA = 0.78, PPA = 0.88. Using the upper cut-offs corresponded to NPA = 0.83, PPA = 0.75. The accuracy value is 0.791 for both lower and upper cut-off values which can be considered as significant. When a linear regression line was added, the coefficient of determination R∧2 was calculated as 0.38.
Clinical validation study
During the clinical validation study, we compared the evaluations of 214 participants using both the MoCA and BPI. Table 4 presents a comprehensive comparison, revealing how the participants’ cognitive states varied between the two tests. In the MoCA assessment, 6.54% of participants were identified as impaired, 40.19% as mildly impaired, and 53.27% as unimpaired. The results from the Beynex BPI evaluation showed that 26.2% of participants were classified as impaired, 16.8% as mildly impaired, and 56.97% as unimpaired.
Table 4
Distribution of participants based on their MoCA and Beynex test scores. The percentage each cell represents is given in parentheses. Here, the number of participants is 214 and this group has been used in the validation study
MoCA∖Beynex | Impaired | Mildly | Unimpaired | Total |
impaired | ||||
Impaired | 11 (5.1%) | 1 (0.5%) | 2 (0.9%) | 14 |
Mildly Impaired | 35 (16.4%) | 22 (10.3%) | 29 (13.6%) | 86 |
Unimpaired | 10 (4.7%) | 13 (6.1%) | 91 (42.5%) | 114 |
Total | 56 | 36 | 122 | 214 |
Figure 2 complements these findings by displaying the test scores of 241 study participants in a scatterplot. The best fitting line was obtained by linear regression and the coefficient of determination R∧2 is 0.36. NPA and PPA were found to be 0.79 and 0.78 respectively for the lower cut-offs while NPA was 0.69 and PPA was 0.8 for the upper cut-offs. These results shows that the data used for validation agrees with the data used for cut-off determination. Accuracy given in Eqn 4 was calculated as 0.77 for lower and 0.75 upper cut-off values.
Fig. 2
Scatterplot of MoCA and BPI scores of participants for validation study. The blue linear regression line, described by the equation y = (0.14±0.01)×BPI + (16.65±0.76), has an R∧2 of 0.36. Green lines correspond to lower cut-offs of the tests whereas yellow ones represent upper cut-offs. NPA = 0.79 and PPA = 0.78 for the lower cut-offs while NPA = 0.69 and PPA = 0.8 for the upper cut-offs.
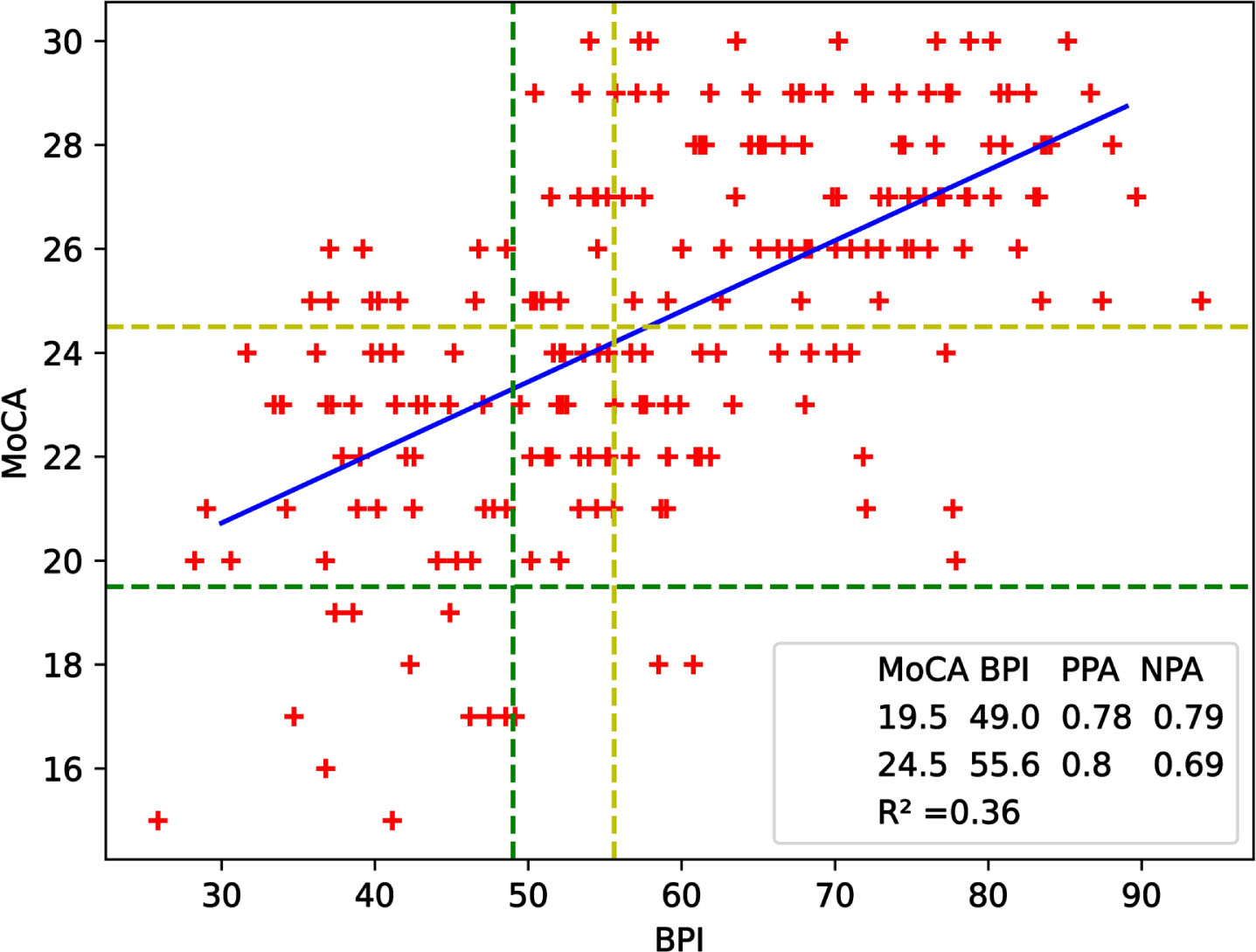
In addition to the NPA, PPA, and accuracy, ROC analysis was performed. The AUC was 0.827 (with [0.778, 0.871] 95% CI) when comparing unimpaired to mildly impaired (Fig. 4a) and the AUC was 0.832 (with [0.756, 0.888] 95% CI) when comparing mildly impaired to impaired (Fig. 4b).
Fig. 3
Scatterplot of the comparison between first and second attempts of subjects at Beynex test. The added regression line has a slope of 0.86 with a 95% confidence interval (0.791, 0.935) which is shown as the shaded blue are about the line. The y-intercept is 10. R∧2 was found to be 0.647.
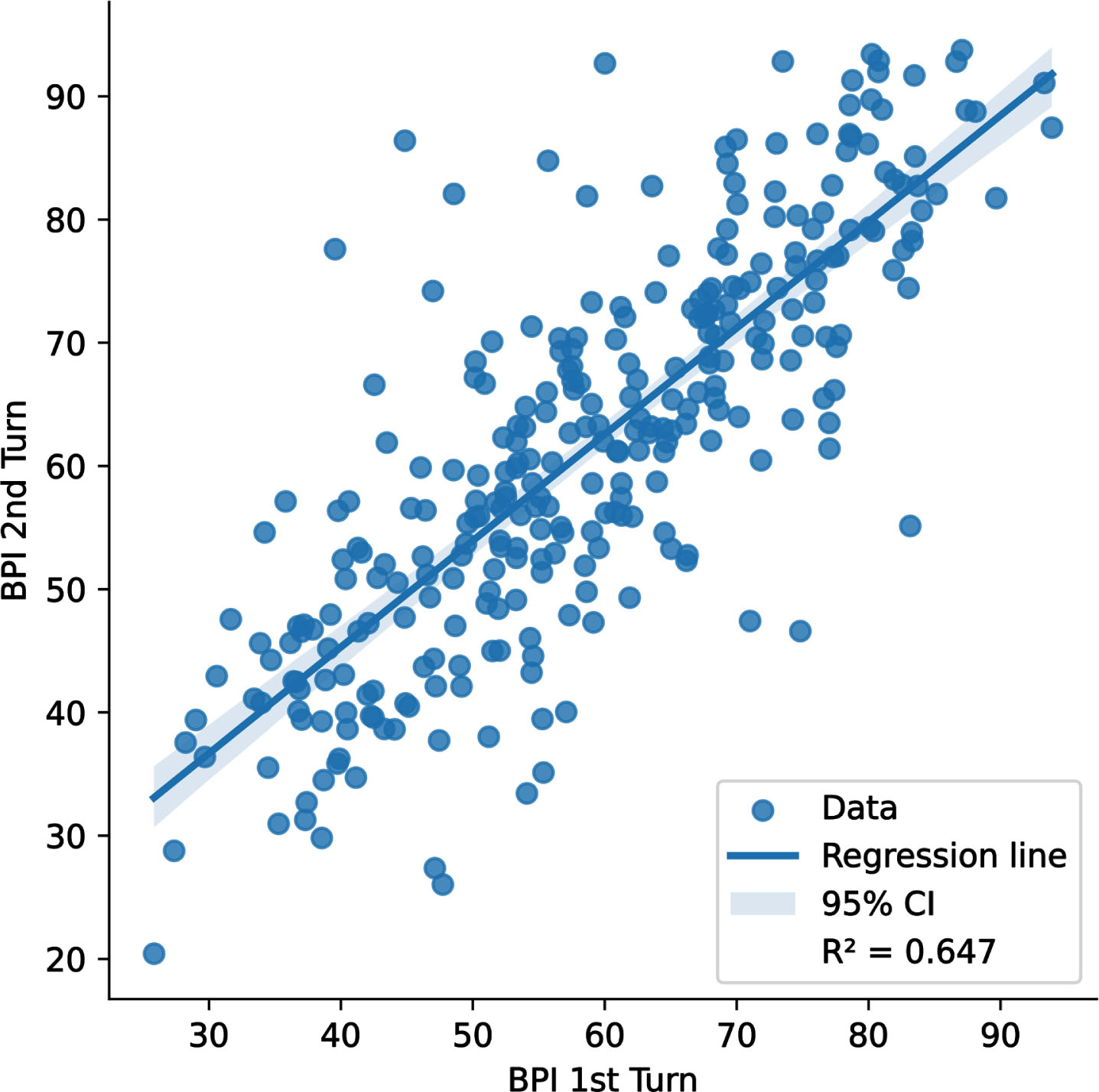
Fig. 4
ROC curve analysis with AUCs comparing Beynex test performance against MoCA diagnoses. The solid blue line signifies Beynex’s efficacy in differentiating unimpaired from mildly impaired in (Fig. 4a) and mildly impaired from impaired in (Fig. 4b). The gray dashed line is the reference line of no-discrimination.
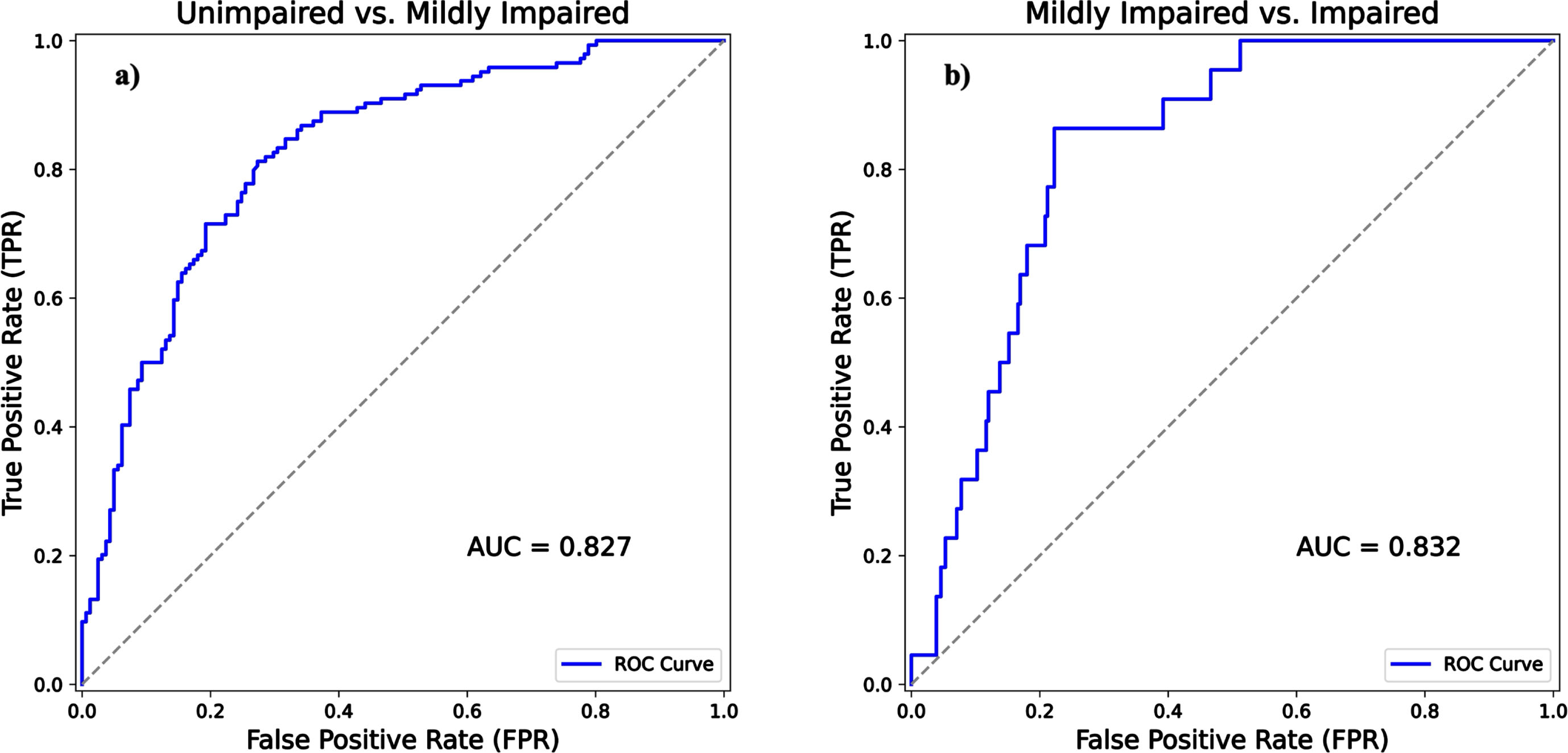
Cohen’s kappa K was calculated to quantify interrater reliability between Beynex and MoCA tests. Quadratic weighting has been used to emphasize the categorical identifications that differ by 2. An example for such a case is when a participant is categorized as impaired in one of the tests while the other categorizes the same participant as unimpaired. K has been found to be 0.4843 with a 95% CI of 0.3759 to 0.5927. This result demonstrates moderate agreement between the two tests.
To assess the reliability and internal consistency of the 7 subtests of Beynex Cronbach’s Alpha has been calculated as 0.823 with a 95% CI of [0.792, 0.852] which indicates that the test has good internal consistency.
Test-retest reliability of the Beynex test was investigated by comparing two consecutive tests of participants that were at least 2 weeks apart. The scatterplot of the two consecutive BPI scores of the participants is given in Fig. 3 along with a linear regression line. R∧2 has been found as 0.647 which is quite good considering the learning effect that takes place during the first taking of the test that boosts the second trial scores of the participants.
DISCUSSION
Quality care for people with AD and other dementias starts with an early, documented diagnosis, including disclosure of the diagnosis. However, most people who have been diagnosed with AD are not aware of their diagnosis. Evidence indicates that only about half of those with AD have been diagnosed. Among those seniors who have been diagnosed with AD, only 33% are even aware that they have the disease. Even when including caregivers, 45 percent of those diagnosed with AD or their caregivers are aware of the diagnosis [11].
Detecting cognitive impairment, diagnosing AD and other dementias, and disclosing that diagnosis to the individual are necessary elements to ensuring that people with dementia, together with their families, have the opportunity to access available treatments, build a care team, participate in support services, enroll in clinical trials, and plan for the future. Furthermore, there is growing consensus that novel disease-modifying therapies for AD would be appropriate and more likely to deliver benefit when used at earlier stages in the disease evolution, potentially at the prodromal stage [21].
The 2017 Geriatric Summit on Assessing Cognitive Disorders among the Aging Population, sponsored by the National Academy of Neuropsychology, emphasized the importance of ongoing screening and the need for automated tools for assessing and recording patients’ results over time [22].
We can list the obstacles to evaluation with cognitive tests in the clinic as high cost, timing, and lack of trained personnel in this field. [23–25]. MCI or milder cognitive impairment often accompanies daily living activity change and has been shown in many studies. Assessment of activities of daily living (ADL) is paramount to underpin accurate diagnostic classification in MCI and dementia. Unfortunately, most common report-based ADL tools have limitations for diagnostic purposes [26–29].
Beynex is self-administered by the patient and can be initiated by non-clinician support personnel. Because of these advantages, it was written for the purpose of long-term cognitive follow-up rather than the dementia screening test. The Beynex software, which is the subject of this research, does not claim to be cognitive training or rehabilitation; it only aims to stimulate the user by performing cognitive follow-up. With the experience obtained from 3 years of user data, we observed that the follow-up of both cognitive and daily living activity changes for at least 3 months or longer is much more meaningful than instant evaluations in people using the application.
This study aimed to verify the BPI as a cognitive assessment tool and establish the cut-off values for the BPI. The scatterplots and linear regression analysis showed a correlation between the BPI scores and MoCA scores. The determined cut-off values for the BPI scores allowed for categorizing participants into different levels of impairment, aligning with the categories defined by the MoCA scores. Additionally, the AUCs for both cases when differentiating unimpaired from mildly impaired and mildly impaired from impaired show that BPI can screen for cognitive performance with good accuracy. The validation study further supported the accuracy and reliability of the BPI test. The agreement between the BPI and MoCA tests, quantified by Cohen’s kappa coefficient, indicated moderate agreement between the two assessments. The internal consistency of the BPI subtests was also found to be good, as evidenced by the calculated Cronbach’s alpha value. The test-retest reliability analysis demonstrated consistent performance of the Beynex test over time, indicating its stability and reliability for measuring cognitive function when administered to participants at least 2 weeks apart.
The findings of this study emphasize the importance of early detection and diagnosis of cognitive impairment, including AD and other dementias. Automated tools like the Beynex test can facilitate ongoing screening and tracking of cognitive function, enabling timely access to available treatments, support services, and future planning for individuals with dementia and their families. Overall, the Beynex test shows promise as a self-administered cognitive assessment tool that can be initiated by non-clinician personnel. Its ease of use, reliability, and ability to track cognitive and daily living activity changes make it a valuable tool for long-term cognitive follow-up. Future research of the Beynex test should focus on further validation by widening the sample population to include a more diverse demography of participants, and people of varying severity of cognitive decline.
ACKNOWLEDGMENTS
We would like to thank Şebnem Barış and BEYNEX Technologies OÜ, who contributed to the organization of data records in this study, meeting the needs of the centers and preparing the participants for the test.
FUNDING
The authors have no funding to report.
CONFLICT OF INTEREST
Sahiner TAH has acted as a consultant for “BEYNEX Technologies OÜ”, Bayındır E, Atam T, Hisarlı E, Akgönül S, Bagatır O, and Sahiner E, are employees of BEYNEX Technologies OÜ.
DATA AVAILABILITY
The data supporting the findings of this study are available on request from the corresponding author. The data are not publicly available due to privacy or ethical restrictions.
REFERENCES
[1] | Valendia PP , Miller-Petrie MK , Chen C , Chakrabarti S , Chapin A , Hay S , Tsakalos G , Wimo A , Dieleman JL ((2022) ) Global and regional spending on dementia care from 2000–2019 and expected future health spending scenarios from 2020–2050: An economic modelling exercise. EClinicalMedicine 45: , 101337. |
[2] | Gauthier S , Rosa-Neto P , Morais JA , Webster C (2021) World Alzheimer’s Report 2021. Journey through the diagnosis of dementia. Alzheimer’s Disease International,https://www.alzint.org/u/World-Alzheimer-Report-2021.pdfposted on September 2021. Accessed 12 January 2022. |
[3] | Bailey C , Poole N , Blackburn DJ ((2018) ) Identifying patterns of communication in patients attending memory clinics: A systematic review of observations and signs with potential diagnostic utility. Br J Gen Pract 68: , 123–138. |
[4] | Albert MS , DeKosky ST , Dickson D , Dubois B , Feldman HH , Fox NC , Gamst A , Holtzman DM , Jagust WJ , Petersen RC , Snyder PJ , Carrillo MC , Thies B , Phelps CH ((2011) ) The diagnosis of mild cognitive impairment due to Alzheimer’s disease: Recommendations from the national institute on aging-Alzheimer’s association workgroups on diagnostic guidelines for Alzheimer’s disease. Alzheimers Dement 7: , 270–279. |
[5] | Liu X , Chen X , Zhou X , Shang Y , Xu F , Zhang J , He J , Zhao F , Du B , Wang X , Zhang Q , Zhang W , Bergeron MF , Ding T , Ashford JW , Zhong L ((2021) ) Validity of the MemTrax memory test compared to the Montreal cognitive assessment in the detection of mild cognitive impairment and dementia due to Alzheimer’s disease in a Chinese cohort. J Alzheimers Dis 80: , 1257–1267. |
[6] | Zhao X , Dai S , Zhang R , Chen X , Zhao M , Bergeron MF , Zhou X , Zhang J , Zhong L , Ashford JW , Liu X ((2023) ) Using MemTrax memory test to screen for post-stroke cognitive impairment after ischemic stroke: A cross-sectional study. Front Hum Neurosci 17: , 1195220. |
[7] | Gualtieri CT , Johnson LG ((2006) ) Reliability and validity of a computerized neurocognitive test battery, CNS Vital Signs. Arch Clin Neuropsychol 21: , 623–643. |
[8] | Weintraub S , Dikmen SS , Heaton RK , Tulsky DS , Zelazo PD , Slotkin J , Carlozzi NE , Bauer PJ , Wallner-Allen K , Fox N , Havlik R , Beaumont JL , Mungas D , Manly JJ , Moy C , Conway K , Edwards E , Nowinski CJ , Gershon R ((2014) ) The cognition battery of the NIH toolbox for assessment of neurological and behavioral function: Validation in an adult sample. J Int Neuropsychol Soc 20: , 567–578. |
[9] | Green RC , Green J , Harrison JM , Kutner MH ((1994) ) Screening for cognitive impairment in older individuals. Validation study of a computer-based test. Arch Neurol 51: , 779–786. |
[10] | Groppell S , Soto-Ruiz KM , Flores B , Dawkins W , Smith I , Eagleman DM , Katz Y ((2019) ) A rapid, mobile neurocognitive screening test to aid in identifying cognitive impairment and dementia (BrainCheck): Cohort study. JMIR Aging 2: , e12615. |
[11] | Jessen F , Amariglio RE , Buckley RF , van der Flier WM , Han Y , Molinuevo JL , Rabin L , Rentz DM , Rodriguez-Gomez O , Saykin AJ , Sikkes SAM , Smart CM , Wolfsgruber S ((2020) ) The characterization of subjective cognitive decline. Lancet Neurol 19: , 271–278. |
[12] | Van der Hoek MD , Nieuwenhuizen A , Keijer J , Ashford JW ((2019) ) The MemTrax test compared to the Montreal cognitive assessment estimation of mild cognitive impairment. J Alzheimers Dis 67: , 1045–1054. |
[13] | Vyshedskiy A , Netson R , Fridberg E , Jagadeesan P , Arnold M , Barnett S , Gondalia A , Maslova V , de Torres L , Ostrovsky S , Durakovic D , Savchenko A , McNett S , Kogan M , Piryatinsky I , Gold D ((2022) ) Boston cognitive assessment (BOCA) — a comprehensive self-administered smartphone- and computer-based at-home test for longitudinal tracking of cognitive performance. BMC Neurol 22: , 92. |
[14] | Zuschnegg J , Schoberer D , Häussl A , Herzog SA , Russegger S , Ploder K , Fellner M , Hofmarcher-Holzhacker MM , Roller-Wirnsberger R , Paletta L , Koini M , Schüssler S ((2023) ) Effectiveness of computer-based interventions for community-dwelling people with cognitive decline: A systematic review with meta-analyses. BMC Geriatr 23: , 229. |
[15] | Chan JYC , Yau STY , Kwok TCY , Tsoi KKF ((2021) ) Diagnostic performance of digital cognitive tests for the identification of MCI and dementia: A systematic review. Ageing Res Rev 72: , 101506. |
[16] | Bergeron MF , Landset S , Zhou X , Ding T , Khoshgoftaar TM , Zhao F , Du B , Chen X , Wang X , Zhong L , Liu X , Ashford JW ((2020) ) Utility of MemTrax and machine learning modeling in classification of mild cognitive impairment. J Alzheimers Dis 77: , 1545–1558. |
[17] | Nasreddine ZS , Phillips NA , Bédirian V , Charbonneau S , Whitehead V , Collin I , Cummings JL , Chertkow H ((2005) ) The Montreal Cognitive Assessment, MoCA: A brief screening tool for mild cognitive impairment. J Am Geriatr Soc 53: , 695–699. |
[18] | Thomann AE , Berres M , Goettel N , Steiner LA , Monsch AU ((2020) ) Enhanced diagnostic accuracy for neurocognitive disorders: A revised cut-off approach for the Montreal Cognitive Assessment. Alzheimers Res Therapy 12: , 39. |
[19] | Bujang MA , Omar ED , Baharum NA ((2018) ) A review on sample size determination for Cronbach’s alpha test: A simple guide for researchers. Malays J Med Sci 25: , 85–99. |
[20] | McHugh ML ((2012) ) Interrater reliability: The kappa statistic. Biochemia Medica 22: , 276–282. |
[21] | Hampel H , Vergallo A , Iwatsubo T , Cho M , Kurokawa K , Wang H , Kurzman HR , Chen C ((2022) ) Evaluation of major national dementia policies and health-care system preparedness for early medical action and implementation. Alzheimers Dement 18: , 1993–2002. |
[22] | Abd Razak MA , Ahmad NA , Chan YY , Mohamad Kasim N , Yusof M , Abdul Ghani MKA , Omar M , Abd Aziz FA , Jamaluddin R ((2019) ) Validity of screening tools for dementia and mild cognitive impairment among the elderly in primary health care: A systematic review. Public Health 169: , 84–92. |
[23] | Cahn-Hidalgo D , Estes PW , Benabou R ((2020) ) Validity, reliability, and psychometric properties of a computerized, cognitive assessment test (Cognivue®). World J Psychiatry 10: , 1–11. |
[24] | Cordell CB , Borson S , Boustani M , Chodosh J , Reuben D , Verghese J , Thies W , Fried LB ((2013) ) Medicare Detection of Cognitive Impairment Workgroup. Alzheimer’s Association recommendations for operationalizing the detection of cognitive impairment during the Medicare Annual Wellness Visit in a primary care setting. Alzheimers Dement 9: , 141–150. |
[25] | Sheehan B ((2012) ) Assessment scales in dementia. Ther Adv Neurol Disord 5: , 349–358. |
[26] | Cornelis E , Gorus E , Beyer I , Bautmans I , de Vriendt P ((2017) ) Early diagnosis of mild cognitive impairment and mild dementia through basic and instrumental activities of daily living: Development of a new evaluation tool. PLoS Med 14: , 1002250. |
[27] | Hesseberg K , Bentzen H , Ranhoff AH , Engedal K , Bergland A ((2013) ) Disability in instrumental activities of daily living in elderly patients with mild cognitive impairment and Alzheimer’s disease. Dement Geriatr Cogn Disord 36: , 146–153. |
[28] | Morris JN , Berg K , Fries BE , Steel K , Howard EP ((2013) ) Scaling functional status within the interRAI suite of assessment instruments. BMC Geriatr 13: , 128. |
[29] | Reppermund S , Brodaty H , Crawford JD , Kochan NA , Draper B , Slavin MJ , Trollor JN , Sachdev PS ((2013) ) Impairment in instrumental activities of daily living with high cognitive demand is an early marker of mild cognitive impairment: The Sydney memory and ageing study. Psychol Med 43: , 2437–2445. |