Relationship Between FERMT2, CELF1, COPI, CHRNA2, and ABCA7 Genetic Polymorphisms and Alzheimer’s Disease Risk in the Southern Chinese Population
Abstract
Background:
Alzheimer’s disease (AD) is a multi-gene inherited disease, and apolipoprotein E (APOE) ɛ4 is a strong risk factor. Other genetic factors are important but limited.
Objective:
This study aimed to investigate the relationship between 17 single-nucleotide polymorphisms (SNPs) and AD in the Southern Chinese populations.
Methods:
We recruited 242 AD patients and 208 controls. The SNaPshot technique was used to detect the SNPs.
Results:
Adjusted for sex and age, we found rs6572869 (FERMT2), rs11604680 (CELF1), and rs1317149 (CELF1) were associated with AD risk in the dominant (rs6572869: p = 0.022, OR = 1.55; rs11604680: p = 0.007, OR = 1.68; rs1317149: p = 0.033, OR = 1.50) and overdominant models (rs6572869: p = 0.001, OR = 1.96; rs11604680: p = 0.002, OR = 1.82; rs1317149: p = 0.003, OR = 1.80). rs9898218 (COPI) was associated with AD risk in the overdominant model (p = 0.004, OR = 1.81). Further, rs2741342 (CHRNA2) was associated with AD protection in the dominant (p = 0.002, OR = 0.5) and additive models (p = 0.002, OR = 0.64). Mutations in rs10742814 (CELF1), rs11039280 (CELF1), and rs3752242 (ABCA7) contributed to AD protection. Among them, rs10742814 (CELF1), rs3752242 (ABCA7), and rs11039280 (CELF1) were more significantly associated with AD carrying APOE ɛ4, whereas rs1317149 (CELF1) showed an opposite trend. Interestingly, rs4147912 (ABCA7) and rs2516049 (HLA-DRB1) were identified to be relevant with AD carrying APOE ɛ4. Using expression quantitative trait locus analysis, we found polymorphisms in CELF1 (rs10742814 and rs11039280), ABCA7 (rs4147912), HLA-DRB1 (rs2516049), and ADGRF4 (rs1109581) correlated with their corresponding gene expression in the brain.
Conclusions:
We identified four risk and four protective SNPs associated with AD in the Southern Chinese population, with different correlations between APOE ɛ4 carriers and non-carriers. rs4147912 (ABCA7) and rs2516049 (HLA-DRB1) were associated with AD carrying APOE ɛ4.
INTRODUCTION
Alzheimer’s disease (AD) is a common age-related neurodegenerative disease associated with genetic factors and accounts for most dementia in the elderly population worldwide [1]. Genetic factors explain 58–79% of AD heritability [2]. Therefore, the identification of AD-related loci can provide a reliable basis for a timely and reliable diagnosis. Apolipoprotein E (APOE) ɛ4 is a major risk factor for sporadic AD [3]. A meta-analysis in Caucasians showed that individuals with the ɛ4 allele were at increased risk of AD (ɛ2/ɛ4: odds ratio [OR] = 2.6; ɛ3/ɛ4: OR = 3.2, ɛ4/ɛ4: OR = 14.9) [4]. However, APOE accounts for only 10–20% of the risk of late-onset AD, suggesting that other genetic risk factors are also important in AD pathogenesis [5].
More than 30 AD-related susceptibility genes have been identified by genome-wide association study (GWAS) meta-analyses, including FERMT2, ABCA7, CR1, HLA-DRB, and CELF1 [6–9]. Furthermore, AD-related variants may affect the expression of nearby genes that influence the pathological processes of brain amyloidosis, tauopathy, and neurodegeneration, which provides valuable insights into the molecular mechanisms of AD pathogenesis [10]. A GWAS analysis of 1,126,563 individuals from European populations recently identified 3,915 variants across 38 independent loci associated with AD and proposed five new susceptibility loci (TNIP1, NTN5, AGRN, LILRB2, HAVCR2) [9]. The association between SNPs within ABCA7, EPHA1, and CD2AP and AD was not observed in an East Asian study, which was identified in Caucasians previously [11].
The genetic basis of race-specific AD susceptibility may be due to differences in minor allele frequency and the complexity of the underlying genetic structure inherent [11, 12]. Most GWAS and subsequent replication studies have been conducted almost exclusively in Caucasian populations, with relatively limited but significant AD inheritance in other populations. Replication of GWAS results in different ethnic populations may help identify SNPs associated with AD [13]. Therefore, in this study, we selected 17 SNP loci in 12 genes that have never been reported in Asian populations (FERMT2 rs6572869, ABCA7 rs4147912 and rs3752242, CELF1 rs10742814, rs1317149, rs11604680 and rs11039280, COPI rs9898218, CHRNA2 rs2741342, ADGRF4 rs1109581, HLA-DRB1 rs2516049, MS4A rs7116190 and rs667897, NRXN1 rs698842, CR1 rs4266886, CSMD1 rs7813641, and OR51I1 rs77336780), to investigate whether these SNPs are independently or in combination with APOE ɛ4 concerning AD risk in southern Chinese populations. In addition, we used expression quantitative trait loci (eQTL) datasets to assess the potential effects of these loci on gene expression, contributing to a better understanding of disease-related mechanisms and the discovery of new therapeutic pathways.
MATERIALS AND METHODS
Subjects
All enrolled subjects were evaluated by at least two experienced neurologists and underwent a standard series of examinations, including medical history, physical examination, systematic neuropsychological, such as standard Mini-Mental State Examination (MMSE) and clock drawing test (CDT) and neuroimaging tests such as magnetic resonance. Among them, 36 healthy controls and 52 AD patients received 18F-florbetapir positron emission tomogram examination. The results of AD patients met the criteria for probable AD defined by National Institute of Neurological and Communicative Disorders and Stroke–Alzheimer’s Disease and Related Disorders Association (NINCDS-ADRDA criteria) [14]. The physicians assessed the healthy controls recruited to confirm that they had no cognitive impairment [15, 16]. All participants were Han Chinese living in southern China. We excluded participants with a history of other neurological disorders that could lead to dementia, such as Parkinson’s disease, stroke, multiple sclerosis, brain tumors, and major depressive disorder [17].
This study conformed with the Declaration of Helsinki and was approved by the Ethics Committee of Ruijin Hospital, affiliated with the Shanghai Jiao Tong University School of Medicine (2018-No.6). All participants and their caregivers were fully informed about the study and signed a written consent form.
Genotype analysis
Genomic DNA was obtained from the peripheral blood leukocytes of the participants using the phenol-chloroform-isopropyl alcohol method. We designed the extension primers and polymerase chain reaction (PCR) using Primer5 software (version 5.00) and used SNaPshot technology (Applied Biosystems, Foster City, CA, United States) to genotype SNPs. SNPs of APOE (rs429358 and rs7412) were detected by polymerase chain reaction-restriction fragment length polymorphism (PCR-RFLP)[3]. The primer sequences are listed in Supplementary Table 1.
Gene expression analysis
Two databases, Braineac and Genotype-Tissue Expression (GTEx), were used to investigate the relationship between genotype and gene expression. The Braineac dataset included eQTL data for ten brain regions from 134 individuals of European descent without neurodegenerative disease. The GTEx database contained genotype and gene expression data of about 1000 individuals, mostly from Caucasian populations, covering 54 non-diseased tissues.
Statistical analyses
Statistical analyses were performed using SPSS software (version 26.0). A t-test and chi-square analysis were used to compare the demographic characteristics of the two groups. We performed a chi-square analysis to assess the distribution of allele and genotype and Hardy-Weinberg equilibrium (HWE) for each SNP. Four genetic models were applied to evaluate the risk of AD for each SNP after adjusting for age and sex. With M defined as the major allele and m as the minor allele, the models are: Dominant: 1 (mm + Mm) versus 0 (MM); Recessive: 1 (mm) versus 0 (MM + Mm); Additive: 0 (MM) versus 1 (Mm) versus 2 (mm); Overdominant: 1 (Mm) versus 0 (MM + mm). Statistical significance was set at p < 0.05. Bonferroni correction was performed.
RESULTS
Demographic characteristics of the study population
A total of 242 patients with AD and 208 healthy controls were recruited in this study. Table 1 shows the demographic and clinical information of the participants. There was no significant difference between the two groups in age and sex. Patients with AD had lower educational attainment than controls, which is consistent with previous studies [18].
Table 1
Characteristics of the study population
Patients (N= 242) | Controls (N= 208) | p | |
Female, n (%) | 157 (64.9%) | 127 (61.1%) | 0.40 |
Male, n (%) | 85 (35.1%) | 81 (38.9%) | |
Age at examination (y, mean±SD) | 72.81±9.56 | 71.19±9.76 | 0.076 |
Education level (y, mean±SD) | 8.32±4.97 | 11.95±3.23 | <0.001 |
MMSE score (mean±SD) | 12.08±5.46 | 29.08±0.76 | <0.001 |
CDT score (mean±SD) | 1.83±1.57 | 3.90±0.14 | <0.001 |
APOE genotype (%) | |||
ɛ2/ɛ2 | 2 (0.8%) | 1 (0.5%) | |
ɛ2/ɛ3 | 13 (5.4%) | 25 (12.0%) | |
ɛ2/ɛ4 | 7 (2.9%) | 5 (2.4%) | |
ɛ3/ɛ3 | 96 (39.7%) | 121 (58.2%) | |
ɛ3/ɛ4 | 102 (42.1%) | 50 (24.0%) | |
ɛ4/ɛ4 | 22 (9.1%) | 6 (2.9%) | |
APOE ɛ4 carriers | 131 (54.1%) | 61 (29.3%) | <0.001 |
MMSE, Mini-Mental State Examination; APOE, apolipoprotein E, APOE ɛ4 carriers have at least one copy of the ɛ4 allele; SD, standard deviation; Bolding indicates statistically significant values; CDT, clock drawing test.
Analysis of the association between SNPs and AD
All genotypes followed the HWE. The allele and genotype frequencies of the SNPs are listed in Supplementary Table 2. rs2741342 of CHRNA was associated with AD in allele and genotype frequencies (allele: p = 0.003, OR = 0.67, 95% confidence interval [CI] = 0.52–0.88; genotype: p = 0.005) (Table 2). Differences persisted after Bonferroni corrections for allele frequencies. The genotype or allele frequencies of rs6572869 in FERMT2, rs11604680, rs1317149, and rs11039280 in CELF1, rs9898218 in COPI, and rs3752242 in ABCA7 were also associated with AD (Table 2). However, these associations vanished after the Bonferroni correction.
Table 2
Association of SNP with AD risk
SNP | Gene | minor | P allele | P genotype | Dominant | Recessive | Additive | Overdominant |
allele | OR | p | p | p | p | |||
(95% CI) | OR (95% CI) | OR (95% CI) | OR (95% CI) | OR (95% CI) | ||||
rs6572869 | FERMT2 | A | 0.35 | 0.005 | 0.022 | 0.13 | 0.33 | 0.001 |
1.15 | 1.55 | 0.63 | 1.15 | 1.96 | ||||
0.87–1.53 | 1.07–2.26 | 0.35–1.14 | 0.87–1.51 | 1.32–2.92 | ||||
rs11604680 | CELF1 | G | 0.078 | 0.007 | 0.007 | 0.53 | 0.065 | 0.002 |
1.31 | 1.68 | 0.81 | 1.32 | 1.82 | ||||
0.98–1.75 | 1.15–2.44 | 0.41–1.56 | 0.98–1.78 | 1.24–2.67 | ||||
rs1317149 | CELF1 | A | 0.35 | 0.009 | 0.033 | 0.13 | 0.33 | 0.003 |
1.15 | 1.50 | 0.6 | 1.16 | 1.80 | ||||
0.87–1.53 | 1.03–2.19 | 0.31–1.16 | 0.87–1.55 | 1.23–2.64 | ||||
rs9898218 | COPI | T | 0.53 | 0.005 | 0.065 | 0.073 | 0.48 | 0.004 |
1.11 | 1.43 | 0.51 | 1.11 | 1.81 | ||||
0.82–1.51 | 0.98–2.10 | 0.24–1.07 | 0.83–1.50 | 1.21–2.71 | ||||
rs2741342 | CHRNA2 | A | 0.003 | 0.005 | 0.002 | 0.068 | 0.002 | 0.16 |
0.67 | 0.5 | 0.64 | 0.64 | 0.77 | ||||
0.52–0.88 | 0.33–0.77 | 0.4–1.03 | 0.48–0.85 | 0.53–1.11 | ||||
rs10742814 | CELF1 | A | 0.23 | 0.17 | 0.61 | 0.039 | 0.17 | 0.39 |
0.84 | 0.91 | 0.55 | 0.83 | 1.18 | ||||
0.63–1.10 | 0.62–1.32 | 0.31–0.97 | 0.63–1.09 | 0.81–1.72 | ||||
rs11039280 | CELF1 | A | 0.046 | 0.064 | 0.26 | 0.016 | 0.048 | 0.63 |
0.75 | 0.81 | 0.49 | 0.76 | 1.1 | ||||
0.57–0.99 | 0.56–1.17 | 0.28–0.88 | 0.58–0.99 | 0.75–1.61 | ||||
rs3752242 | ABCA7 | A | 0.037 | 0.10 | 0.047 | 0.26 | 0.049 | 0.23 |
0.74 | 0.68 | 0.73 | 0.76 | 0.80 | ||||
0.57–0.98 | 0.47–0.99 | 0.43–1.26 | 0.58–1.00 | 0.55–1.16 |
The P allele and P genotype were examined using the Chi-squared test. The p value was adjusted for sex and age using binary logistic regression. Bold indicates statistically significant values.
Four risk SNPs and four protective SNPs associated with AD were identified. Adjusted for sex and age, rs6572869 of FERMT2 and rs11604680 and rs1317149 of CELF1 were associated with the risk of AD in the dominant (rs6572869: p = 0.022, OR = 1.55, 95 % CI = 1.07–2.26; rs11604680: p = 0.007, OR = 1.68, 95% CI = 1.15–2.44; rs1317149: p = 0.033, OR = 1.50, 95% CI = 1.03–2.19) and overdominant models (rs6572869: p = 0.001, OR = 1.96, 95% CI = 1.32–2.92; rs11604680: p = 0.002, OR = 1.82, 95% CI = 1.24–2.67; rs1317149: p = 0.003, OR = 1.80, 95% CI = 1.23–2.64) (Table 2). After the Bonferroni correction, these differences persisted in the overdominant model. In addition, rs9898218 of COPI is associated with AD risk in the overdominant model (p = 0.004, OR = 1.81, 95% CI = 1.21–2.71) (Table 2).
Further, rs2741342 of CHRNA2 was associated with AD protection in the dominant (p = 0.002, OR = 0.5, 95% CI = 0.33–0.77) and additive models (p = 0.002, OR = 0.64, 95% CI = 0.48–0.85), even after Bonferroni correction (Table 2). The recessive model results of rs10742814 and rs11039280 of CELF1 showed that they are protective SNPs associated with AD (rs10742814: p = 0.039, OR = 0.55, 95% CI = 0.31–0.97; rs11039280: p = 0.016, OR = 0.49, 95% CI = 0.28–0.88) (Table 2). These associations vanished after the Bonferroni correction. The results also showed that rs3752242 (ABCA7) was marginally associated with AD protection in the dominant and additive models with AD protection (Table 2).
Association analysis of subgroups stratified by APOE ɛ4 status
As expected, APOE ɛ4 increased susceptibility to AD (Table 1). Next, we stratified the participants according to their APOE ɛ4 carrier status. Other discrepancies were observed in the allele and genotype frequencies of the above risk and protective SNPs combined with APOE ɛ4 (Supplementary Tables 4 and 6).
Adjusted for sex and age, the association of risk SNPs (rs6572869 of FERMT2, rs11604680 of CELF1, rs9898218 of COPI) and protective SNPs (rs2741342 of CHRNA2) persisted in AD with and without APOE ɛ4, consistent with the results when unstratified (Table 3). Protective SNPs (rs10742814 of CELF1, rs3752242 of ABCA7, and rs11039280 of CELF1) were still associated with AD in APOE ɛ4 carriers, and the differences were more pronounced, but not in APOE ɛ4 non-carriers (Table 3). Nonetheless, the risk SNP rs1317149 of CELF1 was significantly associated with AD in APOE ɛ4 non-carriers but not in APOE ɛ4 carriers (Table 3).
Table 3
Association of SNPs of candidate genes with AD risk stratified by APOE ɛ4 status
SNP | Gene | MAF | APOE ɛ4 | Dominant model (adjusted) | Recessive model (adjusted) | ||||
p | OR | 95% CI | p | OR | 95% CI | ||||
rs6572869 | FERMT2 | A | + | 0.009 | 2.33 | 1.23–4.40 | 0.2 | 2.32 | 0.64–8.39 |
– | 0.43 | 1.22 | 0.74–2.01 | 0.014 | 0.33 | 0.14–0.80 | |||
rs11604680 | CELF1 | G | + | 0.016 | 2.20 | 1.16–4.17 | 0.95 | 1.03 | 0.34–3.15 |
– | 0.028 | 1.76 | 1.06–2.92 | 0.38 | 0.65 | 0.25–1.68 | |||
rs9898218 | COPI | T | + | 0.45 | 1.28 | 0.68–2.40 | 0.023 | 0.23 | 0.065–0.81 |
– | 0.066 | 1.61 | 0.97–2.66 | 0.82 | 0.90 | 0.35–2.29 | |||
rs1317149 | CELF1 | A | + | 0.058 | 1.85 | 0.98–3.47 | 0.95 | 1.03 | 0.34–3.15 |
– | 0.038 | 1.72 | 1.03–2.86 | 0.062 | 0.4 | 0.15–1.05 | |||
rs2741342 | CHRNA2 | A | + | 0.028 | 0.43 | 0.20–0.91 | 0.15 | 0.56 | 0.25–1.24 |
– | 0.017 | 0.51 | 0.29–0.89 | 0.41 | 0.77 | 0.42–1.43 | |||
rs10742814 | CELF1 | A | + | 0.39 | 0.76 | 0.40–1.43 | 0.004 | 0.30 | 0.13–0.68 |
– | 0.77 | 0.93 | 0.57–1.52 | 0.35 | 0.66 | 0.28–1.58 | |||
rs3752242 | ABCA7 | A | + | 0.042 | 0.52 | 0.28–0.98 | 0.035 | 0.40 | 0.17–0.94 |
– | 0.69 | 0.90 | 0.54–1.50 | 0.81 | 1.09 | 0.53–2.25 | |||
rs11039280 | CELF1 | A | + | 0.24 | 0.68 | 0.36–1.29 | 0.004 | 0.30 | 0.13–0.68 |
– | 0.31 | 0.77 | 0.47–1.27 | 0.16 | 0.53 | 0.22–1.28 | |||
rs4147912 | ABCA7 | A | + | 0.034 | 2.28 | 1.06–4.87 | 0.68 | 1.60 | 0.17–14.74 |
– | 0.94 | 1.02 | 0.61–1.72 | 0.45 | 2.53 | 0.22–28.51 | |||
rs2516049 | HLA-DRB1 | G | + | 0.69 | 1.14 | 0.60–2.16 | 0.033 | 0.30 | 0.10–0.91 |
– | 0.61 | 0.87 | 0.53–1.45 | 0.69 | 1.30 | 0.36–4.67 | |||
rs1109581 | ADGRF4 | A | + | 0.33 | 0.72 | 0.37–1.40 | 0.81 | 0.83 | 0.19–3.66 |
– | 0.04 | 1.85 | 1.03–3.32 | 0.40 | 0.49 | 0.092–2.58 | |||
SNP | Gene | MAF | APOE ɛ4 | Additive model (adjusted) | Overdominant model (adjusted) | ||||
p | OR | 95% CI | p | OR | 95% CI | ||||
rs6572869 | FERMT2 | A | + | 0.01 | 1.99 | 1.17–3.36 | 0.058 | 1.88 | 0.98–3.61 |
– | 0.53 | 0.89 | 0.62–1.28 | 0.011 | 1.97 | 1.17–3.33 | |||
rs11604680 | CELF1 | G | + | 0.058 | 1.64 | 0.98–2.74 | 0.015 | 2.32 | 1.18–4.56 |
– | 0.18 | 1.31 | 0.88–1.94 | 0.007 | 1.99 | 1.20–3.30 | |||
rs9898218 | COPI | T | + | 0.72 | 0.91 | 0.56–1.50 | 0.044 | 2.02 | 1.02–4.02 |
– | 0.18 | 1.30 | 0.88–1.92 | 0.04 | 1.74 | 1.03–2.94 | |||
rs1317149 | CELF1 | A | + | 0.14 | 1.47 | 0.89–2.43 | 0.056 | 1.90 | 0.98–3.69 |
– | 0.46 | 1.16 | 0.79–1.70 | 0.002 | 2.29 | 1.37–3.82 | |||
rs2741342 | CHRNA2 | A | + | 0.021 | 0.57 | 0.35–0.92 | 0.33 | 0.73 | 0.39–1.38 |
– | 0.044 | 0.69 | 0.48–0.99 | 0.14 | 0.69 | 0.42–1.14 | |||
rs10742814 | CELF1 | A | + | 0.036 | 0.63 | 0.41–0.97 | 0.19 | 1.55 | 0.81–2.95 |
– | 0.52 | 0.88 | 0.60–1.29 | 0.80 | 1.07 | 0.65–1.76 | |||
rs3752242 | ABCA7 | A | + | 0.015 | 0.59 | 0.38–0.90 | 0.57 | 0.83 | 0.43–1.59 |
– | 0.87 | 0.97 | 0.67–1.4 | 0.59 | 0.87 | 0.53–1.43 | |||
rs11039280 | CELF1 | A | + | 0.022 | 0.60 | 0.39–0.93 | 0.32 | 1.39 | 0.73–2.64 |
– | 0.16 | 0.76 | 0.52–1.11 | 0.87 | 0.96 | 0.58–1.59 | |||
rs4147912 | ABCA7 | A | + | 0.046 | 2.03 | 1.01–4.07 | 0.043 | 2.24 | 1.02–4.91 |
– | 0.81 | 1.06 | 0.65–1.74 | 0.92 | 0.97 | 0.58–1.65 | |||
rs2516049 | HLA-DRB1 | G | + | 0.52 | 0.86 | 0.53–1.38 | 0.084 | 1.89 | 0.92–3.88 |
– | 0.76 | 0.93 | 0.60–1.45 | 0.49 | 0.83 | 0.49–1.40 | |||
rs1109581 | ADGRF4 | A | + | 0.38 | 0.78 | 0.46–1.35 | 0.36 | 0.72 | 0.36–1.46 |
– | 0.14 | 1.45 | 0.88–2.39 | 0.013 | 2.18 | 1.18–4.04 |
p-values were adjusted for sex and age using binary logistic regression. Bold indicates statistically significant values.
Interestingly, rs4147912 of ABCA7 and rs2516049 of HLA-DRB1 were identified to be relevant with AD in APOE ɛ4 carriers (dominant model of rs4147912: p = 0.034, OR = 2.28, 95% CI = 1.06–4.87; additive model of rs4147912: p = 0.046, OR = 2.03, 95% CI = 1.01–4.07; overdominant model of rs4147912: p = 0.043, OR = 2.24, 95% CI = 1.02–4.91; recessive model of rs2516049: p = 0.033, OR = 0.30, 95% CI = 0.10–0.91) (Table 3). Moreover, in non-APOE ɛ4 carriers, rs1109581 of ADGRF4 in the dominant and overdominant models was associated with AD (dominant model: p = 0.04, OR = 1.85, 95% CI = 1.03–3.32; overdominant model: p = 0.013, OR = 2.18, 95% CI = 1.18–4.04) (Table 3). However, these correlations were not available after Bonferroni correction (Table 3).
Analysis of the association between SNPs and related gene expression
We applied eQTL analysis to investigate the correlation between genotype and gene expression of the above 11 candidate loci to understand further the relationship between the loci and the pathogenesis of AD. According to GTEx, we found that the TT genotype at rs2516049 was associated with the high expression level of HLA-DRB1 in multiple brain regions, such as the anterior cingulate cortex (BA24), cortex, frontal cortex (BA9), putamen (basal ganglia), amygdala, cerebellum, caudate (basal ganglia), nucleus accumbens (basal ganglia), cerebellar hemisphere, substantia nigra, hypothalamus, and hippocampus (Table 4). In addition, the TT genotype at rs1109581 was associated with a higher level of ADGRF4 in the cerebellum (Table 4). According to the Braineac database, the A allele of rs4147912 was positively correlated with ABCA7 expression in the frontal cortex (p = 0.00012, probe set 3815488). Both A alleles of rs10742814 and rs11039280 increased CELF1 expression in the medulla oblongata (rs10742814: p = 0.00034, probe set 3372267; rs11039280: p = 0.00032, probe set 3372267) (Fig. 1). In the GTEx and Braineac databases, no other genotypes of SNPs were found to be significantly correlated with the expression levels of related genes in the brain.
Fig. 1
Effect of rs4147912, rs10742814, and rs11039280 on ABCA7 and CELF1 expression in normal human brain regions in Braineac. a) The A allele of rs4147912 increased ABCA7 expression in the frontal cortex with probe set 3815488 (p = 0.00012). b) The A allele of rs10742814 increased CELF1 expression in the medulla oblongata with probe set 3372267 (p = 0.00034). c) The A allele of rs11039280 increased CELF1 expression in the medulla oblongata with probe set 3372267 (p = 0.00032).
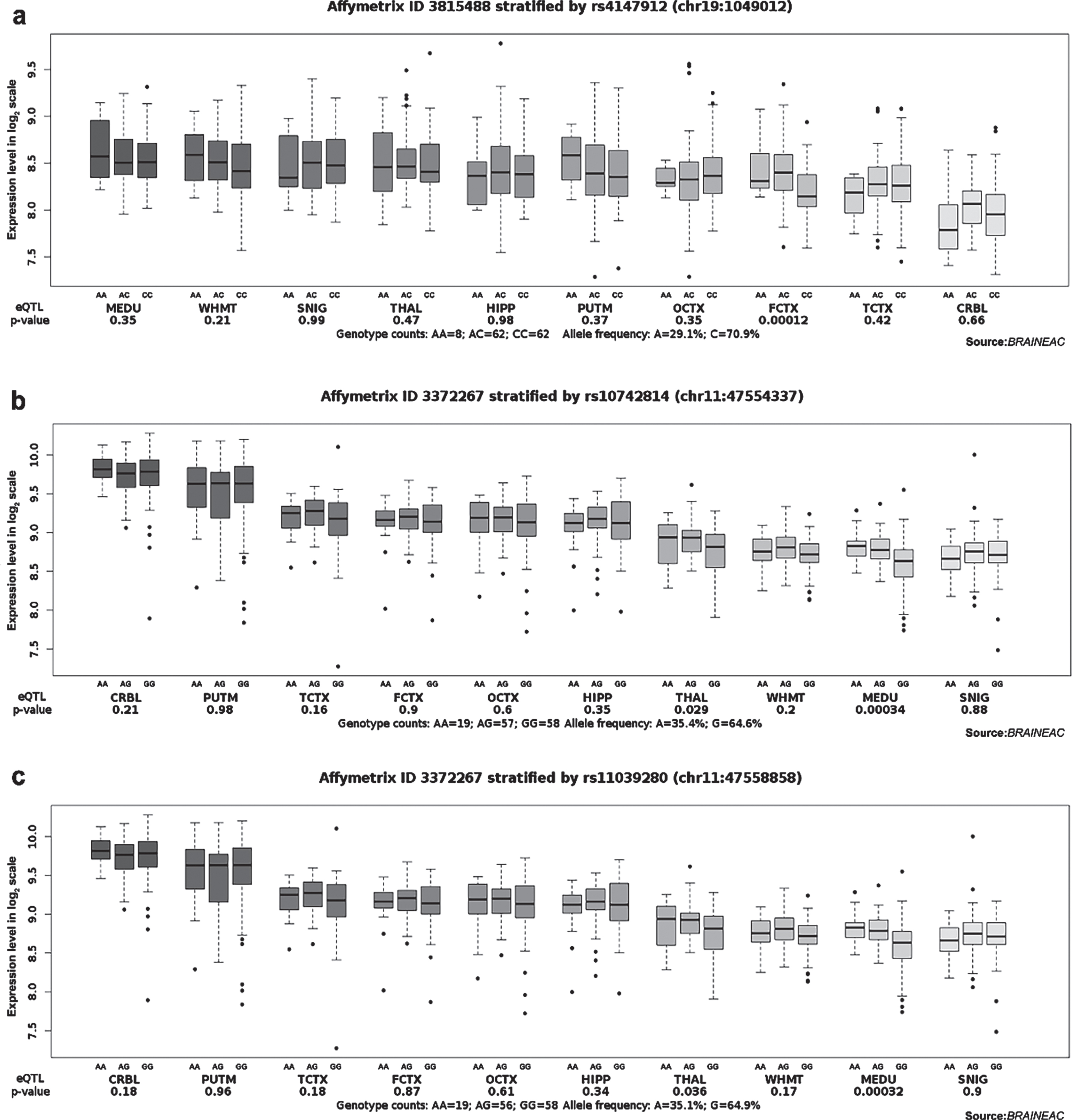
Table 4
Effects of rs2516049 and rs1109581 on HLA-DRB1 and ADGRF4 gene expression in different regions of the normal human brain in GTEx
SNP | Variant ID | Tissue | Samples | Genotype | p | NES | ||
TT | TC | CC | ||||||
rs2516049 | chr6_32602623_T_C_b38 | Brain - Anterior cingulate cortex (BA24) | 147 | 72 | 58 | 17 | 1.00E-16 | –0.59 |
Brain - Cortex | 205 | 115 | 69 | 21 | 8.40E-13 | –0.46 | ||
Brain - Frontal Cortex (BA9) | 175 | 92 | 66 | 17 | 2.30E-11 | –0.44 | ||
Brain - Putamen (basal ganglia) | 170 | 89 | 69 | 12 | 3.80E-11 | –0.51 | ||
Brain - Amygdala | 129 | 58 | 59 | 12 | 2.40E-10 | –0.34 | ||
Brain - Cerebellum | 209 | 105 | 85 | 19 | 1.10E-09 | –0.44 | ||
Brain - Caudate (basal ganglia) | 193 | 103 | 73 | 17 | 1.20E-09 | –0.34 | ||
Brain - Nucleus accumbens (basal ganglia) | 202 | 102 | 81 | 19 | 2.30E-09 | –0.37 | ||
Brain - Cerebellar Hemisphere | 175 | 88 | 68 | 19 | 2.80E-09 | –0.44 | ||
Brain - Substantia nigra | 114 | 58 | 47 | 9 | 7.90E-08 | –0.35 | ||
Brain - Hypothalamus | 170 | 90 | 66 | 14 | 4.00E-07 | –0.28 | ||
Brain - Hippocampus | 165 | 81 | 68 | 16 | 0.0000015 | –0.25 | ||
SNP | Variant ID | Tissue | Samples | Genotype | p | NES | ||
CC | CT | TT | ||||||
rs1109581 | chr6_47710446_C_T_b38 | Brain - Cerebellum | 209 | 114 | 83 | 12 | 0.000082 | 0.35 |
Samples: Number of RNA-seq samples with genotype, p-value: from a t-test that compares observed NES from single-tissue eQTL analysis to a null NES of 0, NES: normalized effect size, the slope of the linear regression of normalized expression data versus the three genotype categories using single-tissue eQTL analysis representing the eQTL effect size.
DISCUSSION
In this study, the associations between 17 SNPs in 12 genes and the risk of AD were validated. To our knowledge, these SNPs have been investigated for the first time in the Southern Chinese population. Four risk SNPs and four protective SNPs were identified. Mutations in rs6572869 (FERMT2), rs11604680 (CELF1), rs1317149 (CELF1), and rs9898218 (COPI) were associated with a higher susceptibility to AD, whereas rs2741342 (CHRNA2), rs10742814 (CELF1), rs11039280 (CELF1), and rs3752242 (ABCA7) were protective against AD pathogenesis. In addition, the correlation between the above-mentioned related SNPs and AD is different when participants are divided into two groups according to their APOE ɛ4 carrier status. Furthermore, individuals with the A allele at rs4147912 had a higher AD susceptibility in APOE ɛ4 carriers, while homozygous GG at rs2516049 reduced AD morbidity. Heterozygote AG at rs1109581 of ADGRF4 in APOE ɛ4 non-carriers increased the risk of AD. The associations of the remaining six SNPs with AD were not replicated in the Southern Chinese group (Supplementary Tables 3, 5, and 7). Similarly, various populations may carry different AD-risk genetic variants.
CELF1 is an essential gene associated with AD genetic factors [19]. Aret, the homologue of CELF1 in fly, was associated with AD by regulating tau protein aggregation [20]. Furthermore, previous studies have found a significant correlation between CELF1 and the establishment of hippocampal white matter integrity [21]. Moreover, the CELF1 variants may correlate with higher levels of Aβ42 in cerebrospinal fluid, thus associated with the AD pathology. In the Caucasian population, when rs11604680, rs1317149, rs10742814, and rs11039280 were aggregated with other SNPs, they were associated with cognition, but the association between a single SNP and AD has not been explored [22]. No studies have investigated the association between rs11604680, rs1317149, rs10742814, and rs11039280 of the CELF1 gene and AD risk in Asian populations. We provide evidence that heterozygous AG at rs11604680 and rs1317149 increased AD susceptibility, while homozygous AA at rs10742814 and rs11039280 decreased AD risk. Moreover, the correlations between these SNPs in APOE ɛ4 carriers and non-carriers were significantly inconsistent. For the first time, we hypothesized that they might interact with APOE and participate in the progression of AD, which needs to be confirmed by future studies.
ABCA7 encodes an ATP-binding cassette transporter that facilitates lipid efflux across membranes [23, 24]. ABCA7 is second only to APOE ɛ4 concerning amyloid deposition, and its loss-of-function increases β-secretase cleavage of the amyloid-β protein precursor (AβPP) [25]. ABCA7 knockout in mice carrying mutant human AβPPs increased insoluble Aβ deposition and amyloid plaques [26]. Two variants of ABCA7 (rs4147912 and rs3752242) were identified to be associated with cerebral amyloidosis and susceptibility to AD, but not with brain atrophy [25]. In this study, we discovered that the A allele at rs3752242 might be protective against AD, particularly in APOE ɛ4 carriers. Moreover, the A allele at rs4147912 is a risk factor for AD susceptibility in APOE ɛ4 carriers. We speculated that ABCA7 might interact with APOE ɛ4 and be associated with AD. APOE ɛ4 may promote the transport of oxidized lipids by ABCA7 through the production of reactive oxygen species (ROS). Studies have shown that APOE ɛ4 in astrocytes and neurons induce greater cPLA2 activation through p38 MAPK pathway, leading to more LTB4, iNOS, and ROS production, increased oxidative stress and neuroinflammation [27]. ROS induces neuronal lipid synthesis, leading to lipid peroxidation isolation in glial lipid droplets to delay neurotoxicity, and ABCA7 in neurons is involved in this process [28]. In addition, studies have shown that APOE ɛ4 is impaired in lipid transport and promotes neurodegeneration [29], which may have a synergistic effect with ABCA7 in this process.
FERMT2 is expressed in the brain, and its corresponding protein targets cell-matrix adhesion structures [30]. Reduction of FERMT2 in human neurons leads to reduced extracellular Aβ40 and Aβ42 levels and reduced total and phosphorylated tau, demonstrating the link between FERMT2 and AD pathology [31]. A recent study revealed that FERMT2 directly regulates AβPP metabolism and that inadequate expression of FERMT2 affects synaptic connectivity and axonal growth in an AβPP-dependent way [32]. GWAS results based on International Genomics of Alzheimer’s Project showed that rs6572869 of FERMT2 were significantly correlated with the incidence of AD [13, 33]. Our study confirmed that heterozygous AG of rs6572869 might be an influential genetic risk factor in AD pathophysiology.
Cell trafficking and recycling are risk mechanisms for AD [34]. COPI-dependent trafficking alters subcellular localization and cell surface expression of AβPP. COPI-dependent trafficking is significantly associated with the reduction of amyloid plaques in the neurocortex and hippocampus of the AD mouse [34]. A large meta-analysis examining transcriptional changes in ageing and AD found that COPI subunit zeta 2 (COPZ2) was upregulated in AD [35]. Analysis of an AD GWAS dataset of European ancestry demonstrated that rs9898218 of COPI is significantly associated with AD risk [36]. Consistent with these results, we provided the first supporting evidence for the association between rs9898218 and AD risk in the Southern Chinese population.
It remains unknown whether rs2741342 of CHRNA2 is relevant to AD risk in the Chinese population. CHRNA2 encodes the alpha-2 subunit of neuronal acetylcholine receptor (nAChR), which is expressed in the hippocampal CA1 region and influences hippocampal-dependent memory and the plasticity of CA1 hippocampal synapses [37, 38]. CHRNA2 is currently one of the targets of AD clinical drug research [39]. rs2741342 has been confirmed to be associated with AD risk in people with Caucasian ancestry [13]. However, our study observed that the A allele of rs2741342 reduces susceptibility to AD, which may be due to race specificity and requires further validation.
HLA-DRB1, a genetic factor in AD pathogenesis, has shown a nominal association with the diagnosis of pathological AD, and methylation in this locus is associated with Aβ loading correlated with tau entanglement density [6, 40]. Analysis of GWAS from North America and Europe found that rs2516049 was significantly associated with neurofibrillary tangle pathology and correlated with AD progression [41]. rs1109581 is in an intron of ADGRF4, which is an adhesion G-protein-coupled receptor [42]. And GWAS results based on IGAP showed that rs1109581 of ADGRF4 were significantly correlated with the incidence of AD [13, 33]. Our results showed that, in the Southern Chinese population, homozygosity for the G allele of rs2516049 (HLA-DRB1) protects APOE ɛ4 carriers from AD susceptibility, whereas mutation of rs1109581 (ADGRF4) may increase the risk of AD in APOE ɛ4 non-carriers. The association of rs2516049 and rs1109581 with HLA-DRB1 and ADGRF4 expression in the brain suggested that mutations in these two loci might be involved in AD pathogenesis by affecting gene expression and interacting with APOE, which requires confirmation in future studies.
The associations between the other six SNPs and AD risk could not be replicated in this study, which may be due to heterogeneity in ethnic origin and/or small sample size [12, 43]. eQTL analysis can establish a link between genotype and gene expression patterns and help identify disease-causing genes. Many eQTLs affect the expression of local transcripts and distal genes [43]. Based on eQTL analysis, we further found that polymorphisms in HLA-DRB1 (rs2516049), ADGRF4 (rs1109581), CELF1 (rs10742814 and rs11039280), and ABCA7 (rs4147912) correlated with their corresponding gene expression in the brain, which demonstrated that polymorphisms in susceptibility loci might affect gene expression in different regions of the brain and thus affect AD pathogenesis. Meanwhile, positive eQTL association analysis could support the validity of this study and contribute to understanding the role of genetic variation in AD-related mechanisms.
In conclusion, our study identified several risk SNPs and protective SNPs associated with AD that have never been reported in Asian populations. Different SNP variants are either a risk factor or protective factor for AD and are associated with APOE ɛ4 carrier status, suggesting that the polymorphism may interact with APOE. Genetic polymorphisms remain insufficiently understood in the development of AD. AD pathogenesis is highly correlated with genetic factors, and the identification of AD-associated loci could provide a reliable basis for timely and reliable diagnosis. Our findings provide new insights into the relationship between risk loci and AD development in a southern Chinese population and contribute to the general understanding of the genetic basis of AD risk. Given the association between these loci and AD risk, future studies must investigate the role of risk genes in the pathogenesis of AD, as demonstrated here, to help further inform future AD-targeted therapies.
ACKNOWLEDGMENTS
We thank all the patients and healthy controls who participated in this study.
FUNDING
This study was supported by grants from the National Natural Science Foundation of China (82171400) and the Natural Science Foundation of Shanghai (19ZR1432500).
CONFLICT OF INTEREST
The authors have no conflict of interest to report.
DATA AVAILABILITY
The data supporting the findings of this study are available on request from the corresponding author. The data are not publicly available due to privacy or ethical restrictions.
SUPPLEMENTARY MATERIAL
[1] The supplementary material is available in the electronic version of this article: https://dx.doi.org/10.3233/ADR-230072.
REFERENCES
[1] | Silva MVF , Loures CMG , Alves LCV , de Souza LC , Borges KBG , Carvalho MDG ((2019) ) Alzheimer’s disease: Risk factors and potentially protective measures. J Biomed Sci 26: , 33. |
[2] | Gatz M , Reynolds CA , Fratiglioni L , Johansson B , Mortimer JA , Berg S , Fiske A , Pedersen NL ((2006) ) Role of genes and environments for explaining Alzheimer disease. Arch Gen Psychiatry 63: , 168–174. |
[3] | Corder EH , Saunders AM , Strittmatter WJ , Schmechel DE , Gaskell PC , Small GW , Roses AD , Haines JL , Pericak-Vance MA ((1993) ) Gene dose of apolipoprotein E type 4 allele and the risk of Alzheimer’s disease in late onset families. Science 261: , 921–923. |
[4] | Liu CC , Liu CC , Kanekiyo T , Xu H , Bu G ((2013) ) Apolipoprotein E and Alzheimer disease: Risk, mechanisms and therapy. Nat Rev Neurol 9: , 106–118. |
[5] | Griciuc A , Tanzi RE ((2021) ) The role of innate immune genes in Alzheimer’s disease. Curr Opin Neurol 34: , 228–236. |
[6] | Lambert JC , Ibrahim-Verbaas CA , Harold D , Naj AC , Sims R , Bellenguez C , DeStafano AL , Bis JC , Beecham GW , Grenier-Boley B , Russo G , Thorton-Wells TA , Jones N , Smith AV , Chouraki V , Thomas C , Ikram MA , Zelenika D , Vardarajan BN , Kamatani Y , Lin CF , Gerrish A , Schmidt H , Kunkle B , Dunstan ML , Ruiz A , Bihoreau MT , Choi SH , Reitz C , Pasquier F , Cruchaga C , Craig D , Amin N , Berr C , Lopez OL , De Jager PL , Deramecourt V , Johnston JA , Evans D , Lovestone S , Letenneur L , Morón FJ , Rubinsztein DC , Eiriksdottir G , Sleegers K , Goate AM , Fiévet N , Huentelman MW , Gill M , Brown K , Kamboh MI , Keller L , Barberger-Gateau P , McGuiness B , Larson EB , Green R , Myers AJ , Dufouil C , Todd S , Wallon D , Love S , Rogaeva E , Gallacher J , St George-Hyslop P , Clarimon J , Lleo A , Bayer A , Tsuang DW , Yu L , Tsolaki M , Bossù P , Spalletta G , Proitsi P , Collinge J , Sorbi S , Sanchez-Garcia F , Fox NC , Hardy J , Deniz Naranjo MC , Bosco P , Clarke R , Brayne C , Galimberti D , Mancuso M , Matthews F , Moebus S , Mecocci P , Del Zompo M , Maier W , Hampel H , Pilotto A , Bullido M , Panza F , Caffarra P , Nacmias B , Gilbert JR , Mayhaus M , Lannefelt L , Hakonarson H , Pichler S , Carrasquillo MM , Ingelsson M , Beekly D , Alvarez V , Zou F , Valladares O , Younkin SG , Coto E , Hamilton-Nelson KL , Gu W , Razquin C , Pastor P , Mateo I , Owen MJ , Faber KM , Jonsson PV , Combarros O , O’Donovan MC , Cantwell LB , Soininen H , Blacker D , Mead S , Mosley TH Jr , Bennett DA , Harris TB , Fratiglioni L , Holmes C , de Bruijn RF , Passmore P , Montine TJ , Bettens K , Rotter JI , Brice A , Morgan K , Foroud TM , Kukull WA , Hannequin D , Powell JF , Nalls MA , Ritchie K , Lunetta KL , Kauwe JS , Boerwinkle E , Riemenschneider M , Boada M , Hiltuenen M , Martin ER , Schmidt R , Rujescu D , Wang LS , Dartigues JF , Mayeux R , Tzourio C , Hofman A , Nöthen MM , Graff C , Psaty BM , Jones L , Haines JL , Holmans PA , Lathrop M , Pericak-Vance MA , Launer LJ , Farrer LA , van Duijn CM , Van Broeckhoven C , Moskvina V , Seshadri S , Williams J , Schellenberg GD , Amouyel P ((2013) ) Meta-analysis of 74,046 individuals identifies 11 new susceptibility loci for Alzheimer’s disease. Nat Genet 45: , 1452–1458. |
[7] | Lambert JC , Heath S , Even G , Campion D , Sleegers K , Hiltunen M , Combarros O , Zelenika D , Bullido MJ , Tavernier B , Letenneur L , Bettens K , Berr C , Pasquier F , Fiévet N , Barberger-Gateau P , Engelborghs S , De Deyn P , Mateo I , Franck A , Helisalmi S , Porcellini E , Hanon O , de Pancorbo MM , Lendon C , Dufouil C , Jaillard C , Leveillard T , Alvarez V , Bosco P , Mancuso M , Panza F , Nacmias B , Bossù P , Piccardi P , Annoni G , Seripa D , Galimberti D , Hannequin D , Licastro F , Soininen H , Ritchie K , Blanché H , Dartigues JF , Tzourio C , Gut I , Van Broeckhoven C , Alpérovitch A , Lathrop M , Amouyel P ((2009) ) Genome-wide association study identifies variants at CLU and CR1 associated with Alzheimer’s disease. Nat Genet 41: , 1094–1099. |
[8] | Seshadri S , Fitzpatrick AL , Ikram MA , DeStefano AL , Gudnason V , Boada M , Bis JC , Smith AV , Carassquillo MM , Lambert JC , Harold D , Schrijvers EM , Ramirez-Lorca R , Debette S , Longstreth WT Jr. , Janssens AC , Pankratz VS , Dartigues JF , Hollingworth P , Aspelund T , Hernandez I , Beiser A , Kuller LH , Koudstaal PJ , Dickson DW , Tzourio C , Abraham R , Antunez C , Du Y , Rotter JI , Aulchenko YS , Harris TB , Petersen RC , Berr C , Owen MJ , Lopez-Arrieta J , Varadarajan BN , Becker JT , Rivadeneira F , Nalls MA , Graff-Radford NR , Campion D , Auerbach S , Rice K , Hofman A , Jonsson PV , Schmidt H , Lathrop M , Mosley TH , Au R , Psaty BM , Uitterlinden AG , Farrer LA , Lumley T , Ruiz A , Williams J , Amouyel P , Younkin SG , Wolf PA , Launer LJ , Lopez OL , van Duijn CM , Breteler MM ((2010) ) Genome-wide analysis of genetic loci associated with Alzheimer disease. JAMA 303: , 1832–1840. |
[9] | Wightman DP , Jansen IE , Savage JE , Shadrin AA , Bahrami S , Holland D , Rongve A , Børte S , Winsvold BS , Drange OK , Martinsen AE , Skogholt AH , Willer C , Bråthen G , Bosnes I , Nielsen JB , Fritsche LG , Thomas LF , Pedersen LM , Gabrielsen ME , Johnsen MB , Meisingset TW , Zhou W , Proitsi P , Hodges A , Dobson R , Velayudhan L , Heilbron K , Auton A , Sealock JM , Davis LK , Pedersen NL , Reynolds CA , Karlsson IK , Magnusson S , Stefansson H , Thordardottir S , Jonsson PV , Snaedal J , Zettergren A , Skoog I , Kern S , Waern M , Zetterberg H , Blennow K , Stordal E , Hveem K , Zwart JA , Athanasiu L , Selnes P , Saltvedt I , Sando SB , Ulstein I , Djurovic S , Fladby T , Aarsland D , Selbæk G , Ripke S , Stefansson K , Andreassen OA , Posthuma D ((2021) ) A genome-wide association study with 1,126,563 individuals identifies new risk loci for Alzheimer’s disease. Nat Genet 53: , 1276–1282. |
[10] | Tan MS , Yang YX , Xu W , Wang HF , Tan L , Zuo CT , Dong Q , Tan L , Suckling J , Yu JT ((2021) ) Associations of Alzheimer’s disease risk variants with gene expression, amyloidosis, tauopathy, and neurodegeneration. Alzheimers Res Ther 13: , 15. |
[11] | Tan L , Yu JT , Zhang W , Wu ZC , Zhang Q , Liu QY , Wang W , Wang HF , Ma XY , Cui WZ ((2013) ) Association of GWAS-linked loci with late-onset Alzheimer’s disease in a northern Han Chinese population. Alzheimers Dement 9: , 546–553. |
[12] | Mao YF , Guo ZY , Pu JL , Chen YX , Zhang BR ((2015) ) Association of CD33 and MS4A cluster variants with Alzheimer’s disease in East Asian populations. Neurosci Lett 609: , 235–239. |
[13] | Leonenko G , Sims R , Shoai M , Frizzati A , Bossù P , Spalletta G , Fox NC , Williams J , Hardy J , Escott-Price V ((2019) ) Polygenic risk and hazard scores for Alzheimer’s disease prediction. Ann Clin Transl Neurol 6: , 456–465. |
[14] | Dubois B , Feldman HH , Jacova C , Dekosky ST , Barberger-Gateau P , Cummings J , Delacourte A , Galasko D , Gauthier S , Jicha G , Meguro K , O’Brien J , Pasquier F , Robert P , Rossor M , Salloway S , Stern Y , Visser PJ , Scheltens P ((2007) ) Research criteria for the diagnosis of Alzheimer’s disease: Revising the NINCDS-ADRDA criteria. Lancet Neurol 6: , 734–746. |
[15] | McKhann GM , Knopman DS , Chertkow H , Hyman BT , Jack CR Jr. , Kawas CH , Klunk WE , Koroshetz WJ , Manly JJ , Mayeux R , Mohs RC , Morris JC , Rossor MN , Scheltens P , Carrillo MC , Thies B , Weintraub S , Phelps CH ((2011) ) The diagnosis of dementia due to Alzheimer’s disease: Recommendations from the National Institute on Aging-Alzheimer’s Association workgroups on diagnostic guidelines for Alzheimer’s disease. Alzheimers Dement 7: , 263–269. |
[16] | Janelidze S , Mattsson N , Stomrud E , Lindberg O , Palmqvist S , Zetterberg H , Blennow K , Hansson O ((2018) ) CSF biomarkers of neuroinflammation and cerebrovascular dysfunction in early Alzheimer disease. Neurology 91: , e867–e877 |
[17] | Jack CR Jr. , Bennett DA , Blennow K , Carrillo MC , Dunn B , Haeberlein SB , Holtzman DM , Jagust W , Jessen F , Karlawish J , Liu E , Molinuevo JL , Montine T , Phelps C , Rankin KP , Rowe CC , Scheltens P , Siemers E , Snyder HM , Sperling R ((2018) ) NIA-AA Research Framework: Toward a biological definition of Alzheimer’s disease. Alzheimers Dement 14: , 535–562. |
[18] | Larsson SC , Traylor M , Malik R , Dichgans M , Burgess S , Markus HS ((2017) ) Modifiable pathways in Alzheimer’s disease: Mendelian randomisation analysis. BMJ 359: , j5375. |
[19] | Hinney A , Albayrak O , Antel J , Volckmar AL , Sims R , Chapman J , Harold D , Gerrish A , Heid IM , Winkler TW , Scherag A , Wiltfang J , Williams J , Hebebrand J ((2014) ) Genetic variation at the CELF1 (CUGBP, elav-like family member 1 gene) locus is genome-wide associated with Alzheimer’s disease and obesity. Am J Med Genet B Neuropsychiatr Genet 165b: , 283–293. |
[20] | Shulman JM , Imboywa S , Giagtzoglou N , Powers MP , Hu Y , Devenport D , Chipendo P , Chibnik LB , Diamond A , Perrimon N , Brown NH , De Jager PL , Feany MB ((2014) ) Functional screening in Drosophila identifies Alzheimer’s disease susceptibility genes and implicates Tau-mediated mechanisms. Hum Mol Genet 23: , 870–877. |
[21] | Horgusluoglu-Moloch E , Xiao G , Wang M , Wang Q , Zhou X , Nho K , Saykin AJ , Schadt E , Zhang B ((2020) ) Systems modeling of white matter microstructural abnormalities in Alzheimer’s disease. Neuroimage Clin 26: , 102203. |
[22] | Nettiksimmons J , Tranah G , Evans DS , Yokoyama JS , Yaffe K ((2016) ) Gene-based aggregate SNP associations between candidate AD genes and cognitive decline. Age (Dordr) 38: , 41. |
[23] | Kaminski WE , Orsó E , Diederich W , Klucken J , Drobnik W , Schmitz G ((2000) ) Identification of a novel human sterol-sensitive ATP-binding cassette transporter (ABCA7). Biochem Biophys Res Commun 273: , 532–538. |
[24] | Kim WS , Weickert CS , Garner B ((2008) ) Role of ATP-binding cassette transporters in brain lipid transport and neurological disease. J Neurochem 104: , 1145–1166. |
[25] | Apostolova LG , Risacher SL , Duran T , Stage EC , Goukasian N , West JD , Do TM , Grotts J , Wilhalme H , Nho K , Phillips M , Elashoff D , Saykin AJ ((2018) ) Associations of the top 20 Alzheimer disease risk variants with brain amyloidosis. JAMA Neurol 75: , 328–341. |
[26] | Kim WS , Li H , Ruberu K , Chan S , Elliott DA , Low JK , Cheng D , Karl T , Garner B ((2013) ) Deletion of Abca7 increases cerebral amyloid-β accumulation in the J20 mouse model of Alzheimer’s disease. J Neurosci 33: , 4387–4394. |
[27] | Wang S , Li B , Solomon V , Fonteh A , Rapoport SI , Bennett DA , Arvanitakis Z , Chui HC , Sullivan PM , Yassine HN ((2022) ) Calcium-dependent cytosolic phospholipase A(2) activation is implicated in neuroinflammation and oxidative stress associated with ApoE4. Mol Neurodegener 17: , 42. |
[28] | Moulton MJ , Barish S , Ralhan I , Chang J , Goodman LD , Harland JG , Marcogliese PC , Johansson JO , Ioannou MS , Bellen HJ ((2021) ) Neuronal ROS-induced glial lipid droplet formation is altered by loss of Alzheimer’s disease-associated genes. Proc Natl Acad Sci U S A 118: , e2112095118. |
[29] | Liu L , MacKenzie KR , Putluri N , Maletić-Savatić M , Bellen HJ ((2017) ) The glia-neuron lactate shuttle and elevated ROS promote lipid synthesis in neurons and lipid droplet accumulation in glia via APOE/D. Cell Metab 26: , 719–737.e716. |
[30] | Pluskota E , Dowling JJ , Gordon N , Golden JA , Szpak D , West XZ , Nestor C , Ma YQ , Bialkowska K , Byzova T , Plow EF ((2011) ) The integrin coactivator kindlin-2 plays a critical role in angiogenesis in mice and zebrafish. Blood 117: , 4978–4987. |
[31] | Sullivan SE , Liao M , Smith RV , White C , Lagomarsino VN , Xu J , Taga M , Bennett DA , De Jager PL , Young-Pearse TL ((2019) ) Candidate-based screening via gene modulation in human neurons and astrocytes implicates FERMT2 in Aβ and TAU proteostasis. Hum Mol Genet 28: , 718–735. |
[32] | Eysert F , Coulon A , Boscher E , Vreulx AC , Flaig A , Mendes T , Hughes S , Grenier-Boley B , Hanoulle X , Demiautte F , Bauer C , Marttinen M , Takalo M , Amouyel P , Desai S , Pike I , Hiltunen M , Chécler F , Farinelli M , Delay C , Malmanche N , Hébert SS , Dumont J , Kilinc D , Lambert JC , Chapuis J ((2021) ) Alzheimer’s genetic risk factor FERMT2 (Kindlin-2) controls axonal growth and synaptic plasticity in an APP-dependent manner. Mol Psychiatry 26: , 5592–5607. |
[33] | Desikan RS , Fan CC , Wang Y , Schork AJ , Cabral HJ , Cupples LA , Thompson WK , Besser L , Kukull WA , Holland D , Chen CH , Brewer JB , Karow DS , Kauppi K , Witoelar A , Karch CM , Bonham LW , Yokoyama JS , Rosen HJ , Miller BL , Dillon WP , Wilson DM , Hess CP , Pericak-Vance M , Haines JL , Farrer LA , Mayeux R , Hardy J , Goate AM , Hyman BT , Schellenberg GD , McEvoy LK , Andreassen OA , Dale AM ((2017) ) Genetic assessment of age-associated Alzheimer disease risk: Development and validation of a polygenic hazard score. PLoS Med 14: , e1002258. |
[34] | Bettayeb K , Hooli BV , Parrado AR , Randolph L , Varotsis D , Aryal S , Gresack J , Tanzi RE , Greengard P , Flajolet M ((2016) ) Relevance of the COPI complex for Alzheimer’s disease progression. Proc Natl Acad Sci U S A 113: , 5418–5423. |
[35] | Ciryam P , Kundra R , Freer R , Morimoto RI , Dobson CM , Vendruscolo M ((2016) ) A transcriptional signature of Alzheimer’s disease is associated with a metastable subproteome at risk for aggregation. Proc Natl Acad Sci U S A 113: , 4753–4758. |
[36] | Yang Y , Wang X , Ju W , Sun L , Zhang H ((2019) ) Genetic and expression analysis of COPI genes and Alzheimer’s disease susceptibility. Front Genet 10: , 866. |
[37] | Demontis D , Rajagopal VM , Thorgeirsson TE , Als TD , Grove J , Leppälä K , Gudbjartsson DF , Pallesen J , Hjorthøj C , Reginsson GW , Tyrfingsson T , Runarsdottir V , Qvist P , Christensen JH , Bybjerg-Grauholm J , Bækvad-Hansen M , Huckins LM , Stahl EA , Timmermann A , Agerbo E , Hougaard DM , Werge T , Mors O , Mortensen PB , Nordentoft M , Daly MJ , Stefansson H , Stefansson K , Nyegaard M , Børglum AD ((2019) ) Genome-wide association study implicates CHRNA2 in cannabis use disorder. Nat Neurosci 22: , 1066–1074. |
[38] | Lotfipour S , Mojica C , Nakauchi S , Lipovsek M , Silverstein S , Cushman J , Tirtorahardjo J , Poulos A , Elgoyhen AB , Sumikawa K , Fanselow MS , Boulter J ((2017) ) α2* Nicotinic acetylcholine receptors influence hippocampus-dependent learning and memory in adolescent mice. Learn Mem 24: , 231–244. |
[39] | Xu Y , Kong J , Hu P ((2021) ) Computational drug repurposing for Alzheimer’s disease using risk genes from GWAS and single-cell RNA sequencing studies. Front Pharmacol 12: , 617537. |
[40] | Yu L , Chibnik LB , Srivastava GP , Pochet N , Yang J , Xu J , Kozubek J , Obholzer N , Leurgans SE , Schneider JA , Meissner A , De Jager PL , Bennett DA ((2015) ) Association of brain DNA methylation in SORL1, ABCA7, HLA-DRB5, SLC24A4, and BIN1 with pathological diagnosis of Alzheimer disease. JAMA Neurol 72: , 15–24. |
[41] | Yokoyama JS , Wang Y , Schork AJ , Thompson WK , Karch CM , Cruchaga C , McEvoy LK , Witoelar A , Chen CH , Holland D , Brewer JB , Franke A , Dillon WP , Wilson DM , Mukherjee P , Hess CP , Miller Z , Bonham LW , Shen J , Rabinovici GD , Rosen HJ , Miller BL , Hyman BT , Schellenberg GD , Karlsen TH , Andreassen OA , Dale AM , Desikan RS ((2016) ) Association between genetic traits for immune-mediated diseases and Alzheimer disease. JAMA Neurol 73: , 691–697. |
[42] | Yoon JH , Cho SG ((2020) ) ADGRF4 regulates non-small cell lung cancer cell invasiveness. Anticancer Res 40: , 6835–6844. |
[43] | Yan Y , Zhao A , Qui Y , Li Y , Yan R , Wang Y , Xu W , Deng Y ((2020) ) Genetic association of FERMT2, HLA-DRB1, CD2AP, and PTK2B polymorphisms with Alzheimer’s disease risk in the southern Chinese population. Front Aging Neurosci 12: , 16. |