Alzheimer’s Disease-Related Proteins Targeted by Secondary Metabolite Compounds from Streptomyces: A Scoping Review
Abstract
Background:
Alzheimer’s disease (AD) is a neurodegenerative disease that is characterized as rapid and progressive cognitive decline affecting 26 million people worldwide. Although immunotherapies are ideal, its clinical safety and effectiveness are controversial, hence, treatments are still reliant on symptomatic medications. Concurrently, the Streptomyces genus has attracted attention given its pharmaceutically beneficial secondary metabolites to treat neurodegenerative diseases.
Objective:
To present secondary metabolites from Streptomyces sp. with regulatory effects on proteins and identified prospective target proteins for AD treatment.
Methods:
Research articles published between 2010 and 2021 were collected from five databases and 83 relevant research articles were identified. Post-screening, only 12 research articles on AD-related proteins were selected for further review. Bioinformatics analyses were performed through the Search Tool for the Retrieval of Interacting Genes/Proteins (STRING) network, PANTHER Go-Slim classification system (PANTHER17.0), and Kyoto Encyclopedia of Genes and Genomes (KEGG) Mapper.
Results:
A total of 20 target proteins were identified from the 12 shortlisted articles. Amyloid-β, BACE1, Nrf-2, Beclin-1, and ATG5 were identified as the potential target proteins, given their role in initiating AD, mitigating neuroinflammation, and autophagy. Besides, 10 compounds from Streptomyces sp., including rapamycin, alborixin, enterocin, bonnevillamides D and E, caniferolide A, anhydroexfoliamycin, rhizolutin, streptocyclinone A and B, were identified to exhibit considerable regulatory effects on these target proteins.
Conclusions:
The review highlights several prospective target proteins that can be regulated through treatments with Streptomyces sp. compounds to prevent AD’s early stages and progression. Further identification of Streptomyces sp. compounds with potential anti-AD properties is recommended.
INTRODUCTION
Neurodegenerative diseases, such as Alzheimer’s disease (AD) and Huntington’s disease (HD), are diseases characterized by the progressive loss of neurons [1]. They are becoming the primary focus within the scientific community given their severe health threats that cause significant disabilities in the ageing population and a burden on families, healthcare, and society in managing patients [2, 3]. Approximately 36–47 million people suffer from dementia, a common symptom of neurodegenerative diseases, and this number is expected to multiply to 66 million and 115 million people by 2030 and 2050, respectively, as a result of the aging global population [4–6]. Specifically, AD is characterized by memory loss due to the severe loss of nucleus basalis cholinergic neurons, typically seen in advanced AD patients. In addition, the accumulation of amyloid-β (Aβ) and phosphorylated tau proteins leads to the deposition of senile plaques and the formation of neurofibrillary tangles (NFTs), respectively, contributing to prototypical lesions [3]. Due to its widespread distribution, AD impairs many essential physiological and cognitive functions, ranging from attention and memory to psychiatric symptoms, such as apathy and depression [7]. AD is the 5th leading cause of death, which claimed 1.55 million lives in 2019, with 1.02 and 0.54 million deaths reported in women and men, respectively [8, 9].
The Streptomyces genus is a major producer of pharmaceutically valuable secondary metabolites with diverse bioactivities, including antibiotics and anti-cancer agents [10]. In recent years, Streptomyces have gained increasing interest as many of its compounds exhibit neuroprotective activities in various neurodegenerative diseases, including multiple sclerosis and Parkinson’s disease [11]. Considering the promising features of Streptomyces-derived compounds, this study performed a scoping review on secondary metabolite compounds or extracts from Streptomyces sp. with regulatory effects on AD-related proteins in the last decade. This approach would determine the interactions between Streptomyces compounds and prospective AD-related target proteins, potentially providing an alternative treatment against AD.
METHODS
The Preferred Reporting Items for Systematic Reviews and Meta-analyses (PRISMA) checklist was used as a guideline for this scoping review [12].
Literature search strategy
A collection of articles on Streptomyces sp. secondary metabolites related to neuroprotection in AD was obtained by conducting an extensive literature search using different keywords and subject headings. Five electronic databases (PubMed, Ovid Medline, ScienceDirect, Embase, and Scopus) were used to search for relevant original research articles published within the last 10 years (January 2010– December 2021). The search terms included “Alzheimer’s disease”, “Alzheimer’s”, “Streptomyces”, “Streptomyces sp.”, “Actinomycete”, “Neuroprotection”, “Neuroprotective”, “Proteins”, “Genes”, “Secondary metabolites”, and “metabolites”. The terms were searched in titles, abstracts, and Medical Subject Headings (MeSH) keywords using Boolean operators for defined search results.
Study eligibility and selection
The eligibility of the compiled articles was determined by screening the title and abstract using a set of inclusion and exclusion criteria (Table 1). The shortlisted research articles were then subjected to full-text review, and only research articles that fulfilled the inclusion criteria were selected for data extraction.
Table 1
Inclusion and exclusion criteria for selecting study
Inclusion Criteria | Exclusion Criteria |
Publication Period (2010–2021) | Publication before 2010 |
English language studies | Non-English studies |
Full-text available | Duplicate articles |
Original research articles | Review articles |
Streptomyces metabolites in Alzheimer’s disease | Irrelevant studies to Streptomyces metabolites in Alzheimer’s disease |
In vitro and in vivo studies | Clinical trials |
Papers on neurodegenerative disease and dementia | Meta-analysis, systematic reviews, mini-reviews |
Screening and data extraction
A preliminary literature search was carried out using specific search terms. After removing duplicate articles, the articles were imported into Covidence (https://www.covidence.org/). Then, the articles were subjected to title and abstract review. Any papers aside from original research articles or those unrelated to AD studies were also removed. Subsequently, the shortlisted articles were subjected to full-text review based on this study’s inclusion and exclusion criteria (Table 1). The review and selection process were assessed by two independent reviewers. If any disagreements or conflicts arose, a third independent reviewer would assist in resolving the decision-making. The selection and exclusion processes are outlined in the PRISMA 2020 flow chart (Fig. 1).
Fig. 1
PRISMA flow chart (2020) PRISMA, Preferred Reported Items for Systematic Review and Meta-analysis [12] outlining the step-by-step process involved in the selection of studies included for this scoping review. *n, number.
![PRISMA flow chart (2020) PRISMA, Preferred Reported Items for Systematic Review and Meta-analysis [12] outlining the step-by-step process involved in the selection of studies included for this scoping review. *n, number.](https://content.iospress.com:443/media/adr/2023/7-1/adr-7-1-adr230065/adr-7-adr230065-g001.jpg)
Bioinformatics analysis
Three different bioinformatics tools were employed to investigate and analyze the potential AD-related proteins: 1) Search Tool for the Retrieval of Interacting Genes/Proteins (STRING) data (http://string-db.org) (version 11.5), 2) PANTHER Classification System (PANTHER17.0) (http://www.pantherdb.org), and 3) Kyoto Encyclopedia of Genes and Genomes (KEGG) Mapper.
RESULTS
Literature search and selection
Using several search terms (Streptomyces AND Neuroprotection AND Alzheimer’s disease AND metabolites), 256 articles were first retrieved from the five databases. After 46 duplicates were removed, the inclusion and exclusion criteria were used to screen the remaining articles, producing 135 full-text articles. These articles underwent further independent screening by two reviewers, of which 83 articles were deemed credible for further screening based on the AD model. Finally, 12 articles were shortlisted for detailed data extraction (Fig. 1).
Characteristics of included studies
The scoping review focused on past studies pertaining to neuroprotection (n = 83) based on initial assessment studies (Fig. 1). Approximately 14.5% of the research articles (12 out of 83 studies) were selected based on the AD model. In addition, data were extensively extracted from these selected studies, primarily focusing on protein regulation by Streptomyces compounds (Table 2). The summary of the compiled studies showed that cumulatively, the highest number of articles on Streptomyces compounds in AD was published in Asia (70%), while the remaining 30% were published equally from Europe, South America, and North America (10% each) (Fig. 2A). Spain has the highest number of published articles with 25% (3 out of 12 research articles), followed by China, India, and South Korea (16.7% each), and the United States of America, Iran, and Chile (8.3% each) (Fig. 2B).
Table 2
Summary of findings extracted from the selected Streptomyces-treated studies (n = 12) in Alzheimer’s disease model. It includes Streptomyces species, secondary compounds, and its effect on the AD-related protein regulations
Streptomyces Species | Country | Secondary compounds | Regulation | Protein | Reference |
Streptomyces sp. CA-237351 | Spain | Streptocyclinones A and B | ↑ | Nrf-2 | [13] |
↓ | iNOS, p65, ERK 1/2, pJNK and p38, IL-1β, IL-10, TNF-α, tau protein, BACE1 | ||||
↔ | GSK3β &IL-6 | ||||
Streptomyces hygroscopicus | China | Rapamycin | ↑ | Aβ degradation enzyme (IDE), neuronal nuclei (NeuN) antigen, autolysosomes, LC3-II/I. Beclin-1, Beta-catenin, Wnt3a, pGSK-3B | [14] |
↓ | AβPP, BACE1, PS1, t-Tau, PHF-1, p62, GSK3β,Aβ | ||||
Streptomyces sp. WON17 | South Korea | Rhizolutin | ↑ | GFAP, BCL-2 | [15] |
↓ | Caspase-3, β-sheet-rich Aβ fibrils, IL-1β | ||||
Streptomyces sp. Lt 005 | Spain | Anhydroexfoliamycin | ↑ | GSK3β | [16] |
↓ | tau, JNK, BACE1 | ||||
↔ | ERK | ||||
Streptomyces caniferus | Spain | Caniferolide A | ↑ | Nrf-2, IL-10 | [17] |
↓ | p65, IL-1β, IL-6, TNF-α, iNOS, p38, JNK, tau, BACE1, ERK | ||||
Streptomyces strain HM4 | Iran | Chloroform Extract | ↓ | Aβ | [18] |
Streptomyces hygroscopicus | Chile | Rapamycin | ↑ | SV-2 | [19] |
Streptomyces sp. UTZ13 | South Korea | Bonnevillamides D and E | ↓ | Aβ | [20] |
Streptomyces hygroscopicus | India | Rapamycin | ↑ | p62, beclin-1, ATG-5, P13K, AKT, CREB,synapsin-I, SYP, PSD95, CHRM2, DAD2 receptor, NMDAR2,AMPAR2, AChE | [21] |
↓ | mTOR | ||||
Streptomyces scabrisporus. | India | Alborixin | ↑ | LC3B-II, beclin-1, ATG7, ATG5 and ATG12, PTEN | [22] |
↓ | SQSTM1, p-AKT p-MTOR and RPTOR | ||||
Streptomyces. qinglanensis 172205 | China | Enterocin | ↓ | Aβ | [23] |
Streptomyces hygroscopicus | USA | Rapamycin | ↑ | β-CTF | [24] |
↓ | sAβPPα, ADAM-10 | ||||
↔ | ADAM-17, BACE1 and PS1, AβPP |
(↑) Upregulated, (↓) Downregulated, (↔) Inconsistent regulation.
Fig. 2
Publication on Streptomyces compounds in Alzheimer’s disease models in the last decade. A) Articles published (%) on implications of Streptomyces compounds in Alzheimer’s disease model in the last 10 years (2010–2021) based on continents. B) Breakdown of published articles (%) by countries in the last decade.
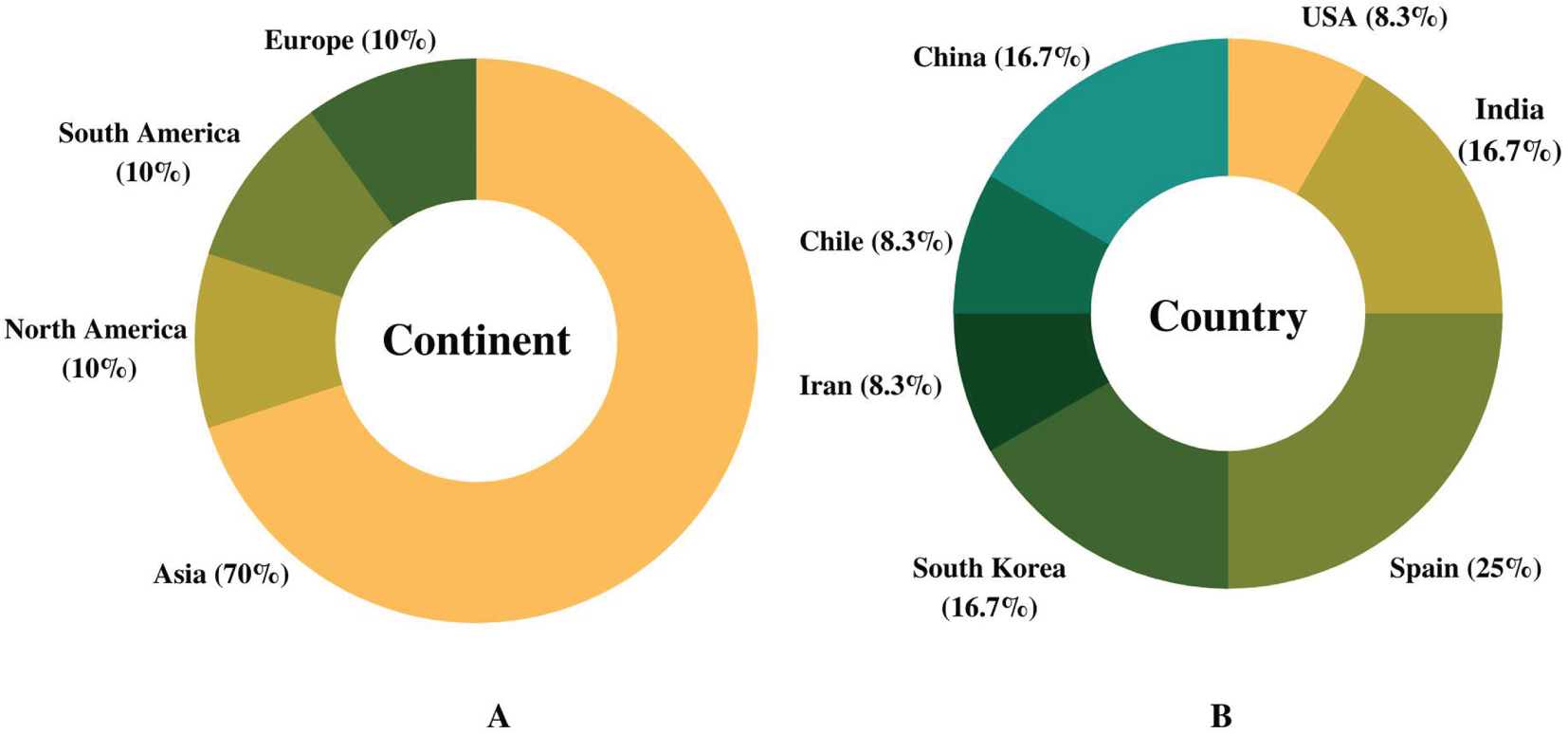
Replicability of proteins
From the 12 shortlisted articles, 53 proteins were extracted, and their replicability was assessed. Only 38% of the proteins (20 out of 53) were reported in two or more research articles and chosen for further analysis (Fig. 3). Notably, 2 proteins (Aβ and BACE1) were reported in 5 articles, while the tau protein was described in 4 articles. Furthermore, 5 proteins (ERK, JNK, IL-1β, BECN1, and GSK3β) were mentioned in 3 articles. Another 2 articles described 13 proteins, and the remaining proteins were stated in a single article (Fig. 4).
Fig. 3
The summary of selected research articles and identified proteins. A) 14.5 % of the total articles (12 out of 83 articles) were selected based on the Alzheimer’s disease model. B) From the 12 independent research articles around 53 proteins were identified to play a role in Alzheimer’s disease. C) Only 38% of total proteins (20 out of 53 proteins) were shortlisted as they were present in more than two research articles. The list of shortlisted proteins used for further STRING, PANTHER, and KEGG analysis.
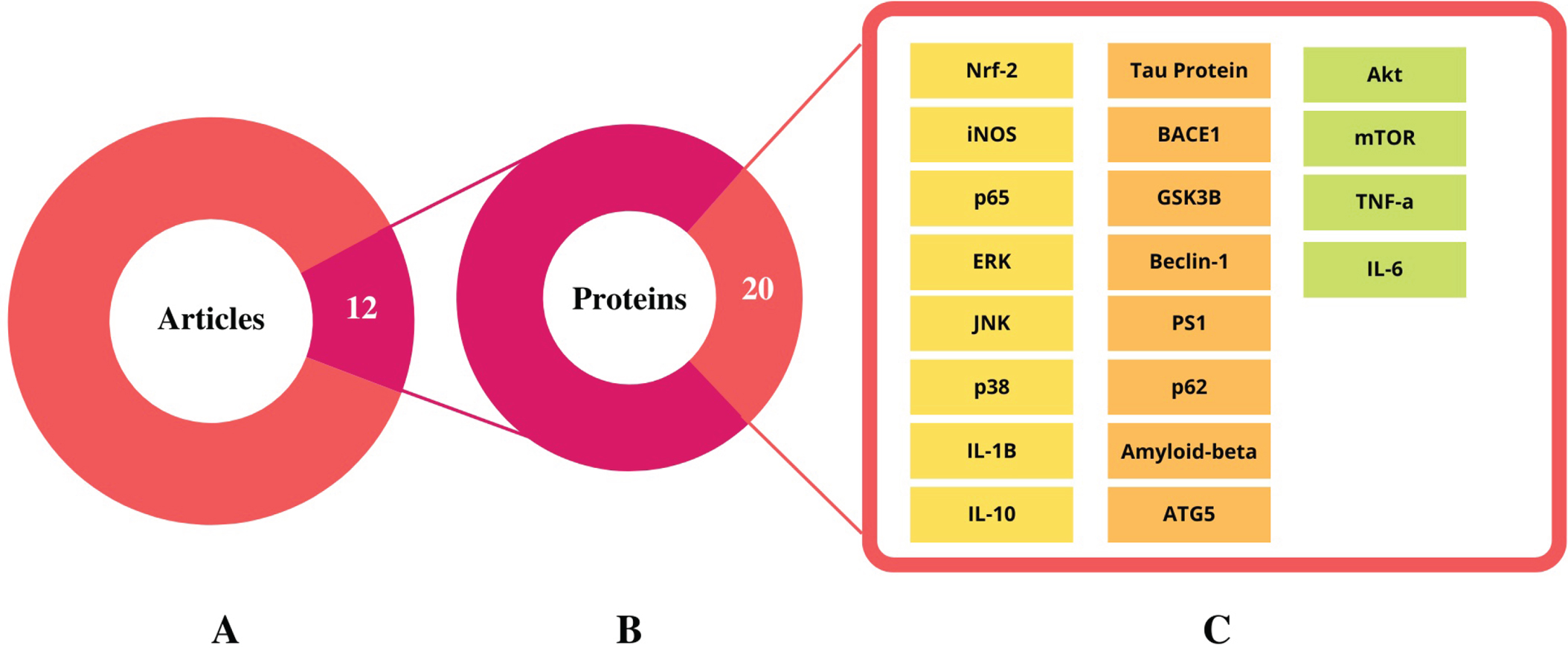
Fig. 4
Replicability of proteins identified across 12 independent Streptomyces-treated studies.(Created in BioRender.com).
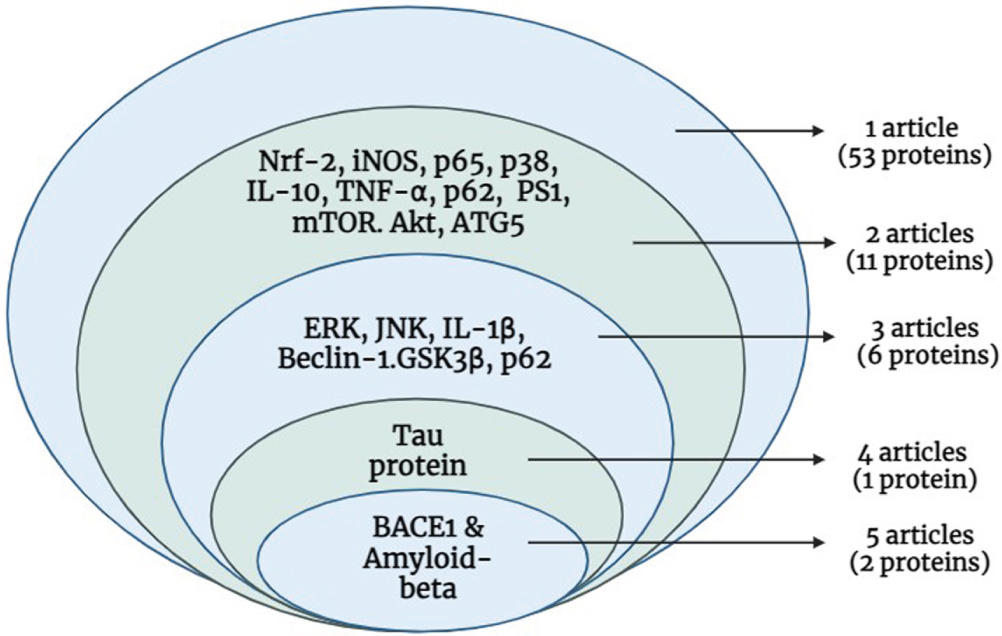
PPI network analysis using STRING
The Protein-Protein Interaction (PPI) network was constructed by uploading the 20 shortlisted proteins to the STRING database (https://string-db.org) to evaluate their differential expression and alteration of protein interactions. The PPI network successfully mapped 20 nodes (interactions) with 63 edges and 14 expected edges, with the PPI enrichment p-value (p < 1.0 e-16) analyzed at high confidence (0.700) minimum required interaction score. In addition, three distinctive protein clusters involved in the Aβ and tau metabolism were generated using the K-means clustering, which were; GSK3β (GSK3B), PS1 (PSEN1), ERK (MAPK1), JNK (MAPK8), BACE1 (BACE1), Aβ(APBB1) and tau (MAPT), neuroinflammation; iNOS(NOS2), IL-6 (IL6), IL-1β (IL1β), p62 (SQSTM1), p65 (RELA), TNF-α (TNF), IL-10 (IL10), p38 (MAPK14); and autophagy: mTOR (MTOR), Beclin-1 (BECN1), ATG5 (ATG5), AKT (AKT1), and Nrf-2 (NFE2L2), as shown in Fig. 5.
Fig. 5
The STRING plot depicts the functional interrelationships of proteins analysed using the STRING 11.5 database. It shows 20 nodes (interactions) and 63 edges represent the protein and protein-protein interaction with an enrichment p-value (p < 1.0e–16). Three distinctive clusters were generated using K-means clustering shown in blue (MTOR, BECN1, ATG5, AKT1, NFE2L2), red (NOS2, IL6, IL1β, SQSTM1, RELA, TNF, IL10, MAPK14) and green (GSK3B, PSEN1, MAPK1, MAPK8, BACE1, MAPT, APBB1) clusters involved autophagy, neuroinflammation and beta-amyloid and tau metabolism respectively. *The genes of proteins are italicized.
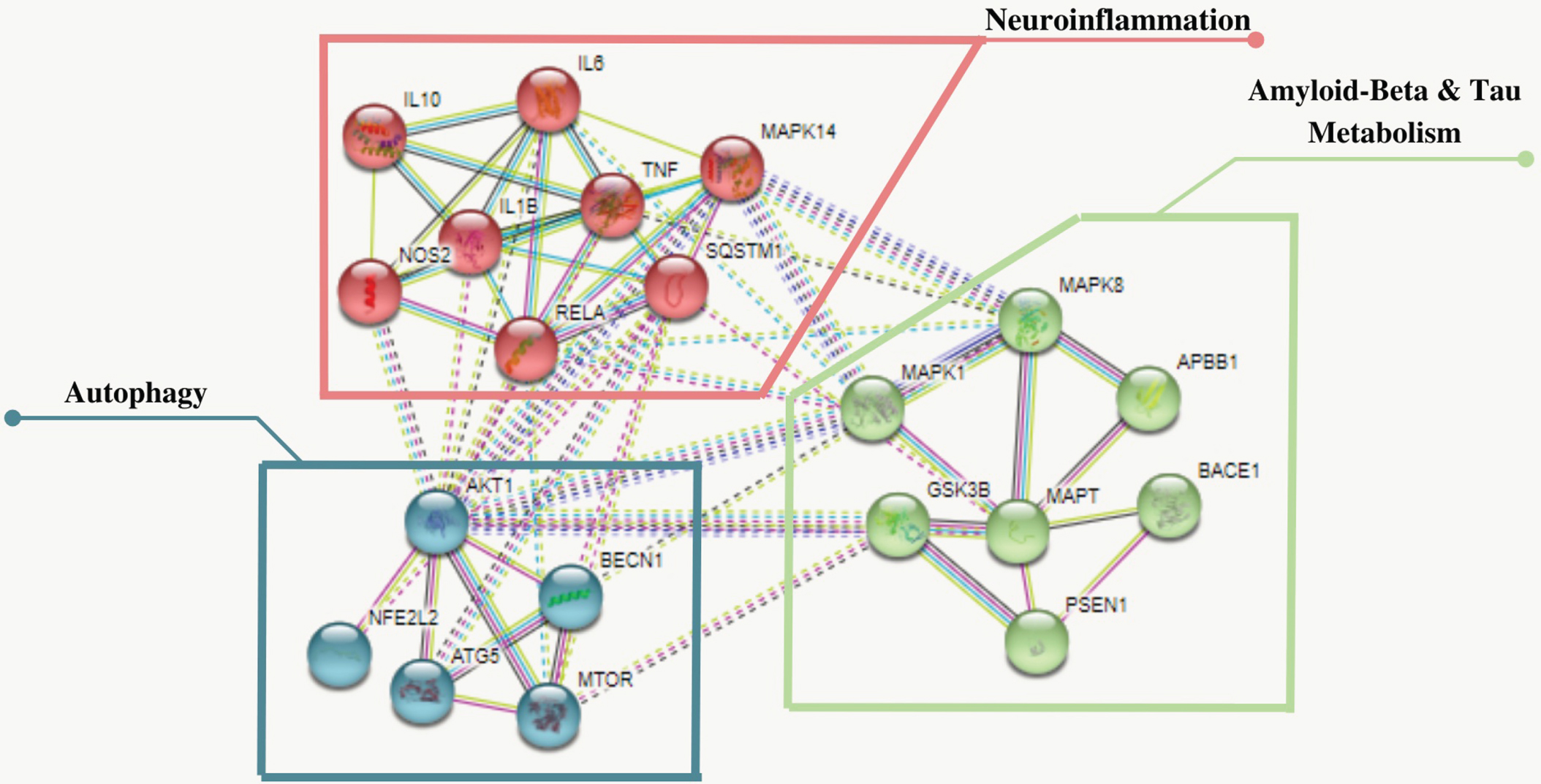
Functional annotation analysis via PANTHER
The 20 shortlisted proteins represented as genes were classified using PANTHER GO-Slim based on the gene ontology domain: PANTHER pathway. Accordingly, nearly 30% of the genes (6 out of 20) were present in the AD-amyloid secretase pathway consisting of MAPK1, PS1, APBB1, MAPK14, BACE1, and MAPK8. On the contrary, 20% of the genes (4 out of 20) comprise the AD-presenilin pathway, including PS1, APBB1, BACE1, and GSK3B. Interestingly, both pathways show three common genes; PS1, APBB1, and BACE1. Figure 6 shows the pathway classification of the 20 proteins in the AD-amyloid secretase pathway (P00003) and AD-presenilin pathway (P00004).
Fig. 6
PANTHER analysis of shortlisted proteins from 12 independent Streptomyces-treated studies. The classification of proteins present in more than two research articles were analysed using PANTHER gene ontology of 20 selected proteins based on PANTHER Pathway; Alzheimer’s disease-amyloid secretase pathway (P00003) and Alzheimer’s disease-presenilin pathway (P00004). The Venn diagram shows overlapping between two pathways producing three (3) specific proteins; PSEN1 (PSEN1), amyloid-β(APBB1) and BACE1(BACE1). (Created in BioRender.com).
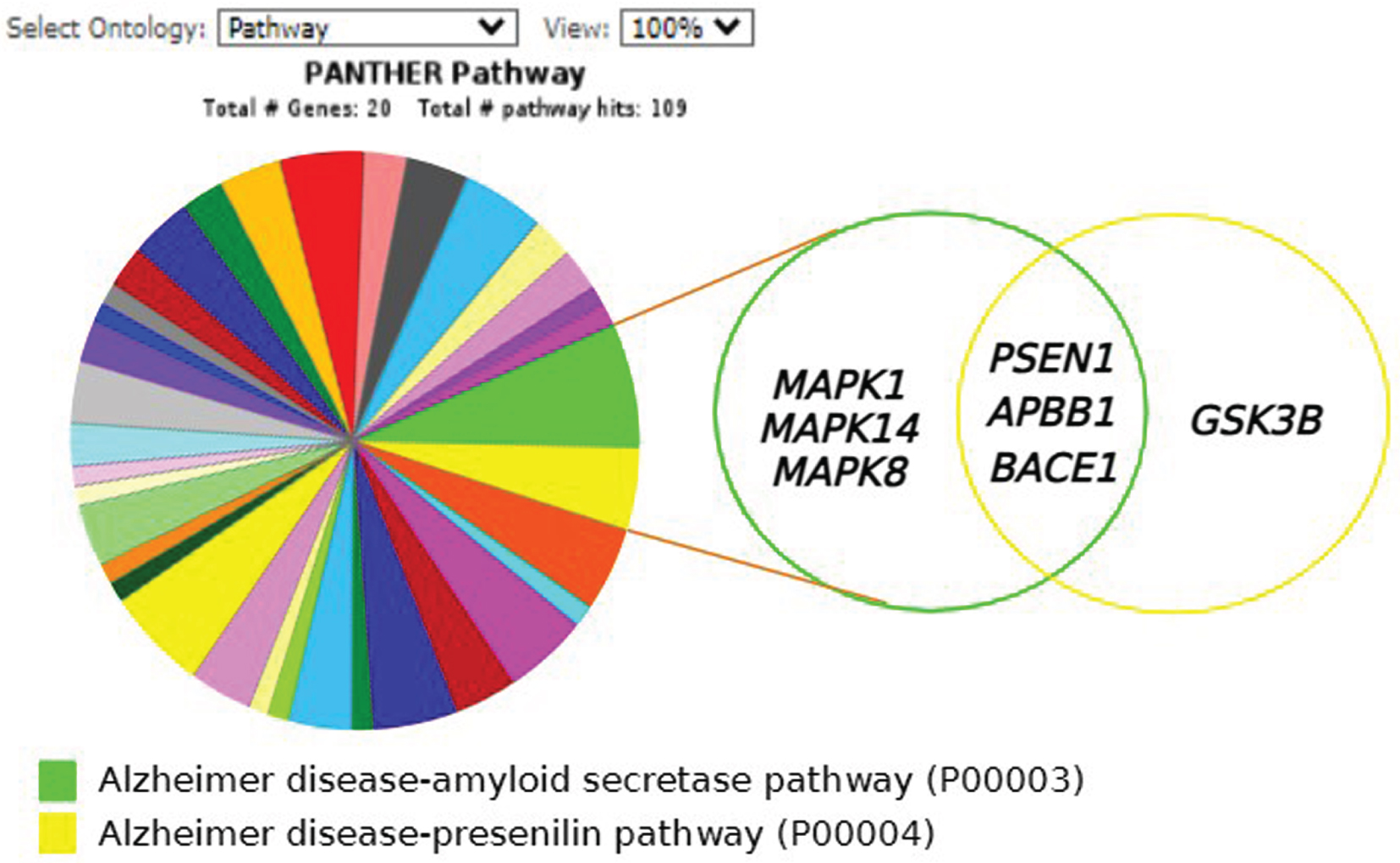
KEGG mapper
The functional role of these 20 shortlisted proteins in AD pathogenesis was assessed using the KEGG pathway. Around 75% of the total proteins (15 out of 20) were successfully mapped in the AD pathway map (hsa05010) in Homo sapiens, as highlighted in green (Fig. 7) indicating these proteins are involved directly in the pathogenesis of AD. A total of 15 proteins, which consisted of AKT (AKT1), BACE1 (BACE1), mTOR (MTOR), GSK3β (GSK3B), Aβ (APBB1), IL-6 (IL6), IL-1β (IL1β), tau (MAPT), iNOS (NOS2), ERK (MAPK1), JNK (MAPK8), PS1 (PSEN1), p65 (RELA), TNF-α (TNF), and Beclin-1 (BECN1), were involved in multiple pathways, including axonal transport defects, impaired autophagy, impaired neuronal insulin signaling, long-term reduction in potentiation, microglial inflammation, Aβ and tau processing, apoptosis, and dysfunctional mitochondria. Noteworthy, 25% of the total proteins (5 out of 20) namely Nrf-2 (NFE2L2), ATG5 (ATG5), IL-10 (IL10), p38 (MAPK14), and p62 (SQSTM1) may play an in-direct role in AD pathogenesis.
Fig. 7
Only 15 proteins (75%) consisted of AKT (AKT1), BACE1 (BACE1), mTOR (MTOR), GSK3β (GSK3B), amyloid-β (APBB1), IL-6 (IL6) IL-1β (IL1β), tau (MAPT), iNOS (NOS2), ERK (MAPK1), JNK (MAPK8), PS1 (PSEN1), p65 (RELA), TNF-α (TNF), and beclin-1 (BECN1) were successfully mapped highlighted in green in the Kyoto Encyclopaedia of Genes and Genomes (KEGG) pathway of Alzheimer’s disease in Homo sapiens (hsa05010). *The genes of respective proteins are italicized. Published with permission from Kanehisa Laboratories.
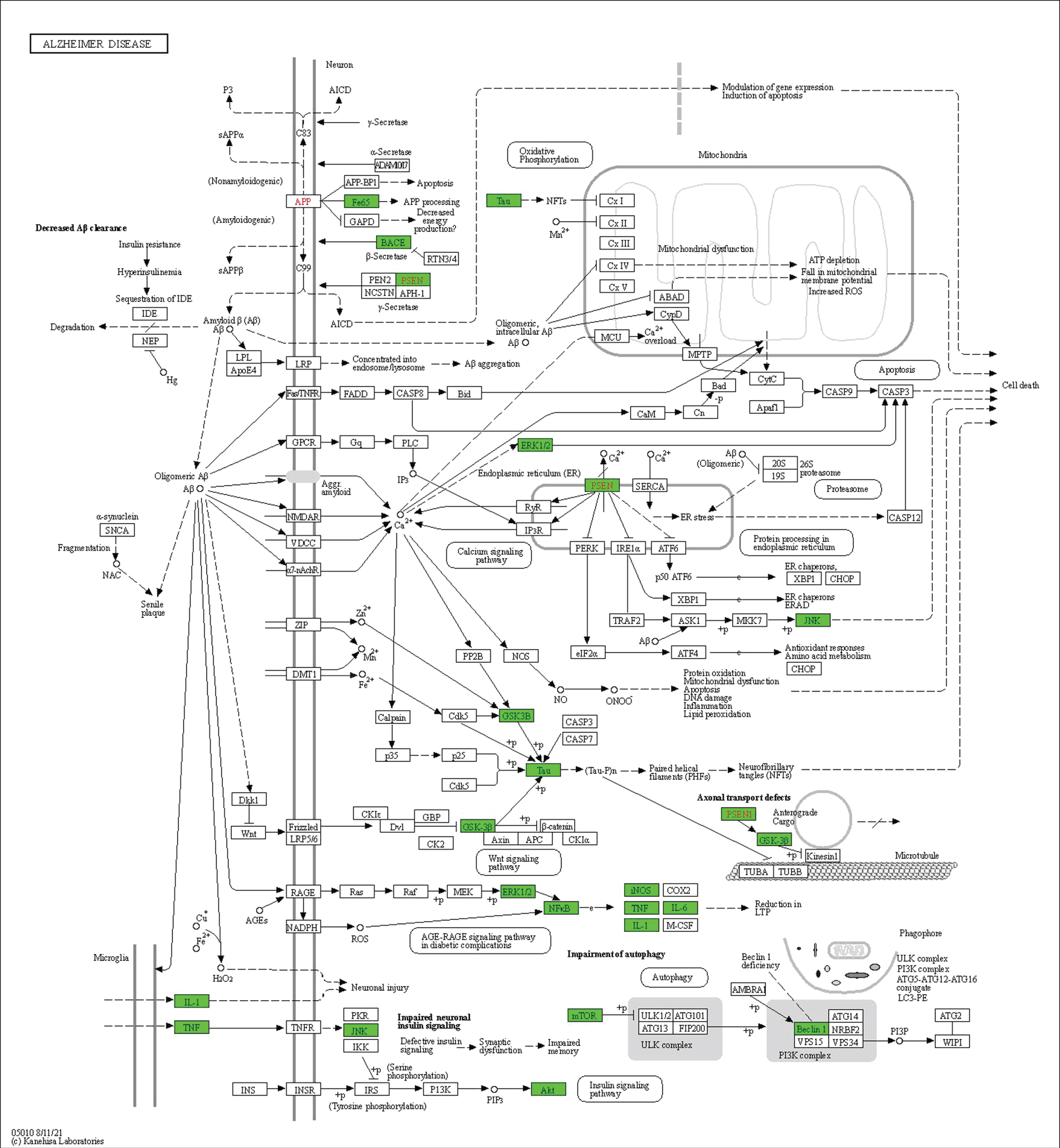
Differentially expressed AD-related proteins
In AD pathogenesis, 70% of the proteins (14 out of 20) are upregulated, while the remaining 30% (6 out of 20) are downregulated. In comparison, it also shows that 35% of the proteins (7 out of 20) were inconsistently regulated when treated with Streptomyces sp. compounds or extract (Table 3). The remaining 45% (9 out of 20) proteins were consistently downregulated while the remaining 20% (4 out of 20) of the proteins were consistently upregulated.
Table 3
The summary of proteins regulations in Alzheimer’s disease compared to Streptomyces studies based on their respective clusters
Pathway (Cluster) | Proteins | Regulation | References | |
Alzheimer’s disease | Streptomyces studies | |||
Autophagy (Blue) | mTOR | ↑ | ↓ | [21, 22, 25] |
Beclin-1 | ↓ | ↑ | [14, 21, 22, 26, 27] | |
ATG5 | ↓ | ↑ | [21, 22, 28] | |
Akt | ↓ | ↑ | [21, 22, 29] | |
Nrf-2 | ↓ | ↑ | [13, 17, 30, 31] | |
Neuroinflammation (Red) | IL-10 | ↓ | ↔ | [13, 17, 32, 33] |
IL-1β | ↑ | ↓ | [15, 17, 34, 35] | |
p62 | ↓ | ↔ | [14, 21, 36] | |
iNOS | ↑ | ↓ | [13, 17, 37] | |
p65 | ↑ | ↓ | [13, 17, 38] | |
TNF-α | ↑ | ↓ | [13, 17, 39] | |
p38 | ↑ | ↓ | [13, 17, 40] | |
IL-6 | ↑ | ↔ | [13, 17, 41] | |
Aβ &tau metabolisms (Green) | GSK3β | ↑ | ↔ | [13, 16, 42] |
PS1 | ↑ | ↔ | [14, 24, 43] | |
ERK | ↑ | ↔ | [13, 17, 44] | |
JNK | ↑ | ↓ | [13, 16, 17, 45] | |
Aβ | ↑ | ↓ | [15, 18, 20, 23, 46] | |
BACE1 | ↑ | ↔ | [13, 14, 16, 17, 47] | |
Tau | ↑ | ↓ | [13, 14, 16, 48] |
(↑) Upregulated, (↓) Downregulated, (↔) Inconsistent regulation.
DISCUSSION
Aβ immunotherapies are designed to target Aβ clearance through either active immunization (vaccines) or passive immunization (monoclonal antibodies). With the latter being favorable for its effectiveness and suitability in elderly population, and convenient termination upon patient’s reports of adverse side effects [49]. However, clinical data were unsatisfactory causing failed drugs including solanezumab, bapineuzumab, and crenezumab showing little-to-no clinical effects [50]. Despite these setbacks, four new monoclonal IgG1 antibody drugs were developed namely donanemab (LY3002813), gantenerumab (RO4909832), aducanumab (BIIB037), and lecanemab (BAN2401) demonstrating promising clinical results leading to gantenerumab (RO4909832) being FDA-granted as breakthrough therapy while the latter two received FDA-approval for Aβ immunotherapies [50, 51]. Despite significant improvement on cognition, these immunotherapies may not have clinical significance due to its effect size [52]. Aβ immunotherapies are shrouded with safety concerns mainly amyloid-related imaging abnormalities (ARIA) including ARIA-edema (ARIA-E) and ARIA-hemosiderosis (ARIA-H) [53]. These drugs have been observed to cause ARIA-E with following frequencies; lecanemab (10%), donanemab (27%), gantenerumab (30%) and aducanumab (35%) [50]. It also requires larger expenditure of $120 billion USD per year [52]. Therefore, it is worth exploring other options as affordable prospective treatments for AD with lesser side effects.
Actinobacteria are prolific producers of natural products, with Streptomyces being the majority genus. Secondary metabolites isolated from Streptomyces species possess various pharmacological properties, including anti-cancer, anti-inflammatory, antimicrobial, and neuroprotective, which are effective against numerous elements, ranging from oxidative stress to neuroinflammation [13]. For instance, flaviogeranin and indanostatin isolated from Streptomyces sp. RAC226 and RAI20, respectively, demonstrated neuroprotective properties [54, 55]. Therefore, this scoping review examined the effects of Streptomyces sp. compounds or extracts on regulating various AD-related proteins and identifying prospective target proteins for AD treatment.
Based on the 12 independent studies selected in this review, 10 compounds (Fig. 8), including rapamycin, alborixin, enterocin, bonnevillamides D and E, caniferolide A, anhydroexfoliamycin, rhizolutin, streptocyclinone A and B, and a chloroform extract (Table 2), were identified to exhibit considerable regulatory effects against 53 proteins. However, only 20 proteins were shortlisted, comprising 3 distinctive clusters from the STRING analysis: autophagy, neuroinflammation, and amyloid-beta and tau metabolism (Fig. 5). The proteins involved in these pathways were either up- or downregulated in AD pathogenesis. Conversely, Streptomyces-treated studies showed that these compounds have opposite effects on the protein regulations, while several proteins, such as IL-6, BACE1, ERK, GSK3β, PS1, p62, and IL-10, were inconsistently regulated (Fig. 9).
Fig. 8
The chemical structures of Streptomyces sp. compounds with neuroprotective potential in Alzheimer’s disease model.
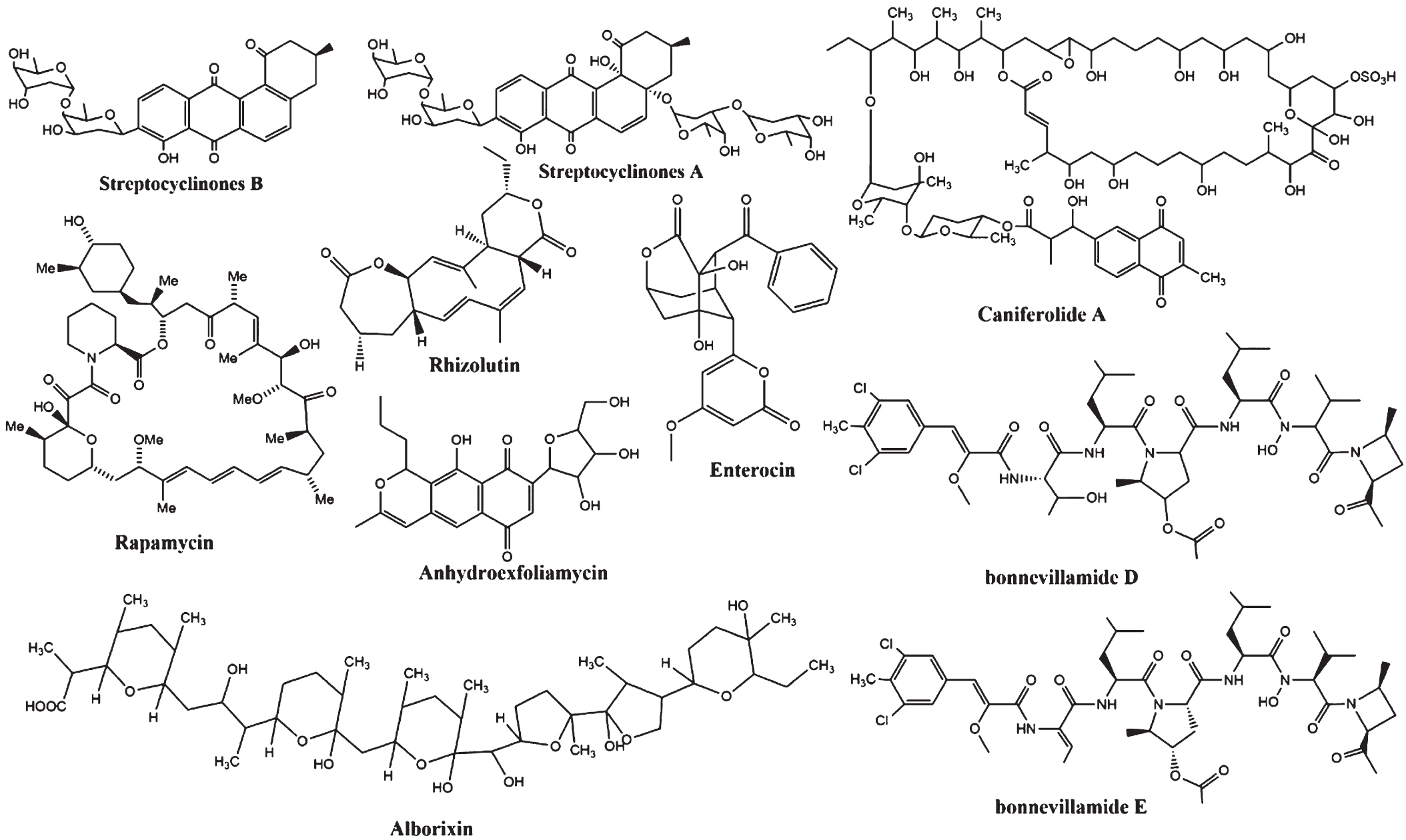
Fig. 9
Protein expression of profiles of 20 proteins obtained from 12 independent Streptomyces-treated studies. A) Shows the protein regulations in Alzheimer’s disease pathogenesis. B) The protein regulations in Streptomyces-treated studies. (Created in BioRender.com).
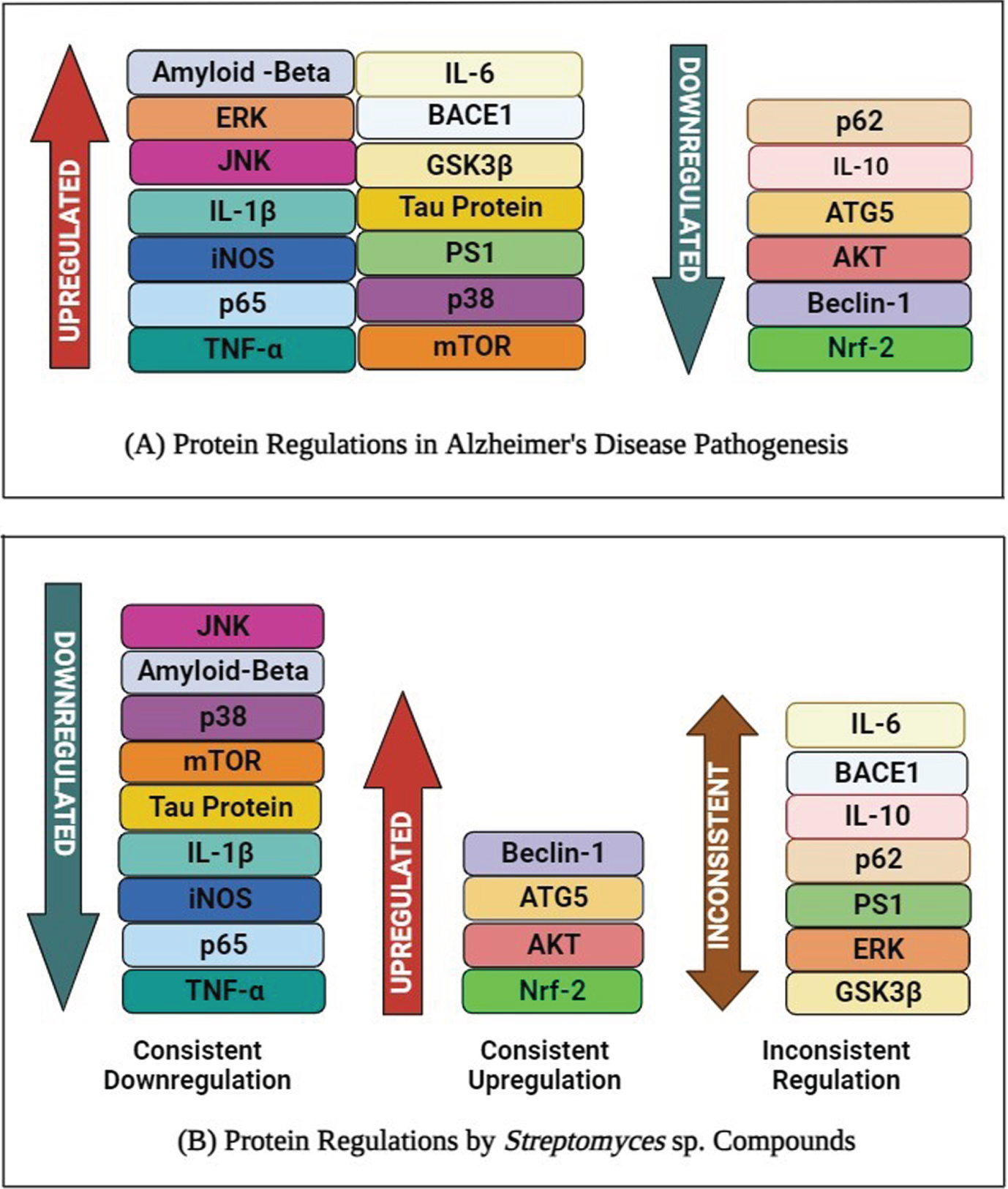
Amyloidosis in Alzheimer’s disease
Five articles showed that Aβ was consistently downregulated when treated with Streptomycessp. compounds, including rhizolutin, rapamycin, enterocin, bonnevillamides D and E, and chloroform extract. In contrast, their expressions were upregulated in AD pathogenesis [14, 15, 18, 20, 23]. In general, Aβ is released from the amyloid-β protein precursor (AβPP) by β-secretase (BACE1) and γ-secretase, producing neurotoxic Aβ fragments. In the first rate-limiting step, the N-terminus of the Aβ is cleaved by β-secretase, followed by γ-secretase cleaving the C-terminus of Aβ. The resulting formation of Aβ oligomers is then polymerized into aggregated Aβ before being converted into plaques. This leads to kinase activation, such as GSK3β, ERK2, and CDK5, leading to the hyperphosphorylation of microtubule-associated tau proteins, which are polymerized into insoluble NFTs. Subsequently, these proteins induce microglia recruitment, triggering a local inflammatory response that causes neurotoxicity and neuronal death, giving rise to AD [56]. Hence, targeting the earlier stages by inhibiting the activity of BACE1 before the formation of Aβ oligomers may provide a novel treatment against AD.
Although BACE1 is an ideal target candidate for treatment as it initiates the amyloidogenic pathway, however, past studies reported that BACE1 was inconsistently regulated [13, 14, 16, 17, 24]. When treated with a well-known and established Streptomyces hygroscopicus-derived compound known as rapamycin, showed that BACE1 expression was insignificant in murine neuroblastoma cells overexpressing the “Swedish” mutant APP6965aa isoform (SweAPP N2a), as reflected by the increased amyloid production. This was also corroborated by the enhanced β-Carboxyl-Terminal Fragment (β-CTF) level of AβPP and the decreased soluble AβPP alpha (sAβPPα), a neuroprotective cleavage product. The findings suggest that the α-secretase activity was inhibited by rapamycin, like the inhibition of α-secretase disintegrin and metallopeptidase domain-10 (ADAM-10) [24]. The suppressed ADAM-10 pathway initiates the amyloidogenic pathways typically reported in AD [57]. However, Chen et al. (2019) refuted the suggestion with a contradicting finding, revealing that the Aβ production in AβPP/PSEN1 transgenic mice was reduced following treatment with rapamycin, which downregulated the expression of AβPP-cleaving enzymes, including BACE1 and PS1.
It should be noted that rapamycin is an established mTOR inhibiting drug, although its downregulation reduces Aβ42, Aβ plaques, and NFT levels and improves AD-like cognitive deficits. It also serves a multifaceted role in normal cellular functions, such as gene translation and cellular growth. However, it was agreed that prolonged intake of rapamycin leads to severe side effects due to the inhibition of the mTOR pathway [28]. Noteworthily, two clinical trials (NCT04200911 and NCT04629495) are being conducted to investigate the long-term use of rapamycin in older adults with MCI and early-stage AD. Although NCT04200911 has reported adverse side effects including altered mental status and headache in participants, however, both studies are still in its preliminary stages and incomplete, as neither could provide supporting evidence on the feasibility of rapamycin as long-term usage in treating AD thus far [58, 59]. Therefore, rapamycin may not be an ideal Streptomyces sp. compound for long-term AD treatment.
The contradicting BACE1 regulation from the two studies supports the notion that rapamycin is unsuitable for AD treatment. Therefore, other Streptomyces sp. compounds with prospective targets against BACE1 should be identified and considered. Examples of Streptomyces sp. compounds that were shown to downregulate BACE1 expression include caniferolide A, anhydroexfoliamycin, and streptocyclinone B isolated from Streptomyces caniferus, Streptomyces sp. Lt 005, and Streptomyces sp. CA-237351, respectively [13, 16, 17]. Therefore, Aβ and BACE1 remain the prospective target proteins to inhibit the amyloidogenic pathway in AD.
Autophagic improvement
The accumulation of autophagosomes containing amyloid-beta and tau proteins contributes to the intracellular build-up of toxic peptides in dystrophic neurites, leading to AD progression [60–62]. Previously, five proteins, consisting of ATG5, AKT, Beclin-1, Nrf-2, and mTOR, were identified to play a role in autophagy through downregulation in AD, except for mTOR. Interestingly, all proteins showed counteracting results when treated with different Streptomyces sp. compounds (Fig. 9), indicating that these proteins are potential targets. Given this, targeting these proteins involved in autophagy is advantageous as an alternative treatment approach.
BECN1 is involved in autophagy by promoting the removal of toxic peptides and preventing neuronal death by maintaining basal autophagy and protein turnover. Additionally, BECN1 plays a crucial role in several membrane transports responsible for autophagy, phagocytosis, and endocytosis [36]. The reduced BECN1 gene and protein expression in AD patients’ brain tissue results in autophagy defects, similar to the abnormal accumulation of subcellular vesicles, producing toxic AβPP metabolites. Meanwhile, BECN1 deletion in human APP (hAPP)-transgenic mice in vivo model increased Aβ plaque deposition, neuronal loss, and the accumulation of AβPP and its metabolites, particularly AβPP C-terminal fragment peptides [27]. On the contrary, mRNA and BECN1 protein expressions were upregulated in mice brains with AD when treated with rapamycin [14, 21], although the latter reported an insignificant Beclin-1 protein expression. Additionally, 24-hour treatment with alborixin, a known Streptomyces scabrisporus compound, enhanced the BECN1 expression in both N9 cells and primary neuronal cells (C57BL/6) [22].
On top of that, ATG5 plays a significant role in autophagy, as its downregulation leads to either partial or total autophagy inhibition [63]. The knockdown effect of the ATG5 gene in AD patients results in the accumulation of ubiquitinated proteins, triggering neuronal loss [26]. When microglial N9 cells were treated with alborixin, the ATG5 expression was upregulated, as reflected by the enhanced autophagic activities in the lysosomal removal of Aβ1–42. Such an occasion was due to the PTEN suppression of the P13K-AKT pathway [22]. In another study, rapamycin treatment in an in vivo Wistar rats model increased the ATG5 mRNA expression in the hippocampal region when treated with Aβ1–42 via autophagic induction [21].
Mitigating neuroinflammation
The accumulation of Aβ and tau proteins caused by autophagic dysregulation activities stimulates microglial cells to remove these proteins. However, the removal process is impaired as the system becomes overwhelmed, leading to prolonged activation and enhanced inflammatory response [41]. Various pro-inflammatory cytokines, including TNF-α, IL-6, IL-1β, and small-molecule messengers, such as nitric oxide further damage the brain cells as they become more susceptible to oxidative stress-mediated cell death [41, 64]. For instance, Nrf-2 was shown to play a dual role in the fine-tuning feedback loop resulting from an elevated level of oxidative stress in combination with the autophagic process through the AMP-activated protein kinase upregulation and mTOR downregulation [65]. Nrf-2 also activates numerous antioxidative enzymes, including catalase, superoxide dismutase, and glutathione peroxide, to mitigate reactive oxygen species and protect from neuroinflammation [16]. Furthermore, Nrf-2 upregulation prevents the transcription of NFkB, which comprises p65, c-Rel, p50, RelB, and p52. Consequently, the nuclear expressions of p65/p50 are downregulated, which prevents the induction of pro-inflammatory genes, suppressing neuroinflammation [13].
Recently, caniferolide A and streptocyclinone A and B were isolated from Streptomyces caniferus and Streptomyces sp. CA-237351, respectively. Interestingly, the nuclear Nrf-2 levels in BV2 microglial cells were significantly enhanced when pre-treated with these compounds. Comparatively, caniferolide A produced the highest effect on its expression at the lowest concentration of 0.001 μM, implying that it is a potent Nrf-2 activator compared to streptocyclinone A and B. The authors also identified that all three compounds downregulated the nuclear NFkB-p65 expression in BV2 microglial cells due to their relation with the NFkB in oxidative stress [13, 17]. This evidence supports the association between the upregulation of Nrf-2 and the suppression of the NFkB expression, subsequently mitigating inflammatory response.
The upregulation of Nrf-2 also provides sufficient neuroprotection against oxidative stresses through increasing antioxidant activities, as reflected by the increased glutathione (GSH) levels when treated using all three compounds. This indicates that these compounds could improve the GSH enzyme expressions, including glutathione peroxidase and glutathione transferase, in tackling oxidative stress. Furthermore, these compounds diminished the release of pro-inflammatory cytokines, including IL-1B, TNF-α, and IL-6. However, IL-6 downregulation was only detected when BV2 microglial cells were treated with caniferolide A. The iNOS expression was also downregulated, reducing the release of nitric oxide in the BV microglial cells [13, 17].
FUTURE DIRECTIONS AND LIMITATIONS
This scoping review sets out to identify the repertoire of research and extent of the knowledge on Streptomyces sp. compounds in AD in both in vitro and in vivo models. These findings provide compelling evidence on utilization of Streptomyces sp. compounds in treating AD as the compounds have demonstrated regulatory effects on the AD-related proteins whether it is involved direct-or-indirectly in the pathogenesis of AD. Although, these findings achieved its objective, it is limited by restricted focus on the primary outcome of the regulatory effect these compounds possess on AD-related proteins. However, a consideration must be taken as these compiled studies used different cell lines and animals for their in vitro and in vivo models respectively. Although commonly used for neurodegenerative disease research, these models have varying phenotypic and genotypic makeups, as well as constraint in their ability in accurately represent the complex physiological, morphological, and functions of the brain when investigating AD pathophysiology. Therefore, it may contribute to the varying effect of regulatory expression of these proteins when treated with Streptomyces sp. compounds seen by the contradicting results when treated with similar compounds in between models. Hence, better models should be considered including 3D models and pathological-specific animal models for preliminary studies for future Streptomyces sp. Compounds [66–68].
The exclusion of clinical trials was due to limited available information on Streptomyces sp. compounds in AD. Hence, clinical trial data are absence in this analysis, although it is not primary focus of this, it provides a future direction on unexplored territories. This highlights the infancy of the area of research due limited clinical studies of Streptomyces sp. compounds in treating AD with the exception of rapamycin. Therefore, it is worth expediting the pre-clinical investigations in supporting the suitability and effectiveness of these compounds as drug-lead candidates prior to clinical trials [69]. This may provide a holistic and comprehensive information in justifying the prospect of Streptomyces sp. compounds as disease-modifying agents in treating AD.
CONCLUSION
The scoping review in this study identified 20 prospective target proteins consist of Aβ, BACE1, JNK, ERK, PS1, GSK3β, IL-6, p38, TNF-α, p65, iNOS, p62, IL-1β, IL-10, Nrf-1, Akt, ATG5, BECN1, and mTOR to treat AD based on their regulatory behaviors when treated with ten Streptomyces sp. compounds. These target proteins are responsible for various pathways, including neuroinflammation, autophagy, Aβ, and tau metabolism. BACE1 and Aβ are involved in AD initiation, and their inhibition may prevent the early stage of AD progression. Similarly, specific proteins involved in autophagy, such as BECN1 and ATG5, are considered target proteins that can improve autophagic activities, such as lysosomal and phagocytotic, to remove toxic Aβ peptides in neuronal cells, subsequently preventing AD. Nrf-2 is also among the target proteins, as it plays a dual role in autophagy and anti-inflammatory mechanisms to treat AD. This protein minimizes neuroinflammation by upregulating the expression of anti-inflammatory proteins, such as GSH and IL-10, while downregulating pro-inflammatory cytokines, including TNF-α, IL-6, and IL-1B. Overall, it is recommended to carry out further research and identification of other Streptomyces sp. compounds with potential anti-AD properties to treat AD effectively.
ACKNOWLEDGMENTS
The authors have no acknowledgments to report.
FUNDING
The authors have no funding to report.
CONFLICT OF INTEREST
The authors have no conflict of interest to report.
DATA AVAILABILITY
The data supporting the findings of this study are available within the article.
REFERENCES
[1] | Dugger BN , Dickson DW ((2017) ) Pathology of neurodegenerative disease. Cold Spring Harb Perspect Biol 9: , a028035. |
[2] | Gilter AD , Dhillon P , Shorter J ((2017) ) neurodegenerative disease: Models, mechanisms and new hope. Dis Model Mech 10: , 499–502. |
[3] | Silva MVF , Loures CMG , Alves LCV , de Souza LC , Borges KBG , Carvalho MDG ((2019) ) Alzheimer’s disease: Risk factors and potentially protective measures. J Biomed Sci 26: , 33. |
[4] | Rashid U , Ansari FL ((2014) ) Chapter 2 – Challenges in designing therapeutic agents for treating alzheimer’s disease-from serendipity to rationality. Drug Design and Discovery in Alzheimer’s Disease, Atta-ur-Rahman, Iqbal Choudhary M, eds. Elsevier, pp. 40–141. |
[5] | Arvanitakis Z , Shah RC , Bennett DA ((2019) ) Diagnosis and management of dementia: Review. JAMA 322: , 1589–1599. |
[6] | Goodson M , McLellan E Rosli R , Tan MP , Kamaruzzaman S , Robinson L , Moloney SA ((2021) ) A qualitative study on formal and informal carers’ perceptions of dementia care provision and management in Malaysia. Front Public Health 9: , 637484. |
[7] | Ferreira-Vieira TH , Guimaraes IM , Silva FR , Ribeiro FM ((2016) ) Alzheimer’s disease: Targeting the cholinergic system. Curr Neuropharmacol 14: , 101–115. |
[8] | GBD 2016 Dementia Collaborators ((2019) ) Global, regional, and national burden of Alzheimer’s disease and other dementias, 1990-2016: A systematic analysis for the Global Burden of Disease Study 2016. Lancet Neurol 18: , 88–106. |
[9] | Nichols E , Vos T ((2020) ) Estimating the global mortality from Alzheimer’s disease and other dementias: A new method and results from the global burden of disease study 2019. Alzheimers Dement 16: (Suppl 10), e042236. |
[10] | Sivalingam P , Hong K , Pote J , Prabakar K ((2019) ) Extreme environment: Potential sources for new antibacterial and anticancer drug leads? Int J Microbiol 2019: , 5283948. |
[11] | Law JFW , Letchumanan V , Tan LTH , Ser LH , Goh BH , Lee LH ((2020) ) Editorial: The rising “modern Actinobacteria” era. Prog Microbe Mol Biol 3: , a0000064. |
[12] | Page MJ , McKenzie JE , Bossuyt PM , Boutrin I , Hoffmann TC , Mulrow CD , Shamseer L , Tetzlaff JM , Akl EA , Brennan SE , Chou R , Glanville J , Grimshaw JM , Hróbjartsson A , Lalu MM , Li T , Loder EW , Mayo-Wilson E , McDonald S , McGuinness LA , Stewart LA , Thomas J , Tricco AC , Welch VA , Whiting P , Moher D ((2021) ) The PRISMA 2020 statement: An updated guideline for reporting systematic reviews. BMJ 372: , n71. |
[13] | Alvariño R , Alonso E , Lacret R , Oves-Costales D , Genilloud O , Reyes F , Alfonso A , Botana LM ((2018) ) Streptocyclinones A and B ameliorate Alzheimer’s disease pathological processes. Neuropharmacology 141: , 283–295. |
[14] | Chen J , Long Z , Li Y , Luo M , Luo S , He G ((2019) ) Alteration of the Wnt/GSK3β/β-catenin signalling pathway by rapamycin ameliorates pathology in an Alzheimer’s disease model. Int J Mol Med 44: , 313–323. |
[15] | Kwon Y , Shin J , Nam K , An JS , Yang SH , Hong SH , Bae M , Moon K , Cho Y , Woo J , Park K , Kim K , Shin J , Kim BY , Kim Y , Oh DC ((2020) ) Rhizolutin, a novel 7/10/6-tricyclic dilactone, dissociates misfolded protein aggregates and reduces apoptosis/inflammation associated with Alzheimer’s disease. Angew Chem Int Ed Engl 59: , 22994–22998. |
[16] | Leirós M , Alonso E , Rateb ME , Ebel R , Jaspars M , Alfonso A , Botana LM ((2015) ) The Streptomyces metabolite Anhydroexfoliamycin ameliorates hallmarks of Alzheimer’s disease in-vitro and in-vivo. Neuroscience 305: , 26–35. |
[17] | Alvariño R , Alonso E , Lacret R , Oves-Costales D , Genilloud O , Alfonso A , Botana LM ((2019) ) Caniferolide A, a macrolide from Streptomyces caniferus, attenuates neuroinflammation, oxidative stress, amyloid-β (Aβ), and tau pathology in vitro. Mol Pharm 16: , 1456–1466. |
[18] | Eftekharzadeh B , Hamedia J , Mohammadipanah F , Khodagholi F , Maghsoudi N , Klenk HP ((2010) ) Inhibition of oxidative stress-induced amyloid β formation in NT2 neurons by culture filtrate of a strain of Streptomyces antibioticus. Appl Microbiol Biotechnol 86: , 1805–1811. |
[19] | Ramírez AE , Pacheco CR , Aguayo LG , Opazo CM ((2014) ) Rapamycin protects against Aβ-induced synaptotoxicity by increasing presynaptic activity in hippocampal neuron. Biochim Biophys Acta 1842: , 1495–1501. |
[20] | Shin YH , Ban YH , Shin J , Park IW , Yoon S , Ko K , Shin J , Nam SJ , Winter JM , Kim Y , Yoon YJ , Oh DC ((2021) ) Azetidine-bearing non-ribosomal peptides, bonnevillamides D and E, isolated from a carrion beetle-associated Actinomycete. J Org Chem 86: , 11149–11159. |
[21] | Singh AK , Kashyap MP , Tripathi VK , Singh S , Garg G , Rizvi SI ((2017) ) Neuroprotection through rapamycin-induced activation of autophagy and PI3K/Akt1/mTOR/CREB signalling against amyloid-β-induced oxidative stress, synaptic/neurotransmission dysfunction, and neurodegeneration in adult rats. Mol Neurobiol 54: , 5815–5828. |
[22] | Wani A , Gupta M , Ahmad M , Shah AM , Ahsan AU , Qazi PH , Malik F , Singh G , Sharma PR , Kaddoumi A , Bharate SB , Vishwakarma RA , Kumar A ((2019) ) Alborixin clears amyloid-β by inducing autophagy through PTEN-mediated inhibition of the AKT pathway. Autophagy 15: , 1810–1828. |
[23] | Xu DB , Ma M , Deng ZX , Hong K ((2015) ) Genotype-driven isolation of enterocin with novel bioactivities from mangrove-derived Streptomyces qinglanensis 172205. Appl Microbiol Biotechnol 99: , 5825–5832. |
[24] | Zhang S , Salemi J , Hou H , Zhu Y , Mori T , Giunta B , Obregon D , Tan J ((2010) ) Rapamycin promotes beta-amyloid production via ADAM-10 inhibition. Biochem Biophys Res Commun 398: , 337–341. |
[25] | Mueed Z , Tandon P , Maurya SK , Deval R , Kamal MA , Poddar NK ((2019) ) Tau and mTOR: The hotspots for multifarious diseases in Alzheimer’s development. Front Neurosci 12: , 1017. |
[26] | Pickford F , Masliah E , Britschgi M , Lucin K , Narasimhan R , Jaeger PA , Small S , Spencer B , Rockenstein E , Levine B , Wyss-Coray T ((2008) ) The autophagy-related protein Beclin-1 shows reduced expression in early Alzheimer disease and regulates amyloid-beta accumulation in mice. J Clin Invest 118: , 2190–2199. |
[27] | Jaeger PA , Wyss-Coray T ((2010) ) Beclin-1 complex in autophagy and Alzheimer disease. Arch Neurol 67: , 1181–1184. |
[28] | Liu J , Li L ((2019) ) Targeting autophagy for the treatment of Alzheimer’s disease: Challenges and opportunities. Front Mol Neurosci 12: , 203. |
[29] | Long HZ , Cheng Y , Zhou ZW , Luo HY , Wen DD , Gao LC ((2021) ) PI3K/AKT signal pathway: A target of natural products in the prevention and treatment of Alzheimer’s disease and Parkinson’s disease. Front Pharmacol 12: , 648636. |
[30] | Ramsey CP , Glass CA , Montgomery MB , Lindl KA , Ritson GP , Chia LA , Hamilton RL , Chu CT , Jordan-Sciutto KL ((2008) ) Expression of Nrf2 in neurodegenerative diseases. J Neuropathol Exp Neurol 66: , 75–85. |
[31] | Bahn G , Park JS , Yun UJ , Lee YJ , Choi Y , Park JS , Baek SH , Choi BH , Cho YS , Kim HK , Han J , Sul JH , Baik SH , Lim J , Wakabayashi N , Bae SH , Han JW , Arumugam TV , Mattson MP , Jo DG ((2019) ) NRF2/ARE pathway negatively regulates BACE1 expression and ameliorates cognitive deficits in mouse Alzheimer’s models. Proc Natl Acad Sci U S A 116: , 12516–12523. |
[32] | Magalhães CA , Carvalho M , Sousa LP , Caramelli P , Gomes KB ((2017) ) Alzheimer’s disease and cytokine IL-10 gene polymorphisms: Is there an association? Arq Neuropsiquiatr 75: , 649–656. |
[33] | Weston LL , Jiang S , Chisholm D , Jantzie LL , Bhaskar K ((2021) ) Interleukin-10 deficiency exacerbates inflammation-induced tau pathology. J Neuroinflammation 18: , 161. |
[34] | Xie L , Lai Y , Lei F , Liu S , Liu R , Wang T ((2015) ) Exploring the association between interleukin-1β and its interacting proteins in Alzheimer’s disease. Mol Med Rep 11: , 3219–3228. |
[35] | Wang WY , Tan MS , Yu JT , Tan L ((2015) ) Role of pro-inflammatory cytokines released from microglia in Alzheimer’s disease. Ann Transl Med 3: , 136. |
[36] | Salminen A , Kaarniranta K , Haapasalo A , Hiltunen M , Soininen H , Alafuzoff I ((2012) ) Emerging role of p62/sequestosome-1 in the pathogenesis of Alzheimer’s disease. Prog Neurobiol 96: , 87–95. |
[37] | Yeo IJ , Yun J , Son DJ , Han SB , Hong JT ((2020) ) Antifungal drug miconazole ameliorated memory deficits in a mouse model of LPS-induced memory loss through targeting iNOS. Cell Death Dis 11: , 623. |
[38] | Chen CH , Zhou W , Liu S , Deng Y , Cai F , Tone M , Tone Y , Tong Y , Song W ((2012) ) Increased NF-κB signalling up-regulates BACE1 expression and its therapeutic potential in Alzheimer’s disease. Int J Neuropsychopharmacol 15: , 77–90. |
[39] | Chang R , Yee KL , Sumbria RK ((2017) ) Tumour necrosis factor α Inhibition for Alzheimer’s disease. J Cent Nerv Syst Dis 9: , 1179573517709278. |
[40] | Kheiri G , Dolatshahi M , Rahmani F , Rezaei N ((2019) ) Role of p38/MAPKs in Alzheimer’s disease: Implications for amyloid-beta toxicity targeted therapy. Rev Neurosci 30: , 9–30. |
[41] | Babić Leko M , Nikolac Perković M , Klepac N , Štrac DŠ , Borovečki F , Pivac N , Hof PR , Šimić G ((2020) ) IL-1 beta, IL-6, IL-10, and TNF alpha single nucleotide polymorphisms in human influence the susceptibility to Alzheimer’s disease pathology. J Alzheimers Dis 75: , 1029–1047. |
[42] | Lauretti E , Dincer O , Praticò D ((2020) ) Glycogen synthase kinase-3 signalling in Alzheimer’s disease. Biochim Biophys Acta Mol Cell Res 1867: , 118664. |
[43] | Borghi R , Piccini A , Barini E , Cirmena G , Guglielmotto M , Tamagno E , Fornaro M , Perry G , Smith MA , Garuti A , Tabaton M ((2010) ) Upregulation of presenilin 1 in brains of sporadic, late-onset Alzheimer’s disease. J Alzheimers Dis 22: , 771–775. |
[44] | Deng Y , Zhang J , Sun X , Ma G , Luo G , Miao Z , Song L ((2020) ) miR-132 improves the cognitive function of rats with Alzheimer’s disease by inhibiting the MAPK1 signal pathway. Exp Ther Med 20: , 159. |
[45] | Yarza R , Vela S , Solas M , Ramirez MJ ((2016) ) c-Jun N-terminal Kinase (JNK) Signaling as a therapeutic target for Alzheimer’s disease. Front Pharmaco l6: , 321. |
[46] | Yu HT , Chan WWL , Chai KH , Lee CWC , Chang RCC , Yu MS , McLoughlin DM , Miller CCJ , Lau KF ((2010) ) Transcriptional regulation of human FE65, a ligand of Alzheimer’s disease amyloid precursor protein, by Sp1. J Cell Biochem 109: , 782–793. |
[47] | Rossner S , Sastre M , Bourne K , Lichtenthaler SF ((2006) ) Transcriptional and translational regulation of BACE1 expression–implications for Alzheimer’s disease. Prog Neurobiol 79: , 95–111. |
[48] | Trabzuni D , Wray S , Vandrovcova J , Ramasamy A , Walker R , Smith C , Luk C , Gibbs JR , Dillman A , Hernandez DG , Arepalli S , Singleton AB , Cookson MR , Pittman AM , de Silva R , Weale ME , Hardy J , Ryten M ((2012) ) MAPT expression and splicing is differentially regulated by brain region: Relation to genotype and implication for tauopathies. Hum Mol Genet 21: , 4094–4103. |
[49] | Song C , Shi J , Zhang P , Zhang Y , Xu J , Zhao L , Zhang R , Wang H , Chen H ((2022) ) Immunotherapy for Alzheimer’s disease: Targeting β-amyloid and beyond. Transl Neurodegener 11: , 18. |
[50] | Söderberg L , Johannesson M , Nygren P , Laudon H , Eriksson F , Osswald G , Möller C , Lannfelt L ((2023) ) Lecanemab, aducanumab, and gantenerumab – binding profiles to different forms of amyloid-beta might explain efficacy and side effects in clinical trials for Alzheimer’s disease. Neurotherapeutics 20: , 195–120. |
[51] | Honig LS , Barakos J , Dhadda S , Kanekiyo M , Reyderman L , Irizarry M , Kramer LD , Swanson CJ , Sabbagh M ((2023) ) ARIA in patients treated with lecanemab (BAN2401) in a phase 2 study in early Alzheimer’s disease. Alzheimers Dement 9: , e12377. |
[52] | Burke JF , Kerber KA , Langa KM , Albin RL , Kotagal V ((2023) ) Lecanemab: Looking Before We Leap. Neurology 101: , 661–665. |
[53] | Hampel H , Elhage A , Cho M , Apostolova LG , Nicoll JAR , Atri A ((2023) ) Amyloid-related imaging abnormalities (ARIA): Radiological, biological and clinical characteristics. Brain 146: , 4414–4424. |
[54] | Hayakawa Y , Kobayashi T , Izawa M ((2013) ) Indanostatin, a new neuroprotective compound from Streptomyces sp. J Antibiot (Tokyo) 66: , 731–733. |
[55] | Hayakawa Y , Yamazaki Y , Kurita M , Kawasaki T , Takagi M , Shin-ya K ((2010) ) Flaviogeranin, a new neuroprotective compound from Streptomyces sp. J Antibiot (Tokyo) 63: , 379–380. |
[56] | Tiwari S , Atluri V , Kaushik A , Yndart A , Nair M ((2019) ) Alzheimer’s disease: Pathogenesis, diagnostics, and therapeutics. Int J Nanomedicine 14: , 5541–5554. |
[57] | García-Ayllón MS , Lopez-Font I , Boix CP , Fortea J , Sánchez-Valle R. Lleó , Molinuevo JL , Zetterberg H , Blennow K , Sáez-Valero J ((2017) ) C-terminal fragments of the amyloid precursor protein in cerebrospinal fluid as potential biomarkers for Alzheimer’s disease. Sci Rep 7: , 2477. |
[58] | ClinicalTrials.gov, Cognition, Age, and RaPamycin Effectiveness – DownregulatIon of thE mTor Pathway (CARPE_DIEM): NCT04200911, https://classic.clinicaltrials.gov/ct2/show/results/NCT04200911?term=NCT04200911&draw=2&rank=1. Last updated March 21, 2023, Accessed on September 2. 2023 |
[59] | ClinicalTrial.gov. Rapamycin – Effects on Alzheimer’s and Cognitive Health (REACH): NCT04629495, https://classic.clinicaltrials.gov/ct2/show/NCT04629495?term=NCT04629495&draw=2&rank=1. Last updated October 12, 2022, Accessed on September 2. 2023 |
[60] | Funderburk SF , Marcellino BK , Yue Z ((2010) ) Cell “self-eating” (autophagy) mechanism in Alzheimer’s disease. Mt Sinai J Med 77: , 59–68. |
[61] | Zare-Shahabadi A , Masliah E , Johnson GV , Rezaei N ((2015) ) Autophagy in Alzheimer’s disease. Rev Neurosci 26: , 385–395. |
[62] | Uddin MS , Stachowiak A , Mamun AA , Tzvetkov NT , Takeda S , Atanasov AG , Bergantin LB , Abdel-Daim MM , Stankiewicz AM ((2018) ) Autophagy and Alzheimer’s disease: From molecular mechanisms to therapeutic implications. Front Aging Neurosci 10: , 04. |
[63] | Ye X , Zhou XJ , Zhang H ((2018) ) Exploring the role of autophagy-related gene 5 (ATG5) yields important insights into autophagy in autoimmune/autoinflammatory diseases. Front Immunol 9: , 2334. |
[64] | Leng F , Edison P ((2021) ) Neuroinflammation and microglial activation in Alzheimer disease: Where do we go from here? Nat Rev Neurol 17: , 157–172. |
[65] | Tang Z , Hu B , Zang F , Wang J , Zhang X , Chen H ((2019) ) Nrf2 drives oxidative stress-induced autophagy in nucleus pulposus cells via a Keap1/Nrf2/p62 feedback loop to protect intervertebral disc from degeneration. Cell Death Dis 10: , 510. |
[66] | Cetin S , Knez D , Gobec S , Kos J , Pišlar A ((2022) ) Cell models for Alzheimer’s and Parkinson’s disease: At the interface of biology and drug discovery. Biomed Pharmacother 149: , 112924. |
[67] | Sharma NS , Karan A , Lee D , Yan Z , Xie J ((2021) ) Advances in modelling Alzheimer’s disease in vitro. Adv Nanobiomed Res 1: , 2100097. |
[68] | Akhtar A , Gupta SM , Dwivedi S , Kumar D , Shaikh MF , Negi A ((2022) ) Preclinical models for Alzheimer’s disease: Past, present, and future approaches. ACS Omega 7: , 47504–47517. |
[69] | Sun D , Gao W , Hu H , Zhou S ((2022) ) Why 90% of clinical drug development fails and how to improve it. Acta Pharm Sin B 12: , 3049–3062. |