Identifying Suitable Targets for Alzheimer’s Disease and Other Eight Common Neurological Disorders Using the Human Plasma Proteome: A Mendelian Randomization Study
Abstract
Background:
Neurological disorders, such as Alzheimer’s disease (AD), comprise a major cause of health-related disabilities in human. However, biomarkers towards pathogenesis or novel targets are still limited.
Objective:
To identify the causality between plasma proteins and the risk of AD and other eight common neurological diseases using a Mendelian randomization (MR) study.
Methods:
Exposure data were obtained from a genome-wide association study (GWAS) of 2,994 plasma proteins in 3,301 healthy adults, and outcome datasets included GWAS summary statistics of nine neurological disorders. Inverse variance-weighted MR method as the primary analysis was used to estimate causal effects.
Results:
Higher genetically proxied plasma myeloid cell surface antigen CD33 level was found to be associated with increased risk of AD (odds ratio [OR] 1.079, 95% confidence interval [CI] 1.047–1.112, p = 8.39×10-7). We also discovered the causality between genetically proxied elevated prolactin and higher risk of epilepsy (OR = 1.068, 95% CI = 1.034–1.102; p = 5.46×10-5). Negative associations were identified between cyclin-dependent kinase 8 and ischemic stroke (OR = 0.927, 95% CI = 0.896–0.959, p = 9.32×10-6), between neuralized E3 ubiquitin-protein ligase 1 and migraine (OR = 0.914, 95% CI = 0.878–0.952, p = 1.48×10-5), and between Fc receptor-like protein 4 and multiple sclerosis (MS) (OR = 0.929, 95% CI = 0.897–0.963, p = 4.27×10-5).
Conclusion:
The findings identified MR-level protein-disease associations for AD, epilepsy, ischemic stroke, migraine, and MS.
INTRODUCTION
Neurological diseases are the leading cause of disability and rank as the second leading cause of death in the world [1, 2]. Given the progressive aging of the global population, the prevalence of neurological diseases, such as Alzheimer’s disease (AD) [3], Parkinson’s disease (PD) [4], stroke [5], and amyotrophic lateral sclerosis (ALS) [6], is gradually increasing. Despite massive investments aimed at finding new therapeutic strategies for neurological disorders [1], most remain essentially intractable, owing to a combination of complex underlying biology and long prodromal phases.
Proteomics has revolutionized neurological research and has boosted the discovery of suitable biomarkers for many neurological diseases [7]. Human blood contains a dynamic flux of proteins important for biological processes [8], including cell signaling, tissue repair, and host defense against external stimuli. Thus, circulating proteins are not merely used as indicators of health status, but deep characterization of blood proteins can inform on pathological mechanisms and help identify clinical intervention strategies for diseases. However, while proteomics can very efficiently identify candidate proteins related to disease, the translation of these findings into practice is complicated by the redundant functions of proteins, the plasticity of biological pathways, and unknown factors that may influence the course of disease or the response to therapeutic intervention. One approach to improve the translatability of proteomics data is to integrate proteomic and genetic datasets. It helps systematically evaluate the putative causal roles and genetic evidence in support of disease-causing pathways [9].
Mendelian randomization (MR) is an effective genetic approach to investigate the causal effect of certain exposures on diseases or clinical traits [10]. This method has been widely used to prioritize targets for neurological diseases [11, 12]. In this study, we applied a two-sample MR method to leverage summary statistics from large-scale genome-wide association study (GWAS) datasets to uncover causal relationships between circulating proteins and neurological diseases, including AD, PD, ALS, multiple sclerosis (MS), epilepsy, ischemic stroke, intracranial hemorrhage (ICH), subarachnoid hemorrhage (SAH), and migraine. We identified a protein-disease associative pair for AD, MS, epilepsy, ischemic stroke, and migraine that warrant further study to determine whether the identified proteins have a causal role in disease pathology.
MATERIALS AND METHODS
Study design
We conducted a two-sample MR analysis to explore the causal relationships between 2,994 plasma proteins and nine neurological diseases (AD, PD, ALS, MS, epilepsy, ischemic stroke, ICH, SAH, and migraine). It is based on 3 core assumptions: 1) genetic variants are strongly associated with the exposure (2,994 plasma proteins); 2) the variants are not associated with confounding factors; 3) the variants affect the outcome (nine neurological diseases) only through their influences on the exposure of interest (2,994 plasma proteins). A graphical overview of the general MR design was shown in Fig. 1. This study follows the guidelines for Strengthening the Reporting of Observational Studies in Epidemiology-Mendelian randomization (Supplementary Table 14) [13].
Fig. 1
The overall design of Mendelian randomization analysis in the present study. Assumption 1, the genetic variants are supposed to be strongly associated with the risk of interest; Assumption 2, the genetic variants should not be associated with any confounding factors; and Assumption 3, the genetic variants should affect the risk of the outcome only mediated by the exposures. IVs, Instrument variants. MR, Mendelian randomization.
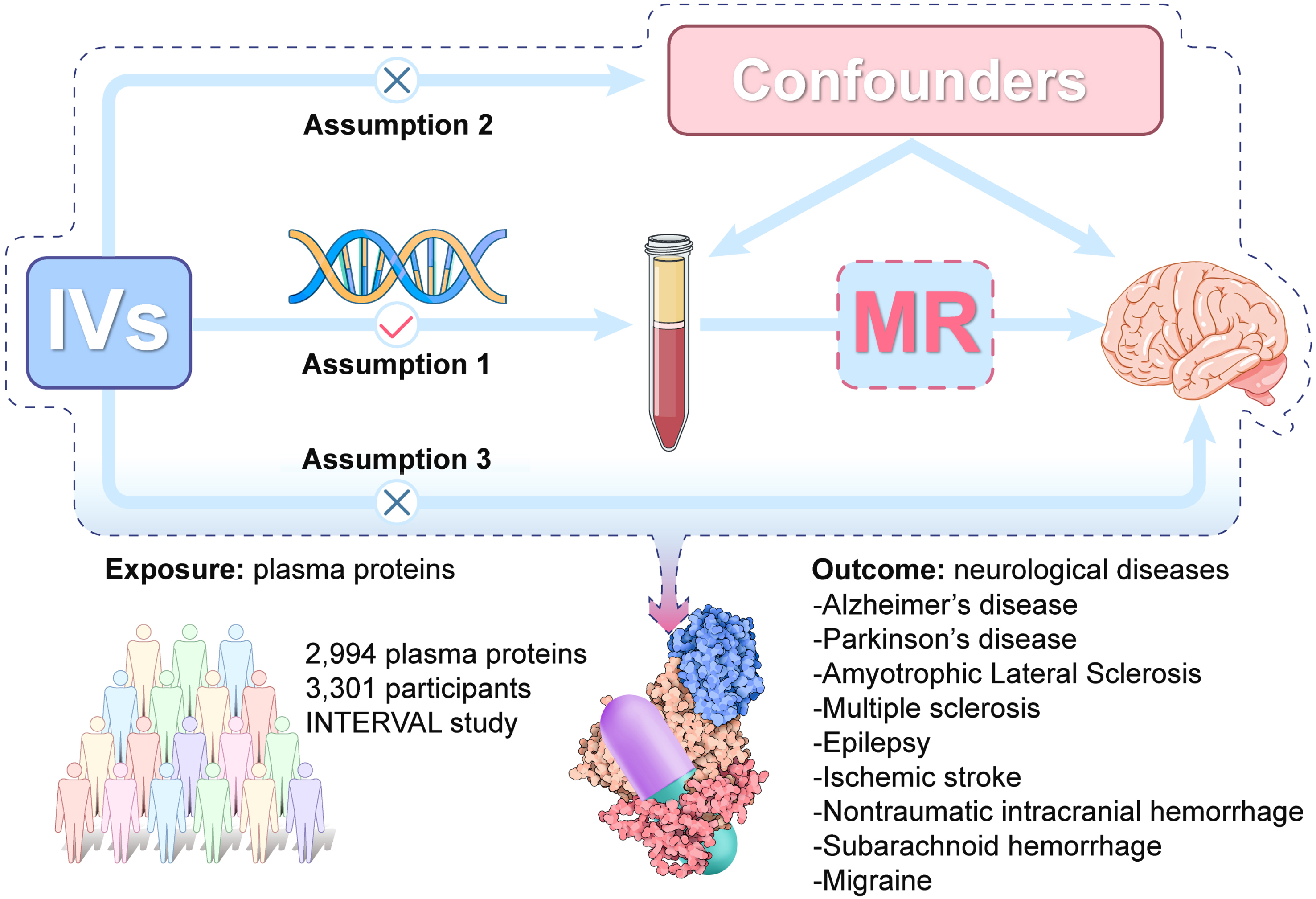
Data sources
Plasma proteins
Summary statistics for plasma proteins were obtained from the genomic atlas of the human plasma proteome, a large-scale GWAS of 3,301 healthy adults from the INTERVAL study [14]. The INTERVAL study is a prospective cohort study that recruited approximately 50,000 blood donors of European ancestry. The plasma proteome was quantified using an aptamer-based, multiplexed approach (SOMAscan assay). After strict quality control, 3,283 SOMAmers that mapped to 2,994 plasma proteins were included in the final GWAS. The GWAS datasets were adjusted for age, sex, duration between blood draw and processing, and the first three principal components of ancestry from multi-dimensional scaling (Table 1).
Table 1
Detailed information of the studies and datasets used for Mendelian randomization analysis
Phenotype | Sample Size (cases/controls) | Ancestry | Consortium | Adjustments |
Plasma proteins | 3301 | European | – | age, sex, duration between blood draw and processing, and the first three principal components of ancestry from multi-dimensional scaling |
Neurodegenerative disease | ||||
Alzheimer’s disease | 21982/ 41944 | European | IGAP | age, sex, and population substructure using principal components |
Parkinson’s disease | 33674/ 449056 | European | IPDGC | age at onset (cases) or exam (controls), sex, the first 2 eigenvectors from principal components analysis |
Amyotrophic lateral sclerosis | 20806/ 59804 | European | AVS | age, gender, and 2 to 4 principal components |
Multiple sclerosis | 47429/ 68374 | European | IMSGC | age and sex |
Epilepsy | 15212/ 29677 | Mixed | ILAE | age, sex, and ethnicity |
Cerebrovascular diseases | ||||
Ischemic stroke | 34217/406111 | European | MEGASTROKE | age and sex |
Intracranial hemorrhage | 2794/203068 | European | FinnGen | age and sex |
Subarachnoid hemorrhage | 1338/201230 | European | FinnGen | age and sex |
Migraine | 48,975/540,381 | European | IHGC | age and sex |
AVS, ALS Variant Server; IGAP, International Genomics of Alzheimer’s Project; IHGC, International Headache Genetics Consortium; ILAE, International League Against Epilepsy; IMSGC, International Multiple Sclerosis Genetics Consortium; IPDGC, International Parkinson’s Disease Genomics Consortium.
Neurological diseases
For AD, PD, and ALS, we used the corresponding GWAS data from the International Genomics of Alzheimer’s Project (21,982 cases, 41,944 controls) [15], the International Parkinson’s Disease Genomics Consortium (33,674 cases, 449,056 controls) [16], and the ALS Variant Server (20,806 cases, 59,804 controls) [17]. Genetic association estimates for MS and epilepsy were obtained respectively from the International Multiple Sclerosis Genetics Consortium (47,429 cases, 68,374 controls) [18] and the International League Against Epilepsy consortium (15,212 cases, 2,9677 controls) [19]. We extracted genetic variants of ischemic stroke from the MEGASTROKE consortium, which included 34,217 cases and 406,111 controls [20]. For ICH and SAH, GWAS data were derived from the FinnGen consortium, which included 2,794 ICH cases and 1,338 SAH cases. We obtained summary-level data for migraine from the International Headache Genetics Consortium, including 48,975 cases and 540,381 controls (participants from 23andMe cohort were not included) [21]. Participants in all studies were of European ancestry, expecting data from the International League Against Epilepsy consortium, which included a small population of Asian and African-American ethnicities. The covariates that were adjusted for age, sex, ethnicity, and population substructure using principal components. Further details regarding the datasets can be found in Table 1.
Selection of instruments
Few significant SNPs for each plasma protein met the significance threshold of 5×10-8 (less than 3 SNPs, mostly). Thus, we set a relatively relaxed threshold of 1×10-5, which is often used in MR analyses [12, 22, 23]. Next, the selected SNPs were clumped for linkage disequilibrium (LD) to r2 <0.001 within a 10 Mb window based on the 1,000 Genomes (EUR) reference panel [24]. When no SNP was available in the outcome dataset, proxy SNPs with LD of r2 > 0.9 were used. In addition, F-statistics was calculated to ensure the strength of exposures and F-statistics >10 was considered sufficient [25].
Statistical analysis
The main analyses were performed using the random-effect inverse-variance weighted (IVW) method [26]. The IVW method would be considered the best causal estimation if none of the instruments were found to have substantial heterogeneity nor horizontal pleiotropy [27]. To allow for multiple testing, we used a Bonferroni-corrected significant threshold of p < 1.52×10-5 (0.05 divided by 3283 SOMAmers). p < 0.05 above the Bonferroni-corrected threshold was suggestive of an association.
For sensitivity analyses, we used alternative MR methods to check the validity of our results, including weighted median, MR-Egger, and weighted mode [27, 28]. When up to 50% of invalid SNPs, weighted median estimates still yield consistent estimates of causal effects [28]. The MR-Egger method was still useful even when up to 100% of the genetic variants were invalid [29]. In addition, we used the Cochran’s Q statistic, MR-Egger intercept, and MR-PRESSO global test to detect the presence of heterogeneity, directional pleiotropy, and potential outliers, respectively [30–32]. Cochran’s Q statistic evaluated heterogeneity across genetic variants [33]. The MR-Egger intercept test was also conducted, and the non-zero intercept indicated that the IVW results might be invalid due to horizontal pleiotropy [29]. MR-PRESSO method provided a correction test by detecting and removing potentially pleiotropic outliers [32]. Moreover, reverse MR analyses were conducted to rule out false positives and power analysis were done to further ensure the robustness of the findings.
The main analyses were performed using the TwoSampleMR (v.0.5.6) in R version 4.1.1 [34]. Statistical power was estimated using the online power calculator (mRnd) (https://cnsgenomics.com/shiny/mRnd/. We also used the PhenoScanner database (Version 2, http://www.phenoscanner.medschl.cam.ac.uk/) [36] to see whether SNPs were associated with potential risk factors (p < 5×10-8), that may affect the neurological diseases being studied.
RESULTS
The MR-predicted causal effects of human plasma proteins on the AD and other eight neurological disorders were shown in Fig. 2. Two risk proteins and three protective proteins were identified in the framework.
Fig. 2
Volcano plot showing the causal effects of human plasma proteins on nine neurological diseases. Data are expressed as raw odds ratios estimated by the IVW method. The red solid line represents the Bonferroni-corrected significant threshold of p < 1.52×10-5. The black dotted line represents the suggestive association threshold of p < 0.05. A) Alzheimer’s disease, B) ischemic stroke, C) migraine, D) epilepsy, E) multiple sclerosis, F) amyotrophic lateral sclerosis, G) Parkinson’s disease, H) intracranial hemorrhage, I) subarachnoid hemorrhage.
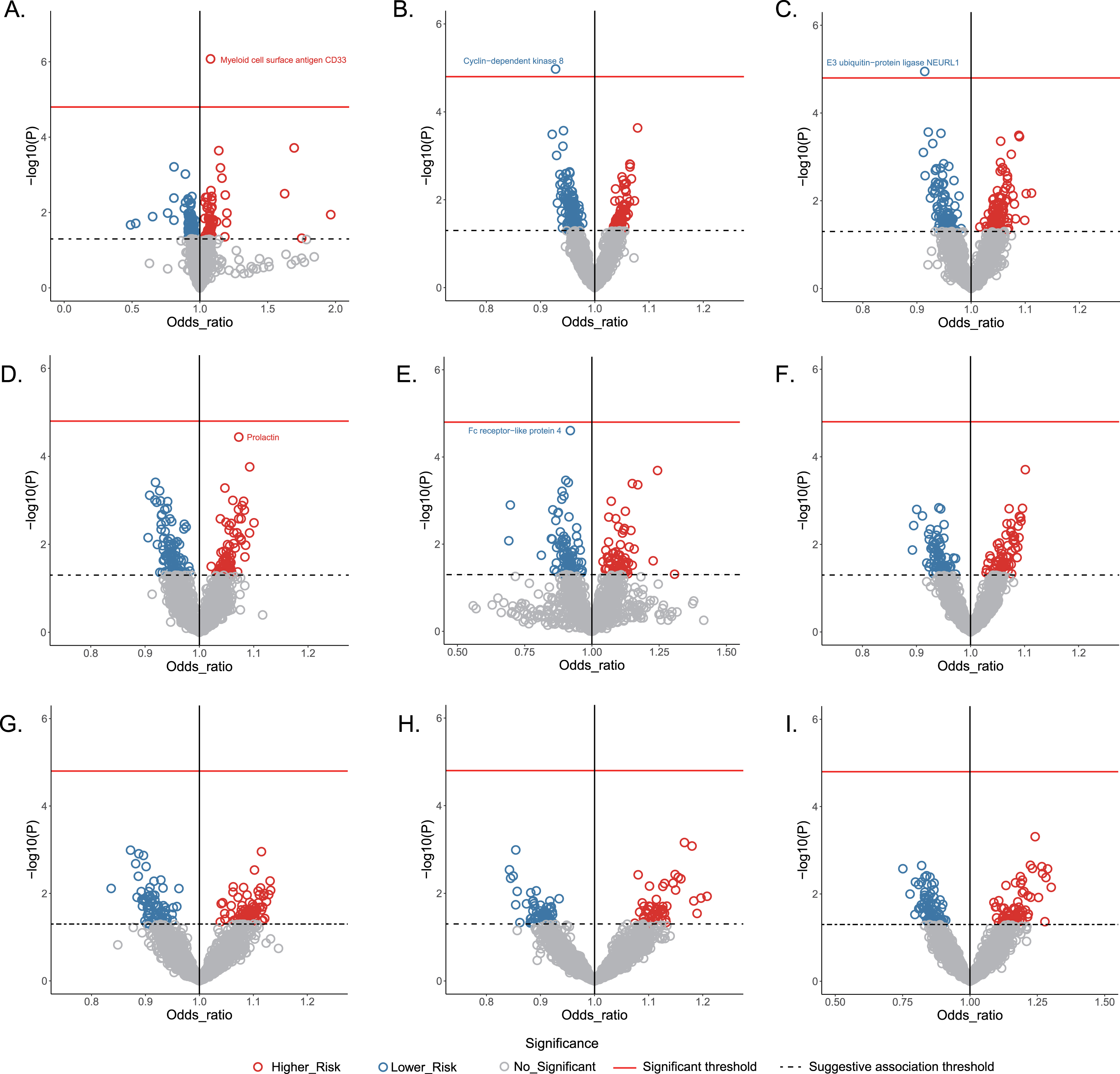
Genetically predicted plasma proteins associated with AD
Based on IVW mode, MR estimates indicated that each standard deviation (SD) increase of plasma myeloid cell surface antigen CD33 was associated with an approximately 8% higher risk of AD (odds ratio [OR] 1.079, 95% confidence interval [CI] 1.047–1.112, p = 8.39×10-7). Across MR-Egger, weighted median, MR-PRESSO and weighted mode, the effect estimates remained directionally consistent and stable with p < 0.05 (Table 2). Importantly, there was no evidence of heterogeneity or horizontal pleiotropy in any of the analyses (p >,0.05).
Table 2
Mendelian randomization results of plasma proteins on neurological diseases
Trait | nSNPs | Method | OR (95% CI) | p | Q pval | Intercept pval | Global pval |
Myeloid cell surface antigen CD33 versus AD | 18 | IVW | 1.079 (1.047, 1.112) | 8.39E-07 | 0.390 | ||
MR Egger | 1.091 (1.043, 1.141) | 2.19E-03 | 0.520 | ||||
MR-PRESSO | 1.076 (1.047, 1.105) | 5.80E-05 | 0.460 | ||||
Weighted median | 1.088 (1.054, 1.124) | 2.96E-07 | |||||
Weighted mode | 1.090 (1.053, 1.128) | 2.30E-04 | |||||
Cyclin-dependent kinase 8 versus ischemic stroke | 28 | IVW | 0.927 (0.896, 0.959) | 9.32E-06 | 0.441 | ||
MR Egger | 0.964 (0.893, 1.041) | 0.355 | 0.274 | ||||
MR-PRESSO | 0.927 (0.896, 0.959) | 1.40E-04 | 0.466 | ||||
Weighted median | 0.927 (0.882, 0.975) | 3.17E-03 | |||||
Weighted mode | 0.936 (0.860, 1.019) | 0.139 | |||||
Neuralized E3 ubiquitin-protein ligase 1 versus migraine | 16 | IVW | 0.914 (0.878, 0.952) | 1.48E-05 | 0.142 | ||
MR Egger | 0.930 (0.828, 1.045) | 0.241 | 0.764 | ||||
MR-PRESSO | 0.914 (0.878, 0.952) | 5.93E-04 | 0.207 | ||||
Weighted median | 0.921 (0.876, 0.969) | 1.36E-03 | |||||
Weighted mode | 0.920 (0.863, 0.982) | 0.024 | |||||
Prolactin versus epilepsy | 19 | IVW | 1.068 (1.034, 1.102) | 5.46E-05 | 0.240 | ||
MR Egger | 1.098 (0.945, 1.277) | 0.237 | 0.710 | ||||
MR-PRESSO | 1.068 (1.034, 1.102) | 7.77E-04 | 0.312 | ||||
Weighted median | 1.057 (1.013, 1.101) | 8.99E-03 | |||||
Weighted mode | 1.052 (0.985, 1.125) | 0.147 | |||||
Fc receptor-like protein 4 versus multiple sclerosis | 18 | IVW | 0.929 (0.897, 0.963) | 4.27E-05 | 0.378 | ||
MR Egger | 0.935 (0.892, 0.980) | 0.012 | 0.702 | ||||
MR-PRESSO | 0.930 (0.898, 0.963) | 6.65E-04 | 0.495 | ||||
Weighted median | 0.939 (0.903, 0.976) | 1.45E-03 | |||||
Weighted mode | 0.940 (0.905, 0.978) | 6.41E-03 |
AD, Alzheimer’s disease; CI, confidence interval; IVW, inverse-variance weighted; MR-PRESSO, Mendelian randomization-Pleiotropy Residual Sum and Outlier, nSNPs, number of single nucleotide polymorphisms; OR, odds ratio; Q_pval, P-value of the Cochran Q statistic.
Genetically predicted plasma proteins associated with ischemic stroke or migraine
MR estimates identified one causal protective plasma protein each for ischemic stroke and migraine. Specially, the genetically predicted level of cyclin-dependent kinase 8 (CDK8) was causally related to lower risk of ischemic stroke (IVW: OR 0.927, 95% CI 0.896–0.959, p = 9.32×10-6), and the effect estimate for the relationship between neuralized E3 ubiquitin-protein ligase 1 (NEURL1) and migraine produced an OR of 0.914 (95% CI, 0.878–0.952, p = 1.48×10-5, IVW mode). The statistically significant associations were broadly stable across the sensitivity analyses. Further, no pleiotropy or heterogeneity were observed using Cochran’s Q test, the MR-Egger intercept test, or the MR-PRESSO global test (p >0.05).
Genetically predicted plasma proteins associated with epilepsy or MS
MR analyses identified marginally significant associations of two plasma proteins with the risk of epilepsy and MS, respectively. Genetically determined prolactin was positively associated with epilepsy risk (OR: 1.068, 95% CI: 1.034–1.102; p = 5.46×10-5; IVW mode). Higher level of genetically predicted Fc receptor-like protein 4 (FCRL4) was inversely associated with the risk of MS (OR: 0.929, 95% CI: 0.897–0.963, p = 4.27×10-5). The effect estimates in IVW mode were similar to those obtained in sensitivity analyses, and there was no evidence of heterogeneity or directional pleiotropy (Table 2).
Genetically predicted plasma proteins associated with PD, ALS, ICH, or SAH
The MR tried to identify potential causal relationships between plasma proteins and PD, ALS, ICH, or SAH; however, none of these associations approached the significance threshold when the Bonferroni correction was applied (Fig. 2).
Reverse analysis between plasma proteins and neurological diseases
Reverse MR analyses were conducted on the aforementioned identified plasma proteins and their associated diseases. They failed to identity significant causality, indicating that the causal effects were statistically robust and not false positives (Supplementary Table 1).
All statistical analyses reached an estimated power analysis of more than 85% (Supplementary Table 2). For the IVs used for these plasma proteins, all F-statistics were above 10 (ranging from 19.27 to 2123.37; Supplementary Tables 3–12). To test whether the estimate was biased by potential risk factors, we searched SNPs for each IV in Phenoscanner database. As a result, in the analysis of CDK8, rs1689804 was found to be associated with high-density lipoprotein (HDL) (p = 9.07×10-9) and HDL cholesterol (p = 1.80×10-8). After removing this SNP, the effect estimates in IVW mode was consistent with previous results (OR: 0.926, 95% CI: 0.89–0.958, p = 1.27×10-5), suggesting that the causality between CDK8 and ischemic stroke was not affected by this factor.
Additionally, we identified six plasma proteins that were significantly associated with AD risk, including leucine-rich repeat neuronal protein 1 (LRRN1), cardiotrophin-1 level (CTP1), MAP kinase-activated protein kinase 5 (MAPKAPK5), vacuolar protein sorting-associated protein 29 (VPS29), Protein S100-A13 (S100A13), and proteasome activator complex subunit 1 (PSME1). However, the estimates for all these associations appear to have been biased by moderate to high pleiotropy and heterogeneity (Supplementary Table 13).
DISCUSSION
Identifying suitable targets for neurological diseases remains a challenging task. This study integrated large-scale GWAS datasets from multiple cohorts and proposes a two-sample MR framework to identify candidate proteins and their links with nine common neurological diseases.
We identified five protein-disease associative pairs, and the robust statistical analysis suggested that these relationships were unlikely to be affected by confounding factors or pleiotropic effects. Among them, increased level of CD33 was prioritized across the models of IVW, MR-Egger, MR-PRESSO, weighted median and weighted mode, as a genetic proxy for higher risk of AD. The role of CD33 in AD is increasingly recognized. The link has been identified through GWAS, wherein rs3826656, rs3865444, and rs12459419 were discovered as susceptibility factors for AD [37]. Further, it has been reported that CD33, which is primarily expressed in microglia, is higher in patients with AD [38]. CD33 could facilitate AD pathology by inhibiting microglia-mediated clearance of amyloid-β [39]. Our results reinforced the association between CD33 and AD by providing MR-validated causality. Moreover, the current findings were consistent with a recent MR study by Gu et al. [40], in which they found that elevated CD33 protein level in plasma was causal to the development of AD. Indeed, the GWAS datasets for exposure (CD33) and outcome (AD) selected in our analysis were also used by Gu and the colleagues [40]. However, our study set a relaxed threshold during the selection of IVs (p = 1×10-5 versus p = 5×10-8 in Gu et al. [40]). As a result, we identified a total of 20 SNPs (compared to 2 SNPs in Gu et al. [40]) (Supplementary Table 3) that were analyzed in our MR model to ensure the accuracy of sensitivity analysis. Although our study re-emphasized the role of circulating CD33 as a causal factor for AD, some studies pointed out that the higher CD33 level could be a response to AD pathology. For instance, factors in neuroinflammation such as platelet derived microparticles or cytokines are able to upregulate the expression of CD33 [41, 42].
Prolactin is a polypeptide hormone, mainly produced and secreted by the lactotroph cells of the anterior pituitary gland [43]. It is implicated in lactation, metabolic homeostasis, maternal behavior, and the adrenal response to stress [43]. The role of prolactin has been well established for epilepsy, with wide application in the diagnosis of epileptic seizures [44]. The epileptic discharge is likely to excite the hypothalamic pituitary axis, inducing secretion of prolactin releasing hormone in the hypothalamus, which can cause the pituitary to secrete prolactin into serum [45]. Elevation of serum prolactin following seizures is considered to be a surrogate marker of epilepsy, and it is informative regarding the progression of the disorder [46]. As expected, our study identified a positive association between circulating prolactin and risk of epilepsy, emphasizing its importance in the disorder. However, studies have found that prolactin can only serve a sensitive and effective biomarker when it is measured 10 to 20 minutes after an epileptic event [46]. Therefore, our finding of evidence that prolactin may have a causative relationship with epilepsy should be applied with caution owing to the complexity and dynamicity of prolactin signaling and secretion. One possible explanation is regarding the negative feedback loop of prolactin secretion. It has been shown that a brief period of restraint stress in male mice results in increased circulating prolactin [47], which then activates tuberoinfundibular dopaminergic neurons. These neurons increase their release of dopamine [48], which suppresses the production of prolactin; thus, a self-regulated framework is formed for prolactin secretion. Therefore, while it is clear that prolactin is a useful adjunct to the diagnosis of epilepsy, its participation in the etiology of epilepsy requires further investigation.
We also found that genetically proxied CDK8 was negatively associated with the risk of ischemic stroke. CDKs and their associations with ischemic stroke stem from the findings of their reactivation in dying neurons [49]. The deregulation of CDKs seems to have a major role in causing neuronal death during ischemic stroke. It has been reported that CDK4 and CDK6 are important factors in ischemic brain that regulate the progression from G1 to S phase in cell cycle [50]. CDK8, however, probably participates in ischemic stroke by regulating the mediator complex and phosphorylating the corresponding transcription factors [51]. Furthermore, CDK8 has been reported to be upregulated in a hyperglycemic state [52]; and Cdk8-knockdown mice exhibited dramatically increased lipid biosynthesis [53]. Therefore, based on the literature and our results, we posit that CDK8 may exert neuroprotective effects against ischemic stroke by regulating transcriptional and metabolic programs.
Interestingly, the association between increasing NEURL1 and lower risk of migraine fits well with a published report demonstrating the reversed trend between NEURL1 expression and genetic susceptibility to arterial fibrillation [54]. It is believed that reduced NEURL1 expression can increase the incidence of arterial fibrillation by regulating the expression of ion transporters that evoke changes in action potential duration [55]. The implication represents an important supplement to the long-running debate regarding the overlap between migraine and heart problems, in which iron-related pathways underlying the two disorders may explain why they can co-occur. Moreover, studies have indicated that NEURL1 is highly expressed in brain, and it modulates the integrity of neurological system through its participation in synaptic plasticity [56]. Variations of the NEURL1 could, therefore, contribute to differences in brain structure and connectivity associated with migraine.
Fc receptor like proteins are members of the immunoglobulin family, that are preferentially expressed in B lymphocytes [57]. Specially, FCRL4+ memory B cells are important mediators in immune responses. They express high levels of CD20 and CD95, and express low level of CD21 [58]. Further, FCRL4 defines atypical memory B cells, which are involved in endoplasmic reticulum stress and IFN-γ responses in autoimmune conditions [59]. Therefore, these findings suggest that FCRL4 should be an active driver in MS. Interestingly, our analysis provided considerable evidence of a negative causal association between FCRL4 and risk of MS, which runs counter to the current understanding of the role of FCRL4 in autoimmune conditions. The possible explanation for the inconsistency could be the influences of the disease stages; that is, it is likely that immune cell phenotypes can change during the course of MS [60]. This should be further investigated if GWAS summary data from different MS stages become available.
Our MR analysis identified six possible AD-associated proteins, but none of the candidate proteins performed satisfactorily in the detection of heterogeneity and horizontal pleiotropy. Although these findings are not statistically significant, they may provoke additional follow-up of the candidate proteins to determine whether they may be relevant to the pathogenesis of AD.
The superior performance of our study should be mentioned. First, we used a multiplex proteomics dataset with a relatively large sample size to explore novel causal targets for a wide range of neurological disorders documented in large GWAS datasets, ensuring the precise estimation of their associations. Second, the two-sample MR design using different models avoided interference by confounding factors and invalid instruments, and the bidirectional design provided evidence that the reverse causation was unlikely to influence our findings. Moreover, the great power across all analyses (>85%) supported biological credibility of our findings.
Nevertheless, our study also had several limitations. First, it is important to note that the phenotypes examined in our framework was susceptibility of the disorder, rather than disease severity or subtype. Therefore, the identified protein-disease causality should be interpreted as nominating biological targets or pathways that may be regulated to alter the risk of developing certain diseases. Though the data may provide clues regarding disease severity or subtype, more specific analyses must be conducted to draw further conclusions. Second, the activities of circulating proteins are diverse and complex, especially regarding their effects on varied cell types in different tissue contexts. Moreover, protein-protein interaction plays an important role in regulating neurological diseases [8], and these could be further studied to help identify proteins that could be repurposed to target the associations we have identified to prevent or treat disease. Third, although we have made efforts to ensure the quality of the included genetic variants in the analysis, pleotropic effects could not be entirely avoided in our analysis of several possible AD-associated proteins. Pleotropic effects could be appropriately addressed if data were analyzed on individual level. Fourth, we used a relatively relaxed threshold (p = 1×10-5) in the selection of SNP to include more protein targets, but more studies should be performed to confirm these links using GWAS with larger sample sizes. Fifth, despite participants in the selected GWAS were all of European ancestry, the possibility of residual confounding from other variables cannot be completely discounted, such as genetic (APOE) and social determinants of health (area level deprivation, access to healthcare). In addition, null findings were observed in the analysis of some disorders; however, MR findings assume a lifetime effect to risk factors and may overestimate the effects on the outcome. Therefore, our findings cannot be assumed to suggest that no suitable targets exist for these diseases. Moreover, some effect values in our study are very small, which may limit their use in practice and require further research. Last, datasets in the study were mostly collected from cohorts of patients of European descent, and it must be noted that protein-disease associations may vary among different ethnic groups. Our findings should therefore be interpreted with caution in populations of non-European ancestry.
CONCLUSION
Our findings identify five candidate protein targets for AD, MS, epilepsy, ischemic stroke, and migraine. Future studies are warranted to clarify the potential mechanisms of these proteins in the associated diseases.
ACKNOWLEDGMENTS
Summary-level data for the genetic associations with plasma proteins, Alzheimer’s disease, Parkinson’s disease, amyotrophic lateral sclerosis, multiple sclerosis, epilepsy, ischemic stroke, and migraine were obtained from GWAS respectively by Sun et al., Kunkle et al., Nalls et al., Nicolas et al., Patsopoulos et al., Abou-Khalil et al., Malik et al., and Hautakangas et al. The intracranial hemorrhage and subarachnoid hemorrhage were from FinnGen consortium. We thank all investigators for sharing the genome-wide summary statistics.
FUNDING
National Natural Science Foundation of China (82220108009, 81970996), National Key R&D Program of China (2022YFC3602600), STI2030-Major Projects (2021ZD0201801), Science and Technology Project of Guangdong Province (2019B030316001), and Guangzhou Municipal Key Discipline in Medicine (2021–2023).
CONFLICT OF INTEREST
The authors have no conflict of interest to report.
DATA AVAILABILITY
All data used here were confined to the GWAS described above and were available from the public domain or cited study authors.
SUPPLEMENTARY MATERIAL
[1] The supplementary material is available in the electronic version of this article: https://dx.doi.org/10.3233/ADR-230058.
REFERENCES
[1] | Feigin VL , Vos T , Nichols E , Owolabi MO , Carroll WM , Dichgans M , Deuschl G , Parmar P , Brainin M , Murray C ((2020) ) The global burden of neurological disorders: Translating evidence into policy. Lancet Neurol 19: , 255–265. |
[2] | ((2017) ) Global, regional, and national burden of neurological disorders during 1990-2015: A systematic analysis for the Global Burden of Disease Study 2015. Lancet Neurol 16: , 877–897. |
[3] | ((2019) ) Global, regional, and national burden of neurological disorders, 1990-2016: A systematic analysis for the Global Burden of Disease Study 2016. Lancet Neurol 18: , 459–480. |
[4] | Dorsey ER , Sherer T , Okun MS , Bloem BR ((2018) ) The emerging evidence of the Parkinson pandemic. J Parkinsons Dis 8: , S3–S8. |
[5] | Feigin VL , Brainin M , Norrving B , Martins S , Sacco RL , Hacke W , Fisher M , Pandian J , Lindsay P ((2022) ) World Stroke Organization (WSO): Global Stroke Fact Sheet 2022. Int J Stroke 17: , 18–29. |
[6] | Xu L , Liu T , Liu L , Yao X , Chen L , Fan D , Zhan S , Wang S ((2020) ) Global variation in prevalence and incidence of amyotrophic lateral sclerosis: A systematic review and meta-analysis. J Neurol 267: , 944–953. |
[7] | O’Connell GC , Alder ML , Smothers CG , Chang JHC ((2020) ) Large-scale informatic analysis to algorithmically identify blood biomarkers of neurological damage. Proc Natl Acad Sci U S A 117: , 20764–20775. |
[8] | Emilsson V , Ilkov M , Lamb JR , Finkel N , Gudmundsson EF , Pitts R , Hoover H , Gudmundsdottir V , Horman SR , Aspelund T , Shu L , Trifonov V , Sigurdsson S , Manolescu A , Zhu J , Olafsson Ö , Jakobsdottir J , Lesley SA , To J , Zhang J , Harris TB , Launer LJ , Zhang B , Eiriksdottir G , Yang X , Orth AP , Jennings LL , Gudnason V ((2018) ) Co-regulatory networks of human serum proteins link genetics to disease. Science 361: , 769–773. |
[9] | Suhre K , McCarthy MI , Schwenk JM ((2021) ) Genetics meets proteomics: Perspectives for large population-based studies. Nat Rev Genet 22: , 19–37. |
[10] | Emdin CA , Khera AV , Kathiresan S ((2017) ) Mendelian randomization. JAMA 318: , 1925–1926. |
[11] | Chong M , Sjaarda J , Pigeyre M , Mohammadi-Shemirani P , Lali R , Shoamanesh A , Gerstein HC , Paré G ((2019) ) Novel drug targets forischemic stroke identified through Mendelian randomization analysisof the blood proteome. Circulation 140: , 819–830. |
[12] | Yang J , He X , Qian L , Zhao B , Fan Y , Gao F , Yan B , Zhu F , Ma X ((2022) ) Association between plasma proteome and childhood neurodevelopmental disorders: A two-sample Mendelian randomization analysis. EBioMedicine 78: , 103948. |
[13] | Skrivankova VW , Richmond RC , Woolf BAR , Yarmolinsky J , Davies NM , Swanson SA , VanderWeele TJ , Higgins JPT , Timpson NJ , Dimou N , Langenberg C , Golub RM , Loder EW , Gallo V , Tybjaerg-Hansen A , Davey Smith G , Egger M , Richards JB ((2021) ) Strengthening the Reporting of Observational Studies in Epidemiology Using Mendelian Randomization: The STROBE-MR Statement. JAMA 326: , 1614–1621. |
[14] | Sun BB , Maranville JC , Peters JE , Stacey D , Staley JR , Blackshaw J , Burgess S , Jiang T , Paige E , Surendran P , Oliver-Williams C , Kamat MA , Prins BP , Wilcox SK , Zimmerman ES , Chi A , Bansal N , Spain SL , Wood AM , Morrell NW , Bradley JR , Janjic N , Roberts DJ , Ouwehand WH , Todd JA , Soranzo N , Suhre K , Paul DS , Fox CS , Plenge RM , Danesh J , Runz H , Butterworth AS ((2018) ) Genomic atlas of the human plasma proteome. Nature 558: , 73–79. |
[15] | Kunkle BW , Grenier-Boley B , Sims R , Bis JC , Damotte V , Naj AC , Boland A , Vronskaya M , van der Lee SJ , Amlie-Wolf A , et al. ((2019) ) Genetic meta-analysis of diagnosed Alzheimer’s disease identifies new risk loci and implicates Aβ, tau, immunity and lipid processing. Nat Genet 51: , 414–430. |
[16] | Nalls MA , Blauwendraat C , Vallerga CL , Heilbron K , Bandres-Ciga S , Chang D , Tan M , Kia DA , Noyce AJ , Xue A , Bras J , Young E , von Coelln R , Simón-Sánchez J , Schulte C , Sharma M , Krohn L , Pihlstrøm L , Siitonen A , Iwaki H , Leonard H , Faghri F , Gibbs JR , Hernandez DG , Scholz SW , Botia JA , Martinez M , Corvol JC , Lesage S , Jankovic J , Shulman LM , Sutherland M , Tienari P , Majamaa K , Toft M , Andreassen OA , Bangale T , Brice A , Yang J , Gan-Or Z , Gasser T , Heutink P , Shulman JM , Wood NW , Hinds DA , Hardy JA , Morris HR , Gratten J , Visscher PM , Graham RR , Singleton AB ((2019) ) Identification of novel risk loci, causal insights, and heritablerisk for Parkinson’s disease: A meta-analysis of genome-wideassociation studies. Lancet Neurol 18: , 1091–1102. |
[17] | Nicolas A , Kenna KP , Renton AE , Ticozzi N , Faghri F , Chia R , Dominov JA , Kenna BJ , Nalls MA , Keagle P , et al. ((2018) ) Genome-wide analyses identify KIF5A as a novel ALS gene. Neuron 97: , 1268–1283.e1266. |
[18] | International Multiple Sclerosis Genetics Consortium ((2019) ) Multiple sclerosis genomic map implicates peripheral immune cells and microglia in susceptibility. Science 365: , eaav7188. |
[19] | International League Against Epilepsy Consortium on Complex Epilepsies ((2018) ) Genome-wide mega-analysis identifies 16 loci and highlights diverse biological mechanisms in the common epilepsies. Nat Commun 9: , 5269. |
[20] | Malik R , Chauhan G , Traylor M , Sargurupremraj M , Okada Y , Mishra A , Rutten-Jacobs L , Giese AK , van der Laan SW , Gretarsdottir S , Anderson CD , Chong M , Adams HHH , Ago T , Almgren P , Amouyel P , Ay H , Bartz TM , Benavente OR , Bevan S , Boncoraglio GB , Brown RD Jr., Butterworth AS , Carrera C , Carty CL , Chasman DI , Chen WM , Cole JW , Correa A , Cotlarciuc I , Cruchaga C , Danesh J , de Bakker PIW , DeStefano AL , den Hoed M , Duan Q , Engelter ST , Falcone GJ , Gottesman RF , Grewal RP , Gudnason V , Gustafsson S , Haessler J , Harris TB , Hassan A , Havulinna AS , Heckbert SR , Holliday EG , Howard G , Hsu FC , Hyacinth HI , Ikram MA , Ingelsson E , Irvin MR , Jian X , Jiménez-Conde J , Johnson JA , Jukema JW , Kanai M , Keene KL , Kissela BM , Kleindorfer DO , Kooperberg C , Kubo M , Lange LA , Langefeld CD , Langenberg C , Launer LJ , Lee JM , Lemmens R , Leys D , Lewis CM , Lin WY , Lindgren AG , Lorentzen E , Magnusson PK , Maguire J , Manichaikul A , McArdle PF , Meschia JF , Mitchell BD , Mosley TH , Nalls MA , Ninomiya T , O’Donnell MJ , Psaty BM , Pulit SL , Rannikmäe K , Reiner AP , Rexrode KM , Rice K , Rich SS , Ridker PM , Rost NS , Rothwell PM , Rotter JI , Rundek T , Sacco RL , Sakaue S , Sale MM , Salomaa V , Sapkota BR , Schmidt R , Schmidt CO , Schminke U , Sharma P , Slowik A , Sudlow CLM , Tanislav C , Tatlisumak T , Taylor KD , Thijs VNS , Thorleifsson G , Thorsteinsdottir U , Tiedt S , Trompet S , Tzourio C , van Duijn CM , Walters M , Wareham NJ , Wassertheil-Smoller S , Wilson JG , Wiggins KL , Yang Q , Yusuf S , Bis JC , Pastinen T , Ruusalepp A , Schadt EE , Koplev S , Björkegren JLM , Codoni V , Civelek M , Smith NL , Trégouët DA , Christophersen IE , Roselli C , Lubitz SA , Ellinor PT , Tai ES , Kooner JS , Kato N , He J , van der Harst P , Elliott P , Chambers JC , Takeuchi F , Johnson AD , Sanghera DK , Melander O , Jern C , Strbian D , Fernandez-Cadenas I , Longstreth WT Jr., Rolfs A , Hata J , Woo D , Rosand J , Pare G , Hopewell JC , Saleheen D , Stefansson K , Worrall BB , Kittner SJ , Seshadri S , Fornage M , Markus HS , Howson JMM , Kamatani Y , Debette S , Dichgans M ((2018) ) Multiancestry genome-wide association study of 520,000 subjectsidentifies 32 loci associated with stroke and stroke subtypes. Nat Genet 50: , 524–537. |
[21] | Hautakangas H , Winsvold BS , Ruotsalainen SE , Bjornsdottir G , Harder AVE , Kogelman LJA , Thomas LF , Noordam R , Benner C , Gormley P , Artto V , Banasik K , Bjornsdottir A , Boomsma DI , Brumpton BM , Burgdorf KS , Buring JE , Chalmer MA , de Boer I , Dichgans M , Erikstrup C , Färkkilä M , Garbrielsen ME , Ghanbari M , Hagen K , Häppölä P , Hottenga JJ , Hrafnsdottir MG , Hveem K , Johnsen MB , Kähönen M , Kristoffersen ES , Kurth T , Lehtimäki T , Lighart L , Magnusson SH , Malik R , Pedersen OB , Pelzer N , Penninx B , Ran C , Ridker PM , Rosendaal FR , Sigurdardottir GR , Skogholt AH , Sveinsson OA , Thorgeirsson TE , Ullum H , Vijfhuizen LS , Widén E , van Dijk KW , Aromaa A , Belin AC , Freilinger T , Ikram MA , Järvelin MR , Raitakari OT , Terwindt GM , Kallela M , Wessman M , Olesen J , Chasman DI , Nyholt DR , Stefánsson H , Stefansson K , van den Maagdenberg A , Hansen TF , Ripatti S , Zwart JA , Palotie A , Pirinen M ((2022) ) Genome-wide analysis of 102,084 migrainecases identifies 123 risk loci and subtype-specific risk alleles. Nat Genet 54: , 152–160. |
[22] | Sanna S , van Zuydam NR , Mahajan A , Kurilshikov A , Vich Vila A , Võsa U , Mujagic Z , Masclee AAM , Jonkers D , Oosting M , Joosten LAB , Netea MG , Franke L , Zhernakova A , Fu J , Wijmenga C , McCarthy MI ((2019) ) Causal relationships among the gut microbiome, short-chainfatty acids and metabolic diseases. Nat Genet 51: , 600–605. |
[23] | Shi X , Wei T , Hu Y , Wang M , Tang Y ((2022) ) The associations between plasma soluble Trem1 and neurological diseases: A Mendelian randomization study. J Neuroinflammation 19: , 218. |
[24] | Auton A , Brooks LD , Durbin RM , Garrison EP , Kang HM , Korbel JO , Marchini JL , McCarthy S , McVean GA , Abecasis GR ((2015) ) A global reference for human genetic variation. Nature 526: , 68–74. |
[25] | Burgess S , Butterworth A , Thompson SG ((2013) ) Mendelian randomization analysis with multiple genetic variants using summarized data. Genet Epidemiol 37: , 658–665. |
[26] | Burgess S , Davey Smith G , Davies NM , Dudbridge F , Gill D , Glymour MM , Hartwig FP , Holmes MV , Minelli C , Relton CL , Theodoratou E ((2019) ) Guidelines for performing Mendelian randomization investigations. Wellcome Open Res 4: , 186. |
[27] | Hartwig FP , Davey Smith G , Bowden J ((2017) ) Robust inference in summary data Mendelian randomization via the zero modal pleiotropy assumption. Int J Epidemiol 46: , 1985–1998. |
[28] | Bowden J , Davey Smith G , Haycock PC , Burgess S ((2016) ) Consistent estimation in Mendelian randomization with some invalid instruments using a weighted median estimator. Genet Epidemiol 40: , 304–314. |
[29] | Bowden J , Davey Smith G , Burgess S ((2015) ) Mendelian randomization with invalid instruments: Effect estimation and bias detection through Egger regression. Int J Epidemiol 44: , 512–525. |
[30] | Bowden J , Del Greco MF , Minelli C , Davey Smith G , Sheehan N , Thompson J ((2017) ) A framework for the investigation of pleiotropy in two-sample summary data Mendelian randomization. Stat Med 36: , 1783–1802. |
[31] | Bowden J , Del Greco MF , Minelli C , Zhao Q , Lawlor DA , Sheehan NA , Thompson J , Davey Smith G ((2019) ) Improving the accuracy of two-sample summary-data Mendelian randomization: Moving beyond the NOME assumption. Int J Epidemiol 48: , 728–742. |
[32] | Verbanck M , Chen CY , Neale B , Do R ((2018) ) Detection of widespread horizontal pleiotropy in causal relationships inferred from Mendelian randomization between complex traits and diseases. Nat Genet 50: , 693–698. |
[33] | Hemani G , Bowden J , Davey Smith G ((2018) ) Evaluating the potential role of pleiotropy in Mendelian randomization studies. Hum Mol Genet 27: , R195–r208. |
[34] | Hemani G , Zheng J , Elsworth B , Wade KH , Haberland V , Baird D , Laurin C , Burgess S , Bowden J , Langdon R , Tan VY , Yarmolinsky J , Shihab HA , Timpson NJ , Evans DM , Relton C , Martin RM , Davey Smith G , Gaunt TR , Haycock PC ((2018) ) The MR-Base platform supports systematic causal inference across the human phenome. Elife 7: , e34408. |
[35] | Brion MJ , Shakhbazov K , Visscher PM ((2013) ) Calculating statistical power in Mendelian randomization studies. Int J Epidemiol 42: , 1497–1501. |
[36] | Staley JR , Blackshaw J , Kamat MA , Ellis S , Surendran P , Sun BB , Paul DS , Freitag D , Burgess S , Danesh J , Young R , Butterworth AS ((2016) ) PhenoScanner: A database of human genotype-phenotype associations. Bioinformatics 32: , 3207–3209. |
[37] | Eskandari-Sedighi G , Jung J , Macauley MS ((2023) ) CD33 isoforms in microglia and Alzheimer’s disease: Friend and foe. Mol Aspects Med 90: , 101111. |
[38] | Karch CM , Jeng AT , Nowotny P , Cady J , Cruchaga C , Goate AM ((2012) ) Expression of novel Alzheimer’s disease risk genes in control and Alzheimer’s disease brains. PLoS One 7: , e50976. |
[39] | Bradshaw EM , Chibnik LB , Keenan BT , Ottoboni L , Raj T , Tang A , Rosenkrantz LL , Imboywa S , Lee M , Von Korff A , Morris MC , Evans DA , Johnson K , Sperling RA , Schneider JA , Bennett DA , De Jager PL ((2013) ) CD33 Alzheimer’s disease locus: Altered monocyte function and amyloid biology. Nat Neurosci 16: , 848–850. |
[40] | Gu X , Dou M , Cao B , Jiang Z , Chen Y ((2022) ) Peripheral level of CD33 and Alzheimer’s disease: A bidirectional two-sample Mendelian randomization study. Transl Psychiatry 12: , 427. |
[41] | Nomura S , Tandon NN , Nakamura T , Cone J , Fukuhara S , Kambayashi J ((2001) ) High-shear-stress-induced activation of platelets and microparticles enhances expression of cell adhesion molecules in THP-1 and endothelial cells. Atherosclerosis 158: , 277–287. |
[42] | Ramsborg CG , Papoutsakis ET ((2007) ) Global transcriptional analysis delineates the differential inflammatory response interleukin-15 elicits from cultured human T cells. Exp Hematol 35: , 454–464. |
[43] | Bernard V , Young J , Binart N ((2019) ) Prolactin - a pleiotropic factor in health and disease. Nat Rev Endocrinol 15: , 356–365. |
[44] | Wang YQ , Wen Y , Wang MM , Zhang YW , Fang ZX ((2021) ) Prolactin levels as a criterion to differentiate between psychogenic non-epileptic seizures and epileptic seizures: A systematic review. Epilepsy Res 169: , 106508. |
[45] | Meierkord H , Shorvon S , Lightman S , Trimble M ((1992) ) Comparison ofthe effects of frontal and temporal lobe partial seizures onprolactin levels. Arch Neurol 49: , 225–230. |
[46] | Chen DK , So YT , Fisher RS ((2005) ) Use of serum prolactin indiagnosing epileptic seizures: Report of the Therapeutics andTechnology Assessment Subcommittee of the American Academy ofNeurology. Neurology 65: , 668–675. |
[47] | Kirk SE , Xie TY , Steyn FJ , Grattan DR , Bunn SJ ((2017) ) Restraintstress increases prolactin-mediated phosphorylation of signaltransducer and activator of transcription 5 in the hypothalamus andadrenal cortex in the male mouse. J Neuroendocrinol 29: , doi: 10.1111/jne.12477. |
[48] | Grattan DR ((2015) ) 60 years of neuroendocrinology: The hypothalamo-prolactin axis. J Endocrinol 226: , T101–122. |
[49] | Osuga H , Osuga S , Wang F , Fetni R , Hogan MJ , Slack RS , Hakim AM , Ikeda JE , Park DS ((2000) ) Cyclin-dependent kinases as a therapeutic target for stroke. Proc Natl Acad Sci U S A 97: , 10254–10259. |
[50] | Wang F , O’Hare MJ , Park DS ((2001) ) Cyclin-dependent kinases and stroke. Expert Opin Ther Targets 5: , 557–567. |
[51] | Szilagyi Z , Gustafsson CM ((2013) ) Emerging roles of Cdk8 in cell cycle control. Biochim Biophys Acta 1829: , 916–920. |
[52] | Friedrichs P , Schlotterer A , Sticht C , Kolibabka M , Wohlfart P , Dietrich A , Linn T , Molema G , Hammes HP ((2017) ) Hyperglycaemic memory affects the neurovascular unit of the retina in a diabetic mouse model. Diabetologia 60: , 1354–1358. |
[53] | Zhao X , Feng D , Wang Q , Abdulla A , Xie XJ , Zhou J , Sun Y , Yang ES , Liu LP , Vaitheesvaran B , Bridges L , Kurland IJ , Strich R , Ni JQ , Wang C , Ericsson J , Pessin JE , Ji JY , Yang F ((2012) ) Regulation of lipogenesis by cyclin-dependent kinase 8-mediated control of SREBP-1. J Clin Invest 122: , 2417–2427. |
[54] | Shoemaker MB , Husser D , Roselli C , Al Jazairi M , Chrispin J , Kühne M , Neumann B , Knight S , Sun H , Mohanty S , Shaffer C , Thériault S , Rinke LL , Siland JE , Crawford DM , Ueberham L , Zardkoohi O , Büttner P , Geelhoed B , Blum S , Aeschbacher S , Smith JD , Van Wagoner DR , Freudling R , Müller-Nurasyid M , Montgomery J , Yoneda Z , Wells Q , Issa T , Weeke P , Jacobs V , Van Gelder IC , Hindricks G , Barnard J , Calkins H , Darbar D , Michaud G , Kääb S , Ellinor P , Natale A , Chung M , Nazarian S , Cutler MJ , Sinner MF , Conen D , Rienstra M , Bollmann A , Roden DM , Lubitz S ((2020) ) Geneticsusceptibility for atrial fibrillation in patients undergoing atrialfibrillation ablation. Circ Arrhythm Electrophysiol 13: , e007676. |
[55] | Sinner MF , Tucker NR , Lunetta KL , Ozaki K , Smith JG , Trompet S , Bis JC , Lin H , Chung MK , Nielsen JB , Lubitz SA , Krijthe BP , Magnani JW , Ye J , Gollob MH , Tsunoda T , Müller-Nurasyid M , Lichtner P , Peters A , Dolmatova E , Kubo M , Smith JD , Psaty BM , Smith NL , Jukema JW , Chasman DI , Albert CM , Ebana Y , Furukawa T , Macfarlane PW , Harris TB , Darbar D , Dörr M , Holst AG , Svendsen JH , Hofman A , Uitterlinden AG , Gudnason V , Isobe M , Malik R , Dichgans M , Rosand J , Van Wagoner DR , Benjamin EJ , Milan DJ , Melander O , Heckbert SR , Ford I , Liu Y , Barnard J , Olesen MS , Stricker BH , Tanaka T , Kääb S , Ellinor PT ((2014) ) Integrating genetic, transcriptional, and functional analyses to identify 5 novel genes for atrial fibrillation. Circulation 130: , 1225–1235. |
[56] | Pavlopoulos E , Trifilieff P , Chevaleyre V , Fioriti L , Zairis S , Pagano A , Malleret G , Kandel ER ((2011) ) Neuralized1 activates CPEB3: A function for nonproteolytic ubiquitin in synaptic plasticity and memory storage. Cell 147: , 1369–1383. |
[57] | Ehrhardt GR , Leu CM , Zhang S , Aksu G , Jackson T , Haga C , Hsu JT , Schreeder DM , Davis RS , Cooper MD ((2007) ) Fc receptor-like proteins (FCRL): Immunomodulators of B cell function. Adv Exp Med Biol 596: , 155–162. |
[58] | Ehrhardt GR , Hijikata A , Kitamura H , Ohara O , Wang JY , Cooper MD ((2008) ) Discriminating gene expression profiles of memory B cell subpopulations. J Exp Med 205: , 1807–1817. |
[59] | Li H , Dement-Brown J , Liao PJ , Mazo I , Mills F , Kraus Z , Fitzsimmons S , Tolnay M ((2020) ) Fc receptor-like 4 and 5 define human atypical memory B cells. Int Immunol 32: , 755–770. |
[60] | Lassmann H ((2013) ) Pathology and disease mechanisms in different stages of multiple sclerosis. J Neurol Sci 333: , 1–4. |