Racial/Ethnic Disparities in the Alzheimer’s Disease Link with Cardio and Cerebrovascular Diseases, Based on Hawaii Medicare Data
Abstract
Background:
There is an expanding body of literature implicating heart disease and stroke as risk factors for Alzheimer’s disease (AD). Hawaii is one of the six majority-minority states in the United States and has significant racial health disparities. The Native-Hawaiians/Pacific-Islander (NHPI) population is well-known as a high-risk group for a variety of disease conditions.
Objective:
We explored the association of cardiovascular disease with AD development based on the Hawaii Medicare data, focusing on racial disparities.
Methods:
We utilized nine years of Hawaii Medicare data to identify subjects who developed heart failure (HF), ischemic heart disease (IHD), atrial fibrillation (AF), acute myocardial infarction (AMI), stroke, and progressed to AD, using multistate models. Propensity score-matched controls without cardiovascular disease were identified to compare the risk of AD after heart disease and stroke. Racial/Ethnic differences in progression to AD were evaluated, accounting for other risk factors.
Results:
We found increased risks of AD for AF, HF, IHD, and stroke. Socioeconomic (SE) status was found to be critical to AD risk. Among the low SE group, increased AD risks were found in NHPIs compared to Asians for all conditions selected and compared to whites for HF, IHD, and stroke. Interestingly, these observations were found reversed in the higher SE group, showing reduced AD risks for NHPIs compared to whites for AF, HF, and IHD, and to Asians for HF and IHD.
Conclusions:
NHPIs with poor SE status seems to be mostly disadvantaged by the heart/stroke and AD association compared to corresponding whites and Asians.
INTRODUCTION
Alzheimer’s disease (AD) is a progressive neurodegenerative disorder that causes a decline in brain function and overall cognitive ability. This impairment affects multiple cognitive domains, resulting in memory loss, language abnormalities, personality changes, and difficulties with judgment [1]. As people are living longer, AD is becoming an increasingly significant burden on society due to its prevalence and its direct and indirect impact on the economy. In the United States (US), it is estimated that the cost of healthcare, including long-term care and hospice services for individuals aged 65 and older with AD, was $305 billion in 2020. This cost is projected to increase to over $1.1 trillion by 2050 (in 2020 dollars) [2].
Cardiovascular disease is a growing concern among elderly individuals aged 65 and above, as vascular insufficiency can potentially impair the function of other organs, including the brain. Research suggests that cardiovascular disease may be a risk factor for AD, with significant anatomical and physiological pathways implicated [3–5]. Heart failure, for example, has been found to contribute to cognitive decline, with the severity of cognitive impairment linked to the severity of heart failure [6]. The Framingham Heart Study has revealed an association between reduced cardiac index and left ventricular ejection fraction with impaired cognition [7]. Studies have also shown that approximately 40–47% of patients hospitalized for heart failure demonstrate some degree of cognitive decline [8, 9]. Recent neuroimaging research has further supported the link between heart and brain dysfunction, with structural brain abnormalities observed after heart failure [10, 11]. In addition, there is growing evidence of a mechanistic association between chronic heart failure (HF), ischemic heart disease (IHD), atrial fibrillation (AF), acute myocardial infarction (AMI), and the development of AD [3, 12]. Cardiovascular risk factors, such as hypertension, diabetes, obesity, hyperhomocystinemia, hyperlipidemia, and smoking, have also been independently associated with the development of AD [3, 12]. Stroke, particularly ischemic stroke, can also lead to biochemical dysfunction and structural damage in the brain, potentially leading to dementia [13]. In fact, up to one-third of elderly patients with stroke develop dementia within three years of the stroke event [13]. Further research is needed to better understand the epidemiological features of the association between heart disease, stroke, and the development of AD. Research also suggests that social and economic factors, such as income level, educational attainment, employment status, access to healthcare, access to healthy food, and neighborhood characteristics, can contribute to a person’s risk for developing diseases such as cardiovascular disease and AD [14–16]. By identifying populations that may be at higher risk and developing targeted interventions to improve health outcomes and reduce disparities, we can potentially mitigate the impact of these diseases on society.
According to the 2020 census, Hawaii has the most diverse ethnic population in the US, with the largest diversity index [17]. Prior epidemiological studies conducted in Hawaii have identified significant racial/ethnic health disparities, highlighting disadvantaged groups in various aspects [18–21]. Notably, the Native Hawaiian and Pacific Islander (NHPI) population has been recognized as a high-risk group for multiple conditions, including cardiovascular disease [18, 19]. Compared to whites, NHPIs report a 1.7 times higher age-adjusted rate of heart disease and a three-fold higher risk of heart failure, as well as an increased risk of ischemic stroke at a younger age [19–21]. Additionally, NHPIs are disproportionately affected by other chronic conditions, such as diabetes and chronic kidney disease [22, 23]. Given that heart conditions and stroke events are known to increase the risk of developing AD, it is likely that NHPIs are also at a higher risk of developing AD, particularly after experiencing a cardiovascular event. In Hawaii, AD is the sixth leading cause of death, with a 258% increase in AD-related deaths since 2000 [24]. Therefore, it is crucial for research studies to examine potential racial and ethnic disparities in AD, especially those linked to cardiovascular disease, to improve our understanding of its epidemiological features.
In this study, we aimed to explore the link between AD and major cardiovascular events, including AF, AMI, HF, IHD, or stroke, in subjects aged 65 and above. We also sought to examine potential racial/ethnic differences in this link. To our knowledge, no prior studies have been conducted in the Hawaii population to assess such associations, nor have there been comprehensive studies done in the US using large longitudinal follow-up datasets that focus on racial and ethnic differences in the progressive dependencies of AD on these conditions. Collecting longitudinal data containing the health status of a large cohort of subjects over a long- and continuous-time frame is generally challenging. However, health insurance databases such as Medicare datasets provide rich sources that offer a natural basis for mining vital information on diseases and their interrelationships. For this study, we used nine years of Hawaii Medicare data to capture vital time-to-event details. We aimed to evaluate the risk of developing AD after observing heart disease and stroke and to assess racial/ethnic differences in developing AD based on these conditions. In this study, we aimed 1) evaluating the risk of developing AD after observing heart disease and stroke, and 2) evaluating racial/ethnic differences in developing AD, based on heart/stroke conditions. To address the research problem, we used the multistate model approach, which turned the problem into a state-transition problem. In this framework, subjects can move among a set of states over time, which allowed us to efficiently handle state transitions and better understand the dynamics of the problem.
MATERIALS AND METHODS
Data
For our study, we conducted a retrospective cohort analysis of the Hawaii Medicare database, which spans nine years (2009–2017) and includes 369,131 individual records and 3.27 million insurance claims. Specifically, we focused on the elderly Medicare population aged 65 years and above, and identified subjects who had experienced AF, AMI, HF, IHD, or stroke conditions between January 1, 2009, and December 31, 2017. We followed these subjects longitudinally for up to nine years to systematically track the occurrence of AD and death and collected demographic data and information on other co-morbidities as covariates. It should be noted that the follow-up lengths of the selected cohort varied. To avoid systematic bias in a time-to-event analytical setting, we excluded individuals with a history of AF, AMI, HF, IHD, stroke, and AD conditions prior to January 1, 2009. We utilized data on both inpatient and outpatient visits and used the International Classification of Diseases, 9th and 10th Revisions (ICD-9/ICD-10) diagnosis codes, along with subject disease history from Medicare beneficiary summary files, to identify disease conditions. Supplementary material (Supplementary Table 1) provides specific ICD codes used to identify conditions such as AD, AF, AMI, HF, IHD, and stroke. Race/ethnicity was categorized as white, Asian, Native Hawaiian, Pacific Islander (NHPI), and other groups, which included American Indian/Alaska Native, African American, Hispanic, and unknown races. We used dual eligibility (DE) status, which indicates an individual who received both Medicare and Medicaid benefits, as a proxy for socioeconomic status since it is common among individuals with lower income [25–27]. The Institutional Review Board at the University of Hawaii (CHS #23362) approved the research protocol.
Multistate model
Multistate models are survival models used in epidemiological research to track the progression of a disease or sequence of conditions/events. They show possible movements of an individual in a set of successive states from an initial state, while accounting for possible competing risks. These models utilize state occupation probabilities and hazard rate functions, which are important temporal functions. However, right-censoring due to partial observations is a significant issue when dealing with time-to-event data, which restricts the utilization of common statistical methods that are limited to fully observed data and can introduce selection bias. In this study, we used a three-state acyclic illness death multistate model shown in Figs. 1 and 2 to address a set of research questions, centered around the progression to AD. We aimed to 1) investigate the risk of progression from heart disease (i.e., AF, AMI, HF, IHD) and stroke conditions to AD (Fig. 1) and 2) investigate racial-ethnic disparities in heart/stroke and AD links highlighted under aim 1 (Fig. 2). Separate models were used for AF, AMI, HF, IHD, and stroke conditions, as appropriate. For example, to examine the risk of developing AD after AF, the model given in Fig. 1 was used with the initial event as “AF”. In the system, there is one clinical state among two that an individual can occupy at a given time point, whereas the final state is the death state (absorbing state). An individual starts from the initial disease state (i.e., AF, AMI, HF, IHD, or stroke) and moves towards the absorbing state of death either directly or through the intermediate state. For example, Fig. 1 shows the progression to AD for an individual with and without observing a condition such as AF, with no history of AD or other conditions: AMI, HF, IHD, and stroke. The basic structure of these models provides the acyclic property that highlights the irreversible transitions. In particular, we present the twin illness death model corresponding to cases and controls under each scenario in Fig. 1. Note that more details of the control group are described in the next section. To analyze a particular model, we first identified a set of subjects who made a claim for the condition between 2009 and 2017 for the first time. We excluded those who had a history of AD, AF, AMI, HF, IHD, or stroke conditions. For each individual, we determined the earliest date of the first disease occurrence and then tracked all subsequent states and corresponding state transition times based on the claims data.
Fig. 1
Progression to AD, followed by the illness and death model. In the figure, the initial event is varying from AF, AMI, HF, IHD, and stroke, generating five different models. The control group corresponds to a set without the initial event of interest (i.e., AF, AMI, HF, IHD, and stroke).
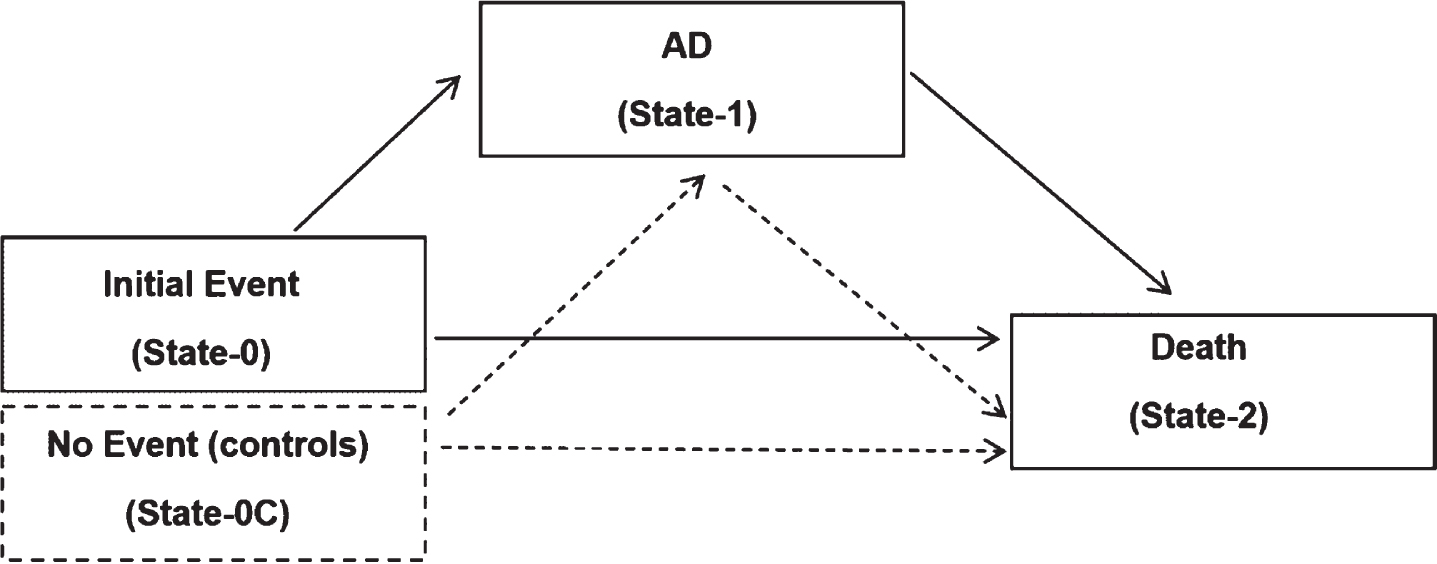
Fig. 2
Progression from cardiovascular disease to AD, followed by the illness and death model. A sub-model of the model given in Fig. 1 only with subjects observed the initial event of interest (i.e., AF, AMI, HF, IHD, and stroke).
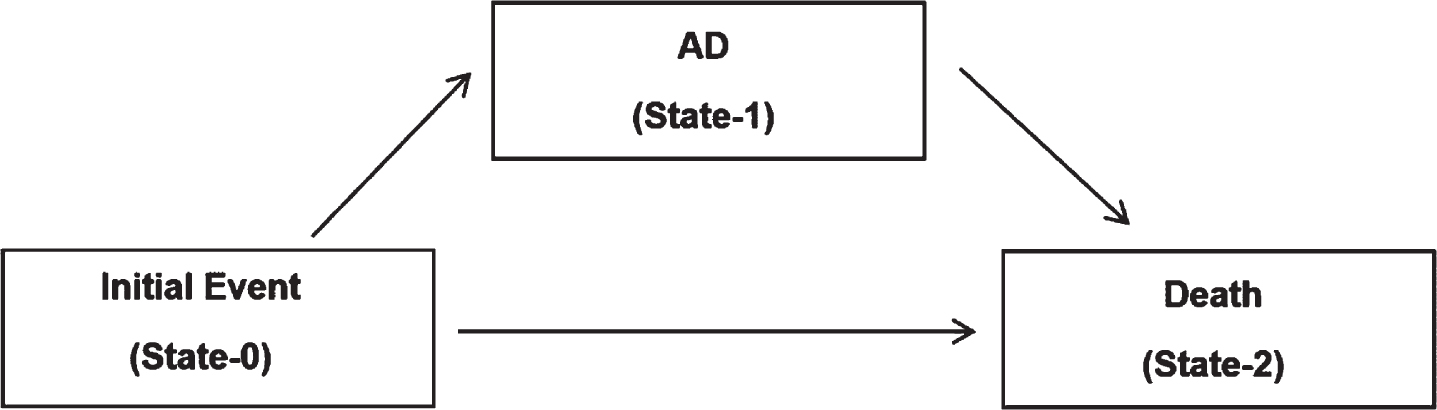
Control group
In this study, under aim 1, we aimed to assess the risk of transitioning to AD after observing heart disease and stroke, compared to a control group of subjects who had not observed the initial condition/event. The following procedure was applied to specify a control group for each model in Fig. 1: a group of subjects who had never experienced any cardiovascular events but were similar to those with heart/stroke conditions (i.e., cases) in terms of their covariate distributions were selected as a control group. The selection step proceeded as follows. First, cases were partitioned based on the age (in years) at the onset of the condition. Let’s denote the set for jth age as Xj. Next, for the age value j, we determined a corresponding same-age sub cohort never experienced AF, AMI, HF, IHD, or stroke events in their lifetimes, indicated by Cj. Let sizes of Xj and Cj sets be
Statistical approach
Statistical techniques for analyzing censored time-to-event data were applied to examine the temporal characteristics and covariate relationships of multistate models used in the study. Subject movements within multistate systems given in Figs. 1 and 2 were tracked over time using Medicare claims ICD9/10 disease codes and corresponding claim dates. We estimated transition hazard functions using the Nelson Aalen hazard rate estimator and used the Aalen-Johansen product limit integral estimator to quantify marginal estimates of state occupation probabilities [29]. The Aalen-Johansen nonparametric techniques are valid even when the restrictive Markov model assumption is not met, offering flexibility in handling problems encountered in real-life situations [30, 31]. Observed transitions were subject to right-censoring due to incomplete follow-up times. The estimation was performed under the assumption that individuals were randomly censored. We calculated 95% point-wise confidence intervals of selected temporal functions using the bootstrap approach with 1,000 bootstrap iterations. To examine covariate effects on temporal functions, we applied the Anderson-Klein pseudo-value regression method, which has been recognized as a flexible, generalizable, and straightforward technique for modeling complex time-to-event data subject to censoring, compared to competing methods [32, 33]. The pseudo-value regression method was initiated with a marginal estimator of the targeted quantity, which could be either parametric or nonparametric, and next utilize the jackknife estimate along with the flexible generalized estimating equations on testing. As propensity score matching was utilized in specifying the control group, all analyses comparing cases and controls were conducted while accounting for the matching, to avoid technical issues in inferencing [34, 35]. Pseudo value approach was applied at a grid of time points ranging from 12 to 96 months. To assess the risk of transitioning from state-0 to state-1 and state-0 to state-2, we focused on the cumulative incidence function whereas state-1 to state-2 was based on the cumulative hazard function. We provide additional details on the statistical approach in the Supplementary Material. Note that a key aspect of this study was to examine the racial/ethnic effects on temporal functions of multistate models. In our approach, we performed these analyses by incorporating the race/ethnicity interaction with the DE status. Note that the DE indicator generally describes low-income beneficiaries enrolled in both Medicare and Medicaid; thus, it serves as a surrogate marker for one’s financial status. We observed that this variable has a critical effect on regulating the racial/ethnic effects on several important transitions, indicating the importance of such interaction. These assessments were performed while accounting for subject age, gender, and multiple disease conditions, including chronic kidney disease, chronic obstructive pulmonary disease, diabetes, depression, osteoporosis, rheumatoid arthritis/osteoarthritis, anemia, asthma, hyperlipidemia, hypertension, and having cancer, under pseudo-regression models. All the analyses were conducted using R version 4.0.1.
RESULTS
Participant characteristics
Supplementary Tables 2–6 provide a summary of the characteristics of the study subjects who experienced initial events of AF, AMI, HF, IHD, and stroke, which correspond to the multistate model shown in Figs. 1 and 2. At the onset of each of these conditions, there were a total of 12,991, 7,856, 10,748, 12,781, and 7,430 subjects, respectively. The average age of these cohorts ranged from 73.5 to 77.1 years, with male subjects comprising 51.2% to 61.1% , and DE subjects comprising 16.3% to 19.5% . In terms of race/ethnicity, the cohorts were composed of whites (27.6% to 34.7% ), Asians (21.5% to 27.9% ), and NIHPIs (21.9% to 24.4% ). Additionally, 25.2% to 41.3% of the subjects had diabetes, 6.5% to 10.8% had depression, 47.0% to 67.67% had hyperlipidemia, and 49.1% to 70.9% had hypertension.
AD risks after heart disease and stroke
Table 1 summarizes the results of our analysis examining the risks of AD for individuals who have experienced heart disease or stroke, as compared to control groups. We used the pseudo-value regression approach to estimate HR for transition probabilities between the two groups. In Table 1, an HR value greater than 1 indicates an increased hazard for the diseased group in the given transition, while an HR value less than 1 indicates a reduced hazard for the diseased group. As described in the methods section, the control group consists of a cohort of disease-free individuals who were matched for covariates. The p-values indicate the statistical significance of the HR, with a null hypothesis that the true parameter HR is equal to 1.
Table 1
Effects of heart disease and stroke on transitions in the multistate model given in Fig. 1
Heart/Stroke to AD | AD to Death | Heart/Stroke to Death | ||||
(State 0 to 1) | (State 1 to 2) | (State 0 to 2) | ||||
HR (95% CI) | p | HR (95% CI) | p | HR (95% CI) | p | |
AF | 1.092(1.045, 1.141) | <0.0001 | 1.369(1.170, 1.601) | <0.0001 | 1.303(1.143, 1.485) | <0.0001 |
AMI | 1.091(0.992, 1.200) | 0.0731 | 1.516(1.240, 1.854) | <0.0001 | 1.394(1.183, 1.643) | <0.0001 |
HF | 1.211(1.100, 1.333) | <0.0001 | 1.794(1.347, 2.39) | <0.0001 | 1.453(1.213, 1.741) | <0.0001 |
IHD | 1.168(1.083, 1.260) | <0.0001 | 1.371(1.138, 1.652) | 0.0009 | 1.150(1.073, 1.232) | <0.0001 |
Stroke | 1.147(1.071, 1.228) | <0.0001 | 1.031(0.856, 1.241) | 0.7474 | 1.122(1.059, 1.188) | <0.0001 |
Estimated hazard ratio (HR) values correspond to the effect of the disease group (i.e., AF, AMI, HF, IHD, and stroke), compared to the control group, by the pseudo value regression approach.
In the multistate model, the transition from state 0 to state 1 indicates the event of diagnosing AD after experiencing AF, AMI, HF, IHD, or stroke. Therefore, one of our primary interests was to understand the relative risks for the diseased compared to the control group in this transition. In Fig. 3, the plots given in the first row show the characteristics of the cumulative hazard functions of the diseased and control groups on this transition. The analysis results in Table 1 (column 1) showed increased hazards for developing AD after observing AF, AMI, HF, IHD, and stroke compared to the control group after following up with subjects for a maximum of 9 years. Except for AMI, all HR values were greater than 1 and statistically significant at a 5% level. The RR values were AF (HR = 1.09), HF (HR = 1.21), IHD (HR = 1.17), and stroke (HR = 1.15). Our assessment of the revealed data showed only borderline significance for the AMI condition (HR = 1.09). In general, the observed risks were modest.
Fig. 3
Estimated transition hazard functions with respect to time, corresponding to multistate model given in Fig. 1, separately by diseased cases: AF, AMI, HF, IHD, and stroke) and controls. Plots given in rows 1, 2, and 3 correspond to state-0 to state-1, state-1 to state-2, and state-0 to state-2, transitions in each of the five models.
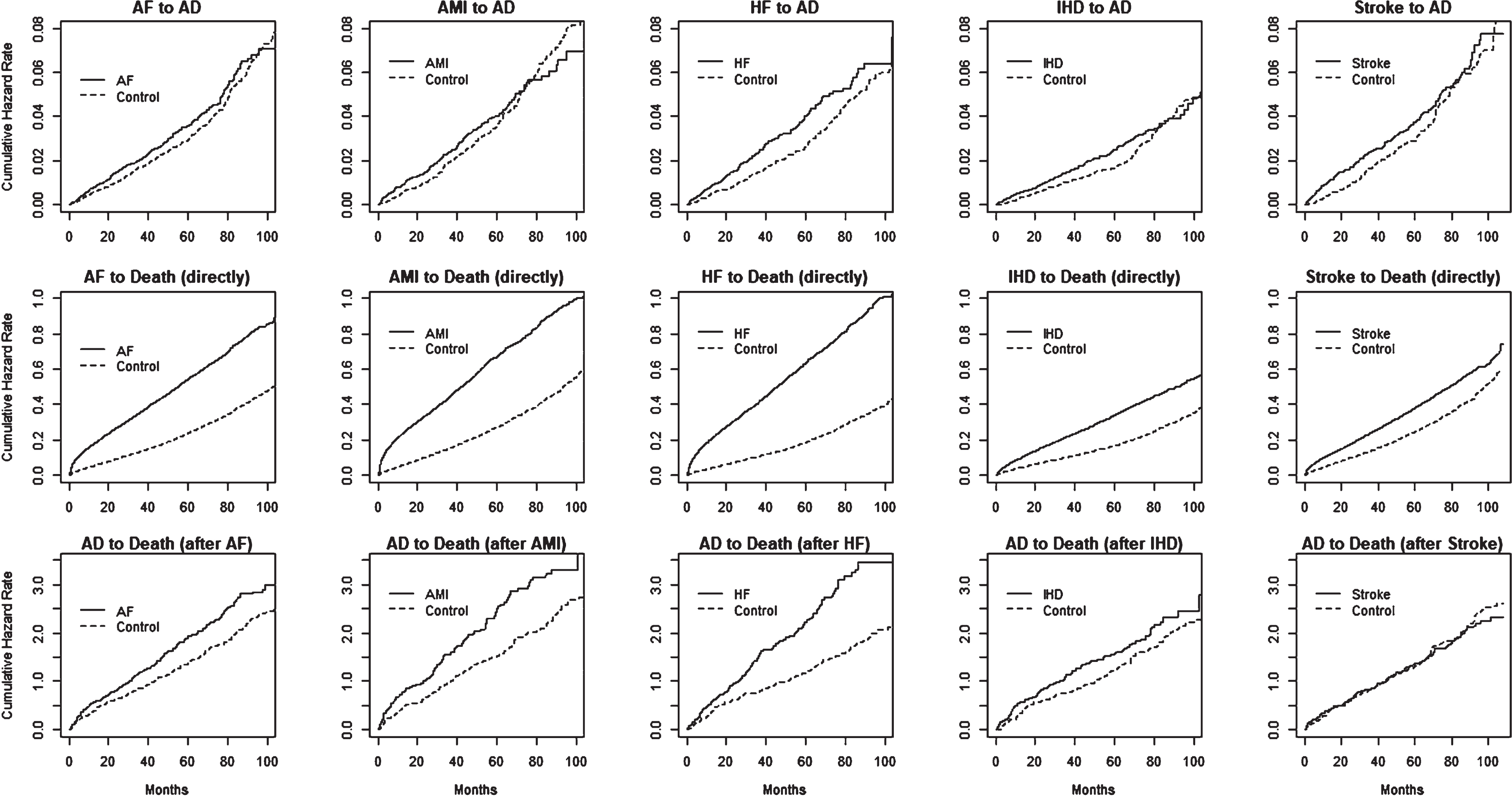
Risk of death after AD, with history of heart disease or stroke
Table 1 (column 2) shows the risks of death after developing AD, given that the subjects had already experienced AF, AMI, HF, IHD, or stroke conditions. The results show increased risks for four out of the five conditions: AF (HR = 1.37), AMI (HR = 1.52), HF (HR = 1.79), and IHD (HR = 1.37), compared to the control group. A relatively stronger group difference was observed for the HF group. However, this analysis did not show any statistically significant risks for subjects who had experienced stroke in the above transition, compared to the corresponding controls. These relationships can also be observed from Fig. 3 (third row).
Direct risk of death after heart disease or stroke
As expected, we found increased hazard rates for death immediately following AF, AMI, HF, IHD, and stroke compared to the corresponding control groups, with HR values of 1.30, 1.39, 1.45, 1.15, and 1.12, respectively. This can be clearly observed in Fig. 3, second row. This result indicates the highest risk of death for the HF condition, whereas the IHD condition had the lowest risk.
State occupational probabilities
We provide estimated state occupational probabilities for the multistate models in Fig. 4. Inspection of the estimated probabilities of state-0 among the heart disease and stroke sets continued to decline while the probabilities of state-2 increased steadily, compared to the control group. As shown in Supplementary Table 7, at 48 months, the estimated state-1 probabilities (% ) for the {diseased versus controls} groups were {62.3:81.6}, {55.5:79.1}, {57.6:85.2}, {74.5:86.3}, and {71.4:81.4} for the AF, AMI, HF, IHD, and stroke models, respectively. On the other hand, the corresponding state-2 probabilities (% ) were {36.6:17.1}, {43.4:19.6}, {41.5:13.6}, {24.7:12.9}, {27.2:17.6}, for the AF, AMI, HF, IHD, and stroke models, respectively. At a given time, estimated state occupational probabilities at state-1 were relatively small due to the state 1 to state 2 transition process.
Fig. 4
The figure displays the estimated state occupational probabilities over time for individuals with different disease conditions (AF, AMI, HF, IHD, and stroke) and controls, based on the multistate model shown in Fig. 1. The plots in the first three rows correspond to state-0, state-1, and state-2, estimates, for each of the five disease models.
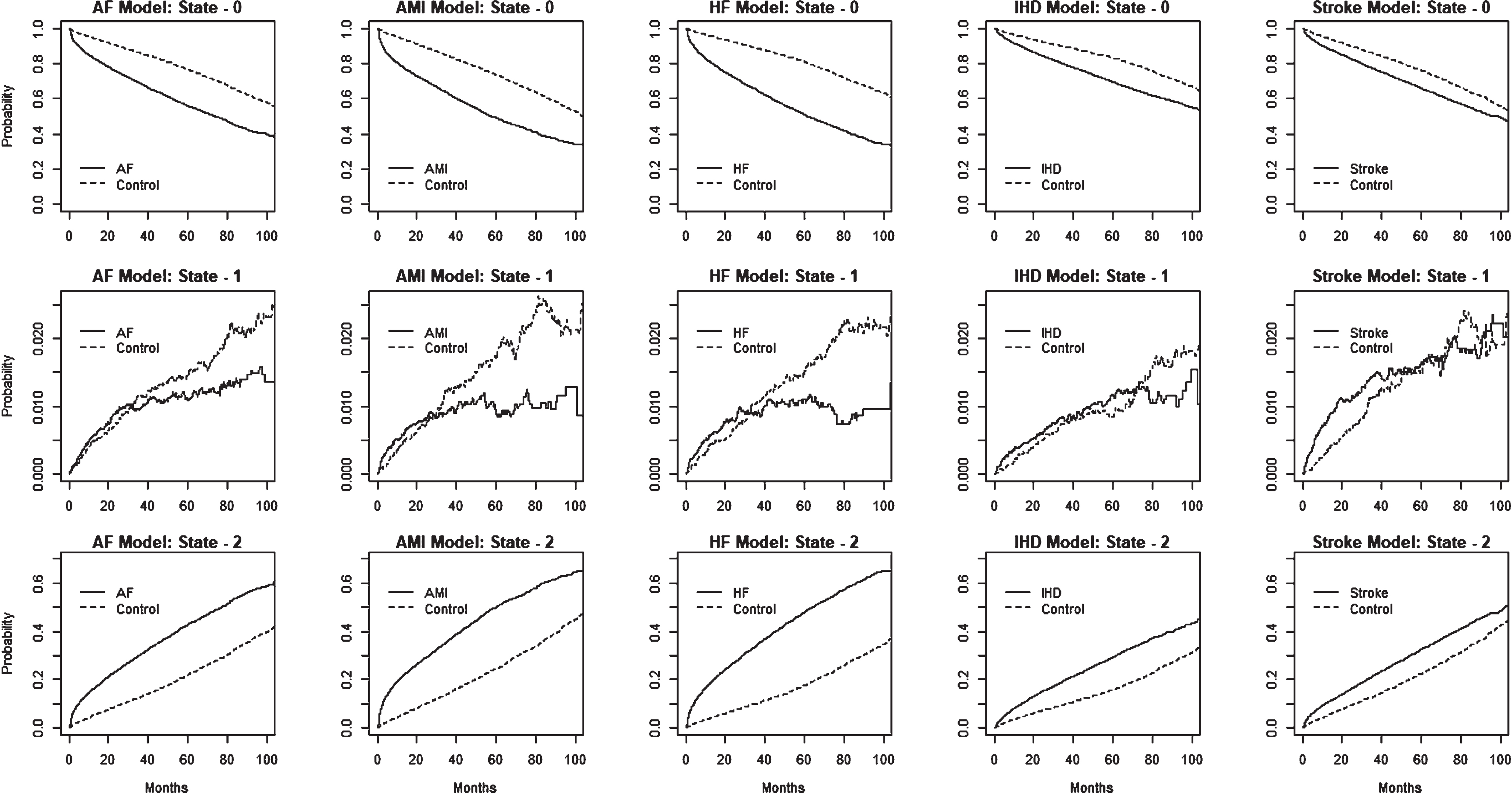
Racial/ethnic differences on AD risks after heart disease and stroke
In Tables 2–4, we provide details on the racial/ethnic effects observed on transitioning from each of the initial states of the multistate model given in Fig. 2: AF, AMI, HF, IHD, and stroke to AD. Note that the analysis results were stratified by the DE indicator that corresponds to having Medicare and Medicaid coverage for low-income beneficiaries. This is because heterogeneous effects were observed between the two groups on transitions, which is explained in a sequel. As previously suggested in the Methods section, DE was considered a surrogate marker of an individual’s financial status. In our discussion of the analysis results, we focus on these two groups separately (i.e., DE and Medicare-only). In the Supplementary Table 8, we provide a summary of marginal effects by DE status on transitions in each of the models. For example, consistently increased risks were observed for the state-0 to state-1 transition for all conditions considered in the study: AF (HR = 1.63), AMI (HR = 3.95), HF (HR = 1.46), IHD (HR = 1.96), and stroke (HR = 1.39). In Tables 2–4, an HR value greater than 1 indicates an increased risk for a given racial group compared to a reference group. Otherwise, a reduced risk. As one of our key interests was to examine effects on NHPIs compared to both whites and Asians in Hawaii, we considered both Asians and whites as reference groups in that aspect.
Table 2
Racial/ethnic effects on developing AD after heart disease and stroke
NHPI versus Whites | NHPI versus Asians | Asians versus Whites | |||||
HR (95% CI) | p | HR (95% CI) | p | HR (95% CI) | p | ||
Medicare only | AF | 0.700(0.501, 0.978) | 0.0366 | 0.734(0.536, 1.005) | 0.0536 | 0.954(0.733, 1.242) | 0.7261 |
AMI | 1.058(0.537, 2.084) | 0.8705 | 0.914(0.434, 1.926) | 0.8131 | 1.158(0.684, 1.961) | 0.5851 | |
HF | 0.542(0.351, 0.837) | 0.0057 | 0.394(0.248, 0.627) | <0.0001 | 1.375(0.998, 1.894) | 0.0513 | |
IHD | 0.750(0.575, 0.978) | 0.0336 | 0.584(0.434, 0.787) | 0.0004 | 1.285(0.988, 1.671) | 0.0613 | |
Stroke | 0.786(0.496, 1.246) | 0.3055 | 0.785(0.488, 1.263) | 0.3182 | 1.002(0.657, 1.528) | 0.9926 | |
Dual Eligible | AF | 0.793(0.536, 1.173) | 0.2455 | 1.544(1.147, 2.079) | 0.0042 | 0.514(0.319, 0.829) | 0.0063 |
AMI | 0.809(0.539, 1.215) | 0.3071 | 1.722(1.073, 2.764) | 0.0244 | 0.47(0.322, 0.686) | <0.0001 | |
HF | 1.316(1.004, 1.724) | 0.0464 | 2.223(1.498, 3.300) | <0.0001 | 0.592(0.397, 0.883) | 0.0101 | |
IHD | 1.369(1.103, 1.699) | 0.0044 | 2.393(1.552, 3.690) | <0.0001 | 0.572(0.436, 0.750) | <0.0001 | |
Stroke | 1.408(1.043, 1.901) | 0.0254 | 1.852(1.297, 2.645) | 0.0007 | 0.76(0.561, 1.030) | 0.0767 |
Table summarizes the race/ethnicity-based hazard ratio (HR) values observed in the transition probabilities from state 0 to state 1 in the multistate model presented in Fig. 2. The estimated HR corresponds to a specific racial/ethnic group in comparison to a reference group, using the pseudo-value regression approach. The results were stratified by Dual Eligibility (DE) status.
Table 3
Racial/ethnic effects on the risk of death after AD with history of heart disease or stroke
NHPI versus Whites | NHPI versus Asians | Asians versus Whites | |||||
HR (95% CI) | p | HR (95% CI) | p | HR (95% CI) | p | ||
Medicare only | AF | 1.091(0.906, 1.314) | 0.3584 | 0.927(0.733, 1.172) | 0.5264 | 1.177(0.942, 1.471) | 0.1525 |
AMI | 0.849(0.546, 1.320) | 0.4672 | 0.977(0.617, 1.547) | 0.921 | 0.869(0.584, 1.293) | 0.4887 | |
HF | 0.665(0.428, 1.032) | 0.069 | 0.639(0.468, 0.872) | 0.0048 | 1.374(0.786, 2.402) | 0.2648 | |
IHD | 0.677(0.436, 1.051) | 0.0823 | 1.554(0.912, 2.648) | 0.1049 | 0.436(0.232, 0.820) | 0.0100 | |
Stroke | 1.845(1.290, 2.639) | 0.0008 | 2.134(1.089, 4.183) | 0.0273 | 0.864(0.440, 1.695) | 0.6708 | |
Dual Eligible | AF | 2.132(1.081, 4.203) | 0.0288 | 1.463(1.075, 1.991) | 0.0156 | 1.458(0.941, 2.260) | 0.0918 |
AMI | 0.719(0.445, 1.162) | 0.1777 | 0.783(0.522, 1.174) | 0.2370 | 0.918(0.383, 2.199) | 0.8478 | |
HF | 1.024(0.923, 1.136) | 0.6545 | 1.245(0.859, 1.804) | 0.2468 | 0.614(0.298, 1.264) | 0.1854 | |
IHD | 0.934(0.743, 1.174) | 0.5585 | 1.389(0.04, 47.825) | 0.8556 | 0.673(0.296, 1.528) | 0.3439 | |
Stroke | 0.705(0.470, 1.057) | 0.0906 | 1.264(0.423, 3.779) | 0.675 | 0.558(0.291, 1.070) | 0.0791 |
Table summarizes the race/ethnicity-based hazard ratio (HR) values observed in the transition probabilities from state 1 to state 2 in the multistate model presented in Fig. 2. The estimated HR corresponds to a specific racial/ethnic group in comparison to a reference group, using the pseudo-value regression approach. The results were stratified by Dual Eligibility (DE) status.
Table 4
Racial/ethnic effects on the risk of death after heart disease and stroke, but without AD
NHPI versus Whites | NHPI versus Asians | Asians versus Whites | |||||
HR (95% CI) | p | HR (95% CI) | p | HR (95% CI) | p | ||
Medicare only | AF | 1.028(0.954, 1.108) | 0.4711 | 0.911(0.840, 0.988) | 0.024 | 1.128(1.051, 1.21) | 0.0008 |
AMI | 0.923(0.832, 1.024) | 0.1315 | 0.95(0.857, 1.053) | 0.3293 | 0.972(0.885, 1.068) | 0.5546 | |
HF | 1.108(1.013, 1.212) | 0.0246 | 0.961(0.88, 1.049) | 0.3753 | 1.153(1.07, 1.243) | 0.0002 | |
IHD | 1.018(0.943, 1.099) | 0.6473 | 0.902(0.831, 0.979) | 0.0139 | 1.129(1.057, 1.206) | 0.0003 | |
Stroke | 0.972(0.880, 1.074) | 0.5776 | 1.048(0.932, 1.178) | 0.4315 | 0.927(0.835, 1.029) | 0.1537 | |
Dual Eligible | AF | 0.894(0.743, 1.076) | 0.2348 | 1.065(0.890, 1.274) | 0.4911 | 0.84(0.729, 0.968) | 0.0159 |
AMI | 0.502(0.355, 0.710) | <0.0001 | 0.611(0.446, 0.838) | 0.0022 | 0.821(0.661, 1.020) | 0.0752 | |
HF | 0.865(0.769, 0.974) | 0.0162 | 0.972(0.569, 1.66) | 0.9172 | 0.89(0.827, 0.958) | 0.0020 | |
IHD | 0.626(0.499, 0.786) | <0.0001 | 0.753(0.544, 1.042) | 0.0868 | 0.831(0.758, 0.911) | <0.0001 | |
Stroke | 0.698(0.504, 0.966) | 0.0300 | 0.917(0.755, 1.114) | 0.383 | 0.760(0.573, 1.009) | 0.0576 |
Table summarizes the race/ethnicity-based hazard ratio (HR) values observed in the transition probabilities from state 0 to state 2 in the multistate model presented in Fig. 2. The estimated HR corresponds to a specific racial/ethnic group in comparison to a reference group, using the pseudo-value regression approach. The results were stratified by Dual Eligibility (DE) status.
Table 2 shows that in the DE group, NHPIs had a higher risk of developing AD compared to whites in three health states: HF (HR = 1.32), IHD (HR = 1.37), and stroke (HR = 1.41). NHPIs also had consistently higher AD risks than Asians in all five health states: AF (HR = 1.54), AMI (HR = 1.72), HF (HR = 2.23), IHD (HR = 2.39), and stroke (HR = 1.85). It’s important to note that in the DE group, Asians had a lower risk of developing AD than whites in all five health states: AF (HR = 0.51), AMI (HR = 0.47), HF (HR = 0.55), IHD (HR = 0.57), and stroke (HR = 0.76). This suggests that Asians have the safest AD risk profiles compared to the other groups. Although we found some racial differences in AD risk following the AMI to AD transition, it’s worth noting that a previous assessment of the risk of developing AD after an AMI, using AMI patients and controls, did not show a substantially elevated risk for AMI patients.
Interestingly, among the Medicare-only cohort, we observed a relationship that was inverted compared to the DE cohort, with reduced AD risks for NHPIs compared to whites for many conditions, including AF (HR = 0.70), HF (HR = 0.54), and IHD (HR = 0.75). NHPIs also had a reduced risk compared to Asians from HF (HR = 0.39) and IHD (HR = 0.58) to AD transition. The effect was only borderline significant for AF (HR = 0.73). In the Medicare-only group, we did not find substantial differences between Asians and whites; however, we found increased risks for HF and IHD with borderline significance.
Racial differences on the risk of death after AD, with history of heart disease or stroke
The analysis of racial-ethnic differences in the risk of death after heart and stroke conditions/events showed only a few differences among the groups, which are presented in Table 3. Among the differences found, in the DE cohort, NHPIs had an increased risk of death compared to both whites (HR = 2.13) and Asians (HR = 1.46) after the AF to AD transition. Among the DE cohort, NHPIs had increased risks compared to both whites and Asians after the stroke to AD transition, but they had a reduced risk of death compared to Asians after HF (HR = 0.64). Additionally, Asians had a reduced risk of death compared to whites after IHD (HR = 0.44).
Racial differences on the direct risk of death after heart disease or stroke
Racial/ethnic differences in the risk of death due to cardiovascular conditions are presented in Table 4. In the DE cohort, NHPIs had a reduced risk of transitioning from state 0 (initial event) to state 1 (death) compared to whites after AMI (HR = 0.50), HF (HR = 0.87), IHD (HR = 0.63), and stroke (RR = 0.698). NHPIs also had a reduced risk compared to Asians from AMI (HR = 0.61). In addition, Asians had reduced risks compared to whites after AF (HR = 0.84), HF (HR = 0.89), and IHD (HR = 0.83), indicating an overall low-risk profile for this group.
In the Medicare-only cohort, we did not find excessively elevated or low risk for any group, however, there were still significant differences for a few cases. NHPIs had an increased risk compared to whites for HF to death (HR = 1.10). They had a reduced risk compared to Asians from AF (HR = 0.91) and IHD (HR = 0.90). Increased risks were found for Asians from AF (HR = 1.12), HF (HR = 1.15), and IHD (HR = 1.12) cases. Overall, the differences found within the Medicare-only cohort were marginal in terms of magnitudes.
DISCUSSION
There is limited literature on the topic of heart disease and stroke’s connection to AD progression. More importantly, this topic has never been studied before in a minority population in a majority-minority state like Hawaii. Hawaii’s population stands out from the rest of the US due to various distinguishing factors such as its ethnic diversity, lifestyle, location, and geography. Numerous studies have shown racial and ethnic health disparities in this population in different contexts, highlighting the NHPI group as a high-risk group for various health conditions, including diabetes [36], chronic kidney disease [23], obesity [37], and cardiovascular conditions [18, 22], compared to other ethnic groups. It is important to emphasize that from 2000 to 2010, the NHPI population grew by approximately 40% , making it one of the fastest-growing populations in the US [38]. If heart disease and stroke are linked to AD, assessing ethnic disparities within the link between heart/stroke and AD is vital. Due to the large presence of Asians, understanding the comparative risks for Asians is also of interest to the state.
Our longitudinal analysis showed that patients who had AF, HF, IHD, and stroke were at an increased risk of developing AD when compared to a matched control group. Furthermore, the risk of death from AD was considerably higher for patients who had transitioned from AF, AMI, HF, and IHD to AD, as compared to the control group. Our findings provide a foundation for further research into potential racial and ethnic disparities. Given that NHPIs have been identified as a high-risk group for heart disease and stroke, this group is also likely to be at an increased risk for AD, based on this link.
It is also important to determine whether heart disease and stroke increase the risk of AD to a greater extent in certain racial groups, or whether the risk is consistent across all races. In this next phase of the study, we investigated this issue and found that there are indeed racial and ethnic differences in the likelihood of developing AD following cardiovascular conditions. We also discovered that socioeconomic status, as measured by the DE index, plays a significant role in how race affects the risk of AD. DE is often used as a proxy for low socioeconomic status and is considered a social determinant of health [39–41]. The DE population has been found to have a higher prevalence of chronic conditions and a larger total number of chronic conditions than those who are only eligible for Medicare [42, 43]. Additionally, the DE population is responsible for a disproportionate amount of Medicare spending [44]. These factors make the DE population an important focus of public health research, particularly regarding racial/ethnic differences in health outcomes. Studies have shown that DE patients experience worse short- and long-term outcomes for several conditions, including AMI, AF, HF, IHD, and stroke, compared to those who are only eligible for Medicare [41, 45, 46]. It is worth noting that DE individuals may fall under different eligibility categories, as described elsewhere. For a detailed explanation of DE eligibility criteria, readers should refer to the Centers for Medicare & Medicaid Services [47].
Previous studies have shown that low socioeconomic status is associated with poor health outcomes [48, 49]. In our current study, we found that DE patients had an increased risk of AD after heart disease and stroke compared to Medicare-only patients. Interestingly, we also found that NHPIs had an increased risk of AD after HF, IHD, and stroke compared to whites within the DE group. Furthermore, NHPIs had a higher AD risk compared to Asians after AF, AMI, HF, IHD, and stroke. However, these observations were different for the Medicare-only group, as NHPIs had a lower AD risk than both whites and Asians in this setting. While this study does not indicate an overall increased AD risk for NHPI patients with heart disease or stroke compared to white patients, there seems to be an important effect of lifestyle, behaviors, health literacy, access to healthcare, and other essential resources mediated by poor socioeconomic status. Therefore, we believe more in-depth studies should focus on this issue in future research. Investigating patterns of care by DE status allows for the incorporation of a subject-level indicator of low income in clinical practice. This study highlights the increased risk of AD development in the DE population, with NHPIs being the most disadvantaged group. We believe that more attention should be focused on these connections to develop interdisciplinary initiatives that increase awareness among both practitioners and patients. In a state such as Hawaii, which has a strong racial diversity and health disparities, understanding the role of race may result in benefits for elderly patients through appropriate evidence-based treatment. Discussion among work groups that include teams of neurologists, cardiologists, patient advocates, and healthcare stakeholders may be appropriate in these aspects. Future health policy developments should focus on high-risk groups for both early prevention and healthcare cost/resource management.
More attention is needed for AD management within the DE population. National-level statistics indicate that nearly 24% of those aged 65 and above living with AD and other dementias have DE status, compared to only 10% of seniors without AD [48]. The per-person Medicaid expenditure for seniors with AD and other dementias is 22 times greater than average per-person Medicaid spending across all seniors without any dementia, placing an enormous burden on the economy [49, 50]. In 2022, Medicaid spent $60 billion on caring for people with AD or other dementia. It is projected that between 2020 and 2025, 30 US states will experience at least a 20% increase in Medicaid spending on AD and other dementias [49].
However, it is important to note that DE status is not solely an indicator of poor socio-economic status. Medicaid coverage provides additional financial assistance for medical care and prescription drugs, which can affect medication adherence, the utility of services, and health outcomes. From a different perspective, it could also be argued that the Medicare database more efficiently captures healthcare claims for the DE group compared to the Medicare-only group, as Medicare-only beneficiaries tend to have additional health insurance coverage, such as private insurance. Therefore, findings from DE claims may provide a more accurate representation of the true status.
There have been many previous studies that have examined the relationship between socioeconomic status and the risk of developing AD [51–53]. For example, Moceri et al. found that AD risk is associated with economic level during childhood, age of parents, and family size [52]. Karp et al. reported an increased risk of AD based on occupational socioeconomic status [15]. Tóth et al. analyzed the relationship between AD and socioeconomic factors such as GDP, wage, and education and found significant associations [53]. It is well known that low socioeconomic status is associated with poor education, and a wealth of literature has examined the inverse relationship between education and the risk of AD or dementia in cross-sectional and case-control studies [52, 54–57]. Previous studies in Hawaii have shown that the level of education, at both individual and neighborhood levels, is associated with self-rated general health [58]. It has been proposed that differences in socioeconomic status mediate the self-rated health differences observed between some ethnic groups in Hawaii [58]. It is important to note that Native Hawaiians have reported the lowest median household income and the highest poverty rate in Hawaii [59]. Low socioeconomic status has also been associated with poor physical and psychological health, with unhealthy lifestyle patterns being mediated by socioeconomic effects [60]. Individuals with low socioeconomic status often have insufficient access to healthcare and high-quality food and nutrition [61, 62]. It is worth noting that an individual’s neighborhood can be influenced by their socioeconomic status. Studies have highlighted the impact of environmental factors such as nutrients, pollutants, chemicals, physical activity, and lifestyle on both physical and mental health [51].
Previous studies have shown the association between heart disease and cognition through systematic pathways and findings from observational analyses. It is widely known that insufficient blood circulation can contribute to systemic alterations in multiple organs, including the brain, leading to organ dysfunction syndrome [63]. For instance, studies indicate that decreased cardiac output due to HF is associated with cognitive impairment [64–66]. Left ventricular ejection fraction (LVEF) was found to be specifically linked to a series of cognitive declines in patients with HF [7, 67, 68]. Moreover, LVEF has been confirmed to be associated with small brain volumes [7, 69, 70]. Neuroimaging studies provide evidence of structural brain abnormalities among individuals with HF, including alterations in the medial temporal lobe and other structures within the Papez circuit, which are closely connected to the medial temporal lobes [8, 11, 71, 72]. In patients with HF, structural alterations in the medial temporal lobe are similar to those observed in AD [72]. Furthermore, critical white matter pathways in the medial temporal lobe have been found to be altered in HF patients [11]. Studies also report alterations in the cingulate gyrus, mammillary bodies and fornix, and thalamus [71, 73, 74]. The medial temporal lobe and related limbic system structures are known to play critical roles in memory functions and have been suggested as early markers of AD [75, 76]. Several epidemiological studies have investigated the link between AD and the heart. In a previous study, a community-based cohort of individuals aged 75 years or older without dementia in Sweden were examined over a 9-year period and found that heart failure was associated with AD with a hazard ratio of 1.80 [77]. The study also suggested that antihypertensive drug therapy may partially counteract the risk effect of heart failure on dementia disorders. In a recent cohort study of patients with incident HF, the burden of AD and AD-related dementias (AD/ADRD) was found to be high as more than 25% of patients with HF received a diagnosis of AD/ADRD either before or after HF diagnosis. Furthermore, AD/ADRD was found to increase the risk of adverse outcomes in HF [78]. A large-scale cohort study conducted in the Danish population showed that HF was associated with an increased risk of all-cause dementia but not necessarily AD [79]. Similarly, a study based on UK Biobank participants of European ancestry found that the dementia risk associated with high cardiometabolic multimorbidity was three times greater than that associated with high genetic risk [80]. Although there is a wide body of literature, including the current study, that supports the link between AD and the heart, the mechanistic relationship remains largely unclear. Reduced cerebral blood flow [72] and progressive dysfunction of the neurovascular unit are commonly cited explanations for this relationship, which likely involve multiple pathways that correspond to differentconditions.
AF is the most common type of arrhythmia, and previous studies have shown impaired cognitive functions in individuals with AF [81–84]. Neuroimaging studies have also revealed brain abnormalities in AF patients, such as reduced hippocampal volume [85]. AF has been suggested as a risk factor for both dementia and AD [86–88]. Cerebral embolism is common in heart failure patients [89], and the risk of embolism is significantly increased in AF. Progressive embolism can cause cerebral damage and result in cognitive impairment, including AD [90, 91].
Several studies have confirmed the association between IHD and cognitive impairment, vascular dementia, and AD development [92–95]. Elderly IHD patients have shown brain MRI abnormalities, including white matter lesions and grey matter changes [96, 97]. Additionally, studies have linked AMI with dementia [98–100], and a cross-sectional assessment of the Rotterdam study found an association between AMI and cognitive impairment resulting from brain hypoperfusion [101]. However, the causality of AMI on AD development remains controversial [102]. Note that in the current study, we were unable to establish a statistically meaningful link between AD development based on AMI.
Also, evidence shows that stroke plays a critical role in AD development [103–107]. In particular, a longitudinal follow-up study of Medicare recipients without dementia in Manhattan, New York, showed that stroke is associated with AD among elderly individuals, and the relationship was found to be strongest in the presence of known vascular risk factors [105]. A systematic review and meta-analysis confirmed that stroke is a risk factor for AD development [106]. However, similar to heart disease, the exact role of stroke in the pathogenesis of AD remains largely unclear [104, 107].
In addition to the principal analytical approach employed in this study, we also performed a sensitivity assessment of key analyses using an alternative Fine and Gray competing risk approach [108]. A summary of these results can be found in Supplementary Tables 15 and 16. Overall, a concurrence between the outcomes obtained from the two distinct analytical approaches was observed.
There are several limitations to this study. Firstly, using ICD codes alone to identify individuals with a disease may not be sufficient. For example, AD may be misdiagnosed in patients with other types of dementia, and co-existing vascular dementia may also be misclassified as AD. This is a common issue with studies that use administrative databases, such as Medicare data. In addition, disease history prior to 2009 was determined based on records for selected major conditions, which may not provide a complete picture of an individual’s disease profile. This could result in misidentification of subjects who had previously been diagnosed with a specific condition as new cases, leading to errors in survival estimates. In the context of utilizing ICD codes for reimbursement purposes within Medicare data, it is plausible that the prevalence of AD may be underestimated in the Medicare database when compared to actual incidence rates. Moreover, the estimation of racial effects may be influenced if race proves to be a significant factor in the timeline of AD diagnosis. Another limitation of this study is the reliance on self-reported race-ethnicity information provided by the Medicare beneficiaries to CMS. Self-reported data can sometimes be subject to biases or inaccuracies, and this could impact the precision and reliability of our findings. The population in Hawaii is highly diverse, and there are important health disparities within the Asian population. While several studies have identified the Hawaiian Filipino population as a disadvantaged group for various health conditions, including heart disease and stroke [109, 110], the Medicare data used in this study does not capture detailed subethnic identity. Further analysis with disaggregated Asian ethnicity would be useful. There were also some reporting errors observed in the claims data, and longer follow-up time may be necessary to examine smaller effects. The study did not account for potential temporal effects due to calendar time, and there may be numerous other factors, such as medication history, that could be important to consider for a better assessment of the relationship between cardiovascular diseases and AD development. Finally, the selected cardiovascular diseases are not homogeneous, and many subtypes exist within each condition. While the study aimed to investigate the influence of cardiovascular diseases on AD development and racial effects on this relationship, further investigations focusing on various subtypes within the selected set of conditions could be considered in future studies.
The use of health insurance databases, such as Medicare, may not be the best choice for evaluating progressive disease models because these data sources are generally not able to efficiently capture an individual’s exact status in real-time. Administrative claim databases only document data when a claim has been processed, which may result in many unreported incidents. These incidents may occur due to various reasons, such as unawareness, claiming through multiple insurance plans or switching between plans, delayed doctor visits, and reporting errors. These issues could lead to the underestimation of transition hazards, rather than overestimation, and can be assumed to occur randomly. Another potential issue with studies conducted based on claims data is limited generalizability of findings to the entire population. The findings could be specific to the population under investigation, which, in this study, is the elderly Medicare population. Despite these limitations, longitudinal health insurance databases provide an excellent opportunity to investigate disease transition problems, as the continuous capturing of health status in real-time faces various challenges in real-life.
We believe this is one of the earliest studies investigating the risk of AD from heart disease and stroke in the context of state transitions with the emphasis on racial/ethnic disparities. This work revealed several important findings that may be helpful for the community and healthcare stakeholders in developing strategies for AD prevention, management, and resource allocation.
Conclusion
This study shows an increased risk of developing AD among subjects who developed heart disease and stroke conditions, suggesting a progressive disease relationship. There exist significant racial/ethnic disparities among the Hawaii population in the link of heart disease and stroke to AD. NHPIs with lower socioeconomic status seems to be mostly disadvantaged by the heart/stroke and AD link compared to corresponding whites and Asians.
ACKNOWLEDGMENTS
The authors have no acknowledgments to report.
FUNDING
Research reported in this publication were supported by the National Institute on Aging of the National Institutes of Health under Award Number R03AG075034 and partially by the National Institute on Minority Health and Health Disparities of the National Institutes of Health under Award Number 2U54MD007601-36. The content is solely the responsibility of the authors and does not necessarily represent the official views of the National Institutes of Health.
CONFLICT OF INTEREST
The authors have no conflict of interest to report.
DATA AVAILABILITY
The datasets generated during and/or analyzed during the current study are not publicly available due to privacy reasons and will not be shared due to third-party agreements.
SUPPLEMENTARY MATERIAL
[1] The supplementary material is available in the electronic version of this article: https://dx.doi.org/10.3233/ADR-230003.
REFERENCES
[1] | Serrano-Pozo A , Frosch MP , Masliah E , Hyman BT ((2011) ) Neuropathological alterations in Alzheimer disease. Cold Spring Harb Perspect Med 1: , a006189. |
[2] | Alzheimer’s Association ((2020) ) 2020 Alzheimer’s disease facts and figures. Alzheimers Dement 16: , 391–460. |
[3] | Cermakova P , Eriksdotter M , Lund LH , Winblad B , Religa P , Religa D ((2015) ) Heart failure and Alzheimer’s disease. J Intern Med 277: , 406–425. |
[4] | Tublin JM , Adelstein JM , Del Monte F , Combs CK , Wold LE ((2019) ) Getting to the heart of Alzheimer disease. Circ Res 124: , 142–149. |
[5] | Wanleenuwat P , Iwanowski P , Kozubski W ((2019) ) Alzheimer’s dementia: Pathogenesis and impact of cardiovascular risk factors on cognitive decline. Postgrad Med 131: , 415–422. |
[6] | Debette S , Bauters C , Leys D , Lamblin N , Pasquier F , de Groote P ((2007) ) Prevalence and determinants of cognitive impairment in chronic heart failure patients. Congest Heart Fail 13: , 205–208. |
[7] | Jefferson AL , Himali JJ , Au R , Seshadri S , Decarli C , O’Donnell CJ , Wolf PA , Manning WJ , Beiser AS , Benjamin EJ ((2011) ) Relation of left ventricular ejection fraction to cognitive aging (from the Framingham Heart Study). Am J Cardiol 108: , 1346–1351. |
[8] | Dodson JA , Truong TT , Towle VR , Kerins G , Chaudhry SI ((2013) ) Cognitive impairment in older adults with heart failure: Prevalence, documentation, and impact on outcomes. Am J Med 126: , 120–126. |
[9] | Hjelm C , Broström A , Dahl A , Johansson B , Fredrikson M , Strömberg A ((2014) ) Factors associated with increased risk for dementia in individuals age 80 years or older with congestive heart failure. J Cardiovasc Nurs 29: , 82–90. |
[10] | Vogels RL , van der Flier WM , van Harten B , Gouw AA , Scheltens P , Schroeder-Tanka JM , Weinstein HC ((2007) ) Brain magnetic resonance imaging abnormalities in patients with heart failure. Eur J Heart Fail 9: , 1003–1009. |
[11] | Kumar R , Woo MA , Macey PM , Fonarow GC , Hamilton MA , Harper RM ((2011) ) Brain axonal and myelin evaluation in heart failure. J Neurol Sci 307: , 106–113. |
[12] | Justin BN , Turek M , Hakim AM ((2013) ) Heart disease as a risk factor for dementia. Clin Epidemiol 5: , 135–145. |
[13] | Vijayan M , Reddy PH ((2016) ) Stroke, vascular dementia, and Alzheimer’s disease: Molecular links. J Alzheimers Dis 54: , 427–443. |
[14] | Schultz WM , Kelli HM , Lisko JC , Varghese T , Shen J , Sandesara P , Quyyumi AA , Taylor HA , Gulati M , Harold JG , Mieres JH , Ferdinand KC , Mensah GA , Sperling LS ((2018) ) Socioeconomic status and cardiovascular outcomes: Challenges and interventions. Circulation 137: , 2166–2178. |
[15] | Karp A , Kåreholt I , Qiu C , Bellander T , Winblad B , Fratiglioni L ((2004) ) Relation of education and occupation-based socioeconomic status to incident Alzheimer’s disease. Am J Epidemiol 159: , 175–183. |
[16] | Evans DA , Hebert LE , Beckett LA , Scherr PA , Albert MS , Chown MJ , Pilgrim DM , Taylor JO ((1997) ) Education and other measures of socioeconomic status and risk of incident Alzheimer disease in a defined population of older persons. Arch Neurol 54: , 1399–1405. |
[17] | US Census Bureau ((2020) ) 2020 Census Redistricting Data - Racial and Ethnic Diversity Index by State: 2020 https://www.census.gov/content/dam/Census/library/visualizations/2021/dec/diversity-index.pdf. Accessed April 3, 2023. |
[18] | Tung WC , Barnes M ((2014) ) Heart diseases among Native Hawaiians and Pacific Islanders. Home Health Care Manag Pract 26: , 110–113. |
[19] | Aluli NE , Reyes PW , Brady SK , Tsark JU , Jones KL , Mau M , Howard WJ , Howard BV ((2010) ) All-cause and CVD mortality in Native Hawaiians. Diabetes Res Clin Pract 89: , 65–71. |
[20] | Siriwardhana C , Lim E , Davis J , Chen JJ ((2018) ) Progression of diabetes, ischemic heart disease, and chronic kidney disease in a three chronic conditions multistate model. BMC Public Health 18: , 752. |
[21] | Balabis J , Pobutsky A , Baker KK , Totori C , Salvali F ((2007) ) The burden of cardiovascular disease in Hawaii 2007 - State Department of Health Hawaii. https://health.hawaii.gov/brfss/files/2013/11/TheBurdenofCVD.pdf. Accessed November 1, 2022. |
[22] | Nakagawa K , Koenig MA , Asai SM , Chang CW , Seto TB ((2013) ) Disparities among Asians and native Hawaiians and Pacific Islanders with ischemic stroke. Neurology 80: , 839–843. |
[23] | Na‘ai D , Raphael KL ((2019) ) CKD in Native Hawaiians and Pacific Islanders: Trouble in paradise. Clin J Am Soc Nephrol 14: , 1661–1663. |
[24] | Alzheimer’s Association ((2018) ) Alzheimer’s statistics Hawaii, 2018 - Alzheimer’s Disease Facts and Figures report at alz.org/facts. https://www.alz.org/media/documents/hawaii-alzheimers-facts-figures-2018.pdf. Accessed April 5, 2023. |
[25] | Gu Q , Koenig L , Faerberg J , Steinberg CR , Vaz C , Wheatley MP ((2014) ) The Medicare Hospital Readmissions Reduction Program: Potential unintended consequences for hospitals serving vulnerable populations. Health Serv Res 49: , 818–837. |
[26] | Wickwire EM , Jobe SL , Oldstone LM , Scharf SM , Johnson AM , Albrecht JS ((2020) ) Lower socioeconomic status and co-morbid conditions are associated with reduced continuous positive airway pressure adherence among older adult Medicare beneficiaries with obstructive sleep apnea. Sleep 43: , zsaa122. |
[27] | Nee R , Yuan CM , Hurst FP , Jindal RM , Agodoa LY , Abbott KC ((2017) ) Impact of poverty and race on pre-end-stage renal disease care among dialysis patients in the United States. Clin Kidney J 10: , 55–61. |
[28] | Ho DE , Imai K , King G , Stuart EA ((2011) ) MatchIt: Nonparametric preprocessing for parametric causal inference. J Stat Softw 42: , 1–28. |
[29] | Aalen OO , Johansen S ((1978) ) An empirical transition matrix for non-homogeneous Markov chains based on censored observations. Scand J Stat 5: , 141–150. |
[30] | Datta S , Satten GA ((2001) ) Validity of the Aalen–Johansen estimators of stage occupation probabilities and Nelson–Aalen estimators of integrated transition hazards for non-Markov models. Stat Prob Lett 55: , 403–411. |
[31] | Datta S , Satten GA ((2002) ) Estimation of integrated transition hazards and stage occupation probabilities for non-Markov systems under dependent censoring. Biometrics 58: , 792–802. |
[32] | Andersen PK , Klein JP ((2007) ) Regression analysis for multistate models based on a pseudo-value approach, with applications to bone marrow transplantation studies. Scand J Stat 34: , 3–16. |
[33] | Klein JP , van Houwelingen HC , Ibrahim JG , Scheike TH ((2013) ) Handbook of Survival Analysis (1st ed). Chapman and Hall/CRC, New York. |
[34] | Zhou B , Fine J , Latouche A , Labopin M ((2012) ) Competing risks regression for clustered data. Biostatistics 13: , 371–383. |
[35] | Austin PC , Fine JP ((2019) ) Propensity-score matching with competing risks in survival analysis. Stat Med 38: , 751–777. |
[36] | McElfish PA , Purvis RS , Esquivel MK , Sinclair KA , Townsend C , Hawley NL , Haggard-Duff LK , Kaholokula JK ((2019) ) Diabetes disparities and promising interventions to address diabetes in Native Hawaiian and Pacific Islander populations. Curr Diab Rep 19: , 19. |
[37] | Bacong AM , Holub C , Porotesano L ((2016) ) Comparing obesity-related health disparities among Native Hawaiians/Pacific Islanders, Asians, and Whites in California: Reinforcing the need for data disaggregation and operationalization. Hawaii J Med Public Health 75: , 337–344. |
[38] | Look MA , Soong S , Kaholokula JK ((2020) ) Assessment and Priorities for Health and Well-Being in Native Hawaiians and Pacific Islanders. Honolulu, HI. Department of Native Hawaiian Health, John A. Burns School of Medicine, University of Hawai‘i. https://dnhh.hawaii.edu/wp-content/uploads/2020/11/NPHI_HlthAssessmentPriorities_Rpt2020.pdf. Accessed November 1, 2022. |
[39] | Roberts ET , Mellor JM , McInerney M , Sabik LM ((2019) ) State variation in the characteristics of Medicare-Medicaid dual enrollees: Implications for risk adjustment. Health Serv Res 54: , 1233–1245. |
[40] | CMS Medicare-Medicaid Coordination Office ((2020) ) Data analysis brief: Medicare-Medicaid dual enrollment 2006 through 2019. https://www.cms.gov/files/document/medicaremedicaiddualenrollmenteverenrolledtrendsdatabrief.pdf. Accessed November 1, 2022. |
[41] | Bosch PR , Karmarkar AM , Roy I , Fehnel CR , Burke RE , Kumar A ((2022) ) Association of Medicare-Medicaid dual eligibility and race and ethnicity with ischemic stroke severity. JAMA Netw Open 5: , e224596. |
[42] | Kasper J , Watts MO , Lyons B ((2010) ) Chronic disease and co-morbidity among dual eligibles: Implications for patterns of Medicaid and Medicare service use and spending. Kaiser Family Foundation. https://www.kff.org/wp-content/uploads/2013/01/8081.pdf. Accessed November 1, 2022. |
[43] | Medicare Payment Advisory Commission and Medicaid and CHIP Payment and Access Commission ((2022) ) Data book: Beneficiaries dually eligible for Medicare and Medicaid – February 2022. https://www.macpac.gov/wp-content/uploads/2022/02/Beneficiaries-Dually-Eligible-for-Medicare-and-Medicaid-February-2022.pdf. Accessed November 1, 2022. |
[44] | Addo J , Ayerbe L , Mohan KM , Crichton S , Sheldenkar A , Chen R , Wolfe CD , McKevitt C ((2012) ) Socioeconomic status and stroke: An updated review. Stroke 43: , 1186–1191. |
[45] | Doll JA , Hellkamp AS , Goyal A , Sutton NR , Peterson ED , Wang TY ((2016) ) Treatment, outcomes, and adherence to medication regimens among dual Medicare Medicaid–eligible adults with myocardial infarction. JAMA Cardiol 1: , 787–794. |
[46] | Bahiru E , Ziaeian B , Moucheraud C , Agarwal A , Xu H , Matsouaka RA , DeVore AD , Heidenreich PA , Allen LA , Yancy CW , Fonarow GC ((2012) ) Association of dual eligibility for Medicare and Medicaid with heart failure quality and outcomes among get with the guidelines-heart failure hospitals. JAMA Cardiol 6: , 791–800. |
[47] | Centers for Medicare & Medicaid Services ((2023) ) Dually Eligible Individuals - Categories. https://www.cms.gov/Medicare-Medicaid-Coordination/Medicare-and-Medicaid-Coordination/Medicare-Medicaid-Coordination-Office/Downloads/MedicareMedicaidEnrolleeCategories.pdf. Accessed March 22, 2023. |
[48] | Faigle R , Cooper LA ((2019) ) Explaining and addressing racial disparities in stroke care and outcomes: A puzzle to solve now. Neurology 93: , 773–775. |
[49] | Alzheimer’s Association ((2023) ) Alzheimer’s Impact Movement, Fact Sheet: Medicaid and Seniors with Alzheimer’s – March 2023. https://portal.alzimpact.org/media/serve/id/5ab115bad5065. Accessed March 20, 2023. |
[50] | ((2022) ) Alzheimer’s disease facts and figures. Alzheimers Dement 18: , 700–789. |
[51] | Nicolia V , Lucarelli M , Fuso A ((2015) ) Environment, epigenetics and neurodegeneration: Focus on nutrition in Alzheimer’s disease. Exp Gerontol 68: , 8–12. |
[52] | Moceri VM , Kukull WA , Emanual I , van Belle G , Starr JR , Schellenberg GD , McCormick WC , Bowen JD , Teri L , Larson EB ((2001) ) Using census data and birth certificates to reconstruct the early-life socioeconomic environment and the relation to the development of Alzheimer’s disease. Epidemiology 12: , 383–389. |
[53] | Tóth P , Gavurová B , Barták M ((2018) ) Alzheimer’s Disease mortality according to socioeconomic factors: Country study. Int J Alzheimers Dis 2018: , 8137464. |
[54] | Gilleard CJ ((1997) ) Education and Alzheimer’s disease: A review of recent international epidemiological studies. Aging Ment Health 1: , 33–46. |
[55] | Hill LR , Klauber MR , Salmon DP , Yu ES , Liu WT , Zhang M , Katzman R ((1993) ) Functional status, education, and the diagnosis of dementia in the Shanghai survey. Neurology 43: , 138–145. |
[56] | Ott A , Breteler MM , van Harskamp F , Claus JJ , van der Cammen TJ , Grobbee DE , Hofman A ((1995) ) Prevalence of Alzheimer’s disease and vascular dementia: Association with education. The Rotterdam Study. BMJ 310: , 970–973. |
[57] | Stern Y , Gurland B , Tatemichi TK , Tang MX , Wilder D , Mayeux R ((1994) ) Influence of education and occupation on the incidence of Alzheimer’s disease. JAMA 271: , 1004–1010. |
[58] | Zhang W , McCubbin H , McCubbin L , Chen Q , Foley S , Strom I , Kehl L ((2010) ) Education and self-rated health: An individual and neighborhood level analysis of Asian Americans, Hawaiians, and Caucasians in Hawaii. Soc Sci Med 70: , 561–569. |
[59] | Economic Development and Tourism state of Hawaii ((2018) ) Demographic, social, economic, and housing characteristics for selected race groups in Hawaii. Research and Economic Analysis Division Department of Business. https://files.hawaii.gov/dbedt/economic/reports/SelectedRacesCharacteristics_HawaiiReport.pdf. Accessed April 12, 2023. |
[60] | Wang J , Geng L ((2019) ) Effects of socioeconomic status on physical and psychological health: Lifestyle as a mediator. Int J Environ Res Public Health 16: , 281. |
[61] | McMaughan DJ , Oloruntoba O , Smith ML ((2020) ) Socioeconomic status and access to healthcare: Interrelated drivers for healthy aging. Front Public Health 8: , 231. |
[62] | Nagata JM , Fiorella KJ , Salmen CR , Hickey MD , Mattah B , Magerenge R , Milner EM , Weiser SD , Bukusi EA , Cohen CR ((2015) ) Around the table: Food insecurity, socioeconomic status, and instrumental social support among women living in a rural Kenyan Island community. Ecol Food Nutr 54: , 358–369. |
[63] | Harjola VP , Mullens W , Banaszewski M , Bauersachs J , Brunner-La Rocca HP , Chioncel O , Collins SP , Doehner W , Filippatos GS , Flammer AJ , Fuhrmann V , Lainscak M , Lassus J , Legrand M , Masip J , Mueller C , Papp Z , Parissis J , Platz E , Rudiger A , Ruschitzka F , Schäfer A , Seferovic PM , Skouri H , Yilmaz MB , Mebazaa A ((2017) ) Organ dysfunction, injury and failure in acute heart failure: From pathophysiology to diagnosis and management. A review on behalf of the Acute Heart Failure Committee of the Heart Failure Association (HFA) of the European Society of Cardiology (ESC). Eur J Heart Fail 19: , 821–836. |
[64] | Jefferson AL ((2010) ) Cardiac output as a potential risk factor for abnormal brain aging. J Alzheimers Dis 20: , 813–821. |
[65] | Leto L , Feola M ((2014) ) Cognitive impairment in heart failure patients. J Geriatr Cardiol 11: , 316–328. |
[66] | Witt LS , Rotter J , Stearns SC , Gottesman RF , Kucharska-Newton AM , Richey Sharrett A , Wruck LM , Bressler J , Sueta CA , Chang PP ((2018) ) Heart failure and cognitive impairment in the Atherosclerosis Risk in Communities (ARIC) Study. J Gen Intern Med 33: , 1721–1728. |
[67] | Putzke JD , Williams MA , Daniel JF , Foley BA , Kirklin JK , Boll TJ ((2000) ) Neuropsychological functioning among heart transplant candidates: A case control study. J Clin Exp Neuropsychol 22: , 95–103. |
[68] | Roman DD , Kubo SH , Ormaza S , Francis GS , Bank AJ , Shumway SJ ((1997) ) Memory improvement following cardiac transplantation. J Clin Exp Neuropsychol 19: , 692–697. |
[69] | Zuccala G , Cattel C , Manes-Gravina E , Di Niro MG , Cocchi A , Bernabei R ((1997) ) Left ventricular dysfunction: A clue to cognitive impairment in older patients with heart failure. J Neurol Neurosurg Psychiatry 63: , 509–512. |
[70] | Shang S , Liu Z , Gao J , Wang J , Lu W , Fei Y , Zhang B , Mi B , Li P , Ma L , Jiang Y , Chen C , Dang L , Liu J , Qu Q ((2022) ) The relationship between pre-existing coronary heart disease and cognitive impairment is partly explained by reduced left ventricular ejection fraction in the subjects without clinical heart failure: A cross-sectional study. Front Hum Neurosci 16: , 835900. |
[71] | Alosco ML , Hayes SM ((2015) ) Structural brain alterations in heart failure: A review of the literature and implications for risk of Alzheimer’s disease. Heart Fail Rev 20: , 561–571. |
[72] | Canu E , McLaren DG , Fitzgerald ME , Bendlin BB , Zoccatelli G , Alessandrini F , Pizzini FB , Ricciardi GK , Beltramello A , Johnson SC , Frisoni GB ((2010) ) Microstructural diffusion changes are independent of macrostructural volume loss in moderate to severe Alzheimer’s disease. J Alzheimers Dis 19: , 963–976. |
[73] | Woo MA , Macey PM , Fonarow GC , Hamilton MA , Harper RM ((2003) ) Regional brain gray matter loss in heart failure. J Appl Physiol 95: , 677–684. |
[74] | Woo MA , Kumar R , Macey PM , Fonarow GC , Harper RM ((2009) ) Brain injury in autonomic, emotional, and cognitive regulatory areas in patients with heart failure. J Card Fail 15: , 214–223. |
[75] | Van der Werf YD , Witter MP , Uylings HB , Jolles J ((2000) ) Neuropsychology of infarctions in the thalamus: A review. Neuropsychologia 38: , 613–627. |
[76] | Hayes SM , Salat DH , Verfaellie M ((2012) ) Default network connectivity in medial temporal lobe amnesia. J Neurosci 32: , 14622–14629. |
[77] | Qiu C , Winblad B , Marengoni A , Klarin I , Fastbom J , Fratiglioni L ((2006) ) Heart failure and risk of dementia and Alzheimer disease: A population-based cohort study. Arch Intern Med 166: , 1003–1008. |
[78] | Manemann SM , Knopman DS , St Sauver J , Bielinski SJ , Chamberlain AM , Weston SA , Jiang R , Roger VL ((2022) ) Alzheimer’s disease and related dementias and heart failure: A community study. J Am Geriatr Soc 70: , 1664–1672. |
[79] | Adelborg K , Horváth-Puhó E , Ording A , Pedersen L , Sørensen HT , Henderson VW ((2017) ) Heart failure and risk of dementia: A Danish nationwide population-based cohort study. Eur J Heart Fail 19: , 253–260. |
[80] | Tai XY , Veldsman M , Lyall DM , Littlejohns TJ , Langa KM , Husain M , Ranson J , Llewellyn DJ ((2022) ) Cardiometabolic multimorbidity, genetic risk, and dementia: A prospective cohort study. Lancet Healthy Longev 3: , E428–E436. |
[81] | Knecht S , Oelschläger C , Duning T , Lohmann H , Albers J , Stehling C , Heindel W , Breithardt G , Berger K , Ringelstein EB , Kirchhof P , Wersching H ((2008) ) Atrial fibrillation in stroke-free patients is associated with memory impairment and hippocampal atrophy. Eur Heart J 29: , 2125–2132. |
[82] | Thacker EL , McKnight B , Psaty BM , Longstreth WT Jr , Sitlani CM , Dublin S , Arnold AM , Fitzpatrick AL , Gottesman RF , Heckbert SR ((2013) ) Atrial fibrillation and cognitive decline: A longitudinal cohort study. Neurology 81: , 119–125. |
[83] | Elias MF , Sullivan LM , Elias PK , Vasan RS , D’Agostino RB Sr , Seshadri S , Au R , Wolf PA , Benjamin EJ ((2006) ) Atrial fibrillation is associated with lower cognitive performance in the Framingham offspring men. J Stroke Cerebrovasc Dis 15: , 214–222. |
[84] | Kalantarian S , Stern TA , Mansour M , Ruskin JN ((2013) ) Cognitive impairment associated with atrial fibrillation: A meta-analysis. Ann Intern Med 158: , 338–346. |
[85] | Wozakowska-Kaplon B , Opolski G , Kosior D , JaskulskaNiedziela E , Maroszynska-Dmoch E , Wlosowicz M ((2009) ) Cognitive disorders in elderly patients with permanent atrial fibrillation. Kardiol Pol 67: , 487–493. |
[86] | Dublin S , Anderson ML , Haneuse SJ , Heckbert SR , Crane PK , Breitner JC , McCormick W , Bowen JD , Teri L , McCurry SM , Larson EB ((2011) ) Atrial fibrillation and risk of dementia: A prospective cohort study. J Am Geriatr Soc 59: , 1369–1375. |
[87] | Miyasaka Y , Barnes ME , Petersen RC , Cha SS , Bailey KR , Gersh BJ , Casaclang-Verzosa G , Abhayaratna WP , Seward JB , Iwasaka T , Tsang TS ((2007) ) Risk of dementia in stroke-free patients diagnosed with atrial fibrillation: Data from a community-based cohort. Eur Heart J 28: , 1962–1967. |
[88] | Ott A , Breteler MM , de Bruyne MC , van Harskamp F , Grobbee DE , Hofman A ((1997) ) Atrial fibrillation and dementia in a population-based study. The Rotterdam Study. Stroke 28: , 316–321. |
[89] | Lip GY , Piotrponikowski P , Andreotti F , Anker SD , Filippatos G , Homma S , Morais J , Pullicino P , Rasmussen LH , Marín F , Lane DA ; Heart Failure Association (EHFA) of the European Society of Cardiology (ESC) and the ESC Working Group on Thrombosis ((2012) ) Thromboembolism and antithrombotic therapy for heart failure in sinus rhythm: An executive summary of a joint consensus document from the ESC Heart Failure Association and the ESC Working Group on Thrombosis. Thromb Haemost 108: , 1009–1022. |
[90] | Russell D ((2002) ) Cerebral microemboli and cognitive impairment. J Neurol Sci 203–204: , 211–214. |
[91] | Purandare N , Burns A , Daly KJ , Hardicre J , Morris J , Macfarlane G , McCollum C. ((2006) ) Cerebral emboli as a potential cause of Alzheimer’s disease and vascular dementia: Case-control study. BMJ 332: , 1119–1124. |
[92] | Wolf PA ((2012) ) Contributions of the Framingham Heart Study to stroke and dementia epidemiologic research at 60 years. Arch Neurol 69: , 567–571. |
[93] | Roberts RO , Knopman DS , Geda YE , Cha RH , Roger VL , Petersen RC ((2010) ) Coronary heart disease is associated with non-amnestic mild cognitive impairment. Neurobiol Aging 31: , 1894–1902. |
[94] | Stellos K , Katsiki N , Tatsidou P , Bigalke B , Laske C ((2012) ) Association of platelet activation with vascular cognitive impairment: Implications in dementia development? Curr Vasc Pharmacol 12: , 152–154. |
[95] | Beeri MS , Rapp M , Silverman JM , Schmeidler J , Grossman HT , Fallon JT , Purohit DP , Perl DP , Siddiqui A , Lesser G , Rosendorff C , Haroutunian V ((2006) ) Coronary artery disease is associated with Alzheimer disease neuropathology in APOE4 carriers. Neurology 66: , 1399–1404. |
[96] | Vidal JS , Sigurdsson S , Jonsdottir MK , Eiriksdottir G , Thorgeirsson G , Kjartansson O , Garcia ME , van Buchem MA , Harris TB , Gudnason V , Launer LJ ((2010) ) Coronary artery calcium, brain function and structure. Stroke 41: , 891–897. |
[97] | Rosano C , Naydeck B , Kuller LH , Longstreth WT Jr , Newman AB ((2015) ) Coronary artery calcium: Association with brain magnetic resonance imaging abnormalities and cognitive status. J Am Geriatr Soc 53: , 609–615. |
[98] | Ikram MA , Hollander M , Bos MJ , Kors JA , Koudstaal PJ , Hofman A , Witteman JC , Breteler MM ((2006) ) Unrecognized myocardial infarction and the risk of stroke: The Rotterdam Study. Neurology 67: , 1635–1639. |
[99] | Sundbøll J , Horváth-Puhó E , Adelborg K , Schmidt M , Pedersen L , Bøtker HE , Henderson VW , Sørensen HT ((2018) ) Higher risk of vascular dementia in myocardial infarction survivors. Circulation 137: , 567–577. |
[100] | Ikram MA , van Oijen M , de Jong FJ , Kors JA , Koudstaal PJ , Hofman A , Witteman JC , Breteler MM ((2008) ) Unrecognized myocardial infarction in relation to risk of dementia and cerebral small vessel disease. Stroke 39: , 1421–1426. |
[101] | Breteler MM , Claus JJ , Grobbee DE , Hofman A ((1994) ) Cardiovascular disease and distribution of cognitive function in elderly people: The Rotterdam Study. BMJ 308: , 1604–1608. |
[102] | Tini G , Scagliola R , Monacelli F , La Malfa G , Porto I , Brunelli C , Rosa GM ((2020) ) Alzheimer’s Disease and cardiovascular disease: A particular association. Cardiol Res Pract 2020: , 2617970. |
[103] | Leys D , Erkinjuntti T , Desmond DW , Schmidt R , Englund E , Pasquier F , Parnetti L , Ghika J , Kalaria RN , Chabriat H , Scheltens P , Bogousslavsky J ((1999) ) Vascular dementia: The role of cerebral infarcts. Alzheimer Dis Assoc Disord 13: , S38–S48. |
[104] | Breteler MM ((2000) ) Vascular risk factors for Alzheimer’s disease: An epidemiologic perspective. Neurobiol Aging 21: , 153–160. |
[105] | Honig LS , Tang MX , Albert S , Costa R , Luchsinger J , Manly J , Stern Y , Mayeux R ((2003) ) Stroke and the risk of Alzheimer disease. Arch Neurol 60: , 1707–1712. |
[106] | Zhou J , Yu JT , Wang HF , Meng XF , Tan CC , Wang J , Wang C , Tan L ((2015) ) Association between stroke and Alzheimer’s disease: Systematic review and meta-analysis. J Alzheimers Dis 43: , 479–489. |
[107] | Tsolaki M , Fountoulakis K , Chantzi E , Kazis A ((1997) ) Risk factors for clinically diagnosed Alzheimer’s disease: A case-control study of a Greek population. Int Psychogeriatr 9: , 327–341. |
[108] | Fine JP and Gray RJ ((1999) ) A proportional hazards model for the subdistribution of a competing risk. JASA 94: , 496–509. |
[109] | Pobutsky A , Cuaresma C , Kishaba G , Noble C , Lung E , Castillo E , Villafuerte A ((2015) ) The social, cultural and behavioral determinants of health among Hawaii Filipinos: The Filipino Healthy Communities Project. Calif J Health Promot 13: , 1–12. |
[110] | Hawaii Department of Health ((2011) ) Hawaii Plan for the Prevention of Heart Disease and Stroke. https://health.hawaii.gov/heart-disease-stroke/files/2013/12/HDSP_Plan.pdf. Accessed November 1, 2022. |