Compensatory Mechanisms in Early Alzheimer’s Disease and Clinical Setting: The Need for Novel Neuropsychological Strategies
Abstract
Despite advances in the detection of biomarkers and in the design of drugs that can slow the progression of Alzheimer’s disease (AD), the underlying primary mechanisms have not been elucidated. The diagnosis of AD has notably improved with the development of neuroimaging techniques and cerebrospinal fluid biomarkers which have provided new information not available in the past. Although the diagnosis has advanced, there is a consensus among experts that, when making the diagnosis in a specific patient, many years have probably passed since the onset of the underlying processes, and it is very likely that the biomarkers in use and their cutoffs do not reflect the true critical points for establishing the precise stage of the ongoing disease. In this context, frequent disparities between current biomarkers and cognitive and functional performance in clinical practice constitute a major drawback in translational neurology. To our knowledge, the In-Out-test is the only neuropsychological test developed with the idea that compensatory brain mechanisms exist in the early stages of AD, and whose positive effects on conventional tests performance can be reduced in assessing episodic memory in the context of a dual-task, through which the executive auxiliary networks are ‘distracted’, thus uncover the real memory deficit. Furthermore, as additional traits, age and formal education have no impact on the performance of the In-Out-test.
INTRODUCTION
The initial clinical manifestations of Alzheimer’s disease (AD) have a high degree of overlap with the cognitive characteristics in normal aging. At initial stages, there are often subjective memory complaints (SMC) not evidenced in neuropsychological tests. The first changes, yet subtle, in neuropsychological performance that do not cause a significant impact on daily activities and the way patients relate to others, describe a clinical stage labelled as mild cognitive impairment (MCI), which is currently considered an initial stage in the development of AD.
The proportion of conversion of MCI to AD dementia is highly variable, depends on the diagnosing criteria used and the age groups studied, and whether the data comes from studies of cases in hospital settings versus population studies. It has been reported a 10–15% conversion in a medical setting studies and 0.5 to 10% for population studies [1–3]. In addition, a recent meta-analysis have concluded that the rate of conversion of MCI to dementia is approximately 5% to 10% over a period of 10 years [4].
Until recently, the only paradigm used in neuropsychology for the early diagnosis of AD was the so-called hippocampal amnesia (HA) paradigm, based on the difference in recalling recent material spontaneously or with clues [5]. HA came to be considered the gold standard for the diagnosis of AD. This paradigm has been useful for the differential diagnosis of AD with other forms of dementia and for establishing disease stages, particularly for dementia and MCI. But psychometric and statistical normality has been taken as biological normality, finding a high level of overlap between healthy people with others who, being psychometrically normal for the HA paradigm, convert to dementia in follow-up studies. Efforts have been made to develop new tests that allow discrimination of true cases from controls, yet most of them essentially maintain HA as the definitive paradigm for the diagnosis of AD; basically, by increasing the degree of difficulty in the tests (binding tests), thus leading to more false positives [6, 7].
A plausible explanation for the disparity between cognitive performance and biomarkers is the fact that there are physiological, psychological, and even personal strategic compensatory mechanisms that allow individuals with positive biomarkers for AD to reach the threshold of ‘normality’ in the different classical tests. Therefore, in this article we discuss the need to renew the clinical-neuropsychological approaches to detect AD in its early stages, since the classic tests have been designed with concepts and purposes that are not consistent with the current knowledge of the disease, nor do them have the capacity to detect subtle cognitive differences between normal and early pathological aging. The changes that have occurred in the perception of AD have move from a relatively passive and linear model to a multidimensional and dynamic one, thanks to technological advances and their impact on neurosciences, ranging from connectomics and intercellular relationships to new findings at the subcellular and molecular level.
ALZHEIMER’S DISEASE: CHRONOPATHOLOGICAL TRAITS
Numerous studies indicate that, in general, the chronopathology of AD follows a specific neuropathological sequence. In the initial stages, prior to clinical manifestation of AD formation of neurofibrillary tangles occur at the medial temporal lobe (MTL) in structures such as the hippocampus, parahippocampus, and entorhinal cortex, in addition to amyloid-β (Aβ) deposition in the neocortex [8, 9]. However, new investigations report that parietal lobe is being affected in early stages [10]. The initial stage is followed by a continual, organized, and progressive spreading through adjacent structures until affecting most area of the cortical mantle [8, 9].
The clinical course of AD correlates with the progressive functional and pathological changes in the tissue that starts at the medial temporal areas. Under normal conditions, these areas transfer the information stored in the dorsolateral frontal buffer to a system that rapidly and efficiently makes the information long-lasting [11]. Sequential studies of atrophy by means of neuropathology, computed tomography (CT) and magnetic resonance image (MRI) as well as functional changes with positron emission tomography (PET) with 2-[F-18] fluoro-2-deoxy-D-glucose show temporal variations that relate to the chronological changes in neuropsychological tests, global function scales, daily activities, and instrumental skills [1, 2, 12–14]. Nevertheless, studies detecting amyloid burden with Pittsburgh compound B (PIB-PET) among others are inadequate for the prognosis of the clinical changes because depositions reach a plateau while neurofibrillary tangles, decrease in neurons, and deterioration of cognitive performance continues. The amyloid cascade theory hypothesizes that ‘deposition of Aβ, the main component of the plaques, is the causative agent of Alzheimer’s pathology and that neurofibrillary tangles, cell loss, vascular damage, and dementia, are consequences from this deposition’ [15, 16]. Other studies suggest that the soluble Aβ, in particular the oligomeric form, has the most of toxicity [17]. In contrast to the Aβ cascade, others suggest that the pattern of Aβ aggregation from simple structures, as are the fibrils, to complex structures, as are the plaques, is a neuroprotective response that might be induced by other primary mechanisms [18]. Clearly, despite years of efforts in the identification of causative factors for AD, there are still essential questions regarding the neuropathogenesis of AD that remain to be answered.
BIOMARKERS IN EARLY DIAGNOSIS OF ALZHEIMER’S DISEASE
The incorporation of biomarkers is paramount in the early diagnosis of AD posing a challenge to design sensitive and accurate tools to predict whether a patient with memory complaints will develop AD and subsequent dementia. Many investigations have looked at how changes in the cerebrospinal fluid (CSF), serum, and blood markers and quantitative neuroimaging may predict the risk of conversion from MCI to dementia [19–21]. Collectively, these studies have demonstrated that changes in cerebral atrophy, regional hypometabolism, Aβ42, total tau (t-tau), phosphorylated forms of tau protein (p-tau), amyloid burden and, more recently, tau burden, correlate with clinical signs of AD and specially with MCI [19, 22]. It has been accepted that decreased levels of Aβ42 in CSF, reduced Aβ42/Aβ40 and t-tau/(p-Thr-181)-tau ratios in the CSF, along with increased PET + amyloid burden, as indicators of the disease, including prodromal stage [22]. More precisely, results from prospective studies in large cohorts of AD and MCI subjects with clinical follow-up, have disclosed a very high diagnostic sensitivity for the combination of low Aβ42 and high CSF T-tau/P-tau to predict AD in the prodromal stages of the disease, as well as a considerable specificity to differentiate AD from stable MCI [23]. This study also reported the ability to discriminate AD from other related dementias, such as frontotemporal dementia and Lewy body dementia [23]. The performance of this CSF biomarker combination for prodromal AD detection has been later verified in other large multicenter studies, i.e. ADNI [24] and DESCRIPA [25] studies [22]. However, subsequent and contemporary studies have detected discrepancies and inconsistencies in the cutoff values difficult to standardize, which have raised concerns on the validity of these criteria for the clinical diagnosis of prodromal AD, and most notably for converters to dementia [22]. In an effort to integrate core biomarkers in the definition of AD continuum, the National Institute on Aging and Alzheimer’s Association (NIA-AA) working group, has proposed the A/T/N classification [19]. This classification system integrates proven AD biomarkers in three binary categories: ‘A’ for Aβ (amyloid PET or CSF Aβ42); ‘T,’ for CSF p-tau, or tau PET; and ‘N,’ for neurodegeneration or neuronal injury (FDG-PET, structural MRI, or CSF t-tau) [19]. Nevertheless, although this unbiased A/T/N classification may help to seed consensus on the terminology used along the AD continuum, it is agnostic to the temporal ordering of mechanisms underlying AD pathogenesis from clinically normal to AD dementia and lacks diagnostic correlates. Therefore, A/T/N classification is suited to population studies of cognitive aging, it may only be applicable for staging purposes.
Another level of complexity regarding core AD biomarkers is the fact that changes in CSF biomarkers precede prodromal and preclinical symptoms of the disease [26]. This suggests the existence of ‘hidden clinical signs’ which escape from classical symptoms-based diagnostic criteria of AD. A limitation that is worsened given the variation among different reports, on the clinical cutoff values for CSF levels of Aβ42, t-tau, p-tau, and their ratios, which has led to discrepancies on the criteria to establish the onset and early progression of the disease based on CSF biomarkers [2, 12, 14].
Numerous evidence accumulated over the last decades have disclosed the great complexity of AD affecting different cellular and subcellular components in brain grey matter, but also the involvement of white matter [27]. This explains why the spectrum of new fluid biomarkers tracking non-Aβ and non-tau pathology in AD has increased enormously. New potential biomarkers detecting AD-related alterations include a wide spectrum of cellular processes, such neuroinflammation (e.g., progranulin, β2-microglobulin, sTREM2, YKL-40, interleukins 1, 6, 10, and 15), neurodegeneration- and synapse-related (e.g., neurofilament light polypeptide, neurogranin, secretogranin, SAP25, chromogranin A, and visinin-like protein), lipid dyshomeostasis (e.g., ApoE4 isoforms, fatty acid-binding protein 3, oxidized LCPUFA, and oxysterols), protein clearance (e.g., transthyretin, clusterin), and signalosome-associated protein complexes (i.e., ERα, VDAC, flotillin-1, prion protein), among many others [28, 29]. Indeed, the list of promising non-Aβ and non-tau-related candidates as biomarkers for AD, which have proven to be altered at some stage of the disease, increases steadily but, in most cases studies are limited to reduced number of patients, without precise selection of susceptible groups, and mostly lack validation in population studies. The current view is that combinations of subsets of new non-Aβ and non-tau biomarkers might enhance the diagnostic characterization of AD-associated pathological changes along the different stages of the disease [28]. These new potential biomarkers will not only allow a more accurate early detection of AD but also will aid to delineate the multifactorial nature and likely causal factors of sporadic AD.
BRAIN CONECTIVITY AND COMPENSATORY MECHANISMS
The emerging field of brain connectomics has endeavored a complete mapping of all brain connections [30, 31] to delve into its structural organization (connectome) and functional roles (functional connectome) to support higher cortical functions, such as memory, language, or consciousness, as well as their alterations under different psychiatric and neurological disorders [32–43]. The current view is that despite the extreme complexity of brain connectivity, there exist discrete centralized and highly connected attractors, designed as hubs, which collectively make the connectome a highly efficient communication network with discrete number of physical connectors to other hubs [44]. There are different subsystems in the human brain that are connected by hubs, which are related to different sensory, motor, and cognitive functions [45]. One such hub is the hippocampus that belongs to the default mode network (DMN) which, in turn, is structurally and functionally connected to other hubs located in the anterior and posterior cingulate, precuneus, and parietal regions [45, 46]. DMN acts as an attractor that turns on at resting when other systems are off and turns off when these are activated. DMN is also one of functional networks where changes due to degenerative diseases are usually found [37, 46].
According to the Schaefer’s model [30], main functional subsystems include: dorsal attention network (DAN), ventral attention network, visual network, auditory network, senso-motor network, salience network, and DMN, all of which exhibit different degrees of higher-order interconnections. Thus, alterations at different levels of the connectome are likely to have a proportional impact on higher cortical functions, depending on the degree and strength of interconnection [41, 43].
Changes in the connectome have been reported during normal aging. Some reports have shown that these alterations occur in critical points and relatively stable networks, such DMN, which plays an key role both in communication between different networks and in adaptation to aging and resistance to degenerative processes [47, 48]. Thus, an increase in connective strength in the DMN domain associated with normal aging has been recently reported [48]. Interestingly, even though older subjects present less integration, this increase in connectivity follow specific pathways in the transition to aging [47, 48]. Besides DMN, the DAN is a major functional network linked to cognitive processes. DAN plays an important role not only in attention but is also involved in multisensory integration with strong connections during aging [37]. Moreover, this relationship between the DMN and DAN is known to play a crucial role in conscious awareness and to be an essential neural substrate for flexibly allocating attentional resources [49] and to serve as the basic connection pattern in the processing of different cognitive functions [50]. Different studies have shown existence of an intrinsic anticorrelation between these DMN and DAN networks in healthy individuals, which is thought to represent a major contributor to normal cognitive function [50]. Indeed, a stronger DAN-DMN anticorrelation has been associated with an index of efficient cognitive processing [51], and it seems that abnormal change in the intrinsic DMN and DAN anticorrelation might underlie cognitive deficits in certain psychiatric and neurologic disorders, including Parkinson’s disease, or AD [52]. Currently, the abnormal anticorrelation between the DAN and DMN is believed to constitute a mechanism for cognitive impairment. A recent study found that the dysfunctional anticorrelation between the DAN and DMN in MCI may have a large impact on behavioral performance, and that the dysconnectivity between the DAN and DMN might be a potential biomarker for evaluating cognitive decline in patients progressing to AD [49].
In addition to the expected decrease in the brain neuronal population in AD patients, different authors have reported increased strength in certain connections or hyperactivity in different cortical areas [9–13, 27, 47, 53, 54]. For instance, strong connections between the poor intraconnected precuneus and the inferior parietal lobes has been demonstrated in cognitively normal elderly subjects who were Aβ+ [53]. A recent study by Ewers at al. [55] showed that patients with AD (either sporadic or by autosomal dominant inheritance) have a slower rate of deterioration when presenting greater functional activity in segregated systems [48, 55, 56]. Noticeably, the authors propose that these findings might reflect an increased cognitive reserve. This proposal is particularly interesting since patients with cognitive impairment have alterations of connectivity and integration of the networks with emphasis on the hubs [55]. Further, hyperconnectivity in the left frontal cortex (LFC) has been reported both in patients with AD [54] and also in asymptomatic subjects but positive for Aβ [57], suggesting a link between Aβ deposition and macroscopic LFC functionality. This is very interesting since the LFC is considered a locus for cognitive reserve and is a hub that interacts and regulates other subsystems involved in compensatory mechanisms. Indeed, it has been shown that LFC connectivity underlies cognitive reserve in prodromal AD [58], and that the increase in connectivity at the LFC level correlates with attenuation of the effects of hippocampal atrophy and the hypometabolism in the precuneus (associated to higher polymodal cognitive cortex), and also with the level of formal education [58]. Further, the authors feature that resting-state connectivity of the left frontal cortex to the DMN and DAN supports reserve in MCI, suggesting the involvement of a rich club as part of the support of cognitive reserve in the early stages of AD [58].
On the other hand, numerous evidence has demonstrated associations between connectivity alterations and Aβ and tau biomarkers [59, 60], as well as with genetics [36]. Aβ initially accumulates in DMN [61, 62] at the level of medial temporal cortex and medial parietal cortex [14, 19, 61] and in the memory system that includes its hub at the level of the MTL. It now seems that although the accumulation of Aβ leads to neuronal hyperactivation, neuronal stimulation also produces an increase in Aβ, and that may even precede the formation of amyloid plaques [63]. There is also evidence that hyperexcitability might promote the propagation of tau pathology from entorhinal cortex to hippocampus and cortex [59, 60, 63]. Similar conclusions regarding the differential association of tau and Aβ to functional network changes using functional MRI have been demonstrated in the aging brain [64].
NEUROPSYCHOLOGY
Cognitive reserve, resilience, and AD
Currently, the terms cognitive reserve and resilience are used in cognitive neuroscience, occupying an important place to explain interindividual differences in cognitive performance when the extent and intensity of brain damage or other parameters such as aging are homogeneous. It can be intuitively understood the terms reserve and resilience as part of everyday physical experiences. From this perspective, the cognitive reserve would be the ‘amount’ of extra cognition, the one that is left over under normal conditions, but which can be used if necessary. Obviously, the brain is not a repository containing a volume of cognition. The same goes for resilience. However, there is no amount of cognition as such and, although there are measurable constructs such as performance on different neuropsychological tests, a difference cannot be measured in terms of the amount of cognition left over. In this sense, some authors have suggested to think of cognitive reserve as a kind of brain ‘software’ whereby individuals who have greater cognitive reserve have a way of processing tasks in a more efficient manner [65].
Superimposed to these concepts, certain indirect features which may affect cognitive skills have been considered. These including years of formal education, head circumference, profession, economic level, spoken languages, dancing, chess playing, and skills with musical instruments, among others. This, in turn, brings up a new dilemma: do those with more years of formal education, polyglots, or those with a higher ELO score in chess have more cognitive reserve than those who lack these conditions and have equal or better performance in adverse situations, with the same degree of brain injury? There is no doubt that the answer is complex, but regardless of the precision that we have, the concept seems to continue to be useful to explain inter-individual differences that cannot be explained by the degrees of injury found, for which great efforts have been made to achieve measurable construct, reliable and valid as well as to achieve a consensus on terminology and that the international community uses the same language [65, 66].
The Collaboratory on Research Definitions for Reserve and Resilience in Cognitive Aging and Dementia, funded by the National Institute on Aging in 2019, proposes resilience as a general concept that encompasses operational definitions of cognitive reserve, brain maintenance and brain reserve. Hence, Stern and collaborators [66] have proposed a framework of operational definitions to homogenize the terminology for characterizing the differential susceptibility to brain aging and disease. Accordingly, Resilience is ‘a general term that subsumes any concept that relates to the capacity of the brain to maintain cognition and function with aging and disease’. Cognitive reserve ‘is a property of the brain that allows for cognitive performance that is better than expected given the degree of life-course related brain changes and brain injury or disease’. Brain maintenance refers to ‘the relative absence of changes in neural resources or neuropathologic change over time as a determinant of preserved cognition in older age’, and finally, Brain reserve ‘the neurobiological status of the brain (numbers of neurons, synapses, etc.) at any time point. It does not involve active adaptation of functional cognitive processes in the presence of injury or disease as does cognitive reserve’ [66].
Despite the operational difficulties that the terms resilience and cognitive reserve entail, there is no doubt that it has opened a path not only to explain the disparate results in cognitive performance between patients with respect to their lesions, but it has also made us aware of the existence of confounding factors for the interpretation of conventional neuropsychological tests and the need to create new paradigms to overcome the biases due to these compensatory mechanisms.
Searching for new paradigms in neuropsychology
Although other deficits and subtle changes in the activities of daily living are present, even at early stages of the disease, it is widely accepted that the cognitive hallmark of AD (MCI due to AD) is the amnestic syndrome of hippocampal type (HA), which may be defined as ‘a recall deficit that does not improve with cueing or recognition procedures, after effective encoding of information’ [5]. Classical test for assessing HA include Free and Cued Selective Reminding Test (FCSRT) [67] and the Rey Auditory Verbal Learning Test [68].
However, to improve sensitivity and specificity of HA-based classical tests in the early diagnosis of AD, their level of difficulty have been increased [67–69], for example, in the Memory Binding Test, 32 items are used compared to the 16 used in the FCSRT. New ways to score them have been proposed after recorded data from retrospective studies as well [70]. On the other hand, new paradigms have been designed to evaluate episodic memory such as the Face-Name test, which is an associative encoding task. This test comprised faces pairs with fictional first names [71]. The authors observed that the pattern on functional MRI activation during the encoding differed between individuals at early stages of AD and controls undergoing normal aging [71]. Interestingly, they also found that test performances associated with amyloid burden in cognitively normal participants [71]. Only individuals with higher education participated in this study, what is a limitation in the external validity [72]. In a recent review by Rubiño et al. [73], the authors report contradictory results in the Face-name retrieval memory test [73].
Other authors have focused their research for the early diagnosis of AD on aspects other than episodic memory, such as working memory, executive functions, language, and semantic memory. Recently, Cejudo et al. [74] published a follow-up study using the Ikos test, based on the ability to make semantic relationships in a selection task with three options where one is the correct. This increases in one option compared to other tests, which assess semantic memory, has allowed the number of items to be reduced and, therefore, the execution time as well. They report excellent discrimination between converters and non-converters [74].
The classical tests capacity HA-based paradigm could be dampened in the early stages of AD because of the presence of compensatory mechanisms. Even though hypertrophy and hyperactivity could be considered paradoxical at the neurodegenerative diseases, they have been found at early stages. This may be the explanation of normal performance in both patients who have fulfill AD criteria and those who show conversion to dementia in longitudinal studies [47, 75–83] with initial hyperactivity in MTL [13, 53, 62, 84] and then other cortical areas being frontals and parietals the most prominent [36, 63, 81].
The hippocampal hyperactivity and hypertrophy and subsequent recruitment of networks related to executive functions could be the cognitive reserve matrix in the early stages of AD. HA-based memory tests could fail due to the presence of these auxiliary executive networks that support the partially damaged memory networks. This possible trade-off may be missed when we assess memory and executive functions separately. Following this order of ideas: a patient in the early AD might be not diagnosed as early stages using conventional tests because they overlook the functional deficits of the memory networks which are relied in executive networks.
Therefore, given the reasoning above, it seems reasonable that an episodic memory task immersed in a dual task context can hamper the compensatory effects of executive networks and thus reveal the underlying mnemonic deficit. Various investigations of memory as a dual task have been performed, particularly for immediate memory including working memory with little success in separating healthy older patients [85, 86]. Since the short-and long-term episodic memory is of greatest relevance in AD, we have designed and validated with classical biomarkers, the In-Out test, which explores episodic memory in a dual task context.
The In-Out-test
The rationale for the design of the In-Out-test was that episodic memory could be more accurately evaluated if non-mnemonic cognitive function could be distracted by a simultaneous executive task [87]. The In-Out-test makes an inference of episodic memory during encoding by proposing a categorization task and memorization of six words simultaneously, which represents a substantial difference compared to other tests which evaluate memory after effective encoding of information [87] (Fig. 1A). More precisely, the overarching structure of In-Out-test includes 1) learning of the Organized Series, 2) memorization the Organized Series, and 3) random recalling of as many words as possible without taking into account the order (Random Memory) [87]. The results obtained in a pilot study (110 subjects, three years successive assessments), predicted conversion to dementia from MCI or SMC with a sensitivity and specificity values of, respectively, 90%, and 94%, being PPV (positive- predictive value) of 0.90 and NPV (negative-predictive value) of 0.94. The results from a subset of subjects who completed the neuropsychological study and donated CSF samples are shown in Fig. 1B and 1C. These results were remarkably better than values reported for other conventional and well-known HA-based memory tests. For instance, PPV values for FCSRT, for conversion to dementia varies between 7% and 30% [88, 89]. This means that between 7% and 30% of the patients with positive results will develop dementia while more than 70% with positive results will not.
Fig. 1
Summary of In-Out-test features and outcomes. A) Work template of In-Out-test (left) with superimposed learning performances of two male patients. Solid black line: 62 years old at basal evaluation: no formal education; In-Out-test total score: 15.2/20 (normal). No dementia after 5 years follows up. Red dashed lines: 60 years old at basal evaluation;>12 years of formal education, three spoken languages; basal In-Out-test score (red): 3.3/20. Developed dementia at month 9 of follow up. Radar plots for In-Out-test outcomes from PROA study. B) Evolution of GDS (1 to 4) for each subject in the two-years follow up. Initial (GDS-i, blue squares) and final (GDS-f, red circles) values for participants diagnosed at the beginning and end of the study. IOT-N: Normalized In-Out test total score (green triangles) as measured at the time of first diagnoses (GDS-i). C) Evolution of Random memory (RAM-N), learning task (Learning-N) and total In-Out (IOT-N) normalized punctuations for patients shown in B. Red squares percent of cases that converted to dementia.
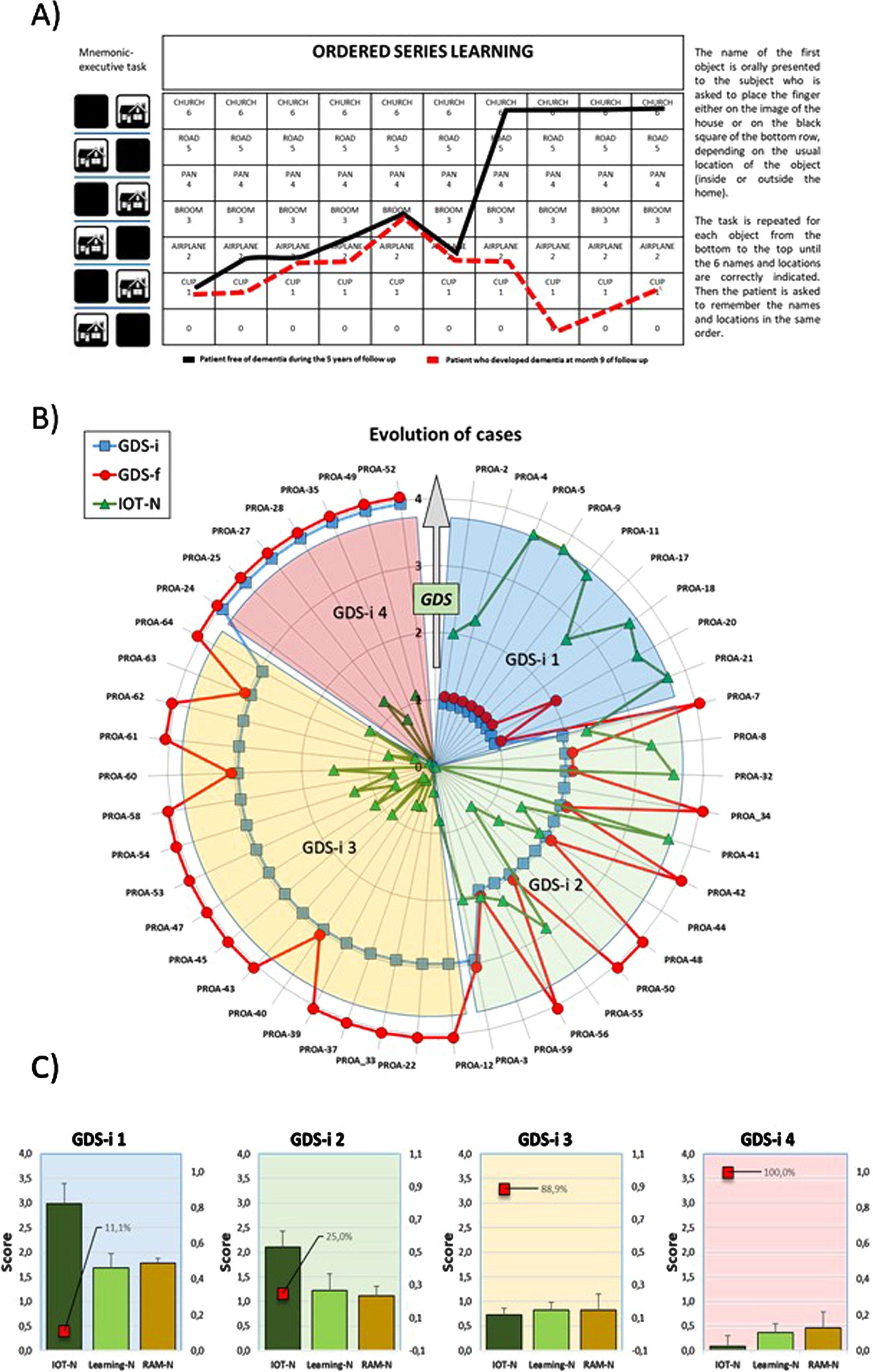
Likewise, the comparative study with biomarkers for the In-Out-test showed sensitivity = 91%; specificity = 77%; PPV = 0.87 and PNV = 0.83. Furthermore, intraclass correlation (ICC) was around 0.7 for In-Out-test in several measurements included p-tau/Aβ42 (in normal patients and Aβ42 in normal, MCI and AD) while ICC for FCSRT in the same patients was less than 0.3 in all groups, indicating that FCSRT is conditioned by large intersubjects variability, which dampens its accuracy value [90]. In-Out-test scores were also related to CSF biomarkers, in particular for p181-tau (Fig. 2A). Hence, a statistically significant positive correlation was found between the In-Out-test and p-tau in subjects with normal neuropsychometry up to a cutoff level of 50 pg/ml (Fig. 1B). However, above 80 pg/ml, the relationship between In-Out-test scores and p-tau became negative and statistically significant. There was a gap between 50 to 80 pg/ml range where no apparent relationship exists between In-Out-test scores and p-tau. These results are particularly interesting, as they agree with some previous reports which have established the cutoff level of 50 pg/ml for patients who convert to MCI or dementia [91]. Moreover, as p-tau levels increase above 80 pg/ml, the massive neuronal loss leads to functional reduction of compensatory mechanisms, making the performance of In-Out-test exclusively dependent on the remaining brain reserve and devoid of resilience. On the other hand, the null correlation in the range 50–80 pg/ml gap reflect a high interindividual variability, which may be interpreted as resulting from MCI and AD patients with different degrees of neuronal loss and reduced compensatory mechanisms typical of aging and others due to AD [90].
Fig. 2
A) Bar chart for relationships between p-tau levels in the CSF and the outcomes of learning task. Bivariate relationships (depicted as dotted lines, along with correlation coefficient r) differed depending on the p-tau range of concentrations (<50, 50–80, and > 80 pg/ml). B) Bivariate plot of In-Out-test punctuations and factor scores for principal component 1 from multivariate analyses of ER-signalosome and scaffolding proteins (modified from reference [29]). C) Bivariate plot of In-Out-test punctuations and factor scores for principal component 1 from multivariate analyses of trace metals, lipoxidative metabolites, antioxidant/detoxifying enzymes (modified from reference [92]). Solid lines in B and C indicate regression lines. 95% CI are represented in dotted lines. r, correlation coefficient; β, regression coefficient.
![A) Bar chart for relationships between p-tau levels in the CSF and the outcomes of learning task. Bivariate relationships (depicted as dotted lines, along with correlation coefficient r) differed depending on the p-tau range of concentrations (<50, 50–80, and > 80 pg/ml). B) Bivariate plot of In-Out-test punctuations and factor scores for principal component 1 from multivariate analyses of ER-signalosome and scaffolding proteins (modified from reference [29]). C) Bivariate plot of In-Out-test punctuations and factor scores for principal component 1 from multivariate analyses of trace metals, lipoxidative metabolites, antioxidant/detoxifying enzymes (modified from reference [92]). Solid lines in B and C indicate regression lines. 95% CI are represented in dotted lines. r, correlation coefficient; β, regression coefficient.](https://content.iospress.com:443/media/adr/2023/7-1/adr-7-1-adr220116/adr-7-adr220116-g002.jpg)
To our knowledge, the In-Out-test is the only neuropsychological test created with the idea that compensatory brain mechanisms exist in the early stages of AD and whose positive effects on conventional test performance can be reduced in an episodic memory task in the context of a dual-task, through which the executive auxiliary networks are ‘distracted’, thus uncovering the real memory deficit. Furthermore, as additional traits, age and formal education have no impact on the performance of the In-Out-test.
Several studies have demonstrated the presence of significant changes in CSF biochemistry in association with early stages of cognitive complaints or decline, even before changes in the levels of classical CSF AD biomarkers can be accurately detected. As these changes do not necessarily lead to AD-type dementia, the question arises of how these alterations may be predictive of a neurodegenerative outcome. We have observed such alterations in neurolipids (docosahexaenoic acid, docosapentaenoic acid), ER-signalosome multiprotein complex (including the estrogen receptor alpha (Erα), insulin-like growth factor 1 receptor beta (IGF-1Rβ), prion protein (PrP), voltage-dependent anion channel 1 (VDAC1)), scaffolding proteins (caeolin-1 and flotilin-1), trace biometals (Cu, Se, Zn, Mn) and related molecules (transferrin), antioxidant/detoxifying enzyme activities (superoxide dismutase (SOD), glutathione peroxidase 4, glutathione-S-transferase, butyrylcholinesterase), and also in oxidative stress-related metabolites (malondialdehyde) reflected in the CSF of individuals diagnosed SMC or MCI [29, 92]. The essential concept of these observations is that these changes reflect biochemical changes occurring in the brain parenchyma in aged individual during initial stages of cognitive alterations, irrespective of whether subjects will develop any type of dementia. Indeed, as observed in Fig. 2B and 2C, highly significant relationships between factors scores obtained by multivariate factor analyses and the In-Out-test punctuations in two different biochemical approaches [29, 92]. In the first one (Fig. 2B), based on the ER-signalosome multiprotein complex [29], factor scores from principal component 1 were highly determined by ERα and IGF-1Rβ as CSF protein markers and IGF-1Rβ/Flotilin-1 and PrP/flotillin-1 as protein ratios. PC1 displayed a significant negative relationship with In-Out scores, with group subjects consistently distributed along the regression line (Fig. 2B). In the second approach, based on CSF contents of trace biometals, antioxidant/detoxifying enzymes, and oxidative stress-related metabolites [92], PC1 (which was mostly determined by Zn, Cu, Se, and SOD) was positively related to In-Out scores (Fig. 2C). Interestingly, neither of these outcomes were conditioned by changes in the CSF levels of tau or Aβ. These results strongly indicate the usefulness In-Out-test as predictive tools for the clinical diagnosis of initial stages of cognitive decline.
ACKNOWLEDGMENTS
We appreciate the helpful intervention of Sofía Torrealba-García y Daniela Torrealba-García during critical moments in the elaboration of this manuscript.
FUNDING
The authors have no funding to report.
CONFLICT OF INTEREST
The authors have no conflict of interest to report.
REFERENCES
[1] | Petersen RC , Smith GE , Waring SC , Ivnik RJ , Tangalos EG , Kokmen E ((1999) ) Mild cognitive impairment: Clinical characterization and outcome. Arch Neurol 56: , 303–308. |
[2] | Larrieu S , Letenneur L , Orgogozo JM , Fabrigoule C , Amieva H , Le Carret N , Barberger-Gateau P , Dartigues JF ((2002) ) Incidence and outcome of mild cognitive impairment in a population-based prospective cohort. Neurology 59: , 1594–1599. |
[3] | Visser PJ , Kester A , Jolles J , Verhey F ((2006) ) Ten-year risk of dementia in subjects with mild cognitive impairment. Neurology 67: , 1201–1207. |
[4] | Mitchell AJ , Shiri-Feshki M ((2009) ) Rate of progression of mild cognitive impairment to dementia–meta-analysis of 41 robust inception cohort studies. Acta Psychiatr Scand 119: , 252–265. |
[5] | Dubois B , Feldman HH , Jacova C , Hampel H , Molinuevo JL , Blennow K , DeKosky ST , Gauthier S , Selkoe D , Bateman R , Cappa S , Crutch S , Engelborghs S , Frisoni GB , Fox NC , Galasko D , Habert M-O , Jicha GA , Nordberg A , Pasquier F , Rabinovici G , Robert P , Rowe C , Salloway S , Sarazin M , Epelbaum S , de Souza LC , Vellas B , Visser PJ , Schneider L , Stern Y , Scheltens P , Cummings JL ((2014) ) Advancing research diagnostic criteria for Alzheimer’s disease: The IWG-2 criteria. Lancet Neurol 13: , 614–629. |
[6] | Mowrey WB , Lipton RB , Katz MJ , Ramratan WS , Loewenstein DA , Zimmerman ME , Buschke H ((2018) ) Memory Binding Test predicts incident dementia: Results from the Einstein Aging Study. J Alzheimers Dis 62: , 293–304. |
[7] | Matias-Guiu JA , Cabrera-Martín MN , Curiel RE , Valles-Salgado M , Rognoni T , Moreno-Ramos T , Carreras JL , Loewenstein DA , Matías-Guiu J ((2018) ) Comparison between FCSRT and LASSI-L to detect early stage Alzheimer’s disease. J Alzheimers Dis 61: , 103–111. |
[8] | Braak H , Braak E ((1995) ) Staging of Alzheimer’s disease-related neurofibrillary changes. Neurobiol Aging 16: , 271–278. |
[9] | Nelson PT , Braak H , Markesbery WR ((2009) ) Neuropathology and cognitive impairment in Alzheimer disease: A complex but coherent relationship. J Neuropathol Exp Neurol 68: , 1–14. |
[10] | Bruner E , Jacobs HIL ((2013) ) Alzheimer’s disease: The downside of a highly evolved parietal lobe? J Alzheimers Dis 35: , 227–240. |
[11] | Dickerson BC , Eichenbaum H ((2010) ) The episodic memory system: Neurocircuitry and disorders. Neuropsychopharmacology 35: , 86–104. |
[12] | Bobinski M , Wegiel J , Wisniewski HM , Tarnawski M , Reisberg B , Mlodzik B , de Leon MJ , Miller DC ((1995) ) Atrophy of hippocampal formation subdivisions correlates with stage and duration of Alzheimer disease. Dementia 6: , 205–210. |
[13] | Putcha D , Brickhouse M , O’Keefe K , Sullivan C , Rentz D , Marshall G , Dickerson B , Sperling R ((2011) ) Hippocampal hyperactivation associated with cortical thinning in Alzheimer’s disease signature regions in non-demented elderly adults. J Neurosci 31: , 17680–17688. |
[14] | Engler H , Forsberg A , Almkvist O , Blomquist G , Larsson E , Savitcheva I , Wall A , Ringheim A , Långström B , Nordberg A ((2006) ) Two-year follow-up of amyloid deposition in patients with Alzheimer’s disease. Brain 129: , 2856–2866. |
[15] | Hardy JA , Higgins GA ((1992) ) Alzheimer’s disease: The amyloid cascade hypothesis. Science 256: , 184–185. |
[16] | Hardy J ((2009) ) The amyloid hypothesis for Alzheimer’s disease: A critical reappraisal. J Neurochem 110: , 1129–1134. |
[17] | Lambert MP , Barlow AK , Chromy BA , Edwards C , Freed R , Liosatos M , Morgan TE , Rozovsky I , Trommer B , Viola KL , Wals P , Zhang C , Finch CE , Krafft GA , Klein WL ((1998) ) Diffusible, nonfibrillar ligands derived from Abeta1-42 are potent central nervous system neurotoxins. Proc Natl Acad Sci U S A 95: , 6448–6453. |
[18] | Castellani RJ , Lee H , Siedlak SL , Nunomura A , Hayashi T , Nakamura M , Zhu X , Perry G , Smith MA ((2009) ) Reexamining Alzheimer’s disease: Evidence for a protective role for amyloid-beta protein precursor and amyloid-beta. J Alzheimers Dis 18: , 447–452. |
[19] | Jack CR Jr, Bennett DA , Blennow K , Carrillo MC , Feldman HH , Frisoni GB , Hampel H , Jagust WJ , Johnson KA , Knopman DS , Petersen RC , Scheltens P , Sperling RA , Dubois B ((2016) ) A/T/N: An unbiased descriptive classification scheme for Alzheimer disease biomarkers. Neurology 87: , 539–547. |
[20] | Ottoy J , Niemantsverdriet E , Verhaeghe J , De Roeck E , Struyfs H , Somers C , wyffels L , Ceyssens S , Van Mossevelde S , Van den Bossche T , Van Broeckhoven C , Ribbens A , Bjerke M , Stroobants S , Engelborghs S , Staelens S ((2019) ) Association of short-term cognitive decline and MCI-to-AD dementia conversion with CSF, MRI, amyloid- and 18 F-FDG-PET imaging. NeuroImage Clin 22: , 101771. |
[21] | Platero C , Tobar MC ((2021) ) Predicting Alzheimer’s conversion in mild cognitive impairment patients using longitudinal neuroimaging and clinical markers. Brain Imaging Behav 15: , 1728–1738. |
[22] | Blennow K , Zetterberg H ((2018) ) Biomarkers for Alzheimer’s disease: Current status and prospects for the future. J Intern Med 284: , 643–663. |
[23] | Hansson O , Zetterberg H , Buchhave P , Londos E , Blennow K , Minthon L ((2006) ) Association between CSF biomarkers and incipient Alzheimer’s disease in patients with mild cognitive impairment: A follow-up study. Lancet Neurol 5: , 228–234. |
[24] | Shaw LM , Vanderstichele H , Knapik-Czajka M , Clark CM , Aisen PS , Petersen RC , Blennow K , Soares H , Simon A , Lewczuk P , Dean R , Siemers E , Potter W , Lee VMY , Trojanowski JQ ((2009) ) Cerebrospinal fluid biomarker signature in Alzheimer’s disease neuroimaging initiative subjects. Ann Neurol 65: , 403–413. |
[25] | Visser PJ , Verhey F , Knol DL , Scheltens P , Wahlund LO , Freund-Levi Y , Tsolaki M , Minthon L , Wallin AK , Hampel H , Bürger K , Pirttila T , Soininen H , Rikkert MO , Verbeek MM , Spiru L , Blennow K ((2009) ) Prevalence and prognostic value of CSF markers of Alzheimer’s disease pathology in patients with subjective cognitive impairment or mild cognitive impairment in the DESCRIPA study: A prospective cohort study. Lancet Neurol 8: , 619–627. |
[26] | Moghekar A , Li S , Lu Y , Li M , Wang M-C , Albert M , O’Brien R , BIOCARD Research Team ((2013) ) CSF biomarker changes precede symptom onset of mild cognitive impairment. Neurology 81: , 1753–1758. |
[27] | Ferrer I ((2022) ) Alzheimer’s disease is an inherent, natural part of human brain aging: An integrated perspective. Free Neuropathol 3: , 17. |
[28] | Park SA , Han SM , Kim CE ((2020) ) New fluid biomarkers tracking non-amyloid-β and non-tau pathology in Alzheimer’s disease. Exp Mol Med 52: , 556–568. |
[29] | Mesa-Herrera F , Marín R , Torrealba E , Santos G , Díaz M ((2022) ) Neuronal ER-signalosome proteins as early biomarkers in prodromal Alzheimer’s disease independent of amyloid-β production and tau phosphorylation. Front Mol Neurosci 15: , 879146. |
[30] | Schaefer A , Kong R , Gordon EM , Laumann TO , Zuo X-N , Holmes AJ , Eickhoff SB , Yeo BTT ((2018) ) Local-global parcellation of the human cerebral cortex from intrinsic functional connectivity MRI. Cereb Cortex 28: , 3095–3114. |
[31] | Rojkova K , Volle E , Urbanski M , Humbert F , Dell’Acqua F , Thiebaut de Schotten M ((2016) ) Atlasing the frontal lobe connections and their variability due to age and education: A spherical deconvolution tractography study. Brain Struct Funct 221: , 1751–1766. |
[32] | Van Den Heuvel MP , Fornito A ((2014) ) Brain networks in schizophrenia. Neuropsychol Rev 24: , 32–48. |
[33] | Aruldass AR , Kitzbichler MG , Morgan SE , Lim S , Lynall ME , Turner L , Vertes P , Cavanagh J , Cowen P , Pariante CM , Harrison NA , Bullmore ET ((2021) ) Dysconnectivity of a brain functional network was associated with blood inflammatory markers in depression. Brain Behav Immun 98: , 299–309. |
[34] | Bullmore E , Sporns O ((2012) ) The economy of brain network organization. Nat Rev Neurosci 13: , 336–349. |
[35] | Zhang K , Sejnowski TJ ((2000) ) A universal scaling law between gray matter and white matter of cerebral cortex. Proc Natl Acad Sci U S A 97: , 5621–5626. |
[36] | Yu M , Sporns O , Saykin AJ ((2021) ) The human connectome in Alzheimer disease—relationship to biomarkers and genetics. Nat Rev Neurol 17: , 545–563. |
[37] | Watanabe H , Bagarinao E , Maesawa S , Hara K , Kawabata K , Ogura A , Ohdake R , Shima S , Mizutani Y , Ueda A , Ito M , Katsuno M , Sobue G ((2021) ) Characteristics of neural network changes in normal aging and early dementia. Front Aging Neurosci 13: , 747359. |
[38] | Pistono A , Pariente J , Bézy C , Lemesle B , Le Men J , Jucla M ((2019) ) What happens when nothing happens? An investigation of pauses as a compensatory mechanism in early Alzheimer’s disease. Neuropsychologia 124: , 133–143. |
[39] | Liloia D , Mancuso L , Uddin LQ , Costa T , Nani A , Keller R , Manuello J , Duca S , Cauda F ((2021) ) Gray matter abnormalities follow non-random patterns of co-alteration in autism: Meta-connectomic evidence. Neuroimage Clin 30: , 102583. |
[40] | Zhang J , Cortese R , De Stefano N , Giorgio A ((2021) ) Structural and functional connectivity substrates of cognitive impairment in multiple sclerosis. Front Neurol 12: , 671894. |
[41] | Berthier ML , García-Casares N , Walsh SF , Nabrozidis A , Ruíz de Mier RJ , Green C , Dávila G , Gutiérrez A , Pulvermüller F ((2011) ) Recovery from post-stroke aphasia: Lessons from brain imaging and implications for rehabilitation and biological treatments. Discov Med 12: , 275–289. |
[42] | Aracil-Bolaños I , Sampedro F , Pujol J , Soriano-Mas C , Gónzalez-de-Echávarri JM , Kulisevsky J , Pagonabarraga J ((2021) ) The impact of dopaminergic treatment over cognitive networks in Parkinson’s disease: Stemming the tide? Hum Brain Mapp 42: , 5736–5746. |
[43] | Bejr-kasem H , Pagonabarraga J , Martínez-Horta S , Sampedro F , Marín-Lahoz J , Horta-Barba A , Aracil-Bolaños I , Pérez-Pérez J , Ángeles Botí M , Campolongo A , Izquierdo C , Pascual-Sedano B , Gómez-Ansón B , Kulisevsky J ((2019) ) Disruption of the default mode network and its intrinsic functional connectivity underlies minor hallucinations in Parkinson’s disease. Mov Disord 34: , 78–86. |
[44] | van den Heuvel MP , Bullmore ET , Sporns O ((2016) ) Comparative connectomics. Trends Cogn Sci 20: , 345–361. |
[45] | Thomas Yeo BT , Krienen FM , Sepulcre J , Sabuncu MR , Lashkari D , Hollinshead M , Roffman JL , Smoller JW , Zöllei L , Polimeni JR , Fisch B , Liu H , Buckner RL ((2011) ) The organization of the human cerebral cortex estimated by intrinsic functional connectivity. J Neurophysiol 106: , 1125–1165. |
[46] | Sperling RA , LaViolette PS , O’Keefe K , O’Brien J , Rentz DM , Pihlajamaki M , Marshall G , Hyman BT , Selkoe DJ , Hedden T , Buckner RL , Becker JA , Johnson KA ((2009) ) Amyloid deposition is associated with impaired default network function in older persons without dementia. Neuron 63: , 178–188. |
[47] | Rao SM , Bonner-Jackson A , Nielson KA , Seidenberg M , Smith JC , Woodard JL , Durgerian S ((2015) ) Genetic risk for Alzheimer’s disease alters the five-year trajectory of semantic memory activation in cognitively intact elders. Neuroimage 111: , 136–146. |
[48] | Moretto M , Silvestri E , Zangrossi A , Corbetta M , Bertoldo A ((2022) ) Unveiling whole-brain dynamics in normal aging through Hidden Markov Models. Hum Brain Mapp 43: , 1129–1144. |
[49] | Wang J , Liu J , Wang Z , Sun P , Li K , Liang P ((2019) ) Dysfunctional interactions between the default mode network andthe dorsal attention network in subtypes of amnestic mild cognitive impairment. Aging (Albany) 11: , 9147–9166. |
[50] | Dixon ML , Andrews-Hanna JR , Spreng RN , Irving ZC , Mills C , Girn M , Christoff K ((2017) ) Interactions between the default network and dorsal attention network vary across default subsystems, time, and cognitive states. Neuroimage 147: , 632–649. |
[51] | Clare Kelly AM , Uddin LQ , Biswal BB , Castellanos FX , Milham MP ((2008) ) Competition between functional brain networks mediates behavioral variability. Neuroimage 39: , 527–537. |
[52] | Whitfield-Gabrieli S , Ford JM ((2012) ) Default mode network activity and connectivity in psychopathology. Annu Rev Clin Psychol 8: , 49–76. |
[53] | Nakamura A , Cuesta P , Kato T , Arahata Y , Iwata K , Yamagishi M , Kuratsubo I , Kato K , Bundo M , DIers K , Fernández A , Maestú F , Ito K ((2017) ) Early functional network alterations in asymptomatic elders at risk for Alzheimer’s disease. Sci Rep 7: , 6517. |
[54] | Peineau S , Rabiant K , Pierrefiche O , Potier B ((2018) ) Synaptic plasticity modulation by circulating peptides and metaplasticity: Involvement in Alzheimer’s disease. Pharmacol Res 130: , 385–401. |
[55] | Ewers M , Luan Y , Frontzkowski L , Neitzel J , Rubinski A , Dichgans M , Hassenstab J , Gordon BA , Chhatwal JP , Levin J , Schofield P , Benzinger TLS , Morris JC , Goate A , Karch CM , Fagan AM , McDade E , Allegri R , Berman S , Chui H , Cruchaga C , Farlow M , Graff-Radford N , Jucker M , Lee JH , Martins RN , Mori H , Perrin R , Xiong C , Rossor M , Fox NC , O’Connor A , Salloway S , Danek A , Buerger K , Bateman RJ , Habeck C , Stern Y , Franzmeier N ((2021) ) Segregation of functional networks is associated with cognitive resilience in Alzheimer’s disease. Brain 144: , 2176–2185. |
[56] | Wu Z , Gao Y , Potter T , Benoit J , Shen J , Schulz PE , Zhang Y ((2021) ) Interactions between aging and Alzheimer’s disease on structural brain networks. Front Aging Neurosci 13: , 639795. |
[57] | Franzmeier N , Düzel E , Jessen F , Buerger K , Levin J , Duering M , Dichgans M , Haass C , Suárez-Calvet M , Fagan AM , Paumier K , Benzinger T , Masters CL , Morris JC , Perneczky R , Janowitz D , Catak C , Wolfsgruber S , Wagner M , Teipel S , Kilimann I , Ramirez A , Rossor M , Jucker M , Chhatwal J , Spottke A , Boecker H , Brosseron F , Falkai P , Fliessbach K , Heneka MT , Laske C , Nestor P , Peters O , Fuentes M , Menne F , Priller J , Spruth EJ , Franke C , Schneider A , Kofler B , Westerteicher C , Speck O , Wiltfang J , Bartels C , Araque Caballero MÁ , Metzger C , Bittner D , Weiner M , Lee JH , Salloway S , Danek A , Goate A , Schofield PR , Bateman RJ , Ewers M ((2018) ) Left frontal hub connectivity delays cognitive impairment in autosomal-dominant and sporadic Alzheimer’s disease. Brain 141: , 1186–1200. |
[58] | Franzmeier N , Duering M , Weiner M , Dichgans M , Ewers M ((2017) ) Left frontal cortex connectivity underlies cognitive reserve in prodromal Alzheimer disease. Neurology 88: , 1054–1061. |
[59] | Hasani SA , Mayeli M , Salehi MA , Barzegar Parizi R ((2021) ) A systematic review of the association between amyloid-β and τ pathology with functional connectivity alterations in the alzheimer dementia spectrum utilizing PET Scan and rsfMRI. Dement Geriatr Cogn Dis Extra 11: , 78–90. |
[60] | King-Robson J , Wilson H , Politis M ((2021) ) Associations between amyloid and tau pathology, and connectome alterations, in Alzheimer’s disease and mild cognitive impairment. J Alzheimers Dis 82: , 541–560. |
[61] | Small SA , Schobel SA , Buxton RB , Witter MP , Barnes CA ((2011) ) A pathophysiological framework of hippocampal dysfunction in ageing and disease. Nat Rev Neurosci 12: , 585–601. |
[62] | Bagarinao E , Watanabe H , Maesawa S , Mori D , Hara K , Kawabata K , Yoneyama N , Ohdake R , Imai K , Masuda M , Yokoi T , Ogura A , Taoka T , Koyama S , Tanabe HC , Katsuno M , Wakabayashi T , Kuzuya M , Ozaki N , Hoshiyama M , Isoda H , Naganawa S , Sobue G ((2019) ) Reorganization of brain networks and its association with general cognitive performance over the adult lifespan. Sci Rep 9: , 11352. |
[63] | Maestú F , de Haan W , Busche MA , DeFelipe J ((2021) ) Neuronal excitation/inhibition imbalance: Core element of a translational perspective on Alzheimer pathophysiology. Ageing Res Rev 69: , 101372. |
[64] | Sepulcre J , Sabuncu MR , Li Q , El Fakhri G , Sperling R , Johnson KA ((2017) ) Tau and amyloid β proteins distinctively associate to functional network changes in the aging brain. Alzheimers Dement 13: , 1261–1269. |
[65] | Stern Y ((2002) ) What is cognitive reserve? Theory and research application of the reserve concept. J Int Neuropsychol Soc 8: , 448–460. |
[66] | Stern Y , Albert M , Barnes C , Cabeza R , Pascual-Leone A , Rapp P ((2023) ) A framework for concepts of reserve and resilience in aging. Neurobiol Aging 124: , 100–110. |
[67] | Grober E , Buschke H , Korey SR ((1987) ) Genuine memory deficits in dementia. Dev Neuropsychol 3: , 13–36. |
[68] | Rey A ((1941) ) L’examen psychologique dans les cas d’encephalopathie traumatique. Arch Psychol (Geneve) 28: , 286–340. |
[69] | Gramunt N , Sánchez-Benavides G , Buschke H , Lipton RB , Masramon X , Gispert JD , Peña-Casanova J , Fauria K , Molinuevo JL ((2016) ) Psychometric properties of the Memory Binding Test: Test-retest reliability and convergent validity. J Alzheimers Dis 50: , 999–1010. |
[70] | Grober E , Veroff AE , Lipton RB ((2018) ) Temporal unfolding of declining episodic memory on the Free and Cued Selective Reminding Test in the predementia phase of Alzheimer’s disease: Implications for clinical trials. Alzheimers Dement (Amst) 10: , 161–171. |
[71] | Rentz DM , Amariglio RE , Becker JA , Frey M , Olson LE , Frishe K , Carmasin J , Maye JE , Johnson KA , Sperling RA ((2011) ) Face-name associative memory performance is related to amyloid burden in normal elderly. Neuropsychologia 49: , 2776–2783. |
[72] | Papp KV , Amariglio RE , Dekhtyar M , Roy K , Wigman S , Bamfo R , Sherman J , Sperling RA , Rentz DM ((2014) ) Development of a psychometrically equivalent short form of the face-name associative memory exam for use along the early Alzheimer’s disease trajectory. Clin Neuropsychol 28: , 771–785. |
[73] | Rubiño J , Andrés P ((2018) ) The Face-Name Associative Memory test as a tool for early diagnosis of Alzheimer’s disease. Front Psychol 9: , 1464. |
[74] | Cejudo JC , Samaniego M , Almeria M , Castrillo S , Medina L , Gil D ((2022) ) Ikos Test: New tool for the assessment of semantic knowledge in early Alzheimer disease. J Alzheimers Dis 90: , 151–160. |
[75] | Cohen AD , Price JC , Weissfeld LA , James J , Rosario BL , Bi W , Nebes RD , Saxton JA , Snitz BE , Aizenstein HA , Wolk DA , Dekosky ST , Mathis CA , Klunk WE ((2009) ) Basal cerebral metabolism may modulate the cognitive effects of Abeta in mild cognitive impairment: An example of brain reserve. J Neurosci 29: , 14770–14778. |
[76] | Belleville S , Clément F , Mellah S , Gilbert B , Fontaine F , Gauthier S ((2011) ) Training-related brain plasticity in subjects at risk of developing Alzheimer’s disease. Brain 134: , 1623–1634. |
[77] | Bondi MW , Houston WS , Eyler LT , Brown GG ((2005) ) fMRI evidence of compensatory mechanisms in older adults at genetic risk for Alzheimer disease. Neurology 64: , 501–508. |
[78] | Grady CL , McIntosh AR , Beig S , Keightley ML , Burian H , Black SE ((2003) ) Evidence from functional neuroimaging of a compensatory prefrontal network in Alzheimer’s disease. J Neurosci 23: , 986–993. |
[79] | Sperling RA , Dickerson BC , Pihlajamaki M , Vannini P , LaViolette PS , Vitolo OV , Hedden T , Becker JA , Rentz DM , Selkoe DJ , Johnson KA ((2010) ) Functional alterations in memory networks in early Alzheimer’s disease. Neuromolecular Med 12: , 27–43. |
[80] | Buldú JM , Bajo R , Maestú F , Castellanos N , Leyva I , Gil P , Sendiña-Nadal I , Almendral JA , Nevado A , del-Pozo F , Boccaletti S ((2011) ) Reorganization of functional networks in mild cognitive impairment. PLoS One 6: , e19584. |
[81] | Maestú F , Fernández A , Simos PG , Gil-Gregorio P , Amo C , Rodriguez R , Arrazola J , Ortiz T ((2001) ) Spatio-temporal patterns of brain magnetic activity during a memory task in Alzheimer’s disease. Neuroreport 12: , 3917–22. |
[82] | Celone KA , Calhoun VD , Dickerson BC , Atri A , Chua EF , Miller SL , DePeau K , Rentz DM , Selkoe DJ , Blacker D , Albert MS , Sperling RA ((2006) ) Alterations in memory networks in mild cognitive impairment and Alzheimer’s disease: An independent component analysis. J Neurosci 26: , 10222–10231. |
[83] | Dickerson BC , Salat DH , Greve DN , Chua EF , Rand-Giovannetti E , Rentz DM , Bertram L , Mullin K , Tanzi RE , Blacker D , Albert MS , Sperling RA ((2005) ) Increased hippocampal activation in mild cognitive impairment compared to normal aging and AD. Neurology 65: , 404–411. |
[84] | Sheng X , Chen H , Shao P , Qin R , Zhao H , Xu Y , Bai F ((2021) ) Brain structural network compensation is associated with cognitive impairment and Alzheimer’s disease pathology. Front Neurosci 15: , 630278. |
[85] | MacPherson SE , Della Sala S , Logie RH ((2004) ) Dual-task interference of encoding and retrieval processes in healthy and impaired working memory. Cortex 40: , 183–184. |
[86] | Foley JA , Kaschel R , Logie RH , Della Sala S ((2011) ) Dual-task performance in Alzheimer’s disease, mild cognitive impairment, and normal ageing. Arch Clin Neuropsychol 26: , 340–348. |
[87] | Torrealba E , Garcia-Morales P , Cejudo JC , Gramunt N , Sánchez-García F , Fabre-Pi O (2012) Inout test: Diagnosis of potential converters from mild cognitive impairment and subjective complaints to dementia by a new paradigm. In 12th International Stockholm/Springfield Symposium on Advances in Alzheimer Therapy, Stockholm, pp. 261. |
[88] | Auriacombe S , Helmer C , Amieva H , Berr C , Dubois B , Dartigues JF ((2010) ) Validity of the free and cued selective reminding test in predicting dementia: The 3C study. Neurology 74: , 1760–1767. |
[89] | Derby CA , Burns LC , Wang C , Katz MJ , Zimmerman ME , L’italien G , Guo Z , Berman RM , Lipton RB ((2013) ) Screening for predementia AD: Time-dependent operating characteristics of episodic memory tests. Neurology 80: , 1307–1314. |
[90] | Torrealba E , Garcia-Morales P , Cejudo JC , Diaz M , Rodriguez-Esparragon F , Fabre O , Mesa-Herrera F , Marin R , Sanchez-Garcia F , Rodriguez-Perez A , Gramunt N ((2019) ) In-Out-Test: A new paradigm for sorting the wheat from the chaff in prodromal Alzheimer’s disease. J Alzheimers Dis 67: , 265–277. |
[91] | Seppälä TT , Koivisto AM , Hartikainen P , Helisalmi S , Soininen H , Herukka S-K ((2011) ) Longitudinal changes of CSF biomarkers in Alzheimer’s disease. J Alzheimers Dis 25: , 583–594. |
[92] | Mesa-Herrera F , Marín R , Torrealba E , Díaz M ((2022) ) Multivariate assessment of lipoxidative metabolites, trace biometals, and antioxidant and detoxifying activities in the cerebrospinal fluid define a fingerprint of preclinical stages of Alzheimer’s disease. J Alzheimers Dis 86: , 387–402. |