Application of Structural Retinal Biomarkers to Detect Cognitive Impairment in a Primary Care Setting
Abstract
Background:
Despite the diagnostic accuracy of advanced neurodiagnostic procedures, the detection of Alzheimer’s disease (AD) remains poor in primary care. There is an urgent need for screening tools to aid in the detection of early AD.
Objective:
This study examines the predictive ability of structural retinal biomarkers in detecting cognitive impairment in a primary care setting.
Methods:
Participants were recruited from Alzheimer’s Disease in Primary Care (ADPC) study. As part of the ADPC Retinal Biomarker Study (ADPC RBS), visual acuity, an ocular history questionnaire, eye pressure, optical coherence tomography (OCT) imaging, and fundus imaging was performed.
Results:
Data were examined on n = 91 participants. The top biomarkers for predicting cognitive impairment included the inferior quadrant of the outer retinal layers, all four quadrants of the peripapillary retinal nerve fiber layer, and the inferior quadrant of the macular retinal nerve fiber layer.
Conclusion:
The current data provides strong support for continued investigation into structural retinal biomarkers, particularly the retinal nerve fiber layer, as screening tools for AD.
INTRODUCTION
Alzheimer’s disease (AD) is the most prevalent form of dementia and a leading cause of death in the elderly. The prevalence of AD will increase 4-fold by 2050, making this disease a serious threat to our healthcare systems [1]. Currently, AD is clinically diagnosed by physician exam, lab work, neurocognitive tests, and magnetic resonance imaging (MRI), which is cost- and time-ineffective. Amyloid positron emission tomography (PET) scans and cerebrospinal fluid (CSF) amyloid confirmatory biomarkers have received FDA approval; however, these modalities are very costly, invasive, and pose substantial access barriers [2]. We have previously proposed that a multi-tiered neurodiagnostic approach that begins in primary care will be of tremendous benefit to the field and follows the models utilized in oncology, cardiology, infectious disease, and other fields [2].
Despite primary care providers being regulated to conduct cognitive examinations per the annual wellness visit, there are no rapid and cost-effective tools available to the providers [3, 4]. The Alzheimer’s Disease in Primary Care (ADPC) study was recently funded explicitly to examine biomarkers for detecting AD, preclinical AD (amyloid positive+normal cognition) and prodromal AD (amyloid positive+mild cognitive impairment) directly within primary care settings. The Alzheimer’s Disease in Primary Care Retinal Biomarker Study (ADPC RBS) is an expansion of the ADPC study and leverages the infrastructure and data to test the hypothesis that structural retinal biomarkers can detect cognitive impairment in primary care settings.
Several studies have found that retinal biomarkers can be used to effectively detect AD. The retina is a projection of the central nervous system (CNS) via the optic nerve; thus, it shares many features with the cerebral cortex, including embryologic origin and blood barriers [5].
Unlike the CNS, the retina can be visualized non-invasively through an ophthalmological examination. Recent advances in ophthalmologic imaging technology have considerably improved the ability to visualize detailed structure and function of the retina in vivo. Consequently, the eye has become an attractive target for detection of neurodegeneration in AD and has potential to serve as an accessible, cost-efficient screening tool for detecting cognitive impairment.
Over the past several decades, research in this area has demonstrated a clear association between retinal and cerebral changes in AD. While numerous studies have demonstrated structural, vascular, and/or proteomic retinal changes in AD, others have failed to establish significant differences in retinal findings between AD patients and cognitively normal individuals [6]. These inconclusive findings may be due to variations in study design and lack of standardization across methodology and data analysis [6]. Further, the majority of studies in this area are cross-sectional in nature, and longitudinal studies are needed to study structural changes in early disease states (preclinical AD and mild cognitive impairment (MCI)). Despite the rapidly growing literature on retinal imaging biomarkers of preclinical AD, prodromal AD, and AD, to date, the utility of retinal imaging as part of a multi-tiered neurodiagnostic process beginning in primary care remains unknown. The goal of the present study is to determine the predictive ability of structural retinal biomarkers to identify cognitive impairment (MCI and AD).
MATERIALS AND METHODS
Participants
Data were analyzed on 91 participants aged 60 and above with and without cognitive impairment (normal control n = 59; MCI n = 26; AD n = 6). This study leverages the ADPC Study (R01AG058537), the first-ever study to directly examine blood-based biomarkers as the first step in a multi-tiered neurodiagnostic process for screening AD in primary care settings. Participants in ADPC were recruited from the University of North Texas Health Science Center (UNTHSC) Department of Family Medicine and Community-Based Primary Care for the Elderly Program. Recruitment for ADPC is based on a referral from a primary care provider; this design was created to align with how a screening test would be implemented within primary care settings. Further, the ADPC study demographic characteristics are designed to reflect those of the Dallas-Fort Worth Metroplex (and the demographics of the U.S. by 2050). The ADPC Retinal Biomarker Study (ADPC RBS) is actively recruiting n = 200 ADPC participants who consented for re-contact and meet the inclusion and exclusion criteria.
ADPC RBS has an IRB-approved protocol with each eligible participant (and/or their informant) providing written informed consent. All procedures were done in accord with the Helsinki Declaration of 1975.
Participant screening
As part of the ADPC protocol, all participants undergo the following: 1) medical and functional exam, 2) clinical interview (medical history, medications, etc.), 3) neuropsychological testing, 4) 3T MRI of the brain using ADNI3 protocols [7], 5) Amyloid PET scan using NeuraCeq per ADNI3 protocols [7], and 6) venipuncture for biobanking purposes. An informant interview was also conducted for completion of the Clinical Dementia Rating (CDR) scale by clinicians with expertise in dementia to evaluate for functional declines.
For the ADPC RBS study, inclusion criteria included completion of the ADPC study and consent for re-contact. Exclusion criteria included: high intraocular pressure defined as greater than or equal to 30 mmHg in either eye, history of adverse effects with pupillary dilation, known hypersensitivity to tropicamide or any ingredient in the formulation, active ocular infection or inflammation, history of angle closure glaucoma, or having undergone ocular surgery within the prior 6 months.
Diagnostic classification
Cognitive diagnoses were assigned algorithmically (decision tree) and verified at consensus review by an expert in the field of dementia as follows: Cognitively Unimpaired (CU) = no cognitive complaints, CDR sum of boxes score of 0 and cognitive test scores broadly within normal limits (i.e., performance greater than that defined as meeting diagnostic criteria for MCI [i.e.,< = 1.5 standard deviations below the normative range]). Of note, participants with an isolated cognitive test score 1.5 SD below adjusted z-scores, who had no cognitive or functional complaints were assigned as CU; Mild Cognitive Impairment (MCI): cognitive complaints (self or other), CDR sum of boxes score between 0.5-2.0 and at least one cognitive test score falling 1.5 standard deviations below normative ranges; Dementia: CDR sum of boxes score> = 2.5 and at least two cognitive test scores 2 standard deviations below normative ranges. In this study, we examined participants classified in the following groups: cognitively impaired (MCI+AD), and cognitively unimpaired.
Ophthalmological assessment
In addition to a detailed ocular history, participants underwent the following ophthalmological examinations at their study visit: best-corrected distance visual acuity, intraocular pressure (IOP), fundus photography, and optical coherence tomography (OCT). One drop of tropicamide 1.0% ophthalmic solution was administered in each eye prior to retinal imaging. All fundus images and OCT scans were assessed by an experienced therapeutic optometrist for unexpected pathology and research diagnoses. Participants with ophthalmological conditions that interfered with image acquisition or image quality were excluded from analysis (cataract, macular hole, diabetic macular edema, etc.).
Optical coherence tomography
Following pupil dilation, the Heidelberg SPECTRALIS Spectral Domain Optical Coherence Tomography (SD-OCT) Plus with BluePeak and NSite Analytics software was used to obtain retinal scans of the optic nerve and macula in the left and right eyes of all participants.
Our imaging protocol followed that proposed by Alber et al. as a minimum dataset framework for SD-OCT image acquisition [8]. The SPECTRALIS has integrated quality control measures that account for image signal to noise ratio. The TruTrack Active Eye Tracking technology ensures high-quality, high-resolution images with reduced noise, and images were reviewed for a minimum quality signal value of 20. Outcome measures included volume and thickness of the total retina, and each retinal neuronal layer through layer segmentation, including: peripapillary retinal nerve fiber layer (pRNFL), macular retinal nerve fiber layer (mRNFL), ganglion cell layer (GCL), inner plexiform layer (IPL), inner nuclear layer, outer plexiform layer, outer nuclear layer, inner and outer photoreceptor segments, and the retinal pigment epithelium. Additionally, macular GC-IPL, macular ganglion cell complex, and the photoreceptor layer were analyzed, as recent studies show these areas are more sensitive indications of neurodegenerative disease than the pRNFL reduction [9, 10]. The mean macular thickness of each retinal layer was averaged across four quadrants extending 3.45 mm from the center of the fovea. The volumes for all layers were calculated separately across the entire macular region. In addition to individual retinal neuronal layer volumes, we expressed the volume of each individual layer as a ratio of the total retinal volume and thickness for each eye. Prior work has demonstrated that volumetric RNFL changes have the potential to represent systemic changes in preclinical AD [11]; thus, the ratio for each neuronal cell layer across both eyes was averaged. Lastly, to ensure measurement of a systemic retinal neuronal layer volume change, a preliminary analysis was conducted to assess for asymmetry between eyes for each layer.
Statistical analyses
Analyses were conducted in R statistical software (v4.1.3). Mean group differences in demographic factors (age, gender, education) between those with and without cognitive impairment were conducted using ANOVA and Chi-square analyses. Random Forest (RF) (v4.7-1) was used as a primary classifier model for distinguishing cognitive impairment (MCI and dementia) using each of the retinal biomarkers as predictors in the models. Given the discovery nature of this preliminary study, all markers were utilized, and subsequent analyses were run using only the top markers from the variable importance plots. The sample size was not sufficient for cross-validation and, therefore, the current results are viewed as discovery.
Analyses were conducted separated by ethnicity (White, Black, Asian).
RESULTS
A total of 94 participants were enrolled in the present study; three subjects were excluded because of poor image quality or the presence of eye disease that affected the analysis. Thus, 91 participants (59 cognitively unimpaired, 32 cognitively impaired (26 MCI, 6 AD)) were included in the preliminary analysis. Table 1 provides the descriptive characteristics of the cohort. Cognitively impaired cases were classified as being significantly older (p = 0.005) as compared to cognitively unimpaired cases. Women made up most of the total sample and were more prevalent in the cognitively unimpaired group than the cognitively impaired group. Gender, race, education, and ethnicity were not significantly different among the two groups.
Fig.1
ADPC RBS visit protocol. ADPC RBS, Alzheimer’s Disease in Primary Care Retinal Biomarker Study; IOP, intraocular pressure.
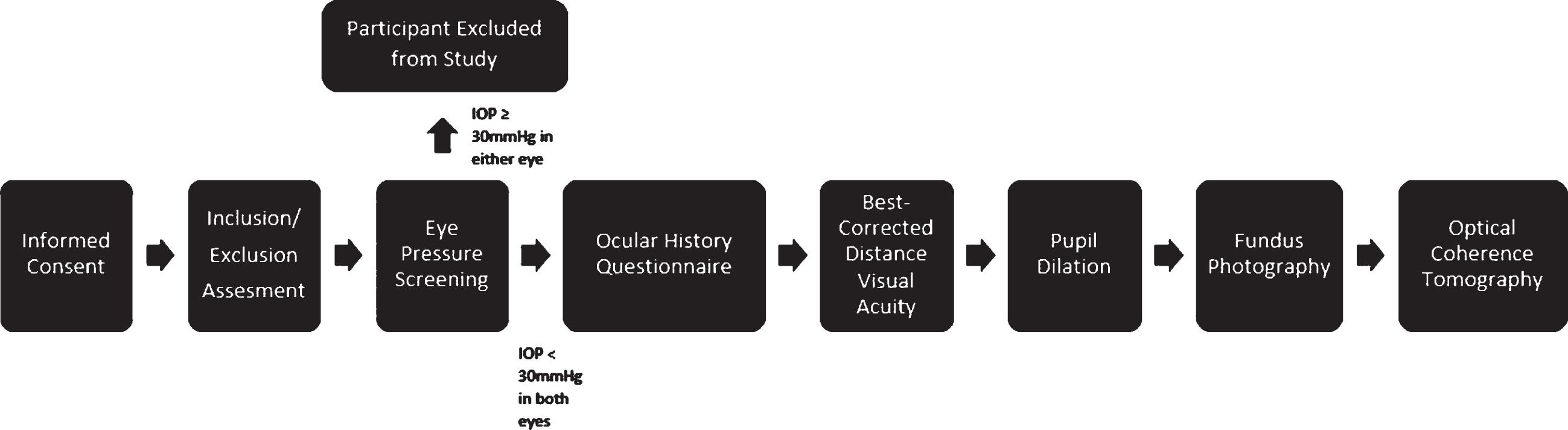
Table 1
Demographic data for ADPC RBS cohort
Cognitively Impaired | Cognitively | p | |||
(MCI+AD) | Unimpaired | ||||
N = 32 | N = 59 | ||||
Age, mean (SD) | 72.9 (7.6) | 70.8±4.4 | 0.0056 | ||
Education, y mean (SD) | 14.8 (3.1) | 16.3±2.6 | 0.2684 | ||
Sex, N (%) | Male | 13 (41%) | Male | 17 (29%) | 0.3504 |
Female | 19 (59%) | Female | 42 (71%) | ||
Ethnicity, N (%) | White | 23 (72%) | White | 52 (88%) | 0.4637 |
Black | 8 (25%) | Black | 6 (10%) | ||
Asian | 1 (3%) | Asian | 1 (2%) |
ADPC RBS, Alzheimer’s Disease in Primary Care Retinal Biomarker Study; MCI, mild cognitive impairment; AD, Alzheimer’s disease.
Due to sample size, MCI and dementia were combined into a cognitively impaired group for the purpose of this analysis. In the initial RF analyses, using a cut-off of 0.5, there was a 100% classification accuracy of cognitive impairment (MCI/dementia) cases (AUC = 1.00, SN = 1.00, SP = 1.00). Analyses were re-run using only the top markers from the variable importance plots. The top retinal structural biomarkers for discriminating cases (MCI/dementia) versus cognitively unimpaired were identified as the inferior quadrant of the outer retinal layer thickness, pRNFL thickness in all 4 quadrants, and mRNFL, particularly in the inferior quadrant. When separated further, diagnostic classification accuracy remained unchanged (100% classification accuracy) for the MCI group compared to the combined cognitively impaired group (AUC = 1.00, SN = 1.00, SP = 1.00). The top markers in distinguishing cognitively unimpaired individuals from MCI were identified as inferior thickness of the outer retinal layers, pRNFL thickness in all 4 quadrants, particularly the superior temporal quadrant, inferior mRNFL thickness and inferior GCL thickness.
The diagnostic classification accuracy was slightly lower for AD (98.4%) compared to the combined model. When detecting dementia, SN = 0.83, SP = 1.00, and AUC = 1.00 (1 false negative identified). The top markers for distinguishing cognitively unimpaired individuals from AD were identified as mRNFL parameters, particularly temporal mRNFL thickness and the inferior quadrant of inner retinal layer thickness.
DISCUSSION
This study was the first to examine the utility of retinal biomarkers in distinguishing cognitive impairment in a primary care setting. Our findings demonstrate a strong importance of structural retinal biomarkers, particularly all four quadrants of the pRNFL, in distinguishing those with cognitive impairment from those cognitively unimpaired. This corroborates prior work in this area, which has consistently demonstrated RNFL thinning in AD patients [12–14]. Several meta-analyses have found preferential thinning of the inner retinal layers in AD compared to healthy controls, most notably the RNFL, GCL, and IPL [15, 16]. It is important to note that some changes have been noted in the outer retinal layers, although they remain inconclusive [17]. In our preliminary analysis, we discovered the inferior quadrant of the outer retinal layers held the strongest importance value in distinguishing cognitive impairment. This warrants further analysis in understanding the utility of outer retinal layers in predicting AD disease state.
The pRNFL contains the ganglion cell axons around the optic nerve and has been the most examined retinal biomarker in AD discovery [18]. Additionally, there has been an established association between pRNFL thickness and brain volume, particularly the hippocampal and entorhinal cortex volume, suggesting the relevance of this retinal biomarker in distinguishing cognitive impairment [19]. The results of our study indicated that while all four quadrants of the pRNFL are highly important biomarkers for identifying those with cognitive impairment, the inferior and superior quadrants displayed higher relative importance compared to the temporal and nasal quadrants. This is consistent with some previous work which also has shown greater thinning in the superior and inferior quadrants in AD patients compared to healthy controls [20–22]. Because the superior and inferior quadrants contain the highest concentration of axon bundles, initial neurodegenerative changes may be first identified in these quadrants.
Our study also revealed mRNFL to be a variable high in importance in models of cognitive dysfunction, although it did not have as high of a relative importance in the model as global pRNFL parameters. When examining the diagnostic groups separately, our findings revealed the possibility that different structural biomarkers are more accurate in identifying MCI compared to AD. While both pRNFL and mRNFL demonstrated strong importance values in detecting cognitive impairment, pRNFL held greater importance in discriminating disease state in MCI and mRNFL was more important in discriminating disease state in AD compared to cognitively unimpaired controls. In contrast, several studies have showed axonal loss in the mRNFL to precede pRNFL degeneration in earlier disease states (preclinical AD and MCI), which is consistent with the knowledge that the mRNFL contains more than half of the total retinal ganglion cells and may be more susceptible to early degenerative changes [23, 24]. The characterization and evolution of these changes, specifically during earlier stages of cognitive impairment, remains uncertain and future studies with standardized methodologies are needed in order to better understand these relationships.
Our study has several limitations. First, the sample of participants with AD was small, limiting the results in comparing the different diagnostic categories (unimpaired, MCI, AD). To address this, we combined the cognitively impaired groups to examine promising findings regarding the utility of retinal biomarkers in detecting cognitive impairment. We are still recruiting participants into this study and plan to examine the findings within our larger cohort in the future. Second, because our study was cross-sectional in nature, we cannot draw conclusions about progression of RNFL thinning with disease state. Future longitudinal studies are needed to understand the diagnostic utility of these retinal neural structural parameters and their association with the progression of AD.
Despite these limitations, our results show that structural retinal parameters, specifically the RNFL thickness, hold strong predictive ability in distinguishing cognitive impairment in our multi-ethnic cohort. As previously mentioned, this is the first study to examine these structural retinal biomarkers in a primary care setting, with models reflecting how it could be employed as a screening tool in practice. These preliminary findings suggest that the RNFL is a promising screening tool for identifying individuals needing further confirmatory diagnostic testing for cognitive impairment and more research is needed to examine volume and thickness changes in the mRNFL and pRNFL over the AD diagnostic spectrum.
In summary, there is an urgent need for a multistage neurodiagnostic screening systems for detecting cognitive loss particularly in primary care settings. If validated, retinal biomarkers can serve as part of a multi-tiered neurodiagnostic process to determine which patients should undergo more invasive and expensive confirmatory procedures. The availability of such a biomarker could be of tremendous value for screening into novel clinical trials as well as increase access to disease modifying treatments once available.
ACKNOWLEDGMENTS
This work would not be possible without the support of the Institute of Translational Research at the University of North Texas Health Science Center.
FUNDING
Research reported in this publication was supported by the National Institute of Aging (NIA) of the National Institutes of Health under Award Number R01AG058537. This study was also funded by the NIA under Award Number U24AG065204, through the Clinician-Scientists Transdisciplinary Aging Research (Clin-STAR) pilot grant, as well as funding from intramural seed grants from the University of North Texas Health Science Center Department of Pharmacology & Neuroscience. The content is solely the responsibility of the authors and does not necessarily represent the official views of the National Institutes of Health.
CONFLICT OF INTEREST
The authors declare no conflicts of interest.
REFERENCES
[1] | Brookmeyer R , Johnson E , Ziegler-Graham K , Arrighi HM ((2007) ) Forecasting the global burden of Alzheimer’s disease. Alzheimers Dement 3: , 186–191. |
[2] | O’Bryant SE , Edwards M , Johnson L , Hall J , Villarreal AE , Britton GB , Quiceno M , Cullum CM , Graff-Radford NR ((2016) ) A blood screening test for Alzheimer’s disease. Alzheimers Dement (Amst) 3: , 83–90. |
[3] | Boustani M , Callahan CM , Unverzagt FW , Austrom MG , Perkins AJ , Fultz BA , Hui SL , Hendrie HC ((2005) ) Implementing a screening and diagnosis program for dementia in primary care. J Gen Intern Med 20: , 572–577. |
[4] | Cotter VT ((2006) ) Alzheimer’s disease: Issues and challenges in primary care, Nurs Clin North Am 41: 83–93, vi. |
[5] | London A , Benhar I , Schwartz M ((2013) ) The retina as a window to the brain-from eye research to CNS disorders. Nat Rev Neurol 9: , 44–53. |
[6] | Alber J , Goldfarb D , Thompson LI , Arthur E , Hernandez K , Cheng D , DeBuc DC , Cordeiro F , Provetti-Cunha L , den Haan J , Van Stavern GP , Salloway SP , Sinoff S , Snyder PJ ((2020) ) Developing retinal biomarkers for the earliest stages of Alzheimer’s disease: What we know, what we don’t, and how to move forward. Alzheimers Dement 16: , 229–243. |
[7] | Jack CR , Jr , Barnes J , Bernstein MA , Borowski BJ , Brewer J , Clegg S , Dale AM , Carmichael O , Ching C , DeCarli C , Desikan RS , Fennema-Notestine C , Fjell AM , Fletcher E , Fox NC , Gunter J , Gutman BA , Holland D , Hua X , Insel P , Kantarci K , Killiany RJ , Krueger G , Leung KK , Mackin S , Maillard P , Malone IB , Mattsson N , McEvoy L , Modat M , Mueller S , Nosheny R , Ourselin S , Schuff N , Senjem ML , Simonson A , Thompson PM , Rettmann D , Vemuri P , Walhovd K , Zhao Y , Zuk S , Weiner M ((2015) ) Magnetic resonance imaging in Alzheimer’s Disease Neuroimaging Initiative 2. Alzheimers Dement 11: , 740–756. |
[8] | Alber J , Arthur E , Sinoff S , DeBuc DC , Chew EY , Douquette L , Hatch WV , Hudson C , Kashani A , Lee CS , Montaquila S , Mozdbar S , Cunha LP , Tayyari F , Van Stavern G , Snyder PJ ((2020) ) A recommended “minimum data set” framework for SD-OCT retinal image acquisition and analysis from the Atlas of Retinal Imaging in Alzheimer’s Study (ARIAS). ,, Alzheimers Dement (Amst) 12: , e12119. |
[9] | Tan O , Chopra V , Lu AT , Schuman JS , Ishikawa H , Wollstein G , Varma R , Huang D ((2009) ) Detection of macular ganglion cell loss in glaucoma by Fourier-domain optical coherence tomography. Ophthalmology 116: , 2305-2314e2301–2302. |
[10] | Mwanza JC , Durbin MK , Budenz DL , Sayyad FE , Chang RT , Neelakantan A , Godfrey DG , Carter R , Crandall AS ((2012) ) Glaucoma diagnostic accuracy of ganglion cell-inner plexiform layer thickness: Comparis with nerve fiber layer and optic nerve head. Ophthalmology 119: , 1151–1158. |
[11] | Snyder PJ , Johnson LN , Lim YY , Santos CY , Alber J , Maruff P , Fernandez B ((2016) ) Nonvascular retinal imaging markers of preclinical Alzheimer’s disease. Alzheimers Dement (Amst) 4: , 169–178. |
[12] | Garcia-Martin ES , Rojas B , Ramirez AI , de Hoz R , Salazar JJ , Yubero R , Gil P , Trivino A , Ramirez JM ((2014) ) Macular thickness as a potential biomarker of mild Alzheimer’s disease. ,, Ophthalmology 121: , 1149–1151 e1143. |
[13] | Cheung CY , Ong YT , Hilal S , Ikram MK , Low S , Ong YL , Venketasubramanian N , Yap P , Seow D , Chen CL , Wong TY ((2015) ) Retinal ganglion cell analysis using high-definition optical coherence tomography in patients with mild cognitive impairment and Alzheimer’s disease. J Alzheimers Dis 45: , 45–56. |
[14] | Mendez-Gomez JL , Rougier MB , Tellouck L , Korobelnik JF , Schweitzer C , Delyfer MN , Amieva H , Dartigues JF , Delcourt C , Helmer C ((2017) ) Peripapillary retinal nerve fiber layer thickness and the evolutionof cognitive performance in an elderly population. FrontNeurol 8: , 93. |
[15] | den Haan J , Verbraak FD , Visser PJ , Bouwman FH ((2017) ) Retinal thickness in Alzheimer’s disease: A systematic review and meta-analysis. Alzheimers Dement (Amst) 6: , 162–170. |
[16] | Salobrar-Garcia E , Hoyas I , Leal M , de Hoz R , Rojas B , Ramirez AI , Salazar JJ , Yubero R , Gil P , Trivino A , Ramirez JM ((2015) ) Analysis of retinal peripapillary segmentation in early Alzheimer’s disease patients. Biomed Res Int 2015: , 636548. |
[17] | Zhang Y , Wang Y , Shi C , Shen M , Lu F ((2021) ) Advances in retina imaging as potential biomarkers for early diagnosis of Alzheimer’s disease. Transl Neurodegener 10: , 6. |
[18] | Majeed A , Marwick B , Yu H , Fadavi H , Tavakoli M ((2021) ) Ophthalmic biomarkers for Alzheimer’s disease: A review. Front Aging Neurosci 13: , 720167. |
[19] | Tao R , Lu Z , Ding D , Fu S , Hong Z , Liang X , Zheng L , Xiao Y , Zhao Q ((2019) ) Perifovea retinal thickness as an ophthalmic biomarker for mild cognitive impairment and early Alzheimer’s disease. Alzheimers Dement (Amst) 11: , 405–414. |
[20] | Iseri PK , Altinas O , Tokay T , Yuksel N ((2006) ) Relationship between cognitive impairment and retinal morphological and visual functional abnormalities in Alzheimer disease. J Neuroophthalmol 26: , 18–24. |
[21] | Berisha F , Feke GT , Trempe CL , McMeel JW , Schepens CL ((2007) ) Retinal abnormalities in early Alzheimer’s disease. Invest Ophthalmol Vis Sci 48: , 2285–2289. |
[22] | Lu Y , Li Z , Zhang X , Ming B , Jia J , Wang R , Ma D ((2010) ) Retinal nerve fiber layer structure abnormalities in early Alzheimer’s disease: Evidence in optical coherence tomography. Neurosci Lett 480: , 69–72. |
[23] | Santos CY , Johnson LN , Sinoff SE , Festa EK , Heindel WC , Snyder PJ ((2018) ) Change in retinal structural anatomy during the preclinical stage of Alzheimer’s disease. Alzheimers Dement (Amst) 10: , 196–209. |
[24] | Lopez-Cuenca I , de Hoz R , Salobrar-Garcia E , Elvira-Hurtado L , Rojas P , Fernandez-Albarral JA , Barabash A , Salazar JJ , Ramirez AI , Ramirez JM ((2020) ) Macular thickness decrease in asymptomatic subjects at high genetic risk of developing Alzheimer’s disease: An OCT study. J Clin Med 9: , 1728. |