Self- and Partner-Reported Subjective Memory Complaints: Association with Objective Cognitive Impairment and Risk of Decline
Abstract
Background:
Episodic memory decline is a hallmark of Alzheimer’s disease (AD). Subjective memory complaints (SMCs) may represent one of the earliest signs of impending cognitive decline. The degree to which self- or partner-reported SMCs predict cognitive change remains unclear.
Objective:
We aimed to evaluate the relationship between self- and partner-reported SMCs, objective cognitive performance, AD biomarkers, and risk of future decline in a well-characterized longitudinal memory center cohort. We also evaluated whether study partner characteristics influence reports of SMCs.
Methods:
758 participants and 690 study partners were recruited from the Penn Alzheimer’s Disease Research Center Clinical Core. Participants included those with Normal Cognition, Mild Cognitive Impairment, and AD. SMCs were measured using the Prospective and Retrospective Memory Questionnaire (PRMQ), and were evaluated for their association with cognition, genetic, plasma, and neuroimaging biomarkers of AD, cognitive and functional decline, and diagnostic progression over an average of four years.
Results:
We found that partner-reported SMCs were more consistent with cognitive test performance and increasing symptom severity than self-reported SMCs. Partner-reported SMCs showed stronger correlations with AD-associated brain atrophy, plasma biomarkers of neurodegeneration, and longitudinal cognitive and functional decline. A 10-point increase on baseline PRMQ increased the annual risk of diagnostic progression by approximately 70%. Study partner demographics and relationship to participants influenced reports of SMCs in AD participants only.
Conclusion:
Partner-reported SMCs, using the PRMQ, have a stronger relationship with the neuroanatomic and cognitive changes associated with AD than patient-reported SMCs. Further work is needed to evaluate whether SMCs could be used to screen for future decline.
INTRODUCTION
Alzheimer’s disease (AD) is the most common form of dementia [1]. While AD is predominantly a disease of the aging population, it is now widely accepted that the molecular pathology underlying disease development begins decades before cognitive and functional decline becomes apparent [2]. Discerning which individuals are at-risk for clinical AD has proven to be challenging [3, 4] but remains a critical pursuit. Early detection and accurate prognostication are important for patients and their families to make appropriate preparations and to ensure they receive the needed support as symptoms emerge and impact day to day function [5]. Additionally, identification of at-risk individuals would provide an appropriate population for secondary prevention trials and identify individuals who would benefit from treatment with potential disease-modifying therapies [6].
In an effort to identify additional tools to inform early detection of cognitive change, multiple sources of information are collected. One such source is subjective memory complaints (SMCs), which are an individual’s perception of their own or a loved one’s cognitive impairment. It has been suggested that SMCs may precede the onset of objective memory impairment [7–9]. Some studies have shown that an individual’s subjective experience of their impairment may be more sensitive to AD-related neurodegenerative changes than certain neurocognitive batteries, particularly early on in the disease course. For this reason, much attention has focused on characterizing the relationship between SMCs and cognitive decline in a variety of populations and clinical settings to determine whether SMCs can serve as a screening tool for individuals at risk for AD [10–12].
Thus far, the literature on the utility of self-reported SMCs as a potential dementia screening tool has been largely conflicting [13–20]. This is, in part, due to the heterogeneity in the population of individuals presenting with SMCs. Many factors have since been reported to influence the perception of one’s own impairment, such as the context in which SMCs are ascertained (community versus clinical), the structure of the SMC questionnaire [21], co-morbid psychiatric symptoms [13, 20, 22, 23], and individual differences in personality traits [24, 25]. These findings have raised questions about how SMCs should be interpreted in clinical and research settings.
To supplement patient-reported information, clinicians often rely on additional input from a proxy or partner, typically a family member or close friend, to assess a patient’s clinical and functional status. In contrast to self-reported SMCs, partner reports of memory impairment have been more reliable in assessing objective impairment and predicting future decline, albeit still somewhat inconsistently [19, 26, 27]. As is the case for self-report, individual characteristics of partners may also impact patterns of reporting about a patient’s cognitive and functional status; however, very few studies to-date have investigated such relationships [28, 29]. A deeper understanding of the variables that impact partner reports will be critical to make effective use of partner reporting tools in both the clinical and research settings.
Beyond these subjective reports, it is also important to demonstrate associations between SMCs, cognitive changes, and AD biomarkers. Prior work has shown that self-reported SMCs are associated with greater cerebral plaque burden on amyloid PET [30–32] as well as hippocampal volume loss [33–35]. Other novel imaging biomarkers have yet to be studied in relation to SMCs. For instance, more granular medial temporal lobe subregional measures that may enhance sensitivity to the earliest neurodegenerative changes of AD [36, 37] or pattern analysis algorithms of structural MRI data may be employed to describe the deviation of an individual’s brain structure from typical brain aging trajectories. The Spatial Pattern of Abnormality for Recognition of Early Alzheimer’s disease index, known as SPARE-AD, is derived from such high dimensional data and indicates whether participants possess spatial patterns of brain atrophy observed in typical AD [38–40]. The SPARE-AD index has been shown to differentiate individuals with normal cognition and MCI [41] as well as predict progression from normal cognition to MCI [38, 42] and from MCI to AD [39, 42]. Similarly, the SPARE-BA (BA = brain age) index measures spatial patterns of brain atrophy associated with aging in cognitively normal individuals and can be used as a biomarker for a person’s brain age [43–45]. In addition to neuroimaging studies, blood-based biomarkers of AD are becoming increasingly attractive due to their low cost and ease of use. Neurofilament light chain (NfL), glial fibrillary acidic protein (GFAP), and phosphorylated tau at threonine 181 (p-tau181) are brain-derived molecules that, when detected in the serum or plasma, are thought to indicate ongoing inflammation and damage in the central nervous system [46]. Elevated plasma concentrations of all three biomarkers have been associated with increased risk of developing AD and cognitive impairment [46–52], and are thus intriguing biomarkers in this disease context. Further work is needed to more comprehensively characterize how SMCs relate to structural and molecular neuroimaging biomarkers as well as more novel blood-based biomarkers in those at risk for cognitive decline due to AD or due to normal aging.
In the present study, we aimed to address the gaps in the literature on SMCs and cognition using a well-characterized longitudinal cohort of individuals across the cognitive spectrum and their study partners. First, we aimed to clarify the relationship between baseline self- and partner-reported SMCs and objective measures of cognitive performance, depression, and imaging and genetic AD biomarkers. Additionally, we investigated how partner characteristics, including the nature of their relationship to the study participant, influence their reports of the partner’s memory impairment. Finally, we investigated whether baseline self- or partner-reported SMCs could predict cognitive and functional decline as well as diagnostic progression over the study period.
MATERIALS AND METHODS
Participants and study design
Participants were selected from the Penn Memory Center and the Penn Alzheimer’s Disease Research Center (ADRC) Clinical Core Integrated Neurodegenerative Disease Database [53], spanning 2009–2019 in accordance with the University of Pennsylvania Institutional Review Board. Data from 758 cohort participants and 690 study partners were included. All participants completed a questionnaire of SMCs at baseline and were classified as having normal cognition (NC; N = 331), mild cognitive impairment (MCI; N = 200), or Alzheimer’s disease (AD; N = 227) by expert clinical consensus diagnosis as previously described [54]. Annual study visits for the cohort consisted of medical history, physical and neurological examinations, and neuropsychological testing. The accompanying study partner was asked to provide their basic demographic data, details about their relationship to the participant, and assessment of the participant’s functional status and cognition, described in greater detail below. The majority of participants were followed annually, and an updated consensus diagnosis was established on a yearly basis upon careful review of the most recent data.
Subjective memory complaints
SMCs were characterized using the Prospective Retrospective Memory Questionnaire (PRMQ) [55, 56]. The PRMQ is a 16-item questionnaire that asks questions such as, “Do you forget something that you were told a few minutes before?”. The subject is asked to rate how frequently these memory lapses occur on a scale of 1 (“Never”) to 5 (“Very Often”), with a total minimum score of 16 and maximum of 80. Higher PRMQ scores indicate more SMCs. The study partner (proxy) was asked to complete an analogous questionnaire about the perceived memory impairment in the study participant. To evaluate discrepancies in self- and study partner-reported SMCs, a PRMQ difference score was calculated (PRMQ Self – PRMQ Proxy) such that positive scores indicate more reported impairment by the subject compared to the study partner.
Neuropsychological assessment
Over the course of the study period (2009–2019), the ADRC Clinical Cohort completed the National Alzheimer’s Coordinating Center (NACC) Uniform Dataset (UDS) Version 2 [57] from 2009–2015, and the UDS Version 3 [58] after 2015. Both batteries assess global cognition, processing speed, executive function, episodic memory, and verbal and category fluency, all of which were included in the analyses. However, the batteries differ in some of the measures used to assess these cognitive domains (Supplementary Table 1). There were strong correlations between all pairs of new and prior tests in the two UDS versions [59]. Nonetheless, to account for differences in domain-specific measures employed by the two batteries (e.g., Logical Memory from Wechsler Memory Scale in UDS2 and the Craft Story in UDS3), z-scores were calculated for each test within the UDS Versions 2 and 3, normalized to the NC group. For measures of global cognition, which include the Mini-Mental State Examination (MMSE) [60] and the Montreal Cognitive Assessment (MoCA) [61], all MoCA scores were converted to MMSE equivalent scores using a previously published MoCA to MMSE conversion algorithm [54].
Affect and function
Participants completed the Geriatric Depression Scale (GDS) [62], a self-report measure of level of depressive symptoms. Study partners completed the Functional Rating Scale (FRS) [63], a measure of the participant’s day to day functional abilities. Briefly, the FRS, which was previously named the Dementia Severity Rating Scale, asks the study partner to rate the participant’s performance in 11 categories of functioning, including memory, orientation, language, judgment, social interaction, home activities, eating, incontinence and mobility. Each category presents a statement indicating a range in behavior from normal (independent) to severely impaired (dependent) functional abilities. Scores range from 0 to 51 points, with higher scores indicating greater impairment.
Study partner data
The study partner provided demographic information, including their age, sex, race, ethnicity, and highest level of education achieved. On the FRS questionnaire described above, the study partner is also asked to define the nature of their relationship with the study participant and the frequency of contact, which includes both in-person visits and phone calls. The study partner relationship is categorized as Spouse/Partner, Child, Sibling, Other Relative, or Friend/Neighbor. Frequency of contact is categorized as < 1 day per week, 2 days per week, 3-4 days per week, or 5 or more days per week.
Genetic, plasma, and neuroimaging biomarkers
The majority of study participants (N = 758) underwent APOE genotyping. Participants possessing either one or two copies of the APOE4 allele were considered to be APOE4 positive, while all other genotypes were considered negative.
For a subset of patients, data on plasma concentrations of NfL, GFAP, and p-tau181 were available. See Supplementary Table 2 for demographics of this sub-cohort. Briefly, participants were recruited, and whole blood samples were collected in EDTA-tubes, spun-down, and stored at –80°C. NfL and p-tau181 were analyzed in plasma samples on the SiMoA platform, as previously described [64, 65]. Plasma samples were analyzed in duplicate for GFAP using the Quanterix Simoa Discovery kit reagents on the Quanterix HD-X automated immunoassay platform [66].
For a subset of patients, neuroimaging data, including amyloid PET status and structural MRI measures, were also collected. Demographic and disease distribution data for these biomarker sub-groups are provided in the Supplementary Table 2. Amyloid status was used to define subgroup analyses in the association of SMCs with cognitive and functional decline in NC and MCI participants only. Participants (N = 121) were stratified into amyloid positive and negative individuals based on expert visual interpretation of amyloid positron emission tomography (PET) scans. PET scans were obtained within five years of clinical testing. Several amyloid PET tracers were used with scanning performed according to manufacturer’s recommendations: 18F-florbetapir (N = 26, 370 MBq±20%, 45-min uptake time, 10-min scan), 18F-florbetaben (N = 106, 300 MBq±20%, 90-min uptake time, 20-min scan), and 18F-flutametamol (N = 2, 185 MBq, 90-min uptake time, 20-min scan).
Additionally, we assessed the relationship between SMCs and medial temporal lobe (MTL) subregion volumes (N = 224) in NC and MCI participants only, as AD participants are already known to harbor significant atrophy in the MTL region [36]. All MRIs were obtained within twelve months of clinical testing. Cross-sectional MTL subregion measures were obtained from 0.8 mm3 T1-MRI using the automated segmentation of hippocampal subfields (ASHS)-T1 pipeline, as previously described [36, 37]. All automated segmentations were visually checked and edited, if necessary. The following regions were included in this analysis: anterior hippocampus, posterior hippocampus, entorhinal cortex (ERC), Brodmann Area (BA) 35, BA36, and parahippocampal cortex (PHC). We computed volume of anterior/posterior hippocampus directly from automatic segmentation. For the MTL cortical subregions (ERC, BA35, BA36, and PHC), a graph-based multi-template thickness analysis pipeline was applied to the automatic segmentation to derive thickness of each subregion [37, 67, 68]. We obtained thickness measures for the cortical subregions because it is less sensitive to segmentation errors of subregion boundaries. Left and right hemisphere were averaged. Intracranial volume (ICV) was also measured using ASHS-T1.
A subset of participants (N = 222) was examined to evaluate whether SMCs were associated with spatial patterns of brain atrophy indicative of advanced brain age (SPARE-BA index) or AD (SPARE-AD index) [38, 40, 43]. Both the SPARE-BA and SPARE-AD indices were generated using machine learning methods described previously [38, 40, 69]. Briefly, the SPARE-AD index is derived from a support vector machine classifier trained for optimal differentiation of cognitively normal individuals and age-matched patients with AD [39]. The SPARE-AD index summarizes the high dimensional data with a single score, wherein higher, more positive scores indicate greater AD-like atrophy and lower, more negative scores indicate greater similarity to normal controls. The SPARE-BA index was calculated using support vector regression to predict brain age from MRI scans, which can then be used to evaluated participants’ advanced brain aging based on the patterns of brain atrophy [43, 45]. A Brain Age Gap was calculated for each subject (predicted age – chronologic age), such that positive scores indicate more advanced brain aging, while negative scores indicate more resilient brain aging.
Statistical analyses
All univariate analyses were performed using either Pearson χ2, Wilcoxon Rank-Sum, or Kruskal-Wallis test with Dunn’s post-test, which accounts for multiple comparisons. These analyses were employed to evaluate differences in SMCs across diagnostic categories, across study partner relationships, and between those with or without the presence of particular AD biomarkers. To evaluate the relationships between SMCs, cognitive performance, and depressive symptoms, either partial correlations or linear regressions were calculated with age, sex, race, and education included as covariates. GDS scores were also included as a covariate for associations between SMCs and cognition. To evaluate whether SMCs were associated with plasma biomarker concentration, MTL volumes/thickness, SPARE-AD and SPARE-BA, multivariate linear regressions were performed with plasma biomarker concentration (NfL, GFAP, or p-tau181), MTL region volume/thickness, SPARE-AD index, or Brain Age Gap (SPARE-BA) as the dependent variables and with SMCs, chronologic age, sex, race, and education as covariates. For analyses of hippocampal volumes, ICV was also included as a covariate. Because we were interested in whether SMCs could predict development of structural brain changes indicative of AD-related neurodegeneration, analyses with neuroimaging data were performed in the NC and MCI group only.
To evaluate the effects of baseline SMCs on change in global cognition (MMSE) and function (FRS), mixed linear regression models were performed with the participant as the hierarchical variable to allow for intra-individual correlation. The mixed-effects model accounted for the varying number of visits and time between visits. A maximum follow-up length of four years was chosen to increase statistical power. Separate models were run to predict the effect of self-reported SMCs and partner-reported SMCs on cognitive and functional decline. Only participants with at least one follow-up visit were included in this analysis. For modeling of functional decline, follow up FRS scores were included if the questionnaire was completed by the same study partner at all time-points to account for inter-individual differences in reporting. The dependent variables in each model were either MMSE or FRS scores at each visit. The following independent variables were included: baseline PRMQ scores (Self or Proxy), time (years from baseline), and, separately, the interaction of these two variables (PRMQ x time). Fixed effects demographic and clinical variables included: age at baseline, sex, race, education, and baseline MMSE or FRS scores depending on the model. The Bayesian information criterion (BIC) was used to choose the most appropriate random effects for the model (e.g., random intercept versus random intercept and random slope). Backward selection of fixed effects was used to optimize each model. Only NC and MCI participants were included in these analyses given our clinical interest in predicting future development of AD-associated cognitive and functional impairment.
To evaluate the effect of baseline SMCs on risk of disease progression, Cox proportional hazards models were used. Disease progression was considered a change in diagnosis from NC to MCI/AD or a change in diagnosis from MCI to AD. Predictor variables of interest were self- and partner-reported SMCs as measured by the PRMQ as well as item 10 on the GDS short form [62], a dichotomous yes/no question: “Do you feel that you have more problems with memory than most?”. GDS item 10 was included in a separate model as a measure of self-reported SMCs relative to peers because such relative measures have been shown to be a more sensitive predictor of cognition and cognitive change [21, 70] and is not explicitly evaluated in the PRMQ. Covariates included were age, sex, race, education and baseline MMSE. Backward selection of covariates was then used to optimize the model. All statistical analyses were performed in STATA, and statistical significance was defined as an alpha level less than 0.05.
RESULTS
Participant characteristics
A total of 758 participants were included in this study. Demographic and neuropsychological characteristics of the total cohort and individuals within each diagnostic group are summarized in Table 1. Overall, this cohort was comprised of predominantly White (72% White; 26% Black; 2% other) and highly educated individuals (Mean = 15.5 years of education, SD = 3.0). There were significant differences in all demographic and clinical variables between diagnostic groups. As expected, age increased, global cognition decreased, and functional independence decreased with greater disease severity (p < 0.0001). Women were over-represented in the NC (70.0%) and AD (62.1%) groups, while sex was more evenly distributed in the MCI group (47.5% women). Race also differed between diagnostic categories, with an increasing proportion of White participants with greater disease severity (p < 0.0001). Education was also significantly lower in the AD group as compared to the NC and MCI groups (p < 0.0001). Analyses involving genetic and imaging biomarkers included a smaller subset of this cohort. Demographic and clinical variables did not differ substantially between the larger cohort and subset cohorts, and results of the latter are summarized in Supplementary Table 2. Due to differences in demographics between each of the diagnostic groups, demographic variables were included as covariates in subsequent multivariate analyses.
Table 1
Participant Demographic and Clinical Variables by Diagnostic Category
Total Cohort (N = 758) | NC (N = 331) | MCI (N = 200) | AD (N = 227) | |
Age, Mean (SD)a,* | 73.2 (8.5) | 71.6 (8.6) | 73.1 (7.9) | 75.7 (8.3) |
Sex, (% Female)b,* | 61.7% | 70.0% | 47.5% | 62.11% |
Race, (%)b,* | ||||
White | 72.4% | 61.3% | 79.2% | 82.7% |
Black | 25.7% | 36.6% | 19.8% | 15.1% |
Other | 1.9% | 2.1% | 1.0% | 2.2% |
Education, Mean (SD)a,* | 15.5 (3.0) | 15.8 (2.7) | 15.8 (3.0) | 14.7 (3.1) |
MMSE, Mean (SD)a,* | 25.9 (4.5) | 29.0 (1.1) | 26.9 (2.4) | 21.1 (4.6) |
FRS, Mean (SD)a,* | 6.7 (7.4) | 0.53 (1.3) | 5.3 (3.9) | 14.1 (6.6) |
Amyloid PET+, N (%) | 22/83 (26.5%) | 30/51 (58.5%) | 13/14 (92.9%) |
AD, Alzheimer’s disease; FRS, Functional Rating Scale; MCI, mild cognitive impairment; MMSE, Mini-Mental State Examination; NC, normal cognition; PET, positron emission tomography. Demographic variables were compared across diagnostic groups using aKruskal-Wallis test with Dunn’s post-test, bPearson’s chi-squared test. All comparisons across diagnostic groups were statistically significant with a p-value < 0.001, denoted by*.
Study partner characteristics
Demographic characteristics of study partners (N = 690) are summarized in Table 2. The study partner cohort was similar to that of the study participants. They were predominantly White (67.7%) and highly educated (M = 15.7 years, SD = 4.8 years). Significant differences in study partner demographics between diagnostic categories was also observed in this cohort. Study partners reporting on participants with MCI and AD tended to be older (p < 0.0001) and a higher proportion were White compared to the NC group (p < 0.001). Study partner sex and education did not differ between diagnostic categories.
Table 2
Study Partner Characteristics by Diagnostic Category
Total Cohort (N = 690) | NC (N = 291) | MCI (N = 183) | AD (N = 216) | |
Age, Mean (SD)a,** | 62.9 (14.2) | 59.8 (14.7) | 65.8 (12.0) | 64.7 (14.4) |
Sex, (% Female)b | 62.41% | 61.36% | 66.67% | 60.26% |
Race (%)b,** | ||||
White | 67.7% | 50.3% | 76.3% | 82.3% |
Black | 29.2% | 45.8% | 21.2% | 15.3% |
Education, Mean (SD)a | 15.7 (4.8) | 16.1 (6.9) | 15.5 (2.8) | 15.5 (2.6) |
Relationship, N (%)b,** | ||||
Spouse | 382 (55.4%) | 124 (42.6%) | 131 (71.6%) | 127 (58.8%) |
Child | 213 (30.9%) | 106 (36.4%) | 31 (16.9%) | 76 (35.2%) |
Sibling | 32 (4.6%) | 19 (6.5%) | 6 (3.3%) | 7 (3.2%) |
Other relative | 20 (2.9%) | 10 (3.4%) | 8 (4.4%) | 2 (0.9%) |
Friend/Neighbor | 43 (6.2%) | 32 (11.0%) | 7 (3.8%) | 4 (1.9%) |
Contact Amount, N (%)b,* | ||||
< 1 time/week | 57 (8.2%) | 34 (11.7%) | 8 (4.4%) | 15 (6.9%) |
1 time/week | 52 (7.5%) | 31 (10.7%) | 10 (5.5%) | 11 (5.0%) |
2 times/week | 28 (4.1%) | 15 (5.2%) | 9 (5.0%) | 4 (1.8%) |
3–4 times/week | 59 (8.6%) | 32 (11.0%) | 12 (6.6%) | 15 (6.9%) |
5+ times/week | 494 (71.6%) | 178 (61.4%) | 143 (78.6%) | 173 (79.4%) |
AD, Alzheimer’s disease; MCI, mild cognitive impairment; NC, normal cognition. Demographic variables were compared across diagnostic groups using aKruskal-Wallis test with Dunn’s post-test, bPearson’s chi-squared test. Statistically significant relationships are denoted as follows: *p < 0.01, **p < 0.001.
In terms of the relationship to the study participant, the largest proportion of study partners were spouses/partners followed by children. Due to the low number of study partners who identified as siblings, other relatives, and friends/neighbors in the total cohort (< 15%), these study partners were grouped together in an “other relationship” category. Spouse study partners were more frequent in the MCI group than in the AD group (72.1% in MCI versus 60.8% in AD, respectively, p < 0.031), while children as study partners were more common in the AD group than in the MCI group (17.0% in MCI versus 33.7% in AD, p = 0.001).
Contact between the participant and study partner included both in-person visits and phone calls. Across diagnostic groups, 70% were in almost everyday contact with the participants (5+ days/week), although a greater proportion of almost everyday contact was seen in MCI and AD groups (77%, p < 0.001), which may be reflective of the greater proportion of spouse study partners in these groups. Additionally, in the NC group, there was a larger proportion of study partners (13%) that had very infrequent contact with study participants (< 1 day/week), compared to 4.1% and 7.7% in MCI and AD, respectively (p = 0.008).
SMCs by diagnostic category
Self- and partner-reported SMCs were compared between each diagnostic category to determine if subjective complaints correlated with objective impairment (Fig. 1A). Median partner-reported SMCs increased with more severe impairment (NC: Median 25, IQR 21–31; MCI: Median 38, IQR 29–44, AD: Median 51, IQR 42–60; p < 0.0001), while self-reported SMCs did not. The highest degree of impairment was reported by participants in the MCI group (Median 42, IQR 36–49), with no difference in self-reported SMCs between participants with NC and AD (NC: Median 38, IQR 32–43; AD: Median 39, IQR 32–45; U = 27860.5, p = 0.1325). PRMQ difference scores highlight the discrepancy in perceptions of memory performance with different levels of clinical impairment. Participants reported significantly more SMCs compared to their study partners in the NC and MCI groups, while study partners reported more SMCs than participants in the AD group (Fig. 1B). All of these relationships between SMCs and diagnostic group were similar in the amyloid+ subgroup of subjects (data not shown). Taken together, partner-reported SMCs varied to a greater extent with diagnostic category than self-reported SMCs.
Fig. 1
PRMQ Scores by Diagnostic Category. A) Comparison of average scores on PRMQ self and PRMQ proxy scales in each diagnostic category. Kruskal-Wallis Test with Dunn’s post-test: ****p < 0.0001. B) Comparison of average PRMQ difference scores in each diagnostic category. PRMQ difference scores were calculated for each subject (PRMQ Self – PRMQ proxy) to represent discrepancies in SMC reports. Wilcoxon Rank-Sum Test: ***p < 0.001, ****p < 0.0001. AD, Alzheimer’s disease; NC, normal cognition; MCI, mild cognitive impairment; PRMQ, Prospective Retrospective Memory Questionnaire.
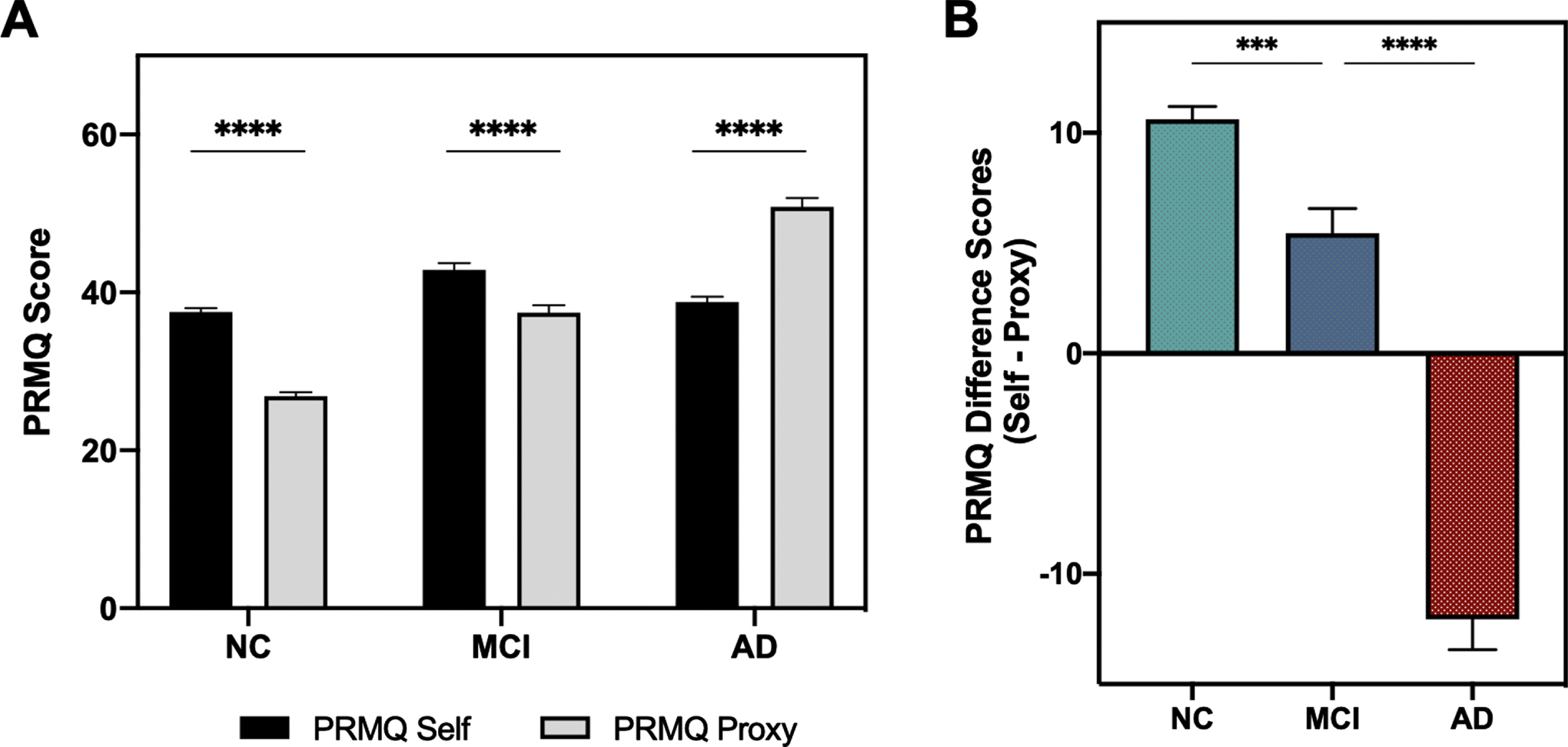
Influence of study partner demographics on SMCs
There were no relationships between partner-reported SMCs and study partner age, sex, or education in the NC or MCI group. In the AD group, female study partners reported higher SMCs than males (males: Median = 46, IQR 39 – 59; females: Median = 52, IQR 43 – 60; U = 2347.5, p = 0.0145), and greater reports of SMCs by both male and female study partners correlated with worse objective global cognitive impairment as measured by the MMSE (males: r = –0.353, p = 0.005 versus females: r = –0.244, p = 0.0185). Younger and more highly educated study partners also tended to report more SMCs, even after controlling for baseline MMSE scores (younger age: r = 0.281, p < 0.001; education: r = 0.279, p = 0.005). Baseline cognition was included as a covariate to account for possible correlations between degree of subject impairment and study partner demographics.
The study partner relationship with the participant also influenced partner-reported SMCs in AD participants. In the AD group, children reported greater SMCs than spouses (spouse: Median = 49; IQR 39 – 58; child: Median = 55, IQR 44 – 64; U = 2478.5, p = 0.0175), which is consistent with the above findings for study partner age. Both children- and spouse-reported SMCs correlated with MMSE scores for AD participants, with a trend for a stronger correlation in children partners (spouse: r = –0.203, p = 0.0376 versus child: r = –0.438, p < 0.001; comparison p = 0.056). This suggests children study partners, at least in the AD group, may be more sensitive to global cognitive change. No relationships between partner-reported SMCs and cognitive performance were observed for either spouse or children study partners of NC or MCI participants.
It is interesting to note that partners of NC participants tended to have less frequent contact than partners of MCI and AD participants (Table 2): up to 22% of NC partners had one or fewer days of contact per week versus 10% and 12% in MCI and AD partners, respectively (Pearson’s χ2: p = 0.01). SMC reports by partners in both NC and AD participants varied based on contact frequency. Partners of NC participants with less frequent contact reported fewer SMCs than those with nearly daily contact (Medain 24, IQR 20–28 versus Median 27, IQR 22–33; U = 3757.5, p = 0.0049). Meanwhile, the opposite was true in AD: partners with less frequent contact reported more SMCs than those with nearly daily contact (Median 57, IQR 51–61 versus Median 50, IQR 39–59, U = 1079, p = 0.0218).
SMCs and depression
Higher self-reported SMCs were associated with greater depressive symptomatology in all diagnostic categories after controlling for age, sex, race, and education (NC: β= 0.068, p < 0.001; MCI: β= 0.067, p = 0.001; AD: β= 0.092, p < 0.001). There were no significant relationships between partner-reported SMCs and depressive symptoms in any diagnostic category. There were significantly higher frequencies of depression in MCI and AD compared to the NC group, as defined by a GDS score > 6 (both p-values < 0.015).
SMCs and objective cognitive performance
Partial correlations of SMCs with cognitive performance were analyzed separately within each diagnostic category, and statistically significant relationships are presented in Fig. 2. Average performance on each cognitive test within a diagnostic category is provided in Supplementary Table 3. Within the NC group, higher self-reported SMCs were associated with slower processing speed (Trail Making Test A time; adjusted r = 0.135, p = 0.033) as well as worse performance on category fluency (Animal Naming; adjusted r = –0.134, p = 0.035). No statistically significant relationships were observed between partner-SMCs and any measure of cognition in the NC group. Within the MCI group, no statistically significant relationships were observed between self-reported SMCs and any measure of cognition, while partner-SMCs were associated with worse performance on delayed recall (adjusted r = –0.231, p = 0.0078). Finally, in the AD group, higher self-reported SMCs were associated with worse performance on tasks of executive function (Trail Making Test B time; adjusted r = 0.200, p = 0.0251) and delayed recall (adjusted r = –0.182, p = 0.0251). Higher partner-reported SMCs in the AD group were associated with worse performance on the MMSE (adjusted r = –0.312, p = 0.002), immediate recall (adjusted r = –0.199, p = 0.020), and delayed recall (adjusted r = –0.195, p = 0.0234). When considering the amyloid+ subgroup of participants, worse performance on delayed recall was observed with higher self-reported SMCs in the NC group (adjusted r = –0.997, p = 0.045) and greater partner-reported SMCs in the MCI group (adjusted r = –0.517, p = 0.012). No other significant relationships between SMCs and cognition were found in the amyloid+ subgroup. Taken together, partner-reported SMCs tended to correlate better with memory impairments in both the MCI and AD group, while associations between self-reported SMCs and memory impairment were only observed in the amyloid+ subgroup.
Fig. 2
Significant Associations between SMCs and cognition at baseline. Partial correlations were calculated between each SMC and cognitive measure across diagnostic groups. All reported R2 values are adjusted for age, sex, race, education, and GDS. p-values are for the SMC term in the model. The left panel includes statistically significant relationships between self-reported SMCs and cognitive measures (A–D), and the right panel includes those for informant-reported SMCs (E–H). The diagnostic category in which the partial correlation was performed is listed above the graph and is further denoted by the colors of the individual dots: normal cognition (NC), green; mild cognitive impairment (MCI), blue; Alzheimer’s disease (AD), red.
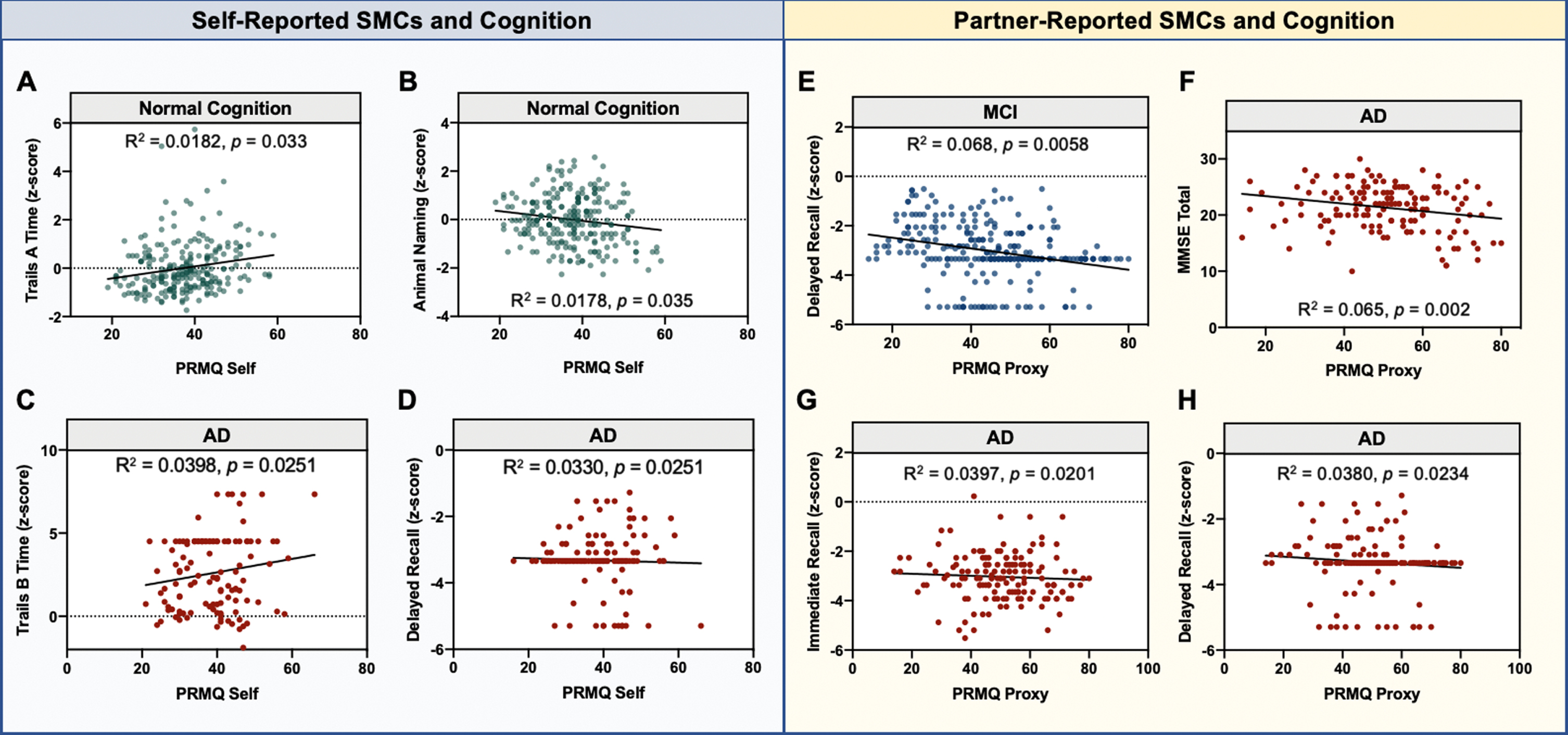
SMCs and genetic, plasma, and neuroimaging biomarkers
There were no significant differences in self- or partner-reported SMCs between those with and without APOE4 alleles in any of the diagnostic categories (Supplementary Table 4). SMCs were associated with changes in plasma biomarkers of neurodegeneration. Overall, concentrations of all plasma biomarkers increased with increasing degree of impairment (Supplementary Table 5). We first divided individuals into those with higher versus lower SMCs, defining those that were SMC+ (upper quartile) and SMC- (lower quartile) within each diagnostic category. We found that higher self- and informant-reported SMCs were associated with higher plasma GFAP concentrations in the NC group (Fig. 3); however, only the relationship with informant-reported SMCs remained significant when considering the entire range of SMCs in the NC cohort and after controlling for age, sex, race, and education (β= 1.438, p = 0.002). We similarly found higher plasma concentrations of p-tau181 for informant-reported SMC+ versus SMC- in the NC group (Median 2.25, IQR 1.75 – 3.09 versus Median 1.99, IQR 1.47 – 2.47, U = 1432, p = 0.042), though this relationship did not remain when considering the entire range of SMCs and controlling for demographic variables. Lastly, higher self-reported SMCs in the AD group were associated with lower plasma NfL concentrations (β= –0.593, p = 0.033), again suggesting impaired insight into the degree of impairment or neurodegeneration. There were no significant relationships between SMCs and plasma biomarkers in the MCI group.
Fig. 3
Plasma GFAP concentrations by SMC quantile in participants with normal cognition. PRMQ Self and Proxy scores were divided into lower and upper quartiles within normal controls, defining participants and their informants as SMC- and SMC+, respectively. A) Differences in plasma concentrations of GFAP between self-reported SMC quartiles. B) Differences in plasma concentrations of GFAP between proxy-reported SMC quartiles. Wilcoxon Rank-Rum Test: *p < 0.05. GFAP, glial fibrillary acidic protein; SMC, subjective memory complaints.
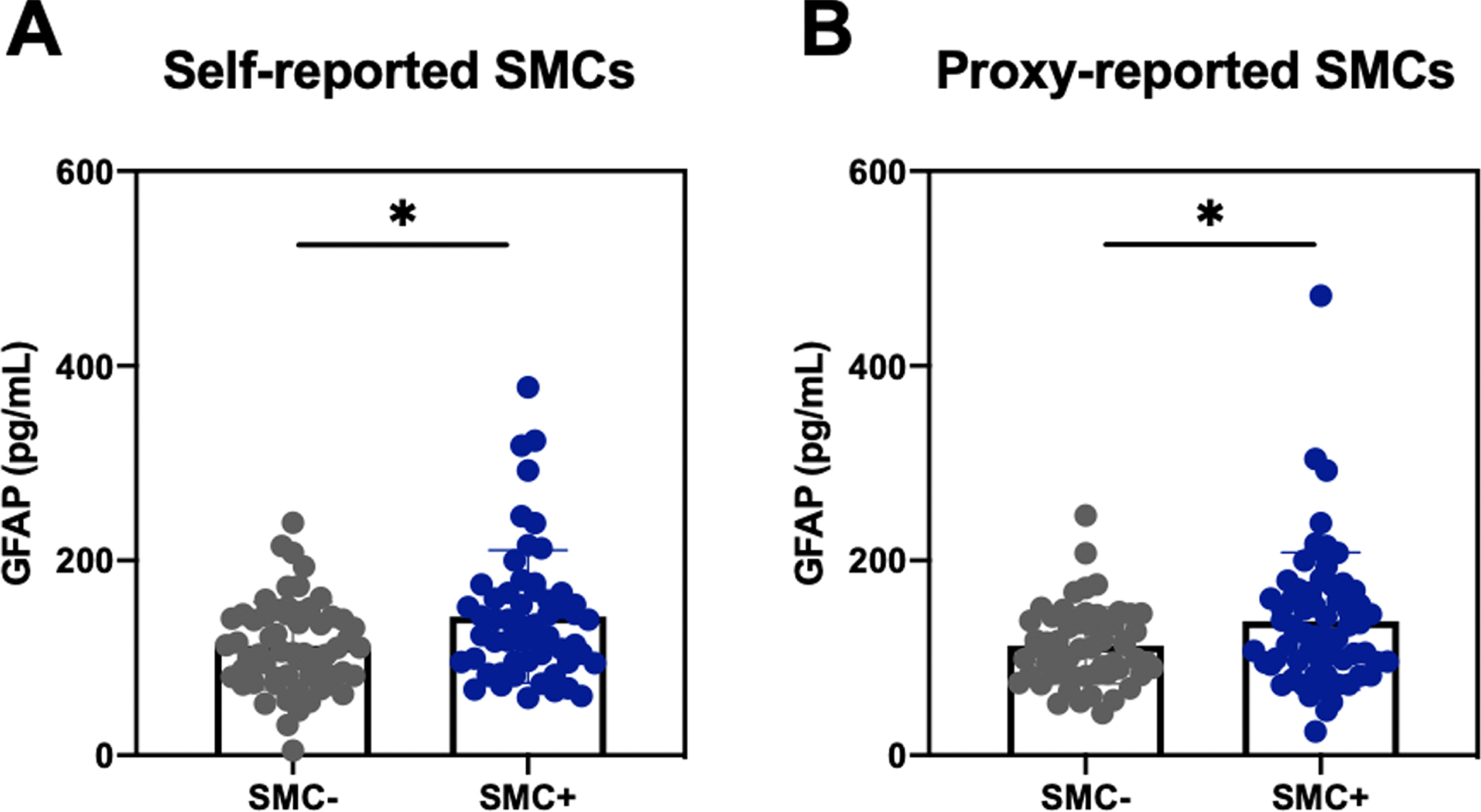
We next investigated the association between measures of medial temporal lobe (MTL) structures and self- and partner-reported SMCs in a subset of participants in the NC and MCI groups. In the NC group, higher self-reported SMCs were associated with lower anterior hippocampal volumes (β= –5.293, p = 0.038) as well as reduced thickness of the parahippocampal cortex (β= –0.005, p = 0.003) (Table 3). Notably, partner-reported SMCs were not associated with any volumetric MTL measurements in the NC group. In the MCI group, self-reported SMCs were associated with reduced thickness in the following areas: the entorhinal cortex (β= –0.0130, p = 0.004), Brodmann area 35 (β= –0.012, p = 0.021), and the parahippocampal cortex (β= –0.008, p = 0.012). Higher partner-reported SMCs in the MCI group were associated with a thinner entorhinal cortex (β= –0.006, p = 0.030). Taken together, SMCs reported by individuals with both NC and MCI were associated with more regional reductions in MTL volumes than partner-reported SMCs in the same diagnostic group.
Table 3
Association between Subjective Memory Complaints and Medial Temporal Lobe Volumes
Normal Cognition | MCI | |||||||
Self SMCs | Partner SMCs | Self SMCs | Partner SMCs | |||||
MTL Region | β | p | β | p | β | p-value | β | p |
Ant. Hippo.† | –5.2929 | 0.038* | –2.3066 | 0.302 | –6.4488 | 0.189 | –2.9801 | 0.350 |
Post. Hippo.† | –3.3442 | 0.117 | –0.4104 | 0.827 | –3.2704 | 0.445 | –1.9313 | 0.440 |
ERC | –0.0026 | 0.209 | –0.0012 | 0.484 | –0.0130 | 0.004* | –0.0062 | 0.030* |
BA35 | –0.0035 | 0.157 | –0.0032 | 0.124 | –0.0115 | 0.021* | –0.0039 | 0.207 |
BA36 | –0.0046 | 0.065 | –0.0036 | 0.092 | –0.0010 | 0.834 | –0.0028 | 0.340 |
PHC | –0.0053 | 0.003* | 0.0017 | 0.267 | –0.0080 | 0.012* | –0.0038 | 0.071 |
Ant Hippo, Anterior hippocampal volume; BA, Brodmann area; ERC, Entorhinal cortex; MTL, Medial temporal lobe; PHC, Parahippocampal cortex; Post. Hippo., Posterior hippocampal volume. Linear regression was performed with age, sex, race, and education as covariates. †measures for which intracranial volume was included as an additional covariate. *and bold font indicate p < 0.05.
We next evaluated the relationship between SMCs and AD- and aging-associated patterns of brain atrophy (Fig. 4). We found a significant positive correlation between partner-reported SMCs for MCI participants and SPARE-AD index, which indicates that the global patterns of brain atrophy were more “AD-like” in those with higher SMCs (β= 0.0409, p = 0.046; Fig. 4B). No significant relationships were observed between the SPARE-AD index and self-reported SMCs in MCI participants or with self/partner-reported SMCs in NC participants (Fig. 4A). Regarding age-related patterns of atrophy, more SMCs were reported by NC participants with more advanced brain aging (β= 0.1620, p = 0.032) after controlling for chronologic age, sex, race, and education (Fig. 4C). No other significant relationships were found between SMCs and brain aging atrophy patterns in NC or MCI (Fig. 4D).
Fig. 4
Relationship between SMCs and patterns of brain atrophy associated with AD and aging. Scatter plots shows the relationships between SPARE-AD index and self- and partner-reported SMCs (PRMQ Self and Proxy Scores) in (A) NC participants and (B) MCI participants. Greater partner-reported SMCs were associated with more pronounced AD-related atrophy. In MCI. C, D) Predicted Age of a subject can be ascertained based on the degree of brain atrophy in areas associated with aging (SPARE-BA index; see Methods). The Brain Age Gap for each subject was calculated by predicted— chronologic age, whereby positive values indicate advanced brain aging (shaded red), and negative values indicate resilient brain aging (shaded blue). Linear regressions were performed to evaluate the relationship between the Brain Age Gap and SMCs in NC and MCI participants after controlling for chronologic age, sex, race, and education. Scatter plots represent the relationship between the Brain Age Gap and self- and partner-reported SMCs (PRMQ Self and Proxy Scores) in (C) NC and (D) MCI participants. Greater self-reported SMCs were associated with more pronounced age-related atrophy in NC.
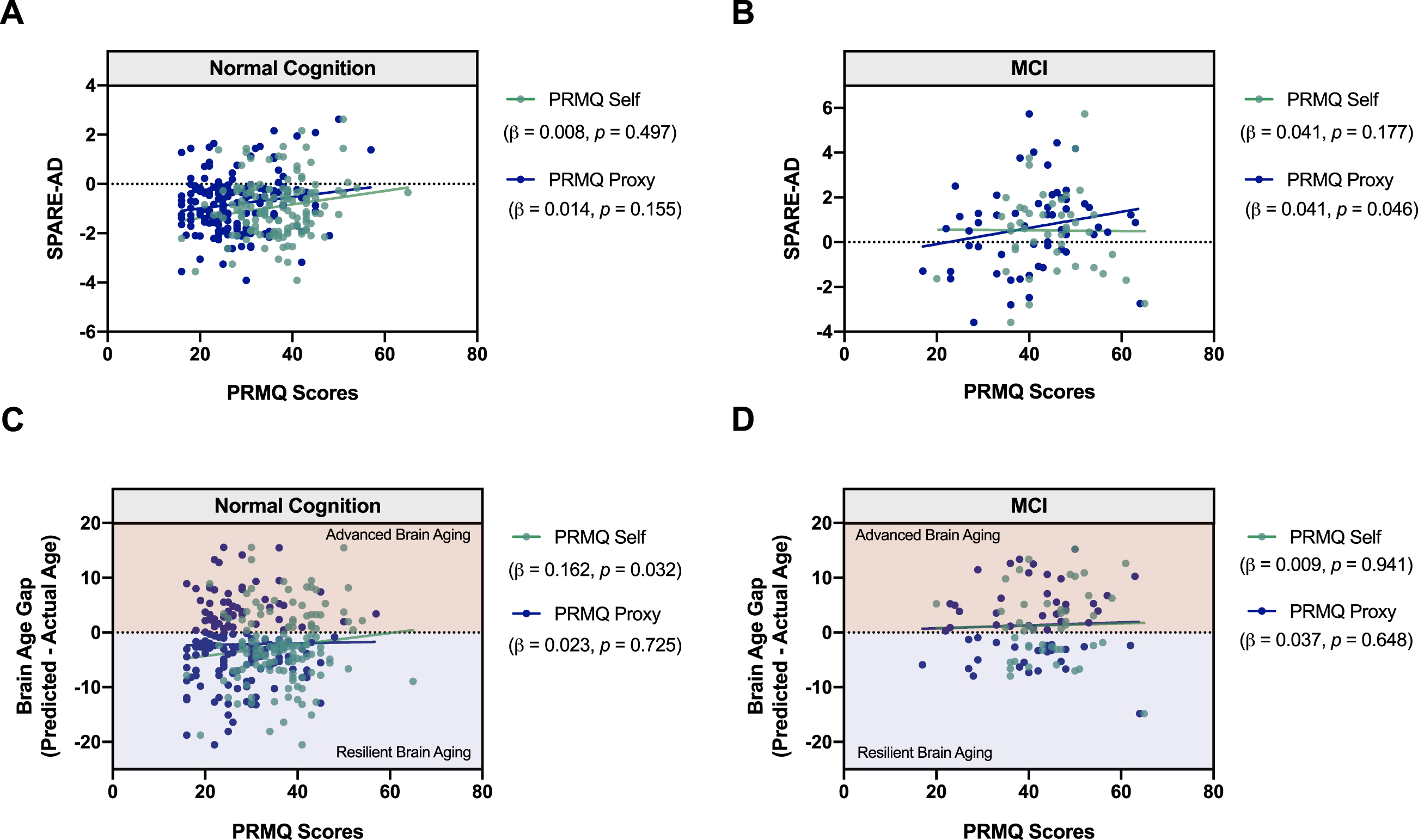
SMCs and cognitive decline
To evaluate the relationship between baseline self- and partner-reported SMCs and global cognitive decline as measured by the MMSE, linear mixed models were performed in participants with NC, MCI, and in a combined group of both NC and MCI. In the combined cohort of NC and MCI, higher baseline partner-reported SMCs were associated with a faster annual rate of decline in cognition over the study period while controlling for diagnostic group (Table 4, upper panels). No significant relationships were found between baseline SMCs and change in MMSE scores over the study period in either the NC group or the MCI group when analyzed separately (Supplementary Table 6).
Table 4
SMCs and baseline factors affecting cognitive decline in NC & MCI participants
95% Confidence Interval | ||||||
Fixed Terms in final model | Estimate | Std. Err. | p | Lower Bound | Upper Bound | |
Total Cohort NC + MCI | PRMQ Self (N = 369) | |||||
PRMQ Self×Time (y) | –0.0076 | 0.006 | 0.195 | –0.0191 | 0.0039 | |
MMSE at baseline | 0.8260 | 0.027 | < 0.001 | 0.7721 | 0.8798 | |
PRMQ Self | 0.0050 | 0.005 | 0.344 | –0.0054 | 0.0154 | |
Time (years) | 0.0090 | 0.235 | 0.969 | –0.4516 | 0.4697 | |
Education | 0.0489 | 0.015 | 0.001 | 0.0190 | 0.7875 | |
Diagnosis (MCI) | –0.4073 | 0.102 | < 0.001 | –0.6078 | –0.2069 | |
PRMQ Proxy (N = 333) | ||||||
PRMQ Proxy×Time (y) | –0.0207 | 0.005 | < 0.001 | –0.0303 | –0.0111 | |
MMSE at baseline | 0.8263 | 0.027 | < 0.001 | 0.7730 | 0.8796 | |
PRMQ Proxy | 0.0040 | 0.005 | 0.444 | –0.0063 | 0.0143 | |
Time (years) | 0.3556 | 0.152 | 0.019 | 0.0574 | 0.6539 | |
Education | 0.0621 | 0.015 | < 0.001 | 0.0324 | 0.0918 | |
Diagnosis (MCI) | –0.3636 | 0.119 | 0.002 | –0.0597 | –0.1305 | |
Amyloid + Subgroup NC + MCI | PRMQ Self (N = 21) | |||||
PRMQ Self×Time (y) | –0.0538 | 0.022 | 0.015 | –0.0974 | –0.0103 | |
MMSE at baseline | 0.8544 | 0.155 | < 0.001 | 0.5501 | 1.159 | |
PRMQ Self | 0.0105 | 0.026 | 0.691 | –0.0413 | 0.0623 | |
Time (years) | 1.7951 | 0.9659 | 0.063 | –0.0981 | 3.6883 | |
Diagnosis (MCI) | –0.5735 | 0.4768 | 0.229 | –1.5081 | 0.3611 | |
PRMQ Proxy (N = 29) | ||||||
PRMQ Proxy×Time (y) | –0.0407 | 0.019 | 0.035 | –0.0786 | –0.0030 | |
MMSE at baseline | 0.9721 | 0.107 | < 0.001 | 0.7623 | 1.1812 | |
PRMQ Proxy | 0.0180 | 0.021 | 0.396 | –0.0236 | 0.0597 | |
Time (years) | 0.8930 | 0.4748 | 0.232 | –0.5727 | 2.3588 | |
Diagnosis (MCI) | –0.3290 | 0.499 | 0.510 | –1.3071 | 0.6490 |
The above fixed effects remained in the final model following optimization with backward selection. Random effects included a random intercept for each subject and a random slope for time in years, for which model statistics are not shown. MCI, mild cognitive impairment; MMSE, Mini-Mental State Examination; NC, normal cognition; PRMQ, Prospective Retrospective Memory Questionnaire.
We next evaluated the relationship between SMCs and cognitive decline in a subgroup of amyloid+ participants to better select for those individuals at greatest risk of later developing AD-related cognitive decline. We found that both higher self- and partner-reported SMCs at baseline were associated with a faster annual rate of cognitive decline in NC and MCI participants after controlling for diagnostic group (Table 4, lower panels). There were no significant relationships between SMCs and change in MMSE scores in either NC or MCI groups when analyzed separately (data not shown).
SMCs and functional decline
To evaluate the relationship between baseline self- and partner-reported SMCs and functional decline, linear mixed models were again performed in participants with NC, MCI, and in a combined group of both NC and MCI. In the combined NC and MCI cohort, higher partner-reported SMCs were associated with a more rapid annual rate of functional decline while controlling for diagnostic group (Table 5, lower panel). In the NC group, somewhat unexpectedly, higher self-reported SMCs were associated with a slower rate of functional decline as measured by the FRS (Table 5, upper panel). No significant relationships were found between baseline SMCs and rate of functional decline over the study period in the MCI group (Supplementary Table 7). As with cognitive decline, above, we also evaluated the relationships between SMCs and functional decline in an amyloid+ subgroup. No significant relationships emerged between SMCs and change in FRS scores in NC, MCI, or the combined group in amyloid positive individuals (data not shown).
Table 5
SMCs and baseline factors affecting functional decline
95% Confidence Interval | |||||
Fixed Terms in final model | Estimate | Std. Err. | p | Lower Bound | Upper Bound |
NC | |||||
PRMQ Self×Time (y) | –0.0114 | 0.005 | 0.028 | –0.0216 | –0.0012 |
FRS at baseline | 0.8428 | 0.031 | < 0.001 | 0.7825 | 0.9031 |
PRMQ Self | 0.0009 | 0.006 | 0.876 | –0.0106 | 0.0125 |
Time (y) | 0.5139 | 0.200 | 0.010 | 0.1226 | 0.9052 |
NC + MCI | |||||
PRMQ Proxy×Time (y) | 0.0197 | 0.007 | 0.005 | 0.0061 | 0.0334 |
FRS at baseline | 0.8315 | 0.029 | < 0.001 | 0.7737 | 0.8892 |
PRMQ Proxy | 0.0089 | 0.009 | 0.329 | –0.0090 | 0.0269 |
Time (years) | –0.2670 | 0.218 | 0.220 | –0.6936 | 0.1596 |
Diagnosis (MCI) | 1.336 | 0.179 | < 0.001 | –0.8255 | 0.1732 |
The above fixed effects remained in the final model following optimization with backward selection. Random effects include a random intercept for each subject and a random slope for time in years, for which model statistics are not shown. MCI, mild cognitive impairment; NC, normal cognition; PRMQ, Prospective Retrospective Memory Questionnaire.
SMCs and diagnostic conversion
Cox-proportional hazards models were used to assess whether baseline SMCs predicted subsequent diagnostic progression. A total of 25 participants initially in the NC and MCI groups progressed to either MCI or AD over a mean follow up of 4.2 years. Partner-reported SMCs at baseline predicted progression to a different diagnostic category over the study period, whereby a ten-point increase of baseline PRMQ Proxy scores increased the annual risk of progression by approximately 70% (Table 6). Notably, and as expected, baseline MMSE scores also predicted diagnostic progression, while no other demographic variables were predictive. When including baseline diagnosis as a covariate, the relationship between SMCs and diagnostic progression was no longer significant. Neither measure of self-reported SMCs (PRMQ Self or GDS item 10) predicted diagnostic progression over the study period (p = 0.335 and p = 0.105, respectively).
Table 6
Baseline partner-reported SMCs and risk of diagnostic conversion
95% Confidence Interval | ||||||
10-point Hazard Ratio | β estimate | Std. Err. | p | Lower Bound | Upper Bound | |
PRMQ Self | ||||||
PRMQ Self | 1.2888 | 0.02537 | 0.023 | 0.251 | 0.9821 | 1.0713 |
Sex | 0.0002 | –0.8398 | 0.156 | 0.020 | 0.2130 | 0.8759 |
MMSE | 0.0073 | –0.4092 | 0.057 | < 0.001 | 0.5621 | 0.7848 |
PRMQ Proxy | ||||||
PRMQ Proxy | 1.6959 | 0.0528 | 0.021 | 0.009 | 1.1424 | 2.5186 |
MMSE | 0.0043 | –0.5459 | 0.083 | < 0.001 | 0.0002 | 0.0710 |
GDS Item 10 | ||||||
GDS Item 10 | 5.7604 | 0.1751 | 0.765 | 0.104 | 0.8739 | 4.1885 |
Sex | 0.0002 | –0.8435 | 0.156 | 0.020 | 0.2115 | 0.8751 |
MMSE | 0.0124 | –0.4388 | 0.051 | < 0.001 | 0.5528 | 0.7520 |
Only covariates remaining statistically significant after model optimization after backward selection are shown. PRMQ, Prospective Retrospective Memory Questionnaire; MMSE, Mini-Mental State Examination.
DISCUSSION
Reliable methods for early detection of preclinical disease are greatly needed to implement effective prevention and treatment of AD. A patient’s experience of SMCs may represent one of the earliest points in time when they will seek medical care for their cognitive health. It is therefore critical to better understand the utility of SMCs in predicting cognitive decline. Furthermore, evaluation of SMCs is efficient, and correlating measures of SMCs with known biomarkers of AD would expand dementia screening and identify those in need of further work-up. In the present study, we aimed to evaluate the relationships between self- and study partner-reported SMCs, objective cognitive performance, AD biomarkers, and risk of future decline in a well-characterized longitudinal memory center cohort. Additionally, we sought to evaluate the relationship between study partner characteristics and their report of cognitive change in their family member/friend. We found that, in general, study partner-reported SMCs were more consistent with cognitive testing and increasing symptom severity than self-reported SMCs. Partner-reported SMC also correlated better with longitudinal cognitive and functional decline. Additionally, we found that depression significantly influenced self- but not study partner-reported SMCs, while partner age, sex, education, and relationship to the study participant, particularly within the AD group, were associated with their reports of SMCs in their partners.
Importantly, this study replicates and adds to a growing body of literature on differences in self- and study partner-reported SMCs in the older adult population, including those at-risk for cognitive decline due to AD. With regard to self-reported SMCs, it is interesting to note that MCI participants reported the highest degree of subjective memory impairment, while individuals with NC and AD reported equal but lower average levels of SMCs. This highlights that the participants with AD demonstrated poor insight into their own memory impairment. Additionally, NC participants are reporting more impairment relative to proxy reports. The extent of SMCs reported by NC participants in this study may be biased by self-selection to participate in research studies focused on memory and aging.
We also found that higher self-reported SMCs at baseline reflected objective memory impairment in the AD group only, while higher self-reported SMCs in NC were associated with worse performance on tasks of processing speed and category fluency. In considering the finding in NC group, it is well-established that processing speed declines with age [71]. The association between SMCs and processing speed, and not memory performance, suggests that NC participant reported SMCs may represent their perceived changes in cognition, more broadly. It is not uncommon for individuals with non-memory cognitive changes to label their concerns as memory impairment. Indeed, NC participants may be perceiving some degree of cognitive decline that are associated with neuroanatomic changes, as NC participants with higher self-reported SMCs exhibited more pronounced brain atrophy indicative of advanced brain aging (SPARE-BA). Such patterns of atrophy in NC participants have previously been associated with deficits in executive function but not in memory domains, similar to our cohort [45]. In longitudinal analyses, higher self-reported SMCs at baseline were not associated with cognitive, functional, or diagnostic decline in the total cohort over the study period. In fact, greater memory complaints in NC were associated with slower functional decline over 4 years of follow up, though a longer study period is typically needed to capture true decline in participants with normal cognition at baseline. The need for prolonged follow up is likely due to the substantial heterogeneity within NC participants. In our cohort, only 27% of NC and 59% of MCI participants with amyloid PET scans had cerebral amyloid and were thus at higher risk of developing AD. In contrast to the findings in the total cohort, a subgroup analysis of amyloid+ individuals did show an association between self-reported SMCs and memory impairment at baseline as well as cognitive decline in NC and MCI over the study period. This suggests that self-reported SMCs may be more useful when applied to a subset of participants with increased risk of progressing to AD.
Despite there being few relationships between self-reported SMCs and cognitive/functional status in the total cohort, study partner-reported SMCs were more consistently associated with objective memory performance in both MCI and AD groups, again suggesting that study participants experience reduced insight as symptom severity increases. Similar findings were demonstrated in longitudinal follow-up, wherein higher study partner-reported SMCs at baseline were associated with more rapid cognitive and functional decline in NC and MCI participants over 4 years of follow up. A subgroup analysis in amyloid+ individuals re-demonstrated the association between partner-reported SMCs and cognitive but not functional decline over the study period, which may indicate that the reports by study partners are sensitive to cognitive changes associated with AD pathology. Separately, partner-reported SMCs were also associated with diagnostic progression in NC and MCI participants over the study period, whereby a ten-point increase in PRMQ proxy score at baseline increased the annual risk of diagnostic progression by 70%. Taken together, these findings highlight the potential for the PRMQ proxy to be used for prognostication in the memory clinic setting and bears replication in additional independent cohorts to inform generalizability.
It should be noted that while the finding that study partner SMCs strongly correlate with cognition and decline is consistent with much of the prior literature [17, 19, 20, 72–74], other reports demonstrate a robust relationship with self-reported SMCs [15, 18, 75]. Discrepancies are likely due to methodologic differences, including the populations studied as well as the metrics used to measure cognition, function, and SMCs. While the total study population is derived from a memory center cohort, the NC group is comprised of volunteers from the community. Discrepancies between self-reported SMCs, objective cognitive performance and neuroimaging biomarkers have been shown in community samples [76, 77], and perhaps explains the weaker associations observed between self-reported SMCs and these data in our sample. Furthermore, there is no consensus in the field regarding the most sensitive SMC questionnaires to detect meaningful cognitive change, and, as such, the varied implementation of SMC questionnaires in research limit comparison across studies and between participants and study partners [16, 20, 70, 73, 78]. Key advantages of the PRMQ are the analogous self and study partner questionnaires and the large dynamic range compared to shorter questionnaires with binary outcomes [55, 56].
It is widely accepted that characteristics of an individual, such as age, sex, education, and mood, can influence perception of one’s own impairment. Indeed, depressive symptoms in this cohort were strongly associated with self-reported SMCs across all diagnostic groups, as previously reported [13, 20, 22, 23]. Though much effort has been dedicated to identifying which attributes influence an individual’s perception of memory performance, relatively little is known about the ways in which study partner characteristics influence their report of patients’ cognition. This is an important area of study given the degree to which clinicians and researchers rely on partner reports to corroborate patient histories and fully evaluate a patient’s cognitive and functional status. Research to-date has focused on the effects of personality traits and affective symptoms in the partner, which have found that, like patients, greater depressive/anxiety symptoms [29] as well as a higher degree of neuroticism [28] correlates with greater reports of SMCs in community-dwelling individuals. We therefore sought to edify the existing literature by evaluating how additional study partner characteristics influence their reports across a range of diagnoses in the memory clinic setting. We demonstrated that younger age, female sex, and higher education were associated with greater study partner-reported SMCs in the AD group only, which may be explained by the greater reports of impairment by partners that are children compared to spouses, considering that the child partners are more likely to be younger females. Additionally, there was a trend for a stronger correlation between children-reported SMCs and objective cognitive performance compared to those reported by spouses of patients with AD. This may be explained by a variety of factors, such as a tendency to minimize the symptoms of disease, frequency of observations, or cognitive decline in similarly aged spouses [1]. It is not currently standard practice to systematically evaluate the cognitive status or other attributes of study partners that present to a clinic with a patient. As an alternative to such measures, Buchanan et al. recently called for the identification of certain SMC scales or sub-scales that are less susceptible to partner reporting bias [28]. Further work is needed to determine the degree to which particular items or sub-scales of the PRMQ or similar questionnaires are influenced by partner characteristics and biases. Moreover, it is interesting to note that frequency of study contact also influenced partner reports, particularly within the NC and AD group. Study partners with less frequent contact tended to under-report SMCs in NC participants and to over-report impairment in AD participants. This suggests that partners with less frequent contact may be less reliable, and given the greater proportion of partners with infrequent contact in NC participants, it may also explain the lack of association between partner SMC reports and cognitive outcomes in the NC group.
In keeping with the field’s move toward a neuropathological definition of AD, we also evaluated the relationship between self- and study partner-reported SMCs and plasma and neuroimaging biomarkers of AD in order to identify those individuals that may be at increased risk for development of AD. Neuroimaging biomarkers explored in this study include subregional MTL atrophy and indices that identify global patterns of brain atrophy associated with AD and aging, SPARE-AD and SPARE-BA, respectively. Each of these measurements possess key advantages in evaluating neuroanatomic correlates of cognitive decline. MTL subregion segmentation provides for evaluation of subtle structural changes in the regions first impacted by tau deposition, which typically begins in BA35, followed by the entorhinal cortex, and the hippocampus [79]. The SPARE-AD and SPARE-BA indices consider all brain regions to identify regions whose volumes maximally differentiate the populations of interest: older from younger in SPARE-BA, and AD from NC in SPARE-AD. In the present study, higher SMCs were reported by study partners of MCI participants who had an atrophy pattern more consistent with AD as well as specific subregion volume loss in the MTL, a region affected early by tau pathology. Similarly, elevated plasma NfL, GFAP, and p-tau181 concentrations have been associated with AD and increased dementia risk [46–50], and partner-reported SMCs were associated with higher levels of plasma GFAP. These neuroimaging and plasma biomarker findings in conjunction with the aforementioned neurocognitive data further strengthens the assertion that study partners can perceive objective changes associated with the neurodegenerative processes underlying AD.
In contrast, self-reported SMCs did not correlate with global patterns of AD-related brain atrophy and instead were associated with patterns of atrophy seen in advanced brain aging (SPARE-BA), as reported above. The lack of association with SPARE-AD may provide a possible neuroanatomic explanation for why self-reported SMCs were not associated with objective memory performance or diagnostic progression. However, self-reported SMCs did correlate with volumetric reductions in certain regions of the MTL in both NC and MCI. Several factors could influence the apparent discrepancy in these findings. First, it has previously been shown that atrophy in the MTL and in the temporal lobe more broadly occurs in physiologic aging [44, 80]. MTL atrophy observed in NC may therefore be reflective of advanced aging as suggested by the association between SPARE-BA and self-reported SMCs. Alternatively, it is possible that self-reported SMCs in NC subject are inconsistent at identifying cognitive, functional, and neuroanatomic changes associated with AD due to methodologic limitations. For instance, it is possible that the cognitive measures included in this study from UDS versions 2 and 3 are not sensitive enough to detect early and more subtle memory changes in the NC population [81], as other cognitive measures utilized in studies of preclinical AD have been shown to be more sensitive to early changes [81, 82]. Furthermore, there are generally low rates of progression to dementia in a memory center population with normal cognition, especially over a follow up period of less than ten years [7, 83]. Longer term follow-up is needed to detect meaningful associations between self-reported SMCs and diagnostic change in NC participants, a concept that is supported by a series of studies on SMCs and dementia in the same longitudinal cohort with varying lengths of follow-up [20, 84].
Strengths of this study include the large sample size, longitudinal study design with an average of four years of follow up, and availability of neuropsychiatric, neuropsychological and neuroimaging data. We also describe the relationship between partner characteristics and perceived impairment of the study participants in a memory center population. One limitation of our study is incomplete characterization of the study cohort: only a subset of participants had data available for various disease biomarkers, and additional medical co-morbidities impacting cognition and cognitive decline (e.g., cerebrovascular disease) were not considered in our analyses. In light of these sample limitations, our amyloid+ subgroup was expanded to include individuals who had an amyloid PET scan within five years of clinical testing, which could conceivably lead to mischaracterization of subjects as amyloid positive when they were negative at the time of cognitive testing. However, data from longitudinal cohorts suggest that conversion from amyloid negative to positive in cognitively normal individuals typically occurs over a longer time period [85]. Other important neuroimaging biomarkers were similarly not included, such as regional glucose hypometabolism measured by FDG-PET, which has been shown to correlate with subjective cognitive decline [86–89]. Subsequent studies should aim to better account for these important factors. Additionally, the majority of our subjects were White, and efforts are currently underway to replicate these findings in a more diverse cohort. Another limitation of all single center studies is generalizability; however, our findings with regard to objective cognitive impairment and subsequent decline are supported by two recent, large longitudinal analyses: one in a memory center cohort in the Netherlands [73] and another in a community-dwelling population in Australia [20]. It has also been suggested that utilizing a memory center population may not be representative of the community at large. Our conception of the clinical relevance of this work is in the development of a screening tool for future cognitive and functional decline; therefore, it will be important to replicate this study in a population of individuals presenting with memory complaints in the primary care setting as well.
In summary, the present study demonstrated that study partner-reported SMCs correlate with measures of objective impairment and greater AD-associated brain atrophy and are also predictive of future decline over four years of follow up. Furthermore, we demonstrated that study partner characteristics, especially the relationship to the study participant and frequency of contact, should be considered when interpreting their reports, especially for older and more impaired patients. Further work is needed to determine the best tool to evaluate both self- and study partner-reported SMCs in the memory center context and to validate these findings in an independent, well-characterized, longitudinal cohort over a longer follow-up period.
ACKNOWLEDGMENTS
The authors would like to thank the participants who generously gave their time and the dedicated clinicians and research staff of the Penn Memory Center and Penn Alzheimer’s Disease Research Center, without whose efforts this study could not have been conducted.
FUNDING
The research was supported by grants from the National Institutes of Health (P30-AG010124; K23AG065499) and the donors of Alzheimer’s Disease Research, a program of the BrightFocus Foundation.
CONFLICT OF INTEREST
IMN receives compensation as an educational speaker for Biogen. LX received personal consulting fees from Galileo CDS, Inc. All other authors have no relevant conflics of interest to report.
SUPPLEMENTARY MATERIAL
[1] The supplementary material is available in the electronic version of this article: https://dx.doi.org/10.3233/ADR-220013.
REFERENCES
[1] | ((2020) ) 2020 Alzheimer’s disease facts and figures. Alzheimers Dement 16: , 391–460. |
[2] | Sperling RA , Aisen PS , Beckett LA , Bennett DA , Craft S , Fagan AM , Iwatsubo T , Jack CR , Kaye J , Montine TJ , Park DC , Reiman EM , Rowe CC , Siemers E , Stern Y , Yaffe K , Carrillo MC , Thies B , Morrison-Bogorad M , Wagster MV , Phelps CH ((2011) ) Toward defining the preclinical stages of Alzheimer’s disease: Recommendations from the National Institute on Aging-Alzheimer’s Association workgroups on diagnostic guidelines for Alzheimer’s disease. Alzheimers Dement 7: , 280–292. |
[3] | Fiandaca MS , Mapstone ME , Cheema AK , Federoff HJ ((2014) ) The critical need for defining preclinical biomarkers in Alzheimer’s disease. Alzheimers Dement 10: (3 Suppl), S196–S212. |
[4] | Dubois B , Hampel H , Feldman HH , Scheltens P , Aisen P , Andrieu S , Bakardjian H , Benali H , Bertram L , Blennow K , Broich K , Cavedo E , Crutch S , Dartigues J-F , Duyckaerts C , Epelbaum S , Frisoni GB , Gauthier S , Genthon R , Gouw AA , Habert M-O , Holtzman DM , Kivipelto M , Lista S , Molinuevo J-L , O’Bryant SE , Rabinovici GD , Rowe C , Salloway S , Schneider LS , Sperling R , Teichmann M , Carrillo MC , Cummings J , Jack CR , Proceedings of the Meeting of the International Working Group (IWG) and the American Alzheimer’s Association on “The Preclinical State of AD”; July 23, 2015; Washington DC, USA ((2016) ) Preclinical Alzheimer’s disease: Definition, natural history, and diagnostic criteria. Alzheimers Dement 12: , 292–323. |
[5] | Leifer BP ((2003) ) Early diagnosis of Alzheimer’s disease: Clinical and economic benefits. J Am Geriatr Soc 51: (5 Suppl Dementia), S281–S288. |
[6] | Sperling R , Mormino E , Johnson K ((2014) ) The evolution of preclinical Alzheimer’s disease: Implications for prevention trials. Neuron 84: , 608–622. |
[7] | Jessen F , Amariglio RE , van Boxtel M , Breteler M , Ceccaldi M , Chételat G , Dubois B , Dufouil C , Ellis KA , van der Flier WM , Glodzik L , van Harten AC , de Leon MJ , McHugh P , Mielke MM , Molinuevo J , Mosconi L , Osorio RS , Perrotin A , Petersen RC , Rabin LA , Rami L , Reisberg B , Rentz DM , Sachdev PS , de la Sayette V , Saykin AJ , Scheltens P , Shulman MB , Slavin MJ , Sperling RA , Stewart R , Uspenskaya O , Vellas B , Visser P , Wagner M , Group S ((2014) ) A conceptual framework for research on subjective cognitive decline in preclinical Alzheimer’s disease. Alzheimers Dement 10: , 844–852. |
[8] | Reisberg B , Prichep L , Mosconi L , John RE , Glodzik-Sobanska L , Boksay I , Monteiro I , Torossian C , Vedvyas A , Ashraf N , Jamil IA , de Leon MJ ((2008) ) The pre–mild cognitive impairment, subjective cognitive impairment stage of Alzheimer’s disease. Alzheimers Dement 4: (1 Suppl 1), S98–S108. |
[9] | Garcia-Ptacek S , Eriksdotter M , Jelic V , Porta-Etessam J , Kåreholt I , Palomo MS ((2016) ) Quejas cognitivas subjetivas: Hacia una identificación precoz de la enfermedad de Alzheimer.í. a 31: , 562–571. |
[10] | van Wanrooij LL , Richard E , Jongstra S , Moll van Charante EP , van Gool WA ((2019) ) Associations of subjective memory complaints and simple memory task scores with future dementia in the primary care setting. Ann Fam Med 17: , 412–418. |
[11] | Choe YM , Byun MS , Lee JH , Sohn BK , Lee DY , Kim JW ((2018) ) Subjective memory complaint as a useful tool for the early detection of Alzheimer’s disease. Neuropsychiatr Dis Treat 14: , 2451–2460. |
[12] | Ramlall S , Chipps J , Bhigjee AI , Pillay BJ ((2013) ) The sensitivity and specificity of subjective memory complaints and the subjective memory rating scale, deterioration cognitive observee, mini-mental state examination, six-item screener and clock drawing test in dementia screening. Dement Geriatr Cogn Disord 36: , 119–135. |
[13] | Buckley R , Saling MM , Ames D , Rowe CC , Lautenschlager NT , Macaulay SL , Martins RN , Masters CL , O’Meara T , Savage G , Szoeke C , Villemagne VL , Ellis KA , and of Group A ((2013) ) Factors affecting subjective memory complaints in the AIBL aging study: Biomarkers, memory, affect, and age. Int Psychogeriatr 25: , 1307–1315. |
[14] | Blackburn DJ , Wakefield S , Shanks MF , Harkness K , Reuber M , Venneri A ((2014) ) Memory difficulties are not always a sign of incipient dementia: A review of the possible causes of loss of memory efficiency. Br Med Bull 112: , 71–81. |
[15] | Caselli RJ , Chen K , Locke D , Lee W , Roontiva A , Bandy D , Fleisher AS , Reiman EM ((2014) ) Subjective cognitive decline: Self and informant comparisons. Alzheimers Dement 10: , 93–98. |
[16] | Rabin LA , Smart CM , Amariglio RE ((2017) ) Subjective cognitive decline in preclinical Alzheimer’s disease. Ann Rev Clin Psychol 13: , 369–396. |
[17] | Nicholas CR , Dowling MN , Racine AM , Clark LR , Berman SE , Koscik RL , Asthana S , Hermann B , Sager MA , Johnson SC ((2017) ) Longitudinal assessment of self- and informant-subjective cognitive complaints in a sample of healthy late-middle aged adults enriched with a family history of Alzheimer’s disease. J Int Neuropsychol Soc 23: , 617–626. |
[18] | Koppara A , Wagner M , Lange C , Ernst A , Wiese B , König H-H , Brettschneider C , Riedel-Heller S , Luppa M , Weyerer S , Werle J , Bickel H , Mösch E , Pentzek M , Fuchs A , Wolfsgruber S , Beauducel A , Scherer M , Maier W , Jessen F ((2015) ) Cognitive performance before and after the onset of subjective cognitive decline in old age. Alzheimers Dement (Amst) 1: , 194–205. |
[19] | Valech N , Mollica MA , Olives J , Tort A , Fortea J , Lleo A , Belén S-S , Molinuevo J , Rami L ((2015) ) Informants’ perception of subjective cognitive decline helps to discriminate preclinical Alzheimer’s disease from normal aging. J Alzheimers Dis 48: (Suppl 1), S87–S98. |
[20] | Numbers K , Crawford JD , Kochan NA , Draper B , Sachdev PS , Brodaty H ((2020) ) Participant and informant memory-specific cognitive complaints predict future decline and incident dementia: Findings from the Sydney Memory and Ageing Study. PloS One 15: , e0232961. |
[21] | Tandetnik C , Farrell MT , Cary MS , Cines S , Emrani S , Karlawish J , Cosentino S ((2015) ) Ascertaining subjective cognitive decline: A comparison of approaches and evidence for using an age-anchored reference group. J Alzheimers Dis 48: (Suppl 1), S43–S55. |
[22] | Zlatar ZZ , Muniz M , Galasko D , Salmon DP ((2018) ) Subjective cognitive decline correlates with depression symptoms and not with concurrent objective cognition in a clinic-based sample of older adults. J Gerontol B Psychol Sci Soc Sci 73: , 1198–1202. |
[23] | Crane MK , CE BHR , Brown GK , Gallo JJ ((2007) ) The link between depressive symptoms, negative cognitive bias and memory complaints in older adults. Aging Mental Health 11: , 708–715. |
[24] | Plotkin DA , Mintz J , Jarvik LF ((1985) ) Subjective memory complaints in geriatric depression. Am J Psychiatry 142: , 1103–1105. |
[25] | Slavin MJ , Brodaty H , Kochan NA , Crawford JD , Trollor JN , Draper B , Sachdev PS ((2010) ) Prevalence and predictors of “subjective cognitive complaints” in the Sydney Memory and Ageing Study. Am J Geriatr Psychiatry 18: , 701–710. |
[26] | Rattanabannakit C , Risacher SL , Gao S , Lane KA , Brown SA , McDonald BC , Unverzagt FW , Apostolova LG , Saykin AJ , Farlow MR ((2016) ) The Cognitive Change Index as a measure of self and informant perception of cognitive decline: Relation to neuropsychological tests. J Alzheimers Dis 51: , 1145–1155. |
[27] | Rabin LA , Wang C , Katz MJ , Derby CA , Buschke H , Lipton RB ((2012) ) Predicting Alzheimer’s disease: Neuropsychological tests, self-reports, and informant reports of cognitive difficulties. J Am Geriatr Soc 60: , 1128–1134. |
[28] | Buchanan T , Loveday C ((2018) ) Informant personality is associated with ratings of memory problems in older adults. Am J Alzheimers Dis Other Demen 33: , 479–489. |
[29] | Jorm AF , Christensen H , Henderson AS , Korten AE , Mackinnon AJ , Scott R ((1994) ) Complaints of cognitive decline in the elderly: A comparison of reports by subjects and informants in a community survey. Psychol Med 24: , 365–374. |
[30] | Buckley R , Saling M , Ellis K , Rowe C , Maruff P , Macaulay LS , Martins R , Masters C , Savage G , Rainey-Smith S , Rembach A , Ames D ((2015) ) Self and informant memory concerns align in healthy memory complainers and in early stages of mild cognitive impairment but separate with increasing cognitive impairment. Age Ageing 44: , 1012–1019. |
[31] | Snitz BE , Lopez OL , McDade E , Becker JT , Cohen AD , Price JC , Mathis CA , Klunk WE ((2015) ) Amyloid-β imaging in older adults presenting to a memory clinic with subjective cognitive decline: A pilot study. J Alzheimers Dis 48: (Suppl 1), S151–S159. |
[32] | Amariglio RE , Becker AJ , Carmasin J , Wadsworth LP , Lorius N , Sullivan C , Maye JE , Gidicsin C , Pepin LC , Sperling RA , Johnson KA , Rentz DM ((2012) ) Subjective cognitive complaints and amyloid burden in cognitively normal older individuals. Neuropsychologia 50: , 2880–2886. |
[33] | Saykin AJ , Wishart HA , Rabin LA , Santulli RB , Flashman LA , West JD , McHugh TL , Mamourian AC ((2006) ) Older adults with cognitive complaints show brain atrophy similar to that of amnestic MCI. Neurology 67: , 834–842. |
[34] | van der Flier WM , van Buchem MA , Weverling-Rijnsburger AWE , Mutsaers ER , Bollen ELEM , Admiraal-Behloul F , Westendorp RGJ , Middelkoop HAM ((2004) ) Memory complaints in patients with normal cognition are associated with smaller hippocampal volumes. J Neurol 251: , 671–675. |
[35] | Rogne S , Vangberg T , Eldevik P , Wikran G , Mathiesen EB , Schirmer H ((2016) ) Magnetic resonance volumetry: Prediction of subjective memory complaints and mild cognitive impairment, and associations with genetic and cardiovascular risk factors. Dement Geriatr Cogn Dis Extra 6: , 529–540. |
[36] | Wolk DA , Das SR , Mueller SG , Weiner MW , Yushkevich PA , Alzheimer’s Disease Neuroimaging Initiative ((2017) ) Medial temporal lobe subregional morphometry using high resolution MRI in Alzheimer’s disease. Neurobiol Aging 49: , 204–213. |
[37] | Xie L , Wisse LEM , Pluta J , de Flores R , Piskin V , Manjón JV , Wang H , Das SR , Ding SL , Wolk DA , Yushkevich PA ((2019) ) Automated segmentation of medial temporal lobe subregions onT1-weighted MRI in early stages of Alzheimer’s disease. Hum Brain Mapp 40: , 3431–3451. |
[38] | Davatzikos C , Xu F , An Y , Fan Y , Resnick SM ((2009) ) Longitudinal progression of Alzheimer’s-like patterns of atrophy in normal older adults: The SPARE-AD index. Brain 132: , 2026–2035. |
[39] | Da X , Toledo JB , Zee J , Wolk DA , Xie SX , Ou Y , Shacklett A , Parmpi P , Shaw L , Trojanowski JQ , Davatzikos C ((2014) ) Integration and relative value of biomarkers for prediction of MCI to AD progression: Spatial patterns of brain atrophy, cognitive scores, APOE genotype and CSF biomarkers. Neuroimage Clin 4: , 164–173. |
[40] | Fan Y , Batmanghelich N , Clark CM , Davatzikos C ((2008) ) Spatial patterns of brain atrophy in MCI patients, identified via high-dimensional pattern classification, predict subsequent cognitive decline. Neuroimage 39: , 1731–1743. |
[41] | Toledo JB , Bjerke M , Chen K , Rozycki M , Jack CR Jr. , Weiner MW , Arnold SE , Reiman EM , Davatzikos C , Shaw LM , Trojanowski JQ ((2015) ) Memory, executive, and multidomain subtle cognitive impairment: Clinical and biomarker findings. Neurology 85: , 144–153. |
[42] | Toledo JB , Weiner MW , Wolk DA , Da X , Chen K , Arnold SE , Jagust W , Jack C , Reiman EM , Davatzikos C , Shaw LM , Trojanowski JQ ((2014) ) Neuronal injury biomarkers and prognosis in ADNI subjects with normal cognition. Acta Neuropathol Commun 2: , 26. |
[43] | Eavani H , Habes M , Satterthwaite TD , An Y , Hsieh M-K , Honnorat N , Erus G , Doshi J , Ferrucci L , Beason-Held LL , Resnick SM , Davatzikos C ((2018) ) Heterogeneity of structural and functional imaging patterns of advanced brain aging revealed via machine learning methods. Neurobiol Aging 71: , 41–50. |
[44] | Habes M , Janowitz D , Erus G , Toledo JB , Resnick SM , Doshi J , Van der Auwera S , Wittfeld K , Hegenscheid K , Hosten N , Biffar R , Homuth G , Völzke H , Grabe HJ , Hoffmann W , Davatzikos C ((2016) ) Advanced brain aging: Relationship with epidemiologic and genetic risk factors, and overlap with Alzheimer disease atrophy patterns. Transl Psychiatry 6: , e775. |
[45] | Habes M , Pomponio R , Shou H , Doshi J , Mamourian E , Erus G , Nasrallah I , Launer LJ , Rashid T , Bilgel M , Fan Y , Toledo JB , Yaffe K , Sotiras A , Srinivasan D , Espeland M , Masters C , Maruff P , Fripp J , Völzk H , Johnson SC , Morris JC , Albert MS , Miller MI , Bryan RN , Grabe HJ , Resnick SM , Wolk DA , Davatzikos C ((2021) ) The Brain Chart of Aging: Machine-learning analytics reveals links between brain aging, white matter disease, amyloid burden, and cognition in the iSTAGING consortium of 10,216 harmonized MR scans. Alzheimers Dement 17: , 89–102. |
[46] | Verberk IMW , Laarhuis MB , Bosch KAvd , Ebenau JL , Leeuwenstijn Mv , Prins ND , Scheltens P , Teunissen CE , Flier WMvd ((2021) ) Serum markers glial fibrillary acidic protein and neurofilament light for prognosis and monitoring in cognitively normal older people: A prospective memory clinic-based cohort study. Lancet Health Longev 2: , e87–e95. |
[47] | de Wolf F , Ghanbari M , Licher S , McRae-McKee K , Gras L , Weverling GJ , Wermeling P , Sedaghat S , Ikram MK , Waziry R , Koudstaal W , Klap J , Kostense S , Hofman A , Anderson R , Goudsmit J , Ikram MA ((2020) ) Plasma tau, neurofilament light chain and amyloid-β levels and risk of dementia; a population-based cohort study. Brain 143: , 1220–1232. |
[48] | Mielke MM , Syrjanen JA , Blennow K , Zetterberg H , Vemuri P , Skoog I , Machulda MM , Kremers WK , Knopman DS , Jack C Jr. , Petersen RC , Kern S ((2019) ) Plasma and CSF neurofilament light: Relation to longitudinal neuroimaging and cognitive measures. Neurology 93: , e252–e260. |
[49] | Oeckl P , Halbgebauer S , Anderl-Straub S , Steinacker P , Huss AM , Neugebauer H , von Arnim CAF , Diehl-Schmid J , Grimmer T , Kornhuber J , Lewczuk P , Danek A , Ludolph AC , Otto M ((2019) ) Glial fibrillary acidic protein in serum is increased in Alzheimer’s disease and correlates with cognitive impairment. J Alzheimers Dis 67: , 481–488. |
[50] | Rajan KB , Aggarwal NT , McAninch EA , Weuve J , Barnes LL , Wilson RS , DeCarli C , Evans DA ((2020) ) Remote blood biomarkers of longitudinal cognitive outcomes in a population study. Ann Neurol 88: , 1065–1076. |
[51] | Moscoso A , Grothe MJ , Ashton NJ , Karikari TK , Lantero Rodríguez J , Snellman A , Suárez-Calvet M , Blennow K , Zetterberg H , Schöll M ((2021) ) Longitudinal associations of blood phosphorylated tau181 and neurofilament light chain with neurodegeneration in Alzheimer disease. JAMA Neurol 78: , 396–406. |
[52] | Hansson O , Cullen N , Zetterberg H , Blennow K , Mattsson-Carlgren N ((2021) ) Plasma phosphorylated tau181 and neurodegeneration in Alzheimer’s disease. Ann Clin Transl Neurol 8: , 259–265. |
[53] | Xie SX , Baek Y , Grossman M , Arnold SE , Karlawish J , Siderowf A , Hurtig H , Elman L , McCluskey L , Van Deerlin V , Lee VM , Trojanowski JQ ((2011) ) Building an integrated neurodegenerative disease database at an academic health center. Alzheimers Dement 7: , e84–93. |
[54] | Roalf DR , Moberg PJ , Xie SX , Wolk DA , Moelter ST , Arnold SE ((2013) ) Comparative accuracies of two common screening instruments for classification of Alzheimer’s disease, mild cognitive impairment, and healthy aging. Alzheimers Dement 9: , 529–537. |
[55] | Crawford J , Smith G , Maylor E , Sala S , Logie R ((2003) ) The Prospective and Retrospective Memory Questionnaire (PRMQ): Normative data and latent structure in a large non-clinical sample. Memory 11: , 261–275. |
[56] | RÖNnlund M , MÄNtylÄ T , Nilsson LG ((2008) ) The Prospective and Retrospective Memory Questionnaire (PRMQ): Factorial structure, relations to global subjective memory ratings, and Swedish norms. Scand J Psychol 49: , 11–18. |
[57] | Weintraub S , Salmon D , Mercaldo N , Ferris S , Graff-Radford NR , Chui H , Cummings J , DeCarli C , Foster NL , Galasko D , Peskind E , Dietrich W , Beekly DL , Kukull WA , Morris JC ((2009) ) The Alzheimer’s Disease Centers’ Uniform Data Set (UDS): The Neuropsychologic Test Battery. Alzheimer Dis Assoc Disord 23: , 91–101. |
[58] | Weintraub S , Besser L , Dodge HH , Teylan M , Ferris S , Goldstein FC , Giordani B , Kramer J , Loewenstein D , Marson D , Mungas D , Salmon D , Welsh-Bohmer K , Zhou X-H , Shirk SD , Atri A , Kukull WA , Phelps C , Morris JC ((2018) ) Version 3 of the Alzheimer Disease Centers’ Neuropsychological Test Battery in the Uniform Data Set (UDS). Alzheimer Dis Assoc Disord 32: , 10–17. |
[59] | Monsell SE , Dodge HH , Zhou XH , Bu Y , Besser LM , Mock C , Hawes SE , Kukull WA , Weintraub S ((2016) ) Results from the NACC uniform data set neuropsychological battery crosswalk study. Alzheimer Dis Assoc Disord 30: , 134–139. |
[60] | Folstein MF , Folstein SE , McHugh PR ((1975) ) “Mini-mental state” A practical method for grading the cognitive state of patients for the clinician. J Psychiatr Res 12: , 189–198. |
[61] | Nasreddine ZS , Phillips NA , Bédirian V , Charbonneau S , Whitehead V , Collin I , Cummings JL , Chertkow H ((2005) ) The Montreal Cognitive Assessment, MoCA: A brief screening tool for mild cognitive impairment. J Am Geriatr Soc 53: , 695–699. |
[62] | Sheikh JI , Yesavage JA ((1986) ) Geriatric Depression Scale (GDS): Recent evidence and development of a shorter version. Clin Gerontol 5: , 165–173. |
[63] | Clark CM , Ewbank DC ((1996) ) Performance of the dementia severity rating scale: A caregiver questionnaire for rating severity in Alzheimer disease. Alzheimer Dis Assoc Disord 10: , 31–39. |
[64] | Tropea TF , Waligorska T , Xie SX , Nasralah IM , Cousins KAQ , Trojanowski JQ , Grossman M , Irwin DJ , Weintraub D , Lee EB , Wolk DA , Chen-Plotkin AS , Shaw LM ((2022) ) Plasma phosphorylated tau181 is a biomarker of Alzheimer’s disease pathology and associated with cognitive and functional decline. SSRN, https://ssrn.com/abstract=4007185 |
[65] | Aamodt WW , Waligorska T , Shen J , Tropea TF , Siderowf A , Weintraub D , Grossman M , Irwin D , Wolk DA , Xie SX , Trojanowski JQ , Shaw LM , Chen-Plotkin AS ((2021) ) Neurofilament light chain as a biomarker for cognitive decline in Parkinson disease. Mov Disord 36: , 2945–2950. |
[66] | Chatterjee P , Pedrini S , Stoops E , Goozee K , Villemagne VL , Asih PR , Verberk IMW , Dave P , Taddei K , Sohrabi HR , Zetterberg H , Blennow K , Teunissen CE , Vanderstichele HM , Martins RN ((2021) ) Plasma glial fibrillary acidic protein is elevated in cognitively normal older adults at risk of Alzheimer’s disease. Transl Psychiatry 11: , 27. |
[67] | Xie L , Das SR , Wisse LEM , Ittyerah R , Yushkevich PA , Wolk DA , Alzheimer’s Disease Neuroimaging Initiative ((2018) ) Early tau burden correlates with higher rate of atrophy in transentorhinal cortex. J Alzheimers Dis 62: , 85–92. |
[68] | Xie L , Pluta JB , Das SR , Wisse LEM , Wang H , Mancuso L , Kliot D , Avants BB , Ding S-L , Manjón JV , Wolk DA , Yushkevich PA ((2017) ) Multi-template analysis of human perirhinal cortex in brain MRI: Explicitly accounting for anatomical variability. Neuroimage 144: , 183–202. |
[69] | Habes M , Toledo JB , Resnick SM , Doshi J , Van der Auwera S , Erus G , Janowitz D , Hegenscheid K , Homuth G , Völzke H , Hoffmann W , Grabe HJ , Davatzikos C ((2016) ) Relationship between APOE genotype and structural MRI measures throughout adulthood in the study of health in Pomerania population-based cohort. AJNR Am J Neuroradiol 37: , 1636–1642. |
[70] | Chapman S , Sunderaraman P , Joyce JL , Azar M , Colvin LE , Barker MS , McKeague I , Kreisl WC , Cosentino S ((2021) ) Optimizing subjective cognitive decline to detect early cognitive dysfunction. J Alzheimers Dis 80: , 1185–1196. |
[71] | Park DC , Reuter-Lorenz P ((2009) ) The adaptive brain: Aging and neurocognitive scaffolding. Ann Rev Psychol 60: , 173–196. |
[72] | Rönnlund M , Sundström A , Adolfsson R , Nilsson LG ((2015) ) Self-reported memory failures: Associations with future dementia in a population-based study with long-term follow-up. J Am Geriatr Soc 63: , 1766–1773. |
[73] | Gruters AAA , Ramakers IHGB , Verhey FRJ , Köhler S , Kessels RPC , de Vugt ME ((2019) ) Association between proxy- or self-reported cognitive decline and cognitive performance in memory clinic visitors. J Alzheimers Dis 70: , 1225–1239. |
[74] | Rahman-Filipiak AM , Giordani B , Heidebrink J , Bhaumik A , Hampstead BM ((2018) ) Self- and informant-reported memory complaints: Frequency and severity in cognitively intact individuals and those with mild cognitive impairment and neurodegenerative dementias. J Alzheimers Dis 65: , 1011–1027. |
[75] | Dufouil C , Fuhrer R , Alpérovitch A ((2005) ) Subjective cognitive complaints and cognitive decline: Consequence or predictor? The Epidemiology of Vascular Aging Study. J Am Geriatr Soc 53: , 616–621. |
[76] | Sohel N , Tuokko H , Griffith L , Raina P ((2016) ) Factors influencing discrepancies in self-reported memory and performance on memory recall in the Canadian Community Health Survey-Healthy Aging, 2008-09. Age Ageing 45: , 280–286. |
[77] | Perrotin A , Joie R , de Sayette V , Barré L , Mézenge F , Mutlu J , Guilloteau D , Egret S , Eustache F , Chételat G ((2017) ) Subjective cognitive decline in cognitively normal elders from the community or from a memory clinic: Differential affective and imaging correlates. Alzheimers Dement 13: , 550–560. |
[78] | Rabin LA , Smart CM , Crane PK , Amariglio RE , Berman LM , Boada M , Buckley RF , Chételat G , Dubois B , Ellis KA , Gifford KA , Jefferson AL , Jessen F , Katz MJ , Lipton RB , Luck T , Maruff P , Mielke MM , Molinuevo J , Naeem F , Perrotin A , Petersen RC , Rami L , Reisberg B , Rentz DM , Riedel-Heller SG , Risacher SL , Rodriguez O , Sachdev PS , Saykin AJ , Slavin MJ , Snitz BE , Sperling RA , Tandetnik C , van der Flier WM , Wagner M , Wolfsgruber S , Sikkes SAM ((2015) ) Subjective cognitive decline in older adults: An overview of self-report measures used across 19 international research studies. J Alzheimers Dis 48: (Suppl 1), S63–S86. |
[79] | Braak H , Braak E ((1991) ) Neuropathological stageing of Alzheimer-related changes. Acta Neuropathol 82: , 239–259. |
[80] | Stoub TR , Barnes CA , Shah RC , Stebbins GT , Ferrari C , deToledo-Morrell L ((2012) ) Age-related changes in the mesial temporal lobe: The parahippocampal white matter region. Neurobiol Aging 33: , 1168–1176. |
[81] | Donohue MC , Sperling RA , Salmon DP , Rentz DM , Raman R , Thomas RG , Weiner M , Aisen PS , Australian Imaging, Biomarkers, and Lifestyle Flagship Study of Ageing; Alzheimer’s Disease Neuroimaging Initiative; Alzheimer’s Disease Cooperative Study ((2014) ) The preclinical Alzheimer cognitive composite: Measuring amyloid-related decline. JAMA Neurol 71: , 961–970. |
[82] | Papp KV , Rentz DM , Orlovsky I , Sperling RA , Mormino EC ((2017) ) Optimizing the preclinical Alzheimer’s cognitive composite with semantic processing: The PACC5. Alzheimers Dement (N Y) 3: , 668–677. |
[83] | Hessen E , Eckerström M , Nordlund A , Almdahl I , Stålhammar J , Bjerke M , Eckerström C , Göthlin M , Fladby T , Reinvang I , Wallin A ((2017) ) Subjective cognitive impairment is a predominantly benign condition in memory clinic patients followed for 6 years: The Gothenburg-Oslo MCI Study. Dement Geriatr Cogn Disord Extra 7: , 1–14. |
[84] | Slavin MJ , Sachdev PS , Kochan NA , Woolf C , Crawford JD , Giskes K , Reppermund S , Trollor JN , Draper B , Delbaere K , Brodaty H ((2014) ) Predicting cognitive, functional, and diagnostic change over 4 years using baseline subjective cognitive complaints in the Sydney Memory and Ageing Study. Am J Geriatr Psychiatry 23: , 906–914. |
[85] | Hatashita S , Wakebe D ((2021) ) Longitudinal assessment of amyloid-beta deposition in initially amyloid-negative non-demented individuals with [11C]-PIB PET imaging. Medicine 100: , e27055. |
[86] | Vannini P , Hanseeuw B , Munro CE , Amariglio RE , Marshall GA , Rentz DM , Pascual-Leone A , Johnson KA , Sperling RA ((2017) ) Hippocampal hypometabolism in older adults with memory complaints and increased amyloid burden. Neurology 88: , 1759–1767. |
[87] | Vergallo A , Lista S , Zhao Y , Lemercier P , Teipel SJ , Potier MC , Habert MO , Dubois B , Lukiw WJ , Hampel H ((2021) ) MiRNA-15b and miRNA-125b are associated with regional Aβ-PET and FDG-PET uptake in cognitively normal individuals with subjective memory complaints. Transl Psychiatry 11: , 78. |
[88] | Nobili F , Mazzei D , Dessi B , Morbelli S , Brugnolo A , Barbieri P , Girtler N , Sambuceti G , Rodriguez G , Pagani M ((2010) ) Unawareness of memory deficit in amnestic MCI: FDG-PET findings. J Alzheimers Dis 22: , 993–1003. |
[89] | Dong QY , Li TR , Jiang XY , Wang XN , Han Y , Jiang JH ((2021) ) Glucose metabolism in the right middle temporal gyrus could be a potential biomarker for subjective cognitive decline: A study of a Han population. Alzheimers Res Ther 13: , 74. |