Is everything under control? An experimental study on how control over data influences trust in and support for major governmental data exchange projects
Abstract
The steep rise in the exchange of (citizen) data through government-wide platforms has triggered a demand for better privacy safeguards. One approach to privacy is to give citizens control over the exchange of personal data, hoping to reinforce trust in and support for data-driven governance. However, it remains unclear whether more control fulfils its promise of more support and higher trust. Using an online survey experiment, we study how 1) textual information on control and 2) direct control (simulated through an exercise in which respondents choose data types that can be shared) affect citizen trust, support and policy concerns. Results suggest that a combination of information on and direct control result in relatively high levels of trust, support and policy concern. Moreover, we observe an interaction effect in which those respondents with low pre-existing trust in government report more positive attitudes when assigned to the full intervention (information on control + direct control) group. Our results imply that perceived control may be especially useful to mitigate negative attitudes of those who have low trust in government.
1.Introduction
An important challenge in modern digital government is how to efficiently organize data collection and exchange between multiple governments and private actors, while simultaneously balancing citizen trust, transparency and respect for privacy (O’Hara, 2012; Lips et al., 2009). Not only do governments have to balance these (sometimes opposing) requirements, they also have to be able to convince citizens that they have done so sufficiently. This task is compounded by the high technical complexity and opaqueness of many data exchange systems in government (O’Hara, 2012; Janssen & Van Den Hoven, 2015), forcing public actors to be selective in what they communicate to the public (O’Hara, 2012; Kleizen et al., 2022). One solution explored in practice is to offer citizens both increased transparency and control over data (e.g. Olsthoorn, 2022; Van Ooijen & Vrabec, 2019), although it remains unknown how such solutions impact citizen attitudes. This contribution provides a first step in addressing the lacuna on the role that control over data may play by examining data from a recent survey experiment held in Belgium. By showing respondents information on control (text explaining how privacy is maintained) and by providing a sense of direct control over data, we examine how attitudes towards data exchange platforms (and other governmental data systems) are influenced differently by types of information.
In doing so, the study ties in to the recent emergence of human-centric data governance. Transparency in particular has long been seen as a panacea to solve trust issues (Grimmelikhuijsen, 2009; Bannister & Connolly, 2011). Transparency is used to signal the trustworthiness of digital government projects and as a means to allow further scrutiny of policies and activities. Efforts to increase citizen control over their personal data are more recent and are seen as an attempt to restore personal autonomy over data in a world where data-gathering and sharing are becoming ever more ubiquitous, while privacy is becoming increasingly elusive (Malhotra et al., 2004; Brandimarte et al., 2013; Van Ooijen & Vrabec, 2019). Examples span from choosing what types of data you want to share on websites (e.g. flowing from GDPR-based informed consent and the right to erasure in the EU, or similar rights and obligations flowing in the Californian CCPA), to ‘digital wallet’ solutions in which citizens can directly see – and in some cases even control – what actor is using their data in what way (Flanders Investment & Trade, 2022). Although some evidence is available regarding the effects on transparency on citizen attitudes (Grimmelikhuijsen, 2022; Aoki, 2020), we lack evidence on the way that perceived control affects citizen attitudes in data-driven projects in government. As argued by Janssen and Kuk (2016) and Giest (2017), trust among all stakeholders is necessary to appropriately adjust digital government to its quickly changing ecosystems. Our contribution adds insight on trust dynamics among one group of these stakeholders: the general public.
Using a survey experiment, we vary the way in which information on the data categories exchanged is shown to citizens, as well as the degree of control available to citizens (with some groups being able to fill in the types of data they would like to share). The first group receives information on a governmental data exchange project and an exercise which simulates a degree of control over data. The second group receives the same information on the data exchange project, this time supplemented with additional information on the control that citizens would have over their data. The final group combines both textual information and direct control. Through this setup, we examine two research questions. First, how does information on the amount on control that respondents have over their data affect trust in and support for a public sector data exchange platform? Second, how does the direct control that respondents have over their data affect trust in and support for that same public sector data platform?
We expect that respondents receiving both information and direct control will report high perceived trust in project and support for the displayed data exchange project as well as low levels of self-reported policy concerns. Conversely, omitting one of these two treatments should reduce trust in project and policy support, while heightening policy concerns. Results are not entirely in line with hypotheses, however, as 1) the treatment group with both conditions displays high levels of policy concern and 2) under some circumstances the information condition seems to adversely influence attitudes. Finally, we observe an interaction effect in which those respondents with low pre-existing levels of trust in government report lower trust in data exchange project and policy support when either the direct control or the information on control treatment is omitted. This implies that control may work to reassure those citizens with lowered general levels of trust in government under certain circumstances.
2.Theoretical framework
2.1Setting the stage: Digital governance, control over data and transparency
Digitalization challenges typical notions of citizen-state interactions and interactions between public organizations (Busuioc, 2020). Effective data collection, storage and data-sharing processes can improve e-government applications (e.g. prefilled taxes and open data), predict citizen needs (e.g. employment training needs) and improve the efficiency of services (e.g. near real-time updates of dossier statuses). Simultaneously, digitalization in government raises questions on the degree to which public organizations should be entrusted with personal data, the reach of the right to a private life and the safety of data managed in governmental projects (e.g. Janssen & Van den Hoven, 2015).
The ethical concerns related to digitalizing governments have resulted in various approaches to make data collection, data sharing and data analytics processes more ‘human-centric’, i.e. putting users and/or stakeholders first in regulation, policy and project design. Such human-centric approaches frequently place a dual focus on transparency and control. For instance, the GDPR is based on the principle that data subjects must be informed in a clear and understandable way what data is processed for what purposes, before consent is given (Van Ooijen & Vrabec, 2019). Moreover, it codifies the right to erasure and right to access stored personal data, making user control increasingly relevant for both private and public sector entities. The more recent California Consumer Privacy Act (CCPA) provides for a similar consent- and rights-based approach, illustrating the glowing clout of control-based regulatory efforts in recent years. Similarly, soft law approaches such as the EU’s and UN’s trustworthy AI guidelines consistently incorporate autonomy, user control and transparency as core principles, suggesting that control and informed consent are internationally becoming important ethical principles (Floridi, 2019; Kozyreva et al., 2020).
Recent technical developments such as Self-Sovereign Identity (SSI) systems take the idea of building trust through transparency and control even further (Naik & Jenkins, 2020; Flanders Investment & Trade, 2022). Such systems would provide citizens with an online ‘digital wallet’, in which they can see what data is available on them and who may access it. For some data categories, citizens might even exercise some degree of control, determining whether to share such data with certain actors or not (Naik & Jenkins, 2020). SSI projects are still in an embryonic state, their emergence and simultaneous developments in the regulatory and policy arena’s (the aforementioned GDPR and guidelines). Nonetheless, their trust-building aim raises the question how control over data psychologically relates to citizen attitudes towards data-driven government projects. Although a new development, digital wallets are no longer entirely theoretical either, with e.g. a Belgian consortium working on an experimental SSI system with an associated digital wallet (Olsthoorn, 2022).
Taking inspiration from both regulatory efforts and technical developments in e.g. the SSI domain, we examine how information on control and actual control would empirically affect citizen attitudes. In the remainder of this section we will therefore first discuss three citizen attitudes that could be affected by information and control (trust in project, policy support and policy concerns). Subsequently, we discuss how information, transparency and control are causally linked with trust, policy support and policy concerns. Finally, we discuss how control over data may affect trust, policy support and policy concerns.
2.2Trust in project, policy support and policy concerns
Citizens possess multiple analytically distinct attitudes towards governmental projects, including public authorities’ data processes. In this study, we examine three different, albeit related attitudes towards data exchange projects in government: trust in (data exchange) project, policy support and policy concerns. Trust in data exchange project is seen as a manifestation of trust in government where a specific project – instead of the government or a public authority as a while – is the trust referent. In a public sector setting, trust is usually defined as the expectation of a trustor that a government will engage in opportunistic behavior that results in harm to the trustor (Hamm et al., 2019; Grimmelikhuijsen & Knies, 2017). We argue that our notion of trust in project is more aligned with trust in government than with trust in technology or trust in systems (see Lankton & McKnight, 2011 for an overview), as citizens will not just evaluate the reliability and functionality of the data exchange system itself, but also the actions, characteristics and intentions of the civil servants and public authorities developing, managing and using it. In our case such evaluations may e.g. also include follow-up decisions by public authorities based on exchanged data, making the trust referent the broader project, rather than ‘just’ the technological system.
Trust is conceptualized as multidimensional in nature, with social scientists commonly distinguishing between the dimensions ability (a trustee’s competence to act in a way beneficial to the trustor), benevolence (the degree to which the trustee takes the trustor’s best interests to heart) and integrity (the degree to which a trustee follows the rule of the game when interacting with the trustor) (O’Hara, 2012; Degli Esposti et al., 2020; Hamm et al., 2019; Grimmelikhuijsen & Knies, 2017). Trustors by definition operate under incomplete information on the behavior and intentions of a trustee, and therefore have to rely on incompletely informed assessments of a trustee’s trustworthiness (Hamm et al., 2019). Faced with limited but gradually updating information, people may change their beliefs regarding the trustworthiness of a trustee – in this case a data exchange project – based on novel information on any of the three trust dimensions (Degli Esposti et al., 2020).
Although important, trust is not the only relevant citizen attitude. One could for instance trust that a government project has the best intentions and will be performed capably and with integrity, without supporting the content of that project. To gain a more comprehensive view of citizen attitudes, we also examine two slightly different – albeit likely correlated – concepts. Policy support captures the degree to which citizens are supportive of data exchange projects in government (Zahran et al., 2006). Slightly different is the policy concerns concept, which concerns the degree to which respondents have reservations on specific aspects of a policy or public sector project. A significant impact of our treatments on either variable would suggest citizens have updated their cognitive assessment of data exchange projects. The following subsections now turn to linking information on control and the exercise of control to trust in project, policy support and policy concerns.
2.3Information on control over data and its impact on citizen attitudes
Although digital government has often been connected with opportunities for increased transparency and – through such transparency – greater citizen trust and accountability (Matheus & Janssen, 2020; Bertot et al., 2010), substantial challenges remain. Previously, we already noted that information on, and control over the way governments process data is becoming increasingly relevant in practice. However, the question remains how governments should provide information and control to citizens, and what transparency exactly means in a digital governance context. More passive, descriptive definitions of transparency emphasize that a transparent government bestows the ability to see through the windows of an institution (Meijer, 2009). Others see transparency as a principle that reinforces other processes. Building on this perspective, Buijze (2013) argues that transparency holds two important functions. First, it facilitates citizen and stakeholder decision-making. By providing citizens with information on what public authorities are doing, citizens become empowered to determine their own opinions and act accordingly. Second, transparency enables outside scrutiny of public authorities’ behavior. Information provided in line with the second function allows citizens to hold governments to account and serves to protect the rights of citizens (Buijze, 2014; see for a similar perspective, Stirton & Lodge, 2001).
The approach provided by Buijze (2013) is useful, as it also implies that information does not necessarily equate to transparency – and in some cases may even adversely affect it. For information provided by a government to contribute to transparency, it should contribute to citizen will-formation, to the monitoring of government actions or to the protection of citizens’ positions. At the same time, excessive or incomplete information may in some cases adversely affect citizen decision-making or the scrutiny of public authorities (Buijze, 2013). This is highly relevant for a digital governance context. As noted by O’Hara (2012), data processes in governments are regularly so complicated (both in technicality and in scope) that any attempt at being transparent on them entails difficult choices what information should be provided and not. Such choices are presented both in terms of the information that is presented to citizens and in terms of the design of the context in which this information is presented (e.g. the website on which it is placed, or regarding the other information sources available on that same website).
Previous work has observed that this design process may have important – and sometimes unexpected – consequences for citizen attitudes, with different forms of presenting information yielding varying levels of trust and privacy concerns (e.g. Brandimarte et al., 2013; Van Ooijen & Vrabec, 2019; Brandimarte et al., 2013). Van Ooijen & Vrabec (2019) e.g. note that even the mere presence of information such as a privacy policy may suggest to users that data may not be shared by the processor, even though the privacy policy in question does not strictly speaking incorporate provisions to that end. Such results suggest that the signals provided by information on websites an important determinant of the concerns and trust that people have regarding data processes (Berliner Senderey et al., 2020), and that some information may even allow for a degree of manipulation of citizen perceptions (Brandimarte et al., 2013; Alon-Barkat, 2020). Moreover, they imply that website design may even provide room for manipulation by the creators of websites. More optimistic on the empowering and trust-building effects of information is the broader public administration literature on transparency and data processes. Matheus and Janssen (2020) note in their recent review that Open Government Data (OGD) is expected by a majority of contributions to have positive effects on citizen trust. Moreover, research on how users respond to the use of AI in government has suggested that explainable models, i.e. systems providing information on how predictions are generated, are generally perceived more favorably by users than non-explainable counterparts (Grimmelikhuijsen, 2022), suggesting ‘algorithmic transparency’ on how data processes work may have positive effects. Aoki (2020) similarly demonstrates that communicating legitimate purposes of chatbots used in governments may enhance public trust. Notable is that such studies focus more on the content of what is communicated, whereas studies such as Brandimarte et al. (2013) and Van Ooijen and Vrabec (2019) focus more on potentially misleading ways information is presented. This again reinforces the idea that transparency and information do not equate to one another, and that in some cases information may even adversely affect transparency (Buijze, 2013). Nonetheless, in both cases, studies seem to show that positive information (whether genuinely transparent or incomplete or misleading) allowed entities to improve attitudes such as trust in and support for their data processes.
In sum, both the literature on privacy options in websites and the public administration literature on transparency in data processes seems to suggest that providing explicit information on the control that citizens would have over their data would have positive effects on attitudes such as trust and support – even if the underlying intentions sometimes seem to differ. We will integrate these insights into our experiment by providing additional information on the control that citizens will have over their data in the context of a novel data exchange platform, and expect that more information on control should result in greater trust and policy support, while reducing citizen concerns on data exchange projects.
H1a: Being presented with information on control over data processing will increase respondents’ trust in project;
H1b: Being presented with information on control over data processing will increase respondents’ policy support;
H1c: Being presented with information on control over data processing will reduce respondents’ policy concerns.
2.4The impact of exercising control on citizen attitudes
Beyond studies on the effects of information and transparency, the impact on control over data has also increasingly received scholarly attention. Prior studies have generally shown that increases in (perceived) control positively affect citizen attitudes. Contributions in this line argue that data collection procedures are often opaque in nature (Malhotra et al., 2004), requiring citizens to place a certain amount of trust in the data processor that data will not be misused. Such misuse might come in various forms, such as the use of data for purposes that are not considered desirable or the recombination of data against the wishes of the person providing the data (Whitley, 2009; Bilogrevic & Ortlieb, 2016; Malhotra et al., 2004). What is more, considering that data is often stored long-term, this brings about further unease on data storage terms and the potential for future misuse (Van Ooijen & Vrabec, 2019). Essential here is that attitudes and behavior will flow from perceived misuse, which does not necessarily align with what is legally possible with the data. For instance, long-term storage may be perfectly legal under some privacy policies agreed to by someone, but may – due to bounded rationality and incomplete information – still be perceived as misuse.
Such fears of misuse may be ameliorated by granting citizens some measure of (perceived) control. Here, it is important to note that control and perceived control are subtly but importantly different. In the context of Facebook, Brandimarte (2013) for instance shows that awareness of control mechanisms to limit whether media can be shared with friends and/or strangers gives respondents a higher level of perceived control, even though actual control over the degree to which personal information is sold to advertisers stays limited. Perceived control may function to reduce uncertainty and unease regarding the potential consequences of sharing data, as the person involved now feels directly empowered to generate a desirable outcome (Whitley, 2009; Van Dyke et al., 2007). Therefore, being provided with a measure of actual control by the data processor may – through improved perceived control – function as a sign of trustworthiness and procedural justice, heightening the degree to which the person involved experiences is willing to provide data to the party in question (Malhotra et al., 2004). Moreover, the role of (perceived) control will likely become stronger as the (perceived) opportunity for abuse becomes higher (Malhotra et al., 2004). Public sector organizations tend to have greater access to personal data than their private counterparts, as well as incentive to share sensitive categories of information (such as data on financial position, criminal convictions, migration status, or previous benefits received). As such, adjusting levels of control over data collection may be a particularly successful predictor of trust in project, policy support and concerns in the context of public sector data projects.
Being granted immediate control over the data categories shared may trigger a process in which respondents cognitively focus on the trustworthiness of the interface directly presented to them – potentially to the detriment of other facets of a governmental projects’ trustworthiness (Brandimarte et al., 2013). Brandimarte et al. (2013) argue that this may even lead to unintended oversharing of data, as limited cognitive capacity (bounded rationality) leads humans to focus on the immediate control they have over data at the expense of what may happen to that data in the future (e.g. during the processing and analysis stages after data is exchanged between governments), leading to reduced perceived risk that data will be abused. This should be visible in our results, as the reduced levels of perceived risk associated with the presented data exchange platform may in turn illicit heightened trust in project and policy support (Brandimarte et al., 2013; Alon-Barkat, 2020), while reducing respondents’ policy concerns.
In sum, we expect that increased control will increase trust and support in data exchange platforms, while reducing policy concerns regarding such platforms.
H2a: Being granted direct control over data processing will increase respondents’ trust in project; H2b: Being granted direct control over data processing will increase respondents’ policy support; H2c: Being granted direct control over data processing will reduce respondents’ policy concerns.
3.Data
3.1Experimental design and treatments
The experiment relies on a group structure in which groups differ on one factor. Three groups are given a short common baseline to which three different additions are made. The common baseline shows information on a hypothetical data exchange project, inspired on existing systems in Belgian governments. More specifically, Belgian governments host platforms such as MAGDA (Flemish regional government (European Commission, n.a.)) and the Crossroads banks (federal government), to which public entities can connect to request data that is stored in a decentralized fashion across multiple (mostly public, but sometimes private) entities (CBSS, n.a.). The commonality between these systems is that they seek to provide efficient, safe and legally compliant platforms for data exchange between public (and sometimes private) organizations. At the same time, many of the data sources that can be connected concern personal and/or sensitive data categories, which may raise concerns among some citizens. This makes data exchange platforms an interesting (and underexamined) context to examine how variations in transparency and control over data may affect attitudes.
The experiment introduces two treatments. Two groups are provided with one treatment, while a third receives a combination of both treatments. The first treatment consists of an exercise where respondents can indicate what data categories they would like to share with various private and public parties. This exercise serves as a direct signal to citizens that they will be able to exercise some degree of control over their data (Brandimarte et al., 2013). The 8 listed data categories were chosen to correspond to both the data categories registered with the Belgian Crossroads bank for social affairs and the data categories that could be shared between governments under the Dutch System Risk Indication (SyRI). This prevents the intervention from being relevant to only one setting. Data categories include identification data, labour data (including career and wage), welfare data (e.g. benefits received), data on prior interactions with the criminal justice system, real estate data, education status and registrations of vehicles. One category incorporated in SyRI but excluded here is migration, as this category may not be relevant for various respondents. In the second treatment, information on a hypothetical governmental project that would improve citizens’ control over their data is provided. The treatment is provided through a brief paragraph of textual information, and is inspired on advances in digital wallet and SSI systems (such as the Belgian SOLID project (Olsthoorn, 2022)), through which citizens would be able to exercise some measure of control over what data is shared with what actor (Naik & Jenkins, 2020).
Table 1
Schematic overview of group structure and vignette components
Components of vignette | Group 1 – full treatment | Group 2 – information on control | Group 3 – direct control |
---|---|---|---|
Baseline | Yes | Yes | Yes |
Information on project improving control | Yes | Yes | No |
Exercise filling in data versus simple list of data categories | Exercise | List | Exercise |
Figure 1.
Screen 1, showing baseline and (for full intervention group and information on control group) the information on control intervention.
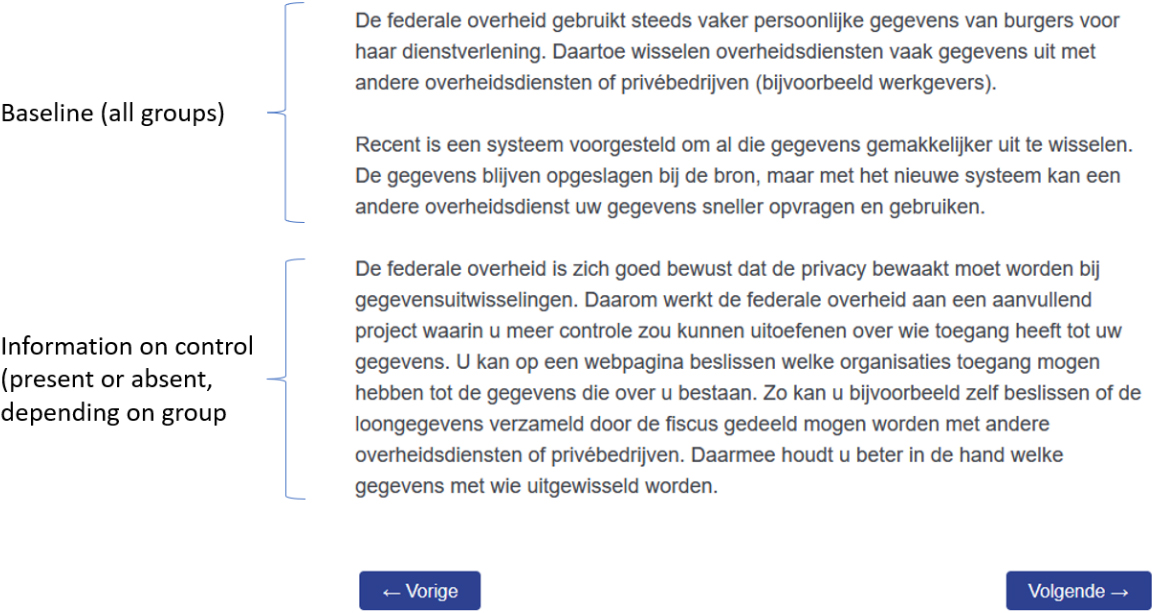
Figure 2.
Screen 2, version 1, showing a list of data types shared between governments to respondents that do not receive the direct control treatment.
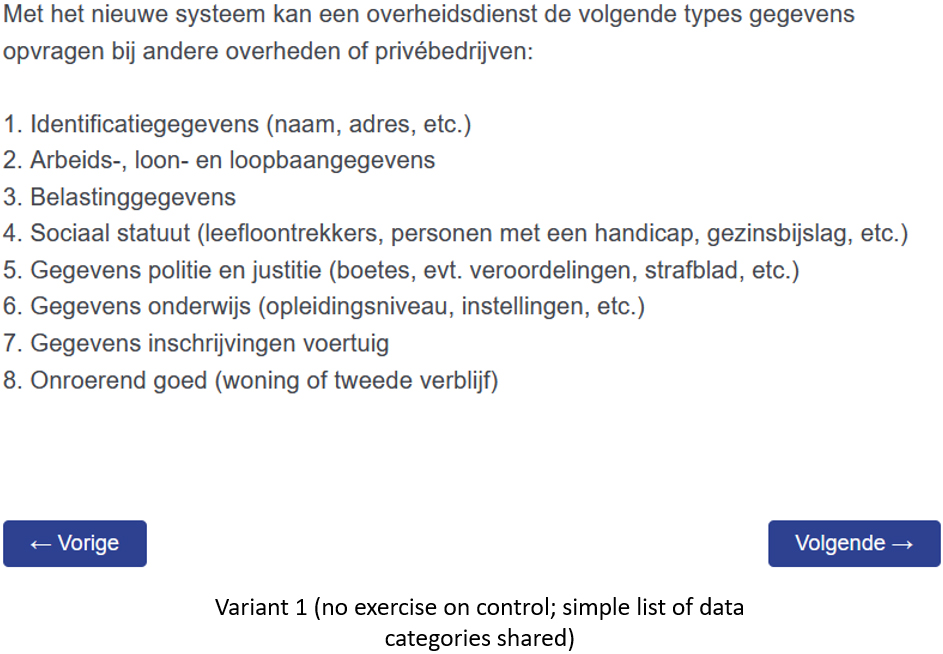
The group receiving the full treatment receives both the exercise on direct control and the textual information on a new project to improve citizen control. This group thus receives the strongest intervention. The group receiving the full intervention is designated as the reference category in regression analysis, as the other two groups each differ on one component with the group receiving the full treatment. Figures 1–3 show the original Dutch screens incorporating the experimental treatments, with Fig. 1 containing the baseline and information on control intervention, while Figs 2 and 3, respectively contain the screen shown to the group of respondents not receiving the direct control and the screen shown to respondents in the group that do receive the direct control. Translated vignettes are available in Appendix 1. A schematic overview of the information available to respondents in the three groups is available in Table 1.
Figure 3.
Screen 2, version 2, showing the direct control treatment (the same list of data types as version 1, but now with the possibility to indicate with whom a respondent would like to share particular data types).
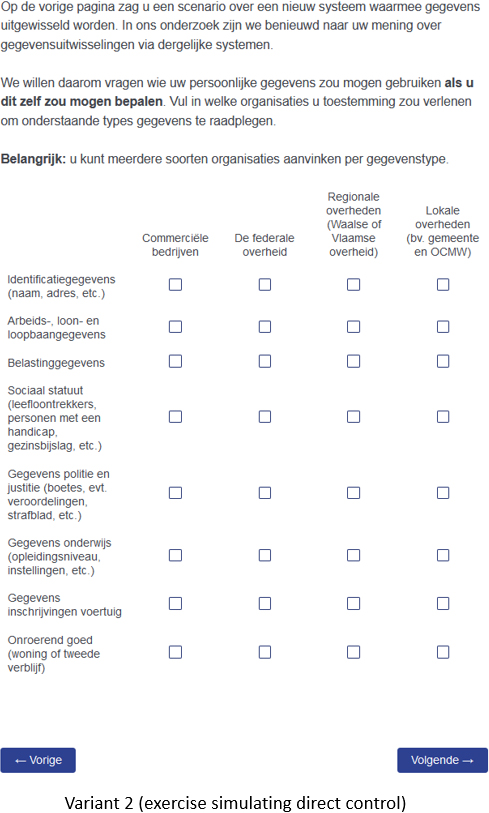
Respondents are randomly assigned to groups, using simple randomization and Qualtrics software to automatize the process, ensuring that unobserved characteristics are distributed randomly across observations. Groups are equal in size (
3.2Sample size, recruitment and pretesting
The survey containing the experiment was held through a marketing company’s panel of Flemish (Dutch-speaking region of Belgium), adult participants. The panel approaches its members until the intended amount of respondents is reached. Although recruitment into the panel is done through self-selection, selection of potential recruits is done so that a representative sample of the Flemish population (based on age and gender and within a weighting factor of 3) is reached. Panel members receive payment through points per survey completed, which they can subsequently spend on offers made by the marketing company. [Anonymized university] is not involved in this payment scheme. Final sample size slightly exceeded our aims, consisting of 402 observations. After random assignment, the full treatment group has 133 observations, the information on control group has 134 observations and the direct control group has 135 observations. This exceeds the aim of our power analyses, which suggested we need just over 100 participants (see Appendix 2).
We held pretest using the cognitive interview (read-out-loud) protocol with 5 respondents. This protocol requires respondents to read out loud every component of the survey and mention any comments they have, for instance on sentence structure, complexity, jargon or issues with the interpretation of items. The benefit of this approach is that a great amount of insight is created on why certain items fail to produce the desired associations among respondents. Subsequently, a soft launch was held with the survey company to test for issues that result in heightened drop-out rates, which revealed no further issues.
3.3Measurements
3.3.1Dependent variables
Three dependent variables are incorporated in this study: trust in project, policy support and policy concerns. Trust in project is measured using a three-item scale based on Grimmelikhuijsen and Knies (2017). The scale uses a sub-distinction between the perceived ability, benevolence and integrity of the trustee. Policy support is measured using a single, newly developed item, measuring the degree to which the respondent believes that the new system will improve services provided by the federal government. Policy concerns are measured using four newly developed items, one measuring expected consequences of governmental data projects, the second measuring concern on data exchanges between governments, the third measuring concern on public-private data exchanges and the fourth measuring concerns that data gathered now will be abused in the future. We opted for newly developed items due to the data exchange project context, which raises several unique issues for which no validated scales were available. Although partially inspired on the control dimension of privacy concerns (e.g. Malhotra et al., 2004), these items are geared more towards general policy concerns of citizens over data exchange platforms. They measure the unease of respondents regarding data exchange platforms generally, respondent concerns on data exchanges between various actors, and unease regarding the long-term storage of (citizen) data. These dependent variables were measured using a 0–100 slider, so as to mitigate common method bias issues with Likert scale items measured before respondents received the experimental treatments. Indices for scales with multiple items are computed using EFA-based factor loadings, using regression scoring (resulting in a standardized distribution (mean 0, standard deviation 1).
Survey items are available in the preregistration: https://osf.io/uycw8/?view_only=85e06856593a48958 654a08c960bcee7.
3.3.2Demographic characteristics and pre-existing attitudes
In addition to the experimental variables, a number of demographic characteristics and attitudinal scales were asked before respondents received the treatments that allowed for exploratory analyses. We incorporate trust in government, measured through a 9-item Likert scale adapted from Hamm et al. (2019). It is notable that we here see trust in government as a pre-existing attitude towards the federal Belgian government as a whole, while our dependent variable trust in project defines a specific data exchange project as the trustee. We also measure privacy concerns, as the desire for control over personal data is intrinsically tied to the desire to maintain privacy (Malhotra et al., 2004). We incorporate a three-item scale measuring pre-existing privacy concerns before respondents enter the experimental phase of the survey. Each item corresponds to one of the dimensions discerned by Malhotra et al. (2004), i.e. concerns on data collection, desire for control over data and awareness of privacy practices. Finally, we incorporate a three-item scale of respondents’ computer self-efficacy as a potential moderator, reasoning that understanding of data tools and data exchanges may alter the perceived threats and benefits of the data exchange systems studied in this experiment (items adapted in part from Murphy et al., 1989; Howard, 2014; Bilici et al., 2013). All indices are again based on EFA-based factor loadings. Regarding demographics, we incorporate education, age, gender and public and/or private sector employment. Controlling for such characteristics is necessary to counter potential model misspecification for attitudes measured before respondents were randomly assigned to groups.
Descriptive statistics on all variables are provided in Table 2.
4.Results
Although the results partially support our initial hypotheses that information and control should increase trust in project and policy support while reducing policy concerns, they also show several unexpected patterns. In regressions only incorporating the experimental variables (see Table 3, columns 1, 4 and 7 as well as Fig. 4), both groups that received the exercise treatment reported higher levels of trust in project and policy support than the group that solely relied on an information-based treatment, in line with hypotheses 2a and 2b. In fact, the group only receiving information on control treatment seemed exhibited notably low levels trust in project and policy support. We reason that the low level of trust and support in the group receiving only information on control and omitting the direct control exercise may be due to the perceived threat of the project as presented to them. Although these respondents did receive positive signals on a project that would enhance control, they were not provided with any simulation of such control. Instead, they saw a simple list of data categories that could be shared between governments or even obtained from the private sector. Considering that this list also contains sensitive data categories such as past interactions with criminal justice, labor data (e.g. pensions) and data on real-estate, the intervention may have been perceived as threatening privacy and/or as overreach by governments. This
Table 2
Descriptive statistics and Pearson correlation coefficients
Variable | Descriptive statistics | Correlations | |||||||||||||||
---|---|---|---|---|---|---|---|---|---|---|---|---|---|---|---|---|---|
Obs. | Mean | Std. Dev. | Min. | Max | (1) | (2) | (3) | (4) | (5) | (6) | (7) | (8) | (9) | (10) | (11) | (12) | |
Trust in data exchange project (1) | 395 | 0,951 | 2,031 | 1 | |||||||||||||
Policy support (2) | 402 | 63,617 | 20,141 | 0 | 100 | 0,629 | 1 | ||||||||||
Policy concerns (3) | 395 | 2,74e-11 | 0,892 | 1,706 | 1 | ||||||||||||
Trust in government (pre-existing) (4) | 401 | 0,904 | 2,749 | 0,453 | 0,348 | 1 | |||||||||||
Privacy concerns (5) | 402 | 0,001 | 0,899 | 1,158 | 0,510 | 1 | |||||||||||
Generalized trust (6) | 402 | 3,767 | 1,462 | 1 | 7 | 0,332 | 0,210 | 0,300 | 1 | ||||||||
Computer self-efficacy (cse) (7) | 402 | 0,006 | 0,890 | 1,350 | 0,047 | 0,120 | 0,125 | 0,026 | 0,153 | 1 | |||||||
Age (8) | 402 | 3,567 | 1,728 | 1 | 7 | 0,074 | 0,077 | 0,025 | 0,062 | 0,094 | 1 | ||||||
Education (9) | 402 | 4,269 | 1,290 | 1 | 7 | 0,077 | 0,083 | 0,116 | 0,025 | 0,076 | 0,170 | 1 | |||||
Gender (10) | 401 | 1,549 | 0,498 | 1 | 2 | 0,140 | 0,063 | 0,013 | 1 | ||||||||
Employed in the public sector (11) | 402 | 0,236 | 0,425 | 0 | 1 | 0,009 | 0,031 | 0,015 | 0,096 | 0,063 | 1 | ||||||
Employed in the private sector (12) | 402 | 0,241 | 0,428 | 0 | 1 | 0,035 | 0,015 | 0,036 | 0,260 | 0,140 | 1 |
illustrates that simply being transparent does not automatically result in increased trust in project and policy support among citizens, with some types of information potentially even negatively affecting citizen attitudes (despite the positive information on a project that would enhance citizen control).
Figure 4.
Treatment effects on trust in project, policy support and policy concerns without control variables.
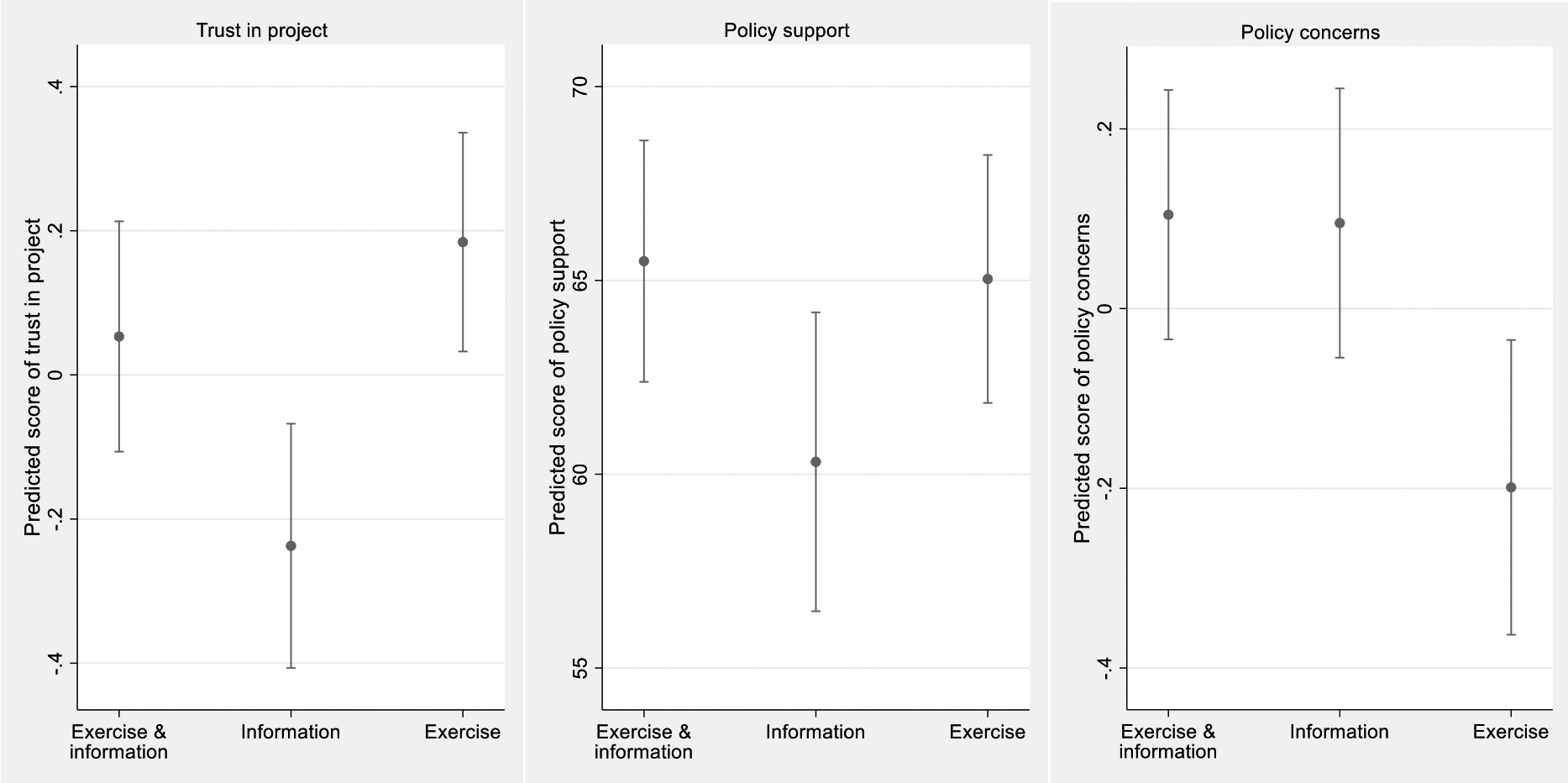
At the same time, the groups respectively receiving only the exercise and receiving the full treatment (both direct control and information on control) exhibited no significant difference between one another, which casts some doubt on the potential positive effects of information on control for trust and policy support (hypotheses 1a and 1b).
The pattern for policy concerns is surprising: although we expected our interventions to reduce concerns (hypotheses 1c and 2c), the full intervention group instead reports the highest levels of policy concerns. Interestingly, this makes the full intervention group the set of respondents with the highest levels of support, single highest levels of trust in project, while also reporting the highest amount of policy concerns. This suggests that combining both interventions does not simply introduce additional degrees of policy support and trust, but may also spur critical attitudes among citizens. From the perspective that effective democratic scrutiny requires transparent information-provision to create a well-informed and critical citizenry (Stirton & Lodge, 2001; Buijze, 2013), this is a highly relevant result. Instead of dismissing the degree of concerns in this group as a normatively negative outcome, we may see increased concerns on data projects in governments as a desirable attitude that stimulates accountability to the public. This will be explored further in the discussion.
Columns 2, 5 and 8 of Table 3, as well as Fig. 5, display the results of exploratory regressions with control variables, which add further nuance. Despite random assignment across groups, the analyses displayed in columns 2 and 5 yield markedly different coefficients for the experimental variables, with some group differences no longer being significant. This suggests that some control variables may have had a moderating effect. To test for the moderation effects, we conducted several exploratory analyses incorporating interaction terms, which indicate that trust in government in particular functions as a
Table 3
OLS regression results for dependents trust in data exchange project, policy support and policy concerns (robust standard errors in parentheses)
Trust in data exchange project | Trust in data exchange project | Trust in data exchange project | Policy support | Policy support | Policy support | Policy concerns | Policy concerns | Policy concerns | |
b/se | b/se | b/se | b/se | b/se | b/se | b/se | b/se | b/se | |
Experimental treatments (full treatment group is reference) | |||||||||
Information | |||||||||
(information on control) | (0.12) | (0.10) | (0.10) | (2.52) | (2.38) | (2.33) | (0.10) | (0.08) | (0.09) |
Exercise (direct | 0.131 | 0.084 | 0.058 | ||||||
control) | (0.11) | (0.10) | (0.10) | (2.27) | (2.19) | (2.24) | (0.11) | (0.09) | (0.09) |
Information*trust in | 0.438 | 8.227 | |||||||
government (general) | (0.12) | (3.11) | (0.10) | ||||||
Exercise*trust in | 0.371 | 7.969 | |||||||
government (general) | (0.11) | (2.64) | (0.10) | ||||||
AGE | 0.020 | 0.018 | 0.947 | 0.875 | |||||
(0.03) | (0.03) | (0.70) | (0.71) | (0.03) | (0.03) | ||||
Education | 0.026 | 0.028 | 0.593 | 0.668 | |||||
(0.03) | (0.03) | (0.67) | (0.67) | (0.03) | (0.03) | ||||
Gender | 0.240 | 0.246 | |||||||
(0.08) | (0.08) | (1.92) | (1.89) | (0.07) | (0.08) | ||||
Public sector | |||||||||
(0.11) | (0.10) | (2.52) | (2.45) | (0.11) | (0.11) | ||||
Private sector | 1.023 | 1.393 | |||||||
(0.10) | (0.10) | (2.41) | (2.42) | (0.10) | (0.10) | ||||
Generalized trust | 0.123 | 0.128 | 1.160* | 1.278* | |||||
(0.03) | (0.03) | (0.69) | (0.68) | (0.03) | (0.03) | ||||
Trust in government | 0.401 | 0.164* | 6.541 | 1.875 | |||||
(pre-existing) | (0.06) | (0.09) | (1.26) | (1.77) | (0.04) | (0.06) | |||
Privacy concerns | 0.491 | 0.489 | |||||||
(0.05) | (0.05) | (1.11) | (1.08) | (0.04) | (0.04) | ||||
Computer self-efficacy | 1.584 | 1.854 | |||||||
(0.05) | (0.05) | (1.31) | (1.29) | (0.05) | (0.05) | ||||
Constant | 0.053 | 65.496 | 60.650 | 61.133 | 0.105 | 0.415 | 0.409 | ||
(0.08) | (0.28) | (0.27) | (1.58) | (6.15) | (6.16) | (0.07) | (0.26) | (0.26) | |
Observations | 395 | 393 | 393 | 402 | 400 | 400 | 395 | 393 | 393 |
F-test | 6.795 | 16.996 | 16.681 | 2.409 | 4.916 | 6.010 | 4.646 | 22.25969 | 19.195 |
R | 0.0345 | 0.347 | 0.384 | 0.014 | 0.180 | 0.2110836 | 0.025 | 0.386 | 0.391 |
Adjusted R | 0.0295 | 0.328 | 0.362 | 0.009 | 0.157 | 0.1845138 | 0.0200745 | 0.368 | 0.370 |
moderator.11 Columns 3 and 6 illustrate that omitting a treatment (either the information on control or the direct control treatment) has a different effect on trust in policy and policy support depending on respondents’ pre-existing levels of trust in government (important note: trust in government concerns general levels of trust in the federal Belgian government measured before respondents were randomly assigned to groups, trust in project concerns their trust in the project presented in the vignettes as was measured after random assignment).
Figure 5.
Treatment effects on trust in project, policy support and policy concerns when including control variables.
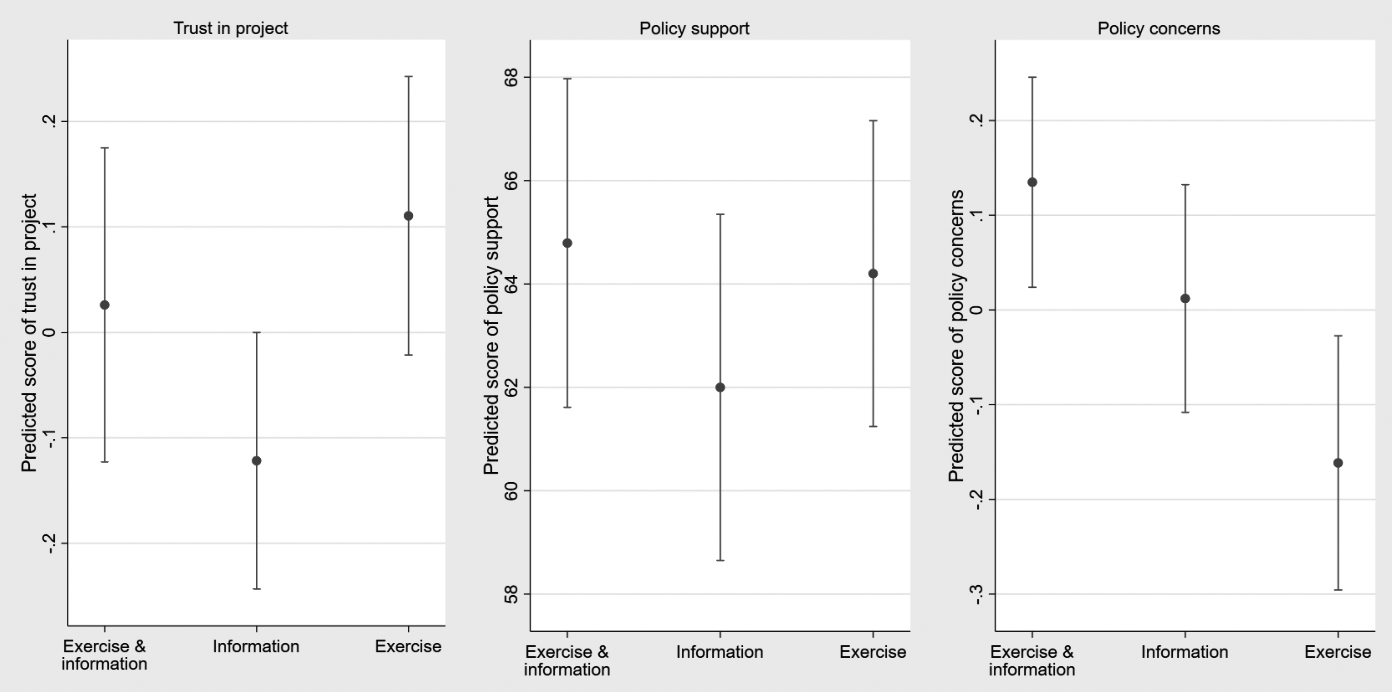
Figure 6.
Interaction effect between trust in government and treatments (dependent variable is trust in project).
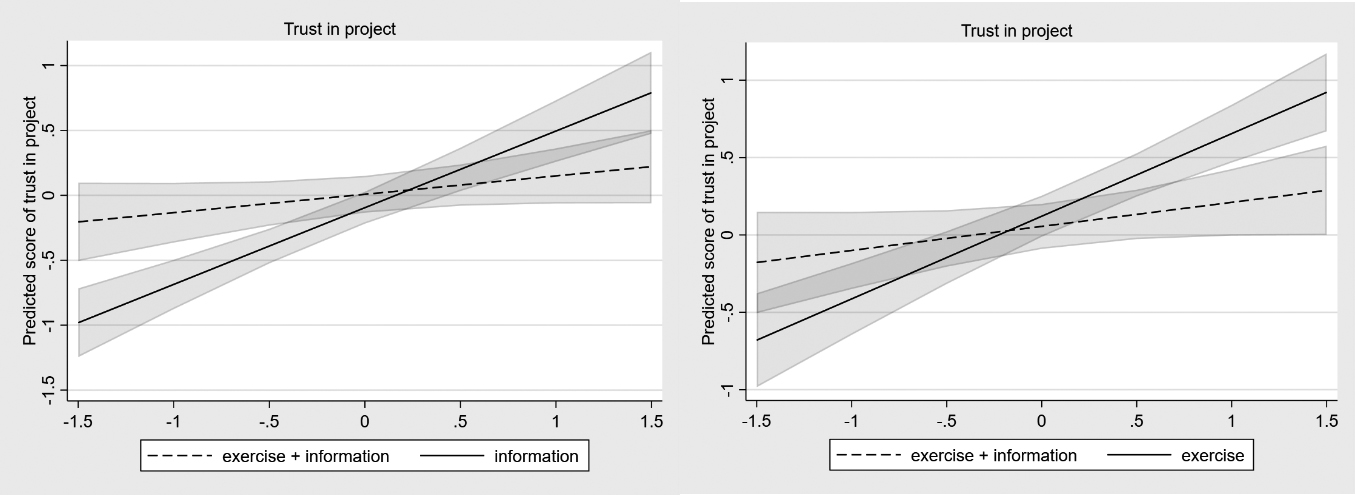
Figure 7.
Interaction effect between trust in government and treatments (dependent variable is policy support).
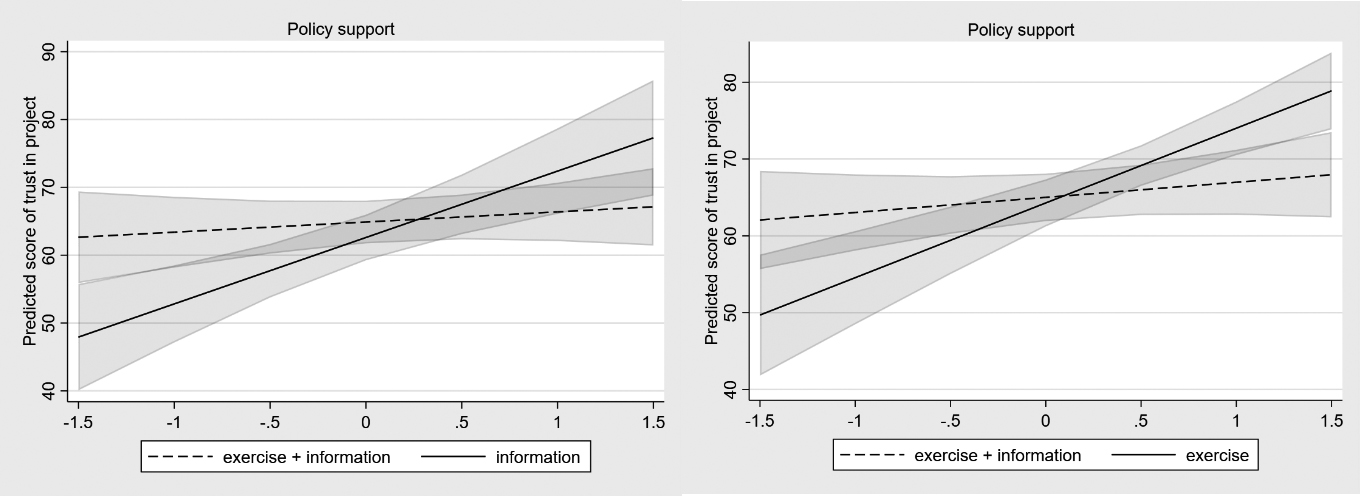
Figures 6 and 7 visualizes how this effect changes. Respondents receiving the full treatment maintain relatively stable levels of predicted trust in project at all levels of pre-existing trust in government. Groups receiving only one out of two treatments display a steeper slope. In the groups omitting one treatment, respondents with low levels of pre-existing trust in government report significantly lower levels of trust in project and policy support than the group receiving the full treatment. At the same time, respondents with higher levels of pre-existing trust in government display higher levels of trust in project and policy support. This interaction effect does not seem to exist when examining policy concerns as the dependent variable.
The significant interactions in the trust in project and policy support models suggest that the two treatments are interpreted differently based on levels of pre-existing trust in government. Trust in government is formed by assessments whether a government is likely to act with high levels of ability, integrity and benevolence (Hamm et al., 2019). Respondents with low pre-existing levels of trust in government that were assigned to the group that receives information on (but no direct) control may believe that projects that enhance citizen control over data are disingenuous or will never come to fruition. As stated earlier, they may also interpret the list of data categories as threatening, with the combination of these effects resulting in lowered trust in project and policy support. Respondents receiving actual control but not receiving the textual information treatment lack knowledge on the positive aims of data exchange projects. This may have caused them to resort to their general perceptions on the trustworthiness of governments as a heuristic. Conversely, in the full intervention group, the combination of information on control and direct control may have increased the knowledge of respondents on governmental intentions, while also taking away the sense of threat produced by the long list of data categories.
In addition to the experimental results, it is relevant to briefly touch on the effects of the control variables. Privacy concerns exhibit a large effect on trust in project, policy support and policy concerns. Generalized trust in others is a significant predictor of trust in project and policy concerns, likely reflecting a generally more critical attitude towards the motivations of others – including governments developing data projects. Gender seems to affect attitudes towards data projects as well, with women generally being somewhat less trusting and supporting and somewhat more concerned. Somewhat surprisingly, we see no effect for computer self-efficacy.
5.Discussion and conclusion
Our results provide initial evidence on the role that control over data may play in governments’ toolbox to maintain trust in and support for major data exchange projects. While we expected a relatively simple relationship in which the introduction of information on control and/or direct control would increase trust in project and policy support while reducing policy concerns, our results suggest greater complexity. It seems that providing both information and direct control did positively influence trust and policy support as compared to only introducing presenting information on control. However, this relationship was itself moderated by pre-existing levels of trust in government. Citizens exhibiting lower trust in government report more positive attitudes in the treatment groups providing them with direct control than in the group where they only received information. Similarly, citizens with low pre-existing trust in government in the full treatment group reported higher levels of support for and trust in the proposed data exchange project than similar citizens in the group only providing direct control. As such, our results challenge the notion that transparently communicating how data processes work will always enhance trust and support (e.g. Bertot et al., 2010; Jobin et al., 2019, although for a nuanced approach, see O’Hara, 2012). Instead, when governments list data categories processed by them without providing control over these categories, they may inadvertently reduce trust in project, as citizens may feel the assurances are disingenuous and/or perceive the list of data categories that can be processed as threatening (Lips et al., 2009). Adding some form of control where possible may allow governments to be transparent while maintaining reasonable degrees of trust in project and policy support.
At the same time, we also observe that the full treatment group exhibits the highest average level of policy concerns, paradoxically combining this with the highest level of policy support and the single-highest level of trust in project. We speculate that this surprising combination may be explained as heightened levels of empowerment in this group, with direct control, information explaining said control and baseline information combining to inform respondents while maintaining their trust and support. The increased cognitive processing of information induced by the treatments may have aided in raising potential concerns with the systems proposed in our vignettes, while information on control and direct control may have simultaneously signaled to citizens that governments’ intentions were benign. As previously mentioned, this suggests that actual control may enhance perceived control (Brandimarte et al., 2013), while transparent information-provision allows for the formation of critical attitudes essential to democratic scrutiny of public policies (Buijze, 2013).
Less optimistic is that the group excluding the textual information on control achieved similar levels of trust in project and policy support as the full treatment group, while the vignettes here were less clear on the control governments actually desired to give citizens. The group not receiving the information on control also reported reduced levels of policy concerns, indicating they were less likely to critically scrutinize the data exchange system. Similar to Alon-Barkat (2020) and Brandimarte et al. (2013), this suggests that providing certain information may elicit some degree of undue trust. It is possible that the exercise present in this group may have given respondents the idea that they already had some degree of immediate control, leading to reduced reasoning on the actual consequences that allowing data-sharing may have (Brandimarte et al., 2013). Whether this is due to bounded rationality (Brandimarte et al., 2013) or due to their attention being drawn to the exercise (Alon-Barkat, 2020) is an interesting question for follow-up research.
Although this contribution only provides tentative insights until it is replicated and extended, we offer some preliminary implications for practice. Supplementing transparency with increased control, while challenging to incorporate in some contexts, may offer benefits in terms of citizen-government relations. Earlier contributions have identified that major data projects in government are sometimes controversial topics within society (Busuioc, 2021; Kleizen, 2022). Large-scale data processing, storage and exchange raises potential tensions with the right to privacy from both legal and ethical perspectives. Such initiatives may even raising fears of a surveillance state creeping into every facet of public and private life (Lips et al., 2009). Providing citizens with some control, whether through submitting preferences when interacting with online systems (Brandimarte et al., 2013) or through the digital wallet systems now in development (Naik & Jenkins, 2020), may contribute to achieving both legal compliance and addressing ethical concerns (Van Ooijen & Vrabec, 2019; Floridi, 2020). Moreover, such control may mitigate citizen concerns in groups that are usually particularly critical of data-driven policy making, making this an important finding for the literature on big and open data in government (see e.g. Janssen & Kuk, 2016). What is more, providing control may signal some degree of trustworthiness on part of a government, allowing governments to be more transparent without incurring trust breaches through data projects that may potentially be seen as threatening by citizens (e.g. major data exchange platforms through which the sharing of sensitive data categories is possible). At the same time, governments must tread carefully, as altering levels of – and information on – control citizens have may alter the ability of citizens to scrutinize public authorities in both positive and negative ways (Brandimarte et al., 2013; Alon-Barkat, 2020).
Choices on control and information provided to citizens also include important normative components. As with Brandimarte et al. (2013), it seemed that providing little contextual information on what guarantees citizens have regarding their data use yielded high levels of trust and support and low levels of policy concerns. If replicable, this might be considered a tempting option for practitioners when purely viewed from the angle of maximizing citizen trust and minimizing legitimacy risks. However, where (personal) data on citizens is still shared, stored and used by governments for various purposes without citizens necessarily knowing that this is happening, this may be at odds with moral (and sometimes legal) obligations of governments to be transparent and open to scrutiny (Lips et al., 2009; Buijze, 2013). In the words of Alon Barkat (2020), governments may be eliciting undue trust.
Under such circumstances, the short-term benefits of limiting control and transparency could reduce legitimacy in the long term, as civil society gradually begins to scrutinize questionable data exchange practices (Kleizen, 2022). Such developments are no longer theoretical, with the recent Dutch SyRI court case e.g. describing a dispute on a data exchange platform designed to combat welfare fraud. Here, the government wanted to curb transparency, as fraudsters might ‘game the system’ if they knew how it worked. The court was unsympathetic to this argument and replied that such strategies may eventually generate a societal chilling effect (Meuwese, 2020). Our contribution suggests that – where possible – complex data exchange projects could benefit from an exploration on the degree of control that can be granted to citizens over their data.
While interesting, our results must be seen as tentative. Further research and replication are necessary to fully chart the relationship between factors such as control, transparency and trust in a public sector data-processing setting. In that context, it is useful to point out a number of limitations. First, due to ethical limitations, it was necessary to indicate in the exercise treatment that respondents were not truly indicating which types of data they would share with governments. This was done by indicating that we as researchers are interested in what types of data citizens would share, if it were up to them. Although necessary as online respondents may 1) not fully read the initial information sheet provided and 2) exit surveys before receiving the debriefing at the end of the survey flow, such choices may influence results. Second, future experiments could benefit from a structure with a more elaborate baseline that would also allow for the removal of both treatments simultaneously, which was not possible here due to sample size reasons (even though all groups still differed on only one factor, allowing us to isolate their effect). Third, future studies should also address issues regarding the amount and scope of control that citizens should have over data in a governmental setting. Some forms of data simply need to be processed by governments to provide services (e.g. in a tax context). As such, providing control over webpage cookie settings may be easier than providing control over data in the context of necessary services provided by public authorities. In the latter case, citizen responses may be more akin to our information on control treatment, causing a drop in trust and support instead of the desired increase. The relationship between transparency, information, control and citizen attitudes may therefore be more complex than is often believed.
Notes
1 Analyses incorporating interactions between the experimental variables and trust in government are therefore reported in columns 3, 6 and 9, with other analyses (yielding no evidence of moderation or mediation) being available on request.
Funding
This work was supported by the Belgian Science Policy Office (Belspo) and the BRAIN-Be framework programme B2/191/P3/DIGI4FED.
References
[1] | Acquisti, A. (2004, May). Privacy in electronic commerce and the economics of immediate gratification. In Proceedings of the 5th ACM conference on Electronic commerce (pp. 21-29). |
[2] | Alon-Barkat, S. ((2020) ). Can government public communications elicit unduetrust? Exploring the interaction between symbols and substantive information in communications. Journal of Public Administration Research and Theory, 30: (1), 77-95. |
[3] | Aoki, N. ((2020) ). An experimental study of public trust in AI chatbots in the public sector. Government Information Quarterly, 37: (4), 101490-101490. |
[4] | Bannister, F., & Connolly, R. ((2011) ). The trouble with transparency: acritical review of openness in e-government. Policy & Internet, 3: (1), 1-30. |
[5] | Berliner Senderey, A., Kornitzer, T., Lawrence, G., Zysman, H., Hallak, Y., Ariely, D., & Balicer, R. ((2020) ). It’s how you say it: Systematic A/Btesting of digital messaging cut hospital no-show rates. PloS One, 15: (6), e0234817. |
[6] | Bertot, J. C., Jaeger, P. T., & Grimes, J. M. ((2010) ). Using ICTs to create a culture of transparency: E-government and social media as openness and anti-corruption tools for societies. Government Information Quarterly, 27: (3), 264-271. |
[7] | Buijze, A. ((2013) ). The six faces of transparency. Utrecht Law Review, 9: (3), 3-25. |
[8] | Bilogrevic, I., & Ortlieb, M. ((2016) , May). “If You Put All The Pieces Together…” Attitudes Towards Data Combination and Sharing Across Services and Companies. In Proceedings of the 2016 CHI Conference on Human Factors in Computing Systems (pp. 5215-5227). |
[9] | Bitektine, A. ((2011) ). Toward a theory of social judgments of organizations: The case of legitimacy, reputation, and status. Academy of Management Review, 36: (1), 151-179. |
[10] | Brandimarte, L., Acquisti, A., & Loewenstein, G. ((2013) ). Misplaced confidences: Privacy and the control paradox. Social Psychological and Personality Science, 4: (3), 340-347. |
[11] | Bilici, S. C., Yamak, H., Kavak, N., & Guzey, S. S. ((2013) ). Technological Pedagogical Content Knowledge Self-Efficacy Scale (TPACK-SeS) for Pre-Service Science Teachers: Construction, Validation, and Reliability. Eurasian Journal of Educational Research, 52: , 37-60. |
[12] | Busuioc, M. ((2021) ). Accountable artificial intelligence: Holding algorithmsto account. Public Administration Review, 81: (5), 825-836. |
[13] | CBSS (n.a.), Information in English, retrieved on 30-05-2022 from: https://www.ksz-bcss.fgov.be/en. |
[14] | Cucciniello, M., Porumbescu, G. A., & Grimmelikhuijsen, S. ((2017) ). 25 years of transparency research: Evidence and future directions. Public Administration Review, 77: (1), 32-44. |
[15] | Degli Esposti, S., Ball, K., & Dibb, S. ((2021) ). What’s In It For Us? Benevolence, National Security, and Digital Surveillance. Public Administration Review, 81: (5), 862-873. |
[16] | European Commission (n.a.), About MAGDA platform, https://joinup.ec.europa.eu/collection/egovernment/solution/magda-platform/about. |
[17] | Flanders Investment & Trade ((2022) ), SolidLab (Flanders) helps set new data safety standard, available at: https://www.flandersinvestmentandtrade.com/invest/en/news/solidlab-flanders-helps-set-new-data-safety-standard-0. |
[18] | Floridi, L. ((2019) ). Establishing the rules for building trustworthy AI. Nature Machine Intelligence, 1: (6), 261-262. |
[19] | Giest, S. ((2017) ). Big data for policymaking: fad or fasttrack? Policy Sciences, 50: (3), 367-382. |
[20] | Hamm, J. A., Smidt, C., & Mayer, R. C. ((2019) ). Understanding the psychological nature and mechanisms of political trust. PloS One, 14: (5), e0215835. |
[21] | Janssen, M., & Kuk, G. ((2016) ). Big and open linked data (BOLD) in government: A challenge to transparency and privacy? Government Information Quarterly, 32: , 363-368. |
[22] | Janssen, M., & van den Hoven, J. ((2015) ). Big and Open Linked Data (BOLD) in government: A challenge to transparency and privacy? Government Information Quarterly, 32: (4), 363-368. |
[23] | Jobin, A., Ienca, M., & Vayena, E. ((2019) ). The global landscape of AIethics guidelines. Nature Machine Intelligence, 1: (9), 389-399. |
[24] | Grimmelikhuijsen, S. ((2009) ). Do transparent government agencies strengthen trust? Information Polity, 14: (3), 173-186. |
[25] | Grimmelikhuijsen, S ((2022) ). Explaining why the computer says no: algorithmic transparency affects the perceived trustworthiness of automated decision-making. Public Administration Review. |
[26] | Grimmelikhuijsen, S., & Knies, E. ((2017) ). Validating a scale for citizentrust in government organizations. International Review of Administrative Sciences, 83: (3), 583-601. |
[27] | Howard, M. C. ((2014) ). Creation of a computer self-efficacy measure: analysis of internal consistency, psychometric properties, and validity. Cyberpsychology, Behavior, and Social Networking, 17: (10), 677-681. |
[28] | Kleizen, B., Van Dooren, W., & Verhoest, K. ((2022) ). Chapter 6: Trustworthiness in an era of data analytics: what are governments dealing with and how is civil society responding? In The new digital eragovernance: How new digital technologies are shaping public governance (pp. 563-574). Wageningen Academic Publishers. |
[29] | Kozyreva, A., Lewandowsky, S., & Hertwig, R. ((2020) ). Citizens versus the internet: Confronting digital challenges with cognitive tools. PsychologicalScience in the Public Interest, 21: (3), 103-156. |
[30] | Lankton, N. K., & McKnight, D. H. ((2011) ). What does it mean to trust facebook? Examining technology and interpersonal trust beliefs. ACM SIGMIS Database: The DATABASE for Advances in Information Systems, 42: (2), 32-54. |
[31] | Lips, A. M. B., Taylor, J. A., & Organ, J. ((2009) ). Managing citizen identity information in E-government service relationships in the UK: theemergence of a surveillance state or a service state? Public Management Review, 11: (6), 833-856. |
[32] | Malhotra, N. K., Kim, S. S., & Agarwal, J. ((2004) ). Internet users’ information privacy concerns (IUIPC): The construct, the scale, and a causal model. Information Systems Research, 15: (4), 336-355. |
[33] | Matheus, R., & Janssen, M. ((2020) ). A systematic literature study to unravel transparency enabled by open government data: The window theory. Public Performance & Management Review, 43: (3), 503-534. |
[34] | Meuwese, A. ((2020) ). Regulating algorithmic decision-making one case at the time: A note on the Dutch ‘SyRI’ judgment. European Review of Digital Administration & Law, 1: (1), 209-212. |
[35] | Meijer, A. ((2009) ). Understanding modern transparency. International Reviewof Administrative Sciences, 75: (2), 255-269. |
[36] | Murphy, C. A., Coover, D., & Owen, S. V. ((1989) ). Development and validation of the computer self-efficacy scale. Educational andPsychological measurement, 49: (4), 893-899. |
[37] | Naik, N., & Paul, J. ((2020) ). Self-Sovereign Identity Specifications: Govern your identity through your digital wallet using blockchain technology. 2020 8th IEEE International Conference on Mobile Cloud Computing, Services, and Engineering (MobileCloud). IEEE. |
[38] | O’Hara, K. ((2012) , June). Transparency, open data and trust in government: shaping the infosphere. In Proceedings of the 4th annual ACM web science conference (pp. 223-232). |
[39] | Olsthoorn, P. ((2022) ), Van wie is al die data? Retrieved on 30-5-2022 from: https://www.ictmagazine.nl/achter-het-nieuws/van-wie-is-al-die-data/. |
[40] | Stirton, L., & Lodge, M. ((2001) ). Transparency mechanisms: Building publicness into public services. Journal of Law and Society, 28: (4), 471-489. |
[41] | Van Dyke, T. P., Midha, V., & Nemati, H. ((2007) ). The effect of consumer privacy empowerment on trust and privacy concerns in e-commerce. Electronic Markets, 17: (1), 68-81. |
[42] | Van Ooijen, I., & Vrabec, H. U. ((2019) ). Does the GDPR enhance consumers’ control over personal data? An analysis from a behavioural perspective. Journal of Consumer Policy, 42: (1), 91-107. |
[43] | Whitley, E. A. ((2009) ). Informational privacy, consent and the “control” of personal data. Information Security Technical Report, 14: (3), 154-159. |
[44] | Zahran, S., Brody, S. D., Grover, H., & Vedlitz, A. ((2006) ). Climate change vulnerability and policy support. Society and Natural Resources, 19: (9), 771-789. |
Appendices
Appendix 1: Full vignettes
Treatment group 1
Baseline: The federal government is increasingly using the personal data of citizens for its services. To that end, public services often exchange data with other public services or private companies (for instance employers).
Recently, a system has been proposed that would make exchanging all this data easier. The data will remain stored at its source, but with the new system a public service will be able to more quickly request and use your data.
Treatment 1: The federal government is well aware that privacy must be safeguarded when exchanging data. For that reason, the federal government is working on an additional project allowing you to exercise more control over who has access to the existing data on you. You could for instance decide yourself whether wage data gathered by the tax service can be shared with other public services or private companies. This gives you more control over what data is exchanged with whom.
[page break]
Treatment 2: On the previous page you saw a scenario on a new system through which data could be exchanged. In our research, we are interested in your opinion on data exchanges through such systems.
We would therefore like to ask who could use your personal data if this were your own choice. Check which organizations you would provide permission to consult the types of data listed below.
Important: you can check multiple types of organization per type of data.
Item list:
[note: categories below are shown as survey items with answer categories: 1. private companies, 2. the federal government, 3. regional governments (Walloon or Flemish government), 4. local governments (e.g. municipality and Public Centre for Social Welfare)]
1. Identification data (name, address, etc.)
2. Labor, wage and career data
3. Tax data
4. Social status data (welfare, persons with a handicap, family subsidies, etc.)
5. Data on police and justice (fines, potential earlier convictions, etc.)
6. Education data
7. Data on registered vehicles
8. Real estate (house or summer residence)
Treatment group 2: Information (information on control)
Baseline: The federal government is increasingly using the personal data of citizens for its services. To that end, public services often exchange data with other public services or private companies (for instance employers).
Recently, a system has been proposed that would make exchanging all this data easier. The data will remain stored at its source, but with the new system a public service will be able to more quickly request and use your data.
The federal government is well aware that privacy must be safeguarded when exchanging data. For that reason, the federal government is working on an additional project allowing you to exercise more control over who has access to the existing data on you. You could for instance decide yourself whether wage data gathered by the tax service can be shared with other public services or private companies. This gives you more control over what data is exchanged with whom.
[page break]
With the new system, a government service could request the following data types from other governments or private companies:
[note: categories below are shown as a simple list of text, not as items that respondents can fill in]
1. Identification data (name, address, etc.)
2. Labor, wage and career data
3. Tax data
4. Social status data (welfare, persons with a handicap, family subsidies, etc.)
5. Data on police and justice (fines, potential earlier convictions, etc.)
6. Education data
7. Data on registered vehicles
8. Real estate (house or summer residence)
Treatment group 3: Exercise (direct control)
Baseline: The federal government is increasingly using the personal data of citizens for its services. To that end, public services often exchange data with other public services or private companies (for instance employers).
Recently, a system has been proposed that would make exchanging all this data easier. The data will remain stored at its source, but with the new system a public service will be able to more quickly request and use your data.
[page break]
On the previous page you saw a scenario on a new system through which data could be exchanged. In our research, we are interested in your opinion on data exchanges through such systems.
We would therefore like to ask who could use your personal data if this were your own choice. Check which organizations you would provide permission to consult the types of data listed below.
Important: you can check multiple types of organization per type of data.
Answer categories: 1. private companies, 2. the federal government, 3. regional governments (Walloon or Flemish government), 4. local governments (e.g. municipality and Public Centre for Social Welfare)
Item list:
1. Identification data (name, address, etc.)
2. Labor, wage and career data
3. Tax data
4. Social status data (welfare, persons with a handicap, family subsidies, etc.)
5. Data on police and justice (fines, potential earlier convictions, etc.)
6. Education data
7. Data on registered vehicles
8. Real estate (house or summer residence)
Appendix 2: Information on power analyses
Power analysis was used to determine necessary sample size, based on a prior experiment in the same research project on trustworthiness and AI, which was considered the most comparable setup. As the effects in this previous experiment were tenuous at best, they provide a conservative starting point for power analysis. In the previous experiment, stronger effect sizes had the following values: mean 1
Author biographies
Bjorn Kleizen is a postdoctoral researcher at the University of Antwerp, Faculty of Social Sciences, research group Politics & Public Governance and is affiliated to the GOVTRUST Centre of Excellence. His research focuses on citizen trust regarding new digital technologies in government.
Wouter Van Dooren is a professor of public administration in the Research Group Politics & Public Governance and the Antwerp Management School. His research interests include public governance, performance information, accountability and learning, and productive conflict in public participation. He is a member of the Urban Studies Institute and the GOVTRUST Centre of Excellence.