How smart can government be? Exploring barriers to the adoption of smart government
Abstract
Smart information and communication technologies (ICTs) are finding their ways into public administration, and numerous smart government efforts are marking the start of a new digitalization wave in the public sector. Despite being in the early stages of development, these initiatives promise a new model for the provision of public services: smart government. Because past technical innovations in the public sector did not reach their full potential, it is crucial to know the difficulties if one is to successfully address them. We explore the perceived barriers to the adoption of smart government in an early phase of implementation. We analyzed barriers, utilizing 32 interviews with actors involved in smart government initiatives. Cluster analysis helped us to identify six barrier groups: a lack of legitimacy, a lack of legal foundations, a lack of policy coherence, a lack of technical infrastructure, cost-benefit considerations, and a lack of innovation capacity. We distinguish between organizational and institutional barriers, and discuss restrictions and implications for praxis and future research.
1.Introduction
Digitalization is crucial if public administration is to thoroughly modernize. Sophisticated technologies such as the internet of things (IoT), sensor systems, big data analytics, and artificial intelligence (AI) have become increasingly important. Many digital initiatives that use these technologies in the public sector are launched under the umbrella term smart government, with the purpose of establishing new service delivery models by connecting and integrating physical, digital, public, and private environments (Gil-Garcia, 2012; Scholl & Scholl, 2014). These approaches go one important step further than past digitalization endeavors, asking how the relationship between public administration and its stakeholders could be rethought by using new technologies (Bright & Margetts, 2016). Thus, smart government is considered to be the next step of electronic government (e-government), mobile government (m-government), or open government (Gascó, 2015; Jimenez et al., 2014).
Starting with e-government, this first digitalization wave sought to create a digital environment in which public authorities provided services to their citizens electronically by utilizing the Internet and the World Wide Web (United Nations & ASPA, 2002). Terms such as Virtual State (Fountain, 2001) or NetState (Lawson, 1998) refer to this phenomenon. With the emergence of portable devices and the widespread availability of broadband wireless networks, the era of m-government has transferred such a “going online” of government from desktops to mobile device screens, without changing any other process parameters or service logics (Albesher & Stone, 2016). In contrast to e-government and m-government, smart government not only wants to digitalize processes, but to fundamentally rethink the way government works (Schedler, 2018). While e-government and m-government are forerunners of smart government, open government initiatives are a precondition for it (Jimenez et al., 2014). Without open data and open action in terms of transparency, collaboration, and participation – as Gascó (2015) describes the criteria and features of open government – no new and smart services are possible and, therefore, no smart government is possible (Guenduez et al., in press).
Smart government promises to success in further modernizing the public sector. However, many initiatives are still in early stages. Especially in early stages, there is a gap between rhetoric and reality, and between expected outcomes and achieved results. Moon (2002) showed this in his analysis of the introduction of e-government in public administration, pointing out that this gap is caused by widely shared barriers that hinder the successful uses of new information and communication technologies (ICTs) in public administration. Many other researchers – including Gilbert et al., (2004), Zakareya and Zahir (2005), and Savoldelli et al., (2014) – have documented barriers to the adoption of e-government. These studies have shown that many e-government initiatives to modernize the public sector did not reach their full potential. Thus, such initiatives are increasingly being questioned, and most fall well short of expectations (Anthopoulos et al., 2016).
We explore the perceived barriers to the adoption of smart government. With this research goal in mind, our study addresses the following questions: What are the specific barriers to the adoption of smart government and how can these barriers be classified? Following Moon (2002), we investigate the barriers in an early phase of smart government implementation, because these barriers especially affect this critical stage. We base our analysis on the perceptions of those involved in smart government initiatives in Switzerland.
We find this particular study objective worthwhile, for two reasons. First, pre-adoption attitudes can serve as an early indicator of the success of smart government implementation in the public sector. Ginsberg and Venkatraman (1992), for instance, showed that actors’ perceptions and interpretations of a new technology for the electronic filing of tax returns in 1987 predicted the introduction of the technology a year later. Similarly, Thomas et al., (1993) showed that hospital managers’ interpretations in 1987 predicted strategic changes over the next three years. Both studies showed that the involved actors’ pre-adoption attitudes and interpretations regarding an emerging technology or a strategic re-orientation predicted the introduction of the technology or chosen strategy in the following years. Thus, pre-adoption attitudes of actors involved in smart government initiatives may be an early indicator of strategic change and the success or failure of smart government implementation. Understanding what difficulties they face or expect can therefore be crucial to the successful implementation of smart government projects. Second, the early detection and understanding of these challenges is crucial to raise awareness about the complexity of smart government projects and to address them early on to successfully overcome them. Early detection enables proactive action, increasing the chances of success of smart government in the modernization of public administration. The remainder of this paper is structured as follows. In Section 2, we review the literature on smart government and on barriers to ICT implementation in public administration, drawing attention to the need for an analysis of the barriers to the adoption of smart government. In Section 3, we apply a mixed-method research approach by performing cluster analysis to coded qualitative interview data. Based on the evidence from interpreting the cluster analysis results, we then discuss our study’s primary contributions and delineate some limitations and our study’s implications for future research and practice.
2.Background
2.1Smart government
Smart government describes a new generation of concepts for the application of information technologies (ITs) in the public sector that collect, connect, and analyze huge volumes and variety of data generated and processed in (near) real-time. The term was used early on, very broadly, to describe a government that finds clever and elegant solutions to complex problems (Kliksberg, 2000). Today, smart government is an umbrella term for many digital initiatives in the public sector that use emerging technologies to creatively link physical, digital, public, and private environments (Bhatti et al., 2015; Rochet & Correa, 2016; Scholl & Scholl, 2014) fostering citizen and customer orientation (Gascó-Hernandez, 2018) as well as citizen and customer participation (Schedler, 2018). To describe smart government initiatives, scholars further refer to innovation (Gil-Garcia et al., 2014; Mergel, 2018), service co-design and co-creation (Guenduez et al., 2017), proactive services (Linders et al., 2015), implementation of business-like service delivery models (Schedler, 2018), agility (Mergel, 2016), a community-based governance model (Coe et al., 2001), extensive uses of technologies by governments (Mellouli et al., 2014), smooth information flows and collaborative decision-making (Chun et al., 2010), and utilizing the power of data (Harsh & Ichalkaranje, 2015). At the heart of smart government initiatives are efforts to creatively use emerging technologies (e.g. sensors, IoT, and big data) to fulfil promises that e-government has been making. These include fostering openness, innovation, citizen-centricity, citizen engagement, improving efficiency and effectiveness, and inter-organizational and intra-organizational collaboration in the public sector, which are considered the core elements of smart government initiatives (Anthopoulos et al., 2016; Scholl & Scholl, 2014). Taken together, smart government promises to fundamentally change how governments work (Schedler, 2018).
2.2Barriers to innovation in the public sector
Many studies have conceptually and empirically examined the challenges of and barriers to technology adoption in public administrations. Fountain’s (2001) framework of technology enactment, as an example of the conceptual work, distinguishes between objective ITs and enacted technologies. Simply put, objective technologies exist ‘out there’ in the public administration environment, while enacted technologies are the technologies that have been applied by public administration, as the process in which objective IT meets an organization, with its specific characteristics (e.g. bureaucracy, networks, and other organizational features), embedded in a political-administrative system’s institutional arrangements. According to Fountain (2001), how and whether an objective technology is enacted and then implemented depends on institutional and organizational arrangements that guide public decision-makers in their everyday behaviors. Fountain’s model is commonly used to describe interactions between organizational forms and institutional arrangements and their impacts on a technological system’s design (Cordella & Iannacci, 2010; Luna-Reyes & Gil-Garcia, 2014). Both factors – organizational forms and institutional arrangements – may hinder the adoption of new technologies in the public sector. For instance, Salvoldelli and colleagues (2014) showed that institutional arrangements impeded the adoption of e-government solutions in the European Union. Conradie and Choenni (2014) showed similar results for open data in the Netherlands concerning organizational factors.
Both organizational forms and institutional arrangements influence whether organizational actors perceive and interpret objective technologies positively or negatively – as potentially useful or useless (Zilber, 2006). Thus, acceptance of objective technologies strongly depends on their compatibility with existing institutional and organizational settings.
Empirical analyses of barriers to ICT implementation in the public sector have mainly focused on e-government – in technological terms, a past public sector innovation. Numerous empirical studies found barriers to the adoption of e-government, including a lack of trust (Gilbert et al., 2004), general concerns about citizen security, privacy, and data ownership (Schwester, 2009; Wing, 2005; Zakareya & Zahir, 2005), information quality (Gilbert et al., 2004), strategy (Wing, 2005; Zakareya & Zahir, 2005), technology (Schwester, 2009; Wing, 2005; Zakareya & Zahir, 2005), policy (Wing, 2005), leadership and management (Kim, 2009; Schedler & Schmidt, 2004; Schwester, 2009), accessibility (Becker, 2004; Gilbert et al., 2004), and organizational shortcomings (Chen & Gant, 2001; Schwester, 2009; Wing, 2005; Zakareya & Zahir, 2005). In their meta-analysis, Savoldelli et al. (2014) found three barrier groups to e-government adoption: technological and economical, managerial and organizational, and institutional and political. While in the first and last phases, institutional and political barriers have been predominant, technological and managerial barriers have been found to be the most important in the strategy implementation phase (Salvoldelli et al., 2014). Thus, barriers’ importances change during the implementation process.
While the research has built up much knowledge about barriers to e-government adoption on conceptual and empirical grounds, very few studies have provided clues to potential barriers to smart government initiatives. In the Continental European jurisdiction this study is located in, smart government initiatives require a legal framework that regulates privacy, access to data, data use, and liability (Conradie & Choenni, 2014; Janssen et al., 2012). Data sensitivity is a major concern for citizens and politicians. Mergel and colleagues (2016, p. 932) argue that, “In public affairs, citizens’ unease with the perceived loss of privacy creates limits on the use of public data for both government operations and public affairs research.” Janssen et al. (2012) also described a series of institutional barriers to publicizing government data; these include an unclear tradeoff between public values; a risk-averse culture (no entrepreneurship); a revenue system based on creating income from data; and conflicts of interest between local organizations and citizens. Hoffmann-Riem (2017) also takes a critical stance, arguing that the fundamental principles of the Rechtsstaat (constitutionality) are challenged because smart technologies, particularly pre-emptive computing systems, undermine every citizen’s autonomy. Kornberger et al. (2017) compared the requirements for open government to the conceptual requirements of a Weberian bureaucracy, finding various institutional contradictions that could lead to conflicts in practical implementation: “ we juxtapose the decentralizing principles of the crowd, such as transparency, participation, and distributed cognition, with the centralizing principles of bureaucracy, such as secrecy, expert knowledge, written files and rules” (p. 180).
2.3Context of the study
We investigate barriers to smart government initiatives in Switzerland. Although Switzerland has been at the very top in the Global Innovation Index for years, it lags behind concerning innovation projects in the public sector. Digital initiatives in Switzerland encounter difficulties. For instance, concerning e-government, these initiatives have only worked partly (Schedler, 2018). More than a decade after the introduction of e-government, the Federal Bureau for Economy concludes that, compared to other European countries, Switzerland needs to catch up (Buess et al., 2017). A number of factors could have prevented digital initiatives from making rapid progress in Switzerland. However, the federal state structure is a key factor. The 26 cantons and around 2500 communes are granted a high degree of self-organization and autonomy in fulfilling their tasks. Thus, implementation of digital initiatives in Switzerland is largely decentralized and involves various stakeholders at different government levels (Mettler, 2018). This can lead to a lengthy process of reaching political consensus (Linder, 2010). According to Mettler (2018, pp. 184–185), “Switzerland’s decentral form of governance significantly increases the complexity of the country’s digital transformation and ultimately negatively influences the pace at which emerging technologies are introduced”.
3.Methodology
We conducted 32 semi-structured interviews to explore the perceived barriers to the adoption of smart government. Because smart government is a new study field, and evidence of its (non)-adoption is fragmented, we chose to conduct semi-structured interviews, which facilitate insights into emerging fields (Gill et al., 2008). Semi-structured interviews have also been proven effective in gleaning broad-based information (Blasius, 1994). The total duration of the interviews (held in German or English) was 30 hours. Data collection took place from April to June 2017.
Sample. For participant selection, we defined three relevant actor categories involved in smart government initiatives: public managers, politicians, and private providers of smart government solutions. The categories provide three perspectives on the barriers to the adoption of smart government. Politicians bring the perspective of legislation, public managers that of executive authorities, and providers an outside view of the political-administrative settings in which smart government initiatives take place. We interviewed 13 public sector managers from different organizational departments located in different communities. Represented were executives from IT departments, organizational development departments, statistics departments, spatial development departments, and municipal utilities. Eight politicians with an interest in smart government initiatives participated; they are active in different parties (all the major Swiss parties were represented in the sample) and at different state levels (communal, cantonal, and federal). Thus, the politicians subsample represents a broad spectrum of observations and opinions. In the group of private providers of smart government solutions, the 11 participants also covered a wide range of perspectives. We interviewed hardware suppliers (e.g. sensors), software suppliers (e.g. applications), and business consultants. For every actor category, we assigned three known experts, each either strategically or operationally involved in smart government initiatives. Using a snowball sampling method, we then recruited new participants. This procedure ensured that the sample captured and represented as many different opinions as possible.
Procedure. The interview guidelines (see Appendix) for collecting raw data contained six topics: strategy, infrastructure (both existing and planned), possible applications, potentials for public service delivery, requirements for a transformation, and limitations. We evaluated the barriers to each of the six topics separately. Fountain’s (2001) model of technology enactment helped us to develop our interview questions, since we addressed organizational forms and institutional arrangements.
First, we wanted to know whether these actors had concrete strategies about smart government implementation. A strategy is often a means to guide an organization and its actors towards an intended direction (in our case, towards the adoption of smart government). Thus, we consider a strategy as an organizational form. The research indicated that conflicting or uncoordinated goals hinder the adoption of e-government; conversely, clear and realistic goals are key success factors for e-government implementation (Gil-Garcia & Pardo, 2005).
The next topic was infrastructure or “objective technology” (Fountain, 2001). Inadequate telecommunication infrastructures, which limit capacity, have been found to be significant barriers in early phases of e-government (Savoldelli et al., 2014). Technical constraints, such as a lack of platforms or meta-standards (an IT supply issue in a broad sense), have also been found to hinder the adoption of open data applications in the public sector (Janssen et al., 2012). Thus, we asked about existing IT infrastructure such as sensors, apps, networks, platforms, or data analysis tools and therefore about currently enacted technologies. We also asked questions about possible plans to extend this infrastructure and, if so, how and why.
We then asked about possible organizational forms or institutional arrangements that foster or hinder the adoption (and therefore the enactment) of smart government initiatives. We asked about possible applications of smart technologies, for examples of their own modernization projects, and about potential improvement of public service outcomes, taking a more normative perspective. These topics provide an overview of existing ideas, but also of many possible pitfalls. We then instructed the participants to consider all the opportunities smart government can provide, asking: Why has your department or public administration not yet implemented these applications? By asking this, we encouraged the subjects to share their thoughts about what hinders the adoption of smart government initiatives at a more implicit level. Further, we wanted them to identify possible limitations of smart government, asking if they saw aspects or application areas that required constraint, and encouraging them to explicitly share the barriers they perceived. We recorded and later transcribed all the interviews.
Analysis of the interview data. We analyzed the data in two stages. In step 1, we developed a codebook containing barriers to the adoption of smart government. To do this, we assessed the literature about barriers to the adoption of innovations in the public sector overall (De Vries et al., 2016), because the public sector has characteristics that play roles in the implementation of innovations (Borins, 2000). We also examined the literature about barriers to e-government initiatives (Savoldelli et al., 2014), since e-government initiatives were one of the most recent major innovation waves in the public sector. We thoroughly assessed the literature about difficulties of open data and open government initiatives (Conradie & Choenni, 2014; Janssen et al., 2012). The literature about open data and open government serves as orientation, because these initiative types contain some elements of smart government, for instance, big data or data analytics. This screening process revealed 17 barriers to the adoption of smart government: legal foundations, technical infrastructure, IT standards, political resources, plurality, silo thinking, the Swiss political system, scarce financial resources, efficiency, discomfort, citizens’ responses, skills and know-how, readiness for innovation, risk-aversion, long-term thinking, management support, and contested benefits. We validated these 17 barriers with the model of technology enactment in order to ensure that the three relevant factors – objective technology, organizational forms, and institutional arrangements – were captured by our data analysis.
Table 1
Barriers to smart government adoption and their operationalizations
Clusters | Barriers | Operationalization of the barriers | Frequencies | |
---|---|---|---|---|
Unit of analysis | ||||
Keywords | Present | Absent | ||
Legal foundations | Legal foundations | Lack of legal foundations | 35 | 157 |
Strict laws | ||||
Constraints in dealing with data | ||||
Constraining effects of laws | ||||
Technical | Technical infrastructure | Insufficient technical infrastructure | 36 | 156 |
infrastructure | Insufficient IT infrastructure | |||
Cost-benefit | Political resources | Lack of political will, interest, or motivation | 20 | 172 |
relationships | Contested | Contested potentials | ||
benefits | Contested needs | 11 | 181 | |
Contested benefits | ||||
Contested relevance | ||||
Efficiency | Constraints about effectiveness and/or efficiency | 17 | 175 | |
Cost-benefit relationships | ||||
Scarce financial | High costs | 33 | 159 | |
resources | A shortage of financial resources | |||
A focus on costs rather than benefits | ||||
Policy coherence | Plurality | Plurality of actors | 29 | 163 |
Democratic system | ||||
Silo thinking | A lack of willingness to cooperate | 26 | 166 | |
Silo thinking | ||||
A lack of connectedness between the actors | ||||
the Swiss political | Federal system | 21 | 171 | |
system | Inertia in the political-administrative system | |||
Autonomy of authorities or political communities | ||||
IT standards | A lack of compatibility of IT structures | 22 | 170 | |
A lack of standardization of ICT | ||||
Long-term thinking | A lack of long-term orientation | 26 | 166 | |
A lack of general strategy | ||||
A lack of vision | ||||
Legitimacy | Discomfort | Emphasis on risks | 30 | 162 |
Fears and concerns | ||||
Insufficient or a lack of security | ||||
Citizens’ responses | Citizens’ resonance | 22 | 170 | |
Citizens’ acceptance | ||||
Citizens’ participation | ||||
Innovativeness | Skills and know-how | A lack of expertise | 32 | 160 |
A lack of interdisciplinariness | ||||
A lack of understanding | ||||
A lack of specialists | ||||
Readiness for | A resistance to change | 30 | 162 | |
innovation | An unwillingness to innovate | |||
Risk-aversion | Insufficient or a lack of tolerance of mistakes | 15 | 177 | |
Risk-avoidance | ||||
Management support | A lack of initiative in political-administrative management | 32 | 160 | |
A lack of support in political-administrative management |
In step 2, we conducted a cluster analysis, which meant that the interviews had to be coded in order to gain analyzable data. We used the variables developed in step 2 of the data analysis. To operationalize the 17 barriers, we defined keywords that served as decision criteria if that barrier was present or absent. For a complete overview of all barriers and their operationalization, see Table 1. We coded a barrier’s presence in the interview data as 1, and its absence as 0. Two coders evaluated the data. Before rating, both coders underwent a training session in which all the barriers and their operationalizations were explained. To assess interrater reliability, they coded 36 randomly selected interview questions. Conformity ranged between 86.1% and 100%, which was satisfactory. The remaining 156 interview questions were randomly assigned to each coder.
We then conducted a variable hierarchical cluster analysis using SPSS to examine whether these variables formed conceptual groups. Cluster analysis is an explorative and structure-detecting method that builds different groups or clusters. While the variables (or cases) in a cluster are very similar, the different clusters are distinct (Blasius & Baur, 2014; Backhaus et al., 2011; Mooi & Sarstedt, 2011). Cluster analysis is commonly used to classify different single cases into groups (Blasius & Baur, 2014; Backhaus et al., 2011; Mooi & Sarstedt, 2011). However, according to Blasius (1994) and Ek (2014), variables (not cases) can also be clustered according to similarities. We used the clustering method, because it allows one to group variables (here, barriers) based on their de facto similarities. Thus, the barriers’ configuration was not based solely on our interpretation, but on the clustering algorithm, which is a more objective criterion than our interpretation.
In the clustering method, we used the subcategory of complete linkage to analyze data. This algorithm, also known as the furthest neighbor method, can be used with binary data and tends to build fairly small groups (Mooi & Sarstedt, 2011), which fits our study aims. We used simple matching to represent the similarities between different variables. This measure is well established in analyzing binary data for a cluster analysis (Backhaus et al., 2011). Since there is no common decision criterion to identify the ideal cluster number, scholars recommend exploring different solutions and choosing the most readily explainable one (Backhaus et al., 2011; Mooi & Sarstedt, 2011). Because there is no appropriate number of clusters, we chose a solution that allowed us to reduce complexity without losing diversity and that is also easy to explain.
4.Results
Our first scan of the literature and our interview data revealed 17 possible barriers. We then applied a cluster analysis to the data to explore whether these barriers were somehow similar. The cluster analysis revealed six clusters containing between one and five variables. We display the results in a dendrogram (see Fig. 1). We will now explain the six clusters, what they consist of, and why these variables fit into the same group.
4.1Cluster 1: Legal foundations
Cluster 1 consisted of a single variable, legal foundations, which was mentioned 35 times. We operationalized this cluster with the keywords lack of legal foundations, strict law, constraints in dealing with data, and constraining effects of the law. It addresses problems with a lack of or too strict laws or regulations. For instance:
“Data protection must be safeguarded by a strong sense of proportion. We probably have the wrong legislation today, which prevents too much. One should punish the abuse and not simply prohibit everything preventatively”.
Further, the cluster contains a more specific topic: concerns about dealing with data. This indicates that legal foundations should simultaneously be loose and protective, to enable the adoption of smart government. The research has identified legal barriers to e-government (Gil-Garcia & Pardo, 2005) and to open data initiatives (Janssen et al., 2012).
4.2Cluster 2: Technical infrastructure
Cluster 2 also contained only one barrier, technical infrastructure, which was mentioned 36 times. It contained two aspects: technical infrastructure (the hardware) and IT infrastructure (the software). We identified the barriers with these keywords: lack of technical infrastructure and lack of IT infrastructure. A sample statement was:
“We need new communication standards such as fifth-generation [5G] mobile networks or long-range wide area networks [LORA] in order to push smart government applications”.
Several studies have shown that technical infrastructure is a key challenge when implementing new technologies into the public sector (Savoldelli et al., 2014; Schwester, 2009; Wing, 2005; Zakareya & Zahir, 2005), especially in early implementation stages (Savoldelli et al., 2014).
Figure 1.
Dendrogram with the complete linkage method and simple matching as a similarity index that shows the six-cluster solution.
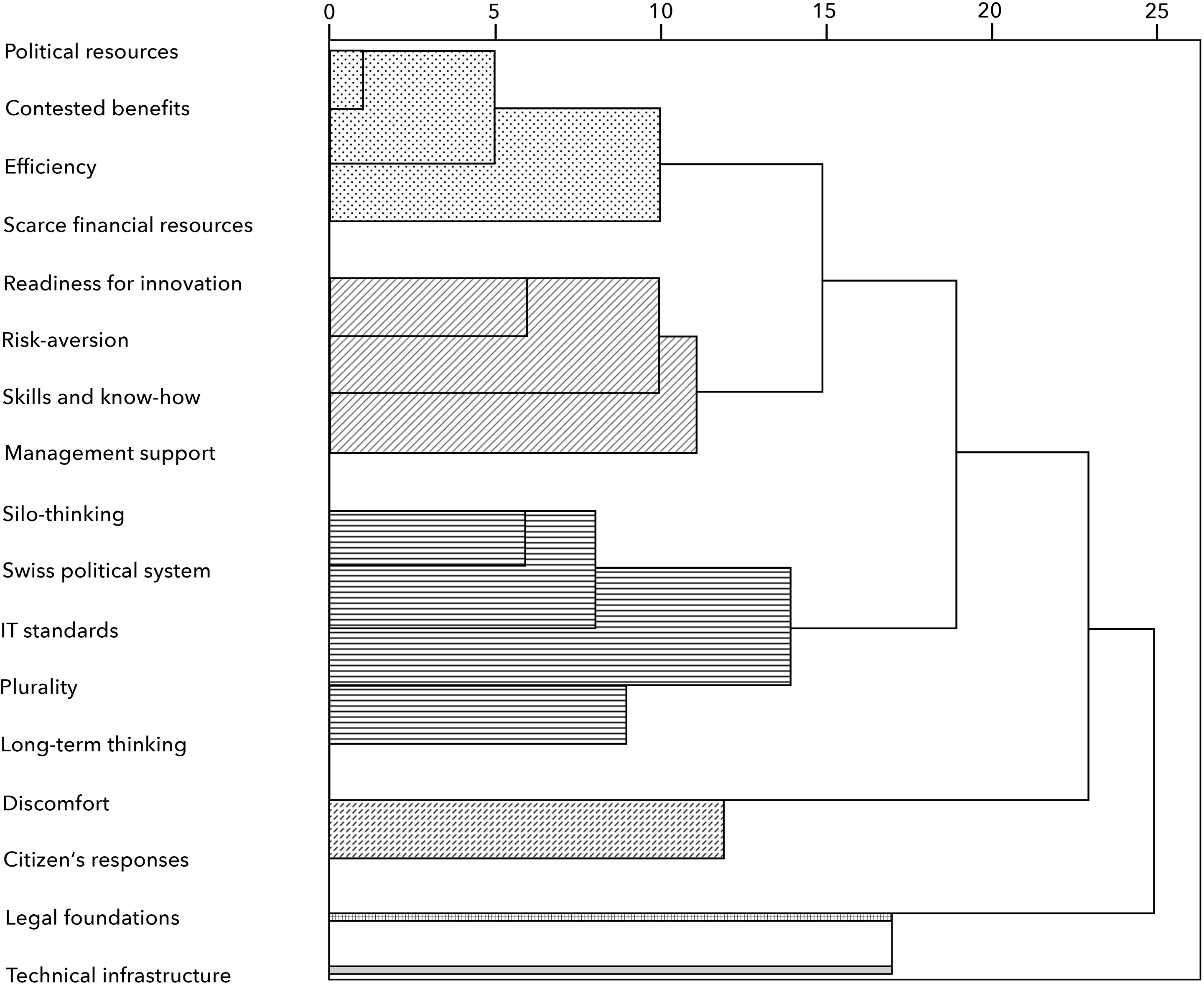
4.3Cluster 3: Cost-benefit relationships
Cluster 3 brings together four barriers: political resources (mentioned 20 times), contested benefits (mentioned 11 times), efficiency (mentioned 14 times), and scarce financial resources (mentioned 33 times). In sum, the barriers in this cluster were mentioned 81 times. They address (financial or personal) resource allocation. We name this cluster cost-benefit relationships, because it is unclear whether financial or reputational risks outweigh the gains. A participant indicated:
“It’s a question of cost-benefit considerations. We don’t yet know whether smart government is at all worthwhile”.
These insufficient profits can be seen in the variables’ content: contested potential, needs, benefits, or relevance (for contested benefits), constraints about efficiency or effectiveness, and cost-benefit relationships if efficiency measures indicate that it is unclear whether the new solutions are better than the existing ones. Scarce financial resources were identified with the keywords high costs, lack of financial resources, and focus on costs rather than benefits. The financial risks are complemented by reputational risks. Reputation in this context can be seen as a political resource, which in this context can be seen as apolitical resource. We operationalized this barrier with the keywords lack of political will, interest, or motivation, suggesting that it is not worth engaging in smart government initiatives. It also subtly indicates that this is not what voters are (now) interested in. The existence of cost-benefit considerations is in line with previous research findings (Zakareya & Zahir, 2005) showed that a lack of central government funding hinders the adoption of innovations in the public sector.
4.4Cluster 4: Innovativeness
Cluster 4 (innovativeness) contains these variables: readiness for innovation (mentioned 30 times), risk-aversion (mentioned 15 times), management support (mentioned 32 times), and skills and know-how (mentioned 32 times). Altogether, issues connected to innovativeness were mentioned 109 times. We called this cluster innovativeness, because it describes the overall problem of bringing innovations into public administration. This barrier group suggests that public administration is not yet ready to implement new technologies or processes, as embodied in smart government, for two reasons. First, public administration does not currently have the necessary technical or managerial skills and know-how for a reform such as smart government. An interviewee noted:
“What is certainly lacking is knowledge, in the political sphere and in public administration. There is insufficient knowledge to tackle important issues.”
These challenges can be seen in the operationalization of two variables: skills and know-how as well as management support. The former was measured with these keywords: lack of expertise, lack of interdisciplinary approach, lack of understanding, and lack of specialists. Management support was identified by these keywords: lack of initiative and/or support in the political-administrative system. Second, characteristics of public administration workplaces, such as risk-aversion and low incentives for innovation, also hindered the implementation of smart government initiatives. As a participant explained: “As operators of so-called critical infrastructures, we traditionally carry out little experimentation. This is in our DNA.” We used the keywords insufficient tolerance of mistakes and risk-avoidance as indicators for risk-aversion and resistance to change and unwillingness to innovate as indicators for readiness for innovation. Janssen and colleagues (2012) also found risk-averse culture to be a barrier to the adoption of open data initiatives. To sum up, this cluster addresses concerns and reservations of people who are implementing smart government initiatives and therefore operate inside the political-administrative system. This stands in contrast to Cluster 5, which addresses citizens’ concerns and thus takes an external perspective of the political-administrative system.
4.5Cluster 5: Legitimacy
We called Cluster 5 legitimacy (as the willingness to accept and support change); it brings together two variables: discomfort (mentioned 30 times) and citizens’ responses (mentioned 22 times). This group shows that, in addition to public administration and politics (as described in Cluster 4), various other actors are reluctant to adopt smart government. Thus, legitimacy represents the external view, i.e. the perspective of smart governments’ target audiences. Fears and objections surface during all aspects of implementation, from broad-based general concerns, to security, to mistrust. A politician stated:
“The general public has a skeptical to negative attitude towards digitalization.”
These aspects can be seen in discomfort, which we operationalized using these keywords: emphasis of risks, fears, and concerns and insufficient or a lack of security. The interviews also indicated that citizens don’t respond to smart government initiatives, are unprepared to accept these solutions, and are unwilling to participate in them, indicating that smart government initiatives lack legitimacy. The variable citizens’ responses represent this issue, and was measured with the keywords citizens’ responses, citizens’ acceptance, and citizens’ participation. This barrier group is also in line with the findings of Savoldelli et al. (2014), who found a lack of citizens’ participation as well as a lack of transparency and trust to be barriers to the adoption of e-government initiatives.
4.6Cluster 6: Policy coherence
Cluster 6 included five variables: silo thinking (mentioned 26 times), the Swiss political system (mentioned 21 times), plurality (mentioned 29 times), IT standards (mentioned 22 times), and long-term thinking (mentioned 26 times). In total, variables in this cluster were mentioned 124 times. Taken together, the variables address issues of collaboration and coordination between and within the three different state levels (in Switzerland: community, canton, and federation). This interviewee statement summarized the overall problem addressed by this cluster’s barriers:
“In other words, responsibility is shifted between departments or state levels. There is no clear responsibility for these projects.”
The cluster is based on factors partly inherent in the Swiss federal system, which emphasizes the autonomy of cantons and communities (Linder, 2010). Accordingly, we measured the barriers in the Swiss political system with these keywords: federal system, inertia, and autonomy. We operationalized plurality with the keywords plurality of actors and democratic system, addressing aspects that are similar to the Swiss political system, yet broader. The policy coherence cluster also addresses insufficient IT standards, which are manifest in the lack of compatibility or standardization of IT infrastructures. Silo thinking refers to problems in collaboration; we measured it with the keywords lack of willingness to cooperate, silo thinking, and lack of connection between relevant actors. With every department, community, or canton acting independently and emphasizing its own autonomy, policies become incoherent. A lack of political commitment, political coordination, and strategy have also been identified in previous studies as barriers to the adoption of e-government (Savoldelli et al., 2014; Wing, 2005). These studies also indicated that policy inconsistencies are not a typical Swiss phenomenon, although the country’s federalist and highly fragmented state structure (Linder, 2010) fosters this; they are widespread.
5.Discussion and conclusion
Smart government is the newest modernization wave in the public sector and promises to bring more customer orientation and effective administrative action via the application of data-driven technologies. Since smart government is still in its infancy (i.e. pre-adoption) phase, the perceptions and expectations of public managers and other actors involved in these projects are highly influential and can even determine their success or failure. We have explored and described which barriers and challenges these people perceive when initiating and adopting such projects.
To understand the perceptions of actors involved in these projects, we explored data from 32 semi-structured interviews. This procedure led to 17 barriers to the adoption of smart government. To explore whether and how these barriers interrelate, we conducted a cluster analysis, which revealed six different barrier groups: legal foundations, technical infrastructure, cost-benefit relationships, innovativeness, legitimacy, and policy coherence.
Fountain’s (2001) distinction between institutional and organizational features for technology adoption is also present in our data. Cost-benefit relationships, innovativeness, and technical infrastructure are organizational barriers, because they occur inside a public administration and its departments. The reported lack of clarity about cost-benefit relationships in smart government initiatives leads decision-makers to exercise restraint. If an organization is able to establish new business models and revenue structures, these concerns are likely to diminish. Innovativeness refers to an organization’s capacity to introduce new processes, products, or ideas into the organization (Hult et al., 2004), and is often studied as an attribute of an organization’s innovation culture (Pervaiz, 1998). Smart government initiatives also require innovativeness, since they seek to establish new models of public service delivery and new products. The innovation cluster combines aspects such as resistance to change, risk-avoidance, inadequate political-administrative management initiatives, and a lack of skills, which fit Pervaiz’s (1998) conclusions. Further, organizations usually decide themselves which hardware and software to use. Providing technical infrastructure that enables smart government initiatives forms part of an organization’s scope. Even if private organizations can decide more independently, this still applies to public organizations, since hardware and software use is rarely a political decision.
It follows that an organization must address issues concerning innovativeness, cost-benefit relationships, and technical infrastructure. Establishing new laws that enable smart government initiatives, overcome existing policy incoherencies, and increase legitimacy among citizens (their willingness to accept and support changes they consider to be beneficial) are also perceived as key for the successful implementation of smart government initiatives. However, an organization can do little about policy coherence, legitimacy, and legal foundations. Based on Fountain’s (2001) framework of technology enactment, we can say that these barriers exist outside the organization and form part of the institutional framework in which a public administration is located.
As the legitimacy cluster suggests, creating and maintaining legitimacy for smart government is a major challenge for project leaders and politicians. Skepticism about the use and abuse of behavioral data is widespread and steadily increasing. However, recent studies show that citizens accept data collection, connection, and analysis more readily if they understand specifically how their government will use such data (van Zoonen, 2016). Further, since citizens’ acceptance and uses of ICT-based smart government services are influenced by their social groups (Yeh, 2017), policymakers should find ways to communicate comprehensively with all these groups in their jurisdictions. These services must also focus on users’ primary needs: service quality, innovative delivery, and privacy.
Further, policy coherence suggests that there are many different interests and actors in smart government initiatives that need to be coordinated. Beyond the peculiarities of Switzerland’s political-administrative system, policy coherence has three aspects: First, collaboration across different entities in a public administration, for instance when inter-agency collaboration is required for specific tasks. Second, collaboration across government levels in cases where multilevel governance solutions are necessary, for instance, when state and city governments share responsibilities for specific issues. Third, collaboration over time, for instance, when smart government is implemented in sequential phases. Clear strategies foster the diffusion of e-government (Savoldelli et al., 2014; Wing, 2005). Thus, the policy coherence cluster describes the institutional setting in which a public administration is embedded. The same applies for legal foundations.
A glance at the research into innovation adoption in the public sector shows that the identified clusters are similar to past research findings. Legal barriers, financial resources, policy coherence, and aspects of the innovation cluster have been found to hinder the adoption of e-government (Gil-Garcia & Pardo, 2005; Savoldelli et al., 2014; Schwester, 2009) and the implementation of open data initiatives (Janssen et al., 2012). A lack of technical infrastructure, cost-benefit considerations, and legitimacy were found to be barriers to the adoption of e-government (Gil-Garcia & Pardo, 2005; Savoldelli et al., 2014; Schwester, 2009; Wing, 2005; Zakareya & Zahir, 2005). Thus, it can be concluded that the barriers to innovation adoption remain the same, regardless of the innovation type, indicating that these barriers are deeply rooted in the public sector and are therefore hard to address or eliminate. Considering this, and considering that these barriers were also present in the advanced stages of e-government implementation, it can also be concluded that they may not exclusively relate to the early stages of smart government adoption, but may also be present in later stages.
Taken together, this paper makes three main contributions. First, the 17 barriers give us clues about what actors involved in smart government have in mind when planning and implementing such initiatives. Our findings suggest that they must address 17 heterogeneous barriers summed up as legal foundations, technical infrastructure, cost-benefit relationships, innovativeness, legitimacy, and policy coherence more or less simultaneously. This indicates that the adoption of smart government is a complex and multifaceted task with many potential pitfalls. The six clusters can provide guidance on the factors that should be considered when implementing smart government projects. Second, the barriers we have found seem to be more or less the same as in the era of e-government. Thus, barriers encountered in the e-government era still strongly impact on new digitalization waves, of which smart government is one. In other words, the successful implementation of smart government is threatened by the same factors as in the case of e-government. Conversely, recipes for success in e-government may also hold true for smart government. Third, there are both organizational and institutional barriers to the adoption of smart government. Thu, the actors involved in the implementation of such initiatives must consider their own organization as well as the institutional framework when implementing them. Addressing organizational barriers (technical infrastructure, cost-benefit relationships, and innovativeness) may be within their scope of action, while overcoming barriers embedded in the institutional framework (policy coherence, legal foundations, and legitimacy) may go beyond their sphere of influence.
5.1Limitations
The technology enactment model, which helped us to develop the interview questions and interpret our results, is usually used to take a processual perspective of technology enactment (Cordella & Iannacci, 2010; Luna-Reyes & Gil-Garcia, 2014). The way we use the model differed – our research is a snapshot of organizational forms and institutional arrangements that currently hinder the enactment of smart technologies in the public sector in Switzerland. It may well be that this snapshot looks different in other countries or changes over time. However, the overview of possible barriers is useful, since public managers and other practitioners can use it as a heuristic overview of possible challenges.
Further, our data are based on subjective perceptions of relevant actors in an early implementation stage, rather than on empirical facts from a later implementation stage. By interviewing 32 actors with different professional backgrounds who are involved in smart government, we sought to capture a wide range of opinions and perceptions to understand what the main barriers to the adoption of smart government are. As noted, our results address smart government challenges in Switzerland, a Continental European country with a particular Napoleonic political-administrative system. This becomes evident in the policy coherence cluster, which refers to federalism, direct democracy, and decentralized political power. Finally, our investigation strongly depended on the barriers identified in past research. By using our iterative method, we may have missed some barriers that our codebook was unable to capture. Although they cover a range of possible barriers, we may have missed some barriers mentioned ‘between the lines’ owing to our method of analysis. In spite of these limitations, in our view, this study had pinpointed and defined clusters and barriers that are relevant to and crucial for both current and future smart government projects.
5.2Implications for research
We focused on an early phase of smart government initiatives in Switzerland, providing temporal and local insights. As evidenced by Savoldelli et al. (2014), barriers to ICT projects can change, strengthen, or weaken over time. This is also true for the institutional and organizational barriers we have identified. Institutional and organizational arrangements are not always linear. They may change in response to external shocks or shifts in the distribution of power. Thus, organizational and institutional barriers to the implementation of smart governments may vary over time, reflecting specific events and local conditions. Additional research is needed to better understand how organizational and institutional barriers evolve and change depending on the study context. Although this was not the study’s focus, it represents an interesting and promising path for future research. In particular, longitudinal analyses in Switzerland and other parts of the world may provide valuable insights. The increasing number of smart government initiatives across the globe also opens the possibility of cross-national comparative analysis. Additional research is also needed to develop a more nuanced picture of what hinders the implementation of smart government initiatives. We have somewhat simplified the complex picture of the adoption of smart government in order to provide an overview. Research designs that allow in-depth analysis of a particular barrier may be fruitful for understanding the adoption of smart government. Finally, we made no assumptions about factors that facilitate or even foster the implementation of smart government; investigating these factors could represent a new and exciting future research direction.
5.3Implications for praxis
We identified 17 barriers that should be considered in smart government initiatives. This is a considerable number, since every barrier addresses a fairly general aspect, rather than a highly specific question or problem. For actors involved in smart government initiatives, this means that these projects are complex and therefore risk failing. Taken together, this emphasizes the complexity of smart government projects and shows that these projects require careful management if they are to succeed. Further, the presence of 17 perceived barriers shows that smart government is much more than just new technologies. Technical problems were outlined in only two barriers; 15 barriers represent institutional and other organizational challenges, which are key. Thus, relevant actors such as public managers should not neglect non-technical barriers if they wish to successfully implement smart government initiatives. However, only the organizational barriers may be approachable in the short term. Institutional barriers are much harder to tackle, since they are deeply rooted in a social and political system. Thus, it may be helpful to focus on organizational barriers when managing smart government initiatives.
References
[1] | Albesher, A. S., & Stone, R. T. ((2016) ). Current state of m-government research: identifying future research opportunities. International Journal of Electronic Governance, 8: (2), 119-139. |
[2] | Anthopoulos, L., Reddick, C. G., Giannakidou, I., & Mavridis, N. ((2016) ). Why e-government projects fail? An analysis of the Healthcare.gov website. Government Information Quarterly, 33: (1), 161-173. |
[3] | Backhaus, K., Erichson, B., Plinke, W., & Weiber, R. ((2011) ). Multivariate Analysemethoden. Eine anwendungsorientierte Einfführung [Multivariate analysis methods. An application-oriented introduction]. Heidelberg: Springer. |
[4] | Becker, S. A. ((2004) ). E-government visual accessibility for older adult users. Social Science Computer Review, 22: (1), 11-23. |
[5] | Bhatti, Z. K., Kusek, J. Z., & Verheijen, T. ((2015) ). Logged on: Smart government solutions from South Asia. Washington, DC, World Bank. |
[6] | Blasius, J., & Baur, N. ((2014) ) Multivariate Datenanalyse [Multivariate data analysis]. In: Baur N., Blasius J. (eds) Handbuch Methoden der empirischen Sozialforschung [Handbook Methods of Empirical Social Research]. Springer VS, Wiesbaden. |
[7] | Blasius, J. ((1994) ). Empirische Lebensstilforschung [Empirical Lifestyle Research]. In J. S. u. B. Dangschat, Jörg (Ed.), Lebensstile in den Städten. Konzepte und Methoden [Lifestyles in the cities. Concepts and methods]. Opladen: Leske |
[8] | Borins, S. ((2000) ). Loose cannons and rule breakers, or enterprising leaders? Some evidence about innovative public managers. Public Administration Review, 60: (6), 498-507. |
[9] | Bright, J., & Margetts, H. ((2016) ). Big Data and Public Policy: Can It Succeed Where E-Participation Has Failed? Policy and Internet, 8: (3), 218-224. |
[10] | Buess, M., Iselin, M., & Bieri, O. ((2017) ). Nationale E-Government Studie 2017. E-Government in der Schweiz aus Sicht der Bevölkerung, der Unternehmen und der Verwaltung. (National e-government study 2017: e-government in Switzerland from the perspective of the population, companies and administration. |
[11] | Chen, Y. C., & Gant, J. ((2001) ). Transforming local e-government services: the use of application service providers. Government Information Quarterly, 18: (4), 343-355. |
[12] | Chun, S. A., Shulman, S., Sandoval, R., & Hovy, E. ((2010) ). Government 2.0: Making connections between citizens, data and government. Information Polity, 15: (1/2), 1-9. |
[13] | Coe, A., Paquet, G., & Roy, J. ((2001) ). E-governance and smart communities - A social learning challenge. Social Science Computer Review, 19: (1), 80-93. |
[14] | Conradie, P., & Choenni, S. ((2014) ). On the barriers for local government releasing open data. Government Information Quarterly, 31: , S10-S17. |
[15] | Cordella, A., & Iannacci, F. ((2010) ). Information systems in the public sector: The e-Government enactment framework. Journal of Strategic Information Systems, 19: (1), 52-66. |
[16] | De Vries, H., Bekkers, V., & Tummers, L. ((2016) ). Innovation in the Public Sector: A Systematic Review and Future Research Agenda. Public Administration, 94: (1), 146-166. |
[17] | Ek, Å, Runefors, M., & Borell, J. ((2014) ). Relationships between safety culture aspects – A work process to enable interpretation. Marine Policy, 44: , 179-186. |
[18] | Fountain, J. E. ((2001) ). Building the Virtual State. Information Technology and Institutional Change. Washinton: Brooking Institution Press. |
[19] | Gascó, M. ((2015) ). Special Issue on Open Government: An Introduction. Social Science Computer Review, 33: (5), 535-539. |
[20] | Gascó-Hernandez, M. ((2018) ). Building a Smart City: Lessons from Barcelona. Communications of the Acm, 61: (4), 50-57. |
[21] | Gil-Garcia, J. R. ((2012) ). Towards a smart state? Inter-agency collaboration, information integration, and beyond. Information Polity, 17: (1), 269-280. |
[22] | Gil-Garcia, J. R., Helbig, N., & Ojo, A. ((2014) ). Being smart: Emerging technologies and innovation in the public sector. Government Information Quarterly, 31), 11-18. aaa(000) Gil-Garcia, J. R., & Pardo, T. A. (2005). E-government success factors: Mapping practical tools to theoretical foundations. Government Information Quarterly, 22(2), 187-216. aaa(000) Gilbert, D., Balestrini, P., & Littleboy, D. (2004). Barriers and benefits in the adoption of e-government. International Journal of Public Sector Management, 17(4), 286-301. aaa(000) Gill, P., Stewart, K., Treasure, E., & Chadwick, B. (2008). Methods of data collection in qualitative research: interviews and focus groups. British Dental Journal, 204(6), 291-295. aaa(000) Ginsberg, A., & Venkatraman, N. (1992). Investing in New Information Technology – The Role of Competitive Posture and Issue Diagnosis. Strategic Management Journal, 13), 37-53. aaa(000) Guenduez, A. A., Mettler, T., & Schedler, K. (in press). Beyond Smart and Connected Governments: Sensors and the Internet of Things in the Public Sector. In: J. Ramon Gil-Garcia, Theresa A. Pardo & Mila Gascó Ramon (eds.), Beyond Smart and Connected Governments: Sensors and the Internet of Things in the Public Sector. Springer. aaa(000) Guenduez, A. A., Mettler, T., & Schedler, K. (2017). Smart Government – Partizipation und Empowerment der Bürger im Zeitalter von Big Data und personalisierter Algorithmen. [Smart Government – Participation and empowerment of citizens in the era of big data and personalized algorithms] HMD. aaa(000) Harsh, A., & Ichalkaranje, N. (2015). Transforming e-Government to Smart Government: A South Australian Perspective. In L. C. Jain, S. Patnaik, & missingN. Ichalkaranje: (Eds.), Intelligent Computing, Communication and Devices: Proceedings of ICCD 2014, Volume 1 (pp. 9-16). New Delhi: Springer India. |
[30] | Hoffmann-Riem, W. ((2017) ). Verhaltenssteuerung durch Algorithmen – Eine Herausforderung für das Recht [Behavioural control through algorithms – A challenge for the law]. Archiv des öffentlichen Rechts, 142: , 1-42. |
[31] | Hult, G. T. M., Hurley, R. F., & Knight, G. A. ((2004) ). Innovativeness: Its antecedents and impact on business performance. Industrial Marketing Management, 33: (5), 429-438. |
[32] | Janssen, M., Charalabidis, Y., & Zuiderwijk, A. ((2012) ). Benefits, Adoption Barriers and Myths of Open Data and Open Government. Information Systems Management,, 29: (4), 258-268. |
[33] | Jimenez, C. E., Solanas, A., & Falcone, F. ((2014) ). E-Government Interoperability: Linking Open and Smart Government. Computer, 47: (10), 22-24. |
[34] | Kim, S. ((2009) ). A case study of local e-government performance in South Korea: Do leadership and management for results matter? International Public Management Review, 10: (1), 170-198. |
[35] | Kliksberg, B. ((2000) ). Rebuilding the state for social development: towards ‘smart government’. International Review of Administrative Sciences, 66: , 241-257. |
[36] | Kornberger, M., Meyer, R., Brandtner, C., & Höllerer, M. A. ((2017) ). When bureaucracy meets the crowd: Studying ’open government’ in the Vienna City Administration. Organization Studies, 38: (2), 179-200. |
[37] | Lawson, G. ((1998) ). NetState: Creating electronic government. London: Demos. |
[38] | Linder, W. ((2010) ). Swiss Democracy: Possible Solutions to Conflict in Multicultural Societies (3rd Edition), New York: Palgrave Macmillan. |
[39] | Linders, D., Liao, Z.-P. C., & Wang, C.-M. ((2015) ). Proactive e-Governance: Flipping the service delivery model from pull to push in Taiwan. Government Information Quarterly, 1-9. |
[40] | Luna-Reyes, L. F., & Gil-Garcia, J. R. ((2014) ). Digital government transformation and internet portals: The co-evolution of technology, organizations, and institutions. Government Information Quarterly, 31: (4), 545-555. |
[41] | Mellouli, S., Luna-Reyes, L. F., & Zhang, J. ((2014) ). Smart Government, Citizen Participation and Open Data. Information Polity, 19: (1), 1-4. |
[42] | Mergel, I. ((2016) ). Agile innovation management in government: A research agenda. Government Information Quarterly, 33: (3), 516-523. |
[43] | Mergel, I. ((2018) ). Open innovation in the public sector: drivers and barriers for the adoption of Challenge.gov. Public Management Review, 20: (5), 726-745. |
[44] | Mergel, I., Rethemeyer, R. K., & Isett, K. ((2016) ). Big data in public affairs. Public Administration Review, 76: (6), 928-937. |
[45] | Mettler, T. ((2018) ). The Road to Digital and Smart Government in Switzerland. In A. Ladner, N. Soguel, Y. Emery, S. Weerts, & S. Nahrath (Eds.), Swiss Public Administration : Making the State Work Successfully (pp. 175-186): Springer International Publishing. |
[46] | Mooi, E., & Sarstedt, M. ((2011) ). Cluster Analysis. In E. Mooi & M. Sarstedt (Eds.), A concise guide to market research. Berlin Heidelberg: Springer-Verlag. |
[47] | Moon, M. J. ((2002) ). The evolution of e-government among municipalities: Rhetoric or reality? Public Administration Review, 62: (4), 424-433. |
[48] | Pervaiz, K. A. ((1998) ). Culture and climate for innovation. European Journal of Innovation Management, 1: (1), 30-43. |
[49] | Rochet, C., & Correa, J. D. P. ((2016) ). Urban Lifecycle Management: A Research Program for Smart Government of Smart Cities. Revista de Gestão e Secretariado, 7: (2), 1-20. |
[50] | Savoldelli, A., Codagnone, C., & Misuraca, G. ((2014) ). Understanding the e-government paradox: Learning from literature and practice on barriers to adoption. Government Information Quarterly, 31: , S63-S71. |
[51] | Schedler, K. ((2018) ). Von Electronic Government und Smart GovernmentMehr als elektrifizieren! [About Electronic Government and Smart Government. More than just electrify!]. IMPuls, 1(1). |
[52] | Schedler, K., & Schmidt, B. ((2004) ). Managing the e-government organization. International Public Management Review, 5: (1), 1-20. |
[53] | Scholl, H. J., & Scholl, M. C. ((2014) ). Smart Governance: A Roadmap for Research and Practice. Paper presented at the iConference 2014 Proceedings. |
[54] | Schwester, R. ((2009) ). Examining the barriers to e-government adoption. Electronic Journal of e-Government, 7: (1), 113-122. |
[55] | Thomas, J. B., Clark, S. M., & Gioia, D. A. ((1993) ). Strategic sensemaking and organizational performance – linkages among scanning, interpretation, action, and outcomes. Academy of Management Journal, 36: (2), 239-270. |
[56] | United Nations, & ASPA, ((2002) ). Benchmarking e-government: A global perspective. New York, NY: U.N. Publications. |
[57] | van Zoonen, L. ((2016) ). Privacy concerns in smart cities. Government Information Quarterly, 33: (3), 472-480. |
[58] | Wing, L. ((2005) ). Barriers to e-government integration. Journal of Enterprise Information Management, 18: (5), 511-530. |
[59] | Yeh, H. ((2017) ). The effects of successful ICT-based smart city services: From citizens’ perspectives. Government Information Quarterly, 34: (3), 556-565. |
[60] | Zakareya, E., & Zahir, I. ((2005) ). E-government adoption: architecture and barriers. Business Process Management Journal, 11: (5), 589-611. |
[61] | Zilber, T. B. ((2006) ). The work of the symbolic in institutional processes: Translations of rational myths in Israeli high tech. Academy of Management Journal, 49: (2), 281-303. |
Appendices
Appendix
Semi-structured Interview Protocol
Personal details
First, I want you to ask about your current job and your past job experience.
• Where do you work?
• What is the job title of your current position?
• Which of the following best describes your position:
(a) Politician
(b) Head official
(c) IT manager
(d) Head of industrial company
(e) Provider of smart government solutions
(f) Consultant
• How experienced are you…
(a) In your current job?
(b) With change processes in public administration?
(c) With digitalization?
Introduction
Before we start the interview, I would like to give you some general information about it. We will talk about your perceptions of smart government. In our view, smart government deals with sensors, connectivity, data analysis, and automation. However, our idea of smart government is not important here. What we want to hear is your opinion.
Have you already dealt with smart government or digitalization (or similar) in your area or your community?
Topic 1: Vision/Strategy
We start with strategy, since it is usually present, even if smart technologies are not currently used.
Is there a smart government strategy?
If the answer is yes, please tell us about the strategy.
If the answer is no, why is there no strategy?
Topic 2: Infrastructure
[Acknowledge what has been explained.]
We now want to talk about what is already there in terms of infrastructure. Infrastructure may include IoT, sensors, fiber-optic networks, apps, and GPS tracking, to name a few.
What infrastructure for smart government solutions do you have in your organization or your community?
Are there any plans to extend the existing infrastructure?
Topic 3: Possible applications
We now go one step further, to talk about possible applications of these new technologies.
Where do you see possible applications for smart technologies in your organization or your area?
Topic 4: Potentials for public service delivery
[Acknowledge what has been said.]
As I explained at the start, we are investigating smart technologies in the context of public administration. We are especially interested in possible applications for the public sector.
Where do you see possible applications of smart technologies to improve public service delivery?
Topic 5: Requirements for a transformation
Are there any reasons why public administration has not yet implemented these possibilities?
Topic 6: Boundaries
Usually, not everything that is possible is not necessarily also desirable.
Do you see limits to the use of smart technologies in public administration?
Closing the conversation
Thank you for sharing your thoughts and opinions. If you would like the study results, kindly provide your e-mail address.