Collecting, exploring and sharing personal data: Why, how and where
Abstract
New, multi-channel personal data sources (like heart rate, sleep patterns, travel patterns, or social activities) are enabled by ever increased availability of miniaturised technologies embedded within smartphones and wearables. These data sources enable personal self-management of lifestyle choices (e.g., exercise, move to a bike-friendly area) and, on a large scale, scientific discoveries to improve health and quality of life. However, there are no simple and reliable ways for individuals to securely collect, explore and share these sources. Additionally, much data is also wasted, especially when the technology provider ceases to exist, leaving the users without any opportunity to retrieve own datasets from “dead” devices or systems. Our research reveals evidence of what we term human data bleeding and offers guidance on how to address current issues by reasoning upon five core aspects, namely technological, financial, legal, institutional and cultural factors. To this end, we present preliminary specifications of an open platform for personal data storage and quality of life research. The Open Health Archive (OHA) is a platform that would support individual, community and societal needs by facilitating collecting, exploring and sharing personal health and QoL data.
1.Introduction
The qualitative and quantitative data collection of biological, physiological, psychological and social-environmental factors may tell the beholder unprecedented information about the data subject – his behaviours, health risks and life quality in the long term. The World Health Organization (WHO) defines health as “a state of complete physical, mental and social well-being and not merely the absence of disease or infirmity.” This definition has recently been challenged by a team of international experts to be shifted to “the ability to adapt and self-manage in the face of social, physical, and emotional challenges” [1]. Health is directly related and contributes significantly to the quality of life (QoL) [2]. QoL can be defined via a more global approach (ex. happiness vs. unhappiness), a categorical breakdown (e.g., physical or psychological aspects) and field-specific definitions (Liver QoL) [2,3]. However, across different QoL definitions, there is some agreement that QoL integrates an individual’s multidimensional evaluation of his/her life [4]. Forging meaning from multidimensional factors in order to get a better understanding and outcomes of own quality of life (QoL) is undoubtedly a good reason to gather personal data. In this paper, we elaborate on the opportunities and challenges of collecting, exploring and sharing personal data to find effective solutions across the individual-community-society spectrum that help to improve QoL. A query in Google Trends11 for the worldwide search volume of the term “personal data” over 2004-present exhibits three peaks that coincide with relevant events related to EU policy for protecting privacy (Fig. 1). Those peaks appear in: Mar 2005: Recommendation Rec(2005)7 of the Committee of Ministers to Member States Concerning Identity and Travel Documents and the Fight Against Terrorism; Dec 2011: Agreement between the United States of America and the European Union on the use and transfer of Passenger Name Records (PNR) to the United States Department of Homeland Security; May 2018: General Data Protection Regulation (GDPR) came into force. Thus, we follow the definition of personal data given by the EU General Data Protection Regulation (GDPR). In brief, personal data is “any information relating to an identifiable person who can be directly or indirectly identified in particular by reference to an identifier”.22
Fig. 1.
The term “Personal Data” – Google global search trends reached peaks that coincide with relevant events related to EU policy for protecting privacy.
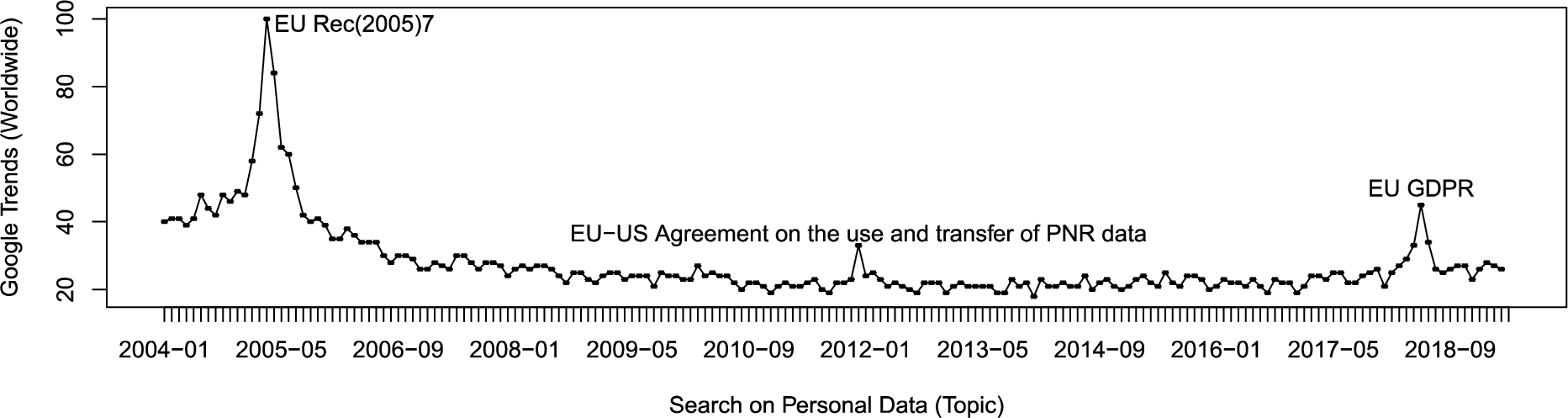
Alongside the developments within the personal data sphere, there is a demand of “multidimensional measurement of QoL” [5] at the individual and the population level. The World Health Organization (WHO) indicates that measuring QoL can provide valuable information in medical practice, for improving the doctor-patient relationship, as well as for assessing the effectiveness and merits of treatments, in health service evaluation, in research and policy making [6].
At the same time, the use of non-medical devices to maintain or restore health [7,8], reinforces the importance of patient-driven health care models [9] and consumer health care models [10] that include mobile health (mHealth) applications to track lifestyle habits, wrist-band trackers, or other kind of Internet of Things (IoT)/wearable devices. There is a plethora of scientific peer-review pervasive computing systems for health care [11–14]. Big data and IoT open the opportunity to bring global health innovations. They come with the promise of an algorithm-supported healthcare system that will be more efficient than the current one, prone to human errors, and will save more lives. However, digital technology produces these main trade-offs: privacy vs security, control vs freedom, dependence vs independence [15].
This dilemma raises tensions and concerns that lead to the question on why data controllers and data processors are usually data holders in the first place, suggesting the need for revisions on regulations to protect the right of the data subject to choose the storage location. One pitfall of EU data protection regulation is the “passive” role for the consumer/patient or study participant. The use of the term data subject does not empower the individual, whose voice is ultimately reduced to a signed data consent policy. Meanwhile, applications that target powerless consumers by claiming to give them control over personal data are multiplying. Some solutions offer personal data markets for helping individuals to generate revenue. Such business requires transparency to avoid facing legal, economic, technical and social challenges [16].
Overall, despite the data deluge of modern life, researchers rarely gain access to significant sources of personal data that integrate the multiple aspects of life. The wearable market will continue to grow while databases are kept behind commercial, ethical and legal barriers. The market will have a compound annual growth rate of 18.4% over the next three years, i.e., 222.3 million shipments in 2021 [17]. Based on the predictions, it is reasonable to expect a growing research interest in data retrieved from wearable technologies. Furthermore, projections for the year 2020 indicated that healthcare data would require 2,314 exabytes of storage space if it continues with a growing annual rate of 48% [18]. Thus it makes sense to rethink solutions for collecting, exploring and sharing personal data and its applications for quality of life.
Towards this end, in this paper, we elaborate on strategies to manage and preserve the sheer amount of personal data from a quality of life perspective. Building on a considerable body of literature, we strive for answers that respect individual, community and societal values, encourage individuals to be more engaged in healthy behaviours without losing control over their digital traces, and empower researchers and citizen scientists with an ever-growing health data source.
This article extends our paper [19] presented at the 4th Workshop on “Data-driven self-regulating systems” as part of the Foundations and Applications of Self* Systems (FAS* 2018). The main novel contributions are: Why, a semi-systematic review of the status of data providers as evidence of the human data bleeding defined later in this paper; How, our argumentation about five main issues to promote data access and sharing in this area; and Where, the set of requirements and design considerations for the Open Health Archive (OHA) derived from the literature and properties of existent solutions.
2.Personal health information
In this section, we briefly examine two approaches that impact on the design of health information systems, namely, the disease-centric and the patient-centric approaches. Then, we consolidate the different elements that create personal health information and analyze the role of emerging technologies as health outcomes. Finally, we elaborate on the difficulties to integrate emerging technologies into traditional healthcare systems.
2.1.Health information systems
Health information systems refer to systems that deal with health data and are used by patients, doctors, and decision-makers. By contrast, we use the term personal health information (ϕ)33 to indicate the interrelation between personal and health data. Personal data augment research studies based on medical and health records with more dimensions on health, quality of life and well-being.
Due to the myriad of technology innovations that provide sources for personal health information, we loosely characterize ϕ (uncountable) dimensions. We hypothesize that leveraging these dimensions can help researchers to get a holistic view of the person’s quality of life unfolding and changing over time, as well as a better understanding of health outcomes. This hypothesis is supported by numerous studies and reviews of the use of technology in health conditions, e.g., mental illness, injuries and disabilities, and cardiovascular diseases [20–25].
Decision making in healthcare systems is mostly based on health outcomes. Health outcomes are measurements of an aspect of health that provide a unique view of a health condition. Health outcomes could be generic or disease-specific [26], and both can be used in combination to gain more insight and compare alternative treatments. Disease-specific outcomes are considered ill-suited for the general population or patients with multiple chronic conditions; instead, the alternative patient-centric models that considered the perspective of the patient to measure health outcomes are promising [27].
Meanwhile, the vast bulk of patient data is collected in clinical studies through clinician-reported (ClinRO), performance-based (PerfO), self-reported (SRO) outcomes and emerging technologies (TechOs) [28]. Professionals assess activities in PerfO, while the participant is required to fill SRO. However, PerfO are momentary and expensive and SRO may be inaccurate due to perception bias.
Patient-centric approaches aim at providing a holistic approach that takes into account the multidimensional aspects of health, QoL and well-being. There is general agreement that QoL and well-being measurements have to be sought from the individual own’s perception, and wearable devices and smartphones facilitate its quantification in daily life activities. Consumers and patients with chronic conditions keep showing interest in these devices. In particular, quantified-self trackers are extreme consumers that wear multiple sensors and may develop their solution to capture data and run analytics if the market fails to satisfy their needs. In a sense, self-quantification and life-logging technologies are invaluable data sources for patient-centric research. These data collections can augment patient-supplied data in healthcare systems even for individuals who are not patients at any given moment.
2.2.Emerging technologies contributing to health outcomes
The spiral shown in Fig. 2 illustrates the ever-growing ecosystem of emerging technologies that create personal health information. Its inner sections correspond to records generated by healthcare/domain experts. Its outer sections incorporate data generated by non-experts and with less discrimination extends to the rich variety of lifelog data sources, examples can be found in the reference [29]. We assume that the integration of non-expert sources contributes to reducing data acquisition costs and increases the possibilities to capture with higher fidelity the “dynamic nature of quality of life” [30]. At least for healthy patients, the innermost section generates records every couple of months or years and frequency increases as we move towards the outer sections. An exception can be found in patients treated with complex medical procedures, where the innermost section becomes a source of high-dimensional high-resolution data too.
Fig. 2.
The main sources for personal health information are electronic medical records, electronic health records, patient-generated health data, wearable devices and mHealth apps. They contribute to fill the ever-growing volume of phi dimensional data about an individual.
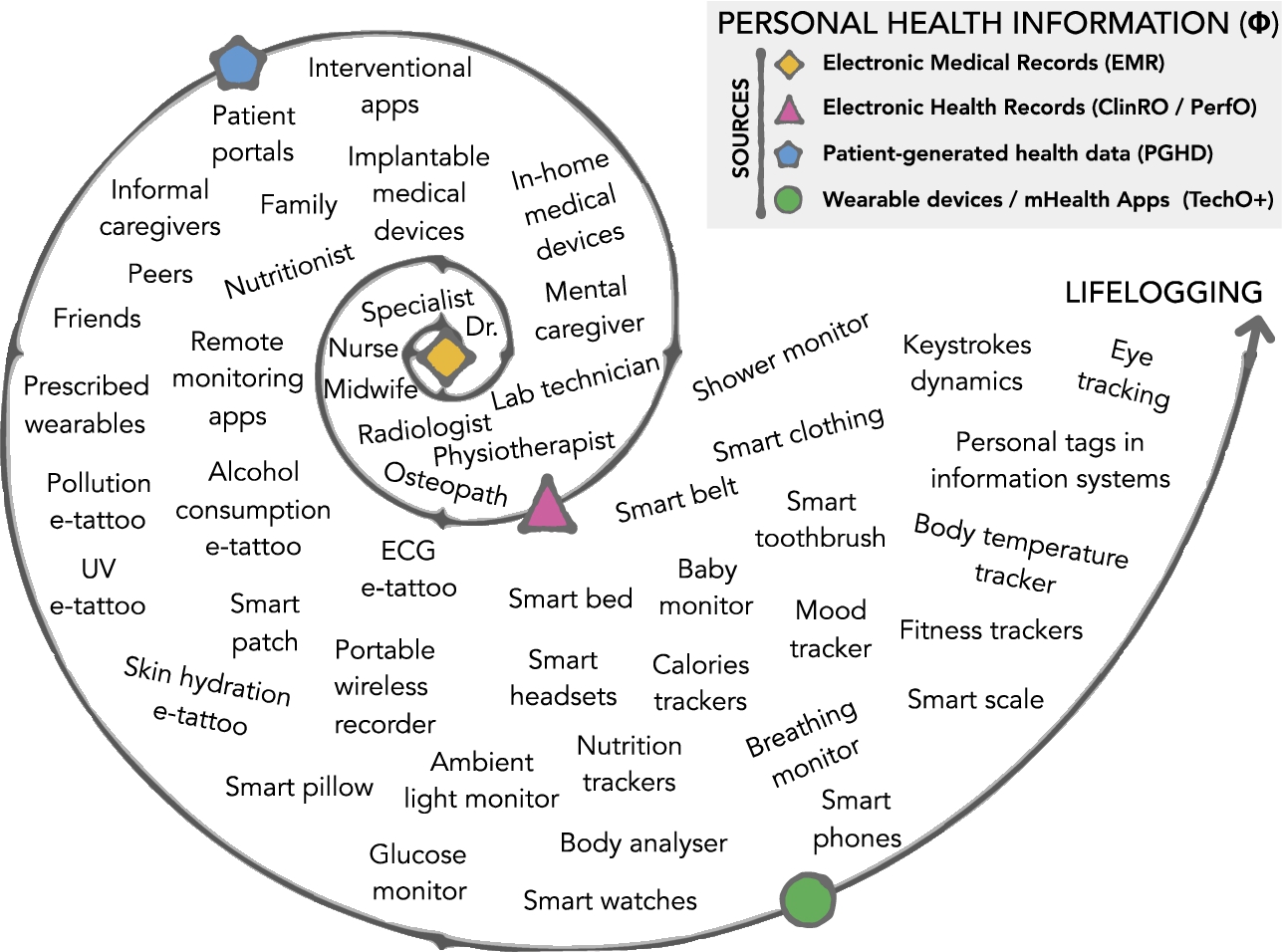
In patient-centric models, emerging technologies are likely to improve health outcomes. The time interval between measurements taken by experts can be long (except for patients with critical health conditions who are closely monitored in hospitals). The use of dynamic data is encouraging to understand if interventions are working or not. In other words, laying data out over time is useful to understand trends and improve analytics. Thus, measurements taken at home may unveil relevant patterns even if the measuring device was not designed for medical purposes. Furthermore, in the QoL domain, Evans and other researchers have argued that objective measurements should rely more on the actual manifested behaviour and current circumstances of the person compared with an external baseline [31]. Overall, dynamic data can provide a more comprehensive quantification than static data or self-reports used alone.
Table 1
Prevalent raw and algorithm-driven data in TechO+
TechO+ | |||
Algorithm-driven data | |||
Physical health | Mental health | Social relationships | Environment |
Fitness: duration, time, distance, calories burned, elevation, activity type Sleep: sleep cycle time distribution, wake up times, circadian rhythm, movements Nutrition: calorie intake, nutrition values, carbohydrates intake, fibre, water, protein Body mass: weight, height, fat percentage Vital signals: blood pressure, hormones, glucose levels, HR | Mood: via face gesture recognition, via voice recognition Mental disorder: early detection via keystroke dynamics and touch screen tracking Stress levels: via heart rate variability (HRV) Emotional arousal intensity: via GSR peaks Seasonal affective disorder (SAD): basedon climate data | Social network: interactions with close friends, interactions with family, interaction with colleagues | Ambient light: lux Location: via GPS Climate: temperature, number of daylight hours, extreme rain, etc. Time: time zone difference, shift work |
Raw data input | |||
Accelerometer – Gyroscope – Location GPS – Microphone – Thermometer – Oximeter – Altimeter – Lumen per m2 (LUX) Tooth brush pressure – Foot pressure – Air pressure – Keylogger – Touch screen events – ECG – EMG – Digital camera User inputs – Bluetooth proximity – Electrodermal activity/galvanic skin response (GSR) – Event tracking |
The spiral in Fig. 2 follows the distinction between “medical records” and “health records” given by the Office of the National Coordinator for Health Information Technology (ONC), US Department of Health and Human Services (HHS),44 and the taxonomy given in the Montreal accord on patient-reported outcomes [28]. The innermost section includes electronic medical records (EMRs), a digital version of the traditional paper patient’s history written in a 5 × 7 inch card. Electronic health records (EHRs) expand the value of EMRs. They contain information from all the clinicians involved in the patient’s care. Patient-generated health data (PGHD) expand the value of EMRs even more with health-related data created, recorded, or gathered by or from patients, family members or other caregivers. They include but are not restricted to patient-reported outcome measures (PROMs), observer-reported outcomes (ObsRO) and proxy reported outcomes (ProxRO). Examples are self-reported questionnaires, face to face interviews and telephone interviews about physical, mental, and social health. In the Montreal accord, technology-reported outcome (TechO) is vaguely mentioned as part of the clinician-reported outcomes (ClinRO) branch and directly linked with performance-reported outcomes (PerfO), which are assessments done by clinicians in close cooperation with the patient. However, health-related quality of life (HRQoL) measurements done with emerging technologies are gaining preponderance. We propose TechO+ to distinguish and take into consideration the data that is collected by apps, sensors, trackers and other IoT devices either worn by the user or available in the user’s environment, e.g., smart home or a smart car, to quantify different aspects of their life. TechO+ makes the outermost section of the spiral. It comprises raw data and algorithm-driven data that quantifies physical health, psychological health and social relationships, and the environment as described in Table 1. The main difference between Tech and TechO+ is that clinicians do not control data generated by TechO+.
2.3.TechO+ in health information systems
The introduction of emerging technologies in the healthcare sector is not new. A central argument is that PGHD and TechO+ data open the opportunity to develop new clinical decision-making metrics, where these new data sources can be leveraged for better diagnosis, assignment of treatment, prognosis and prediction of future health outcomes. The use of personal devices to create digital biomarkers can help researchers and doctors to diagnose diseases. For example, researchers are experimenting with keystroke and touch-screen tracking to early detect mental disorders [32]. ‘Bring your own device’ and similar policies can provide the means for more active patients, hence increasing the levels of patient engagement. However, the integration of emerging technologies into healthcare systems is not close to becoming a reality. The adoption level of patient engagement oriented technologies is varied, and there is space for improvements [33]. Countries like US and UK are just progressing towards nationwide electronic health systems, but migration is costly and complicated [34]. Healthcare information systems are dominated with inflexible legacy systems that do not integrate ClinRO, PerfO and SRO with external data sources. One main difficulty is the role of security and privacy in medical device software [35]. The WannaCry ransomware put in evidence that hospitals infrastructures are populated with outdated software [36]. Other challenges are the cost and legal implications in the case of devices that are prescribed by doctors. On a growing scale, questions are raised concerning data ownership, access rights and sharing of data collected with wearable medical devices due to intellectual property protections [37]. More questions are raised concerning the validity of measurements taken with consumer-grade devices, for example, the sensor placement plays a vital role [38]. Although techniques to minimize these errors are available, the position of the sensor is not always registered with the measurements. In addition, most vendors do not make their algorithms public; versions and update dates to these algorithms are not well documented. The same measurement can have different meanings across data captured with sensors from different vendors or across the same database during different periods.55 High data resolution may be unreliable if the device is in low power mode (not enough battery), manufacturers recommend using only daily aggregates for those cases.66 Researchers need access to data about battery life and syncing behavior in order to assess participant compliance and data reliability. Open questions remain regarding how to preserve the source of uncertainties, how to verify measurements reliability, and how to communicate uncertainties [39]. Nevertheless, there are research and development efforts that help the incorporation of wearables as medical technologies [40].
3.Why: The challenges of personal health information
The human body and behaviour is a source of big data, especially in the context of health. For example, a single X-ray accounts for 30 MB of data, a mammogram for 120 MB, 3D MRI for 150 MB, while 3D CT scan for 1 GB. Raw sequencing data per person accounts for 4 TB [41]. Stored healthcare data will continue to grow, and it is expected to be around 2,300 EB in 2020 [42]. Medical image archives are increasing 20–40% annually [43]. Electronic tattoos (e-tattoos) used together with a portable wireless multichannel electrical potential recorder can synchronously record three-channel ECG with a sampling rate of 1 kHz [44]. The Artemis system [45] supports the acquisition and storage of raw physiological streaming and clinical data to monitor multiple premature-born babies. Data channels such as ECGs and electroencephalograms are time sampled at 0.5–1 kHz. The initial pilot test generates less than 0.2 Mb/s network traffic. A soccer players dataset, which includes field position, heading, and speed, was collected with a highly accurate tracking system taking samples at 20 Hz [46]. That means a player who participates in a full game generates approximately 108,000 records. A coronary artery bypass grafting surgery is another example of a high-dimensional, high-resolution data source. This procedure can produce more than 200,000 points with potential value for outcome prediction and research. Nonetheless, data is discarded using a reductionist process to reduce investment in proper infrastructure [47].
Based on the above observations, we examine the challenges for collecting, exploring and sharing personal health from the individual’s and researcher’s perspective.
3.1.Individual perspective
Lifelogging, as defined by Microsoft MyLifeBits research project (1998–2007) [48], is the storage of selected qualitative and quantitative facets of life, namely professional communications, personal records and family activities. Based on MyLifeBits, it has been estimated that a 10-year personal collection required approximately 100 GB. This space requirement may change from one person to another, but such collections would fit in standard consumer hard disks. However, the big data and cloud storage market promotes a different approach. Lifelogging repositories are fragmented, in the sense that personal collections are stored in remote locations and distributed across different vendors. In this scenario, individuals may give away privacy based on illusions of what the solution claim to provide. A study in the US showed that 58.23% (934/1604) mobile phone users downloaded health-related mobile apps mostly with a focus on fitness and nutrition [49]. Many of these applications are unreliable. There are multiple examples of negligence and/or abuses of individual users’ trust that can lead to vulnerabilities and/or security breaches to gain more information about a subject [50–52]. If human blood is for traditional (analogue) healthcare the equivalent to human data for digital healthcare, we can talk about human data bleeding.
Furthermore, if not bleeding, the data may become inaccessible and completely lost when companies go out of business. Namely, nowadays, the app stores, as well as consumer electronics stores, host a variety of mobile health solutions. Smartphone users can choose from tens of hundreds of applications, designed to assess the behaviors (e.g., physical activity) and states (e.g., mood) and enable to prevent or manage certain diseases, or induce behavior change to improve health and life quality in general. There are also a variety of affordable, miniaturized and fashionable wearable devices that are designed to assess various behaviors (e.g., sleep, physical activity) unobtrusively to the wearer. In addition, there are patient-focused social networks that create an ecosystem to discuss health conditions with family, friends, informal caregivers, and health professionals. However, it is also observed that these apps, devices, and social networks get discontinued due to company acquisitions, business shifting focus, companies shutdown, and when research project supporting the wearable/apps has been completed. The disappearing networks, apps and dead wearables often imply that their data collections get lost, in most cases – without offering data migration solutions to the users.
To illustrate the wasted resources we discuss the state of the “lost data” evidence via a semi-systematic review of providers, as follows. We review 438 latest available personal wearable technologies enabling self-monitoring, as previously surveyed by Wac [53]. With respect to the wearables, 265 or 438 wearables surveyed by Wac, do not exist anymore. Many of the mobile apps and wearables that disappeared do not even have a corresponding website with information to their users anymore (e.g., web link returns an error or the domain is on sale). For the devices, we estimate the amount of data being lost assuming three (3) months of use, having different data channels (e.g., accelerometer, GPS) collected via a device. For each data channel, based on our past and ongoing experience with body area networks and wearable psycho-physiological devices [54,55] we firstly assume a continuous data stream ranging from high frequency/high resolution, for example, ECG (assumed 128 leads, each 256 Hz frequency, 16 bits per sample) to low frequency, for example, button interaction (assumed 1 event/minute on average, 8 bits per sample). We assume that the psycho-physiological data stream is collected 24/7, and an audio/video stream is collected on average an hour a day. Based on that, we calculate the total data loss incurred in three months for all discontinued devices [56]. We only compile it for the wearables, because we were able to archive the wearables database entries used at that time.77 We are unable to compile that for the mobile apps because we have not archived the details about the mobile apps themselves. The estimate of the data lost due to discontinuity of the wearables (and their corresponding apps) is as follows. For 265 discontinued devices, the overall data loss is estimated to be in the range of 6 TB (out of 9.85 TB for all the devices), with an average of 22.71 GB per a discontinued device providing on average four different data channels. Data waste is substantial.
Other complications appear with patient- and consumer-generated data from wearables and other sensors used for life tracking. The common practice is that devices synchronize their data to cloud-based storage systems directly or via smartphone applications. It becomes challenging to keep track all together with the data from multiple sensors all along the life span of the person. We conjecture that the lack of a reliable storage platform to keep personal health and health-related records may have a repercussion in the user engagement with mHealth technologies and health self-management.
3.2.QoL researcher perspective
From the perspective of researchers, there is no clear line between data ownership and data stewardship. In practice, the researcher takes the role of data owner, and study participants donate data for particular studies. Despite the tacit donation “to science”, databases are kept in silos to the detriment of large scale studies, cross-validation and data reuse. In some countries, study participants have the right to request their data, but that may require complex procedures. The archives of universities, institutions, and research labs are not meant as a backup for study participants.
Undoubtedly, access to data is essential to conduct data-driven research [57]. However, academic labs often lack resources to gather data on a large geographical scale. A remedy could be to conduct research in association with an industry partner to gain access to big data repositories. But, that introduces the risks of statistical bias since data collected by users of a single product brand, e.g. Apple Watch or Fitbit trackers, may not represent the general population. Another problem is that research projects that involve personal health data require ethical approvals. From our own experience, and that of others, the application process can be complex, time-consuming and not necessarily successful.
Clinical studies usually involved large cohorts (in the order of thousands) and some studies extend across 10–30 years. Conversely, the studies in the QoL Technologies Lab extend on average 6 months, involve small cohorts (20–30, sometimes up to 100 participants) and involve analytic systems for high-dimensional, high-resolution data (the outer layers of the ϕ spiral). Similarly, the studies based on smartwatches for health and wellness applications report promising solutions but most of them are only based on small sample sizes [58].
To sustain innovation in the QoL domain, we need data to conduct measurements covering physical health, psychological, social relationships and the environment. To the best of our knowledge, there are no such large vendor-independent data banks for data gathered with wearable devices, i.e., wearable data. Large biobanks have gather wearable data from large cohorts, but only for short periods, e.g., UK Biobank includes a repository of 1-week wearable data used by more than 100K participants. Other sources for research studies are community-based projects and crowdsourcing data. In this direction, we collaborate with the Open Humans (OH) Foundation. This non-profit organization built and support a community-based platform that collects personal data for personal exploration and research study participation [59]. OH has, as 30 of May of 2019, 6,976 members and 42% of them contribute or have contributed to a myriad of private and/or public databases. The most successful projects are related to genetic data. Through this collaboration, we could obtain data from approximately 400 activity trackers (OH’s Fitbit database). Some self-trackers have data from 2008; a pitfall is that OH’s Fitbit database only kept a single summary record per day. Crowdsourcing data is being tested in many areas, e.g., epidemiology studies, infection diseases, nutrition, and diabetes [60–63]. We hope that crowdsourcing mHealth systems may speed research and innovation with high-fidelity and reliable data.
To sum up, we identify two critical aspects for bringing data-driven research and innovation in the QoL domain: (1) increasing the user engagement to quantify and track QoL measurements; (2) facilitating data gathering and data sharing. In this paper, we provide some design guidelines for a system addressing these two key aspects.
Fig. 3.
The hierarchical value of health and health-related data.
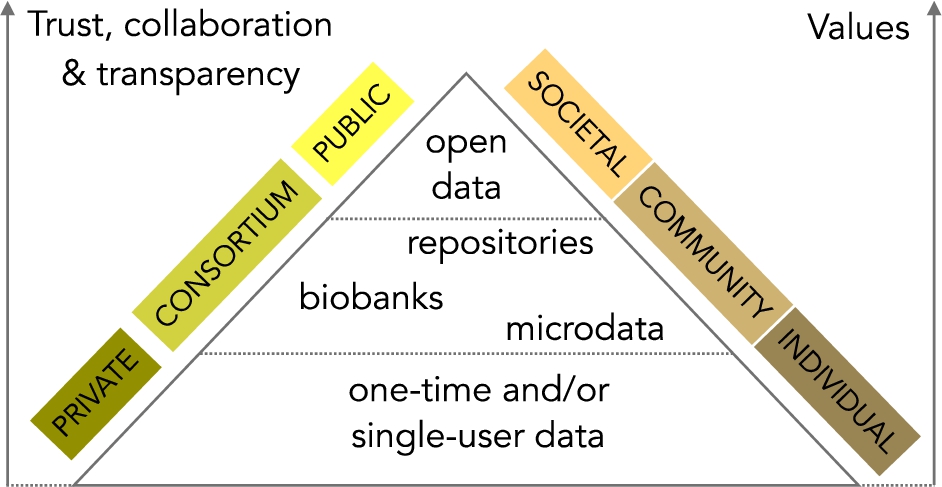
4.How: Individual, community and societal values
The value of personal data goes beyond the individual. There are countless examples of the benefits of personal data to communities and the society as a whole, but lack of transparency erodes trust and the willingness of individuals to share sensitive personal data such as health data [64]. Organizations with collaborative relationships that highly invest in trust can bring considerable innovation [65]. From what has been said above, we assign a hierarchical value to health and health-related data to classify systems in a pyramid of three non-exclusive categories, as illustrated in Fig. 3 and described as follows. Individual value is attainable by products or services designed for (a) one-time use, e.g., a particular clinical study conducted with the help of a mHealth app, or (b) single-user, e.g. commercial applications that satisfy the needs of individuals. Community value is possible when data is kept in well-curated institutional repositories, biobanks or public microdata collections. Temporal, economic, geographical and other restrictions may apply; consequently, benefits are subscribed to a group of individuals. Open, and ideally raw, data generate more opportunities to create societal value. Solutions that gain space in the upper part of this pyramid can bring social innovation since QoL measurements can have a significant impact in many fields that need creative solutions to meet social goals. Some of these fields are the rising incidence of long-term conditions, behavioural problems, happiness, rising life expectancy, and more examples given in the literature [66].
Certainly, the need for more trust, collaboration and transparency is a requirement to attain community and societal value. On the contrary, as we move towards the solutions located at the bottom of the pyramid, privacy prevails. However, high trust compensates for low privacy and vice versa [67]. In that study, the authors did not find any substantial decrease on self-disclosure of personal information in environments that combined high trust with weak privacy policies, or its counterpart, low trust with strong privacy policies. Then, it seems reasonable to think that organizations that design solutions to fit in all three pyramid levels may strive to increase the levels of trust, collaboration and transparency progressively. A pitfall is that the all-or-nothing approach that prevails in privacy policies found in mainstream products/services is not adequate to design innovative solutions for managing the broad diversity of personal data sources throughout a human lifetime and give back value to each level of the pyramid.
Modernising this area – with improved data access, sharing and long-term archiving methods – requires to consider multiple aspects. We reason upon five groups of issues to promote data access and sharing regimes identified by members of an Organization for Economic Cooperation and Development (OECD) follow-up working group [57]: (a) technological, (b) financial and budgetary, (c) legal and policy, (d) institutional and managerial, and, (e) cultural and behavioural factors. The rest of this section examines these aspects and place them in context with previous work and existent community and commercial solutions to manage and archive personal health-related data.
4.1.Technological issues
A system that collects data from many sources relies on the definition of data standards to implement interoperability solutions. The caveat is that the definition of data standards is affected by competition, overlapping goals, and poor coordination at national and international levels [68]. Particular to health standards, the Health Level Seven (HL7) specifications are widely used across the globe. HL7 specifications are designed for semantic interoperability, i.e., “the ability for data shared by systems to be understood at the level of fully defined domain concepts” [69]. Fast Healthcare Interoperability Resources (FHIR) is a draft HL7 standard describing data formats and elements and an application programming interface for exchanging electronic health records.
Consumer applications can use FHIR to import patient health data directly to mobile phones. There is a large ecosystem of apps that using free, open healthcare application programming interfaces (APIs) can connect to any EHR [70]. For instance, the Health app88 from Apple is a hub that integrates data from multiple devices and offers limited functionality for accessing electronic health record databases from partner providers. Researchers can make use of open development resources, e.g., Apple ResearchKit99 and Google Fit SDK,1010 to create data connectors to access EHR and TechO+ data from study participants. Hubs are single-points of data integration that include multiple aspects of life and facilitate data exploration.
Data exploration that goes beyond the default tools and features is available in the market. Knowledge sharing via community-driven and research-driven software seeks to reduce the burden of building ad hoc solutions. As examples, we can mention PACO1111 and QuantifyMe [71], both are open-source research frameworks that allow single-case self-experiments.
The development of personalised solutions requires expertise to implement data connectors that integrate data sources in own research hub. The creation of connectors is time consuming due to the myriad of consumer devices that are ingesting human data. Some of the data management platforms that provide data connectors to facilitate development are vendor-agnostic while others only provide connectors for a particular vendor, e.g., Fitabase.1212 A system that ingests data from multiple sources may need from ten to hundreds or thousands of connectors to provide real value to the large diversity of products and individual choice. Data connectors can be leased by paying a subscription fee to third-party vendors. Such fees could be expensive for non-profit projects or for individuals trying to keep a long-term personal data archive. Finally, data connectors may move data to a location that is not selected by the data owner.
Almost any data aggregation solution moves data to cloud-based infrastructure without giving the option to choose the storage location. The use of public cloud storage means that the provider has some control over the data. A claim to sovereignty is a claim of domain under control. Hence, embracing claims to sovereignty is crucial to assure data privacy in a trustless system. Blockchain, often in combination with off-chain data, has gained much attention to address self-sovereignty and manage healthcare data [72–74]. With the freedom to choose the storage location, individuals could opt for personal data vaults (PDV) [75], their proprietary storage media (such available space in local disk), a hybrid storage solution [76] or other communal/cooperative in-house storage systems. For example, a hybrid storage solution may combine media owned by the user and remote resources owned by peers organised in a decentralised architecture. Distribute medical data in different places would be a safer way to protect privacy and information; in fact, many countries have implemented decentralised systems that include data sharing and informed consents [77,78] many years before the boom of blockchain-based solutions. Communal/cooperative in-house storage systems require an upfront investment, but the cost could be afforded with a registration fee. On-premise solutions can keep the data where it should be, in the hands of the cooperative and, preferable, in the hands of the data subject only.
4.2.Financial and budgetary issues
Government incentives can help to speed up the adoption of new technologies. For instance, EHR adoption among US hospitals gained eight percentage points in the five years after the implementation of the Health Information Technology for Economic and Clinical Health Act (HITECH Act) [79]. The All of Us Research Program plans to build a database of 1 million US citizen profiles that incorporate lifestyle, environment and biologic factors. The program received $130 million from the US National Health Institute to accelerate precision medicine [80].
Worldwide, independent programs are run and financed cooperatively. A health cooperative model can be self-sustainable by reinvesting earnings from data but challenges remain [81]. Pilot studies based on the cooperative model have been built in different places, Swiss1313 and Dutch1414 initiatives promote new forms of citizen-driven data collection, and cooperatives at the local level and a federation of cooperatives at a global level [82]. Healthcare professionals and many individuals may, nonetheless, restrain from participation. In the late summer of 2010, an organization tried to build a health record bank (HRB) for a community in Phoenix, Arizona (USA). During the process, a national market research firm found that 20% of the survey respondents (1044 participants) were likely to pay $100–150 as an annual fee for a health record bank [83]. The initial aim was to engage 200K users (5% of the population), but the project was abandoned in April 2011 due to insufficient registrations.
Concerning data storage, a cost-effective model is needed, whether it is done by the individual or by a steward organization. The economics of long-term archives are different from models develop to store data that has a lifespan shorter than the life of the storage device [84]. Long-term data preservation has maintenance costs to avoid physical and logical obsolescence. Carefully chosen data types and formats can reduce migrations due to logical obsolescence. The selection of the storage medium, e.g., hard disk (HDD), solid-state device (SDD), tape, dictates physical replacement cycles. Tape storage [85] is a winner technology for archived data due to cost per Gigabyte and duration. However, tape is more susceptible to environmental damage; consequently, it may not be the best solution for individuals who wish to keep personal data at home.
For steward organizations, three main models for long-term preservation systems are: (a) rented storage in a public cloud, (b) monetised storage, and (c) endowed storage. In addition, developing markets for rented storage proliferate in P2P networks. Individuals may keep storage at home and share some resources with other individuals to build a P2P system with higher performance and reliability. Shared resources can be used to increase the performance and reliability of the system by storing redundant encrypted data. Sharing quotas can help to balance the equation costs for each user. But more research is needed to assess the cost-effectiveness of P2P-based solutions and their ability to provide long-term storage services. In a separate project, one of us is conducting experiments to further investigate P2P-based solutions. The monetised model is not a valid option neither for open data nor private data. While we do not suggest that individuals should not monetised data, this model is not compatible with our ultimate aim at designing a system that promotes open data for research and innovation. However, monetary incentives could be introduced for data generated during participatory studies as there is evidence that incentives are effective to improve the response rate in a survey [86]. In the endowment model, individuals pay endowed money that should cover the entire lifetime of data with some part used to pay the initial setup costs such as buying media and the rest is invested. Under the hypothesis that a system designed for long-term archiving of personal data will attract many users, endowed storage seems to be cost-effective in comparison with rented storage. Researchers showed that own private cloud is cost-effective with large clusters and that the cost of ownership of raw storage is almost 100× cheaper than the costs cloud storage providers service [87]. Other study highlighted that over the long term owning the infrastructure can save money [88]. Finally, researchers that studied the endowment model over a 100 years simulated archive founded that SSDs are a better choice media to keep the costs low [89].
4.3.Legal and policy issues
Regulations give a tool to public officers to control what companies are doing with data. Controls are only a first step, as stated by the Open Data Institute (ODI) in its guidance [90]: “Data-related activity can be unethical but still lawful.” Besides, regulations serve to accelerate innovation by providing government incentives, e.g. the case of personalised medicine in the US [91]. Although there are multiple cases of users showing autonomy and “wilfully sharing data”, there is limited protection to open data initiatives [92].
In the US, the Health Insurance Portability and Accountability Act (HIPAA) is the legal framework to protect patient’s privacy and guarantee that patients can have their data on request. It should be noticed that companies that provide services using health data may not be healthcare providers under the provisions of HIPAA; hence, HIPAA is not mandatory to those companies. For example, the website of Lydia, the suggested replacement for the Microsoft Health Vault service,1515 (last accessed 27 April 2019) says “The Service is not subject to HIPAA because our organization is not a healthcare provider”. Additionally, the US Affordable Care Act seeks to improve healthcare and encourage the use of technologies to increase patient engagement.
In Europe, individuals, so-called “data subjects”, are protected through the General Data Protection Regulation (GDPR) – (EU) 2016/679. GDPR imposes obligations to data controllers and data processors, among other protections, to defend the individual’s right to access, move and forget data.
The level of data protection legislation around the globe may differ from country to country.1616 Transborder data flow, sometimes cross-border data flow, is meant to protect data sovereignty and to avoid transferring data to countries that do not offer the same level of guarantees. For instance, taking in consideration that countries may take different approaches to protect data privacy, personal data transfers between the US, EU countries and Switzerland are protected by the Privacy Shield Framework.1717 GDPR is gaining global impact, and its core principles are evolving in a de-facto globalised standard as companies perceive that the adoption of a global compliant standard can save costs [93].
Compliance to regulations often means the obligation to obtain informed consent from users. But decades of raised concerns about the reading level of those privacy statements, which may be intelligible only to a minority or inadequate to vulnerable populations [94–96], suggest room for improvement. Individuals may give consent to gather data based on the expectations to address health concerns. In this context, tech/health giants keep exploiting business opportunities with closed data, although it may affect individuals who become exposed to privacy and security tensions in an undisclosed algorithmic decision-making world [15,97].
Data subjects are powerless with respect to data control and data process. The mere term “data subject” imposes a passive role to the individual that actually generates data. Data subjects are expected to give consent to others to act on their behalf. An active role requires participatory engagement. For that, we need systems that empower users and regulatory frameworks that act accordingly.
4.4.Institutional and managerial issues
In the governance of health data, trust in developers and regulators is a key condition to generate innovations in digital health [98]. Trust may be only generated with secure technologies and individuals with control [99]. Solutions that gather personal data without empowering the individual to take control may leave the data subject at the mercy of technology providers, researchers, healthcare providers, doctors, wellness centers, and others. All of them may have in place their systems to manage and store data from their patients/consumers, hence multiplying silos and points of attacks and vulnerabilities. Even if systems must comply with the law, there is still room for arbitrarily decisions in privacy and security policies, and for ambiguities that can lead to different interpretations affecting data subjects. For that reason, interpretative guidance and compliance with standards could become useful aids to organizations.
Transparency is closely related to trust. It helps the data subject to know how the data is processed, supporting the principle of accountability. A transparent system, which is now a GDPR requirement, needs to provide the data subject with the means to verify that the usage policies are being followed and that the business process complies with the policies consented by the user and the applicable data protection regulations [100]. Transparency suggests a socio-technical concept of global interest. Transparency Enhancing Tools (TETs) can help to accomplish transparency, at least as a technical principle [101].
The FAIR principles were designed to ensure transparency, reproducibility and reusability of scientific data [102]. FAIR stands for findability, accessibility, interoperability, and reusability. These principles are described as guidance to support proper data management and stewardship As an example, in the Netherlands, the personal health train initiative promotes the use of FAIR principles and citizen-controlled health data lockers [103]. Trains are a metaphor for data workflows, each responding to specific research questions, built and maintained by a researcher.
4.5.Cultural and behavioural factors
The OECD working group conclude in their study [57] that reward structures for those who produce and those who manage research data are a necessary component for promoting data access and sharing practices. In the context of our study, self-tracking cultures [104] are sustained by selfhood, i.e., the motto “self-knowledge through numbers”, and communities. Online communities of practice promote the engagement of healthy individuals and patients in their health care [105]. They are vehicles for sharing their personal self-tracking experiences which other members. The Quantified Self community1818 is a global movement of individuals and developers who share an interest in self-tracking tools. The Open Humans community1919 is a diverse community of people who want to learn more about themselves by forging meaning from personal data. It is governed democratically, and its board of directors include a seat elected by the community. Both communities have intersections in the private and communal self-tracking mode. But other cultures have emerged, these are pushed, imposed and exploited self-tracking [106]. Another issue is that online communities may disappear, altogether with the knowledge database and the networks created. We have surveyed the communities listed by Swan [9] in 2008, and almost half of the links do not work. Some of the disappeared communities had info about hundreds of health conditions and hundreds of groups.
Self-tracking practices may bring into effect the values of autonomy, solidarity, and authenticity. In practice, these values are enacted differently, and consequently, researchers have begun to ask what type of practices will guarantee these values in meaningful ways to individuals and communities [107].
5.Where: Towards an open health archive (OHA)
Long-lasting designed solutions that encompass a holistic view of human data can help to overcome the lack of persistent solutions and fragmented databases. A large number of platforms can be used to collect, explore and sharing personal data; surveying all of them is outside the scope of this work. Table 2 lists some examples to show the diversity of approaches in this field. The projects are sustained via three main models: non-profit/community, commercial projects and cooperatives. They target different audiences: end-users, as well as developers, healthcare partners and researchers. The table includes a column for a perceived value that indicates, when available, the number of members. The numbers may be imprecise due to artificially inflating the number of Twitter followers [108] or fabricated download figures to make the app seem more popular [109].
Table 2
Examples of existent solutions that can be used to collect, explore and share data
Solution | Twitter account | Website | Origin – Year | Operational model | Architecture | Data sources | Description | Current state of the evidence of value |
PatientsLikeMe @patientslikeme https://www.patientslikeme.com US – 2004 | Comercial, raised $127M | Centralised | Disease conditions and treatments | Members can see in a global network what treatments other members are trying and discuss health issues in an open environment. Anything that a member shares in the profile is visible to other members. Members can ask questions in a forum and get answers from the community. Data is shared with community, employees, partners and vendors. | 700K members, 31.5K Twitter followers |
Microsoft Health Vault @HealthVault https://www.healthvault.com US – 2007 | Commercial | Centralised (cloud) | Health records, health apps, personal health and fitness devices | Consumers can use the service to gather, store, use and share data of themselves, children and other family members. Partners: hospitals, pharmacies and lab testing companies. It is limited to some countries and languages. It will be shut down as of November 20, 2019. Consumers can have data migrated to Lydia. | 9K Twitter followers, 19 apps, 244 devices connections |
Digi.me @digime https://digi.me UK – 2009* | Commercial, raised $10.6M, transaction fees paid by companies for getting/giving data in user app | Distributed. Cloud options: Google Drive, Ms OneDrive, Dropbox | Social, medical, financial, health, fitness, music, entertainment | It allows users to retrieve copies of data from different services and stored in a user-centric cloud repository. It offers an ecosystem that permits sharing data privately with other apps. It offers enterprises a way to give back data to customers. It offers developers 1000s data sources (mostly from banks) and health records from US, UK and Iceland. It claims not to touch, hold or see data. To archive data it requires daily user intervention. | 5.6K Twitter followers, 400K users in 2017 |
HealthBank @healthbankcoop https://www.healthbank.coop CH – 2013 | For-profit, cooperative member minimum fee CHF 100, researchers pay for data, raised CHF 6.3M | Servers in Switzerland. Blockchain for the market model | Currently a platform with basic functions | Users can use the app for free. They can share data with doctors and others. Users receive internal token for sharing data. No feature to download data in a single operation. If user gave consent, data can be donated after death for research purposes. Before closure of the account users will have the possibility to donate health data. Individuals with cooperative member share can vote. The old platform is no longer valid, users are requested to migrate data to platform 2.0. | 200K users, 2.6K Twitter followers |
Open Humans @OpenHumansOrg https://www.openhumans.org US – 2015 | Non-profit, community project. Storage is free for users | Centralised storage (US Amazon cloud). User-centric data exploration | Genomes, physical activity, sleep, music, health, google search, etc. | This project aims at providing a path to share data, such as genetic, activity, or social media data, with researchers. Community members can contribute to more data connectors. Users can store data privately or opt to contribute data to projects proposed by members. Connection with Jupyter notebooks for data exploration. Website and other tools are open source. Project membership is public information. | 6.8K members, 1K Twitter followers, 30 active projects, 61 open-source repositories |
Wolfram Data Drop @WolframDataDrop https://datadrop.wolframcloud.com US – 2015 | Commercial | Centralised (cloud) | Web-API, Twitter, Arduino, Raspberry PI, Email, IFTTT, Web Form, etc | Data collection from multiple sources in databins for exploration using Wolfram computational resources. Wolfram Data Drop Starter is free for low-frequency and low-size data with databins expiring in 30 days. Subscriptions are available for more intense use. Data from expired databins is deleted. Users can administer the databin by assigning its creator, owner, and one or more administrators and grant permissions. | 400+ data types and 6,500+ measured quantities, Wolfram Notebooks for data exploration |
MIDATA @midata_coop https://www.midata.coop CH – 2015 | Cooperative, funded via paid research trials | Redundant servers hosted in Switzerland. FHIR API interoperability | Health records, medication, images (MRI) | MIDATA enables users to gather all health-relevant and personal data in a single repository. Subsets of data can be shared with friends, physicians or researchers. Developed at ETH Zurich and University of Bern (Switzerland). Users can share but do not sell data. Users can request to have their data automatically deposited in their account (e.g. via Apple Healthkit) [110]. Partnerships with EU countries/cities and low and middle income countries. | 0.3K Twitter followers, tracking pollen allergies app, sense app |
Dat protocol @dat_project https://datproject.org US – 2017 | Non-profit community project, in 2017 received five grants $1.48M | Decentralised | Not applicable | Data sharing protocol supported by a decentralised architecture. Its initial goal was to archive and share scientific data, although the project is evolving and expanding but without specific focus on QoL domain. It makes possible to store and sharing data in a decentralised way. It targets developers. | 9.3K Twitter followers, 46 open-source repositories |
* In 2017 Personal merged with Digi.me
K = 1000
M = 1,000,000
Raised funding information derived from https://www.crunchbase.com
From the perspective of a researcher it is important to know how many members are active, the number of days/months/years contributed, the number of data points registered, the variables, and if the platform has multiple projects, it is essential to know which users join which project. Researchers need all this information to judge possible partnerships for their studies. PatientsLikeMe has the largest number of users and presents open statistics about the members living conditions, e.g., the number of patients with certain symptoms, or a chart with age/sex of patients with liver cancer. Open Humans is open with respect to membership information and provides resources to citizen scientist and research institutions. It has a smaller database more focused on TechO+ and genome data.
From the perspective of individuals, it is almost impossible to evaluate an app without signing consent and give away personal data. Further, user needs may change throughout life and to choose the best solution for that particular moment in time, better information is needed. Migrating data from one solution to another may not be possible, or at least easy, and concerns about data loss may make users dependent on the same product. Open Humans and Wolfram Data Drop seem to offer the most flexible solution. The platforms offer a way to aggregate data from multiple sources and then explore all together with the help of powerful and stable resources such Jupyter Notebooks and Wolfram Notebooks. Overall, it is difficult to judge the value yield across the individual-community-society spectrum by the eight solutions. Some are more focused on individual values; some include interesting features for particular communities, etc.
The solutions listed in Table 2 may disappear. Product discontinuity, interoperability issues and geographical limitations are typical. For example, Google Health launched in May 2008 and was dissolved in January 2012 based on the lack of broad impact and Microsoft Health Vault will shutdown this year. Instead, Microsoft launched early this year Microsoft Health Bot, a cloud service based on AI algorithms, to take medical data from trusted sources at the conversational level. Medical data includes conditions, symptoms, specialists, medications and procedures.
There is room for improvements in the management of personal health information to reduce human data bleeding and disappearing communities built in dead applications. We believe that the lack of stable infrastructures to systematically collect data throughout all stages of human life penalize research and innovation. This section outlines the requirements and design choices to set the foundations for the creation of a platform, called Open Health Archive (OHA), that we derived based on the analysis of past experiences and initiatives as presented above and Sections 4 and 3.
The main design principle aims at helping individuals with the preservation of health-related data generated throughout life in a user-controlled and open infrastructure that together protect private, shared and public data. We argue that in order to have control over personal data collections, individuals should participate in the system administration and, ideally, have physical access to the storage devices that hold their data repositories. A user-controlled and open infrastructure built with Free/Libre and Open Source Software (FLOSS) can provide more control and more options to customize solutions to individual specific health and life conditions. Furthermore, FLOSS enjoys transparency and other characteristics that facilitate transdisciplinary research dialog [111].
Our vision for OHA is to emphasize the creation of value in a pyramid structure that benefits the individual, communities and society. To generate sustainable benefits to communities and societies, we propose a bottom-up innovation that emerges from empowered individuals and transparent management of personal data. Little has been done to provide individuals with tools and resources that empower them with independence and self-sufficiency, in other words, to become more active with their health-related data. In this regard, a case study on the Camfield Estates-MIT project that gives thought to community and individual empowerment point out that information and a sense of control are instrumental in participatory behavior [112]. FLOSS is a key factor in individual and community empowerment since everyone can contribute and customize tools to help individuals in longitudinal self-quantifying own experiments. That may help to achieve low participation attrition in future studies conducted inside OHA. OHA will allow gradual openness to protect and respect the privacy according to individual preferences while making easier the path to share data whenever the data subject decides it, including consenting options for posthumous donations. We discuss strategies that can create value such as the creation of a knowledge base and pseudo-anonymous profiles for each of the core QoL domains.
We envision a self-regulated system that shares computer resources in a decentralised storage system, assures patient/consumer-centric data management administration, and provides for each user a monolithic view of their life-tracking log. Developers could offer services or applications running on top of OHA but, at least, the OHA system’s core should be sustained by an open-source project. In this paper, we put more emphasis on the two outermost sections of the spiral, PGHD and TechO+ data. We assume that the data subject has already some control over these data collections, for example, access to fitness data. The ultimate mission is to preserve all type of personal health information. However, accessing medical records and stored them under the data subject realm is a complex issue with resistance and concerns, which fall outside the scope of this work.
The platform should not become a vehicle to push, impose and exploit self-tracking. Most of the data collections kept in the platform may remain private. Data is stored privately by default; gradually open data will be an option. Individuals may opt to participate in research studies and donate data to communities.
Building a cooperative, self-organized health archive system is a complex and long-term endeavour. We suggest that the initiative to create OHA must be taken by a non-profit organization that acts in the best interest of its members and adopts conflict of interests policies to assure that OHA remains open and has a transparent development and administration. This section elaborates more about our vision and outlines what can be the initial OHA requirements.
Fig. 4.
Overview of the open health archive (OHA).
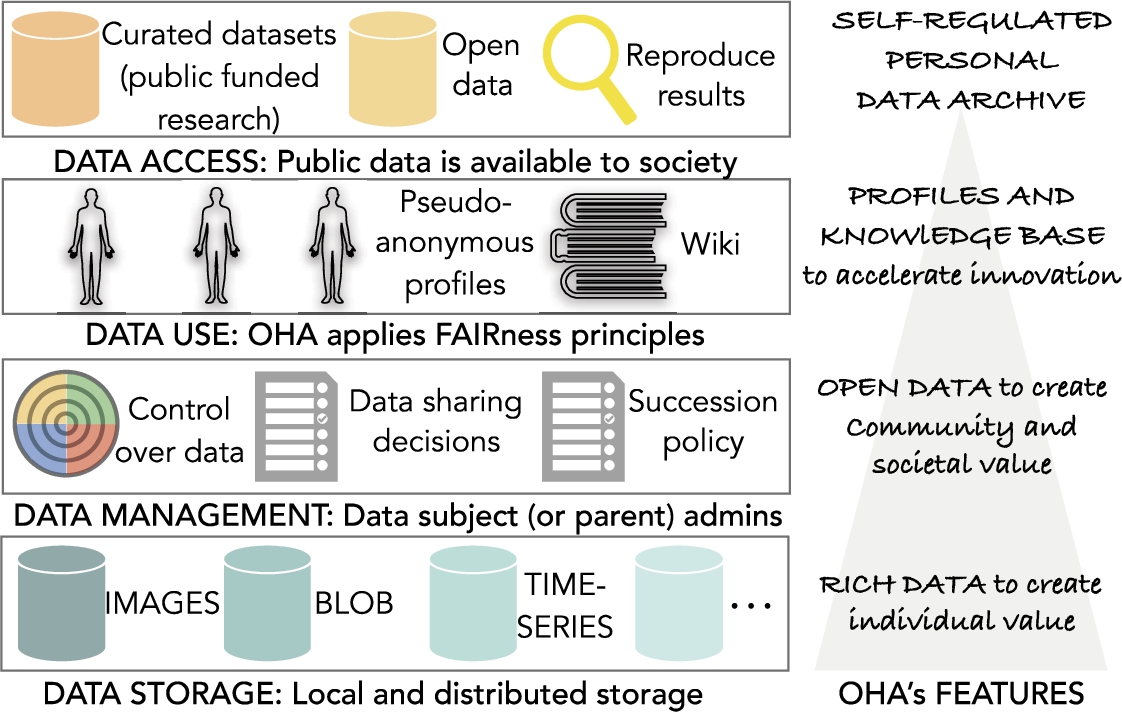
5.1.OHA: Preliminary specifications
At this stage of the research, our specifications are necessarily speculative since OHA is not yet implemented. However, given the crescent attention to health data governance and stewardship, this subsection presents some of the novel aspects of our design. We propose and examine the following OHA’s core features; an overview diagram is shown in Fig. 4.
5.1.1.Data storage
Shared and public/open datasets, namely curated datasets, will be supported economically by independent non-profit foundations, institutional repositories, and biobanks. Other datasets will reside in a community-based database supported by a decentralized infrastructure where individuals share resources such as storage and bandwidth for the benefit of the commons.
OHA moves the focus of attention from big data and features rich data. Rich data is constructed by aggregate inputs from different sources (or connectors) that create personal health information. Gathering data from different sources is possible via the integration of open standards, e.g., open mHealth standards [113]. Data sources include time-series, images, and other data formats that may require different repositories. These repositories will be kept in a hybrid infrastructure that combines local storage owned by the user and distributed storage shared or leased to the user. The sole use of traditional cloud storage service is discouraged to avoid single points of failure, expensive long-term storage costs and privacy threats often associated with central administrations.
These rich data repositories per individual give the possibility to design a monolith life-tracking log. The log will enable time travel visualizations through lifestyle patterns. Thus, the individual can monitor themselves their quality of life, and provided that they have the right instruments designed by experts in the field, visualize the correlations between different factors that may impact on their health.
5.1.2.Data management
OHA allows gradual openness to promote data sharing and open data. Users may opt to share partial information with formal (i.e., medical experts) and informal caregivers, specific community groups or with the whole society. Sharing can be done at any time (for example, information can be shared after the appearance of a disease), including automatically after subject death with previous consent. When records are inserted in open datasets, in the form of aggregated records, it is not technically feasible to delete them. However, those records do not contain information that can be associated with an individual. However, shared datasets should comply with state-of-the-art data protection requirements like GDPR.
OHA enables learning from patients with similar health concerns and learning from individuals with similar lifestyle patterns. It may be possible to conduct performance tests, e.g., 6-minute walk test, and compare results with previous personal measurements, community members or standard references.
To protect open data, OHA adopts a small number of possible licenses to donate content. End-users may opt among standards such Open Data Commons Public Domain Dedication and License (PDDL), Open Data Commons Attribution License (ODC-by), Public Domain Dedication (CCZero or CC0), or Community Data License Agreement (CDLA). Also, succession policies are put in place so that users state clear instructions regarding data deletion or posthumous data donation to family members and health research institutions. Decisions are modifiable at any time by the data subject. Succession process starts with a valid death certificate.
OHA collects data that (optionally) spans the whole life of a human (suggested 100-year data retention). Parents or guardians are named data administrators until the subject achieves legal adult age.
5.1.3.Data use
OHA is a computational stakeholder powered by algorithms developed by the community to help humans with the management and archival of personal health information. Non-experts are guided to gather more reliable and reusable data for investigations. Mechanisms to discover public data are put in place. Towards that goal, data stewardship follows good practices for scientific data management such the FAIR Guiding Principles, a recently published recommendation to “support knowledge discovery and innovation” [102]. FAIRness data means that data and metadata are findable, accessible, interoperable, and reusable. However, data is stored privately by default and sharing is not mandatory in OHA. Detailed analysis of FAIR data access in the cases of shared and open data is postponed for future work.
OHA repositories are a source to generate statistical knowledge using validated instruments such as WHOQOL-BREF 36 self-questionnaire. Users may participate in surveys to contribute to a large-scale statistical knowledge base and get feedback without fears of hidden data sharing policies. Optionally, this information is included in a pseudo-anonymous vector profile that is used by OHA’s knowledge discovery mechanisms to connect people with compatible needs, e.g. a patient with type II diabetes and a researcher studying the effects of depression on patients with diabetes type II.
5.1.4.Data access
Researchers who are granted total or partial access to private monolith logs will be able to conduct retrospective or prospective studies that take into consideration QoL dynamics and multidimensions. Participants may expect to receive economic incentives or other benefits from sharing data.
In addition, researchers will have access to a large-scale repository built with donated data. Then, multiple scientists can reproduce scientific findings based on the open repository. OHA will stimulate investigations based on the open repository to accelerate health innovation.
6.Discussion
Solutions are starting to emerge with alternatives to give people control over their personal data. Though personal data management still suffers from ambiguities or undefined policies. Who is the data owner [114]? Who is the data holder? On the contrary, answers to these two questions are evident in the domain of personal computers. Users can collect, explore, organize and share documents using the file system installed in their personal computers. They can decide whether the documents are stored locally or in the cloud. But file systems are not designed to receive input from the myriad of devices that generate TechO+ data. If personal computers empower individuals, systems or platforms designed to handle TechO+ data empower individuals too. We expect that next-generation systems will let consumers decide where TechO+ personal data should be stored. In the current status-quo, most app vendors store personal data in the cloud, usually in the US, with consequences in privacy, sovereignty and quality of service (latency) [115–117]. The same is true with IoT and smart devices, which could synchronize data directly with local computers, but instead send to cloud repositories and, only after that, the user may exercise the right to download data. The following questions remain: If users have full control of their personal data repositories, will they need to sign others consent policy terms? Instead, will users have the right to define their own consent policies when they share data? The initiative for democratizing data access via cooperatives can provide new models for informed consent policies where the individuals gain growing recognition of their rights [118].
7.Concluding remarks
Health and health-related data are being jeopardised by patient portals, mHealth apps and non-medical IoT devices that populate the market but with high probability disappear in a short time. Common pitfalls are lack of transparency and a shortage of simple procedures to move/share data into systems controlled by the data subject. Databases that are fragmented and hidden between walls hinder coordination among all healthcare actors and discourage individuals. The focus on big data ignores the fact that individuals are sources of rich data. Much of these rich data is lost. As a result, individuals may become less engaged in improving their health and well-being. To address these challenges, we propose to consider the individual-community-society spectrum and provide the requirements for OHA, a FLOSS platform that collects, explores and shares personal data to speed up research and innovation on quality of life.
Notes
3 We prefer the abbreviated form ϕ instead of “PHI” to avoid the confusion with the term “protected health information” used in the context of US HIPAA regulation. PHI refers to 18 identifiers, typically numbers and addresses that HIPAA distinguishes to protect individual’s privacy from re-identification.
Acknowledgements
We thank Bastian Greshake Tzovaras and Mad Price Ball from Open Humans Foundation for sharing valuable insights and expertise that improved this work although they may not agree with all the interpretations provided in this paper.
References
[1] | M. Huber, J.A. Knottnerus, L. Green, H. van der Horst, A.R. Jadad, D. Kromhout, B. Leonard, K. Lorig, M.I. Loureiro, J.W. van der Meer et al., How should we define health?, BMJ: British Medical Journal 343: ((2011) ), d4163. doi:10.1136/bmj.d4163. |
[2] | M. de Wit and T. Hajos, Health-related quality of life, in: Encyclopedia of Behavioral Medicine, (2013) , pp. 929–931. doi:10.1007/978-1-4419-1005-9_753. |
[3] | E. Kalaitzakis, Quality of life in liver cirrhosis, in: Handbook of Disease Burdens and Quality of Life Measures, (2010) , pp. 2239–2254. doi:10.1007/978-0-387-78665-0_131. |
[4] | M. de Wit and T. Hajos, Quality of life, in: Encyclopedia of Behavioral Medicine, M.D. Gellman and J.R. Turner, eds, Springer, New York, (2013) , pp. 1602–1603. ISBN 978-1-4419-1005-9. doi:10.1007/978-1-4419-1005-9_1196. |
[5] | D. Felce and J. Perry, Quality of life: Its definition and measurement, Research in Developmental Disabilities 16: (1) ((1995) ), 51–74. doi:10.1016/0891-4222(94)00028-8. |
[6] | W.H. Organization, WHOQOL: Measuring quality of life. https://web.archive.org/web/20180715074316/http://www.who.int/healthinfo/survey/whoqol-qualityoflife/en/index3.html. |
[7] | G. Caggianese, M. Calabrese, V. De Maio, G. De Pietro, A. Faggiano, L. Gallo, G. Sannino and C. Vecchione, A rehabilitation system for post-operative heart surgery, in: International Conference on Intelligent Interactive Multimedia Systems and Services, Springer, (2017) , pp. 554–564. doi:10.1007/978-3-319-59480-4_55. |
[8] | Y.R. Park, Y. Lee, J.Y. Kim, J. Kim, H.R. Kim, Y.-H. Kim, W.S. Kim and J.-H. Lee, Managing patient-generated health data through mobile personal health records: Analysis of usage data, JMIR mHealth and uHealth 6: (4) ((2018) ), e89. doi:10.2196/mhealth.9620. |
[9] | M. Swan, Emerging patient-driven health care models: An examination of health social networks, consumer personalized medicine and quantified self-tracking, International Journal of Environmental Research and Public Health 6: (2) ((2009) ), 492–525. doi:10.3390/ijerph6020492. |
[10] | G.K. Garge, C. Balakrishna and S.K. Datta, Consumer health care: Current trends in consumer health monitoring, IEEE Consumer Electronics Magazine 7: (1) ((2018) ), 38–46. doi:10.1109/MCE.2017.2743238. |
[11] | A. Bodaghi, A novel pervasive computing method to enhance efficiency of walking activity, Health and Technology 6: (4) ((2016) ), 269–276. doi:10.1007/s12553-016-0138-2. |
[12] | J. Casselman, N. Onopa and L. Khansa, Wearable healthcare: Lessons from the past and a peek into the future, Telematics and Informatics 34: (7) ((2017) ), 1011–1023. doi:10.1016/j.tele.2017.04.011. |
[13] | J. Lopes, R. Souza, C. Geyer, A. Souza, P. Davet, A. Pernas and A. Yamin, A situation-aware pervasive approach for assessing therapeutic goals in healthcare environment, in: Proceedings of the 31st Annual ACM Symposium on Applied Computing, ACM, (2016) , pp. 125–130. doi:10.1145/2851613.2851684. |
[14] | C. Orwat, A. Graefe and T. Faulwasser, Towards pervasive computing in health care – A literature review, BMC Medical Informatics and Decision Making 8: (1) ((2008) ), 26. doi:10.1186/1472-6947-8-26. |
[15] | S. Newell and M. Marabelli, Strategic opportunities (and challenges) of algorithmic decision-making: A call for action on the long-term societal effects of ‘datification’, Journal of Strategic Information Systems 24: (1) ((2015) ), 3–14. doi:10.1016/j.jsis.2015.02.001. |
[16] | S. Spiekermann, A. Acquisti, R. Böhme and K.-L. Hui, The challenges of personal data markets and privacy, Electronic Markets 25: (2) ((2015) ), 161–167. doi:10.1007/s12525-015-0191-0. |
[17] | IDC, IDC forecasts shipments of wearable devices to nearly double by 2021 as smart watches and new product categories gain traction, 2017. https://web.archive.org/web/20180525103053/https://www.idc.com/getdoc.jsp?containerId=prUS43408517. |
[18] | EMC, The digital universe: Driving data growth in healthcare, 2014. https://web.archive.org/web/20180525094214/https://www.emc.com/analyst-report/digital-universe-healthcare-vertical-report-ar.pdf. |
[19] | V. Estrada-Galinanes and K. Wac, Visions and challenges in managing and preserving data to measure quality of life, in: 2018 IEEE 3rd International Workshops on Foundations and Applications of Self* Systems (FAS* W), IEEE, (2018) , pp. 92–99. doi:10.1109/FAS-W.2018.00031. |
[20] | J. Kvedar, M.J. Coye and W. Everett, Connected health: A review of technologies and strategies to improve patient care with telemedicine and telehealth, Health Affairs 33: (2) ((2014) ), 194–199. doi:10.1377/hlthaff.2013.0992. |
[21] | R.V. Milani, R.M. Bober and C.J. Lavie, The role of technology in chronic disease care, Progress in Cardiovascular Diseases 58: (6) ((2016) ), 579–583. doi:10.1016/j.pcad.2016.01.001. |
[22] | J.A. Naslund, K.A. Aschbrenner and S.J. Bartels, Wearable devices and smartphones for activity tracking among people with serious mental illness, Mental Health and Physical Activity 10: ((2016) ), 10–17. doi:10.1016/j.mhpa.2016.02.001. |
[23] | J.A. Naslund, L.A. Marsch, G.J. McHugo and S.J. Bartels, Emerging mHealth and eHealth interventions for serious mental illness: A review of the literature, Journal of Mental Health 24: (5) ((2015) ), 321–332. doi:10.3109/09638237.2015.1019054. |
[24] | M. Schootman, E.J. Nelson, K. Werner, E. Shacham, M. Elliott, K. Ratnapradipa, M. Lian and A. McVay, Emerging technologies to measure neighborhood conditions in public health: Implications for interventions and next steps, International Journal of Health Geographics 15: (1) ((2016) ), 20. doi:10.1186/s12942-016-0050-z. |
[25] | P.H. Wise, Emerging technologies and their impact on disability, The Future of Children 22: ((2012) ), 169–191. https://www.jstor.org/stable/41475651. |
[26] | D.L. Patrick and R.A. Deyo, Generic and disease-specific measures in assessing health status and quality of life, Medical Care 27: ((1989) ), S217–S232. https://www.jstor.org/stable/3765666. |
[27] | M.E. Tinetti, A.D. Naik and J.A. Dodson, Moving from disease-centered to patient goals-directed care for patients with multiple chronic conditions: Patient value-based care, JAMA Cardiology 1: (1) ((2016) ), 9–10. doi:10.1001/jamacardio.2015.0248. |
[28] | N.E. Mayo, S. Figueiredo, S. Ahmed and S.J. Bartlett, Montreal accord on patient-reported outcomes (PROs) use series – Paper 2: Terminology proposed to measure what matters in health, Journal of Clinical Epidemiology 89: ((2017) ), 119–124. doi:10.1016/j.jclinepi.2017.04.013. |
[29] | C. Gurrin, A.F. Smeaton, A.R. Doherty et al., Lifelogging: Personal big data, Foundations and Trends® in Information Retrieval 8: (1) ((2014) ), 1–125. doi:10.1561/1500000033. |
[30] | P.J. Allison, D. Locker and J.S. Feine, Quality of life: A dynamic construct, Social Science & Medicine 45: (2) ((1997) ), 221–230. doi:10.1016/S0277-9536(96)00339-5. |
[31] | D.R. Evans, Enhancing quality of life in the population at large, Social Indicators Research 33: (1–3) ((1994) ), 47–88. doi:10.1007/BF01078958. |
[32] | T. Arroyo-Gallego, M.J. Ledesma-Carbayo, I. Butterworth, M. Matarazzo, P. Montero-Escribano, V. Puertas-Martín, M.L. Gray, L. Giancardo and Á. Sánchez-Ferro, Detecting motor impairment in early Parkinson’s disease via natural typing interaction with keyboards: Validation of the neuroQWERTY approach in an uncontrolled at-home setting, Journal of Medical Internet Research 20: (3) ((2018) ), e89. doi:10.2196/jmir.9462. |
[33] | A. Scheck McAlearney, C.J. Sieck, T. Menser, D.M. Walker and T.R. Huerta, Information technology to support patient engagement: Where do we stand and where can we go?, Journal of the American Medical Informatics Association 24: (6) ((2017) ), 1088–1094. doi:10.1093/jamia/ocx043. |
[34] | K. Wilson and L. Khansa, Migrating to electronic health record systems: A comparative study between the United States and the United Kingdom, Health Policy 122: (11) ((2018) ), 1232–1239. doi:10.1016/j.healthpol.2018.08.013. |
[35] | K. Fu, Trustworthy medical device software, in: Public Health Effectiveness of the FDA 510(k) Clearance Process, (2011) . https://www.ncbi.nlm.nih.gov/books/NBK209656/. |
[36] | G. Martin, S. Ghafur, J. Kinross, C. Hankin and A. Darzi, WannaCry – A year on, BMJ: British Medical Journal 361: ((2018) ), k2381. doi:10.1136/bmj.k2381. |
[37] | E.A. Rowe, Sharing data, Iowa Law Rev. 104: ((2018) ), 287–323. doi:10.2139/ssrn.3141890. |
[38] | N. Amini, M. Sarrafzadeh, A. Vahdatpour and W. Xu, Accelerometer-based on-body sensor localization for health and medical monitoring applications, Pervasive and Mobile Computing 7: (6) ((2011) ), 746–760. doi:10.1016/j.pmcj.2011.09.002. |
[39] | B. Knowles, A. Smith-Renner, F. Poursabzi-Sangdeh, D. Lu and H. Alabi, Uncertainty in current and future health wearables, Communications of the ACM 61: (12) ((2018) ), 62–67. doi:10.1145/3199201. |
[40] | A.K. Yetisen, J.L. Martinez-Hurtado, B. Ünal, A. Khademhosseini and H. Butt, Wearables in medicine, Advanced Materials 30: (33) ((2018) ), 1706910. doi:10.1002/adma.201706910. |
[41] | H. Chen, R.H.L. Chiang and V.C. Storey, Business intelligence and analytics: From big data to big impact, MIS Quarterly 36: (4) ((2012) ), 1165–1188. doi:10.2307/41703503. |
[42] | H. Maier and A. Schreiber, Thoughts on the future of medical imaging, 2016. https://web.archive.org/web/20190425170356/https://www.bgmassociates.net/uploads/6/8/9/2/68925041/bgm_associates_-_futureofimaging_industryperspective.pdf. |
[43] | NetApp, The power of healthcare data – The body as a source of big data. https://web.archive.org/web/20180715174221/https://cdn.datafloq.com/cms/bds_20141120195421_The-Body-as-a-Source-of-Big-Data-Inforgraphic.jpg. |
[44] | Y. Wang, Y. Qiu, S.K. Ameri, H. Jang, Z. Dai, Y. Huang and N. Lu, Low-cost, μm-thick, tape-free electronic tattoo sensors with minimized motion and sweat artifacts, npj Flexible Electronics 2: (1) ((2018) ), 6. doi:10.1038/s41528-017-0019-4. |
[45] | M. Blount, M.R. Ebling, J.M. Eklund, A.G. James, C. McGregor, N. Percival, K.P. Smith and D. Sow, Real-time analysis for intensive care, IEEE Engineering in Medicine and Biology Magazine 29: (2) ((2010) ), 110–118. doi:10.1109/MEMB.2010.936454. |
[46] | S.A. Pettersen, D. Johansen, H. Johansen, V. Berg-Johansen, V.R. Gaddam, A. Mortensen, R. Langseth, C. Griwodz, H.K. Stensland and P. Halvorsen, Soccer video and player position dataset, in: Proceedings of the 5th ACM Multimedia Systems Conference, MMSys ’14, ACM, New York, (2014) , pp. 18–23. ISBN 978-1-4503-2705-3. doi:10.1145/2557642.2563677. |
[47] | M. Mori, W.L. Schulz, A. Geirsson and H.M. Krumholz, Tapping into underutilized healthcare data in clinical research, Annals of Surgery 270: (2) ((2019) ), 227–229. doi:10.1097/SLA.0000000000003329. |
[48] | G. Bell, Counting every heart beat: Observation of a quantified selfie, Technical Report MSR-TR-2015-53, 2015. https://www.microsoft.com/en-us/research/wp-content/uploads/2015/06/MSR-TR-2015-53-Collecting-Every-Heart-Beat.pdf. |
[49] | P. Krebs and D.T. Duncan, Health app use among US mobile phone owners: A national survey, JMIR mHealth and uHealth 3: (4) ((2015) ), e101. doi:10.2196/mhealth.4924. |
[50] | Y.-A. De Montjoye, C.A. Hidalgo, M. Verleysen and V.D. Blondel, Unique in the crowd: The privacy bounds of human mobility, Scientific Reports 3: ((2013) ), 1376. doi:10.1038/srep01376. |
[51] | Fitness tracking app Strava gives away location of secret US army bases, 2018. https://web.archive.org/web/20180612140709/https://www.theguardian.com/world/2018/jan/28/fitness-tracking-app-gives-away-location-of-secret-us-army-bases. |
[52] | L. Sweeney, k-anonymity: A model for protecting privacy, International Journal on Uncertainty, Fuzziness and Knowledge-Based Systems 10: (5) ((2002) ), 557–570. doi:10.1142/S0218488502001648. |
[53] | K. Wac, From quantified self to quality of life, in: Digital Health, H. Rivas and K. Wac, eds, Springer, Cham, (2018) , pp. 83–108. ISBN 978-3-319-61446-5. doi:10.1007/978-3-319-61446-5_7. |
[54] | T. Boillat, H. Rivas and K. Wac, “Healthcare on a wrist”: Increasing compliance through checklists on wearables in obesity (self-)management programs, in: Digital Health, H. Rivas and K. Wac, eds, Springer, Cham, (2018) , pp. 65–81. ISBN 978-3-319-61446-5. doi:10.1007/978-3-319-61446-5_6. |
[55] | K. Wac, A.K. Dey and A.V. Vasilakos, Body area networks for ambulatory psychophysiological monitoring: A survey of off-the-shelf sensor systems, in: Proceedings of the Fifth International Conference on Body Area Networks, BodyNets ’10, ACM, New York, (2010) , pp. 181–187. ISBN 978-1-4503-0029-2. doi:10.1145/2221924.2221959. |
[56] | C. Maher, J. Ryan, C. Ambrosi and S. Edney, Users’ experiences of wearable activity trackers: A cross-sectional study, BMC Public Health 17: (1) ((2017) ), 880. doi:10.1186/s12889-017-4888-1. |
[57] | P. Arzberger, P. Schroeder, A. Beaulieu, G. Bowker, K. Casey, L. Laaksonen, D. Moorman, P. Uhlir and P. Wouters, Promoting access to public research data for scientific, economic, and social development, Data Science Journal 3: ((2004) ), 135–152. doi:10.2481/dsj.3.135. |
[58] | B. Reeder and A. David, Health at hand: A systematic review of smart watch uses for health and wellness, Journal of Biomedical Informatics 63: ((2016) ), 269–276. doi:10.1016/j.jbi.2016.09.001. |
[59] | B. Greshake Tzovaras, M. Angrist, K. Arvai, M. Dulaney, V. Estrada-Galiñanes, B. Gunderson, T. Head, D. Lewis, O. Nov, O. Shaer et al., Open humans: A platform for participant-centered research and personal data exploration, GigaScience 8: (6) ((2019) ), giz076. doi:10.1093/gigascience/giz076. |
[60] | J.S. Brownstein, C.C. Freifeld, B.Y. Reis and K.D. Mandl, Surveillance sans frontieres: Internet-based emerging infectious disease intelligence and the HealthMap project, PLoS Medicine 5: (7) ((2008) ), e151. doi:10.1371/journal.pmed.0050151. |
[61] | E. Dunford, H. Trevena, C. Goodsell, K.H. Ng, J. Webster, A. Millis, S. Goldstein, O. Hugueniot and B. Neal, FoodSwitch: A mobile phone app to enable consumers to make healthier food choices and crowdsourcing of national food composition data, JMIR mHealth and uHealth 2: (3) ((2014) ), e37. doi:10.2196/mhealth.3230. |
[62] | C.C. Freifeld, R. Chunara, S.R. Mekaru, E.H. Chan, T. Kass-Hout, A.A. Iacucci and J.S. Brownstein, Participatory epidemiology: Use of mobile phones for community-based health reporting, PLoS Medicine 7: (12) ((2010) ), e1000376. doi:10.1371/journal.pmed.1000376. |
[63] | D. Paolotti, A. Carnahan, V. Colizza, K. Eames, J. Edmunds, G. Gomes, C. Koppeschaar, M. Rehn, R. Smallenburg, C. Turbelin et al., Web-based participatory surveillance of infectious diseases: The Influenzanet participatory surveillance experience, Clinical Microbiology and Infection 20: (1) ((2014) ), 17–21. doi:10.1111/1469-0691.12477. |
[64] | T. Morey, T. Forbath and A. Schoop, Customer data: Designing for transparency and trust, Harvard Business Review 93: (5) ((2015) ), 96–105. https://web.archive.org/web/20190428175024/https://hbr.org/2015/05/customer-data-designing-for-transparency-and-trust. |
[65] | R.A. Hattori and T. Lapidus, Collaboration, trust and innovative change, Journal of Change Management 4: (2) ((2004) ), 97–104. doi:10.1080/14697010320001549197. |
[66] | G. Mulgan, S. Tucker, R. Ali and B. Sanders, Social innovation: What it is, why it matters and how it can be accelerated, Skoll Centre for Social Entrepreneurship, 2007. https://web.archive.org/web/20130612102633/http://www.sbs.ox.ac.uk:80/centres/skoll/research/Documents/SocialInnovation.pdf. |
[67] | A.N. Joinson, U.-D. Reips, T. Buchanan and C.B.P. Schofield, Privacy, trust, and self-disclosure online, Human–Computer Interaction 25: ((2010) ), 1–24. doi:10.1080/07370020903586662. |
[68] | W.E. Hammond, The making and adoption of health data standards, Health Affairs 24: (5) ((2005) ), 1205–1213. doi:10.1377/hlthaff.24.5.1205. |
[69] | R.H. Dolin and L. Alschuler, Approaching semantic interoperability in health level seven, Journal of the American Medical Informatics Association 18: (1) ((2010) ), 99–103. doi:10.1136/jamia.2010.007864. |
[70] | K.D. Mandl, J.C. Mandel and I.S. Kohane, Driving innovation in health systems through an apps-based information economy, Cell Systems 1: (1) ((2015) ), 8–13. doi:10.1016/j.cels.2015.05.001. |
[71] | S. Taylor, A. Sano, C. Ferguson, A. Mohan and R.W. Picard, QuantifyMe: An open-source automated single-case experimental design platform, Sensors 18: (4) ((2018) ), 1097. doi:10.3390/s18041097. |
[72] | X. Liang, S. Shetty, J. Zhao, D. Bowden, D. Li and J. Liu, Towards decentralized accountability and self-sovereignty in healthcare systems, in: International Conference on Information and Communications Security, Springer, (2017) , pp. 387–398. doi:10.1007/978-3-319-89500-0_34. |
[73] | X. Liang, J. Zhao, S. Shetty, J. Liu and D. Li, Integrating blockchain for data sharing and collaboration in mobile healthcare applications, in: 2017 IEEE 28th Annual International Symposium on Personal, Indoor, and Mobile Radio Communications (PIMRC), IEEE, (2017) , pp. 1–5. doi:10.1109/PIMRC.2017.8292361. |
[74] | C. McFarlane, M. Beer, J. Brown and N. Prendergast, Patientory: A healthcare peer-to-peer EMR storage network v1.0, Entrust Inc., Addison, TX, USA, 2017. https://patientory.com. |
[75] | M.Y. Mun, D.H. Kim, K. Shilton, D. Estrin, M. Hansen and R. Govindan, PDVLoc: A personal data vault for controlled location data sharing, ACM Trans. Sen. Netw. 10: (4) ((2014) ), 58:1–58:29. http://doi.acm.org/10.1145/2523820. doi:10.1145/2523820. |
[76] | D. Tarrant, T. Brody and L. Carr, From the desktop to the cloud: Leveraging hybrid storage architectures in your repository, in: The 4th Annual International Open Repositories Conference (or09), (2009) . https://eprints.soton.ac.uk/id/eprint/267084. |
[77] | C.M. O’Keefe, P. Greenfield, A. Goodchild et al., A decentralised approach to electronic consent and health information access control, Journal of Research and Practice in Information Technology 37: (2) ((2005) ), 161–178. https://search.informit.com.au/documentSummary;dn=015439491208510;res=IELHSS. |
[78] | C. Quantin, G. Coatrieux, M. Fassa, V. Breton, D.-O. Jaquet-Chiffelle, P. De Vlieger, N. Lypszyc, J.-Y. Boire, C. Roux and F.-A. Allaert, Centralised versus decentralised management of patients’ medical records, in: MIE, (2009) , pp. 700–704. doi:10.3233/978-1-60750-044-5-700. |
[79] | J. Adler-Milstein and A.K. Jha, HITECH act drove large gains in hospital electronic health record adoption, Health Affairs 36: (8) ((2017) ), 1416–1422. doi:10.1377/hlthaff.2016.1651. |
[80] | G.S. Ginsburg and K.A. Phillips, Precision medicine: From science to value, Health Affairs 37: (5) ((2018) ), 694–701. doi:10.1377/hlthaff.2017.1624. |
[81] | I. van Roessel, M. Reumann and A. Brand, Potentials and challenges of the health data cooperative model, Public Health Genomics 20: ((2017) ), 321–331. doi:10.1159/000489994. |
[82] | E. Hafen, D. Kossmann and A. Brand, Health data cooperatives – Citizen empowerment, Methods of Information in Medicine 53: (2) ((2014) ), 82–86. doi:10.3414/ME13-02-0051. |
[83] | W.A. Yasnoff and E.H. Shortliffe, Lessons learned from a health record bank start-up, Methods of Information in Medicine 53: (2) ((2014) ), 66–72. doi:10.3414/ME13-02-0030. |
[84] | D.S. Rosenthal, D.C. Rosenthal, E.L. Miller, I.F. Adams, M.W. Storer and E. Zadok, The economics of long-term digital storage, 2012. https://www.crss.ucsc.edu/papers/rosenthal-unesco12.pdf. |
[85] | M. Lantz, Why the future of data storage is (still) magnetic tape, 2018. https://spectrum.ieee.org/computing/hardware/why-the-future-of-data-storage-is-still-magnetic-tape. |
[86] | S. Yu, H.E. Alper, A.-M. Nguyen, R.M. Brackbill, L. Turner, D.J. Walker, C.B. Maslow and K.C. Zweig, The effectiveness of a monetary incentive offer on survey response rates and response completeness in a longitudinal study, BMC Medical Research Methodology 17: (1) ((2017) ), 77. doi:10.1186/s12874-017-0353-1. |
[87] | I. Sadooghi, D. Zhao, T. Li and I. Raicu, Understanding the cost of cloud computing and storage, in: 1st Greater Chicago Area System Research Workshop, (2012) . http://citeseerx.ist.psu.edu/viewdoc/summary?doi=10.1.1.646.4673. |
[88] | C. Fisher, Cloud versus on-premise computing, American Journal of Industrial and Business Management 8: (9) ((2018) ), 1991–2006. doi:10.4236/ajibm.2018.89133. |
[89] | P. Gupta, A. Wildani, E.L. Miller, D. Rosenthal, I.F. Adams, C. Strong and A. Hospodor, An economic perspective of disk vs. flash media in archival storage, in: 2014 IEEE 22nd International Symposium on Modelling, Analysis & Simulation of Computer and Telecommunication Systems, IEEE, (2014) , pp. 249–254. doi:10.1109/MASCOTS.2014.39. |
[90] | Open Data Institute, ODI ethical data handling 2017-09-13, 2017. https://www.scribd.com/document/358778144/ODI-Ethical-Data-Handling-2017-09-13. |
[91] | F.R. Vogenberg, C.I. Barash and M. Pursel, Personalized medicine: Part 1: Evolution and development into theranostics, Pharmacy and Therapeutics 35: (10) ((2010) ), 560–576. 21037908. |
[92] | A. Karanasiou and S. Kang, My quantified self, my FitBit and I: The polymorphic concept of health data and the sharer’s dilemma, Digital Culture and Society 2: (1) ((2016) ), 123–142. doi:10.14361/dcs-2016-0109. |
[93] | M.L. Rustad and T.H. Koenig, Towards a global data privacy standard, Florida Law Review 71: ((2018) ), 365–453. https://ssrn.com/abstract=3239930. |
[94] | G. Das, C. Cheung, C. Nebeker, M. Bietz and C. Bloss, Privacy policies for apps targeted toward youth: Descriptive analysis of readability, JMIR mHealth and uHealth 6: (1) ((2018) ), e3. doi:10.2196/mhealth.7626. |
[95] | M.A. Graber, D.M. D’Alessandro and J. Johnson-West, Reading level of privacy policies on Internet health web sites, Journal of Family Practice 51: (7) ((2002) ), 642–645. 12160504. |
[96] | J.R. Ogloff and R.K. Otto, Are research participants truly informed? Readability of informed consent forms used in research, Ethics & Behavior 1: (4) ((1991) ), 239–252. doi:10.1207/s15327019eb0104_2. |
[97] | J.T. Wilbanks and E.J. Topol, Stop the privatization of health data, Nature News 535: (7612) ((2016) ), 345–348. doi:10.1038/535345a. |
[98] | E. Vayena, T. Haeusermann, A. Adjekum and A. Blasimme, Digital health: Meeting the ethical and policy challenges, Swiss Medical Weekly 148: ((2018) ), w14571. doi:10.3929/ethz-b-000239873. |
[99] | F. Ferretti, Data protection and the legitimate interest of data controllers: Much ado about nothing or the winter of rights?, Common Market Law Review 51: (3) ((2014) ), 843–868. https://web.archive.org/web/20190427161200/http://www.kluwerlawonline.com/abstract.php?area=Journals&id=COLA2014063. |
[100] | P. Bonatti, S. Kirrane, A. Polleres and R. Wenning, Transparent personal data processing: The road ahead, in: International Conference on Computer Safety, Reliability, and Security, Springer, (2017) , pp. 337–349. doi:10.1007/978-3-319-66284-8_28. |
[101] | D. Spagnuelo, A. Ferreira and G. Lenzini, Accomplishing transparency within the general data protection regulation, in: 5th International Conference on Information Systems Security and Privacy, (2018) . http://insticc.org/node/TechnicalProgram/icissp/presentationDetails/73665. |
[102] | M.D. Wilkinson, M. Dumontier, I.J. Aalbersberg, G. Appleton, M. Axton, A. Baak, N. Blomberg, J.-W. Boiten, L.B. da Silva Santos, P.E. Bourne et al., The FAIR guiding principles for scientific data management and stewardship, Scientific Data 3: ((2016) ), 160018. doi:10.1038/sdata.2016.18. |
[103] | W. Kraaij, Citizen controlled health data lockers as game changer, 2017. http://publications.tno.nl/publication/34625969/RhGMOU/TNO-2017-citizen.pdf. |
[104] | D. Lupton, Self-tracking cultures: Towards a sociology of personal informatics, in: Proceedings of the 26th Australian Computer–Human Interaction Conference on Designing Futures: The Future of Design, ACM, (2014) , pp. 77–86. doi:10.1145/2686612.2686623. |
[105] | G. Appelboom, M. LoPresti, J.-Y. Reginster, E.S. Connolly and E.P.L. Dumont, The quantified patient: A patient participatory culture, Current Medical Research and Opinion 30: (12) ((2014) ), 2585–2587. doi:10.1185/03007995.2014.954032. |
[106] | D. Lupton, Self-tracking modes: Reflexive self-monitoring and data practices, 2014. doi:10.2139/ssrn.2483549. |
[107] | T. Sharon, Self-tracking for health and the quantified self: Re-articulating autonomy, solidarity, and authenticity in an age of personalized healthcare, Philosophy & Technology 30: (1) ((2017) ), 93–121. doi:10.1007/s13347-016-0215-5. |
[108] | Y. Zhang and J. Lu, Discover millions of fake followers in Weibo, Social Network Analysis and Mining 6: (1) ((2016) ), 16. doi:10.1007/s13278-016-0324-2. |
[109] | H. Tu, Failed cases of mobile healthcare apps: Why QPG health is doomed to fail?, mHealth 1: ((2015) ), 8. doi:10.3978/j.issn.2306-9740.2015.03.06. |
[110] | E. Hafen, O.5.4.2.005: MIDATA cooperatives–citizen-controlled use of health data is a pre-requiste for big data analysis, economic success and a democratization of the personal data economy, Tropical Medicine & International Health 20: ((2015) ), 129. https://onlinelibrary.wiley.com/doi/epdf/10.1111/tmi.12575. |
[111] | G. Von Krogh and S. Spaeth, The open source software phenomenon: Characteristics that promote research, Journal of Strategic Information Systems 16: (3) ((2007) ), 236–253. doi:10.1016/j.jsis.2007.06.001. |
[112] | R. Pinkett and R. O’Bryant, Building community, empowerment and self-sufficiency, Information, Communication & Society 6: (2) ((2003) ), 187–210. doi:10.1080/1369118032000093888. |
[113] | D. Estrin and I. Sim, Open mHealth architecture: An engine for health care innovation, Science 330: (6005) ((2010) ), 759–760. doi:10.1126/science.1196187. |
[114] | P. Kostkova, H. Brewer, S. de Lusignan, E. Fottrell, B. Goldacre, G. Hart, P. Koczan, P. Knight, C. Marsolier, R.A. McKendry et al., Who owns the data? Open data for healthcare, Frontiers in Public Health 4: ((2016) ), 7. doi:10.3389/fpubh.2016.00007. |
[115] | Z.N. Peterson, M. Gondree and R. Beverly, A position paper on data sovereignty: The importance of geolocating data in the cloud, 2011. https://www.usenix.org/legacy/events/hotcloud11/tech/final_files/Peterson.pdf. |
[116] | D. Svantesson and R. Clarke, Privacy and consumer risks in cloud computing, Computer Law & Security Review 26: (4) ((2010) ), 391–397. doi:10.1016/j.clsr.2010.05.005. |
[117] | J. Xu, E.-C. Chang and J. Zhou, Weak leakage-resilient client-side deduplication of encrypted data in cloud storage, in: Proceedings of the 8th ACM SIGSAC Symposium on Information, Computer and Communications Security, ACM, (2013) , pp. 195–206. doi:10.1145/2484313.2484340. |
[118] | A. Blasimme, E. Vayena and E. Hafen, Democratizing health research through data cooperatives, Philosophy & Technology 31: (3) ((2018) ), 473–479. doi:10.1007/s13347-018-0320-8. |