Rho GTPase-activating protein 4 is upregulated in Kidney Renal Clear Cell Carcinoma and associated with poor prognosis and immune infiltration
Abstract
BACKGROUND:
Kidney Renal Clear Cell Carcinoma (KIRC) is a malignant tumor that seriously threatens human health. Rho GTPase-activating protein 4 (ARHGAP4) plays an important role in the occurrence and development of tumors.
OBJECTIVE:
The purpose of this study was to explore the role of ARHGAP4 in the progression of KIRC and its diagnostic and prognostic value.
METHODS:
Multiple analytical methods and in vitro cell assays were used to explore the expression of ARHGAP4 and its value in the progression, diagnosis and prognosis of KIRC. The biological function of ARHGAP4 was studied by GO analysis and KEGG pathway analysis, and then the relationship between ARHGAP4 and immune infiltration was analyzed.
RESULTS:
The expression of ARHGAP4 was significantly up-regulated in KIRC. We found that the high expression of ARHGAP4 was related to the progression of KIRC and suggested a poor prognosis. Compared with normal tissues, ARHGAP4 had a better diagnostic value in KIRC. The biological function of ARHGAP4 was related to immunity, and its expression was also closely related to tumor immune infiltration and immune checkpoints.
CONCLUSIONS:
Our study demonstrated that ARHGAP4 may be a biomarker, which is related to the progression, diagnosis and prognosis of KIRC. Its biological functions are related to tumor immune infiltration.
1.Introduction
Renal cell carcinoma (RCC) is one of the most common malignant tumors of the urinary system, with the highest incidence in North America, Australia and Europe and the lowest in India, Japan, Africa and China [1]. RCC is also a serious threat to human health. It is reported that the 5-year disease-specific survival rate of patients with RCC stage IV is less than 10% [2]. KIRC is the main subtype of RCC, with the highest incidence, accounting for about 80% of all RCCs. The symptoms of KIRC are hidden and appear late, about 25% of patients have metastases at the time of initial diagnosis [3, 4]. Nowadays, more and more treatments are used for RCC, including surgery, chemotherapy, radiotherapy and targeted therapy, which show a certain effect on the survival of RCC. However, compared with other types of RCC, KIRC has a poorer therapeutic effect and prognosis [5, 6]. In addition, the median survival time of metastatic KIRC was only about 10 months [7].
Immune response is closely related to the clinical outcome and prognosis of cancer. Different from most cancers, the increase of CD8 + T cells and neutrophils in KIRC are predictors of poor prognosis [8]. Tumor infiltrating immune cells (TIIC) play an important role in tumor microenvironment. In many studies, it has been proved that KIRC is significantly related to immune infiltration [9]. In recent years, immunotherapy, as a new treatment for RCC, is an effective supplement to surgery, radiotherapy and chemotherapy, especially in the treatment of metastatic RCC. However, it is estimated that only about 20% of RCC patients could achieve better results from immunotherapy. It is necessary to look for some predictive biomarkers of RCC to evaluate the potential response and prognosis of patients receiving immunotherapy [10, 11].
ARHGAP4 is a small GTP enzyme that can degrade activated GTP into inactivated GDP and negatively regulate Rho A protein. It not only plays an important role in inhibiting the movement of cells and axons, but also closely related to the occurrence and development of many kinds of tumors [12, 13]. It has been reported that ARHGAP4 can regulate the migration and invasion of pancreatic cancer cells, and its mechanism may be related to HDAC2/
We analyzed the mRNA expression level and protein expression level of ARHGAP4 in KIRC in TCGA, GEO and UALCAN databases. Then, the data of KIRC in TCGA were used to analyze the relationship between the expression of ARHGAP4 and clinical features and prognosis. In addition, we further studied the biological functions and pathways of differentially expressed genes related to ARHGAP4 by GO and KEGG analysis. Finally, we systematically evaluated the state of infiltrating immune cells and clarified the relationship between ARHGAP4 and KIRC immunity. Our study suggested that ARHGAP4 is associated with the progression and clinical outcome of KIRC. Furthermore, it could be a new prognostic marker related to immune infiltration in KIRC.
2.Materials and methods
2.1Data collection
The RNA sequencing data of 539 cases of KIRC and 72 cases of para-carcinoma tissues were obtained from TCGA database, as well as the clinicopathological data of the patients (https://portal.gdc.cancer.gov/). We converted the RNA sequencing data in FPKM format into transcript per million readings (TPM) format for further analysis. And when we carried on the classified statistical analysis, we discarded the data without clinical information.
In addition, we also obtained the RNA sequencing data of three data sets (GSE66270, GSE66271, GSE71963) from the GEO database (https://www.ncbi. nlm.nih.gov/geo/).
2.2Analysis of ARHGAP4 expression level in cancer and normal tissues
The mRNA expression of ARHGAP4 was analyzed by RNA sequencing data of 33 kinds of tumors and corresponding para-carcinoma tissues in TCGA database. Similarly, RNA sequencing data of GSE66270, GSE66271 and GSE71963 datasets were extracted from GEO database to verify the mRNA expression of ARHGAP4 in KIRC.
UALCAN database (http://ualcan.path.uab.edu/) is a free and open data analysis website, which has three main modules: TCGA analysis module, CPTAC analysis module and children’s brain tumor (CBTTC) analysis module. We used CPTAC analysis module to analyze the difference of ARHGAP4 expression at the protein level.
2.3Analysis of correlation between ARHGAP4 expression and clinicopathological characteristics in KIRC
According to the median of ARHGAP4 mRNA expression, 539 patients with KIRC were divided into high expression group and low expression group. The differences of clinicopathological parameters such as TNM stage, pathological stage, histological grade, overall survival time (OS), disease specific survival time (DSS) and disease progression-free interval (PFI) between ARHGAP4 high and low expression group were compared with R packages. Dunn’s multiple hypothesis test, Kruskal-Wallis Test and Shapiro-Wilk normality test were used to analyze the differences of pathological parameters between the two groups. We used Logistic regression analysis to study the correlation between the expression level of ARHGAP4 and the clinicopathological features of KIRC.
2.4Analysis of ARHGAP4 expression and its diagnostic and prognostic value in KIRC
The survival data were statistically analyzed with “survival” package (Version 3.2-10), and the data visualization was carried out with “survminer” package (Version 0.4.9). Univariate and multivariate COX regression analysis were used to evaluate the relationship between ARHGAP4 expression and prognosis of KIRC patients. The diagnostic ROC curve and time-dependent survival ROC curve were performed with “pROC” (Version 1.17.0.1) and “timeROC” (Version 0.4) packages to evaluate the value of ARHGAP4 expression in the diagnosis and prognosis of KIRC.
2.5Functional analysis of ARHGAP4-related differentially expressed genes in KIRC
The differentially expressed genes (DEGs) between ARHGAP4 low expression group and high expression group were analyzed by “DESeq2” package (Version 1.26.0), and genes screening was carried out with the absolute value of logarithmic variation
2.6Analysis of correlation between the expression of ARHGAP4 and immune cell infiltration in KIRC
TIMER (https://cistrome.shinyapps.io/timer/) is an open and comprehensive database that can be used to analyze immune infiltration of different types of cancer. We used this database to analyze the correlation between ARHGAP4 and immune cell infiltration. The “GSVA” package (Version 1.34.0) and Spearman correlation analysis were used to evaluate the correlation between ARHGAP4 expression level and 24 kinds of tumor immune cells in KIRC.
2.7Analysis of correlation between the expression of ARHGAP4 and immune checkpoint genes in KIRC
The correlation between the level of ARHGAP4 in KIRC and immune checkpoint genes was explored by Spearman correlation analysis.
2.8Tissue specimens and Immunohistochemical (IHC) staining
In this study, 50 pairs of tumor tissues and matched para-carcinoma tissues were collected from the second Hospital of Tianjin Medical University. In addition, the clinicopathological data of these patients were obtained, including age, sex, tumor stage and Fuhrman grade. These patients had the following inclusion criteria: (1) all of these patients were diagnosed with KIRC; (2) none of the patients had other tumors; (3) none of the patients received radiotherapy or chemotherapy; (4) the patients underwent radical nephrectomy or partial nephrectomy. The study was approved by the informed consent of the patients and the Ethics Committee of the second Hospital of Tianjin Medical University.
We embedded these tissues in paraffin and sliced them, and then dewaxed and dehydrated the paraffin sections. Then, these sections were treated with citrate buffer for antigen repair, endogenous peroxidase activity was inactivated with 3% hydrogen peroxide, followed by blocking of tissue sections with 5% bovine serum albumin (BSA). Next, they were incubated overnight with antibody ARHGAP4 (1:60,16697-1-AP, Proteintech) at 4∘C. The secondary antibody (ZSGB-BIO, China) was incubated at room temperature for 1 h, then they were colored with HRP DAB detection kit (ZSGB-BIO, China) and re-stained with hematoxylin, and finally sealed with neutral resin. The staining of different tissue sections was observed under an optical microscope. It was evaluated by two independent pathologists based on staining intensity and the proportion of positively stained tumor cells. The evaluation criteria are as follows: (1) staining intensity: 0, negative; 1, moderate (yellowish brown); 2, strong (brown), (2) the proportion of tumor cells with positive staining: 0, 0–1%; 1, 1%–5%; 2, 6%–10%; 3, 11%–20%; 4, 21%–50% and 5, 51%–100%. The final score is the sum of intensity and percentage, which is divided into negative (0–3) and positive (4–7).
2.9Cell lines and culture methods
All the cell lines used were purchased from the American Type Culture Collection Library (ATCC), including human embryonic kidney cells HEK-293T and human renal cancer cells ACHN and 786-O.
ACHN used MEM medium (Viva Cell), 786-O and HEK-293T cells used DMEM medium (Viva Cell), and all cell media were supplemented with 10% fetal bovine serum (FBS, Viva Cell) and 1% penicillin-streptomycin (PS, Bioind). The cells were cultured in an incubator containing 5% CO2 at 37∘C.
2.10Construction of plasmid and stable cell lines
We designed the sgRNA of ARHGAP4 usingCRISPOR website (http://crispor.tefor.net/) and ligated sgRNA (F-CACCGTTCATGCGGCGCCGCGCTG, R-AAACCAGCGCGGCGCCGCATGAAC) into lentiCRISPRv2 plasmid. Then the empty plasmid (WT) and ARHGAP4-KO plasmid were transfected into 786-O and ACHN cell lines by lentivirus infection. Puromycin was used for screening, and Western blotting was used to verify the gene knockout efficiency.
2.11Western blotting
We added SDS protein lysate and protease inhibitor to the cell, shake on the ice for 10 minutes, ultrasonicate at 30% power for 20 seconds, then heat at 97∘C for 10 minutes, and finally use BCA kit (Thermo) to determine the protein concentration. The proteins were fractionated in 10% acrylamide gel and then transferred to the PVDF membrane. After 5% skimmed milk powder was sealed at room temperature for 2 hours, the corresponding primary antibodies were incubated overnight at 4∘C, and the second antibodies labeled by HRP were incubated at room temperature for 1 hour, then the chemiluminescence instrument was used to detect the target band.
2.12Colony formation assay
The cells in logarithmic growth phase were digested and resuscitated, and the cell density was adjusted to 1000 cells/ml. The cells were inoculated in 12-well plate with 100 ul in each well. After 7 days of cell culture, the cells were fixed with 4% paraformaldehyde for 20 minutes, stained with Giemsa’s dye for 10 minutes, washed in distilled water until the background was clean, dried and photographed under the microscope.
2.13Cell proliferation assay
The cells in logarithmic growth phase were inoculated in 96-well plate with 1000 cells per well. After the cells were adhered to the wall (4–8 hours), 100 ul CCK8 (Biosharp) mixed reagent was added to each well and incubated at 37∘C for 2 hours, the OD value (450 nm) was measured by enzyme labeling instrument for 0 h, and then the OD value was measured every 24 hours to calculate the cell proliferation rate.
Figure 1.
ARHGAP4 is highly expressed in KIRC. (A) The mRNA expression level of ARHGAP4 in 33 kinds of cancer tissues and their corresponding normal tissues in TCGA database. (B) The mRNA expression level of ARHGAP4 in paired KIRC carcinoma tissues and adjacent renal tissues. (C) The mRNA expression level of ARHGAP4 in unmatched KIRC carcinoma tissues and normal renal tissues in the TCGA-KIRC dataset. (D–F) The mRNA expression level of ARHGAP4 in KIRC carcinoma tissues and normal renal tissues in (D) GSE71963, (E) GSE66270, (F) GSE66271 dataset of GEO database. (G) The protein expression level of ARHGAP4 in KIRC tissues and para-carcinoma tissues from CPTAC samples. *p < 0.05; **p < 0.01; ***p < 0.001.
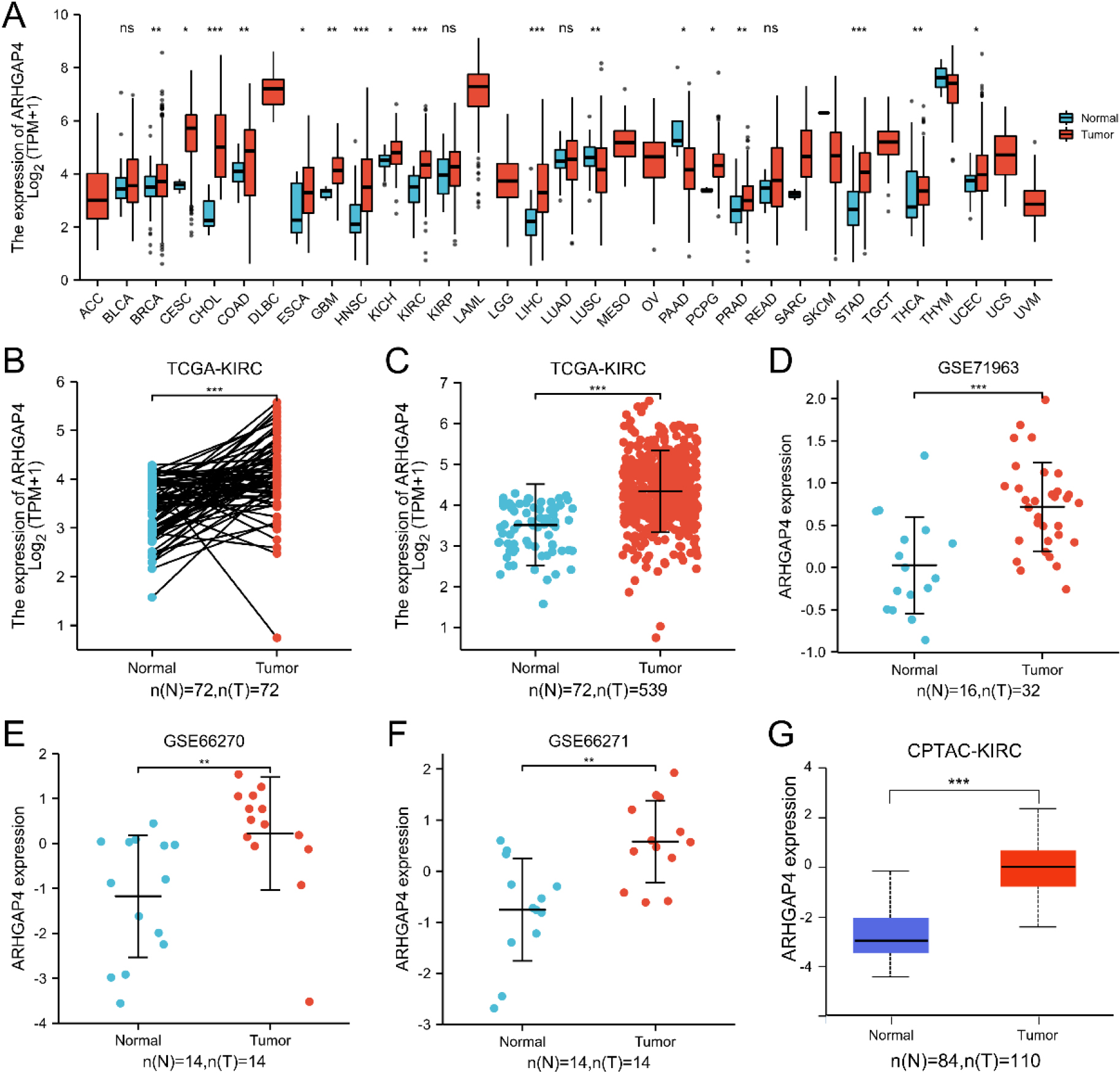
2.14Scratch healing assay
Draw horizontal lines on the back of the six-well plate, inoculate the cells into the 6-well plate. After the cells were covered with the bottom of the wells, the cells were scratched perpendicular to the plate and washed with PBS for 3 times before adding the serum-free medium. The photo field was determined according to the intersection of the scratch and the horizontal line on the back, and the photos were taken at 0, 6 and 18 hours.
2.15Cell migration and invasion assay
The cells in logarithmic growth phase were digested with trypsin and re-suspended with serum-free culture medium, and the cell density was adjusted to 2
2.16Statistical analysis
Statistical analysis was performed using IBM SPSS Statistics 25 and GraphPad Prism software 8.0. The relationship between ARHGAP4 protein expression and clinicopathological features was analyzed by Chi-square test. Significance was considered when
3.Results
3.1ARHGAP4 was upregulated in KIRC
Figure 2.
Expression of ARHGAP4 in KIRC tissues. Low expression of ARHGAP4 in para-carcinoma tissues of KIRC (A, B). High expression of ARHGAP4 in KIRC tissues (C, D).
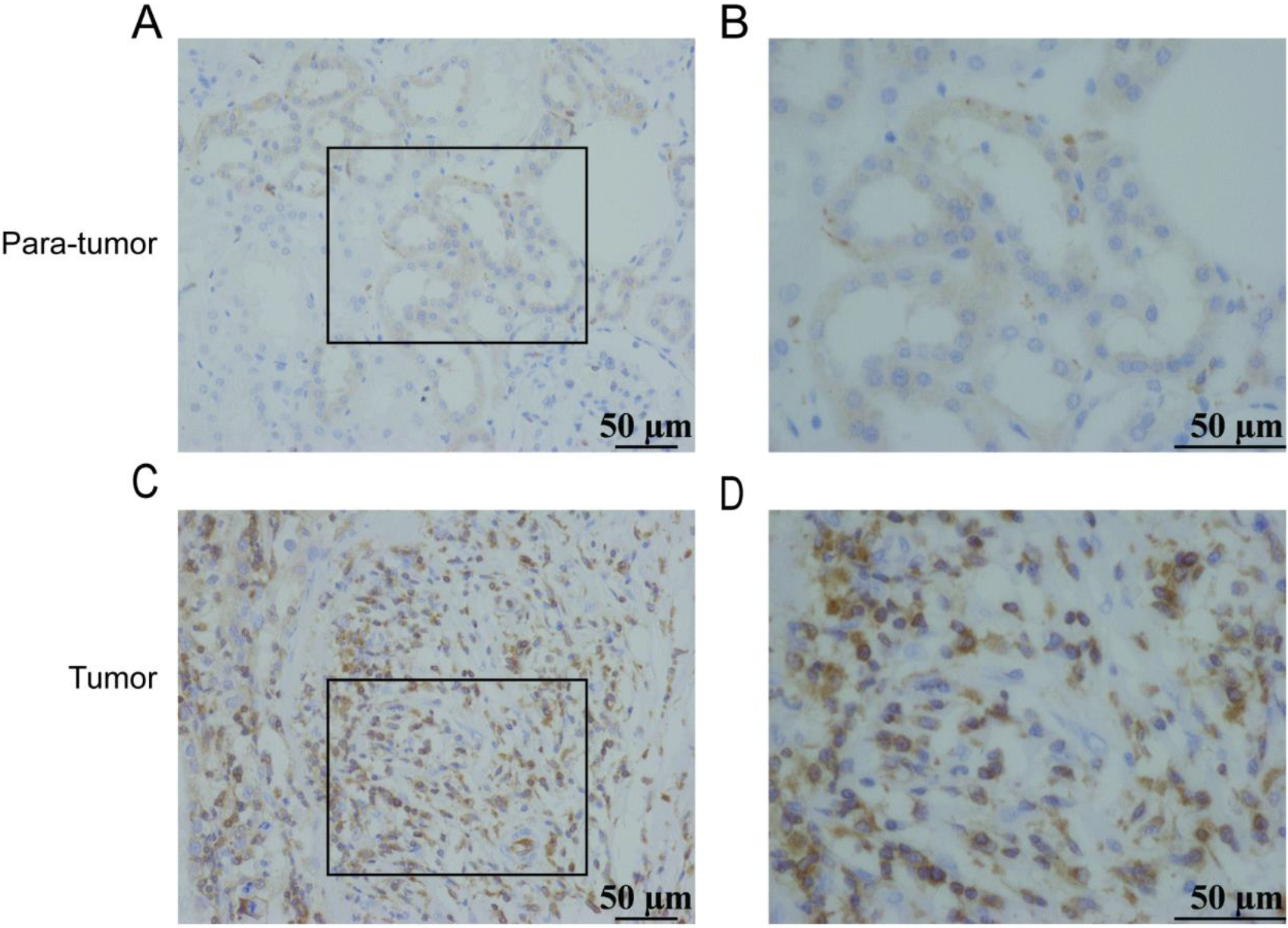
We analyzed the expression of ARHGAP4 using 33 cancer datasets in TCGA database. The results showed that compared with the para-carcinoma tissues, the mRNA expression of ARHGAP4 was significantly upregulated in BRCA, CESC, CHOL, COAD, ESCA, GBM, HNSC, KIRH, KIRC, LIHC, PRAD, PCPG, STAD, THCA and UCEC tissues. Conversely, we found that there was significant decrease of ARHGAP4 expression in LUSC and PAAD tissues (Fig. 1A).
Particularly, we downloaded the RNA-seq data of 539 cases of KIRC and 72 cases of para-carcinoma tissues from TCGA and analyzed the mRNA expression of ARHGAP4. The results of paired test showed that the mRNA expression of ARHGAP4 in KIRC tissues was significantly higher than that in para-carcinoma tissues (
Table 1
Expression of ARHGAP4 and clinical characteristics in clinical tissue samples
Characteristic | ARHGAP4 | |||
---|---|---|---|---|
N | Negative | Positive |
| |
Gender | ||||
Man | 26 | 6 | 20 | 0.571 |
Woman | 24 | 4 | 20 | |
Age | ||||
| 19 | 3 | 16 | 0.56 |
| 31 | 7 | 24 | |
T stage | ||||
T1–2 | 28 | 9 | 19 | 0.015 |
T3–4 | 22 | 1 | 21 | |
Fuhrman grade | ||||
I, II | 28 | 6 | 22 | 0.776 |
III, IV | 22 | 4 | 18 | |
Tissue | ||||
Tumor | 50 | 10 | 40 | |
Para-tumor | 50 | 42 | 8 |
Considering the specificity of KIRC with high expression of ARHGAP4, this marker was verified in the KIRC tissue via IHC. The IHC staining image was randomly selected as shown in the figures (Figs 2A–D), which showed that the expression of ARHGAP4 in KIRC tissues was significantly higher than that in para-carcinoma tissues. Then we analyzed the relationship between ARHGAP4 expression and clinicopathological parameters of KIRC patients, including gender, age, T stage and Fuhrman grade. The results showed that the expression level of ARHGAP4 in KIRC patients was related to T stage (Table 1).
Figure 3.
The relation between ARHGAP4 expression and clinicopathological characteristics of KIRC. The correlation between ARHGAP4 and race (A), TNM stage (B-D), pathological stage (E) and (F) histological grade in the TCGA-KIRC dataset. **p < 0.01; ***p < 0.001.
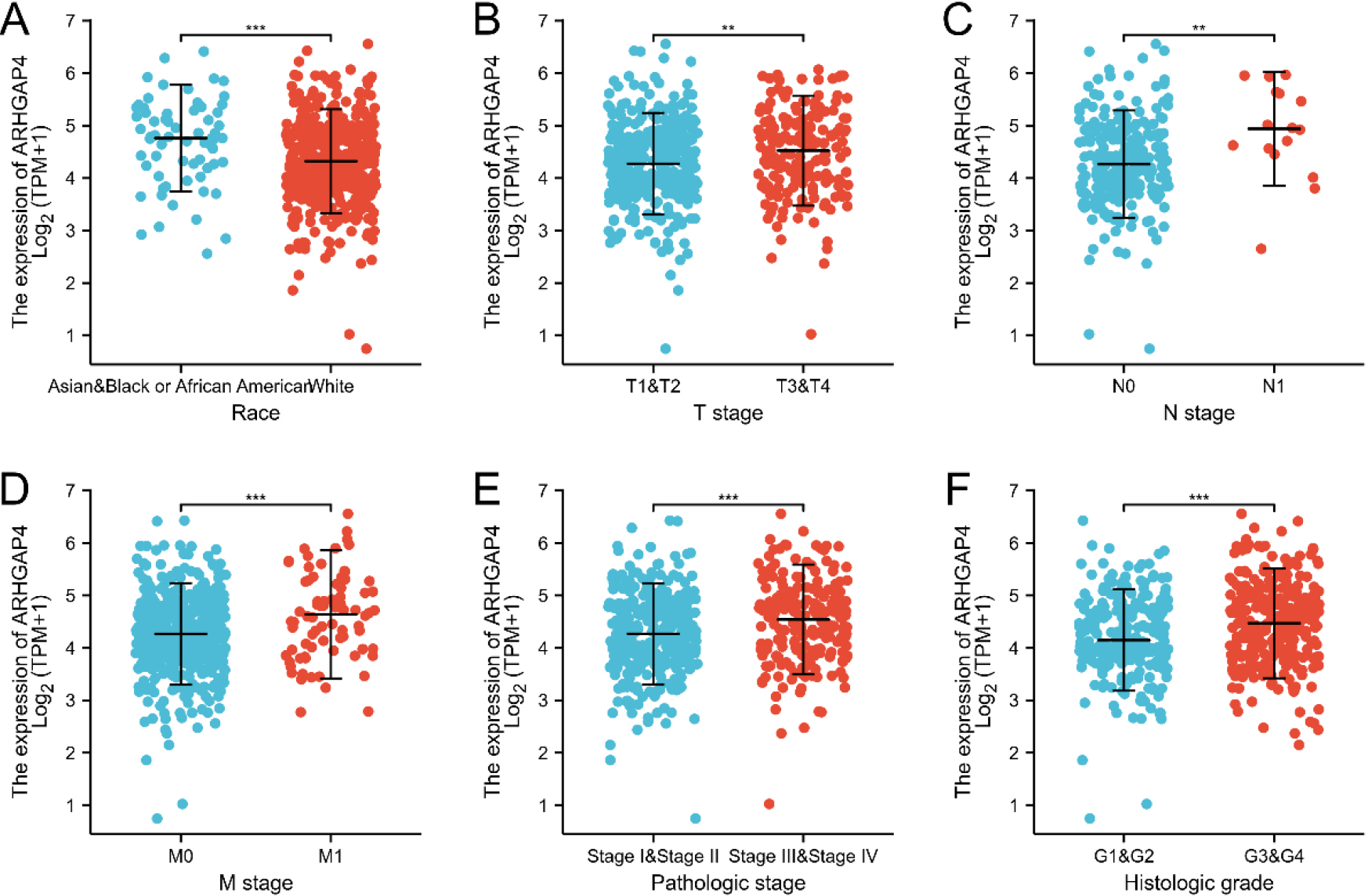
Table 2
Clinical and pathological characteristics of patients with high and low ARHGAP4 expression levels in the TCGA-KIRC dataset
Characteristic | Low expression of ARHGAP4 | High expression of ARHGAP4 |
|
---|---|---|---|
| 269 | 270 | |
Age, | 0.83 | ||
| 136 (25.2%) | 133 (24.7%) | |
| 133 (24.7%) | 137 (25.4%) | |
Gender, | 0.903 | ||
Female | 94 (17.4%) | 92 (17.1%) | |
Male | 175 (32.5%) | 178 (33%) | |
Race, | 0.012* | ||
Asian | 2 (0.4%) | 6 (1.1%) | |
Black or African American | 19 (3.6%) | 38 (7.1%) | |
White | 242 (45.5%) | 225 (42.3%) | |
T stage, | 0.005** | ||
T1 | 157 (29.1%) | 121 (22.4%) | |
T2 | 33 (6.1%) | 38 (7.1%) | |
T3 | 77 (14.3%) | 102 (18.9%) | |
T4 | 2 (0.4%) | 9 (1.7%) | |
N stage, | 0.018* | ||
N0 | 127 (49.4%) | 114 (44.4%) | |
N1 | 3 (1.2%) | 13 (5.1%) | |
M stage, | 0.012* | ||
M0 | 234 (46.2%) | 194 (38.3%) | |
M1 | 30 (5.9%) | 48 (9.5%) | |
Pathologic stage, | 0.009** | ||
Stage I | 155 (28.9%) | 117 (21.8%) | |
Stage II | 27 (5%) | 32 (6%) | |
Stage III | 54 (10.1%) | 69 (12.9%) | |
Stage IV | 32 (6%) | 50 (9.3%) | |
Histologic grade, | 0.004** | ||
G1 | 7 (1.3%) | 7 (1.3%) | |
G2 | 137 (25.8%) | 98 (18.5%) | |
G3 | 91 (17.1%) | 116 (21.8%) | |
G4 | 29 (5.5%) | 46 (8.7%) | |
OS event, | 0.011* | ||
Alive | 197 (36.5%) | 169 (31.4%) | |
Dead | 72 (13.4%) | 101 (18.7%) | |
DSS event, | |||
Alive | 228 (43.2%) | 192 (36.4%) | |
Dead | 35 (6.6%) | 73 (13.8%) | |
PFI event, | 0.026* | ||
Alive | 201 (37.3%) | 177 (32.8%) | |
Dead | 68 (12.6%) | 93 (17.3%) |
Table 3
Logistic regression analysis of the relationship between the expression level of ARHGAP4 and clinical pathological characteristics in the TCGA-KIRC dataset
Characteristics | Total (N) | Odds ratio (OR) |
|
---|---|---|---|
Race (White vs. Asian & Black or African American) | 532 | 0.444 (0.252–0.761) | 0.004** |
T stage (T3 & T4 vs. T1 & T2) | 539 | 1.679 (1.176–2.405) | 0.004** |
N stage (N1 vs. N0) | 257 | 4.827 (1.510–21.444) | 0.016* |
M stage (M1 vs. M0) | 506 | 1.930 (1.184–3.191) | 0.009** |
Pathologic stage (Stage III & Stage IV vs. Stage I & Stage II) | 536 | 1.690 (1.190–2.408) | 0.003** |
Histologic grade (G3 & G4 vs. G1 & G2) | 531 | 1.851 (1.313–2.619) |
3.2Relationship between the expression of ARHGAP4 and clinicopathological characteristics in KIRC
Then, we evaluated the relationship betweenARHGAP4 expression and various clinicopathological parameters in KIRC patients. 539 samples of KIRC were downloaded from TCGA, including ARHGAP4 expression data obtained from patients with different clinical characteristics (Table S1). According to the median level of ARHGAP4 expression, we divided 539 KIRC patients into high expression group and low expression group. Table 2 showed the relationship between the level of ARHGAP4 expression and the clinicopathological characteristics of KIRC patients. We found that there was significant difference in race, TNM stage, pathological stage, histological grade, overall survival time (OS), disease-specific survival time (DSS) and disease progression-free interval (PFI) between KIRC patients with high and low expression of ARHGAP4. The expression level of ARHGAP4 was significantly correlated with race (Fig. 3A), TNM stage (Figs 3B–D), pathological stage (Fig. 3E) and histological grade (Fig. 3F). In KIRC patients, the expression of ARHGAP4 in Caucasians was significantly higher than that in other races. Moreover, patients with advanced cancer stages and high histological grades had higher levels of ARHGAP4 expression. In addition, we used Logistics regression analysis to evaluate the relationship between ARHGAP4 and different clinical characteristics. From the results, it can be known that the expression of ARHGAP4 was positively correlated with TNM stage, pathological stage and histological grade (Table 3).
3.3Predictive effect of ARHGAP4 on the diagnosis and prognosis of KIRC
Figure 4.
The value of ARHGAP4 in the diagnosis and prognosis of patients with KIRC. (A–C) The effects of ARHGAP4 expression on (A) overall survival time (OS), (B) disease-specific survival time (DSS) and (C) progression-free interval (PFI) of patients in the TCGA-KIRC dataset were analyzed by survival curve. (D) The diagnostic ROC curve of differentiating KIRC tissue from normal tissue by ARHGAP4 in the TCGA-KIRC dataset. (E–H) The ROC curves of ARHGAP4 in the TCGA-KIRC dataset differentiate normal tissues from KIRC tissues of different stages. (I) The time-dependent ROC curves of 1-, 3-and 5-year overall survival (OS) predicted by ARHGAP4 in the TCGA-KIRC dataset.
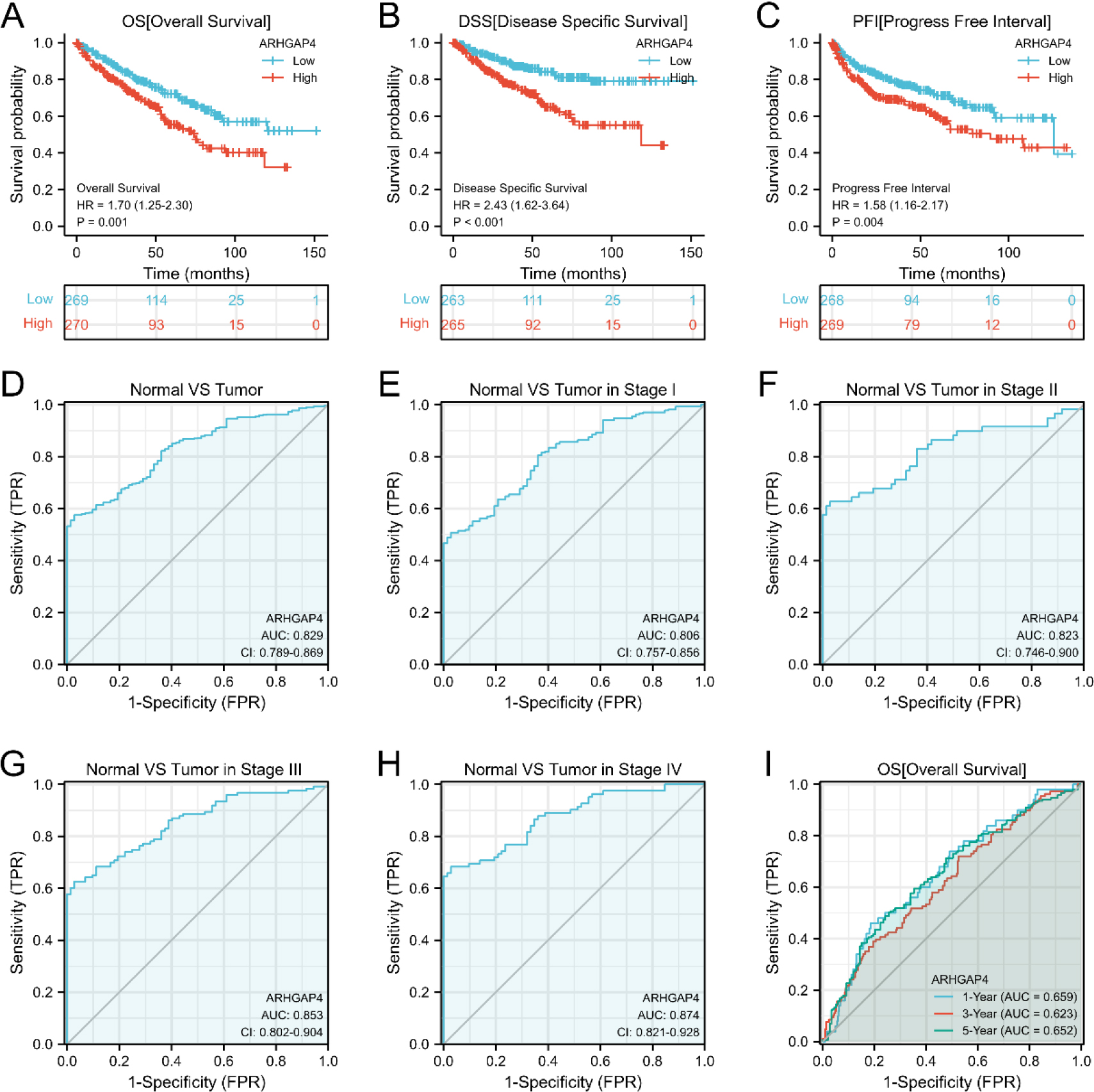
Table 4
Univariate and multivariate COX regression analysis showed the impact of ARHGAP4 expression levels and different clinical pathological characteristics on overall survival (OS) in the TCGA-KIRC dataset
Characteristics | Total (N) | Univariate analysis | Multivariate analysis | ||
---|---|---|---|---|---|
Hazard ratio (95% CI) |
| Hazard ratio (95% CI) |
| ||
Age | 539 | ||||
| 269 | Reference | |||
| 270 | 1.765 (1.298–2.398) | 1.659 (1.083–2.543) | 0.020* | |
Race | 532 | ||||
Asian & Black or African American | 65 | Reference | |||
White | 467 | 1.222 (0.678–2.201) | 0.505 | ||
T stage | 539 | ||||
T1 & T2 | 349 | Reference | |||
T3 & T4 | 190 | 3.228 (2.382–4.374) | 1.504 (0.664–3.410) | 0.328 | |
N stage | 257 | ||||
N0 | 241 | Reference | |||
N1 | 16 | 3.453 (1.832–6.508) | 1.540 (0.764–3.102) | 0.227 | |
M stage | 506 | ||||
M0 | 428 | Reference | |||
M1 | 78 | 4.389 (3.212–5.999) | 2.764 (1.635–4.672) | ||
Pathologic stage | 536 | ||||
Stage I & Stage II | 331 | Reference | |||
Stage III & Stage IV | 205 | 3.946 (2.872–5.423) | 1.240 (0.490–3.139) | 0.65 | |
Histologic grade | 531 | ||||
G1 & G2 | 249 | Reference | |||
G3 & G4 | 282 | 2.702 (1.918–3.807) | 1.563 (0.941–2.598) | 0.085 | |
ARHGAP4 | 539 | ||||
Low | 269 | Reference | |||
High | 270 | 1.681 (1.240–2.279) | 1.627 (1.034–2.562) | 0.035* |
We analyzed the effect of ARHGAP4 expression on the survival rate of KIRC patients by K-M survival curve. From the results, it can be found that the overall survival time (OS) of ARHGAP4 low expression group is significantly higher than that of high expression group. Similarly, the disease-specific survival time (DSS) and progression-free interval (PFI) of patients showed the same trend (Figs 4A–C). Next, we evaluated the diagnostic value of ARHGAP4 expression level in KIRC by ROC curve. As shown in the figure (Fig. 4D), the AUC value is 0.829, indicating that the expression level of ARHGAP4 has a high accuracy in the differential diagnosis of tumor and normal tissue. In addition, compared with tumors in early stage, ARHGAP4 has higher differential ability (Figs 4E–H) for tumors in late stage. Then, univariate and multivariate COX regression analysis was used to evaluate the effects of ARHGAP4 expression and common clinicopathological features on OS, DSS and PFI in KIRC patients (Table 4 and Tables S2, S3). It can be seen from these results that the high expression of ARHGAP4, like age, TNM stage, pathological stage and histological grade, is also a factor affecting the prognosis of KIRC patients. Furthermore, the expression of ARHGAP4 could be an independent risk factor affecting OS in KIRC patients. The results of time-dependent ROC curve (Fig. 4I) analysis showed that according to the expression level of ARHGAP4, the AUC values of OS in patients with KIRC at 1, 3 and 5 years were all above 0.6. In addition, the results of the ROC curve (Fig. S1) of DSS and PFI suggested that the expression of ARHGAP4 could also predict DSS and PFI in KIRC patients.
Figure 5.
Verification of the effect of ARHGAP4 on growth and proliferation in vitro. (A) Expression of ARHGAP4 protein in 786-O and ACHN cells in control group and ARHGAP4-KO group. (B, C) Cell proliferation rate of control group and ARHGAP4-KO group in 786-O and ACHN cells. (D, E) Number of colonies in control group and ARHGAP4-KO group in 786-O and ACHN cells. **p < 0.01; ***p < 0.001.
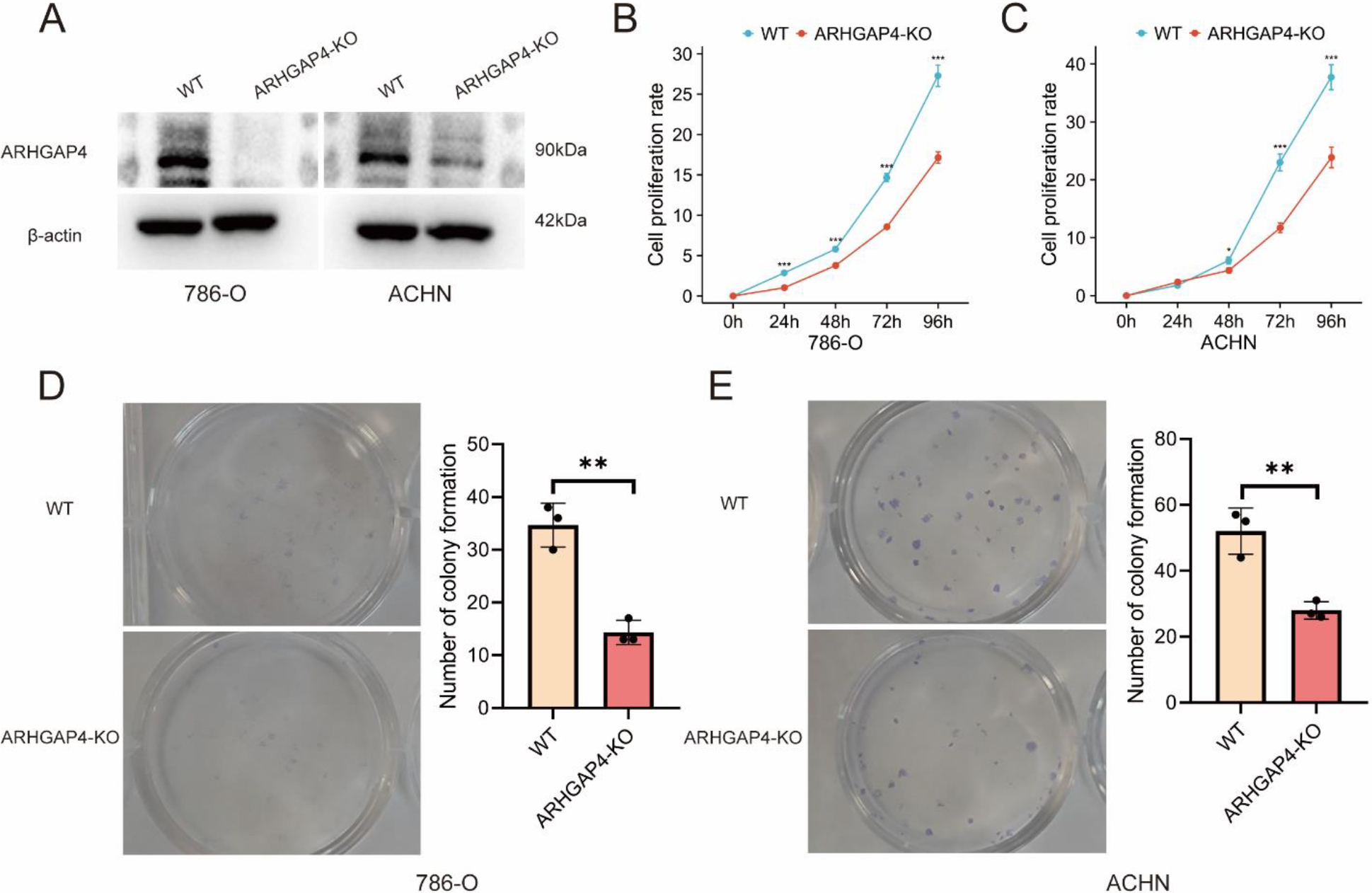
Figure 6.
Verification of the effect of ARHGAP4 on migration and invasion in vitro. (A, B) Scratch healing distance between control group and ARHGAP4-KO group in 786-O and ACHN cells. (C, D) Number of cell migration in control group and ARHGAP4-KO group in 786-O and ACHN cells. (E, F) Number of cell invasion in control group and ARHGAP4-KO group in 786-O and ACHN cells. **p < 0.01; ***p < 0.001.
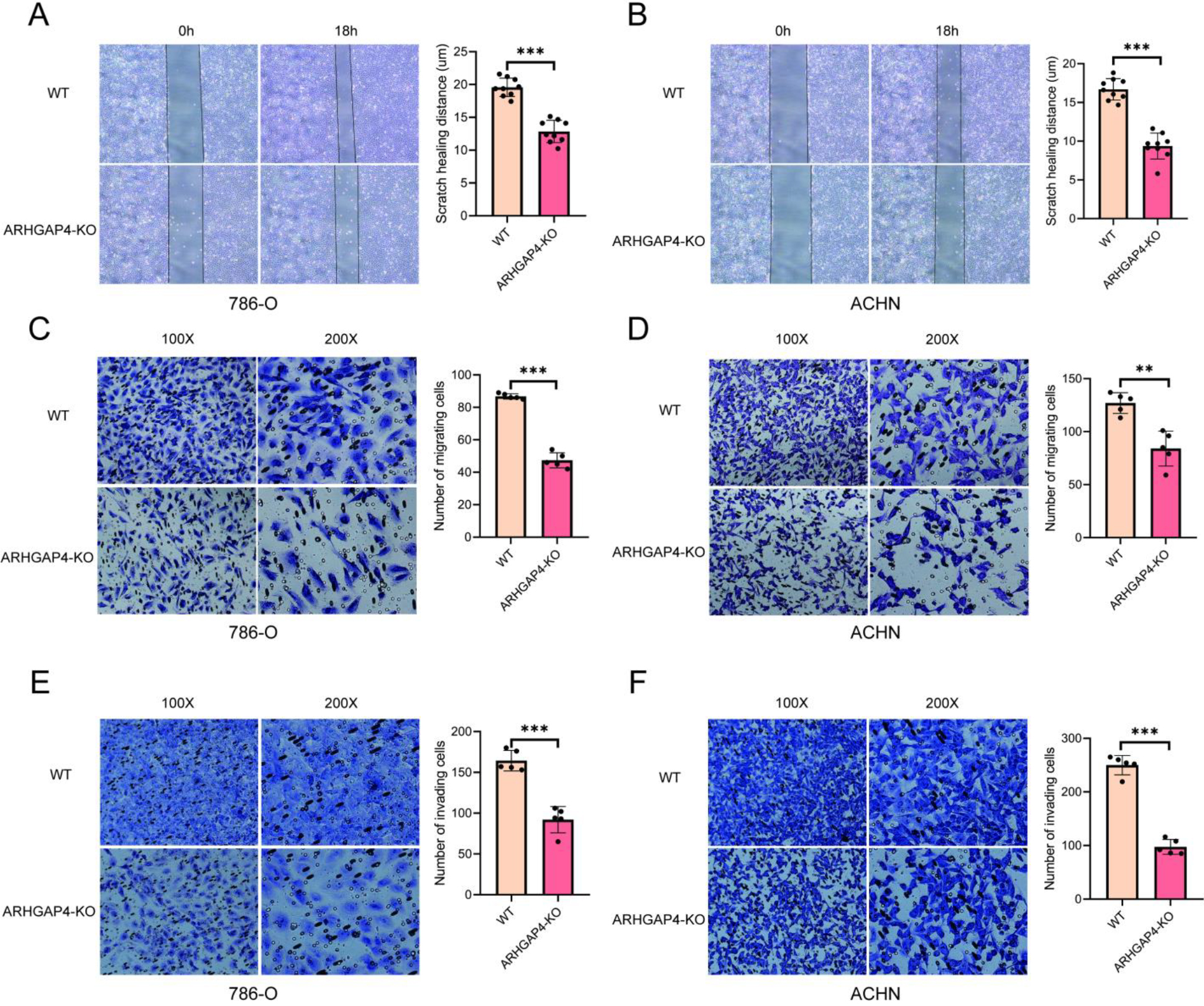
3.4Verification of the effect of ARHGAP4 on the progression of KIRC in vitro
In order to further explore and verify the role of ARHGAP4 in KIRC, we used CRISPR technique to down-regulate the expression of ARHGAP4 in 786-O and ACHN cell lines, and verified the down-regulation efficiency of ARHGAP4 in cells by Western blotting analysis (Fig. 5A). Subsequently, the cell proliferation assays showed that down-regulation of ARHGAP4 significantly inhibited the proliferation and growth of these cells (Figs 5B, C). Similarly, the results of colony formation assays showed that both the number of colonies and the colony size in the ARHGAP4-KO groups were lower than those in the control groups suggesting that the knockout of ARHGAP4 significantly reduced the colony formation ability of the two cell lines (Figs 5D, E). Then we carried out the scratch healing assays to study the effect of ARHGAP4 on the migration ability of renal carcinoma cells (Figs 6A, B). The migration ability of ARHGAP4 knockout cells was significantly weaker than that of control cells, indicating that the down-regulation of ARHGAP4 could inhibit the migration ability of renal carcinoma cells. In order to further verify the effect of ARHGAP4 on the migration and invasion of renal carcinoma cells, we carried out Transwell migration and invasion assays. The number of cells passing through the chambers in the ARHGAP4 knockout groups was significantly lower than that in the control groups, which were consistent with the results of scratch assays (Figs 6C, D). Similarly, the down-regulation of ARHGAP4 also inhibited the ability of cells to pass through Matrigel (Figs 6E, F).
Figure 7.
Biological function analysis of ARHGAP4-related differentially expressed genes (DEGs) in KIRC. (A) Volcanic map of ARHGAP4-related DEGs in the TCGA-KIRC dataset. (B) KEGG pathway analysis showed the functions and pathways of ARHGAP4-related DEGs in the TCGA-KIRC dataset. (C-E) GO analysis showed the (C) biological processes (BP), (D) cellular components (CC) and (E) molecular functions (MF) of ARHGAP4-related DEGs in the TCGA-KIRC dataset.
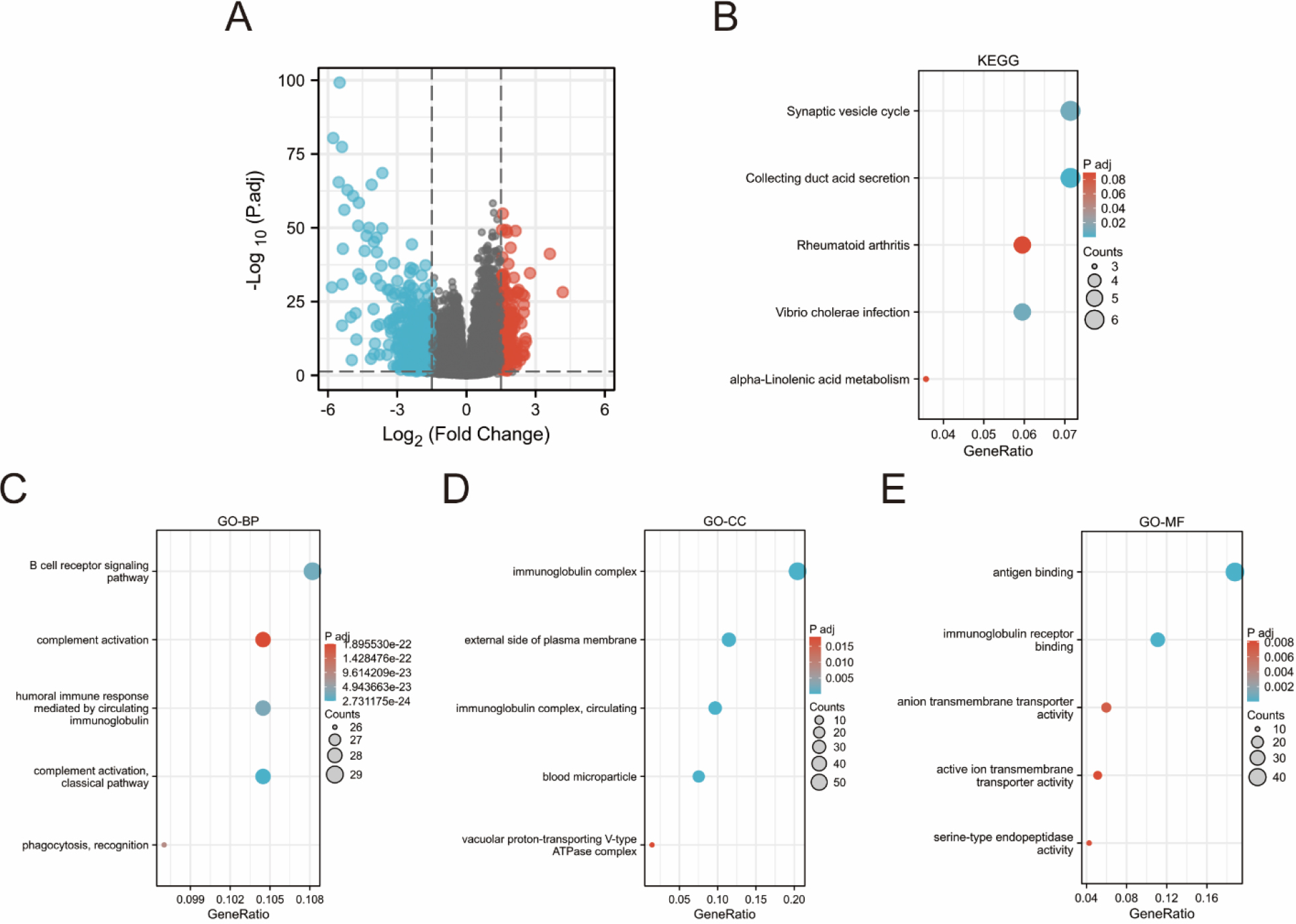
3.5Biological function Analysis of ARHGAP4-related differentially expressed genes in KIRC
Using absolute logarithmic variation
Figure 8.
The expression level of ARHGAP4 in KIRC was related to immune infiltration. (A, B) The correlation between the expression level of ARHGAP4 in KIRC tissues and the level of immune cell infiltration in TIMER database. (C) The infiltration level of 24 kinds of immune cells in the TCGA-KIRC dataset with high expression group and low expression group of ARHGAP4. (D) The correlation between the expression level of ARHGAP4 and the infiltration level of 24 kinds of immune cells in the TCGA-KIRC dataset. *p < 0.05; **p < 0.01; ***p < 0.001.
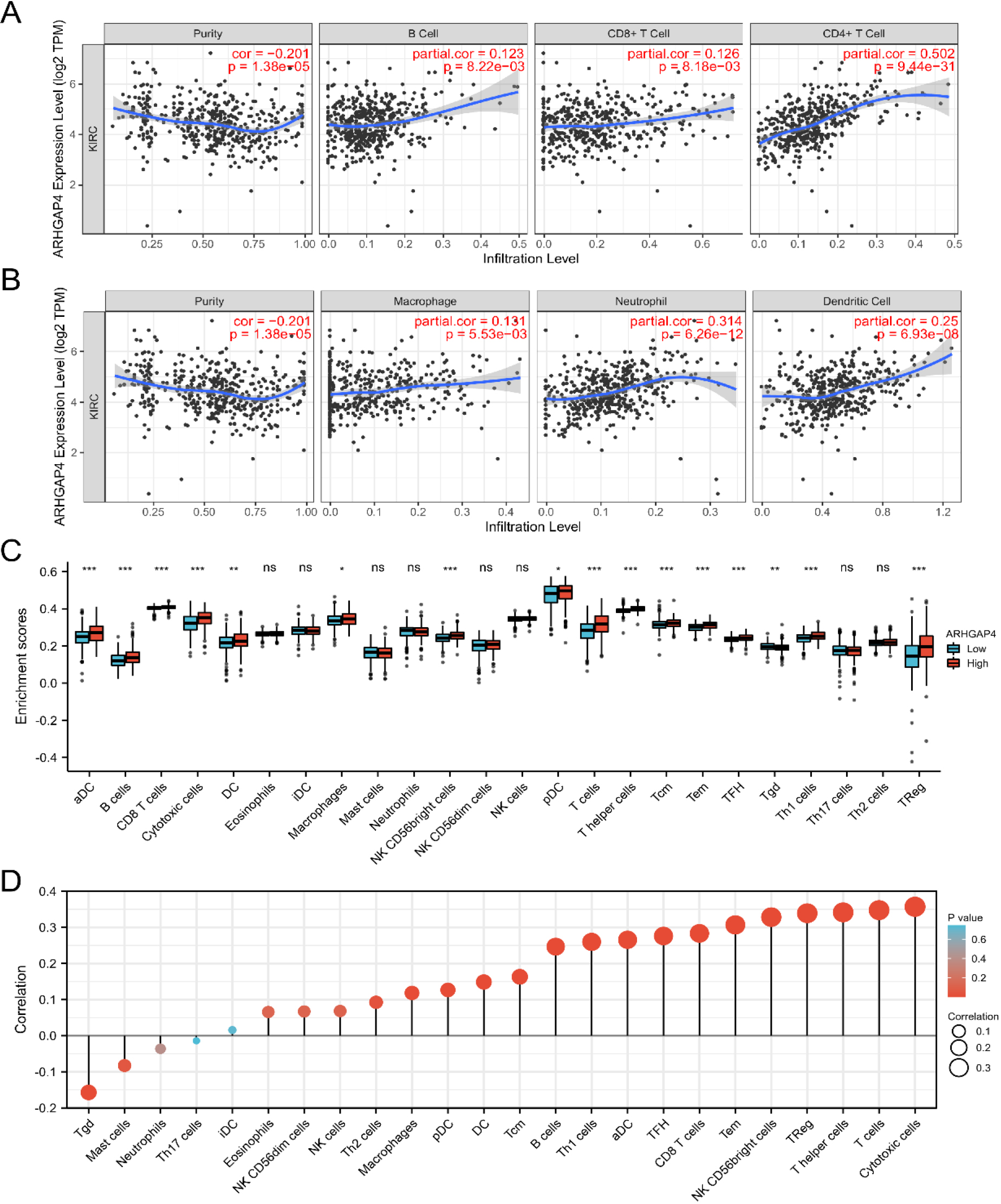
Figure 9.
ARHGAP4 is associated with immune checkpoint genes in KIRC. (A-I) Correlation analysis between the expression levels of ARHGAP4 and PDCD1, PDCD-L1 (CD274), CTLA-4LAG3, TIGIT, OX40 (TNFSF4), B7-H3 (CD276), BTLA, and TNFRSF9 in the TCGA-KIRC dataset.
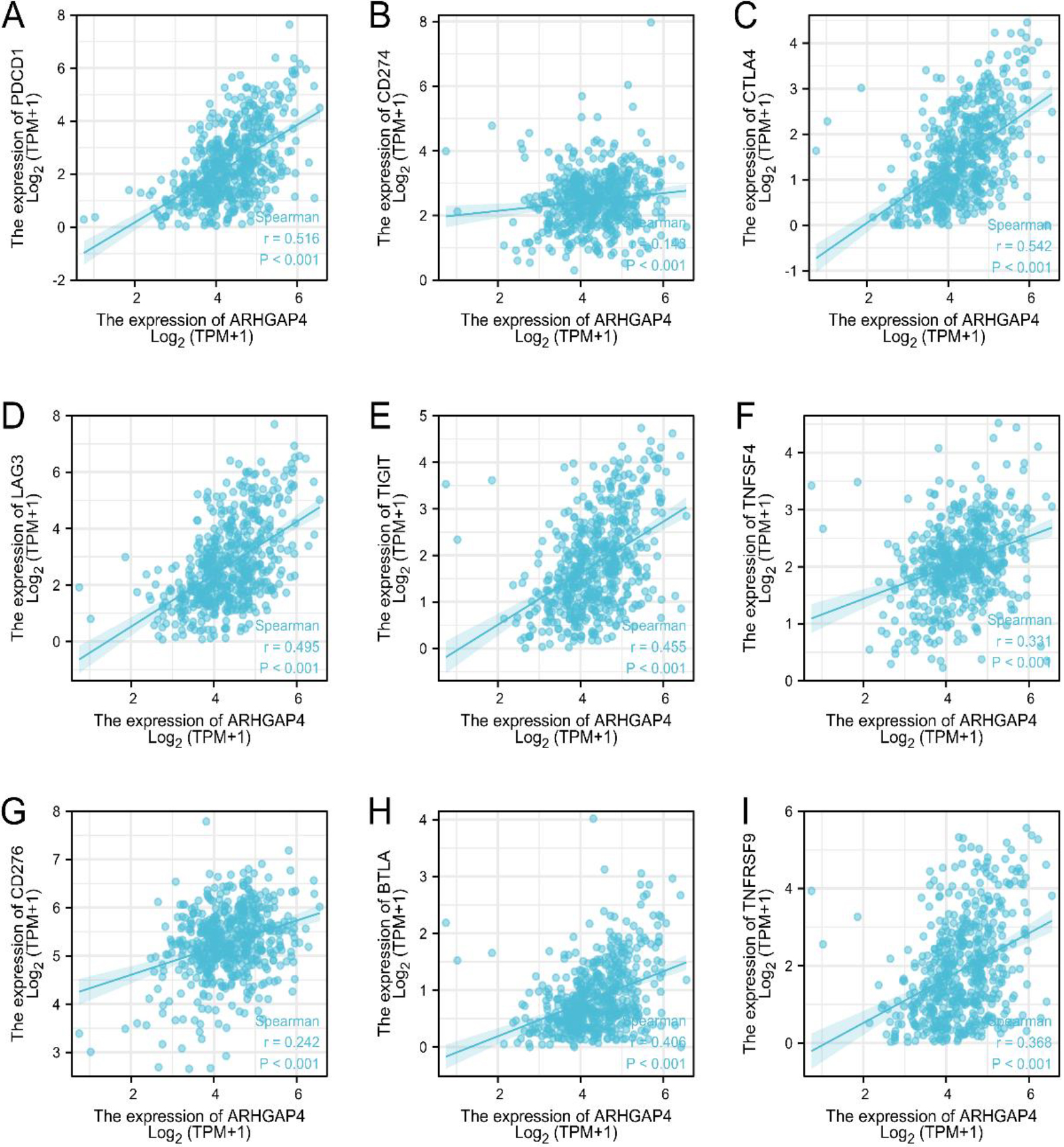
3.6Relationship between ARHGAP4 expression and immune infiltration in KIRC
Tumor immunity plays an important role in the overall survival rate and prognosis of patients. Previous biological functional analysis showed that ARHGAP4 was related to tumor immune cells. Therefore, we evaluated the relationship between the expression level of ARHGAP4 in KIRC and immune cell infiltration using the Timer database. As shown in the following figures, ARHGAP4 was positively correlated with CD4 + T cells, DC cells, B cells, Macrophages, CD8+ T cells and neutrophils, especially with CD4 + T cells (Figs 8A, B). Next, we evaluated the difference in tumor immunity between patients with high and low levels of ARHGAP4 expression. We used ssGSEA algorithm to evaluate the difference of expression of 24 kinds of immune cells between the two groups (Fig. 8C). We also evaluated the correlation between ARHGAP4 and 24 kinds of immune cells in KIRC by Spearman correlation analysis. The results showed that the expression of ARHGAP4 was positively correlated with some kinds of immune cells in KIRC, including cytotoxic cells (
3.7The relationship between ARHGAP4 and immune checkpoint genes expression in KIRC
Tumor immunotherapy is a new treatment with broad prospects, which is often used as an adjuvant therapy, combined with conventional treatments such as surgery, chemotherapy and radiotherapy, playing an increasingly important role in tumor treatment. However, the tumor immune escape mechanism will significantly reduce the effectiveness of immunotherapy, and immune checkpoint proteins are closely related to tumor immune escape [23]. Programmed cell death 1 (PDCD1), programmed cell death ligand-1 (PDCD-L1, CD274) and cytotoxic T lymphocyte antigen 4 (CTLA-4) are the most widely studied and recognized immune checkpoint proteins [24]. In addition, Lymphocyte activation gene-3 (LAG3), B and T cell lymphocyte attenuator (BTLA), OX40 (TNFSF4), B7 homolog 3 (B7-H3, CD276), T cell immunoglobulin and ITIM domain (TIGIT) and tumor necrosis factor receptor superfamily member 9 (TNFRSF9) are promising genes of new immune checkpoint pathway. Our results proved that in the KIRC samples of TCGA, the expression level of ARHGAP4 was positively correlated with the expression level of these immune checkpoint genes (Figs 9A–I), especially with the expression of PDCD1 (
4.Discussion
Renal cell carcinoma (RCC) is a malignant tumor that seriously affects human health, and its morbidity and mortality remain high [25]. KIRC is the main subtype of RCC. Surgical treatment is mainly used in patients with early KIRC, but the effect of surgical treatment for advanced and metastatic tumors is limited. With the development of medical technology, although great progress has been made in drug treatment, chemotherapy and radiotherapy, the clinical results of patients with advanced KIRC are still not satisfactory due to the influence of tumor drug resistance and radiation resistance [26]. As a new treatment, immunotherapy plays an increasingly important role in tumor treatment, which is often used in combination with radiotherapy, chemotherapy and other treatment methods to improve the therapeutic effect of patients. In addition, immune checkpoint inhibitors (ICIs), such as CTLA-4 inhibitors, PDCD1 inhibitors and PDCD-L1 inhibitors, have been used in the clinical treatment of various malignant tumors, such as lung cancer, breast cancer, gastric cancer, renal cell carcinoma and Hodgkin’s lymphoma [23, 27, 28, 29]. However, the specific biomarkers for diagnosis, individualized treatment and predicting prognosis of KIRC are still needed to be evaluated.
Some investigations have shown that ARHGAP4 is highly expressed in colorectal cancer, gastric cancer and cervical cancer, and is related to tumor progression and prognosis [30, 31, 32]. Similarly, the expression of ARHGAP4 has a certain effect on the effect of chemotherapy in ovarian tumors [33]. However, the role of ARHGAP4 in KIRC is not clear. In this research, we proved the different ARHGAP4 expression in human cancer tissues. It was significantly high expression in 14 cancers, including KIRC, and low expression in 2 cancers, indicating that ARHGAP4 has cancer specificity. According to the RNA sequencing data of KIRC patient samples from TCGA and GEO databases, we observed that ARHGAP4 was upregulated in cancer tissues compared with the corresponding normal tissues. In addition, immunohistochemical staining showed that ARHGAP4 was significantly upregulated in KIRC tissues and correlated with tumor malignancy.
From our results, we can find that the expression level of ARHGAP4 was significantly correlated with race, TNM stage, clinical stage, pathological stage, OS, DSS and PFI in KIRC patients. In addition, univariate and multivariate COX regression analysis results showed that ARHGAP4 was an independent prognostic factor in patients with KIRC, and the higher ARHGAP4 expression meant the worse prognosis. The larger the AUC value of the ROC curve, the stronger the diagnostic and predictive ability. The AUC value of ARHGAP4 differential diagnosis KIRC was 0.829, which indicated that it had great diagnostic value. Meanwhile, the 1-, 3-and 5-year AUC values of predicted overall survival were all higher than 0.6. As we know, the proliferation, migration and invasion of tumor cells are closely related to the progression of tumor, and also affect the survival and prognosis of patients. Next, we carried out a series of cell assays in vitro to further verify the role of ARHGAP4 in the progression of KIRC. The results of colony formation assay and cell proliferation assay suggested that knockout of ARHGAP4 can significantly inhibit the growth and proliferation of renal carcinoma cells. Scratch healing assay, Transwell migration and invasion assays showed that down-regulation of ARHGAP4 expression decreased the migration and invasion ability of renal carcinoma cells. These data suggested that ARHGAP4 may be a diagnostic and prognostic biomarker for KIRC.
Next, we functionally enriched ARHGAP4-related DEGs by GO analysis and KEGG pathway enrichment analysis to further study the biological functions of ARHGAP4. The results suggested that these differentially expressed genes are enriched in immune and transport-related functions. High expression of ARHGAP4 in KIRC patients may affect tumor progression and prognosis by regulating tumor immune response.
It is precisely because tumor immunity is closely related to tumor progression, immunotherapy strategy is also considered as a very promising method of tumor therapy. In recent years, immunotherapy for RCC has developed rapidly, from non-specific immunotherapy to targeted immunotherapy, and targeted immunotherapy is also considered to be a more accurate and effective new treatment strategy, which will greatly improve the therapeutic effect of patients [29]. Therefore, exploring the relationship between ARHGAP4 and tumor immunity in KIRC is of great significance for the prognosis and treatment of KIRC.
Subsequently, our research showed that ARHGAP4 is positively correlated with CD4+ T cells, DC cells, B cells, Macrophages, CD8 + T cells and neutrophils. In addition, the expression of ARHGAP4 was positively correlated with the immune infiltration level of some immune cells in KIRC, including cytotoxic cells, T cells, T helper cells, Tregs, NK CD56 bright cells, Tems, CD8 + T cells, TFHs, aDCs, Th1s, B cells and so on. The main function of CD8 + T cells is to provide protective immunity and play a key role in the immunotherapy of infections and tumors [34]. In addition, CD4 + T cells contribute to the formation of protective memory CD8 + T cells after infection or immunization [35]. Mass spectrometry and digital cell analysis have found that the most common types of immune cells in KIRC tumors are CD4 + T cells, CD8 + T cells, and macrophages. Interestingly, the percentage of CD8 + T cells is highly positively correlated with the expression level of PDCD1 [36]. In addition, studies have shown that high levels of CD4 + T cells and CD8 + T cell abundance in KIRC patients may indicate shorter survival and poorer surgical outcomes, and their underlying mechanisms may be related to tumor immune escape [37, 38]. DCs are special antigen presenting cells, which are powerful and play a key role in initiating, regulating and maintaining immune responses [39]. B cell-mediated humoral immunity can effectively supplement T cell-mediated anti-tumor immunity [40]. In general, macrophages not only have the function of maintaining balance in the body, but also play an important role in immunity. However, tumor-associated macrophages (TAM) in tumor microenvironment (TME) can promote tumor cell proliferation and angiogenesis, participate in tumor immunosuppression, and thus promote tumor progression [41]. Neutrophils have powerful antibacterial functions, however, improper activation or imbalance of neutrophils can also damage the host and lead to autoimmune and inflammatory diseases [42]. Therefore, our data show that the overexpression of ARHGAP4 may be related to immune cell infiltration in KIRC, which can promote the progression of KIRC.
Renal cell carcinoma has high immune infiltration and low mutation load, and it is highly sensitive to immunotherapy. Therefore, it is of great significance to explore the therapeutic targets and biomarkers that affect the efficacy of renal cell carcinoma. From the initial therapeutic drugs that regulate the immune system, such as interleukin-2 (IL-2) and interferon (IFN), to immune checkpoint inhibitors, such as PDCD1 inhibitors, PDCD-L1 inhibitors and CTLA-4 inhibitors, these drugs have achieved good clinical efficacy [43, 44]. The immune checkpoint pathway is related to tumor immune escape. after being activated, this pathway can make T cells fail and can’t kill tumor cells normally, thus suppressing tumor immunity. Our research revealed that there was a positive correlation between ARHGAP4 and tumor immune checkpoints such as PDCD1, PDCD-L1 (CD274), CTLA-4, LAG3, TIGIT, OX40 (TNFSF4), B7-H3 (CD276), BTLA and TNFRSF9, especially with the expression of PDCD1 and CTLA-4 genes. Immunotherapy, based on PDCD1 and CTLA-4 pathways, has achieved excellent results in the treatment of a variety of cancers, including KIRC [45, 46, 47]. In addition, studies have shown that the survival time of patients can be significantly prolonged when immune checkpoint inhibitors (Navulizumab) and anti-vascular endothelial growth factor drugs (Carbotinib) are used together [48]. Beyond that, some good immunotherapeutic potential drugs including LAG3, TIGIT, OX40 (TNFSF4), B7-H3 (CD276), BTLA and TNFRSF9 are under research and development [25]. The correlation between ARHGAP4 and these immune checkpoints genes suggested that it may affect the immune escape pathway of tumors and may be a therapeutic target to improve the efficacy of KIRC tumor immunotherapy.
However, there are some limitations in our research. First of all, it is necessary to verify the clinical value of ARHGAP4 in KIRC through a larger cohort and more detailed clinical data, because our database-based analysis can’t determine whether other diseases affect the expression of ARHGAP4. Secondly, we need to further verify the role of ARHGAP4 in KIRC in vivo. Although our results suggest that ARHGAP4 may play a role in the immune infiltration of KIRC, the pathway and mechanism of its effect are not clear, and we will verify it through more experiments in the future.
5.Conclusion
In summary, our investigation explored the progress, diagnostic and prognostic value of ARHGAP4 in KIRC patients for the first time. ARHGAP4 was upregulated in KIRC patients and indicates a poor prognosis. ARHGAP4 was mainly related to immune and transport-related functions in KIRC. ARHGAP4 was significantly correlated with some kinds of immune cells, suggesting that it may affect the immune infiltration of KIRC. In addition, ARHGAP4 was positively correlated with a variety of immune checkpoint genes, which may affect the prognosis and treatment of KIRC. Therefore, ARHGAP4 may be a biomarker of KIRC, which is beneficial to the diagnosis of tumors. At the same time, it was related to the immune cell infiltration of tumors and may be a therapeutic target to improve the efficacy of immunotherapy.
Author contributions
Conception: Xuesong Xiao.
Interpretation or analysis of data: Xuesong Xiao, Xiaofei Lv, Tianyu Lin.
Preparation of the manuscript: Xuesong Xiao.
Revision for important intellectual content: Jianqiao Li, Rui Wang, Shaoping Tian, Xinyu Liu, Shiming Liu, Huamao Jiang.
Supervision: Yong Wang, Dan Yue.
Supplementary data
The supplementary files are available to download from http://dx.doi.org/10.3233/CBM-230388.
Acknowledgments
This work was supported by the National Natural Science Foundation of China (grant number 81872078 and 82203360), the Natural Science Foundation of Tianjin (grant number 21JCYBJC01430), National Training Program of innovation and Entrepreneurship for undergraduates (grant number 202310062054 and 202310062006), and Education Teaching Reform Research Project (2023jxyb05).
References
[1] | B. Escudier, C. Porta, M. Schmidinger, N. Rioux-Leclercq, A. Bex, V. Khoo, V. Grunwald, S. Gillessen, A. Horwich and E.G. Comm, Renal cell carcinoma: ESMO Clinical Practice Guidelines for diagnosis, treatment and follow-up, Annals of Oncology 30: ((2019) ), 706–720. |
[2] | C.J. Chen and Y.Y. Sheng, Prognostic Impact of MITD1 and Associates With Immune Infiltration in Kidney Renal Clear Cell Carcinoma, Technology in Cancer Research & Treatment 20: ((2021) ). |
[3] | H. Moch, A.L. Cubilla, P.A. Humphrey, V.E. Reuter and T.M. Ulbright, The 2016 WHO Classification of Tumours of the Urinary System and Male Genital Organs-Part A: Renal, Penile, and Testicular Tumours, Eur Urol 70: ((2016) ), 93–105. |
[4] | W.M. Linehan, Genetic basis of kidney cancer: role of genomics for the development of disease-based therapeutics, Genome Res 22: ((2012) ), 2089–100. |
[5] | F.E. Vera-Badillo, A.J. Templeton, I. Duran, A. Ocana, P. de Gouveia, P. Aneja, J.J. Knox, I.F. Tannock, B. Escudier and E. Amir, Systemic Therapy for Non-clear Cell Renal Cell Carcinomas: A Systematic Review and Meta-analysis, European Urology 67: ((2015) ), 740–749. |
[6] | S. Fernandez-Pello, F. Hofmann, R. Tahbaz, L. Marconi, T.B. Lam, L. Albiges, K. Bensalah, S.E. Canfield, S. Dabestani, R.H. Giles, M. Hora, M.A. Kuczyk, A.S. Merseburger, T. Powles, M. Staehler, A. Volpe, B. Ljungberg and A. Bex, A Systematic Review and Meta-analysis Comparing the Effectiveness and Adverse Effects of Different Systemic Treatments for Non-clear Cell Renal Cell Carcinoma, European Urology 71: ((2017) ), 426–436. |
[7] | B. Kasenda, J. Larkin and M. Gore, Immunotherapies in Early and Advanced Renal Cell Cancer, Immuno-Oncology 42: ((2015) ), 1–10. |
[8] | C. Jochems and J. Schlom, Tumor-infiltrating immune cells and prognosis: the potential link between conventional cancer therapy and immunity, Experimental Biology and Medicine 236: ((2011) ), 567–579. |
[9] | W.H. Xu, Y. Xu, J. Wang, F.N. Wan, H.K. Wang, D.L. Cao, G.H. Shi, Y.Y. Qu, H.L. Zhang and D.W. Ye, Prognostic value and immune infiltration of novel signatures in clear cell renal cell carcinoma microenvironment, Aging-Us 11: ((2019) ), 6999–7020. |
[10] | D.A. Braun, Z. Bakouny, L. Hirsch, R. Flippot, E.M. Van Allen, C.J. Wu and T.K. Choueiri, Beyond conventional immune-checkpoint inhibition – novel immunotherapies for renal cell carcinoma, Nat Rev Clin Oncol 18: ((2021) ), 199–214. |
[11] | S. Zhang, E. Zhang, J. Long, Z. Hu, J. Peng, L. Liu, F. Tang, L. Li, Y. Ouyang and Z. Zeng, Immune infiltration in renal cell carcinoma, Cancer Sci 110: ((2019) ), 1564–1572. |
[12] | D.L. Vogt, C.D. Gray, W. Young, S.A. Orellana and A.T. Malouf, ARHGAP4 is a novel RhoGAP that mediates inhibition of cell motility and axon outgrowth, Molecular and Cellular Neuroscience 36: ((2007) ), 332–342. |
[13] | Y. Shen, G. Chen, L. Zhuang, L. Xu, J. Lin and L. Liu, ARHGAP4 mediates the Warburg effect in pancreatic cancer through the mTOR and HIF-1alpha signaling pathways, Onco Targets Ther 12: ((2019) ), 5003–5012. |
[14] | Y. Shen, L. Xu, Z. Ning, L. Liu, J. Lin, H. Chen and Z. Meng, ARHGAP4 regulates the cell migration and invasion of pancreatic cancer by the HDAC2/beta-catenin signaling pathway, Carcinogenesis 40: ((2019) ), 1405–1414. |
[15] | Y. Shen, G. Chen, H. Gao, Y. Li, L. Zhuang, Z. Meng and L. Liu, miR-939-5p Contributes to the Migration and Invasion of Pancreatic Cancer by Targeting ARHGAP4, Onco Targets Ther 13: ((2020) ), 389–399. |
[16] | N. Kang, T.S. Matsui, S. Liu and S. Deguchi, ARHGAP4-SEPT2-SEPT9 complex enables both up- and down-modulation of integrin-mediated focal adhesions, cell migration, and invasion, Mol Biol Cell 32: ((2021) ), ar28. |
[17] | F. Liu, H. Guo, M. Ou, X. Hou, G. Sun, W. Gong, H. Jing, Q. Tan, W. Xue, Y. Dai and W. Sui, ARHGAP4 mutated in a Chinese intellectually challenged family, Gene 578: ((2016) ), 205–9. |
[18] | L. Anesi, P. de Gemmis, D. Galla and U. Hladnik, Two new large deletions of the AVPR2 gene causing nephrogenic diabetes insipidus and a review of previously published deletions, Nephrology Dialysis Transplantation 27: ((2012) ), 3705–3712. |
[19] | Y. Bai, Y. Chen and X. Kong, Contiguous 22.1-kb deletion embracing AVPR2 and ARHGAP4 genes at novel breakpoints leads to nephrogenic diabetes insipidus in a Chinese pedigree, BMC Nephrol 19: ((2018) ), 26. |
[20] | M. Fujimoto, K. Imai, K. Hirata, R. Kashiwagi, Y. Morinishi, K. Kitazawa, S. Sasaki, T. Arinami, S. Nonoyama and E. Noguchi, Immunological profile in a family with nephrogenic diabetes insipidus with a novel 11 kb deletion in AVPR2 and ARHGAP4 genes, BMC Med Genet 9: ((2008) ), 42. |
[21] | S.Y. Cho, C.Y. Law, K.L. Ng and C.W. Lam, Novel large deletion in AVPR2 gene causing copy number variation in a patient with X-linked nephrogenic diabetes insipidus, Clinica Chimica Acta 455: ((2016) ), 84–86. |
[22] | H. Zeng, X.D. Song, J.R. Ji, L.Y. Chen, Q.M. Liao and X.L. Ma, HPV infection related immune infiltration gene associated therapeutic strategy and clinical outcome in HNSCC, Bmc Cancer 20: ((2020) ). |
[23] | T. Makino, S. Kadomoto, K. Izumi and A. Mizokami, Epidemiology and Prevention of Renal Cell Carcinoma, Cancers 14: ((2022) ). |
[24] | P.C. Barata and B.I. Rini, Treatment of renal cell carcinoma: Current status and future directions, Ca-a Cancer Journal for Clinicians 67: ((2017) ), 507–524. |
[25] | P. Darvin, S.M. Toor, V.S. Nair and E. Elkord, Immune checkpoint inhibitors: recent progress and potential biomarkers, Experimental and Molecular Medicine 50: ((2018) ). |
[26] | G. Morad, B.A. Helmink, P. Sharma and J.A. Wargo, Hallmarks of response, resistance, and toxicity to immune checkpoint blockade, Cell 185: ((2022) ), 576. |
[27] | H.O. Alsaab, S. Sau, R. Alzhrani, K. Tatiparti, K. Bhise, S.K. Kashaw and A.K. Iyer, PD-1 and PD-L1 Checkpoint Signaling Inhibition for Cancer Immunotherapy: Mechanism, Combinations, and Clinical Outcome, Front Pharmacol 8: ((2017) ), 561. |
[28] | Y. Diesendruck and I. Benhar, Novel immune check point inhibiting antibodies in cancer therapy-Opportunities and challenges, Drug Resist Updat 30: ((2017) ), 39–47. |
[29] | G. Courthod, M. Tucci, M. Di Maio and G.V. Scagliotti, Papillary renal cell carcinoma: A review of the current therapeutic landscape, Crit Rev Oncol Hematol 96: ((2015) ), 100–12. |
[30] | M.S. Fu, S.X. Pan, X.Q. Cai, Y.X. Hu, W.J. Zhang and Q.C. Pan, Analysis of ARHGAP4 Expression With Colorectal Cancer Clinical Characteristics and Prognosis, Front Oncol 12: ((2022) ), 899837. |
[31] | T. Sera, T. Sakuma, K. Maruo, G. Tsujio, Y. Yamamoto, A. Sugimoto, S. Kushiyama, S. Togano, K. Kuroda, H. Kasashima, M. Ohira and K. Maeda, Candidate Oncogenes, ARHGAP4, NOS3, and OR51B5, for the Development of Scirrhous-type Gastric Cancer, Anticancer Res 42: ((2022) ), 5195–5203. |
[32] | X. Lu, Y. Ying, W. Zhang, J. Zhang, R. Li and W. Wang, Prognosis, immune microenvironment, and personalized treatment prediction in Rho GTPase-activating protein 4-mutant cervical cancer: Computer strategies for precision oncology, Life Sci 315: ((2023) ), 121360. |
[33] | S. L’Esperance, I. Popa, M. Bachvarova, M. Plante, N. Patten, L. Wu, B. Tetu and D. Bachvarov, Gene expression profiling of paired ovarian tumors obtained prior to and following adjuvant chemotherapy: Molecular signatures of chemoresistant tumors, International Journal of Oncology 29: ((2006) ), 5–24. |
[34] | M. Reina-Campos, N.E. Scharping and A.W. Goldrath, CD8(+) T cell metabolism in infection and cancer, Nat Rev Immunol 21: ((2021) ), 718–738. |
[35] | B.J. Laidlaw, J.E. Craft and S.M. Kaech, The multifaceted role of CD4(+) T cells in CD8(+) T cell memory, Nat Rev Immunol 16: ((2016) ), 102–11. |
[36] | S. Su, S. Akbarinejad and L. Shahriyari, Immune classification of clear cell renal cell carcinoma, Scientific Reports 11: ((2021) ). |
[37] | K. Nishida, A. Kawashima, T. Kanazawa, Y. Kidani, T. Yoshida, M. Hirata, K. Yamamoto, Y. Yamamoto, M. Sawada, R. Kato, T. Kato, K. Hatano, T. Ujike, K. Fujita, M. Uemura, A. Morimoto-Okazawa, K. Iwahori, M. Yamasaki, N. Ohkura, S. Sakaguchi, N. Nonomura, Y. Doki and H. Wada, Clinical importance of the expression of CD4+CD8+ T cells in renal cell carcinoma, International Immunology 32: ((2020) ), 347–357. |
[38] | S. Dai, H. Zeng, Z. Liu, K. Jin, W. Jiang, Z. Wang, Z. Lin, Y. Xiong, J. Wang, Y. Chang, Q. Bai, Y. Xia, L. Liu, Y. Zhu, L. Xu, Y. Qu, J. Guo and J. Xu, Intratumoral CXCL13+CD8+T cell infiltration determines poor clinical outcomes and immunoevasive contexture in patients with clear cell renal cell carcinoma, Journal for ImmunoTherapy of Cancer 9: ((2021) ). |
[39] | S.K. Wculek, F.J. Cueto, A.M. Mujal, I. Melero, M.F. Krummel and D. Sancho, Dendritic cells in cancer immunology and immunotherapy, Nat Rev Immunol 20: ((2020) ), 7–24. |
[40] | R. Tokunaga, M. Naseem, J.H. Lo, F. Battaglin, S. Soni, A. Puccini, M.D. Berger, W. Zhang, H. Baba and H.J. Lenz, B cell and B cell-related pathways for novel cancer treatments, Cancer Treatment Reviews 73: ((2019) ), 10–19. |
[41] | C. Ngambenjawong, H.H. Gustafson and S.H. Pun, Progress in tumor-associated macrophage (TAM)-targeted therapeutics, Advanced Drug Delivery Reviews 114: ((2017) ), 206–221. |
[42] | P.X. Liew and P. Kubes, The Neutrophil’s Role during Health and Disease, Physiological Reviews 99: ((2019) ), 1223–1248. |
[43] | M.C. Kim, Z. Jin, R. Kolb, N. Borcherding, J.A. Chatzkel, S.M. Falzarano and W. Zhang, Updates on Immunotherapy and Immune Landscape in Renal Clear Cell Carcinoma, Cancers (Basel) 13: ((2021) ). |
[44] | J.J. Hsieh, M.P. Purdue, S. Signoretti, C. Swanton, L. Albiges, M. Schmidinger, D.Y. Heng, J. Larkin and V. Ficarra, Renal cell carcinoma, Nat Rev Dis Primers 3: ((2017) ), 17009. |
[45] | J.A. Marin-Acevedo, B. Dholaria, A.E. Soyano, K.L. Knutson, S. Chumsri and Y.Y. Lou, Next generation of immune checkpoint therapy in cancer: new developments and challenges, Journal of Hematology & Oncology 11: ((2018) ). |
[46] | J.J. Havel, D. Chowell and T.A. Chan, The evolving landscape of biomarkers for checkpoint inhibitor immunotherapy, Nature Reviews Cancer 19: ((2019) ), 133–150. |
[47] | S.H. Baumeister, G.J. Freeman, G. Dranoff and A.H. Sharpe, Coinhibitory Pathways in Immunotherapy for Cancer, Annual Review of Immunology 34: ((2016) ), 539–573. |
[48] | A.M. Kase, D.J. George and S. Ramalingam, Clear Cell Renal Cell Carcinoma: From Biology to Treatment, Cancers (Basel) 15: ((2023) ). |