Cuproptosis-related gene-located DNA methylation in lower-grade glioma: Prognosis and tumor microenvironment
Abstract
Cuproptosis a novel copper-dependent cell death modality, plays a crucial part in the oncogenesis, progression and prognosis of tumors. However, the relationships among DNA-methylation located in cuproptosis-related genes (CRGs), overall survival (OS) and the tumor microenvironment remain undefined. In this study, we systematically assessed the prognostic value of CRG-located DNA-methylation for lower-grade glioma (LGG). Clinical and molecular data were sourced from The Cancer Genome Atlas (TCGA) and Gene Expression Omnibus (GEO) databases. We employed Cox hazard regression to examine the associations between CRG-located DNA-methylation and OS, leading to the development of a prognostic signature. Kaplan-Meier survival and time-dependent receiver operating characteristic (ROC) analyses were utilized to gauge the accuracy of the signature. Gene Set Enrichment Analysis (GSEA) was applied to uncover potential biological functions of differentially expressed genes between high- and low-risk groups. A three CRG-located DNA-methylation prognostic signature was established based on TCGA database and validated in GEO dataset. The 1-year, 3-year, and 5-year area under the curve (AUC) of ROC curves in the TCGA dataset were 0.884, 0.888, and 0.859 while those in the GEO dataset were 0.943, 0.761 and 0.725, respectively. Cox-regression-analyses revealed the risk signature as an independent risk factor for LGG patients. Immunogenomic profiling suggested that the signature was associated with immune infiltration level and immune checkpoints. Functional enrichment analysis indicated differential enrichment in cell differentiation in the hindbrain, ECM receptor interactions, glycolysis and reactive oxygen species pathway across different groups. We developed and verified a novel CRG-located DNA-methylation signature to predict the prognosis in LGG patients. Our findings emphasize the potential clinical implications of CRG-located DNA-methylation indicating that it may serve as a promising therapeutic target for LGG patients.
1.Introduction
Lower-grade glioma (LGG) represents around 22% of all adult brain tumors [1]. Although clinical histopathological characteristics and traditional molecular markers like isocyanate dehydrogenase (IDH) mutations and 1p/19q co-deletion status help to further differentiate most LGGs and enhance patient prognosis [2], the progression-free survival for cases with inoperable or residual disease necessitating therapy is only about 40%, resulting in a poor prognosis [3]. Multiple studies have developed biomarkers that improve LGG prognostication. For example, Song et al. defined indent a 21-mRNA signature which has reliable prognostic value for LGGs and might facilitate the effective stratification and individualized management of patients [4]. Zhang et al. constructed a six immune-related gene signature and established a prognostic nomogram effective in risk stratification and prediction of overall survival in LGG [5]. Guo et al. found a nomogram based on methylation-driven genes combined with clinicopathological parameters for predicting prognosis in LGG [6]. Nevertheless, even with molecular and clinicopathological factors considered, their ability to precisely forecast patient outcomes remains limited. Consequently, the development and validation of novel practical molecular signatures to better assess prognosis and facilitate personalized therapy in LGG patients remain a pressing need.
Copper is a vital nutrient that plays a crucial role in numerous biological processes. Its importance is evident in cell proliferation, where it contributes to the division and multiplication of cells, enabling growth and tissue repair [7]. Copper is also integral to the process of cell growth, as it regulates the synthesis and activation of various enzymes and proteins [8]. Furthermore, copper is implicated in metabolism, where it functions as a cofactor for essential enzymes involved in energy production and the breakdown of various nutrients [9]. Cuproptosis, a novel type of non-apoptotic programmed cell death, plays a critical role in cell biology [10] and has been connected to numerous death pathways, such as apoptosis, autophagy, kinase signaling and anti-angiogenesis [11]. Recent studies consistently illuminated that copper metabolism and cuproptosis-related genes (CRGs) might influence tumor progression and act as potential indicators for prognosis [12, 13, 14]. For instance, FDX1 has been found to impact tumor cell metabolism and immune microenvironment [15, 16], while LIPT1 expression is strongly correlated with immune infiltration and can predict melanoma prognosis [12].
Past research has shown that DNA-methylation status proves to be more dependable than gene expression when it comes to tumour prognosis [17, 18], playing an influential role in tumour development [19], patient stratification [20] and targeted treatment [21]. Owing to its high dependability, prevalence and accessibility, DNA-methylation is extensively utilized as a prognostic biomarker for ovarian cancer [22], cutaneous melanoma [23], vulvar squamous cell carcinoma [24] and lower-grade glioma [25]. At present, several studies have demonstrated the impact of CRGs on the prognosis of LGG. For example, Bao et al. established a CRG risk model for LGG patients’ therapy status and prognosis and highlight the potential clinical implications of CRGs, suggesting that cuproptosis may be the potential therapeutic target for patients with LGG [26]. Chen et al. found that high-risk individuals in LGG can be reliably identified by the model based on cuproptosis-related genes [27]. However, the prognostic performances of CRG-located DNA-methylation in LGG remain uncertain, making it crucial to further investigate the connection between CRG-located DNA-methylation and LGG.
Here, we aimed to investigate the significance of CRG-located DNA-methylation in the prognosis and tumor microenvironment (TME) infiltration in LGG. Using LGG DNA-methylation-level, gene expression data, clinical data, and combined CRGs, we established a CRG-located DNA-methylation signature. After validation, we also examined patient prognosis and immune status based on this signature and conducted functional enrichment analysis to uncover possible mechanisms of action. Our findings provide valuable insights into CRG-located DNA-methylation in LGG and an effective prognosis prediction tool for patients.
2.Materials and methods
2.1Acquisition of dataset from public online databases and preprocessing
We collected DNA-methylation data, transcriptional profiles and clinical data from the TCGA database. The GEO database (GSE104293,
Table 1
Three CRG-located DNA-methylation sites that was significantly related to survival of LGG patients in TCGA dataset
ID | Location | Gene | Gene location | Type | ||
---|---|---|---|---|---|---|
cg04174091 | chr11: 123064514–123064515 | HSPA8 | chr11: 123057489–123063230 | S_Shore | 2.39E-09 | 1.54E-09 |
cg07562918 | chr9: 21994436–21994437 | CDKN2A | chr10: 21967752–21995301 | Island | 1.92E-10 | 1.48E-09 |
cg08795279 | chr16: 56589904–56589905 | MT3 | chr16: 56589074–56591088 | Island | 2.21E-13 | 1.96E-04 |
1. in univariate-Cox-regression-analysis; 2. in multivariate-Cox-regression-analysis.
2.2CRG-located DNA-methylation signature construction
We conducted a univariate-Cox-regression-analysis to preliminarily identify 414 prognostic CRG DNA-methylation sites related to OS from 1116 DNA-methylation site located in the CRGs using R package “survival” [31]. A multivariate-Cox-regression-analysis was then performed to estimate and weight the regression coefficients of the prognostic DNA methylation sites, and construct all possible models of 2–3 sites in 414 prognostic CRG DNA-methylation sites. Finally, a CRG-located DNA-methylation signature was selected as the best prognostic model for predicting survival through ROC analyses. The risk scores for each LGG patient were calculated based on the CRG-located DNA methylation signature.
2.3Validation of the CRG-located DNA-methylation signature
We also assessed the differences in OS among different subgroups using Kaplan-Meier survival curves. To verify the independence of the signature in predicting prognosis, Cox-regression-analyses of the risk score and clinical characteristics were performed. To individualize the predicted survival probability of LGG patients, a nomogram of clinical characteristics and the riskscore was used to verify the signature. The specific methods are the same as in the literature [32].
2.4Evaluation of the immunogenomic landscape
We used the ESTIMATE [33] and CIBERSORT [34] algorithm to calculate vaious immune-related score, and 22 immune cells infiltration for each CM patient. The Spearman correlation of riskscore with immunogenomic landscape and ICGs [32, 35, 36] were calculated. The difference in the immunogenomic landscape and ICGs expression between high- and low-risk groups were compared using Mann-Whitney U-test.
2.5Functional enrichment analysis
To examine the difference in biological function between high- and low-risk groups, GSEA was performed using the sample groups and gene expression data from TCGA database. “c5.go.v2022.1.Hs.symbols.gmt”, “c2.cp.kegg.v2022.1.Hs.symbols.gmt” were chosen as the gene sets database. Setting the permutations value to default value 1000 for computing Normalized enrichment score (NES). Signaling pathways with adjusted
2.6Statistical analysis
Statistical analyses in this study were conducted using R.4.0.3. Correlation coefficients were assessed using Spearman analysis. The Kaplan-Meier analysis was conducted to demonstrate the difference in prognosis between the two groups, and Wilcoxon-rank test was employed to identify the significance of differences. The Mann-Whitney-U test was conducted to illustrate the variables between the two groups.
3.Results
3.1Screening and construction of prognostic signature based on CRG-located DNA-methylation
We extracted 96 CRGs and their corresponding 1116 DNA-methylation sites. After filtering for 414 DNA-methylation sites significantly correlated with patient prognosis (
3.2Evaluating the established CRG-located DNA-methylation signature
The Cox regression analysis, which utilized the riskscore as a consecutive variable demonstrated that our CRG-located DNA-methylation signature was significantly correlated to the patients’ OS (
Figure 1.
Correlation between the signature and OS in the TCGA cohort. (A) The distribution of risk score. (B) The distribution of OS and survival status of each patient as risk score increases. Patients were divided into high- and low-risk groups using the median score as the cut-off value. (C) The distribution of OS and survival status of each patient as DNA methlation level increases. (D) DNA methylation levels of three DNA methylation sites in patients with short (OS
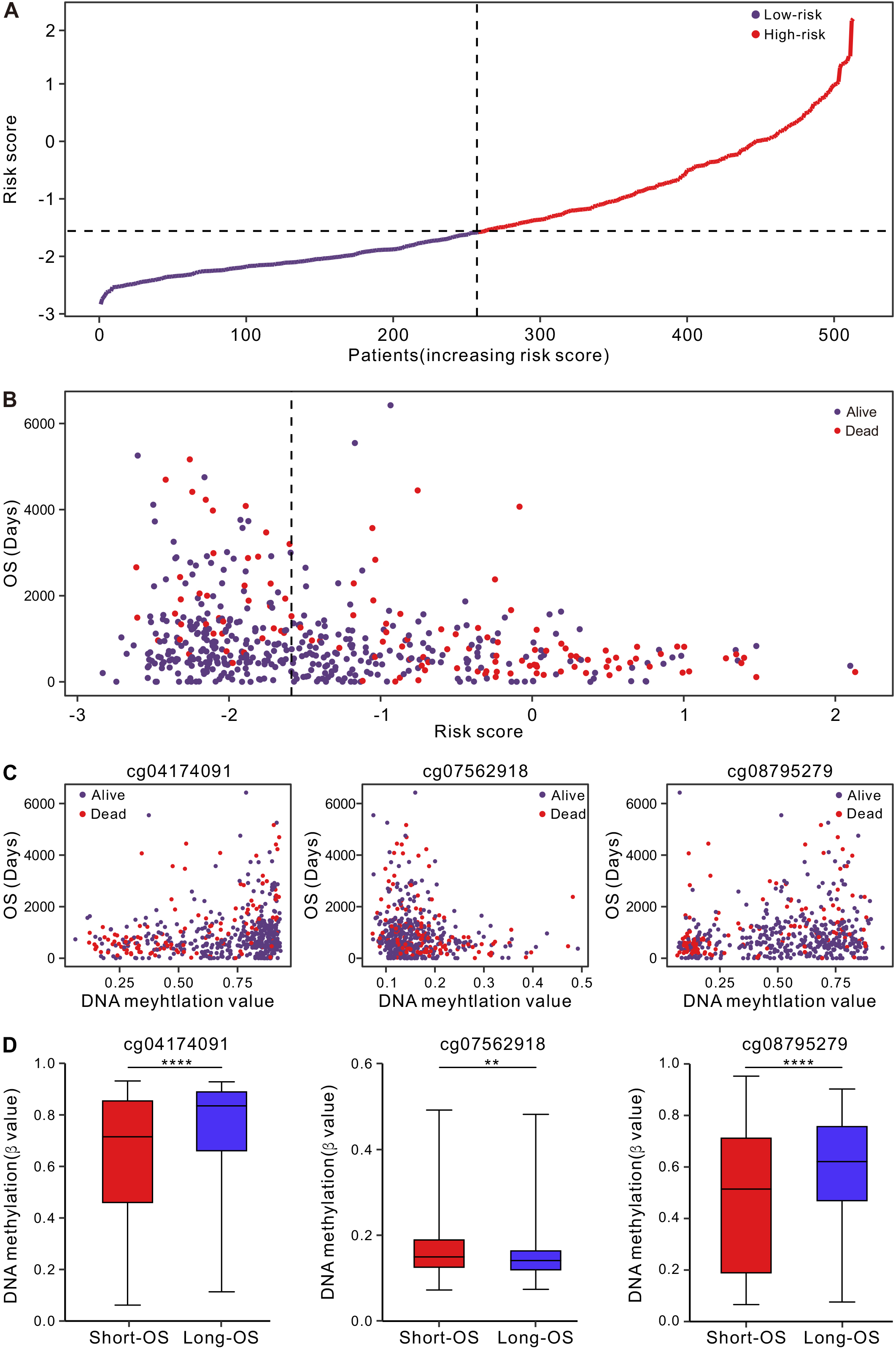
Figure 2.
Kaplan-Meier and ROC curves of the CRG-located DNA-methylation signature in the discovery (A-B) and validation sets (C-D). Kaplan-Meier analysis combined with Wilcoxon test was used to visualize and compare the OS in low- and high-risk groups. AUC values for the 1, 3, and 5-year survival to show the prognostic performance of the signature.
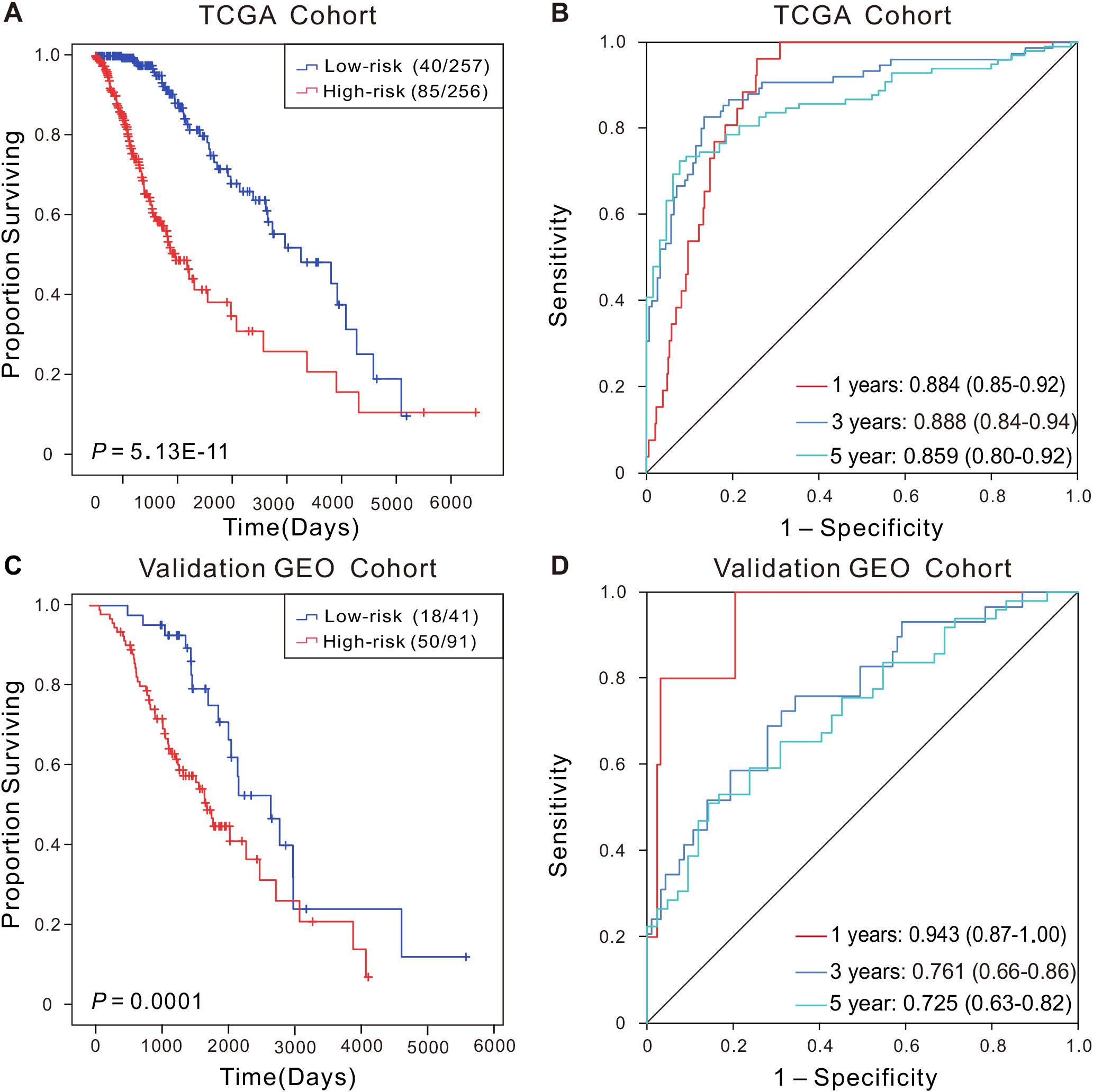
Kaplan-Meier-survival-analysis indicated that the high-risk group experienced poorer OS (
To further confirm the prognostic value of this CRG-located DNA-methylation signature, we evaluated its impact on an independent dataset of LGG patients from GEO database. As anticipated, Kaplan-Meier-analysis revealed that cases in the high-risk group had significantly shorter OS (
3.3Relationships between CRG-located DNA-methylation signature and clinicopathologic factors
Many clinical factors can influence patient survival [37]. To assess the connection between the CRG-located DNA-methylation signature and clinical characteristics, we in turn examined the differences in clinical features between the two group and the performance of the signature among patients categorized by different clinical aspects in TCGA. The findings showed no notable distinctions between patients categorized based on gender in both analysis (Fig. 3A and G). Older individuals (
Figure 3.
Association between the CRG-located DNA-methylation signature and clinical factors in the TCGA set. (A–F) The constituent ratio of clinical factors in the low- or high-risk group, and distribution of risk-score in different groups based on pathological characteristics. ****P < 0.0001, ***P < 0.001, **P < 0.01, *P < 0.05. (G–L) Kaplan-Meier curves of LGG patients stratified by clinical factors.
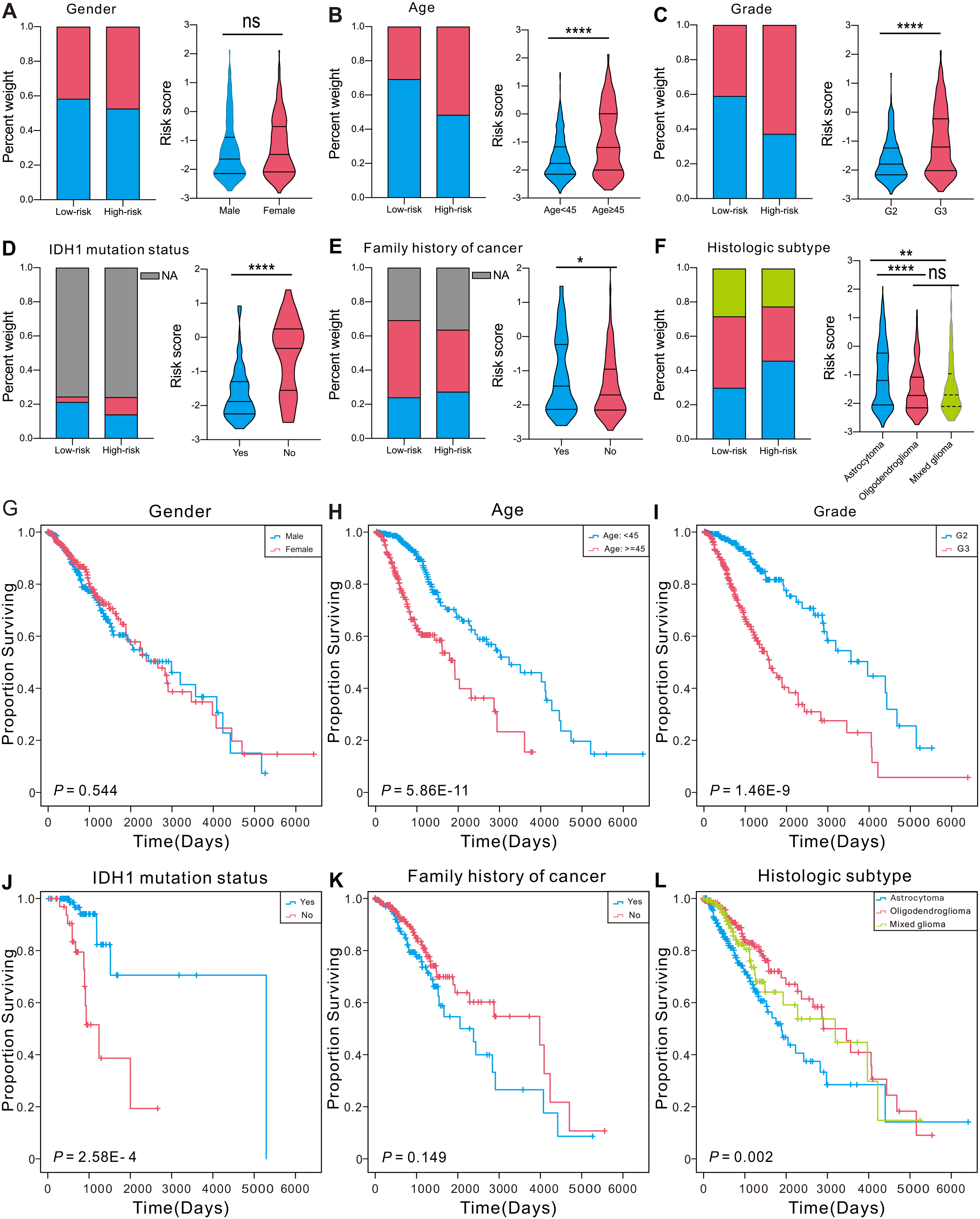
3.4Analysis of independence and nomogram construction
Figure 4.
The Cox regression (A-B) of risk scores and clinical characteristics. ****P < 0.0001, ***P < 0.001, **P < 0.01, *P < 0.05. (C) The nomogram for OS prediction, which consisted of age, grade, IDH1 mutation status, histologic subtype and the riskscore.
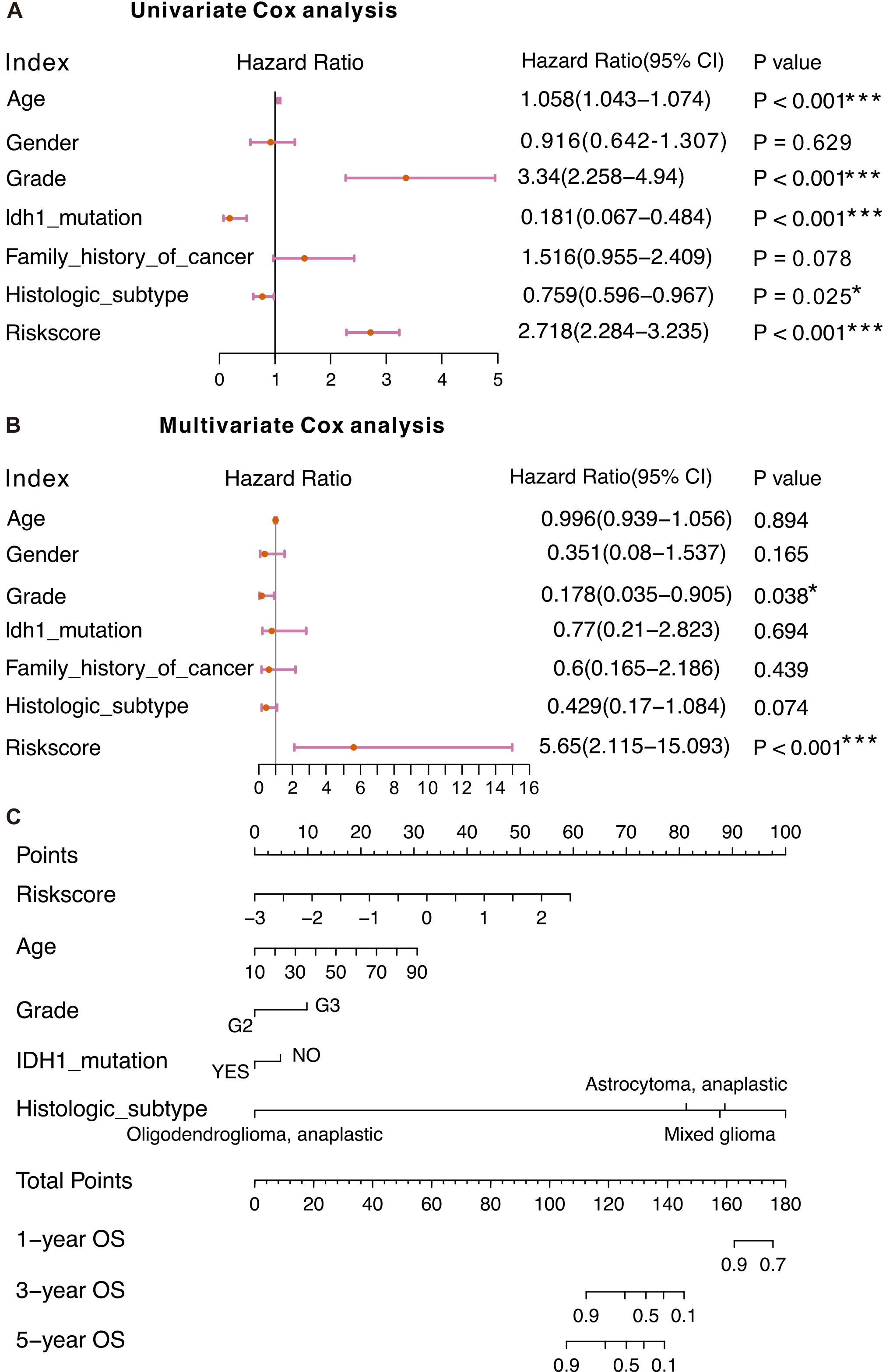
To investigate the independence of the CRG-located DNA-methylation signature from other clinical factors, we conducted Cox-regression-analyses (Fig. 4A and B). Univariate-Cox-regression results uncovered that riskscore, age, grade, IDH1 mutation status, and histologic subtype were significantly associated with LGG patients’ OS. Multivariate-Cox-regression-analysis demonstrated that the riskscore remained significantly connected to patients’ OS, suggesting the signature could function as an independent prognostic biomarker for OS in LGG.
To precisely predict survival probability, we devised a nomogram for OS estimation by incorporating the signature and other clinicopathological features, such as age, grade, IDH1 mutation status and histologic subtype (Fig. 4C). The findings indicated that the predictive nomogram was valuable for guiding clinical management.
3.5Association of CRG-located DNA-methylation signature with immune landscape
In order to investigate the differences in the immune microenvironment between the two groups, we studied the connection between the immunogenomic profile and riskscore. Our findings indicated a negatively correlation between the risk-score and various characteristics, including ESTIMATE scores, immune scores, stromal scores, and specific cell types such as Plasma cells, CD8 T cells, naive CD4 T cells, regulatory T cells, M0 Macrophages, M2 Macrophages, resting Mast cells, activated Mast cells, and Neutrophils (Fig. 5A). The CIBERSORT analysis supported the existence of unique ESTIMATE scores, immune scores, stromal scores for the high- and low-risk groups (Fig. 5B). The analysis of immune cell infiltration in the TME showed that high-risk patients exhibited increased infiltration of CD8
Figure 5.
Estimated infiltration level of tumor-infiltrating leukocytes (TILs) in individuals based on the DNA-methylation signature. (A) Correlation between signature and immune infiltration. (B-C) The differences in various immune score and infiltration levels of 22 immune cells in different groups. ****P < 0.0001, ***P < 0.001, **P < 0.01, *P < 0.05.
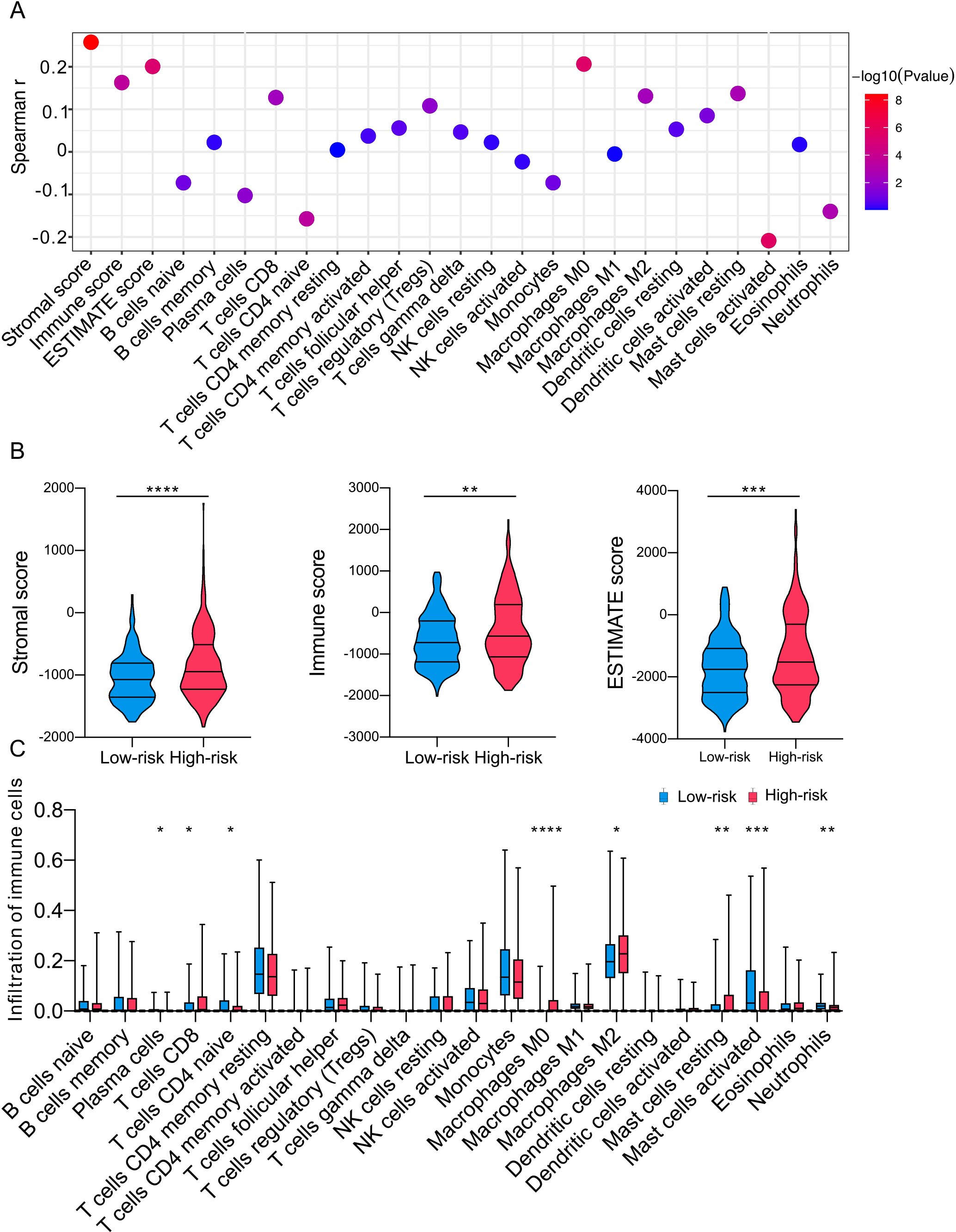
Figure 6.
Correlation analysis of the signature. (A) Correlation of CRG-located DNA-methylation signature with ICGs. The colors above represent the Spearman’s correlation coefficients, and the colors below represent
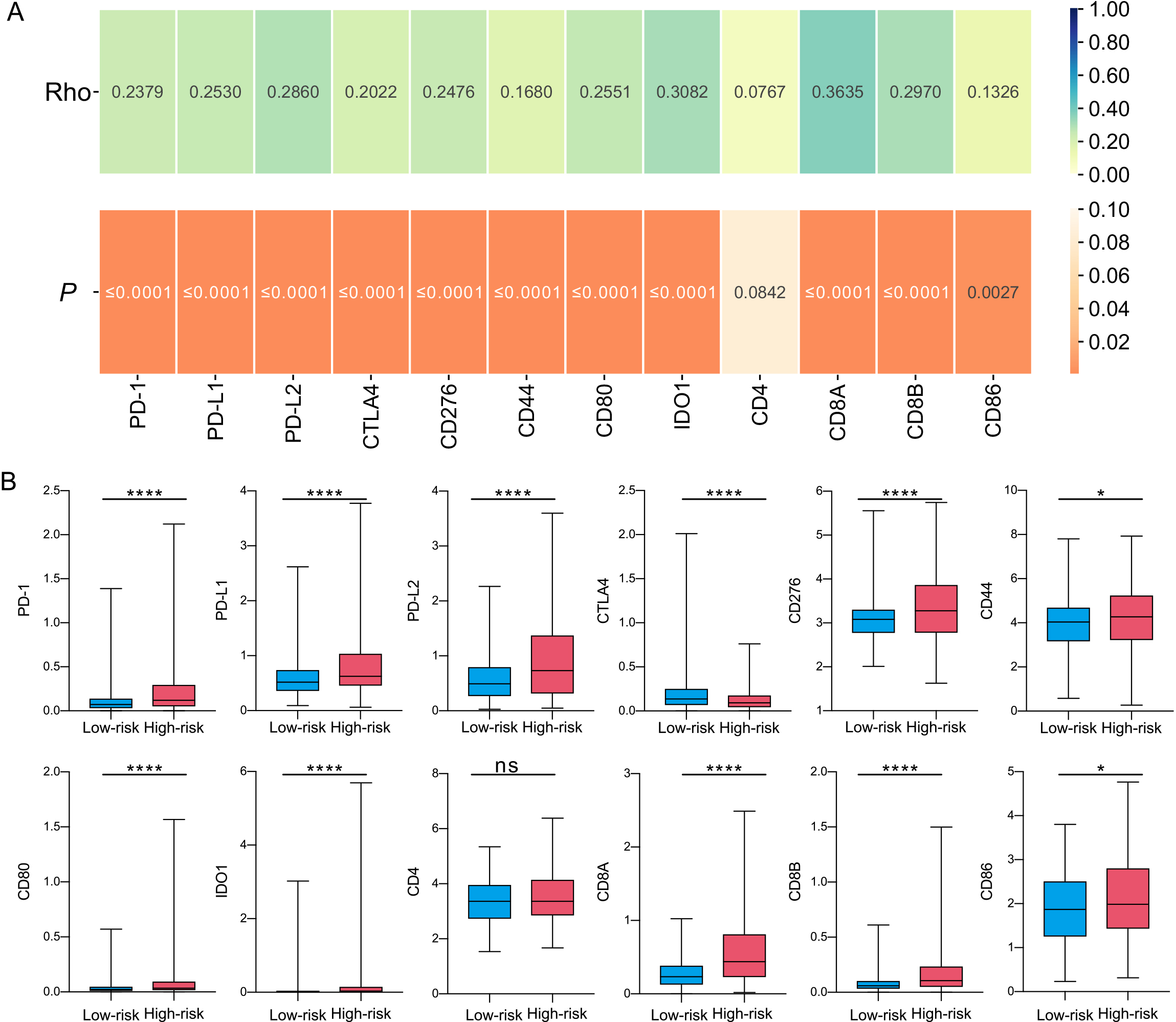
As the relevance of immunotherapy in clinical treatment is undeniable, it is essential to investigate the distinctions in immune checkpoint gene (ICG) expression between the high- and low-risk categories. Our study discovered a direct relationship between the riskscore and ICG expression (Fig. 6A), and the demonstrated notably increased ICG expression in comparison to the low-risk group (Fig. 6B). This implies that the high-risk group may be more closely linked to compromised tumor immune, which in turn influences patient outcomes.
3.6Functional enrichment analyses
We further investigated the disparities in signaling pathways between high- and low-risk patients by employing GSEA to reveal specific enrichment pathways. GO analysis demonstrated that significant activation occurred in low-risk patients regarding hindbrain cell differentiation, cytoplasmic translation, positive regulation of nuclear transcriptional mRNA catabolic predenylation dependent decay belonging to biological process aspect, protein phosphatase type 2a complex belonging to cellular component aspect, and demethylase activity belonging to molecular function aspect (Fig. S5A). KEGG analysis exposed enriched pathways in the high-risk group, such as pantothenate and co-biosynthesis pathway, drug metabolism and other enzymes pathway, as well as ECM receptor interactions (Fig. S5B). Lastly, Hallmark analysis revealed an overrepresentation of peroxisome, glycolysis, reactive oxygen species pathway and estrogen response late in high-risk group (Fig. S5C).
3.7Correlation between gene expression and methylation status
Epigenetic modifications have been demonstrated to affect RNA content and in turn plays a key role in the development of tumour. The genes coverd cg04174091, cg07562918, cg08795279 were HSPA8, CDKN2A, and MT3. Our analysis found that the methylation-levels of cg07562918 and cg08795279 were found to be significantly negatively linked with their gene expression (Fig. S6B and C). Meanwhile, we found that HSPA8 and CDKN2A expression were significantly higher in LGG than the normal tissues, while MT3 expression was lower (Fig. S6D–F). In addition, the combined signature of these three methylations can offer better potential than single DNA methylation in LGG (Fig. S7).
4.Discussion
Copper is an essential element involved in cell proliferation and death and serves as a crucial cofactor for enzymes and transport proteins [38]. Recently, several researches have indicated a significant increase in copper levels in malignancies and the vital role of cuproptosis in cancer progression [15]. Consequently, CRG-located DNA-methylation warrants attention and prognostic biomarkers based on CRG-located DNA-methylation may help improve the survival rate of LGG patients. To date, no research has focused on prognostic biomarkers based on CRG-located DNA-methylation. In the current study, we constructed a CRG-located DNA-methylation signature for LGG, which would probably be the first risk-assessment signature of its kind and could lay the foundation for novel therapeutic targets in prognosis and clinical treatment.
Aberrant DNA-methylation has been linked to the development of various malignancies [39, 40]. Epigenetic modifications have been demonstrated to affect RNA content and in turn plays a key role in the development of tumour [41]. Interestingly, the methylation-levels of cg07562918 and cg08795279 were found to be significantly negatively linked with their gene expression. Several studies have highlighted that the corresponding genes of three DNA-methylation sites may play an essential role in cancer development. HSPA8, a crucial mediator in maintaining intracellular proteins and regulating cellular activity [42]. Its function include folding and transporting newly synthesized polypeptides, activating proteolysis of misfolded proteins and formimg and dissociating protein complexes [43]. HSPA8 expression has been positively correlated with glioma grade, and its knockdown effectively inhibited cell proliferation and increased apoptosis [44]. Additionally, HSPA8-dependent activation of FAK-Src signaling has been hypothesized to partially induce the migratory and invasive ability of glioma cells [45]. CDKN2A frequently mutated or deleted in a variety of tumors is considered an important tumor suppressor gene. CDKN2A hypermethylation can cause gene loss, an important event in some cancers, and patients with hypermethylation have poorer prognosis in colorectal, hepatocellular and lung cancers [46, 47]. Homozygous deletion of CDKN2A has been recognized as a prognostic indicator in glioma as well [48]. MT3, a member of the metallothionein family (MTF) is very important in metal homeostasis, heavy metal toxicity prevention, DNA damage and oxidative stress. MT3 is involved in the formation, development and drug resistance of various malignancies by participating in the Metal ion SLC transporters pathway and the Cellular responses to stimuli pathway [49]. High MT3 expression has been associated with patient survival and can be used as a prognostic marker in gliomas [50]. Furthermore, MT3 has been implicated in autophagy of astrocytes and may be a therapeutic target in glioma [51]. Even though more research and supplementary experiments are needed to understand the function of these three genes in LGG, the DNA-methylation at these locations serves as fitting biomarkers for forecasting patient outcomes.
Tumor immunotherapy is a new therapeutic approach that has made substantial progress in both fundamental research and clinical practice in recent decades. Complex tumor microenvironment is known to regulate tumor development and growth in gliomas, and tumor-infiltrating immune cells are an important part of this microenvironment [52]. Here we found that ESTIMATE scores, immune scores, and stromal scores, and the expression of ICGs were significantly higher in the high-risk group. The analysis of immune cell infiltration in the TME showed that high-risk patients exhibited increased infiltration of CD8
Investigating the biological characteristics and signaling pathways associated with cuproptosis can offer valuable insights into the role of cuproptosis in tumor initiation and progression. This deeper understanding can aid in the identification of novel prognostic factors and potential therapeutic targets for LGG [10]. While certain DNA methylation prognostic biomarkers demonstrate superior prognostic performance [56, 57], CRG-located DNA methylation markers are particularly associated with cuproptosis. Integrating these markers with other cuproptosis-related prognostic biomarkers, such as RNA expression, protein expression, and histone modifications, may enhance patient risk stratification and inform individualized treatment strategies [58]. The specific biological context of cuproptosis-related markers facilitates the discovery of direct connections between them, providing a more comprehensive understanding of the relationship between cuproptosis processes and LGG tumor biology. This approach enables the assessment of patients’ prognostic risk from multiple omics perspectives, elucidating cuproptosis mechanisms and delivering more accurate and comprehensive information for LGG clinical treatment decision-making [59]. Moreover, it may help identify biologically relevant therapeutic targets within cuproptosis-related biological processes or signaling pathways, ultimately improving treatment outcomes for LGG patients [60]. Furthermore, analyzing the associations between different biomarkers can enhance our understanding of cuproptosis mechanisms in tumor initiation, progression, and prognosis, offering theoretical support for the development of innovative treatment methods [61].
Looking ahead, further improvement is still needed in the CRG-located DNA-methylation signature constructed in this study. The combination of DNA-methylation sites was based on the information of LGG patients in the TCGA dataset, and it was verified only in the GEO datasets. To further confirm the applicability of the signature, more experimental validation were needed to be accumulated in the future. At the same time, the effect of signature in improving the quality of life in patients was still needed to be verified in clinical practice. Furthermore, we anticipate further advances in the glioma field using CRG-located DNA methylation prognostic markers. Given the complex etiology and development mechanisms of LGG [62], abnormalities in the cuproptosis pathway may directly impact disease progression in only a subset of patients. Future research should explore strategies to identify this patient subgroup through stratification studies, as CRG-located DNA methylation markers may exhibit greater specificity and sensitivity within this population. Simultaneously, with advancements in DNA methylation-based liquid biopsy techniques [63], we aim to integrate CRG-located DNA methylation prognostic markers with these methodologies. By quantifying tumor-derived DNA fragments in blood or cerebrospinal fluid using DNA methylation-based liquid biopsy techniques, abnormal methylation patterns can be detected, facilitating disease monitoring and therapeutic efficacy assessment for glioma patients [64].
5.Conclusions
We developed and validated a CRG-located DNA-methylation signature by analyzing TCGA and GEO DNA-methylation data, which can function as a dependable and effective independent prognostic biomarker for LGG patients. This signature was associated with tumor immune infiltration and the expression of ICGs, potentially influencing LGG patients outcomes by altering the tumor microenvironment (TME). Although further investigation is needed to fully understand the specific function this present research offers theoretical basis for enhancing clinical management and fostering the advancement of personalized therapy for LGG patients.
Funding
This research was funded by The National Natural Science Foundation of China (No. 32070395, No. 82302642 and No. 82103055).
Author contributions
Conception: Wenna Guo and Rui Zhu.
Interpretation or analysis of data: Liucun Zhu and Fa Yuan.
Preparation of the manuscript: Fa Yuan and Xue Wang.
Revision for important intellectual content: Liucun Zhu, Rui Zhu and Wenna Guo.
Supervision: Wenna Guo.
Institutional review board statement
Not applicable.
Informed consent statement
Not applicable.
Ethics statement
Approval of the research protocol by an Institutional Reviewer Board: N/A.
Informed Consent: N/A.
Registry and the Registration No. of the study/trial: N/A.
Animal Studies: N/A.
Data availability statement
Data presented in this study are contained within this article and in the supplementary materials, or are available upon request to the corresponding author.
Supplementary data
The supplementary files are available to download from http://dx.doi.org/10.3233/CBM-230341.
Acknowledgments
We would like to thank the TCGA, GEO databases for the availability of the data.
Conflict of interest
The authors declare no conflict of interest.
References
[1] | D. Schiff et al., Recent developments and future directions in adult lower-grade gliomas: Society for Neuro-Oncology (SNO) and European Association of Neuro-Oncology (EANO) consensus, Neuro Oncol 21: (7) ((2019) ), 837–853. |
[2] | N. Cancer Genome Atlas Research et al., Comprehensive, integrative genomic analysis of diffuse lower-grade gliomas, N Engl J Med 372: (26) ((2015) ), 2481–2498. |
[3] | J.L. Ater et al., Randomized study of two chemotherapy regimens for treatment of low-grade glioma in young children: A report from the Children’s Oncology Group, J Clin Oncol 30: (21) ((2012) ), 2641–2647. |
[4] | L.R. Song et al., Identification and validation of a 21-mRNA prognostic signature in diffuse lower-grade gliomas, J Neurooncol 146: (1) ((2020) ), 207–217. |
[5] | M. Zhang, X. Wang, X. Chen, Q. Zhang and J. Hong, Novel immune-related gene signature for risk stratification and prognosis of survival in lower-grade glioma, Front Genet 11: ((2020) ), 363. |
[6] | Y. Guo, Y. Li, J. Li, W. Tao and W. Dong, DNA methylation-driven genes for developing survival nomogram for low-grade glioma, Front Oncol 11: ((2021) ), 629521. |
[7] | M.C. Linder and M. Hazegh-Azam, Copper biochemistry and molecular biology, Am J Clin Nutr 63: (5) ((1996) ), 797S–811S. |
[8] | J.Y. Uriu-Adams and C.L. Keen, Copper, oxidative stress, and human health, Mol Aspects Med 26: (4–5) ((2005) ), 268–298. |
[9] | J. Camakaris, I. Voskoboinik and J.F. Mercer, Molecular mechanisms of copper homeostasis, Biochem Biophys Res Commun 261: (2) ((1999) ), 225–232. |
[10] | P. Tsvetkov et al., Copper induces cell death by targeting lipoylated TCA cycle proteins, Science 375: (6586) ((2022) ), 1254–1261. |
[11] | Y. Jiang, Z. Huo, X. Qi, T. Zuo and Z. Wu, Copper-induced tumor cell death mechanisms and antitumor theragnostic applications of copper complexes, Nanomedicine (Lond) 17: (5) ((2022) ), 303–324. |
[12] | H. Lv et al., Comprehensive analysis of cuproptosis-related genes in immune infiltration and prognosis in melanoma, Front Pharmacol 13: ((2022) ), 930041. |
[13] | S. Sha et al., Prognostic analysis of cuproptosis-related gene in triple-negative breast cancer, Front Immunol 13: ((2022) ), 922780. |
[14] | Y. Yun, Y. Wang, E. Yang and X. Jing, Cuproptosis-Related Gene – SLC31A1, FDX1 and ATP7B – polymorphisms are associated with risk of lung cancer, Pharmgenomics Pers Med 15: ((2022) ), 733–742. |
[15] | Z. Zhang et al., FDX1 can impact the prognosis and mediate the metabolism of lung adenocarcinoma, Front Pharmacol 12: ((2021) ), 749134. |
[16] | Z. Bian, R. Fan and L. Xie, A novel cuproptosis-related prognostic gene signature and validation of differential expression in clear cell renal cell carcinoma, Genes (Basel) 13: (5) ((2022) ). |
[17] | A. Paziewska et al., DNA methylation status is more reliable than gene expression at detecting cancer in prostate biopsy, Br J Cancer 111: (4) ((2014) ), 781–789. |
[18] | X. Hao et al., DNA methylation markers for diagnosis and prognosis of common cancers, Proc Natl Acad Sci U S A 114: (28) ((2017) ), 7414–7419. |
[19] | A.A. Emran et al., Targeting DNA methylation and EZH2 activity to overcome melanoma resistance to immunotherapy, Trends Immunol 40: (4) ((2019) ), 328–344. |
[20] | A. Wenger and H. Caren, Methylation profiling in diffuse gliomas: Diagnostic value and considerations, Cancers (Basel) 14: (22) ((2022) ). |
[21] | A. Ozair et al., DNA methylation and histone modification in low-grade gliomas: Current understanding and potential clinical targets, Cancers (Basel) 15: (4) ((2023) ). |
[22] | W. Guo et al., A five-DNA methylation signature act as a novel prognostic biomarker in patients with ovarian serous cystadenocarcinoma, Clin Epigenetics 10: (1) ((2018) ), 142. |
[23] | V. Aleotti et al., Methylation markers in cutaneous melanoma: Unravelling the potential utility of their tracking by liquid biopsy, Cancers (Basel) 13: (24) ((2021) ). |
[24] | J.C. Rotondo et al., Hypermethylation-induced inactivation of the IRF6 gene as a possible early event in progression of vulvar squamous cell carcinoma associated with lichen sclerosus, JAMA Dermatol 152: (8) ((2016) ), 928–933. |
[25] | S. Ferreyra Vega et al., DNA methylation profiling for molecular classification of adult diffuse lower-grade gliomas, Clin Epigenetics 13: (1) ((2021) ), 102. |
[26] | J.H. Bao et al., Identification of a novel cuproptosis-related gene signature and integrative analyses in patients with lower-grade gliomas, Front Immunol 13: ((2022) ), 933973. |
[27] | P. Chen, H. Han, X. Wang, B. Wang and Z. Wang, Novel cuproptosis-related gene signature for precise identification of high-risk populations in low-grade gliomas, Mediators Inflamm 2023: ((2023) ), 6232620. |
[28] | P. Bady et al., The DNA methylome of DDR genes and benefit from RT or TMZ in IDH mutant low-grade glioma treated in EORTC 22033, Acta Neuropathol 135: (4) ((2018) ), 601–615. |
[29] | A.J. Gentles et al., The prognostic landscape of genes and infiltrating immune cells across human cancers, Nat Med 21: (8) ((2015) ), 938–945. |
[30] | X. Zhao et al., The expression of cuproptosis-related genes in hepatocellular carcinoma and their relationships with prognosis, Front Oncol 12: ((2022) ), 992468. |
[31] | P.C. van Dijk, K.J. Jager, A.H. Zwinderman, C. Zoccali and F.W. Dekker, The analysis of survival data in nephrology: basic concepts and methods of Cox regression, Kidney Int 74: (6) ((2008) ), 705–709. |
[32] | W. Guo et al., Identification and validation of ferroptosis-related DNA methylation signature for predicting the prognosis and guiding the treatment in cutaneous melanoma, Int J Mol Sci 23: (24) ((2022) ). |
[33] | K. Yoshihara et al., Inferring tumour purity and stromal and immune cell admixture from expression data, Nat Commun 4: ((2013) ), 2612. |
[34] | A.M. Newman et al., Robust enumeration of cell subsets from tissue expression profiles, Nat Methods 12: (5) ((2015) ), 453–457. |
[35] | F.F. Hu, C.J. Liu, L.L. Liu, Q. Zhang and A.Y. Guo, Expression profile of immune checkpoint genes and their roles in predicting immunotherapy response, Brief Bioinform 22: (3) ((2021) ). |
[36] | S. Bagchi, R. Yuan and E.G. Engleman, Immune checkpoint inhibitors for the treatment of cancer: Clinical impact and mechanisms of response and resistance, Annu Rev Pathol 16: ((2021) ), 223–249. |
[37] | R. Dubrow and A.S. Darefsky, Demographic variation in incidence of adult glioma by subtype, United States, 1992–2007, BMC Cancer 11: ((2011) ), 325. |
[38] | K. Michalczyk and A. Cymbaluk-Ploska, The role of zinc and copper in gynecological malignancies, Nutrients 12: (12) ((2020) ). |
[39] | V. Koroknai et al., DNA hypermethylation is associated with invasive phenotype of malignant melanoma, Exp Dermatol 29: (1) ((2020) ), 39–50. |
[40] | G. Micevic, N. Theodosakis and M. Bosenberg, Aberrant DNA methylation in melanoma: biomarker and therapeutic opportunities, Clin Epigenetics 9: ((2017) ), 34. |
[41] | F. Kottakis et al., LKB1 loss links serine metabolism to DNA methylation and tumorigenesis, Nature 539: (7629) ((2016) ), 390–395. |
[42] | F. Stricher, C. Macri, M. Ruff and S. Muller, HSPA8/HSC70 chaperone protein: Structure, function, and chemical targeting, Autophagy 9: (12) ((2013) ), 1937–1954. |
[43] | Y.H. Yamamoto et al., A novel ER J-protein DNAJB12 accelerates ER-associated degradation of membrane proteins including CFTR, Cell Struct Funct 35: (2) ((2010) ), 107–116. |
[44] | G. Sun, Y. Cao, X. Dai, M. Li and J. Guo, Hsc70 interacts with beta4GalT5 to regulate the growth of gliomas, Neuromolecular Med 21: (1) ((2019) ), 33–41. |
[45] | G. Sun et al., Overexpression of Hsc70 promotes proliferation, migration, and invasion of human glioma cells, J Cell Biochem 120: (6) ((2019) ), 10707–10714. |
[46] | K. Shima et al., Prognostic significance of CDKN2A (p16) promoter methylation and loss of expression in 902 colorectal cancers: Cohort study and literature review, Int J Cancer 128: (5) ((2011) ), 1080–1094. |
[47] | D.P. Bradly et al., CDKN2A (p16) promoter hypermethylation influences the outcome in young lung cancer patients, Diagn Mol Pathol 21: (4) ((2012) ), 207–213. |
[48] | R. Appay et al., CDKN2A homozygous deletion is a strong adverse prognosis factor in diffuse malignant IDH-mutant gliomas, Neuro Oncol 21: (12) ((2019) ), 1519–1528. |
[49] | M. Si and J. Lang, The roles of metallothioneins in carcinogenesis, J Hematol Oncol 11: (1) ((2018) ), 107. |
[50] | B. Masiulionyte, I. Valiulyte, A. Tamasauskas and D. Skiriute, Metallothionein genes are highly expressed in malignant astrocytomas and associated with patient survival, Sci Rep 9: (1) ((2019) ), 5406. |
[51] | Y.H. Cho, S.H. Lee, S.J. Lee, H.N. Kim and J.Y. Koh, A role of metallothionein-3 in radiation-induced autophagy in glioma cells, Sci Rep 10: (1) ((2020) ), 2015. |
[52] | P. Domingues et al., Tumor infiltrating immune cells in gliomas and meningiomas, Brain Behav Immun 53: ((2016) ), 1–15. |
[53] | X. Chen et al., Comprehensive characterization of extracellular matrix-related genes in PAAD identified a novel prognostic panel related to clinical outcomes and immune microenvironment: A silico analysis with in vivo and vitro validation, Front Immunol 13: ((2022) ), 985911. |
[54] | E.C. Cheung and K.H. Vousden, The role of ROS in tumour development and progression, Nat Rev Cancer 22: (5) ((2022) ), 280–297. |
[55] | Y. Zhang et al., The role of histone methylase and demethylase in antitumor immunity: A new direction for immunotherapy, Front Immunol 13: ((2022) ), 1099892. |
[56] | F. Ohka et al., The global DNA methylation surrogate LINE-1 methylation is correlated with MGMT promoter methylation and is a better prognostic factor for glioma, PLoS One 6: (8) ((2011) ), e23332. |
[57] | X. Wu et al., Epigenetic Activation of lncRNA MIR155HG Mediated by Promoter Hypomethylation and SP1 is Correlated with Immune Infiltration in Glioma, Onco Targets Ther 15: ((2022) ), 219–235. |
[58] | I. Magen et al., Circulating miR-181 is a prognostic biomarker for amyotrophic lateral sclerosis, Nat Neurosci 24: (11) ((2021) ), 1534–1541. |
[59] | S. Shen et al., A multi-omics study links TNS3 and SEPT7 to long-term former smoking NSCLC survival, NPJ Precis Oncol 5: (1) ((2021) ), 39. |
[60] | X. Jiang, B.R. Stockwell and M. Conrad, Ferroptosis: Mechanisms, biology and role in disease, Nat Rev Mol Cell Biol 22: (4) ((2021) ), 266–282. |
[61] | H. Zhao et al., Inflammation and tumor progression: Signaling pathways and targeted intervention, Signal Transduct Target Ther 6: (1) ((2021) ), 263. |
[62] | D.N. Louis et al., The 2021 WHO classification of tumors of the central nervous system: A summary, Neuro Oncol 23: (8) ((2021) ), 1231–1251. |
[63] | E. Heitzer, I.S. Haque, C.E.S. Roberts and M.R. Speicher, Current and future perspectives of liquid biopsies in genomics-driven oncology, Nat Rev Genet 20: (2) ((2019) ), 71–88. |
[64] | Y. Li, Z. Fan, Y. Meng, S. Liu and H. Zhan, Blood-based DNA methylation signatures in cancer: A systematic review, Biochim Biophys Acta Mol Basis Dis 1869: (1) ((2023) ), 166583. |