Potential association of HSPD1 with dysregulations in ribosome biogenesis and immune cell infiltration in lung adenocarcinoma: An integrated bioinformatic approach
Abstract
BACKGROUND:
Lung adenocarcinoma (LUAD) is a major histological subtype of lung cancer with a high mortality rate worldwide. Heat shock protein family D member 1 (HSPD1, also known as HSP60) is reported to be increased in tumor tissues of lung cancer patients compared with healthy control tissues.
OBJECTIVE:
We aimed to investigate the roles of HSPD1 in prognosis, carcinogenesis, and immune infiltration in LUAD using an integrative bioinformatic analysis.
METHODS:
HSPD1 expression in LUAD was investigated in several transcriptome-based and protein databases. Survival analysis was performed using the KM plotter and OSluca databases, while prognostic significance was independently confirmed through univariate and multivariate analyses. Integrative gene interaction network and enrichment analyses of HSPD1-correlated genes were performed to investigate the roles of HSPD1 in LUAD carcinogenesis. TIMER and TISIDB were used to analyze correlation between HSPD1 expression and immune cell infiltration.
RESULTS:
The mRNA and protein expressions of HSPD1 were higher in LUAD compared with normal tissues. High HSPD1 expression was associated with male gender and LUAD with advanced stages. High HSPD1 expression was an independent prognostic factor associated with poor survival in LUAD patients. HSPD1-correlated genes with prognostic impact were mainly involved in aberrant ribosome biogenesis, while LUAD patients with high HSPD1 expression had low tumor infiltrations of activated and immature B cells and CD4+ T cells.
CONCLUSIONS:
HSPD1 may play a role in the regulation of ribosome biogenesis and B cell-mediated immunity in LUAD. It could serve as a predictive biomarker for prognosis and immunotherapy response in LUAD.
1.Introduction
Lung cancer is one of the most common cancers with a high mortality rate worldwide [1]. The majority of lung cancer is non-small cell lung cancer (NSCLC), in which lung adenocarcinoma (LUAD) is the most common subtype [2]. Although treatment options are currently available, LUAD patients still have a poor prognosis and low survival rate [2, 3]. Moreover, the effectiveness of chemotherapeutic and targeted drugs is often limited by the drug resistance [3, 4]. Therefore, identifying new prognostic markers and therapeutic targets for LUAD is necessary.
Tumor-infiltrating immune cells (TIICs) are one of the key components in the tumor microenvironment (TME) that influence tumor progression and aggressiveness [5]. Previous studies have shown that TIICs are related to clinical outcomes and prognosis of LUAD patients [6, 7, 8]. The composition of TIICs also affects patients’ response to immunotherapy [9, 10, 11]. Hence, TIICs are promising targets for predicting the prognosis and immune response in LUAD patients.
Heat shock protein family D member 1 (HSPD1), also known as HSP60, is a molecular chaperone that participates in various cellular functions. It is present in different cellular compartments and implicated in both intracellular and extracellular processes [12, 13, 14, 15]. Accumulating evidence has highlighted the critical role of HSPD1 in cancer development, progression, and chemoresistance across various types of cancer [16, 17]. HSPD1 has been shown to exert its oncogenic functions through several mechanisms, such as p53, Erk1/2,
Previous studies have reported a correlation between HSPD1 overexpression and poor prognosis in NSCLC patients [25, 26]. HSPD1 has been shown to promote NSCLC development through modulation of mitochondrial metabolisms [27]. Additionally, extracellular HSPD1 can induce an inflammatory response in bronchial epithelial cells [28] and modulate the effector functions of immune cells, such as macrophages and neutrophils [23, 24]. The involvement of HSPD1 in regulating these critical aspects of tumor biology suggests its potential as a prognostic marker in LUAD. A deeper understanding of the precise mechanisms by which HSPD1 modulates these processes could provide valuable insights into LUAD pathogenesis and potentially reveal therapeutic targets.
In this study, we analyzed HSPD1 expression and its correlation with clinicopathologic features in LUAD patients using various databases, including TIMER, GEPIA2, HPA, and UALCAN. Prognostic value of HSPD1 expression in LUAD was investigated by KM plotter and OSluca. Survival prognosis, interaction network, and enrichment analyses of HSPD1-correlated genes in LUAD were performed using GEPIA2, GeneMANIA, and DAVID, respectively. In addition, association between HSPD1 expression and immune cell infiltration was also explored with TIMER and TISIDB. Our findings provide new insights into the role of HSPD1 and its relationship to tumor immunity in LUAD.
2.Materials and methods
2.1Differential expression analysis of HSPD1 in LUAD and normal tissues – mRNA level
We investigated the mRNA expression of HSPD1 in tumor and normal samples across various human cancers using Tumor Immune Estimation Resource (TIMER) database (https://cistrome.shinyapps.io/timer/) [29, 30]. The Gene Expression Profiling Interactive Analysis 2 (GEPIA2) database (http://gepia2.cancer-pku.cn/) [31] was used to analyze the mRNA expression of HSPD1 in normal (
2.2Differential expression analysis of HSPD1 in LUAD and normal tissues – protein level
Differential protein expression of HSPD1 was analyzed from normal (
2.3Association analysis of HSPD1 expression and clinicopathologic features of LUAD patients
Relationships between HSPD1 mRNA expression and clinicopathologic features, including age, race, gender, and stages were analyzed in normal (
2.4Survival analysis
To evaluate the prognostic value of HSPD1 in LUAD, we used two different tools: Kaplan-Meier (KM) plotter database (www.kmplot.com/lung) [35] and Online consensus Survival for Lung Cancer (OSluca) database (http://bioinfo.henu.edu.cn/LUCA/LUCAList.jsp) [36] In KM plotter, the Affymetrix probe ID: 200806_s_at was used to analyze the overall survival (
2.5Integrative gene interaction network and enrichment analyses of HSPD1-correlated genes
To comprehensively analyze the potential role of HSPD1 in LUAD, we retrieved the top 25 genes that are positively and negatively correlated with HSPD1 in the LUAD-TCGA dataset based on the Pearson correlation coefficient value from the UALCAN database. Survival impact of HSPD1-correlated genes on overall survival in LUAD patients was explored using GEPIA2. The GeneMANIA (https://genemania.org/) [37] was used to construct an interaction network of HSPD1-correlated genes. Gene Ontology (GO) and Kyoto Encyclopedia of Genes and Genomes (KEGG) pathway enrichment analyses were performed using the Database for Annotation, Visualization and Integrated Discovery (DAVID) (https://david.ncifcrf.gov/summary.jsp) [38, 39]. The dot plots of enriched pathways were depicted by using GraphBio online tool (http://www.graphbio1.com/en/) [40].
2.6Analysis of correlation between HSPD1 and ribosome biogenesis marker gene expression
Correlation between HSPD1 expression and ribosome biogenesis marker genes (i.e. NHP2, H/ACA ribonucleoprotein complex subunit 2; NMD3, 60S ribosomal export protein NMD3; NOP56, Nucleolar protein 56; RCL1, RNA 3’-terminal phosphate cyclase-like protein; and TCOF1, Treacher Collins syndrome protein) expression was analyzed in LUAD-TCGA dataset using GEPIA2 with Pearson’s correlation analysis.
2.7Analysis of correlation between HSPD1 expression and immune cell infiltration in LUAD
TIMER was used to analyze the correlation between HSPD1 expression with tumor infiltrating immune cells in LUAD, including B cells, CD8+ T cells, CD4+ T cells, neutrophils, macrophages, and dendritic cells. KM survival curves of each immune cell infiltration were plotted with the log-rank
Figure 1.
The workflow chart of this study.
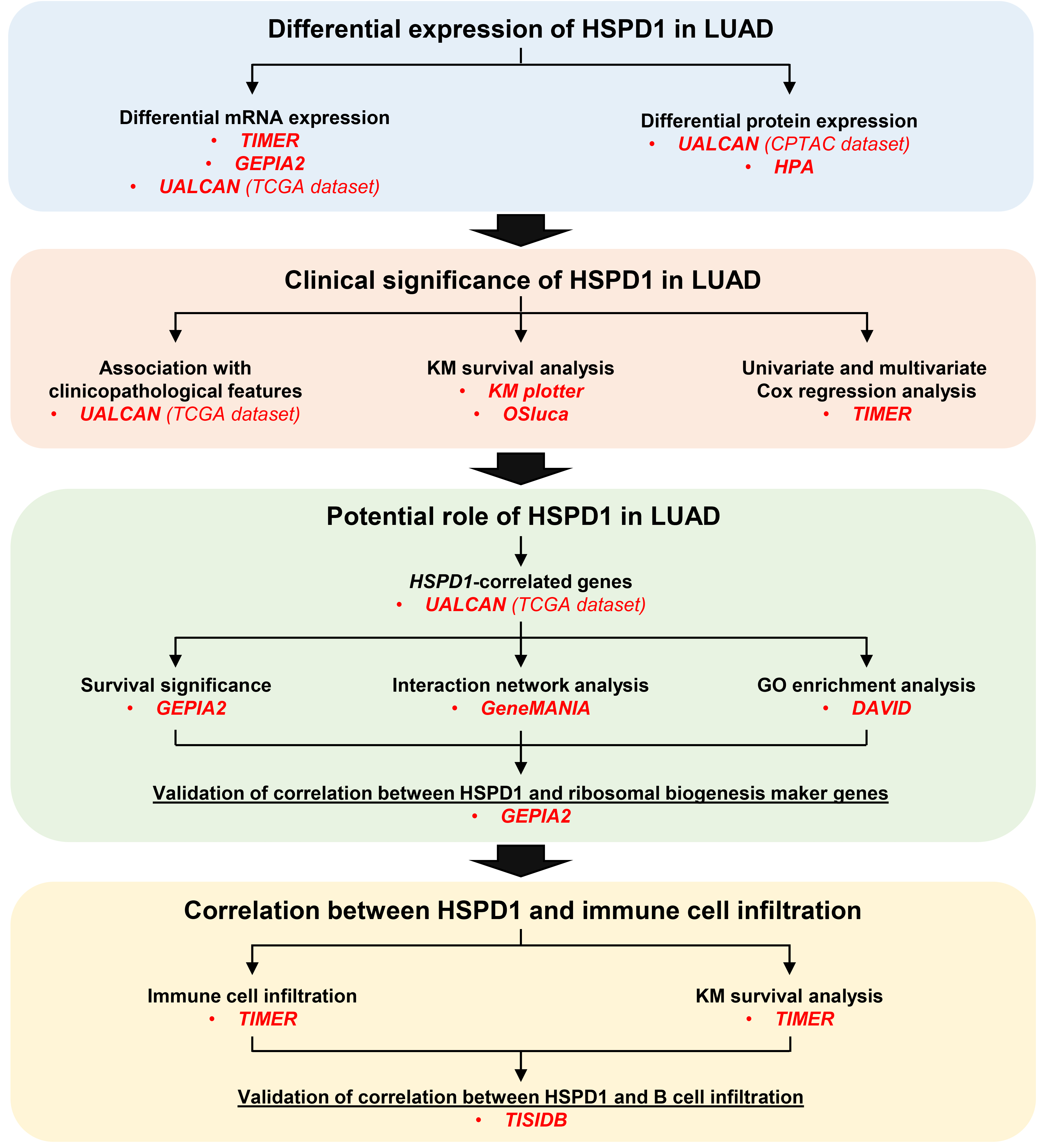
Figure 2.
Expression levels of HSPD1 in human cancers and LUAD. (A) HSPD1 mRNA expression in different TCGA tumor types in TIMER; red, tumor tissues; blue, normal adjacent tissues; purple, metastatic tissues. (B) mRNA expression of HSPD1 in normal and LUAD tissues in GEPIA2. (C) mRNA expression of HSPD1 in normal and LUAD tissues from TCGA dataset in UALCAN. (D) Protein expression of HSPD1 in normal and LUAD tissues from CPTAC dataset in UALCAN. (E) Immunohistochemistry staining images of HSPD1 protein in normal lung and LUAD tissues obtained from HPA. *p < 0.05, **p < 0.01, ***p < 0.001.
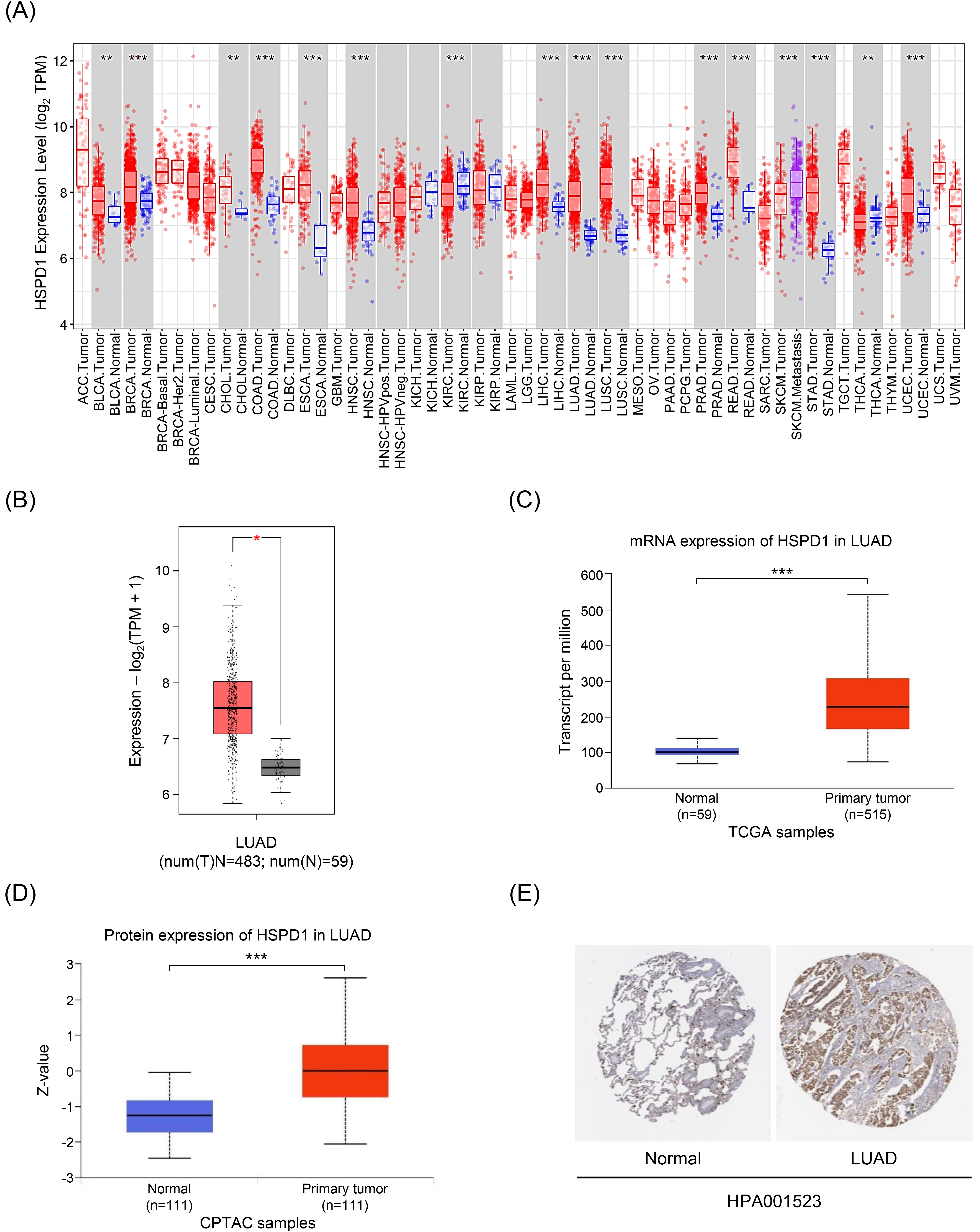
3.Results
3.1Expression of HSPD1 in human cancers and LUAD tumor tissues
The workflow chart of this study is showed in Fig. 1. The expression level of HSPD1 in human cancers was initially investigated using TIMER database. As shown in Fig. 2A, HSPD1 mRNA expression was markedly increased in LUAD and various other types of cancers. Similarly, an elevated HSPD1 mRNA expression in LUAD tissues was found in GEPIA2 database (Fig. 2B). Furthermore, UALCAN analysis demonstrated a significant increase in HSPD1 expression in LUAD at both mRNA and protein levels (Fig. 2C and 2D). Immunohistochemistry results obtained from HPA database also showed that HSPD1 protein level was higher in LUAD compared with normal lung tissues (Fig. 2E). Consistently, HSPD1 expression is upregulated in LUAD tumor tissues at both mRNA and protein levels among multiple databases, which therefore supports further investigations on its correlation with clinical characteristics of LUAD patients.
3.2Association between HSPD1 expression and clinicopathologic features of LUAD patients
Table 1
Univariate and multivariate Cox analysis of clinicopathological parameters and HSPD1 expression for survival in LUAD patients estimated by TIMER
Parameters | Univariate analysis | Multivariate analysis | ||
---|---|---|---|---|
HR (95% CI) | HR (95% CI) | |||
Age | 1.008 (0.992–1.024) | 0.33 | 1.008 (0.990–1.026) | 0.393 |
Gender (Male) | 1.065 (0.796–1.424) | 0.672 | 0.956 (0.688–1.328) | 0.786 |
Race (Black) | 2.031 (0.270–15.289) | 0.491 | 2.399 (0.313–18.364) | 0.399 |
Race (White) | 2.788 (0.390–19.939) | 0.307 | 2.938 (0.405–21.336) | 0.287 |
Stage 2 | 2.417 (1.68–3.476) |
| 2.205 (1.478–3.288) |
|
Stage 3 | 3.584 (2.450–5.244) |
| 2.715 (1.796–4.104) |
|
Stage 4 | 3.828 (2.206–6.642) |
| 2.771 (1.481–5.187) | 0.001 |
HSPD1 | 1.466 (1.189–1.808) |
| 1.382 (1.093–1.747) | 0.007 |
HR: Hazard ratio, CI: confidence interval.
Figure 3.
Relationship between HSPD1 expression and clinicopathological parameters in LUAD patients from TCGA dataset in UALCAN. HSPD1 expression in LUAD patients based on (A) age, (B) race, (C) gender, and (D) individual cancer stages. *p < 0.05, **p < 0.01, ***p < 0.001.
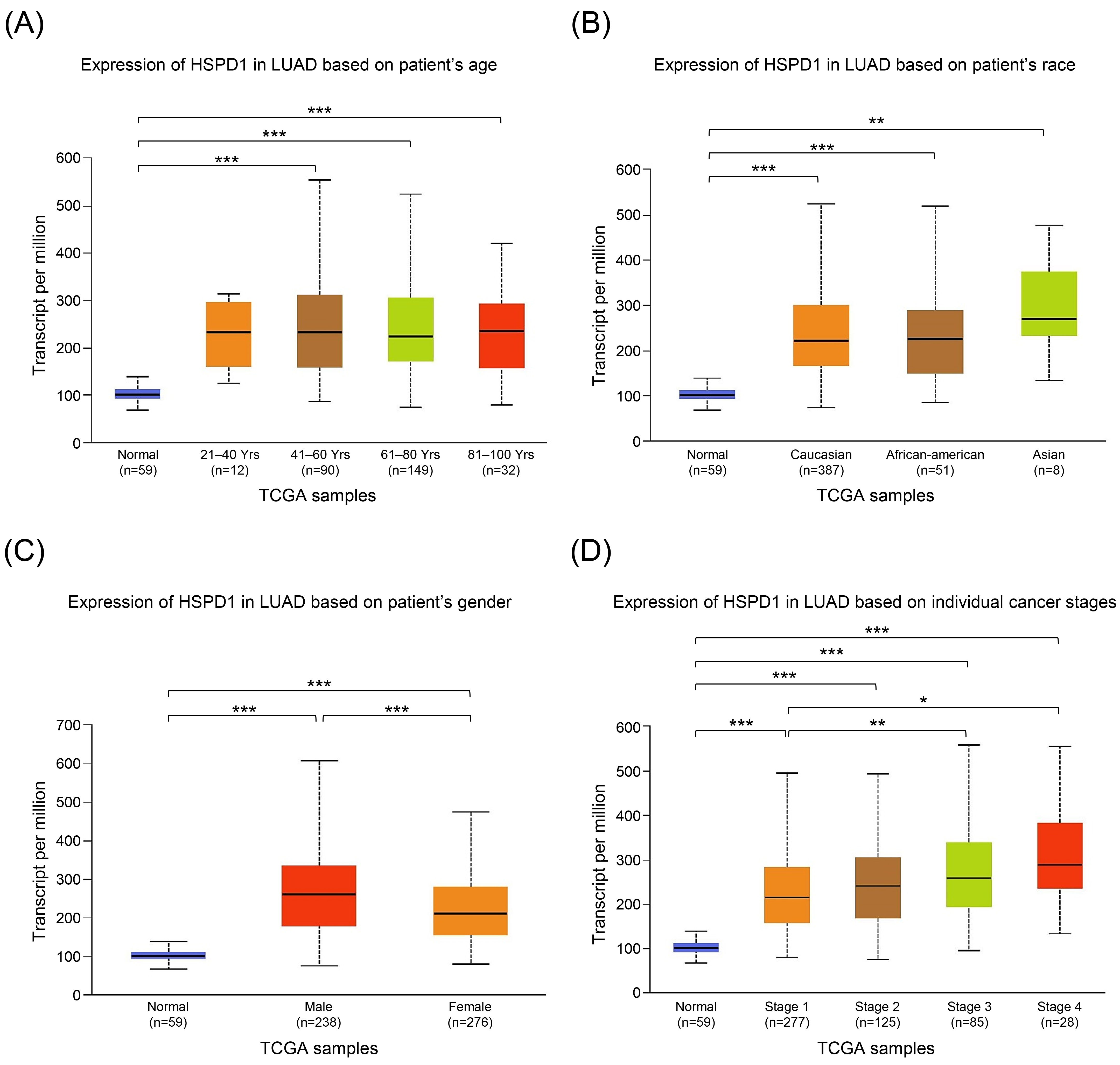
We employed UALCAN analysis to evaluate the relationship between HSPD1 mRNA expression in tumor tissues and clinicopathologic features of LUAD patients. As expected, the results showed that HSPD1 expression was significantly higher in LUAD patients compared with normal control in all subgroup analyses categorized by age, race, gender, and cancer stages (Fig. 3). While HSPD1 expression was not different among patients with various age and race groups (Fig. 3A and 3B) the significantly higher HSPD1 expression was found in male patients compared to female patients (Fig. 3C). Patients with LUAD stage 3 and stage 4 had significantly higher HSPD1 expression than those with stage 1 (Fig. 3D). This result also suggested that HSPD1 may exhibit a dose-dependent effect on the disease severity and probably determine the prognosis in LUAD patients.
Figure 4.
Relationship between HSPD1 expression and survival outcome of LUAD patients. KM survival curves for (A) overall survival and (B) first progression survival of LUAD patients in KM plotter. KM survival curves for (C) overall survival and (D) disease-specific survival of LUAD patients in OSluca.
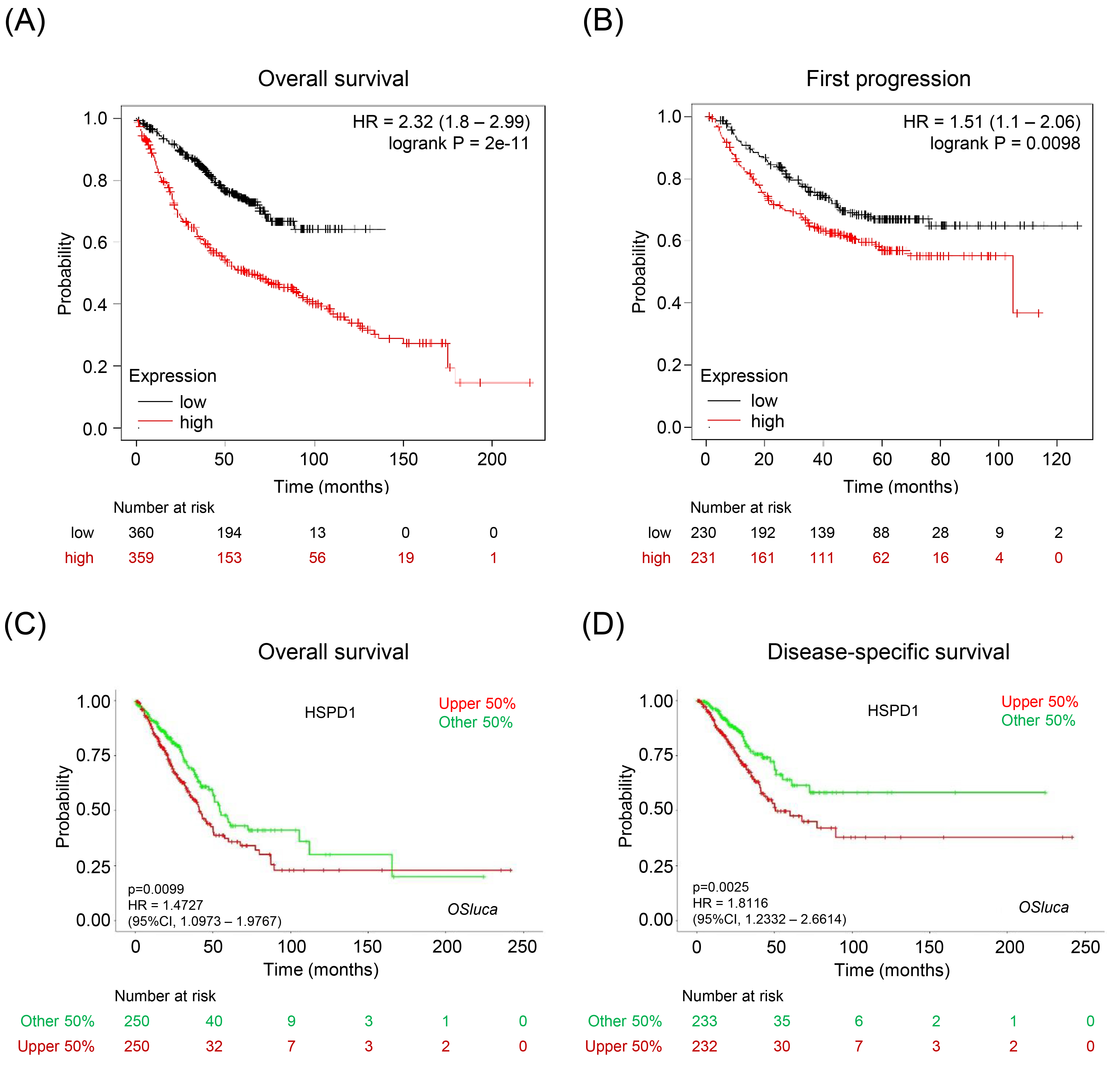
In this direction, we then performed univariate and multivariate Cox regression analyses of HSPD1 expression and clinicopathologic parameters for the overall survival of LUAD patients by using TIMER database. The univariate analysis showed that HSPD1 expression (HR
To further validate the prognostic impact of HSPD1 expression in LUAD, we performed survival analysis by using two databases. In KM plotter database, LUAD patients with high HSPD1 expression had significantly shorter overall survival (HR
Figure 5.
HSPD1-correlated genes in LUAD and their interaction network. (A) Expression heatmaps of top 25 genes that are positively and negatively correlated with HSPD1 in normal and LUAD tissues visualized by UALCAN. (B) Survival heatmaps of HSPD1-correlated genes in LUAD created by GEPIA2. Heatmaps show hazard ratio for different genes in overall survival of LUAD patients. Framed blocks represent statistical significance in prognostic analysis. (C) Interaction network of HSPD1-correlated genes constructed by GeneMANIA.
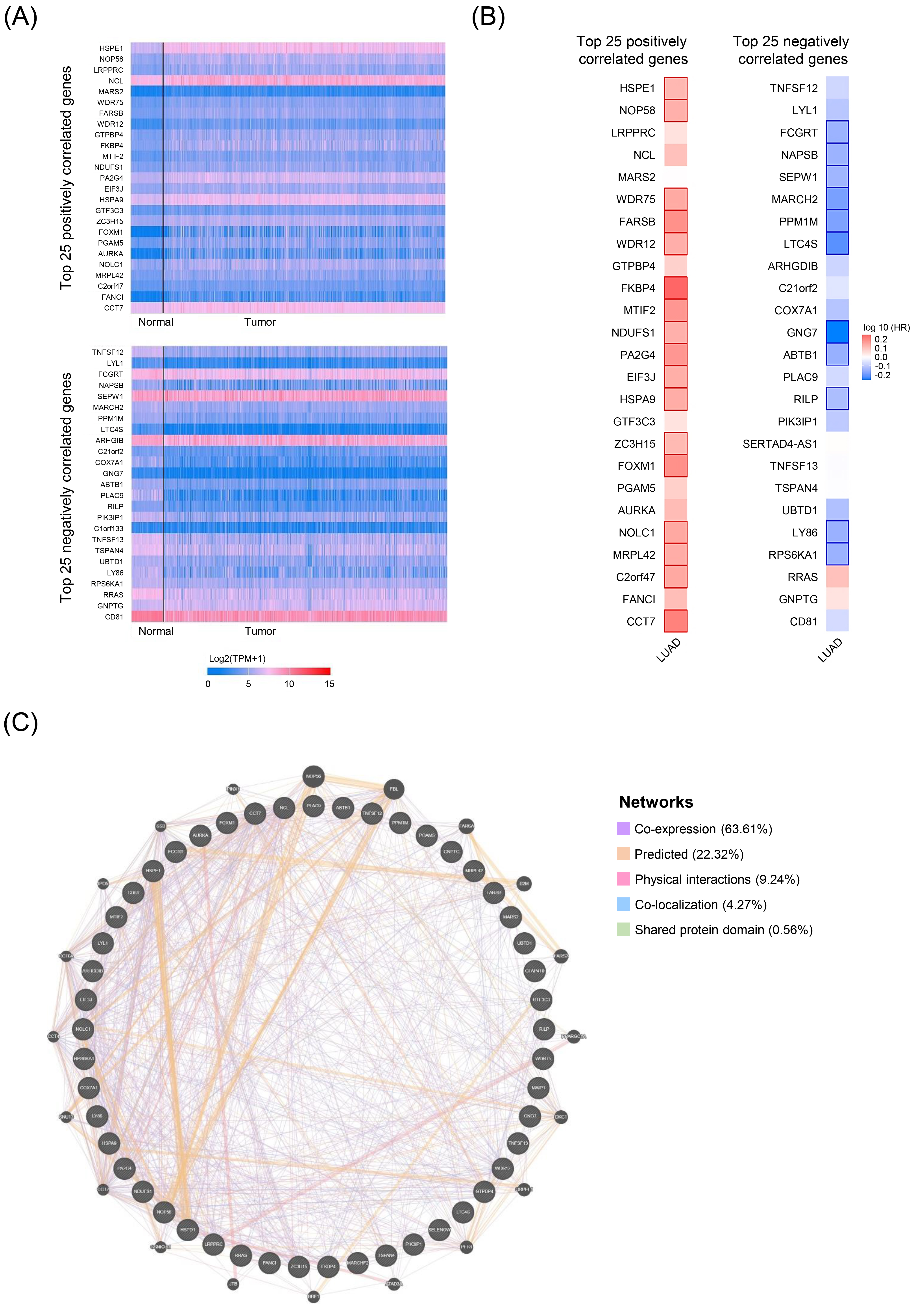
Figure 6.
GO and KEGG pathway enrichment analysis of HSPD1-correlated genes in LUAD. GO terms for biological process (A), cellular component (B), and molecular function (C), and KEGG pathway (D) assigned to HSPD1-correlated genes by using DAVID online tool.
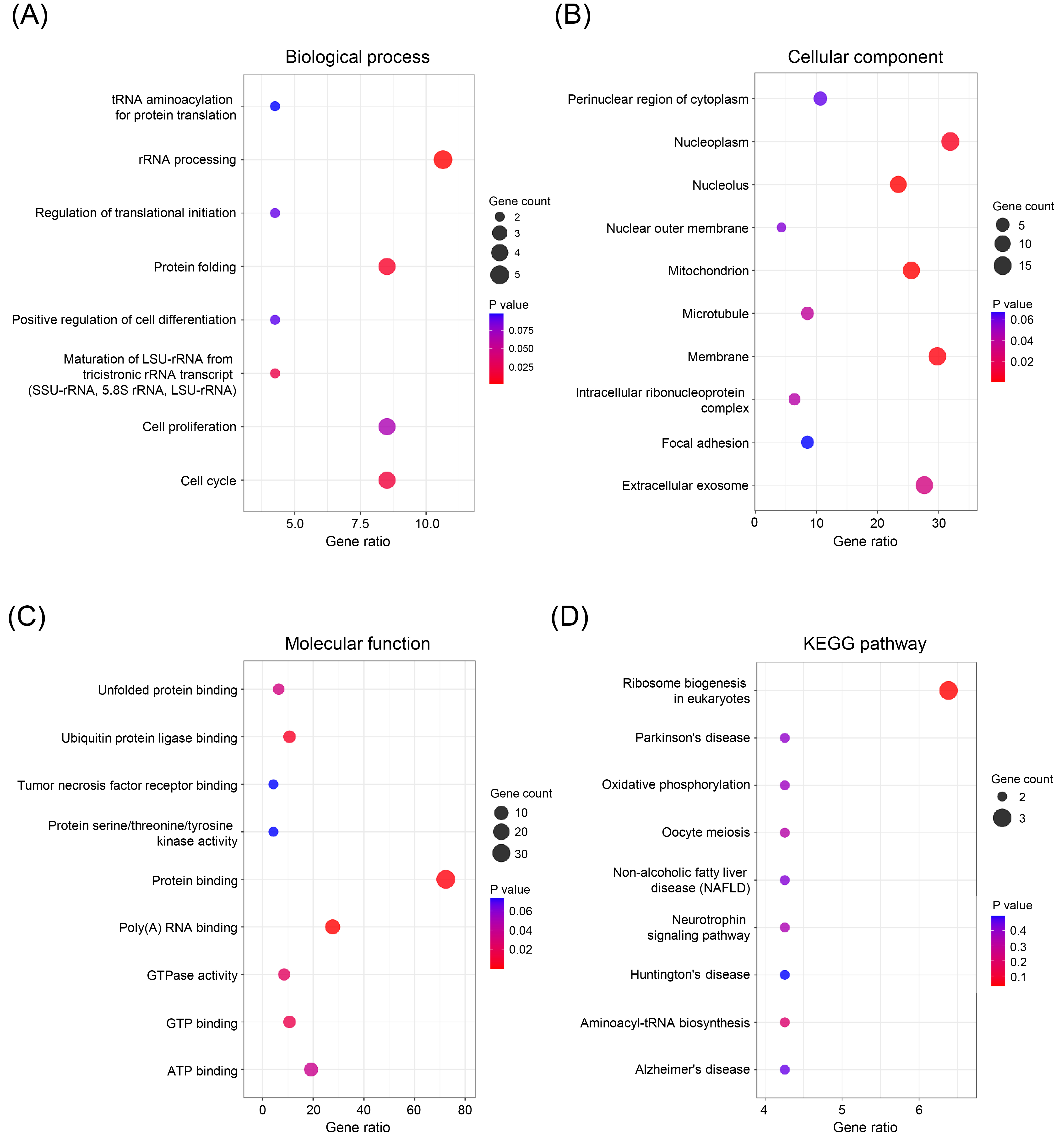
Figure 7.
Ribosome biogenesis in eukaryotes pathway and its correlation with HSPD1 in LUAD. (A) Ribosome biogenesis in eukaryotes pathway obtained from KEGG. Red stars denote components regulated by HSPD1-correlated genes. Genes that were selected for further correlation analysis are indicated by red dotted boxes. (B) Correlation of HSPD1 with TCOF1, NOP56, NHP2, RCL1, and NMD3 in LUAD analyzed by GEPIA2.
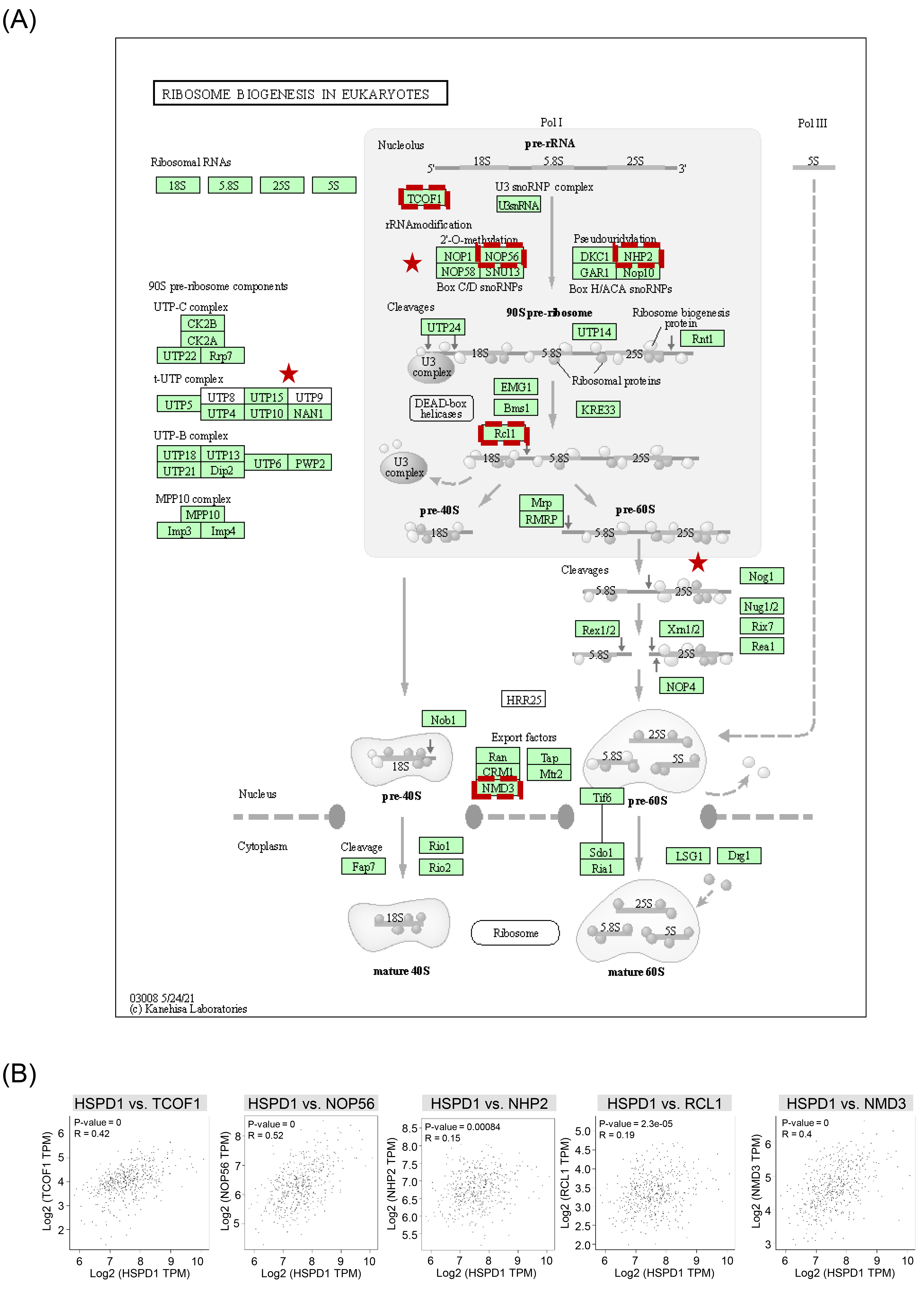
Table 2
Univariate and multivariate Cox analysis of infiltrating immune cells and HSPD1 expression for survival in LUAD patients estimated by TIMER
Variables | Univariate analysis | Multivariate analysis | ||
---|---|---|---|---|
HR (95% CI) | HR (95% CI) | |||
B cell | 0.024 (0.004–0.142) |
| 0.006 (0.000–0.076) |
|
CD8+ T cell | 0.299 (0.083–1.074) | 0.064 | 1.195 (0.190–7.526) | 0.850 |
CD4+ T cell | 0.346 (0.078–1.541) | 0.164 | 32.942 (2.091–518.996) | 0.013 |
Macrophage | 0.577 (0.094–3.539) | 0.552 | 1.524 (0.114–20.382) | 0.750 |
Neutrophil | 0.366 (0.055–2.452) | 0.300 | 0.415 (0.009–18.781) | 0.651 |
Dendritic cell | 0.553 (0.308–0.993) | 0.047 | 0.879 (0.233–3.310) | 0.849 |
HSPD1 | 1.466 (1.189–1.808) |
| 1.415 (1.124–1.781) | 0.003 |
HR: Hazard ratio, CI: confidence interval.
3.3Survival prognosis, interaction network, and enrichment analysis of HSPD1-correlated genes related to LUAD
In order to investigate the potential roles of HSPD1 in LUAD carcinogenesis, top 25 genes that are positively and negatively correlated with HSPD1 expression were retrieved from UALCAN database. Expression heatmaps of HSPD1-correlated genes are represented in Fig. 5A and details of these genes are summarized in Supplementary Table S1. Then, the prognosis values of HSPD1-correlated genes in LUAD patients was explored by the survival heatmap using GEPIA2. As shown in Fig. 5B, 17 genes of the top 25 positively correlated genes had high HR (
3.4Correlation between HSPD1 and ribosome biogenesis marker genes expression in LUAD
We further assessed whether HSPD1 expression was related to ribosome biogenesis in LUAD. Details of ribosome biogenesis in eukaryotes pathway and components regulated by HSPD1-correlated genes are represented in Fig. 7A. Correlation between HSPD1 and ribosome biogenesis marker gene expression, including TCOF1, NOP56, NHP2, RCL1, and NMD3 was analyzed in LUAD dataset in GEPIA2. As shown in Fig. 7B, HSPD1 expression was significantly positive correlated with all of these marker genes, indicating the potential involvement of HSPD1 in ribosome biogenesis pathway in LUAD.
Figure 8.
Relationship between HSPD1 expression and immune cell infiltration in LUAD. (A) Correlation of HSPD1 expression with immune cell infiltration in LUAD patients evaluated by TIMER. (B) KM survival curves of each type of immune cells in LUAD patients obtained from TIMER. (C) Correlation of HSPD1 expression with activated B (Act B) cells, immature B (Imm B) cells, and memory B (Mem B) cells in LUAD from TISIDB.
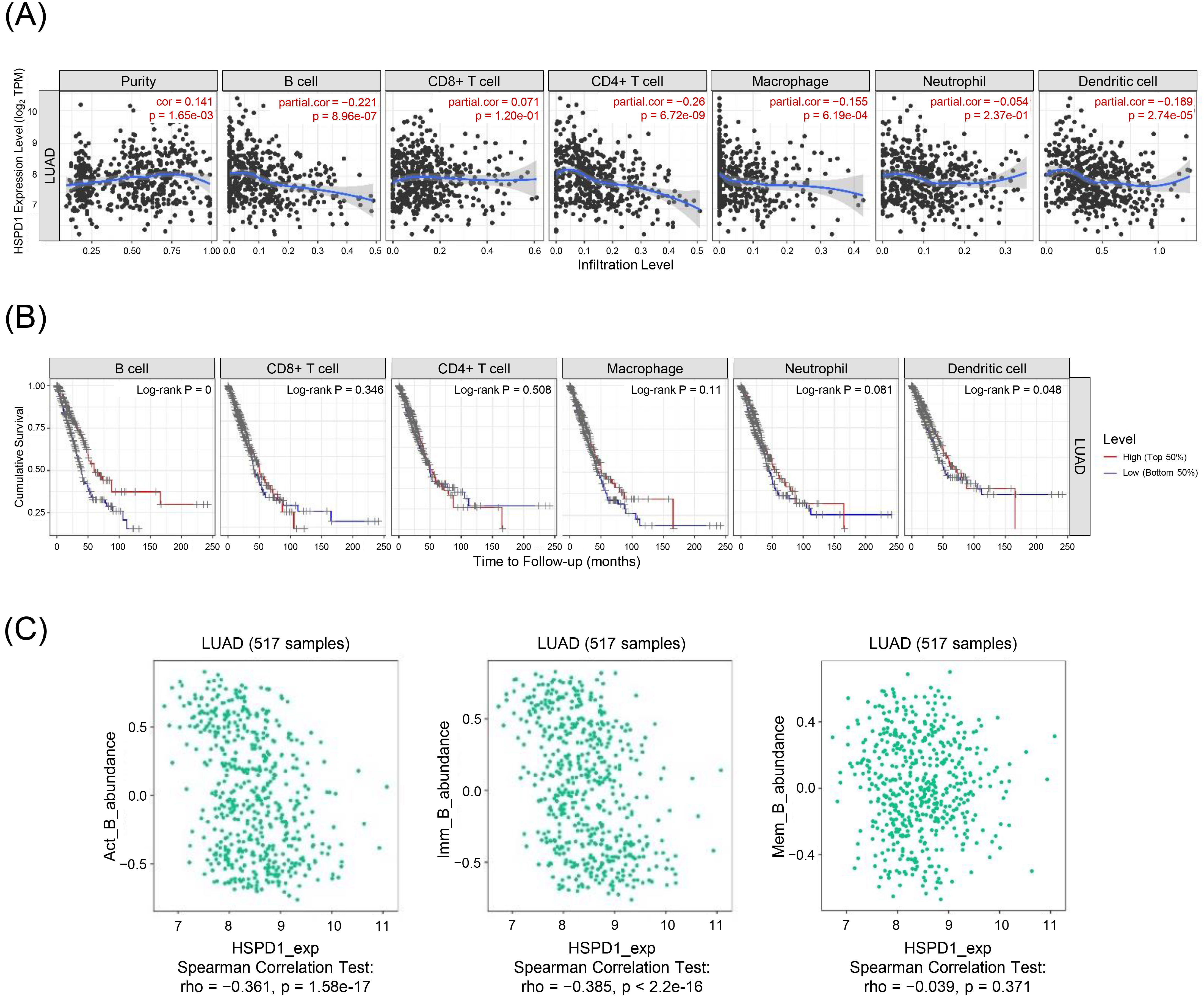
3.5Relationship between HSPD1 expression and immune cell infiltration in LUAD
Aberrant ribosome biogenesis is not only involved with tumorigenesis, e.g., MDM2/p53-mediated ribosome biogenesis checkpoint [42, 43, 44], but also regulates immune responses via multiple mechanisms, such as interferon-
4.Discussion
HSPD1 has been reported to play a role in the development and progression of many cancers, including LUAD [16, 27]. Its overexpression is associated with poor prognosis in LUAD patients [25, 26] and chemoresistance in NSCLC cells [27]. Additionally, HSPD1 is widely recognized for its interaction with the immune system. Extracellular or secreted HSPD1 can modulate the activation and function of various kinds of immune cells [15]. TIICs serve as key modulators in TME, and their composition is related to the prognosis and therapeutic response of LUAD patients [6, 7, 8, 9, 10, 11]. Therefore, HSPD1 is an interesting molecule for further investigations of its potential as a biomarker for predicting prognosis and immune response and as a therapeutic target for LUAD.
A previous study using immunohistochemistry has reported an increased expression of HSPD1 and its significant correlation with the prognosis of patients with LUAD [25]. Overexpression of HSPD1 has been shown to correlate with HSP10 expression, and LUAD patients with high expression of HSP60 and HSP10 proteins show poor overall survival [26]. Nevertheless, these studies aimed to assess the prognostic impact of HSPD1 for LUAD patients, but the role of HSPD1 in cancer development and progression has not been fully explored. To our knowledge, only a study by Parma et al. has demonstrated the role of HSPD1 in promoting lung cancer development through the modulation of mitochondrial metabolism [27]. However, the precise role and underlying mechanism of HSPD1 in LUAD carcinogenesis remain largely unknown, and its association with the immune response has not been reported.
Recently, several omics datasets and bioinformatics tools have become publicly available, enabling further comprehensive studies of cancers. In this study, we thus applied an integrated bioinformatic approach to gain a more comprehensive insight into prognostic and functional significance of HSPD1 in LUAD. We first investigated the expression and prognostic significance of HSPD1 in LUAD using several databases. A significantly increased expression of HSPD1 in LUAD tissue was found at both mRNA and protein levels in multiple databases. The HSPD1 expression was significantly associated with cancer stages and was an independent prognostic factor for survival in LUAD patients. The patients with high HSPD1 expression had a poorer prognosis than those with low HSPD1 expression, confirming the prognostic significance of HSPD1 expression in LUAD. Further analyses also showed that most of HSPD1-positively correlated genes were significantly predictive of poor overall survival, while the favorable outcome of LUAD patients was associated with HSPD1-negatively correlated genes. Our findings were consistent with previous studies [25, 26, 27] in supporting the role of HSPD1 as a prognostic marker for LUAD. Additionally, our findings provided novel insights into the potential underlying mechanism of HSPD1 in LUAD progression and metastasis through regulation of ribosome biogenesis, and demonstrated a possible relationship between HSPD1 and immune cell infiltration in LUAD.
GO and KEGG pathway enrichment analyses of HSPD1-correlated genes highlighted a potential role of HSPD1 involved in ribosome biogenesis in LUAD. Several studies have demonstrated that ribosome biogenesis and ribosomal protein (RP) play important roles in tumorigenesis and cancer progression. An increase in ribosome biogenesis is associated with tumor growth, progression, and drug resistance in many cancers [46, 47, 48]. In LUAD, an elevated level of phosphorylated-RPS6 was associated with metastasis and an unfavorable prognosis [49, 50]. Knockdown of RPS6 suppressed the growth of NSCLC cells through induction of the cell cycle arrest [51]. A recent study has reported an increased expression of RPL32 in LUAD tissues. Its overexpression was associated with poor prognosis. Knockdown of RPL32 induced ribosomal stress, resulting in the accumulation of p53 and inhibition of lung cancer cell proliferation [52]. Furthermore, it has been shown that treatment with triptolide, an extract from the Chinese herb Tripterygium wilfordii, induced cell cycle arrest and apoptosis in NSCLC cells through perturbation of ribosome biogenesis [53]. These findings indicated the crucial roles of RPs and ribosome biogenesis in LUAD development. Although a direct function of HSPD1 in ribosome biogenesis has not been clearly elucidated, previous studies have demonstrated their relationship in cancer development. It has been shown that knockdown of HSPD1 results in the downregulation of RPs and a decrease in protein synthesis, leading to growth inhibition of ovarian cancer cells and glioblastoma cells [54, 55]. Therefore, the positive correlation between HSPD1 and ribosome biogenesis marker gene expressions observed in our analyses suggested that HSPD1 may exert a tumor-promoting activity in LUAD through the regulation of ribosome biogenesis.
HSPD1 is well known for its modulatory activity on many types of immune cells [15]. Composition of TIICs is related with clinical outcomes and therapeutic response in LUAD patients [6, 7, 8, 9, 10, 11]. Therefore, it was of interest to investigate an association between HSPD1 expression and TIICs in LUAD. Among several types of TIICs, our analyses showed that HSPD1 expression was negatively correlated with infiltration level of B cell, particularly activated B cells and immature B cells. A low abundance of tumor-infiltrating B cells was strongly associated with poor overall survival of the patients, suggesting a protective role of B cells in LUAD. There is increasing evidence demonstrating that B cells play an important role in anti-tumor immunity in lung cancers [56]. A decrease in tumor-infiltrating B cells was associated with poor survival in LUAD patients [8]. The presence of B cells in tertiary lymphoid structures in TME was positively correlated with tumor-specific antibody response, high level of activated CD4+T cells and low level of regulatory T cells in NSCLS patients [57, 58, 59]. Recently, it has been shown that depletion of B cells by anti-CD20 antibody accelerates tumorigenesis and tumor progression in a murine lung cancer model through suppression of tumor infiltration of immune cells [59]. Hence, it was possible that HSPD1 expression might affect B cell-mediated immune response, thereby regulating anti-tumor immunity in LUAD.
Speculatively, it is possible that HSPD1 overexpression could potentially contribute to the occurrence of abnormal ribosome biogenesis and disrupt immune responses, resulting in limited B cell and CD4+ T cell infiltration. However, some limitations of this study should be noted. First, our analyses were based on the limited datasets publicly available online on internet. More datasets would help strengthen the findings of this study. Second, bioinformatic analyses are susceptible to various confounding factors, and the obtained data may not be entirely accurate, necessitating experimental validations. Examining the effects of HSPD1 using gene knockdown and overexpression experiments in relevant cell lines or animal models, followed by comprehensive molecular and cellular analyses in response to subsequent changes in specific signaling pathways and its interaction, would provide direct evidence of the functional role of HSPD1 in aberrant ribosome biogenesis and dysregulated immune responses. Further studies are warranted to assess clinical significance of HSPD1 in LUAD development and immunotherapy responses
In summary, HSPD1 expression was significantly higher in LUAD tissues. Its overexpression was associated with poor survival in patients with LUAD. HSPD1 had a potential role involved in the dysregulation of ribosome biogenesis. HSPD1 expression was negatively correlated with B cell infiltration and might be a predictive biomarker for prognosis and immunotherapy response in LUAD.
Author contributions
Conception: SA.
Interpretation or analysis of data: SA, KS, and JN.
Preparation of the manuscript: SA, SC, and SP.
Revision for important intellectual content: SA, SC, and SP.
Supervision: SC and SP.
Supplementary data
The supplementary files are available to download from http://dx.doi.org/10.3233/CBM-220442.
Acknowledgments
This work (Grant No. RGNS 65-164) was supported by Office of the Permanent Secretary, Ministry of Higher Education, Science, Research and Innovation (OPS MHESI), Thailand Science Research and Innovation (TSRI) and Mae Fah Luang University. Sutatip Pongcharoen received research grant from the National Science, Research and Innovation Fund (NSRF, grant no. R2565B001 and R2566B001 as a continual grant).
References
[1] | C. Mattiuzzi and G. Lippi, Current Cancer Epidemiology, J Epidemiol Glob Health 9: ((2019) ), 217–222. |
[2] | C. Zappa and S.A. Mousa, Non-small cell lung cancer: current treatment and future advances, Transl Lung Cancer Res 5: ((2016) ), 288–300. |
[3] | T.V. Denisenko, I.N. Budkevich and B. Zhivotovsky, Cell death-based treatment of lung adenocarcinoma, Cell Death Dis 9: ((2018) ), 117. |
[4] | V. Sosa Iglesias, L. Giuranno, L.J. Dubois, J. Theys and M. Vooijs, Drug Resistance in Non-Small Cell Lung Cancer: A Potential for NOTCH Targeting? Front Oncol 8: ((2018) ), 267. |
[5] | C. Jochems and J. Schlom, Tumor-infiltrating immune cells and prognosis: the potential link between conventional cancer therapy and immunity, Exp Biol Med (Maywood) 236: ((2011) ), 567–579. |
[6] | K. Suzuki, S.S. Kachala, K. Kadota, R. Shen, Q. Mo, D.G. Beer, V.W. Rusch, W.D. Travis and P.S. Adusumilli, Prognostic immune markers in non-small cell lung cancer, Clin Cancer Res 17: ((2011) ), 5247–5256. |
[7] | X. Liu, S. Wu, Y. Yang, M. Zhao, G. Zhu and Z. Hou, The prognostic landscape of tumor-infiltrating immune cell and immunomodulators in lung cancer, Biomed Pharmacother 95: ((2017) ), 55–61. |
[8] | H.E. Lee, L. Luo, T. Kroneman, M.R. Passow, K.M. Del Rosario, M.R. Christensen, M.E. Francis, J.W. O’Shaughnessy, A.J. Blahnik, P. Yang and E.S. Yi, Increased Plasma Cells and Decreased B-cells in Tumor Infiltrating Lymphocytes are Associated with Worse Survival in Lung Adenocarcinomas, J Clin Cell Immunol 11: ((2020) ). |
[9] | J. Sun, Z. Zhang, S. Bao, C. Yan, P. Hou, N. Wu, J. Su, L. Xu and M. Zhou, Identification of tumor immune infiltration-associated lncRNAs for improving prognosis and immunotherapy response of patients with non-small cell lung cancer, Journal for Immunotherapy of Cancer 8: ((2020) ), e000110. |
[10] | J.E. Schoenhals, S.N. Seyedin, C. Anderson, E.D. Brooks, Y.R. Li, A.I. Younes, S. Niknam, A. Li, H.B. Barsoumian, M.A. Cortez and J.W. Welsh, Uncovering the immune tumor microenvironment in non-small cell lung cancer to understand response rates to checkpoint blockade and radiation, Translational Lung Cancer Research 6: ((2017) ), 148–158. |
[11] | L. Li, G. Lu, Y. Liu, L. Gong, X. Zheng, H. Zheng, W. Gu and L. Yang, Low Infiltration of CD8+ PD-L1+ T Cells and M2 Macrophages Predicts Improved Clinical Outcomes After Immune Checkpoint Inhibitor Therapy in Non-Small Cell Lung Carcinoma, Frontiers in Oncology 11: ((2021) ). |
[12] | H.H. Kampinga, J. Hageman, M.J. Vos, H. Kubota, R.M. Tanguay, E.A. Bruford, M.E. Cheetham, B. Chen and L.E. Hightower, Guidelines for the nomenclature of the human heat shock proteins, Cell Stress Chaperones 14: ((2009) ), 105–111. |
[13] | S. Aluksanasuwan, K. Sueksakit, K. Fong-Ngern and V. Thongboonkerd, Role of HSP60 (HSPD1) in diabetes-induced renal tubular dysfunction: regulation of intracellular protein aggregation, ATP production, and oxidative stress, Faseb J 31: ((2017) ), 2157–2167. |
[14] | F. Cappello, E. Conway de Macario, L. Marasà, G. Zummo and A.J. Macario, Hsp60 expression, new locations, functions and perspectives for cancer diagnosis and therapy, Cancer Biol Ther 7: ((2008) ), 801–809. |
[15] | F.J. Quintana and I.R. Cohen, The HSP60 immune system network, Trends Immunol 32: ((2011) ), 89–95. |
[16] | C.W. Yun, H.J. Kim, J.H. Lim and S.H. Lee, Heat Shock Proteins: Agents of Cancer Development and Therapeutic Targets in Anti-Cancer Therapy, Cells 9: ((2019) ). |
[17] | A. Fucarino and A. Pitruzzella, Role of HSP60/HSP10 in Lung Cancer: Simple Biomarkers or Leading Actors? J Oncol 2020: ((2020) ), 4701868. |
[18] | J. Wu, T. Liu, Z. Rios, Q. Mei, X. Lin and S. Cao, Heat Shock Proteins and Cancer, Trends in Pharmacological Sciences 38: ((2017) ), 226–256. |
[19] | Y.-P. Tsai, M.-H. Yang, C.-H. Huang, S.-Y. Chang, P.-M. Chen, C.-J. Liu, S.-C. Teng and K.-J. Wu, Interaction between HSP60 and β-catenin promotes metastasis, Carcinogenesis 30: ((2009) ), 1049–1057. |
[20] | C. Campanella, F. Bucchieri, N.M. Ardizzone, A. Marino Gammazza, A. Montalbano, A. Ribbene, V. Di Felice, M. Bellafiore, S. David, F. Rappa, M. Marasà, G. Peri, F. Farina, A.M. Czarnecka, E. Conway de Macario, A.J. Macario, G. Zummo and F. Cappello, Upon oxidative stress, the antiapoptotic Hsp60/procaspase-3 complex persists in mucoepidermoid carcinoma cells, Eur J Histochem 52: ((2008) ), 221–228. |
[21] | A. Marino Gammazza, C. Campanella, R. Barone, C. Caruso Bavisotto, M. Gorska, M. Wozniak, F. Carini, F. Cappello, A. D’Anneo, M. Lauricella, G. Zummo, E. Conway de Macario, A.J. Macario and V. Di Felice, Doxorubicin anti-tumor mechanisms include Hsp60 post-translational modifications leading to the Hsp60/p53 complex dissociation and instauration of replicative senescence, Cancer Lett 385: ((2017) ), 75–86. |
[22] | C. Zhou, H. Sun, C. Zheng, J. Gao, Q. Fu, N. Hu, X. Shao, Y. Zhou, J. Xiong, K. Nie, H. Zhou, L. Shen, H. Fang and J. Lyu, Oncogenic HSP60 regulates mitochondrial oxidative phosphorylation to support Erk1/2 activation during pancreatic cancer cell growth, Cell Death Dis 9: ((2018) ), 161. |
[23] | W. Pei, K. Tanaka, S.C. Huang, L. Xu, B. Liu, J. Sinclair, J. Idol, G.K. Varshney, H. Huang, S. Lin, R.B. Nussenblatt, R. Mori and S.M. Burgess, Extracellular HSP60 triggers tissue regeneration and wound healing by regulating inflammation and cell proliferation, NPJ Regen Med 1: ((2016) ), 16013. |
[24] | A. Osterloh, F. Geisinger, M. Piédavent, B. Fleischer, N. Brattig and M. Breloer, Heat shock protein 60 (HSP60) stimulates neutrophil effector functions, J Leukoc Biol 86: ((2009) ), 423–434. |
[25] | X. Xu, W. Wang, W. Shao, W. Yin, H. Chen, Y. Qiu, M. Mo, J. Zhao, Q. Deng and J. He, Heat shock protein-60 expression was significantly correlated with the prognosis of lung adenocarcinoma, J Surg Oncol 104: ((2011) ), 598–603. |
[26] | Y. Tang, Y. Yang, J. Luo, S. Liu, Y. Zhan, H. Zang, H. Zheng, Y. Zhang, J. Feng, S. Fan and Q. Wen, Overexpression of HSP10 correlates with HSP60 and Mcl-1 levels and predicts poor prognosis in non-small cell lung cancer patients, Cancer Biomark 30: ((2021) ), 85–94. |
[27] | B. Parma, V. Ramesh, P.N. Gollavilli, A. Siddiqui, L. Pinna, A. Schwab, S. Marschall, S. Zhang, C. Pilarsky, F. Napoli, M. Volante, S. Urbanczyk, D. Mielenz, H.D. Schrøder, M. Stemmler, H. Wurdak and P. Ceppi, Metabolic impairment of non-small cell lung cancers by mitochondrial HSPD1 targeting, J Exp Clin Cancer Res 40: ((2021) ), 248. |
[28] | C. Sangiorgi, D. Vallese, I. Gnemmi, F. Bucchieri, B. Balbi, P. Brun, A. Leone, A. Giordano, E. Conway de Macario, A.J. Macario, F. Cappello and A. Di Stefano, HSP60 activity on human bronchial epithelial cells, Int J Immunopathol Pharmacol 30: ((2017) ), 333–340. |
[29] | T. Li, J. Fan, B. Wang, N. Traugh, Q. Chen, J.S. Liu, B. Li and X.S. Liu, TIMER: A Web Server for Comprehensive Analysis of Tumor-Infiltrating Immune Cells, Cancer Res 77: ((2017) ), e108–e110. |
[30] | B. Li, E. Severson, J.C. Pignon, H. Zhao, T. Li, J. Novak, P. Jiang, H. Shen, J.C. Aster, S. Rodig, S. Signoretti, J.S. Liu and X.S. Liu, Comprehensive analyses of tumor immunity: implications for cancer immunotherapy, Genome Biol 17: ((2016) ), 174. |
[31] | Z. Tang, B. Kang, C. Li, T. Chen and Z. Zhang, GEPIA2: an enhanced web server for large-scale expression profiling and interactive analysis, Nucleic Acids Res 47: ((2019) ), W556–w560. |
[32] | D.S. Chandrashekar, B. Bashel, S.A.H. Balasubramanya, C.J. Creighton, I. Ponce-Rodriguez, B. Chakravarthi and S. Varambally, UALCAN: A Portal for Facilitating Tumor Subgroup Gene Expression and Survival Analyses, Neoplasia 19: ((2017) ), 649–658. |
[33] | F. Pontén, K. Jirström and M. Uhlen, The Human Protein Atlas–a tool for pathology, J Pathol 216: ((2008) ), 387–393. |
[34] | M. Uhlén, L. Fagerberg, B.M. Hallström, C. Lindskog, P. Oksvold, A. Mardinoglu, Å. Sivertsson, C. Kampf, E. Sjöstedt, A. Asplund, I. Olsson, K. Edlund, E. Lundberg, S. Navani, C.A. Szigyarto, J. Odeberg, D. Djureinovic, J.O. Takanen, S. Hober, T. Alm, P.H. Edqvist, H. Berling, H. Tegel, J. Mulder, J. Rockberg, P. Nilsson, J.M. Schwenk, M. Hamsten, K. von Feilitzen, M. Forsberg, L. Persson, F. Johansson, M. Zwahlen, G. von Heijne, J. Nielsen and F. Pontén, Proteomics. Tissue-based map of the human proteome, Science 347: ((2015) ), 1260419. |
[35] | B. Györffy, P. Surowiak, J. Budczies and A. Lánczky, Online survival analysis software to assess the prognostic value of biomarkers using transcriptomic data in non-small-cell lung cancer, PLoS One 8: ((2013) ), e82241. |
[36] | Z. Yan, Q. Wang, Z. Lu, X. Sun, P. Song, Y. Dang, L. Xie, L. Zhang, Y. Li, W. Zhu, T. Xie, J. Ma, Y. Zhang and X. Guo, OSluca: An Interactive Web Server to Evaluate Prognostic Biomarkers for Lung Cancer, Front Genet 11: ((2020) ), 420. |
[37] | D. Warde-Farley, S.L. Donaldson, O. Comes, K. Zuberi, R. Badrawi, P. Chao, M. Franz, C. Grouios, F. Kazi, C.T. Lopes, A. Maitland, S. Mostafavi, J. Montojo, Q. Shao, G. Wright, G.D. Bader and Q. Morris, The GeneMANIA prediction server: biological network integration for gene prioritization and predicting gene function, Nucleic Acids Res 38: ((2010) ), W214–220. |
[38] | W. Huang da, B.T. Sherman and R.A. Lempicki, Systematic and integrative analysis of large gene lists using DAVID bioinformatics resources, Nat Protoc 4: ((2009) ), 44–57. |
[39] | W. Huang da, B.T. Sherman and R.A. Lempicki, Bioinformatics enrichment tools: paths toward the comprehensive functional analysis of large gene lists, Nucleic Acids Res 37: ((2009) ), 1–13. |
[40] | T. Zhao and Z. Wang, GraphBio: A shiny web app to easily perform popular visualization analysis for omics data, Front Genet 13: ((2022) ), 957317. |
[41] | B. Ru, C.N. Wong, Y. Tong, J.Y. Zhong, S.S.W. Zhong, W.C. Wu, K.C. Chu, C.Y. Wong, C.Y. Lau, I. Chen, N.W. Chan and J. Zhang, TISIDB: an integrated repository portal for tumor-immune system interactions, Bioinformatics 35: ((2019) ), 4200–4202. |
[42] | C. Deisenroth and Y. Zhang, Ribosome biogenesis surveillance: probing the ribosomal protein-Mdm2-p53 pathway, Oncogene 29: ((2010) ), 4253–4260. |
[43] | I. Oršolić, S. Bursać, D. Jurada, I. Drmić Hofman, Z. Dembić, J. Bartek, I. Mihalek and S. Volarević, Cancer-associated mutations in the ribosomal protein L5 gene dysregulate the HDM2/ p53-mediated ribosome biogenesis checkpoint, Oncogene 39: ((2020) ), 3443–3457. |
[44] | A. Zisi, J. Bartek and M.S. Lindström, Targeting Ribosome Biogenesis in Cancer: Lessons Learned and Way Forward, Cancers (Basel) 14: ((2022) ). |
[45] | X. Zhou, W.J. Liao, J.M. Liao, P. Liao and H. Lu, Ribosomal proteins: functions beyond the ribosome, J Mol Cell Biol 7: ((2015) ), 92–104. |
[46] | A. Pecoraro, M. Pagano, G. Russo and A. Russo, Ribosome Biogenesis and Cancer: Overview on Ribosomal Proteins, Int J Mol Sci 22: ((2021) ). |
[47] | S. Nait Slimane, V. Marcel, T. Fenouil, F. Catez, J.C. Saurin, P. Bouvet, J.J. Diaz and H.C. Mertani, Ribosome Biogenesis Alterations in Colorectal Cancer, Cells 9: ((2020) ). |
[48] | M. Penzo, L. Montanaro, D. Treré and M. Derenzini, The Ribosome Biogenesis-Cancer Connection, Cells 8: ((2019) ). |
[49] | J.M. McDonald, C.E. Pelloski, A. Ledoux, M. Sun, G. Raso, R. Komaki, Wistuba, II, B.N. Bekele and K. Aldape, Elevated phospho-S6 expression is associated with metastasis in adenocarcinoma of the lung, Clin Cancer Res 14: ((2008) ), 7832–7837. |
[50] | B. Chen, Z. Tan, J. Gao, W. Wu, L. Liu, W. Jin, Y. Cao, S. Zhao, W. Zhang, Z. Qiu, D. Liu, X. Mo and W. Li, Hyperphosphorylation of ribosomal protein S6 predicts unfavorable clinical survival in non-small cell lung cancer, J Exp Clin Cancer Res 34: ((2015) ), 126. |
[51] | B. Chen, W. Zhang, J. Gao, H. Chen, L. Jiang, D. Liu, Y. Cao, S. Zhao, Z. Qiu, J. Zeng, S. Zhang and W. Li, Downregulation of ribosomal protein S6 inhibits the growth of non-small cell lung cancer by inducing cell cycle arrest, rather than apoptosis, Cancer Lett 354: ((2014) ), 378–389. |
[52] | J. Xie, W. Zhang, X. Liang, C. Shuai, Y. Zhou, H. Pan, Y. Yang and W. Han, RPL32 Promotes Lung Cancer Progression by Facilitating p53 Degradation, Mol Ther Nucleic Acids 21: ((2020) ), 75–85. |
[53] | J. Wang, Z.Q. Zhang, F.Q. Li, J.N. Chen, X. Gong, B.B. Cao and W. Wang, Triptolide interrupts rRNA synthesis and induces the RPL23-MDM2-p53 pathway to repress lung cancer cells, Oncol Rep 43: ((2020) ), 1863–1874. |
[54] | J. Guo, X. Li, W. Zhang, Y. Chen, S. Zhu, L. Chen, R. Xu, Y. Lv, D. Wu, M. Guo, X. Liu, W. Lu and H. Deng, HSP60-regulated Mitochondrial Proteostasis and Protein Translation Promote Tumor Growth of Ovarian Cancer, Sci Rep 9: ((2019) ), 12628. |
[55] | H. Tang, J. Li, X. Liu, G. Wang, M. Luo and H. Deng, Down-regulation of HSP60 Suppresses the Proliferation of Glioblastoma Cells via the ROS/AMPK/mTOR Pathway, Sci Rep 6: ((2016) ), 28388. |
[56] | S.S. Wang, W. Liu, D. Ly, H. Xu, L. Qu and L. Zhang, Tumor-infiltrating B cells: their role and application in anti-tumor immunity in lung cancer, Cell Mol Immunol 16: ((2019) ), 6–18. |
[57] | C. Germain, S. Gnjatic, F. Tamzalit, S. Knockaert, R. Remark, J. Goc, A. Lepelley, E. Becht, S. Katsahian, G. Bizouard, P. Validire, D. Damotte, M. Alifano, P. Magdeleinat, I. Cremer, J.L. Teillaud, W.H. Fridman, C. Sautès-Fridman and M.C. Dieu-Nosjean, Presence of B cells in tertiary lymphoid structures is associated with a protective immunity in patients with lung cancer, Am J Respir Crit Care Med 189: ((2014) ), 832–844. |
[58] | T.C. Bruno, P.J. Ebner, B.L. Moore, O.G. Squalls, K.A. Waugh, E.B. Eruslanov, S. Singhal, J.D. Mitchell, W.A. Franklin, D.T. Merrick, M.D. McCarter, B.E. Palmer, J.A. Kern and J.E. Slansky, Antigen-Presenting Intratumoral B Cells Affect CD4(+) TIL Phenotypes in Non-Small Cell Lung Cancer Patients, Cancer Immunol Res 5: ((2017) ), 898–907. |
[59] | C. Germain, P. Devi-Marulkar, S. Knockaert, J. Biton, H. Kaplon, L. Letaïef, J. Goc, A. Seguin-Givelet, D. Gossot, N. Girard, P. Validire, M. Lefèvre, D. Damotte, M. Alifano, F.M. Lemoine, K.E. Steele, J.L. Teillaud, S.A. Hammond and M.C. Dieu-Nosjean, Tertiary Lymphoid Structure-B Cells Narrow Regulatory T Cells Impact in Lung Cancer Patients, Front Immunol 12: ((2021) ), 626776. |