Modulation of Distinct Intrinsic Resting State Brain Networks by Acute Exercise Bouts of Differing Intensity
Abstract
Acute exercise bouts alter resting state functional connectivity (rs-FC) within cognitive, sensorimotor, and affective networks, but it remains unknown how these effects are influenced by exercise intensity.
Twenty-five male athletes underwent individual fitness assessments using an incremental treadmill test. On separate days, they performed ‘low’ (35% below lactate threshold) and ‘high’ (20% above lactate threshold) intensity exercise bouts of 30 min. Rs-fMRI and Positive and Negative Affect Scale (PANAS) were acquired before and after each exercise bout. Networks of interest were extracted from twenty-two participants (3 dropouts). Pre-to-post changes and between conditions effects were evaluated using FSL’s randomise by applying repeated measures ANOVA. Results were reported at p < 0.05, corrected for multiple comparisons using threshold free cluster enhancement.
PANAS revealed a significant increase in positive mood after both exercise conditions. Significant effects were observed between conditions in the right affective and reward network (ARN), the right fronto parietal network (FPN) and the sensorimotor network (SMN). Pre-to-post comparisons after ‘low’ exercise intensity revealed a significant increase in rs-FC in the left and right FPN, while after ‘high’-intensity exercise rs-FC decreased in the SMN and the dorsal attention network (DAN) and increased in the left ARN.
Supporting recent findings, this study is the first to report distinct rs-FC alterations driven by exercise intensity: (i) Increased rs-FC in FPN may indicate beneficial functional plasticity for cognitive/attentional processing, (ii) increased rs-FC in ARN may be linked to endogenous opioid-mediated internal affective states. Finally, (iii) decreased rs-FC in the SMN may signify persistent motor fatigue. The distinct effects on rs-FC fit with theories of transient persistent network alterations after acute exercise bouts that are mediated by different exercise intensities and impact differentially on cognitive/attentional or affective responses.
INTRODUCTION
Acute physical exercise bouts are known to enhance affective, cognitive and attentional processes in a range of minutes to hours after exercise bouts. One possible mechanism by which such transient improvements appear to be mediated is an alteration of coherent neuronal activity that persists after exercise. Such coherent neuronal activity is termed “resting state functional connectivity” (rs-FC) and brain regions that are functionally connected via distinct temporo-spatial properties are referred to as “functional networks”. Resting state functional magnetic resonance imaging (rs-fMRI) is a potential tool to measure such transient coherent neuronal activity alterations that persist after acute exercise bouts.
Most of the previous rs-fMRI literature was, however, not focused on investigating the transient effects of acute exercise bouts, but rather on long-term effects of repeated exercise training over several months [1–4]: Stronger rs-FC was reported between the right parahippocampal gyrus and motor, sensory, and mood regulating areas after 16 weeks of training [4]. On the other hand, 12 weeks of exercise induced increased FC between the default mode network (DMN) and primary motor areas [3], eventually indicating improved motor performance. Conversely, another study found no significant changes in intrinsic brain activity after a six months of aerobic exercise [2].
Less is known about the transient effects of acute exercise bouts on rs-FC: A recently published study using rs-fMRI in both older and younger healthy volunteers reported a modulation of rs-FC after a moderate exercise session on a bicycle ergometer compared to a passive control condition [5]. The authors found a significant increase in rs-FC in the affective-reward network (ARN), the hippocampal and the right executive control network (ECN) after exercising at 65% of their HRmax (maximum heart rate). Another study investigating the effects of a single moderate aerobic exercise session in young healthy subjects found transient changes in FC in the auditory resting state network (RSN), the sensorimotor network (SMN) and the thalamic-caudate RSN [6]. Taken together, these initial findings in the acute setting suggest that single exercise bouts induce transient changes in intrinsic brain activity that can be captured by rs-fMRI. Although, these studies generally report enhanced FC of intrinsic brain networks, it still remains unknown how these effects are influenced by the intensity of the exercise bout. It is relevant to point out, based on meta-analytical data (independent of the imaging context) that the effect on cognitive performance after exercise is influenced by the intensity of the exercise and notably also by the time between the end of the exercise bout and the start of the cognitive testing [7]. When cognitive performance is acquired with a delay of more than 1 min, more intense exercise (very hard) appears to be more beneficial than lighter intensity exercise [7], whereas, when cognitive testing is performed immediately after the exercise bout, lighter intensity exercise (very light, light, moderate) appears to be more beneficial than high intensity exercise [7]. Chang and colleagues found that distinct types of brain executive control functions tested with the ‘Tower of London’ task are differentially improved by different intensities of acute exercise [8, 9].
Studies using MRI to investigate the neuronal underpinnings of transient effects of acute exercise bouts are rare. To the best of our knowledge, there have been only two studies comparing cerebral blood flow (CBF) after different intensities of acute exercise [10, 11], showing exercise intensity dependent changes in CBF. One of these studies found decreases in CBF after moderate exercise intensity in the anterior cingulate, bilateral thalamus, and bilateral insula, but not after low exercise intensity [11]. The other study found intensity dependent changes in CBF in the right somatosensory cortex in individuals with cerebrovascular disease. The CBF in the sensorimotor cortices was reduced following low-intensity exercise and increased following moderate-intensity exercise [10]. A recently published study by our own group comparing the effect of low and high intensity exercise bouts on emotional face processing could show distinct changes in the brain during fearful face processing [12]: Low-intensity exercise resulted in reduced brain activations in PCC / precuneus and high intensity exercise was associated with reduced fear-related activity in ventral basal ganglia structures. Taken together, the mentioned behavioral and imaging studies indicate distinct changes in cognitive, affective and brain function, dependent on exercise intensity.
In order to determine how the intensity of acute exercise transiently affects the intrinsic neuronal activity at rest, we designed this study to assess rs-FC changes after defined ‘low’ and ‘high’ exercise intensities (adapted to the individual fitness level of each participant). These two forms of exercise intensity (‘low’ and ‘high’) were chosen in this study in order to fill the open gap (i.e. effects of different exercise intensities on rs-fMRI) in the literature. We predicted that ‘high’-intensity (20% above lactate threshold) exercise would alter the FC at rest differently than ‘low’-intensity (35% below lactate threshold) exercise. This could be helpful in understanding the relation between exercise intensity and to identify interventions that specifically enhance the FC in different cognitive and affective networks.
MATERIALS AND METHODS
Participants
Twenty-five right-handed and trained male subjects (exercising at least 3 times / week for 45 minutes for the past two years) were recruited. Requirements for participation in the study were no current or prior history of head injury, no neurological or psychiatric illness, no orthopedic or general health problems that may have prohibited physical activity. Participants with MRI-contraindications (i.e. metal and/or electronic implants, claustrophobia, etc.) were excluded. To assemble a set of descriptive characteristics (e.g. age, education, handedness) several questionnaires were collected. Additionally, psychiatric questionnaires were carried out, including the Mini International Neuropsychiatric Interview, German Version 5.0.0 (MINI) [13], the State and Trait Anxiety Inventory (STAI) [14], and the Beck Depression Inventory (BDI) [15].
Three of 25 subjects had to be excluded from our final analyses due to injuries that prohibited exercise (caused by private activities, N = 1) or dropouts (N = 2), resulting in a final sample size of N = 22 subjects. According to the Edinburgh Handedness Inventory (EHI) [16], N = 21 subjects were right dominant (mean laterality quotient 83.4±15.1) and N = 1 was ambidextrous (laterality quotient: 21.74). None of the 22 participants showed results indicating psychiatric diseases (MINI). At study entrance, the volunteers’ mean BDI score was 1.1±1.9, indicating that all participants scored below the threshold value for clinically relevant depression (score ≤9: no depression). STAI trait scores were on average 30.8±6.2 (range: 20 = not being afraid to 80 = maximum intensity of anxiety). BDI and STAI state scores did not differ significantly between examination days. The mean verbal intelligence quotient estimated by a German vocabulary test was 106.4±9.8 [17], indicating a normal verbal intelligence distribution in this cohort. For a summary of the volunteer demographics see Table 1.
Table 1
Participants demographics
Variable | N = 22 |
Age (years) | 27.1±4.0 |
Education (years) | 17.9±2.7 |
Height (cm) | 182±6 |
Weight (kg) | 78.3±6.8 |
BMI (kg/m2) | 23.7±1.4 |
Resting HR (bpm) | 75.9±15.1 |
HRmax (bpm) | 194.2±7.2 |
peak running speed (km/h) | 16.1±1.3 |
Note: Data are presented as mean (±standard deviation). BMI = body mass index; bpm = beats per minute; HRmax =maximum heart rate.
Participants were informed about the aim of the study and received a written detailed explanation regarding all tests, potential discomforts, risks, and procedures employed in the investigation, after which they gave written consent. The study was approved by the local Ethics Committee of the University Hospital Bonn (Lfd. 340/13), according to national legislation and the Declaration of Helsinki.
Experimental procedure
The study consisted of 4 examination days: a pre-experimental session and three experimental sessions that were separated by at least 2 days (maximum 12 weeks; time period for completing the whole study including all three exercise conditions: 58±47 days). As this manuscript focuses exclusively on the comparison of standardized and metabolically defined exercise intensities, data from the self-selected condition will not be presented here and will be reported elsewhere. The time period for completing only the ‘low’ and the ‘high’ exercise condition was 37±46 days. Subjects were instructed not to change their training behavior and to continue their training as usual throughout the study (in order to maintain their fitness level). During the pre-experimental session, participants completed a screening by a physician at the German Sport University Cologne, including medical history, stethoscopy, and a 12 channel resting electrocardiogram (ECG: MAC 1200 ST, GE Medical Systems Information Technologies GmbH, Freiburg, Germany). In the following fitness assessment in form of an incremental treadmill test (without spiroergometry), the subjects’ physical performance was examined. The incremental treadmill test started at a speed of 5 km/h, with a 1% gradient set throughout (Woodway PPS Med, Woodway GmbH, Germany). Each stage lasted 3 minutes and running speed was increased by 1 km/h per stage. After each stage, a blood sample (20μL) was taken from the earlobe and used to measure blood lactate concentration. Additionally, at the end of each stage heart rate (POLAR, Kempele, Finland) and a rating of perceived exertion (RPE; via the BORG Scale) [18] were recorded. The RPE scale ranges from “6 – no exertion at all / extremely light” to “20 – maximal exertion”. Participants performed the incremental test until volitional exhaustion. Exhaustion was considered with the attainment of at least two of the following criteria: high levels of blood lactate (8–10 mmol·L-1); a perceived rate of exertion of ≥18 and/or a HR of±10 (beats per minute) of age-predicted maximum (220 - age) [19]. Blood lactate samples were analyzed using the BIOSEN C-Line lactate analyzer (EKF-diagnostic GmbH, Barleben, Germany).
To ensure comparable relative exercise intensities across participants, the lactate threshold (LT) was individually determined from a plot of blood lactate concentration against running speed. LT was defined as the first workload at which there was an increase in blood lactate concentration of 0.4 mmol/l above the baseline lactate [20]. During the three experimental sessions, different 30 minutes exercise bouts (i.e. ‘low’, ‘high’ and ‘self-selected’) were performed on a treadmill. Each exercise session consisted of a five minutes warm up at 5 km/h, followed by the main 30 minutes exercise and ended with a five minutes cool-down period at 5 km/h. Before exercising, resting blood pressure, HR, and blood lactate were recorded. During exercise, speed, HR, blood lactate, physical exertion were monitored. HR was recorded every minute, and blood lactate and physical exertion were recorded every 5 minutes. Exercise intensities were randomized across the three experimental sessions [21]. The ‘low’ exercise intensity was performed at 35% under LT to ensure a very easy exercise condition that challenges primarily the aerobic energy supply. Since an exercise intensity with a similar percentage above LT would not be feasible for a duration of 30 min, exercise intensity for the ‘high’ condition was set at 20% above LT. Directly pre and post exercise the mood questionnaire Positive and Negative Affect Schedule (PANAS) [22] was acquired. Rs-fMRI scans were performed on all three examination days pre and approximately 18±5 min post exercise bouts. To account for possible prolonged effects of exercise on resting HR, HR was monitored during MRI scans using a pulse oximetry transducer placed on the left index finger (BIOPAC Systems Inc., Goleta, CA, USA). Data was recorded with Software of Biopac (AcqKnowledge 4.1). In addition to the rs-fMRI, we also acquired other fMRI sequences (B0, CASL, task-based, structural). All participants were instructed to avoid exercise training 24 hours prior each testing session to minimize the acute influences of exercise prior to assessments. They were also instructed to refrain from alcohol intake 24 hours prior to examination and to refrain from drinking caffeine and eating two hours before the experiment.
MRI acquisition
All MRI examinations were performed at the Department of Radiology, University Hospital Bonn, with a 3T clinical MRI System (Ingenia 5.1.7, Philips Healthcare, Best, The Netherlands), equipped with an 8-channel head coil. Rs-fMRI data were collected using a T2*-weighted gradient-echo, echo-planar imaging (EPI) protocol sensitive to the blood oxygenation level-dependent (BOLD) contrast with 250 volumes: TR = 2595 ms, TE = 35 ms, FOV = 230×230×147 mm, image matrix = 64×64, flip angle = 90°, 3.59×3.59×3.59 mm3 voxels, interleaved acquisition (1,3,5,7…41; 2,4,6,8…40; first odd, then even) of 41 contiguous axial slices of 3.59 mm thickness. These images were obtained parallel to the anterior-posterior commissure plane with no inter-slice gap. For all rs-fMRI scans, we instructed participants to lay still with their eyes closed and to stay awake without thinking about anything in particular for 11 minutes. For anatomical reference a 3D-T1 weighted data set was acquired within each run with the following parameters: slice orientation: sagittal, acquisition matrix: 256×256, acquired voxel size: 1×1×1 mm3, sequence type: 3D FFE, TR: 7.6 ms, TE: 3.9 ms, flip angle: 15°, total scan duration: 4:39 min.
MRI data preprocessing
First, an average T1 image of the acquired structural images was created using the paired longitudinal registration [23] in SPM12 (Statistical Parametric Mapping version; Wellcome Department of Imaging Neuroscience, London, UK) implemented in Matlab (The Mathworks Inc., Sherborn, MA, USA). The averaged T1 image was then skull-stripped using FSL’s BET (Brain Extraction Technique) algorithm [24]. Functional data from all time points were evaluated using FSL toolbox (version 5.0.9; FMRIB Software Library, http://www.fmrib.ox.ac.uk/fsl). The preprocessing of the individuals’ rs-fMRIs included motion correction, brain extraction, and spatial smoothing with 6 mm FWHM (full width at half maximum). Due to the interleaved acquisition mode and the minimal effects of slice timing on rs-fMRI (as previously described in the literature [25]), we did not perform slice time correction. Functional images from each subject were registered to their average T1 structural image and then optimized using the boundary-based registration approach. Later, structural images were transformed to standard space using a non-linear registration tool (FNIRT). The resulting warp fields were then applied to the functional images. An additional motion correction was performed to remove the physiological noise components (preserving the autocorrelations) by using a robust ICA-based strategy for automatic removal of motion artifacts (ICA-AROMA) [26]. This approach removes (motion-related) spurious noise from rs-fMRI data to a larger extent than the regression approach by using 24 motion parameters or spike regression. To avoid the further loss of signals of interest, we did not regress out white matter (WM) and cerebrospinal fluid (CSF) in our main analysis; rather we used a gray matter (GM) mask to extract the independent components, later on [27]. The gray matter (GM) mask was created from the GM segments available in the segmentation toolbox of SPM12 (https://www.fil.ion.ucl.ac.uk/spm/software/spm12/). We thresholded GM segment at 0.2 to further remove overlapping voxels with WM and CSF and binarized the mask. We additionally performed a second analysis with nuisance regression before performing gICA. Results of this analysis are presented in the supplement.
Finally, we obtained denoised filtered functional data by band pass filtering the non aggressive denoised data from the ICA-AROMA to remove frequencies other than 0.009 to 0.1 Hz.
Creating functional connectivity maps
The denoised filtered functional data from every participant pre and post exercise of the ‘high’ and ‘low’ exercise conditions were registered from native EPI space to the MNI152 template (2 mm3 resolution) by applying the previously computed EPI-to-MNI transform matrices. After transformation, the denoised filtered functional data were inserted to the group-level independent components analysis (gICA) to create data-driven multivariate components. To do so, we temporally concatenated the time-points with the dimensional reduction approach in FSL melodic and constrained it to a GM mask to avoid the noise from WM and CSF signals. Twenty-five independent spatiotemporal components (ICs) were derived commonly across the study sample. On the basis of the previous literature [5, 6] and to further add to the knowledge of the effects of acute exercise on rs-FC, we selected the following 12 components (involved in affective processing, motor function, attention, executive and cognitive function) by visually inspecting every component and matching them with previous defined network templates [28]. The selected networks were: 1) Default Mode Network (DMN); 2) Sensorimotor Network (SMN); 3) Executive Control Network (ECN); 4) Salience Network (SAL); 5) Left Affective and Reward Network (LARN); 6) Right Affective and Reward Network (RARN); 7) Left Fronto Parietal Network (LFPN); 8) Right Fronto Parietal Network (RFPN); 9) Dorsal Attention Network (DAN), 10) Basal Ganglia Network (BGN), 11) Cerebellar Network (CBN), and (12) Hippocampal Network (HIP) (Fig. 1). In order to create the individual FC maps of each selected RSN per condition, we applied the dual regression approach [29, 30]. This is a two stage process: In the first stage, we regressed the averaged group map of each network to the individual denoised filtered functional data to get the timeseries - one timeseries per group-ICA component. In the second stage, we further generated the FC maps of each groupICA component for each individual subject from the regressed time series. Later on, these FC maps of each ICA per individual subject were inserted in the statistical analysis to evaluate the effect of exercise condition.
Fig. 1
Networks derived from group ICA decomposition. The colored network maps are presented at a threshold of Z > 2.3 based on the group ICA. BGN = Basal Ganglia Network; ECN = Executive Control Network; CBN = Cerebellar Network; DAN = Dorsal Attention Network; DMN = Default Mode Network; HIP = Hippocampal Network; LARN = Left Affective and Reward Network; LFPN = Left Fronto Parietal Network; RARN = Right Affective and Reward Network; RFPN = Right Fronto Parietal Network; SMN = Sensorimotor Network; R = right; L = left.
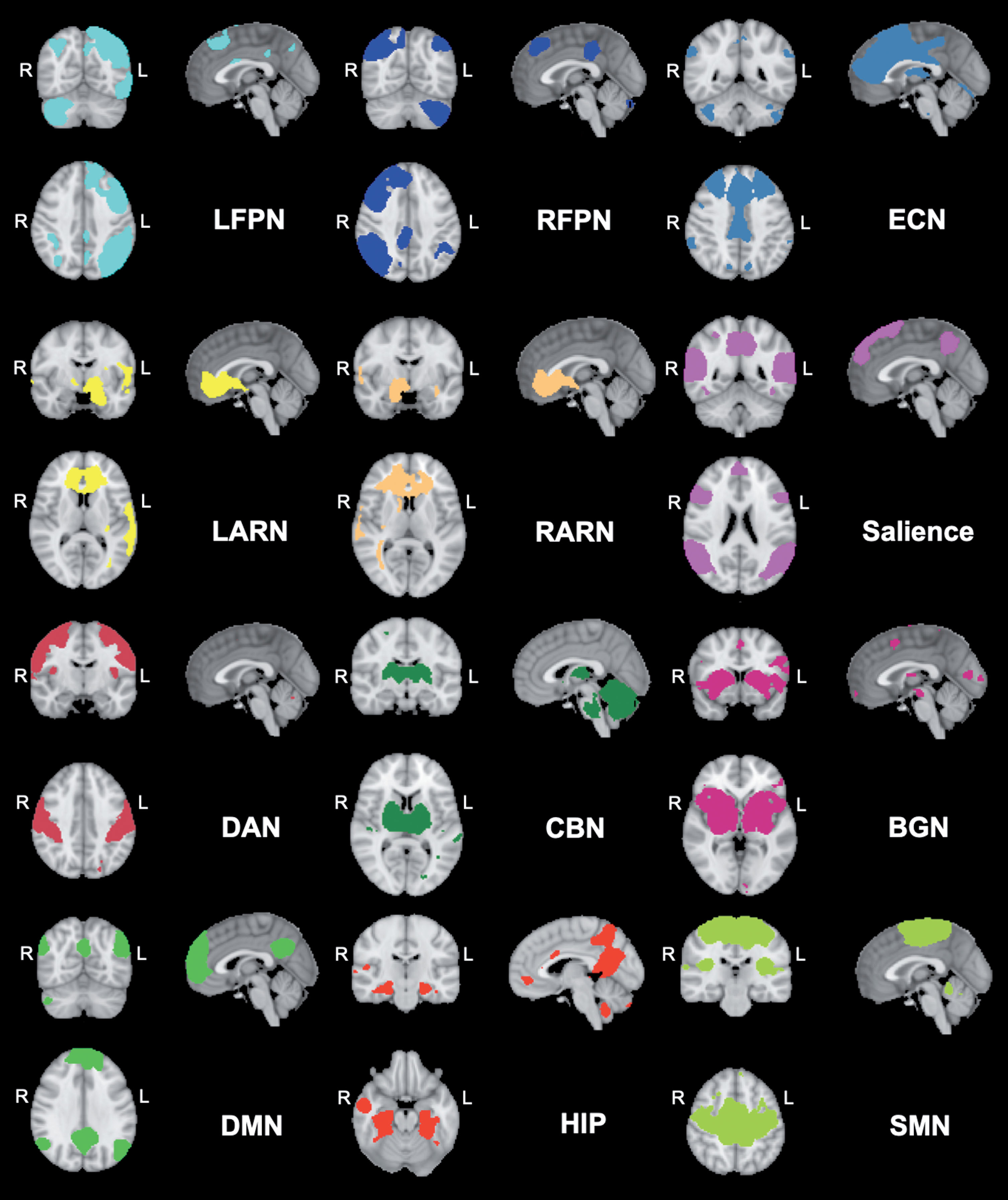
Statistical analysis
Behavioral data
All statistical analyses of behavioral data were performed using SPSS 25 (SPSS Inc., Chicago, Illinois). Using the Shapiro-Wilk-Test, data was tested for normal distribution. Tests revealed that the data of the PANAS negative affect scale were not normally distributed. However, simulation studies have shown that repeated measures ANOVA is relatively robust against violations of normal distribution assumptions, provided it is the only assumption that has been violated [31]. Therefore, PANAS scores for positive affect and negative affect were entered in two separate 2 (condition: ‘low’ / ’high’)×2 (time: pre / post exercise) repeated measures ANOVAs. Post hoc paired t-tests with Bonferroni correction were performed.
Physiological data
To compare ‘low’ vs ‘high’ exercise intensity, exercise-related parameters were analyzed using a paired t-test. For the exercise-related parameters speed and HR, average values for the 30 minutes of each exercise condition were used. Additionally, maximum values of blood lactate, HR and RPE were taken.
To analyze the HR monitored during MRI sessions, means for every single session were calculated and analyzed with a repeated-measures 2×2 ANOVA (with factors condition: ‘low’ / ‘high’ and time: pre / post exercise). Due to artifacts we could only include 18 subjects in the ANOVA that had a complete data set for ‘low’ and ‘high’. Additionally, post hoc paired t-tests with Bonferroni correction were performed. Significant results were reported at p-values of less than 0.05.
fMRI-data
To evaluate the modulation of rs-FC in the selected RSN’s in each condition from pre to post exercise, we created within-subject difference maps (post minus pre) for both exercise conditions. Then, we applied a one-tailed one sample t-test on these individual difference maps for each network to measure within condition effects. However, to evaluate the rs-FC changes between conditions (‘high vs low’) in each RSN (N = 12) individual difference maps were inserted into a paired t-test design. To apply for possible effects of HR changes induced by exercise, we transformed the mean HR-values during the resting state scan via Fisher's transformation into z-values and inserted these as covariate of no interest in both aforementioned designs. This analysis was only performed for sub-groups as we needed to exclude some data with artifacts in the HR recording. Due to missing HR data, the one sample t-test for ‘low’ and ‘high’ included N = 20 subjects. As the two subjects excluded for the ‘low’ condition were not the same two subjects which needed to be excluded in the ‘high’ condition, the analysis for comparing the exercise conditions included only N = 18 subjects (results are presented in the supplementary material). Modulations were assessed by randomizing each component per subject using non-parametric 5,000 random permutations, incorporating a threshold-free cluster enhancement technique (TFCE) [32]. The resulting clusters at p < 0.05 (surviving Z > 2.3 threshold after multiple comparison correction), were labeled using the AAL WFU PickAtlas [33, 34] and the Harvard-Oxford Cortical and Subcortical Structural Atlas provided by FSL’s software package [35]. Z-statistic values from normalized differential FC maps of pre to post scan in each condition were extracted per network using a mask created from the significant clusters of the ‘low’, ‘high’ and ‘low vs high’ conditions.
RESULTS
Acute exercise manipulation
During the ‘low’ exercise condition HR increased in the mean from HRrest (resting heart rate) of 62.0±6.3 to 119.2±15.8 bpm, which corresponds to 61.5±8.4% of the subjects HRmax. In the ‘high’ exercise condition subjects HR increased from a HRrest of 65.5±12.1 to 176.8±6.9 bpm, which corresponds to 91,1±3.3% of the subjects HRmax. These HR correspond to light (57–63% HRmax) and vigorous (77–95% HRmax) aerobic exercise intensity as defined by the American College of Sports Medicine (ACSM) [36]. Paired t-tests showed that the HRmax during ‘low’ (128.2±17.2 bpm) and ‘high’ (186.5±6.5 bpm) exercise intensity differ significantly (t(21) = –16.5; p < 0.001). The values of the mean perceived exertion of the ‘low’ exercise condition (9±2) and the ‘high’ exercise condition (17±1) also matched the intensity categorizations of the ACSM (light: 9–11; vigorous: 14–17) and differ significantly from each other (t(21) = –21.1; p < 0.001). Significant differences between the two exercise intensities were also found for the mean speed (‘low’: 7.1±0.7 km/h; ‘high’: 12.8±1.3 km/h; t(21) = –22.0; p < 0.001) and the maximum blood lactate concentration (‘low’: 2.8±1.9 mmol/L; ‘high’: 10.2±3.2 mmol/L; t(21) = –10.6; p < 0.001).
Physiological monitoring
Due to artifacts, N = 4 subjects needed to be removed from the analysis between conditions, resulting in a sample size of N = 18. The 2×2 ANOVA showed a significant main effect of time (F(1,17) = 115.93, p < 0.001), a main effect of condition (F(1,17) = 41.92, p < 0.001), and a significant time×condition interaction (F(1,17) = 82.24, p < 0.001). In the post hoc analysis N = 2 subjects needed to be excluded for the ‘low’ and also N = 2 for the ‘high’ exercise condition. Comparisons revealed that HR was significantly increased after the ‘high’ exercise bout compared to pre exercise (t(19) = –14.99, p < 0.001; pre ‘high’: 58.9±9.0 bpm, post ‘high’: 78.0±9.6 bpm). No significant difference in HR was found pre to post ‘low’ exercise (t(19) = –0.88, p = 0.390; pre ‘low’: 57.7±8.1 bpm, post ‘low’: 58.6±8.7 bpm). Comparing the differences of pre-post ‘low’ vs pre-post ‘high’ revealed a significant effect (t(17) = 9.07, p < 0.001), i.e. the increase from pre to post ‘high’ (19.1±5.7 bpm) was significantly greater than the increase from pre to post ‘low’ (1.0±5.5 bpm). No significant difference was found for HR between the two conditions pre exercise (baseline) (t(19) = –0.65, p = 0.524).
PANAS
Analyzing the positive affect scale using a 2×2 ANOVA revealed a significant main effect of time (F(1,21) = 27.09, p < 0.001). Post-hoc comparisons showed a significant increase for the ‘low’ exercise condition pre (34.0±8.5) to post (38.7±6.6) (t(21) = –5.47, p < 0.001) and the ‘high’ exercise condition pre (32.3±10.6) to post (38.8±6.9) (t(21) = –3.94, p = 0.001).
The ANOVA for the negative affect scale only revealed a significant main effect of condition (F(1,21) = 6.29, p = 0.020). Comparing ‘low’ versus ‘high’ post-hoc did not show any significant differences.
Acute exercise-induced changes in the functional connectivity
In our data analysis we focused on two aspects for each RSN of interest: 1) the interaction effects between time and intensity of the exercise bout and 2) the change from pre to post exercise bout for each intensity separately.
Right affect and reward network
In the RARN a significant time×condition interaction in rs-FC in the right insula has been observed (Fig. 2A, Table 2). This interaction was driven by an increase from pre to post ‘high’ exercise condition (trend only).
Fig. 2
FC changes observed significantly different between conditions (‘low’ and ‘high’ intensity). Significant clusters in the interaction of the: A) RARN in the right anterior insula; B) RFPN in the right superior frontal gyrus (SFG); C) SMN in the left and right postcentral gyrus, the precuneus and the right supplementary motor area (SMA); Data shown is corrected for p < 0.05, after multiple comparison correction using TFCE approach; z-values were normalized by dividing them by SD; For enhancing the contrast between the activation clusters and the RSN masks, we chose either blue or red for the activation clusters. The colors do not indicate increases or decreases. N = 22; R = right; L = left.
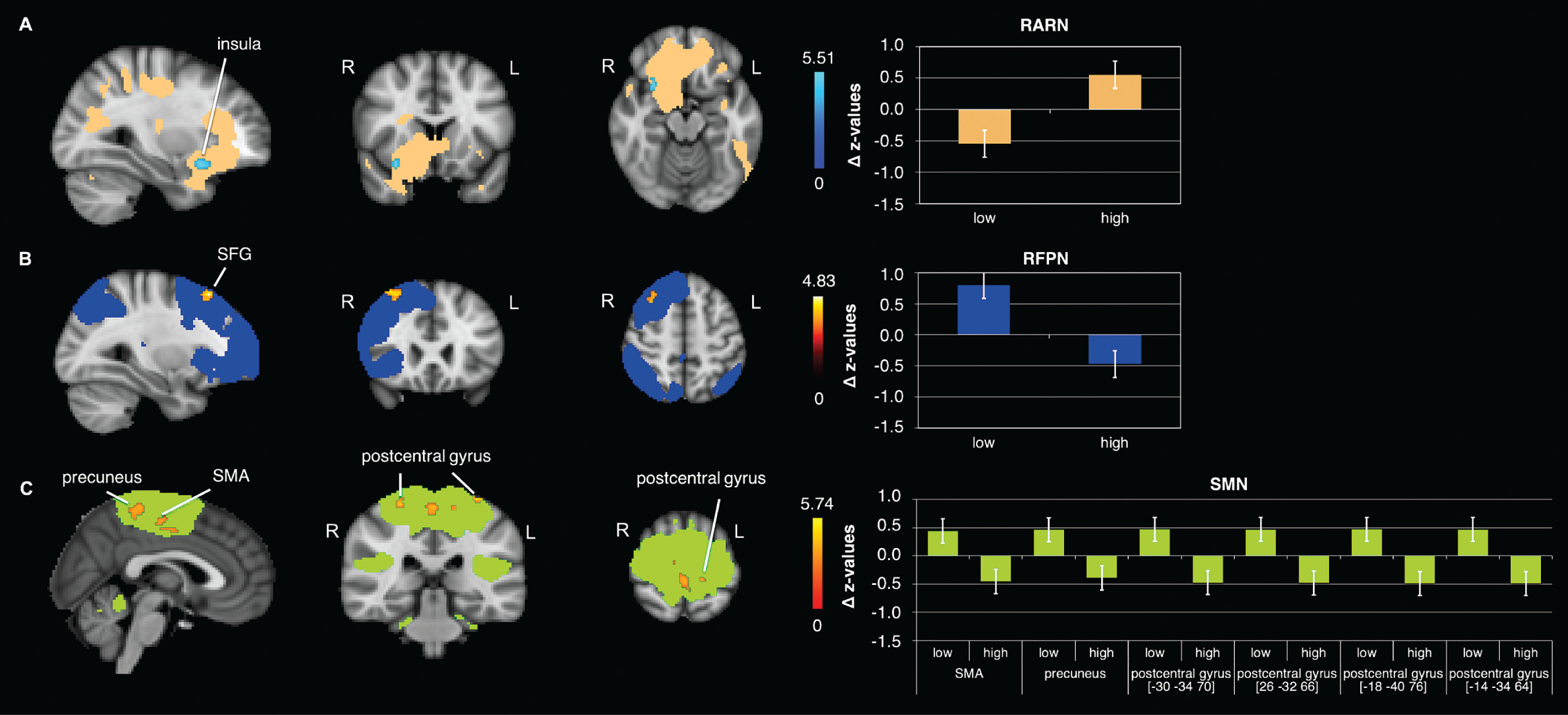
Table 2
Brain regions observed significantly different between conditions (‘low’ and ‘high’ intensity)
Brain region | side | k | x | y | z | p-value (tfce) |
RARN –‘high’ > ‘low’ | ||||||
anterior insula | R | 37 | 30 | 14 | –16 | 0.013 |
RFPN –‘low’> ‘high’ | ||||||
superior frontal gyrus | R | 103 | 30 | 26 | 54 | 0.022 |
SMN - ‘low’> ‘high’ | ||||||
precuneus | L | 184 | –6 | –42 | 56 | 0.015 |
supplementary motor area | R | 111 | 10 | –18 | 50 | 0.038 |
postcentral gyrus | L | 38 | –30 | –34 | 70 | 0.026 |
postcentral gyrus | R | 23 | 26 | –32 | 66 | 0.040 |
postcentral gyrus | L | 11 | –18 | –40 | 76 | 0.046 |
postcentral gyrus | L | 10 | –14 | –34 | 64 | 0.046 |
Coordinates presented are in MNI space; k = cluster size; R = right; L = left.
Right fronto parietal network
Results of the rs-FC analysis in the RFPN also indicated a significant time×condition interaction in the right superior frontal gyrus (SFG) (Fig. 2B, Table 2). This effect was driven by a strong increase in rs-FC from pre to post ‘low’ exercise condition (Fig. 3E, Table 3).
Fig. 3
Differential effects in rs-FC from pre to post within exercise condition. Rs-FC changes in the: A) ECN within the ‘low’ condition (post>pre) in the anterior cingulate cortex (ACC) (trend only, results are presented at p = 0.1), B) DAN within the ‘high’ condition (pre>post) in the postcentral gyrus, C) LARN within the ‘high’ condition (post>pre) in the cerebellar lobule 6, D) LFPN within the ‘low’ condition (post > pre) in the inferior parietal lobule (IPL), E) RFPN within the ‘low’ condition (post>pre) in the middle frontal gyrus (MFG), middle orbitofrontal gyrus (MOFG), inferior frontal gyrus (IFG) and superior frontal gyrus (SFG), F) SMN within the ‘high’ condition (pre>post) in the postcentral gyrus; data shown is corrected for p < 0.05, after multiple comparison correction using TFCE approach; *indicates significant changes in FC; z-values were normalized by dividing them by SD; For enhancing the contrast between the activation clusters and the RSN masks, we chose either blue or red for the activation clusters. The colors do not indicate increases or decreases. N = 22; R = right; L = left.
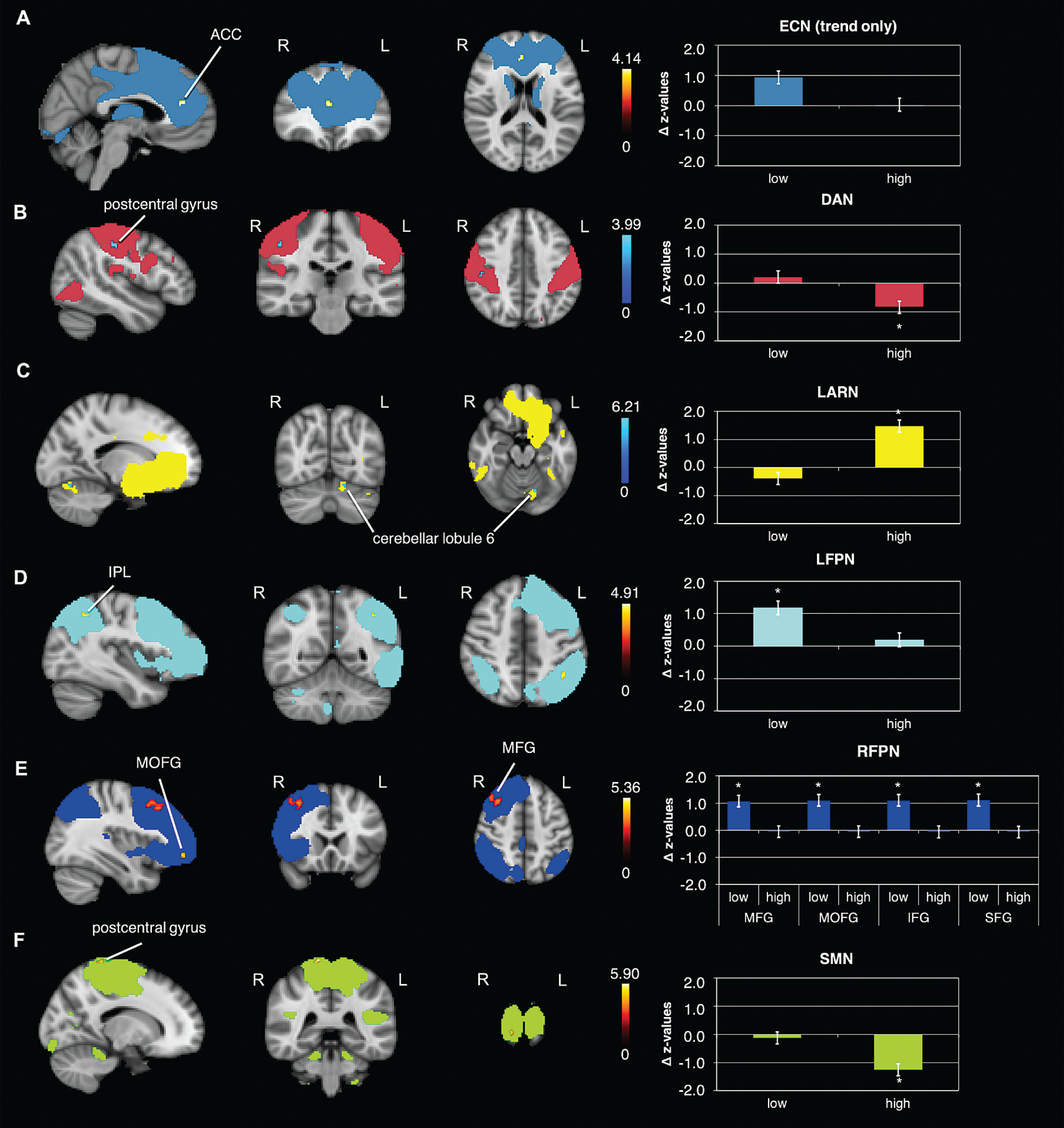
Table 3
Brain regions observed significantly different between pre to post within each exercise condition
Brain region | side | k | x | y | z | p-value (tfce) |
ECN –‘low’ post>pre | ||||||
anterior cingulate cortex | R | 19 | 4 | 40 | 18 | 0.078** |
DAN –‘high’ pre>post | ||||||
postcentral gyrus | R | 13 | 48 | –22 | 44 | 0.045 |
LARN –‘high’ post>pre | ||||||
cerebellar lobule 6 | L | 7* | –14 | –70 | –18 | 0.015 |
LFPN –‘low’ post>pre | ||||||
inferior parietal lobule | L | 15 | –40 | –50 | 44 | 0.033 |
RFPN –‘low’ post>pre | ||||||
middle frontal gyrus | R | 568 | 44 | 36 | 34 | 0.004 |
middle orbitofrontal gyrus | R | 23 | 36 | 50 | –8 | 0.034 |
inferior frontal gyrus (p. opercularis) | R | 10 | 46 | 12 | 38 | 0.046 |
superior frontal gyrus | L | 9* | –2 | 30 | 52 | 0.041 |
SMN –‘high’ pre > post | ||||||
postcentral gyrus | R | 15 | 14 | –38 | 78 | 0.027 |
Coordinates presented are in MNI space; k = cluster size; R = right; L = left; *small cluster size, needs to be treated with caution; **represents a trend.
Sensorimotor network
The rs-FC analysis in the SMN revealed a significant time×condition interaction in the postcentral gyrus and, the supplementary motor area (SMA) and the precuneus (Fig. 2C, Table 2). Comparing pre versus post ‘high’ exercise condition showed a significant decrease in rs-FC in the postcentral gyrus (Fig. 3F, Table 3).
Dorsal attention network
The DAN revealed no significant time×condition interaction in rs-FC, although we detected a strong trend (p = 0.053). However, there was a significant decrease in FC from pre to post ‘high’ exercise condition in postcentral gyrus (Fig. 3B, Table 3).
Left affect and reward network
The LARN did not reveal a significant time×condition interaction in rs-FC. However, there was a significant increase in FC from pre to post ‘high’ exercise condition in the left cerebellar lobule 6 (Fig. 3C, Table 3).
Left fronto parietal network
The LFPN did not reveal a significant time×condition interaction, but a significant increase in rs-FC from pre to post ‘low’ exercise condition in the left inferior parietal lobule (Fig. 3D, Table 3).
Other networks
In the other examined RSNs we could not detect any further significant changes in FC. However, we could detect statistical trends in some of the RSNs. The ECN showed a trend in the interaction time×condition (p = 0.053) and an increase in rs-FC from pre to post ‘low’ exercise condition in the anterior cingulate cortex (ACC) (Fig. 3A). In the Salience network we could also detect a strong trend in form of an increase from pre to post ‘low’ exercise condition (p = 0.06).
Sub-group analysis including HR as covariate of no interest
Including the HR as covariate of no interest in our analysis led to almost the same results, which we found for the whole group analysis without HR as covariate. The RARN showed a significant interaction effect in the analysis without HR, but only a very strong trend in the analysis with HR (p = 0.051) (see supplementary material: Figure S1, Table S1). The interaction effects in the RFPN and the SMN remained stable (see supplementary material: Figure S1, Table S1).
In the direct pre versus post comparisons the results for the ‘high’ exercise condition stayed the same, whereas the results of the pre versus post ‘low’ exercise condition changed slightly (see supplementary material: Figure S2, Table S2). Where we initially found a trend only in the ECN, results got significant in the ECN after inserting the HR as covariate. The significant change in the RFPN and the LFPN stayed the same when including HR. Detailed results are summarized in the supplement.
Additional analysis including nuisance regression
Including nuisance regression (WM and CSF) in our analysis pipeline led to similar results as without nuisance regression. Our main findings could be replicated: the RARN showed a significant interaction effect in the analysis without HR (see supplementary material: Figure S3, Table S3), but no significances with HR. The interaction effects in the RFPN and the SMN remained stable with and without HR as well as with and without nuisance regression (see supplementary material: Figure S3 + S4, Table S3 + S4).
In the direct pre versus post comparisons we could not detect significant changes in the DAN and ECN anymore, however we could detect significant chances in the DMN, which we haven’t in the analysis without nuisance regression (see supplementary material: Figure S5 + S6, Table S5 + S6). Detailed results are summarized in the supplement.
DISCUSSION
This is the first study to investigate the differential effects of aerobic exercise bouts of ‘low’ (35% below lactate threshold) and ‘high’ (20% above lactate threshold) intensity on RSN activations in young and healthy recreational athletes. Behavioral data revealed a significant increase in positive affect after both exercise intensities. Moreover, while providing further evidence for persisting rs-FC increases in cognitive, sensorimotor, and affective networks after acute exercise bouts [5, 6], the results of this study suggest additional exercise intensity-dependent modulatory effects on distinct resting state brain networks. Interaction effects depending on the factors pre / post and ‘high’ / ‘low’ were found in the RARN, the SMN and the RFPN. Whereas ‘low’-intensity exercise bouts resulted in increased rs-FC in brain networks implicated in cognitive and attentional processing (LFPN and RFPN), ‘high’-intensity exercise increased rs-FC in affective brain networks (LARN) and decreased FC in the sensorimotor (SMN) network. In general terms, the observed locoregional effects on rs-FC suggest transient persistent network alterations after acute exercise bouts that are mediated by different exercise intensities in a distinct manner and are discussed in the following with reference to cognitive/attentional (FPN), affective/reward (RARN), and sensorimotor (SMN) function.
Modulation of the FPN after ‘low’-intensity exercise
The FPN plays a fundamental role in attentional monitoring [37], cognitive processing [38], and higher order motor control [39–41]. Our data revealed an increase in rs-FC after ‘low’-intensity exercise in the left and right FPN, which is a large-scale network representing a major functional hub in terms of distributed connectivity [42–44]. Meta-analyses also support the notion that rs-FC strength across the wide-range FPN network is crucial for cognitive and emotional self-regulation [45, 46]. Top-down attentional control processes are regulated by the superior frontal gyrus [47, 48] and the inferior parietal lobule [49]; for instance, Yantis et al. suggested that the inferior parietal lobule is a source of brief attention-related control signals that indicate top-down shifts in attentive states [50].
Unfortunately, we have no neuropsychological test data on attention or executive control tasks (e.g. Stroop, Flanker, Simon tasks, etc.) available. It is thus not possible to directly infer on the impact of exercise on FPN-associated behavioral effects. Nevertheless, it is highly interesting that the connectivity strength within the FPN was increased by physical exercise of ‘low’ intensity: The superior frontal gyrus and the inferior parietal lobule showed a significant increase in rs-FC after ‘low’-intensity exercise, while a significant decrease in rs-FC in the superior frontal gyrus was found after ‘high’-intensity exercise. Our findings on elevated FC in networks mediating attentional control processes specifically after ‘low’-intensity exercise bouts are well in line with a number of studies in the literature that have reported improved attention and cognitive performance after low to moderate exercise bouts [51–53]. According to several studies and meta-analysis the relationship between exercise intensity and cognitive performance is U-shaped [54–57], i.e. suggesting that the beneficial effects occur preferentially with light to moderate exercise intensities, while they then deteriorate with high-intensity anaerobic exercise [58]. Also, effects of different exercise intensities on cerebral oxygenation tested with near infrared spectroscopy (NIRS) point in this direction, i.e. indicating a quadratic relation of prefrontal cortex oxygenation with incremental exercise: first rising between moderate and hard intensities, and then falling at very hard intensities [59].
In conclusion, our data seem to agree with the general notion, that beneficial effects of exercise are preferentially found in low- to intermediate exercise ranges (according to physiological exercise recommendations). Whether the quadratic relation between exercise intensity and functional outcomes described above can conclusively explain why enhanced FPN rs-FC changes in our study were found exclusively for the ‘low’-intensity condition remains uncertain: First of all, one needs to consider that several studies have reported improvements in cognitive states also in the context of high intensity anaerobic exercise bouts [54, 60, 61]. Moreover, our rs-fMRI measurements were undertaken on average 18 minutes after the acute exercise bout, i.e. when any deteriorating impact of ‘high’-intensity exercise on cognitive states should have already leveled off (see introduction). Thus, future studies will need to address the relationship between rs-FC in dedicated cognitive / attentional networks and exercise intensity and / or delay time, before conclusive interpretations can be drawn.
Modulation of the ARN after ‘high’-intensity exercise
The significant interaction effect in the ARN was driven by an almost significant increase of rs-FC after the ‘high’-intensity exercise condition and a decrease after the ‘low’ exercise condition in the right anterior insula. Weng et al. reported a significant rs-FC increase in the right anterior insula after moderate aerobic exercise, as compared to a passive control condition, and a previous study investigating the connectivity of the insula at rest showed it to be functionally integrated in two distinct neural networks [5]: 1) The ventral most anterior insula is part of the anterior network, which is connected to the rostral anterior cingulate cortex, the middle and inferior frontal cortex, and the temporo parietal cortex [62]. This anterior network is involved in emotional salience detection and attentional control [63–66] and is mostly related to the integration of multiple cognitive, homeostatic and emotional (i.e. interoceptive) functions. 2) The dorsal posterior insula is part of the posterior network and is connected to the dorsal posterior cingulate cortex, along with sensorimotor, premotor, supplementary motor, temporal, and occipital areas [62]. This network promotes skeleton-motor body orientation, environmental monitoring, and response selection [67–71]. Reiman et al. had posited that the anterior insula may function as an alarm center for internally-sensed dangers or homeostatic changes [72]. Indeed, this hypothesis is also supported by meta-analytical data, reporting that the anterior insula is more involved in cognitively demanding emotional tasks, and suggesting preferential recruitment for evaluative, experimental, or expressive aspects of internally generated emotions [73]. Gasquoine reported that the insula is the primary reception region of the brain for interoceptive information, in particular for deciphering changes in autonomic nervous system activity levels [74], and Gu et al. showed that the anterior insula responds to the interaction of cognitive demand and stimulus valence [75]. Based on the role of the anterior insula in encoding interoceptive signals from the body’s internal milieu that reflect autonomic activity, Damasio argued that the insula is the site within the brain where subjective feelings of emotion are generated [76].
Our findings might reflect elevated processing of interoceptive signals and internally generated emotions, and suggest a possible neural mechanism underlying the subjective affective experiences after strenuous exercise [77, 78]. Why these findings in the RARN are reduced when including HR as covariate of no interest remains unclear. In fact, it could either be due to the HR difference from pre to post exercise, or it could be related to the reduced power after the exclusion of 4 subjects from this analysis due to artifacts.
The assessments of mood changes using the PANAS revealed a significant increase after both exercise interventions (‘low’ and ‘high’) in the positive affect scale. These findings are in line with previous literature reporting PANAS ratings after a low and a high exercise intervention in students [79]. Pre-exercise, the values of the negative affect variable were already near the bottom of the range, thus representing a floor effect and leaving little / no room for further decreases in negative affect. This might explain why we could not detect any significant changes in negative affect. The results of these behavioral data are not completely in line with the imaging findings in the ARN, as we found a significant increase in positive affect after both exercise intensities in the mood questionnaire, but differential effects induced by exercise intensity in the ARN. One possible reason might be the timing of the acquisition of the data. While the PANAS positive scale was acquired directly post exercise, the rs-fMRI was acquired approximately 18 minutes after exercise.
Modulation of the SMN after ‘high’-intensity exercise
Interestingly, ‘high’-intensity exercise was associated with decreased intrinsic FC in the SMN. The SMN includes the sensorimotor cortex and extends to the SMA. Studies have indicated that the SMN is activated during motor tasks like finger tapping [80]. Previous work investigating the effect of moderate exercise compared to rest could detect increased co-activation in the pre and / or postcentral gyri [6]. Together with our results, different exercise intensities seem to alter SMN brain activity in a differential manner, namely increasing rs-FC after low and moderate exercise, but decreasing rs-FC after high intensity exercise. This may be related to persistent fatigue after strong exercise intensities, i.e. either long & enduring or short & of high intensity as in the current study. Indeed, acute exhaustive exercise has been shown to cause reduced excitability of the SMA [81], which could provide an explanation for the decrease in functional connectivity within the SMN after ‘high’-intensity exercise. In the same line, reduced connectivity between the SMA and the sensorimotor cortex was found in patients with chronic fatigue syndrome during motor preparation [82]. However, rs-FC analyses in multiple sclerosis patients with fatigue also reported opposite effects [83]. Therefore, it is interesting to consider experimental data on ‘fatigue caused by sustaining submaximal-intensity muscle contraction(s)’ during scanning: a significant increase in FC was reported in M1, S1, and SMA [84]. The authors interpreted their finding of strengthened FC with increasing muscle fatigue as a compensatory phenomenon ‘by enhancing the descending command for greater muscle recruitment to maintain the same force’ [84]. As our data were acquired 18 minutes after the strenuous exercise, such compensatory effects are likely to be no longer in place, leaving the sensorimotor output system in a persisting post-exhaustive state.
Possible underlying mechanisms
A variety of possible underlying mechanisms, coming from both human and animal studies, are to be considered to explain our findings, notably neurochemical changes (lactate, cortisol, neurotrophins, neurotransmitters and neuromodulators) that possibly relate to the persistent changes in brain function after acute exercise bouts [85]. Best described are the mechanisms underlying mood changes. Potentially, these affective responses are mediated by levels of endogenous opioids, which are found to be elevated after acute bouts of exercise in both humans and animals in the peripheral blood; this effect seems to be intensity-dependent and has been linked to mood improvements [86–90]. Independent PET ligand activation studies from different groups were able to report local exercise-induced central opioid release and related opioid tracer binding changes in the anterior insula [91–93] after strenuous exercise, which fits well with brain areas found in the ARN in our study.
We also found FC changes in the FPN, which is involved in cognitive processes. Acute exercise stimulates the hypothalamic-pituitary-adrenal (HPA) axis in an intensity-dependent fashion, resulting in increased cortisol levels [94–96]. Some studies have shown that moderate increases in cortisol enhance working memory [97, 98], whereas the elevation of cortisol over a certain level serves to impair memory [99, 100]. Prefrontal cortex is characterized by a high cortisol receptor density [101].
Another possible mechanism would be the level of the brain derived neurotrophic factor (BDNF), which is one of the main regulators of neurogenesis. Studies investigating the effect of BDNF after acute exercise bouts on cognitive function reported mixed effects. Some reported an impairment, some an enhancement and other reported no effect on cognition [102–104]. Protocols that were too exhaustive rather led to an impairment in cognitive function [105]. Although, there are more neurotransmitters that are involved in cognitive processes (e.g. norepinephrine and acetylcholine) [106, 107], little has been done to investigate the influence of these transmitters after acute exercise on cognitive function.
Limitations
There are some limitations that must be considered in the current study. The study population consisted only of males in order to exclude potential hormone cycle-linked humoral factors. To generalize findings, future studies need to investigate gender-balanced cohorts. Additionally, no randomized control condition was implemented, so it cannot be excluded with certainty that effects shown in the pre-post comparisons are also driven by factors other than exercise. We consider this to be unlikely, as the modulations of rs-FC maps induced by exercise were generally in line with previous initial findings in the literature (as mentioned above), with the additional description of exercise intensity effects. Future studies will also need to combine rs-FC measurements, exercise conditions of different intensities, and dedicated neuropsychological tests to shed further light on the prolonged neurobiological mechanisms at the brain network level mediated by acute exercise bouts. Finally, direct comparisons with other studies are hampered by the fact, that standardization is lacking throughout the exercise and imaging literature with regards to age, fitness level, exercise intensity, exercise duration, and the time point of brain imaging after the exercise conditions.
CONCLUSIONS
This is the first study demonstrating differential effects of two different individually titrated exercise intensities on resting state networks. The ‘low’ and ‘high’ exercise intensities affect prolonged network alterations in attentional / cognitive and affective / reward networks in a differential manner. Future research in this area will help to provide neurobiological evidence which exercise intensity is best suited for either cognitive / attentional or affective modulation and may pave the way for supportive clinical applications in patients or for enhancing brain plasticity.
The authors have no conflict of interest to report.
This research did not receive any specific grant from funding agencies in the public, commercial, or not-for-profit sectors.
ETHICS STATEMENT
The study was approved by the local Ethics Committee of the University Hospital Bonn (Lfd. 340/13), according to national legislation and the Declaration of Helsinki.
CONFLICT OF INTEREST
None of the participating scientists have any competing financial interests.
SUPPLEMENTARY MATERIAL
[1] Analogue to the described results in the main manuscript, the supplement includes the results of the rs-FC analysis including HR as covariate of no interest. Additionally, we present the results from an analysis using nuisance regression (WM and CSF). The supplement describes the interaction effects and the pre / post comparison within each exercise condition for the RSN that revealed significant changes in FC.
The supplementary material is available in the electronic version of this article: https://dx.doi.org/10.3233/BPL-190081.
REFERENCES
[1] | Chirles TJ , Reiter K , Weiss LR , Alfini AJ , Nielson KA , Smith JC . Exercise training and functional connectivity changes in mild cognitive impairment and healthy elders. J Alzheimers Dis. (2017) ;57: (3):845–56. |
[2] | Flodin P , Jonasson LS , Riklund K , Nyberg L , Boraxbekk CJ . Does aerobic exercise influence intrinsic Bbrain activity? An aerobic exercise intervention among healthy old adults. Front Aging Neurosci. (2017) ;9: :267. |
[3] | McGregor KM , Crosson B , Krishnamurthy LC , Krishnamurthy V , Hortman K , Gopinath K , et al. Effects of a 12-week aerobic spin intervention on resting state networks in previously sedentary older adults. Front Psychol. (2018) ;9: :2376. |
[4] | Tozzi L , Carballedo A , Lavelle G , Doolin K , Doyle M , Amico F , et al. Longitudinal functional connectivity changes correlate with mood improvement after regular exercise in a dose-dependent fashion. Eur J Neurosci. (2016) ;43: (8):1089–96. |
[5] | Weng TB , Pierce GL , Darling WG , Falk D , Magnotta VA , Voss MW . The acute effects of aerobic exercise on the functional connectivity of human brain networks. Brain Plast. (2017) ;2: (2):171–90. |
[6] | Rajab AS , Crane DE , Middleton LE , Robertson AD , Hampson M , MacIntosh BJ . A single session of exercise increases connectivity in sensorimotor-related brain networks: A resting-state fMRI study in young healthy adults. Front Hum Neurosci. (2014) ;8: :625. |
[7] | Chang YK , Labban JD , Gapin JI , Etnier JL . The effects of acute exercise on cognitive performance: A meta-analysis. Brain Res. (2012) ;1453: :87–101. |
[8] | Chang YK , Chu IH , Chen FT , Wang CC . Dose-response effect of acute resistance exercise on Tower of London in middle-aged adults. J Sport Exerc Psychol. (2011) ;33: (6):866–83. |
[9] | Chang YK , Etnier JL . Exploring the dose-response relationship between resistance exercise intensity and cognitive function. J Sport Exerc Psychol. (2009) ;31: (5):640–56. |
[10] | Robertson AD , Crane DE , Rajab AS , Swardfager W , Marzolini S , Shirzadi Z , et al. Exercise intensity modulates the change in cerebral blood flow following aerobic exercise in chronic stroke. Exp Brain Res. (2015) ;233: (8):2467–75. |
[11] | Williamson JW , McColl R , Mathews D . Changes in regional cerebral blood flow distribution during postexercise hypotension in humans. J Appl Physiol (1985). (2004) ;96: (2):719–24. |
[12] | SchmittA, MartinJA, RojasS, VafaR, ScheefL, StruderHK, BoeckerH. Effects of low- and high-intensity exercise on emotional face processing: an fMRI face-matching study. Soc Cogn Affect Neurosci. (2019) ;14: (6):657–5. doi:10.1093/scan/nsz042 |
[13] | Sheehan DV , Lecrubier Y , Sheehan KH , Amorim P , Janavs J , Weiller E , et al. The Mini-International Neuropsychiatric Interview (M.I.N.I.): The development and validation of a structured diagnostic psychiatric interview for DSM-IV and ICD-10. J Clin Psychiatry. (1998) ;59: (Suppl 20):22–33;quiz 4-57. |
[14] | Spielberger CD , Gorsuch RL , Lushene R , Vagg PR , Jacobs GA . Manual for the State-Trait Anxiety Inventory: Palo Alto, CA: Consulting Psychologists Press, (1983) . |
[15] | Hautzinger M , Bailer M , Worall H , Keller F . Beck Depressions-Inventar: (BDI); Testhandbuch. [Beck Depression Inventory (BDI): Test manual]: Bern; 1994. |
[16] | Oldfield RC . The assessment and analysis of handedness: The Edinburgh inventory. Neuropsychologia. (1971) ;9: (1):97–113. |
[17] | Schmidt KH , Metzler P . Wortschatztest: WST:Weinheim: Beltz Test GmbH; 1992. |
[18] | Borg GA . Psychophysical bases of perceived exertion. Med Sci Sports Exerc. (1982) ;14: (5):377–81. |
[19] | Hollmann W , Knigge H , Knicker A , Strüder HK . Methods for Measurement of Physical Fitness and Training Recommendations in Studies on Humans. Functional Neuroimaging in Exercise and Sport Sciences. New York, NY: Springer New York; (2012) , pp. 79–107. |
[20] | Bishop D , Jenkins DG , Mackinnon LT . The relationship between plasma lactate parameters, Wpeak and 1-h cycling performance in women. Med Sci Sports Exerc. (1998) ;30: (8):1270–5. |
[21] | Williams EJ . Experimental designs balanced for the estimation of residual effects of treatments. Aust J Sci Res Ser A. (1949) ;2: (2):149–68. |
[22] | Krohne HW , Egloff B , Kohlmann CW , Tausch A . Untersuchungen mit einer deutschen Version der "Positive and Negative Affect Schedule“ (PANAS). Diagnostica. (1996) ;42: :139–56. |
[23] | Ashburner J , Ridgway GR . Symmetric diffeomorphic modeling of longitudinal structural MRI. Front Neurosci. (2012) ;6: :197. |
[24] | Smith SM . Fast robust automated brain extraction. Hum Brain Mapp. (2002) ;17: (3):143–55. |
[25] | Wu CW , Chen CL , Liu PY , Chao YP , Biswal BB , Lin CP . Empirical evaluations of slice-timing, smoothing, and normalization effects in seed-based, resting-state functional magnetic resonance imaging analyses. Brain Connect. (2011) ;1: (5):401–10. |
[26] | Pruim RHR , Mennes M , van Rooij D , Llera A , Buitelaar JK , Beckmann CF . ICA-AROMA: A robust ICA-based strategy for removing motion artifacts from fMRI data. Neuroimage. (2015) ;112: :267–77. |
[27] | Bright MG , Tench CR , Murphy K . Potential pitfalls when denoising resting state fMRI data using nuisance regression. Neuroimage. (2017) ;154: :159–68. |
[28] | Smith SM , Fox PT , Miller KL , Glahn DC , Fox PM , Mackay CE , et al. Correspondence of the brain’s functional architecture during activation and rest. Proc Natl Acad Sci U S A. (2009) ;106: (31):13040–5. |
[29] | Beckmann CF , Mackay CE , Filippini N , Smith SM . Group comparison of resting-state FMRI data using multi-subject ICA and dual regression. NeuroImage. (2009) ;47: :S148. |
[30] | Filippini N , MacIntosh BJ , Hough MG , Goodwin GM , Frisoni GB , Smith SM , et al. Distinct patterns of brain activity in young carriers of the APOE-epsilon4 allele. Proc Natl Acad Sci U S A. (2009) ;106: (17):7209–14. |
[31] | Berkovits I , Hancock GR , Nevitt J . Bootstrap resampling approaches for repeated measure designs: Relative robustness to sphericity and normality violations. Educ Psychol Meas. (2000) ;60: (6):877–92. |
[32] | Smith SM , Nichols TE . Threshold-free cluster enhancement: Addressing problems of smoothing, threshold dependence and localisation in cluster inference. Neuroimage. (2009) ;44: (1):83–98. |
[33] | Maldjian JA , Laurienti PJ , Kraft RA , Burdette JH . An automated method for neuroanatomic and cytoarchitectonic atlas-based interrogation of fMRI data sets. Neuroimage. (2003) ;19: (3):1233–9. |
[34] | Tzourio-Mazoyer N , Landeau B , Papathanassiou D , Crivello F , Etard O , Delcroix N , et al. Automated anatomical labeling of activations in SPM using a macroscopic anatomical parcellation of the MNI MRI single-subject brain. Neuroimage. (2002) ;15: (1):273–89. |
[35] | Desikan RS , Segonne F , Fischl B , Quinn BT , Dickerson BC , Blacker D , et al. An automated labeling system for subdividing the human cerebral cortex on MRI scans into gyral based regions of interest. Neuroimage. (2006) ;31: (3):968–80. |
[36] | American College of Sports Medicine, Riebe D , Ehrman JK , Liguori G , Magal M . ACSM’s guidelines for exercise testing and prescription. Tenth edition. ed. Philadelphia: Wolters Kluwer; (2018) . xxx, p. 472. |
[37] | Ptak R . The frontoparietal attention network of the human brain: Action, saliency, and a priority map of the environment. Neuroscientist. (2012) ;18: (5):502–15. |
[38] | Marek S , Dosenbach NUF . The frontoparietal network: Function, electrophysiology, and importance of individual precision mapping. Dialogues Clin Neurosci. (2018) ;20: (2):133–40. |
[39] | Wise SP , Boussaoud D , Johnson PB , Caminiti R . Premotor and parietal cortex: Corticocortical connectivity and combinatorial computations. Annu Rev Neurosci. (1997) ;20: :25–42. |
[40] | Wu T , Wang L , Chen Y , Zhao C , Li K , Chan P . Changes of functional connectivity of the motor network in the resting state in Parkinson’s disease. Neurosci Lett. (2009) ;460: (1):6–10. |
[41] | Wymbs NF , Bassett DS , Mucha PJ , Porter MA , Grafton ST . Differential recruitment of the sensorimotor putamen and frontoparietal cortex during motor chunking in humans. Neuron. (2012) ;74: (5):936–46. |
[42] | Marek S , Hwang K , Foran W , Hallquist MN , Luna B . The contribution of network organization and integration to the development of cognitive control. PLoS Biol. (2015) ;13: (12):e1002328. |
[43] | Power JD , Cohen AL , Nelson SM , Wig GS , Barnes KA , Church JA , et al. Functional network organization of the human brain. Neuron. (2011) ;72: (4):665–78. |
[44] | Power JD , Schlaggar BL , Lessov-Schlaggar CN , Petersen SE . Evidence for hubs in human functional brain networks. Neuron. (2013) ;79: (4):798–813. |
[45] | Chen T , Becker B , Camilleri J , Wang L , Yu S , Eickhoff SB , et al. A domain-general brain network underlying emotional and cognitive interference processing: Evidence from coordinate-based and functional connectivity meta-analyses. Brain Struct Funct. (2018) ;223: (8):3813–40. |
[46] | Langner R , Leiberg S , Hoffstaedter F , Eickhoff SB . Towards a human self-regulation system: Common and distinct neural signatures of emotional and behavioural control. Neurosci Biobehav Rev. (2018) ;90: :400–10. |
[47] | Casey BJ , Thomas KM , Welsh TF , Badgaiyan RD , Eccard CH , Jennings JR , et al. Dissociation of response conflict, attentional selection, and expectancy with functional magnetic resonance imaging. Proc Natl Acad Sci U S A. (2000) ;97: (15):8728–33. |
[48] | Colcombe SJ , Kramer AF , Erickson KI , Scalf P , McAuley E , Cohen NJ , et al. Cardiovascular fitness, cortical plasticity, and aging. Proc Natl Acad Sci U S A. (2004) ;101: (9):3316–21. |
[49] | Hopfinger JB , Buonocore MH , Mangun GR . The neural mechanisms of top-down attentional control. Nat Neurosci. (2000) ;3: (3):284–91. |
[50] | Yantis S , Schwarzbach J , Serences JT , Carlson RL , Steinmetz MA , Pekar JJ , et al. Transient neural activity in human parietal cortex during spatial attention shifts. Nat Neurosci. (2002) ;5: (10):995–1002. |
[51] | Coleman M , Offen K , Markant J . Exercise similarly facilitates men and women’s selective attention task response times but differentially affects memory task performance. Front Psychol. (2018) ;9: :1405. |
[52] | Chang YK , Alderman BL , Chu CH , Wang CC , Song TF , Chen FT . Acute exercise has a general facilitative effect on cognitive function: A combined ERP temporal dynamics and BDNF study. Psychophysiology. (2017) ;54: (2):289–300. |
[53] | Loprinzi PD , Kane CJ . Exercise and cognitive function: A randomized controlled trial examining acute exercise and free-living physical activity and sedentary effects. Mayo Clin Proc. (2015) ;90: (4):450–60. |
[54] | Alves CR , Tessaro VH , Teixeira LA , Murakava K , Roschel H , Gualano B , et al. Influence of acute high-intensity aerobic interval exercise bout on selective attention and short-term memory tasks. Percept Mot Skills. (2014) ;118: (1):63–72. |
[55] | Chmura J , Nazar K , Kaciuba-Uscilko H . Choice reaction time during graded exercise in relation to blood lactate and plasma catecholamine thresholds. Int J Sports Med. (1994) ;15: (4):172–6. |
[56] | McMorris T , Graydon J . The effect of exercise on the decision-making performance of experienced and inexperienced soccer players. Res Q Exerc Sport. (1996) ;67: (1):109–14. |
[57] | McMorris T , Sproule J , Turner A , Hale BJ . Acute, intermediate intensity exercise, and speed and accuracy in working memory tasks: A meta-analytical comparison of effects. Physiol Behav. (2011) ;102: (3-4):421–8. |
[58] | Brisswalter J , Collardeau M , Rene A . Effects of acute physical exercise characteristics on cognitive performance. Sports Med. (2002) ;32: (9):555–66. |
[59] | Rooks CR , Thom NJ , McCully KK , Dishman RK . Effects of incremental exercise on cerebral oxygenation measured by near-infrared spectroscopy: A systematic review. Prog Neurobiol. (2010) ;92: (2):134–50. |
[60] | Du Rietz E , Barker AR , Michelini G , Rommel AS , Vainieri I , Asherson P , et al. Beneficial effects of acute high-intensity exercise on electrophysiological indices of attention processes in young adult men. Behav Brain Res. (2019) ;359: :474–84. |
[61] | Gmiat A , Micielska K , Kozlowska M , Flis DJ , Smaruj M , Kujach S , et al. The impact of a single bout of high intensity circuit training on myokines’ concentrations and cognitive functions in women of different age. Physiol Behav. (2017) ;179: :290–7. |
[62] | Cauda F , D’Agata F , Sacco K , Duca S , Geminiani G , Vercelli A . Functional connectivity of the insula in the resting brain. Neuroimage. (2011) ;55: (1):8–23. |
[63] | Corbetta M , Shulman GL . Control of goal-directed and stimulus-driven attention in the brain. Nat Rev Neurosci. (2002) ;3: (3):201–15. |
[64] | Dosenbach NU , Visscher KM , Palmer ED , Miezin FM , Wenger KK , Kang HC , et al. A core system for the implementation of task sets. Neuron. (2006) ;50: (5):799–812. |
[65] | Fox MD , Corbetta M , Snyder AZ , Vincent JL , Raichle ME . Spontaneous neuronal activity distinguishes human dorsal and ventral attention systems. Proc Natl Acad Sci U S A. (2006) ;103: (26):10046–51. |
[66] | Seeley WW , Menon V , Schatzberg AF , Keller J , Glover GH , Kenna H , et al. Dissociable intrinsic connectivity networks for salience processing and executive control. J Neurosci. (2007) ;27: (9):2349–56. |
[67] | Craig AD . How do you feel? Interoception: The sense of the physiological condition of the body. Nat Rev Neurosci. (2002) ;3: (8):655–66. |
[68] | Flynn FG . Anatomy of the insula functional and clinical correlates. Aphasiology. (1999) ;13: (1):55–78. |
[69] | Kurth F , Eickhoff SB , Schleicher A , Hoemke L , Zilles K , Amunts K . Cytoarchitecture and probabilistic maps of the human posterior insular cortex. Cereb Cortex. (2010) ;20: (6):1448–61. |
[70] | Kurth F , Zilles K , Fox PT , Laird AR , Eickhoff SB . A link between the systems: Functional differentiation and integration within the human insula revealed by meta-analysis. Brain Struct Funct. (2010) ;214: (5-6):519–34. |
[71] | Taylor KS , Seminowicz DA , Davis KD . Two systems of resting state connectivity between the insula and cingulate cortex. Hum Brain Mapp. (2009) ;30: (9):2731–45. |
[72] | Reiman EM , Lane RD , Ahern GL , Schwartz GE , Davidson RJ , Friston KJ , et al. Neuroanatomical correlates of externally and internally generated human emotion. Am J Psychiatry. (1997) ;154: (7):918–25. |
[73] | Phan KL , Wager T , Taylor SF , Liberzon I . Functional neuroanatomy of emotion: A meta-analysis of emotion activation studies in PET and fMRI. Neuroimage. (2002) ;16: (2):331–48. |
[74] | Gasquoine PG . Contributions of the insula to cognition and emotion. Neuropsychol Rev. (2014) ;24: (2):77–87. |
[75] | Gu X , Liu X , Van Dam NT , Hof PR , Fan J . Cognition-emotion integration in the anterior insular cortex. Cereb Cortex. (2013) ;23: (1):20–7. |
[76] | Damasio A . Feelings of emotion and the self. Ann N Y Acad Sci. (2003) ;1001: :253–61. |
[77] | Niven A , Thow J , Holroyd J , Turner AP , Phillips SM . Comparison of affective responses during and after low volume high-intensity interval exercise, continuous moderate- and continuous high-intensity exercise in active, untrained, healthy males. J Sports Sci. (2018) ;36: (17):1993–2001. |
[78] | Malik AA , Williams CA , Weston KL , Barker AR . Perceptual responses to high- and moderate-intensity interval exercise in adolescents. Med Sci Sports Exerc. (2018) ;50: (5):1021–30. |
[79] | Bixby WR , Spalding TW , Hatfield BA . Temporal dynamics and dimensional specificity of the affective response to exercise of varying intensity: Differing pathways to a common outcome. J Sport Exercise Psy. (2001) ;23: (3):171–90. |
[80] | Biswal B , Yetkin FZ , Haughton VM , Hyde JS . Functional connectivity in the motor cortex of resting human brain using echo-planar MRI. Magn Reson Med. (1995) ;34: (4):537–41. |
[81] | Coco M , Perciavalle V , Cavallari P , Perciavalle V . Effects of an exhaustive exercise on motor skill learning and on the excitability of primary motor cortex and supplementary motor area. Medicine (Baltimore). (2016) ;95: (11):e2978. |
[82] | van der Schaaf ME , Roelofs K , de Lange FP , Geurts DEM , van der Meer JWM , Knoop H , et al. Fatigue is associated with altered monitoring and preparation of physical effort in patients with chronic fatigue syndrome. Biol Psychiatry Cogn Neurosci Neuroimaging. (2018) ;3: (4):392–404. |
[83] | Bisecco A , Nardo FD , Docimo R , Caiazzo G , d’Ambrosio A , Bonavita S , et al. Fatigue in multiple sclerosis: The contribution of resting-state functional connectivity reorganization. Mult Scler. (2018) ;24: (13):1696–705. |
[84] | Jiang Z , Wang XF , Kisiel-Sajewicz K , Yan JH , Yue GH . Strengthened functional connectivity in the brain during muscle fatigue. Neuroimage. (2012) ;60: (1):728–37. |
[85] | Basso JC , Suzuki WA . The effects of acute exercise on mood, cognition, neurophysiology, and neurochemical pathways: A review. Brain Plast. (2017) ;2: (2):127–52. |
[86] | Brooks S , Burrin J , Cheetham ME , Hall GM , Yeo T , Williams C . The responses of the catecholamines and beta-endorphin to brief maximal exercise in man. Eur J Appl Physiol Occup Physiol. (1988) ;57: (2):230–4. |
[87] | Colt EW , Wardlaw SL , Frantz AG . The effect of running on plasma beta-endorphin. Life Sci. (1981) ;28: (14):1637–40. |
[88] | Goldfarb AH , Hatfield BD , Armstrong D , Potts J . Plasma beta-endorphin concentration: Response to intensity and duration of exercise. Med Sci Sports Exerc. (1990) ;22: (2):241–4. |
[89] | McMurray RG , Forsythe WA , Mar MH , Hardy CJ . Exercise intensity-related responses of beta-endorphin and catecholamines. Med Sci Sports Exerc. (1987) ;19: (6):570–4. |
[90] | Schwarz L , Kindermann W . Changes in beta-endorphin levels in response to aerobic and anaerobic exercise. Sports Med. (1992) ;13: (1):25–36. |
[91] | Boecker H , Sprenger T , Spilker ME , Henriksen G , Koppenhoefer M , Wagner KJ , et al. The runner’s high: Opioidergic mechanisms in the human brain. Cereb Cortex. (2008) ;18: (11):2523–31. |
[92] | Hiura M , Sakata M , Ishii K , Toyohara J , Oda K , Nariai T , et al. Central mu-opioidergic system activation evoked by heavy and severe-intensity cycling exercise in humans: A pilot study using positron emission tomography with 11C-carfentanil. Int J Sports Med. (2017) ;38: (1):19–26. |
[93] | Saanijoki T , Tuominen L , Tuulari JJ , Nummenmaa L , Arponen E , Kalliokoski K , et al. Opioid release after high-intensity interval training in healthy human subjects. Neuropsychopharmacology. (2018) ;43: (2):246–54. |
[94] | Hill EE , Zack E , Battaglini C , Viru M , Viru A , Hackney AC . Exercise and circulating cortisol levels: The intensity threshold effect. J Endocrinol Invest. (2008) ;31: (7):587–91. |
[95] | Kraemer RR , Blair S , Kraemer GR , Castracane VD . Effects of treadmill running on plasma beta-endorphin, corticotropin, and cortisol levels in male and female 10K runners. Eur J Appl Physiol Occup Physiol. (1989) ;58: (8):845–51. |
[96] | Luger A , Deuster PA , Kyle SB , Gallucci WT , Montgomery LC , Gold PW , et al. Acute hypothalamic-pituitary-adrenal responses to the stress of treadmill exercise. Physiologic adaptations to physical training. N Engl J Med. (1987) ;316: (21):1309–15. |
[97] | Yuen EY , Liu W , Karatsoreos IN , Feng J , McEwen BS , Yan Z . Acute stress enhances glutamatergic transmission in prefrontal cortex and facilitates working memory. Proc Natl Acad Sci U S A. (2009) ;106: (33):14075–9. |
[98] | Yuen EY , Liu W , Karatsoreos IN , Ren Y , Feng J , McEwen BS , et al. Mechanisms for acute stress-induced enhancement of glutamatergic transmission and working memory. Mol Psychiatry. (2011) ;16: (2):156–70. |
[99] | de Quervain DJ , Roozendaal B , Nitsch RM , McGaugh JL , Hock C . Acute cortisone administration impairs retrieval of long-term declarative memory in humans. Nat Neurosci. (2000) ;3: (4):313–4. |
[100] | Tollenaar MS , Elzinga BM , Spinhoven P , Everaerd WA . The effects of cortisol increase on long-term memory retrieval during and after acute psychosocial stress. Acta Psychol (Amst). (2008) ;127: (3):542–52. |
[101] | Heffelfinger AK , Newcomer JW . Glucocorticoid effects on memory function over the human life span. Dev Psychopathol. (2001) ;13: (3):491–513. |
[102] | Gutin B , Di Gennaro J . Effect of a treadmill run to exhaustion on performance of long addition. Res Q. (1968) ;39: (4):958–64. |
[103] | Pontifex MB , Hillman CH , Fernhall B , Thompson KM , Valentini TA . The effect of acute aerobic and resistance exercise on working memory. Med Sci Sports Exerc. (2009) ;41: (4):927–34. |
[104] | Tomporowski PD . Effects of acute bouts of exercise on cognition. Acta Psychol (Amst). (2003) ;112: (3):297–324. |
[105] | Wrisberg CA , Herbert WG . Fatigue effects on the timing performance of well-practiced subjects. Res Q. (1976) ;47: (4):839–44. |
[106] | Ferreira-Vieira TH , Guimaraes IM , Silva FR , Ribeiro FM . Alzheimer’s disease: Targeting the Cholinergic System. Curr Neuropharmacol. (2016) ;14: (1):101–15. |
[107] | McMorris T . Developing the catecholamines hypothesis for the acute exercise-cognition interaction in humans: Lessons from animal studies. Physiol Behav. (2016) ;165: :291–9. |