Big data and artificial intelligence in post-stroke aphasia: A mapping review
Abstract
BACKGROUND:
Aphasia is an impairment of language as a result of brain damage which can affect individuals after a stroke. Recent research in aphasia has highlighted new technologies and techniques that fall under the umbrella of big data and artificial intelligence (AI).
OBJECTIVES:
This review aims to examine the extent, range and nature of available research on big data and AI relating to aphasia post stroke.
METHODS:
A mapping review is the most appropriate format for reviewing the evidence on a broad and emerging topic such as big data and AI in post-stroke aphasia. Following a systematic search of online databases and a two-stage screening process, data was extracted from the included studies. This analysis process included grouping the research into inductively created categories as the different areas within the research topic became apparent.
RESULTS:
Seventy-two studies were included in the review. The results showed an emergent body of research made up of meta-analyses and quasi-experimental studies falling into defined categories within big data and AI in post-stroke aphasia. The two largest categories were automation, including automated assessment and diagnosis as well as automatic speech recognition, and prediction and association, largely through symptom-lesion mapping and meta-analysis.
CONCLUSIONS:
The framework of categories within the research field of big data and AI in post-stroke aphasia suggest this broad topic has the potential to make an increasing contribution to aphasia research. Further research is needed to evaluate the specific areas within big data and AI in aphasia in terms of efficacy and accuracy within defined categories.
1Introduction
Aphasia is the loss or impairment of language as a result of brain damage. Those affected can suffer from difficulty speaking, understanding, reading and writing. Approximately 5,500 people experienced a stroke in Ireland in 2020 and 40% of stroke patients presented with aphasia (NOCA, 2021). The Collaboration of Aphasia Trialists (CATs) described research into aphasia as facing methodological and infrastructural challenges, namely being language, region and discipline specific, which limits the efficiency, strength and wider utility of the body of evidence (COSTAction-IS1208, 2013-2017). A large number of aphasia treatment studies have involved single-subject research in the form of case reports or single-subject experimental studies, making it difficult to evaluate treatments and synthesise findings (Beeson & Robey, 2006). A Cochrane Systematic Review of aphasia trials found a wide variety of methodologies, and levels of data reporting, with little consistency between papers (Brady et al., 2016). This hampered the synthesis of evidence resulting in a lack of clarity regarding the efficacy of interventions and their optimum intensity, dosage and duration. The development of consensus outcome measures, endorsed by CATs (Wallace et al., 2019), provides researchers with guidance on measures that are recommended to be routinely included in aphasia research studies. The use of consensus outcome measures allows for the aggregation of data from multiple studies that use those recommended research outcome measures in their research designs. This provides opportunities for meta-analyses of high-quality randomised controlled trials and the development of a large aphasia research database (Brady et al., 2022).
More recently, research has emerged utilising a variety of new technologies and techniques, which can broadly be described as big data and artificial intelligence (AI). The term big data refers to the extraction and analysis of information from the large amounts of data available in an increasingly digital and connected world (Gandomi & Haider, 2015). The data can be varied and unstructured, often acquired from a multitude of sources. It is produced at an ever-increasing rate, including in real time, such as in the case of wearable devices (Sagiroglu & Sinanc, 2013). As well as this, big data analysis can take many forms, from the application of comparatively simple statistical methods through to AI and machine learning (Faroqi-Shah, 2016). Big data is a growing part of the healthcare industry, where various sources of data exist including hospital records, medical records of patients, results of medical examinations, biomedical research and devices that are a part of the “internet of things” (Dash et al., 2019). The amount of data captured is also growing with the increasing adoption of technology like remote forms of service delivery. Additionally, corpora such as TalkBank provide datasets specifically created for use by aphasia researchers under the principles of data-sharing (MacWhinney & Fromm, 2016). The variety of both data available and methods of analysis is evident in aphasia studies. This includes research into areas as diverse as aphasia classification models, semantic analysis, speech recognition, improving diagnosis and predicting patient outcomes through data from MRI scans, electroencephalogram tests, mobile therapy applications, aphasic speech corpora and client satisfaction surveys (MacWhinney & Fromm, 2016).
It appears likely that continued advances in this research field will result in real-world clinical applications that could potentially improve assessment, diagnosis and management of aphasia in the future. However, the dissemination of this AI and big data research in post-stroke aphasia spreads across journals and conference proceedings from the differing fields of aphasia, stroke, neurology, computing and mathematics. Additionally, the majority of this research has taken the form of quasi-experimental studies, further compounding the issue of variation between studies. As a result, this body of research is compromised by the same issues of disciplinary separation and disparate methods, data sets and outcomes as originally described by Collaboration of Aphasia Trailists (COSTAction-IS1208, 2013-2017). A synthesis of the evidence is required in order for it to be fully understood and utilised. We have carried out the first step in this synthesis process, which is a mapping review that has collated, categorised and labelled research (Grant & Booth, 2009) under the umbrella of big data and AI in aphasia. Mapping reviews are a relatively new form of review. This method is part of a growing body of evidence synthesis methodologies, intended to map out and categorise existing literature and can then guide subsequent reviews and/or primary research by identifying gaps in the research literature. Analysis within mapping reviews looks to characterise quantity and quality of literature and synthesis can be tabular or graphical (Grant & Booth, 2009). The need for mapping reviews have emerged in response to the increasing volume and variation in academic research published (Altbach & De Wit, 2018). The issues identified by CATs in terms of range of disciplines, methodological challenges and varied outcome measures continue in the research field of big data and AI in aphasia (COSTAction-IS1208, 2013-2017). Mapping reviews aim to systematically search a broad research field to establish available evidence, map key concepts and identify gaps in this knowledge as well as future research needs (Grant & Booth, 2009; Miake-Lye et al., 2016).
The aim of this mapping review is to examine the extent, range and nature of available research on big data and AI relating to aphasia post stroke. In this review, we gathered information on research design, methods of data collection and research findings which form the different branches of our mapping framework. It is intended that this framework will be used to understand the value of big data and AI in post-stroke aphasia, its contribution to aphasia research generally and identify areas for further research.
2Methods
A mapping review methodology was selected to examine this topic due to the anticipated heterogeneity of the available evidence across healthcare, multidisciplinary and computer science fields. This method was developed by the Evidence for Policy and Practice Information and Co-ordinating Centre (EPPI-Centre), Institute of Education, London, to investigate research on a broad subject of interest (Grant & Booth, 2009; James et al., 2016). The aim of this type of review is to categorise, describe and map available evidence on a broad subject of interest, into an inductively developed framework. No standardised guidance document exists equivalent to the PRISMA-P for mapping review protocols. The methodology used followed the process outlined in James et al. (2016) and O’Cathain et al. (2013). Quality assessment of included papers is not required in mapping reviews; instead, studies are characterised based on study design (Grant & Booth, 2009; James et al., 2016).
In order to define the review question the population, concept and healthcare problem question type from Whittemore and Knafl (2005) was employed. In this review, population refers to people with post-stroke aphasia and concept includes big data and AI/machine-learning algorithms. These concepts informed the development of the search terms in the search strategy. The healthcare problem is any clinical practice issue in aphasia. As this was expected to be varied across studies, it was not defined in the search strategy. Instead, this was identified during the screening process.
2.1Search strategy
The search strategy was developed to ensure a broad and comprehensive search of the literature. Preliminary searches were conducted to refine the research question, search terms and list of databases. Published reviews in the fields of post-stroke aphasia research as well as big data and AI in healthcare (Senders et al., 2018) were examined and a specialist librarian was consulted when developing the search strategy. Online databases CINAHL, Scopus, PsycINFO, MEDLINE, Cochrane Collaboration, EMBASE, ACM and IEEE were searched in August 2021 with a follow up search in November 2022. The search included all literature published without year of publication, peer-review, full-text or participant age limiters, and included conference proceedings, abstracts and dissertations. The search terms used are detailed in Table 1.
Table 1
Search strategy
Search terms | |
S1 Population | aphasia OR “naming deficit” OR “word finding difficulty” OR anomia OR “acquired language disorder” OR anomic OR aphasic OR dysphasic |
AND S2 concept | “big data” OR dataset OR “machine learning” OR Machine OR “bayesian learning” OR “random forest” OR “machine learning” OR “artificial intelligence” OR “neural network*” OR “natural language processing” OR “support vector*” OR boosting OR “deep learning” OR “random forest*” OR “naive bayes” OR “machine intelligence” OR “computational intelligence” OR “computer reasoning” |
2.2Eligibility criteria
Studies with participants with aphasia over the age of 18 years were included irrespective of stroke classification, stage of recovery or type of intervention. Studies investigating non-stroke aphasia (e.g. primary progressive aphasia) were excluded. Where there was a mixture of stroke and non-stroke participants, studies were only included if data from stroke participants with aphasia could be separated out. Any studies that included artificial intelligence or machine learning techniques were included as well as studies with large datasets (n=>900) with or without AI analysis techniques. This number was considered sufficient to meet the classification of big data within this population and reflects numbers presented in a recent systematic review and meta-analysis in aphasia (Ali et al., 2021). All studies, including peer-reviewed articles, conference abstracts and grey literature were included. Studies without participant data, for example theoretical papers on algorithms, and articles not published in English, were excluded.
2.3Study selection
Following database searches, all records were imported to EndNote and duplicates were removed. Two reviewers independently conducted title and abstract screening. This process was managed, with reasons for exclusion recorded, using Rayyan review software, Rayyan Systems Inc., Doha, Qatar (available at www.rayyan.ai). There was moderate agreement (Cohen’s Kappa 0.49) for title and abstract review. All disagreements were discussed in order to reach consensus. The final stage of screening included full-text review of articles, if available, before final inclusion decisions were made. Data extraction was managed using Microsoft Office Excel. A Preferred Reporting Items for Systematic reviews and Meta-Analyses (PRISMA) flow diagram was used to guide the review process of identification, screening, eligibility and inclusion (Page et al., 2021), see Fig. 1 for details.
Fig. 1
PRISMA flow diagram.
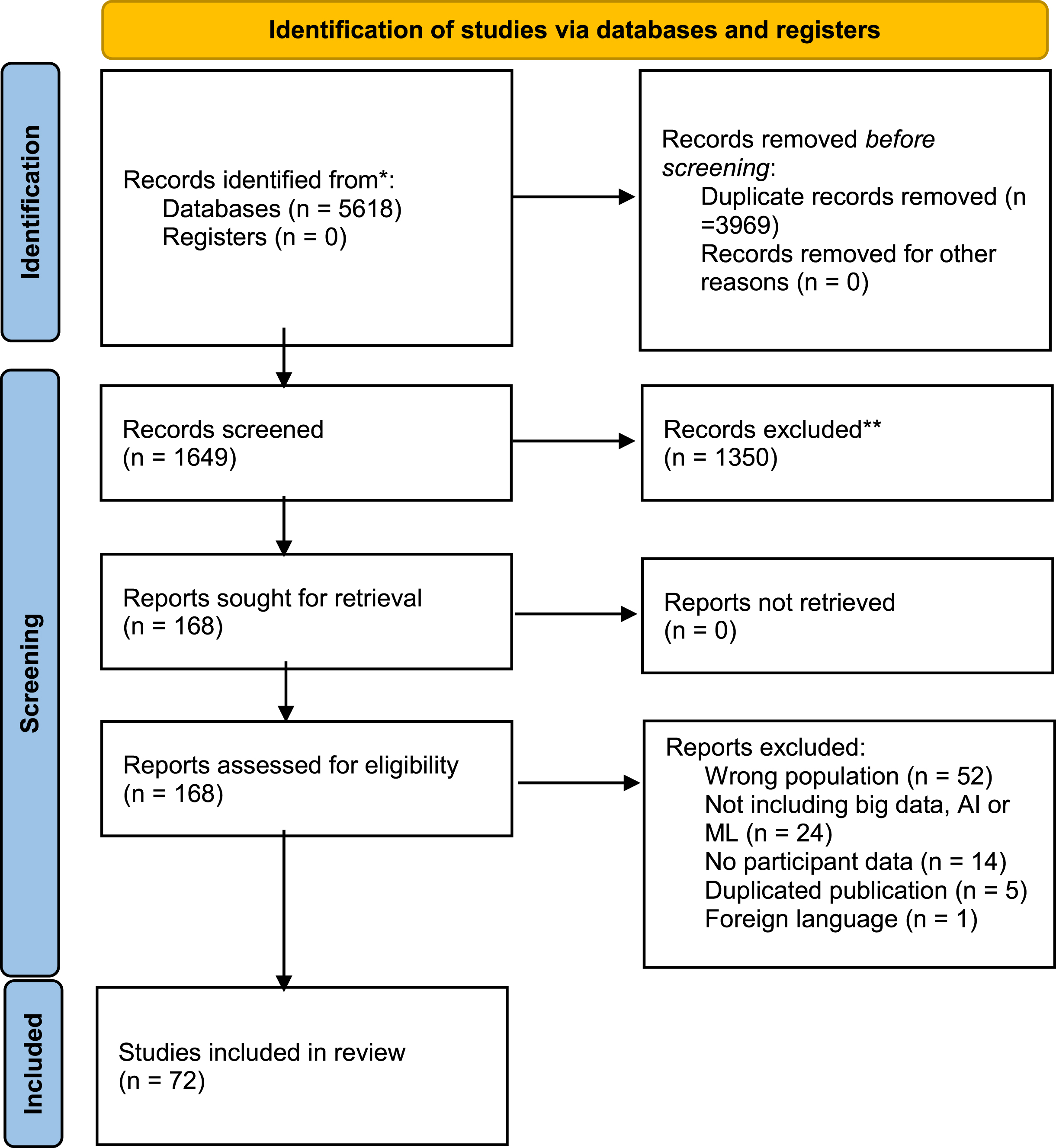
2.4Data extraction
The data extracted from included articles included: title, year of publication, authors, publication name, type of publication, country, study aim, study type, study method of analysis, study data type, participant data, summary of findings and study recommendations.
2.5Data analysis
Following review of the extracted data, inductive coding was carried out creating categories that reflect the areas of research. These categories were developed by the authors, who are speech and language therapists. They were informed by the aims and methods of the included studies. For example, the application of AI techniques in the use of automated systems to assess speech (Le et al., 2018) was categorised as automation. These categories were further divided into subcategories to give a deeper understanding of the nature of research for example, differentiating the category of automation into assessment, automatic speech recognition or diagnosis.
3Results
5,618 records were retrieved after database searches. Following duplicate removal, title and abstract screening of 1649 records was carried out and 168 records underwent full-text review with 72 articles included in the mapping review (Fig. 1). Data from the studies were extracted into predefined categories including type of publication, country, study aim, method of analysis, data type, participant data, findings and recommendations. This is available in supplemental materials.
3.1Characteristics of included studies
Just under half of the studies (32) were published by authors working in the USA (See Fig. 2). This is followed by eight from the UK, six each from Hong Kong and Germany, four from China, three from Greece, two from Korea and India respectively, and one each from Canada, Sweden and the Netherlands. There are six studies with authors’ afflictions and/or data collection from multiple countries.
Fig. 2
Country of publication.
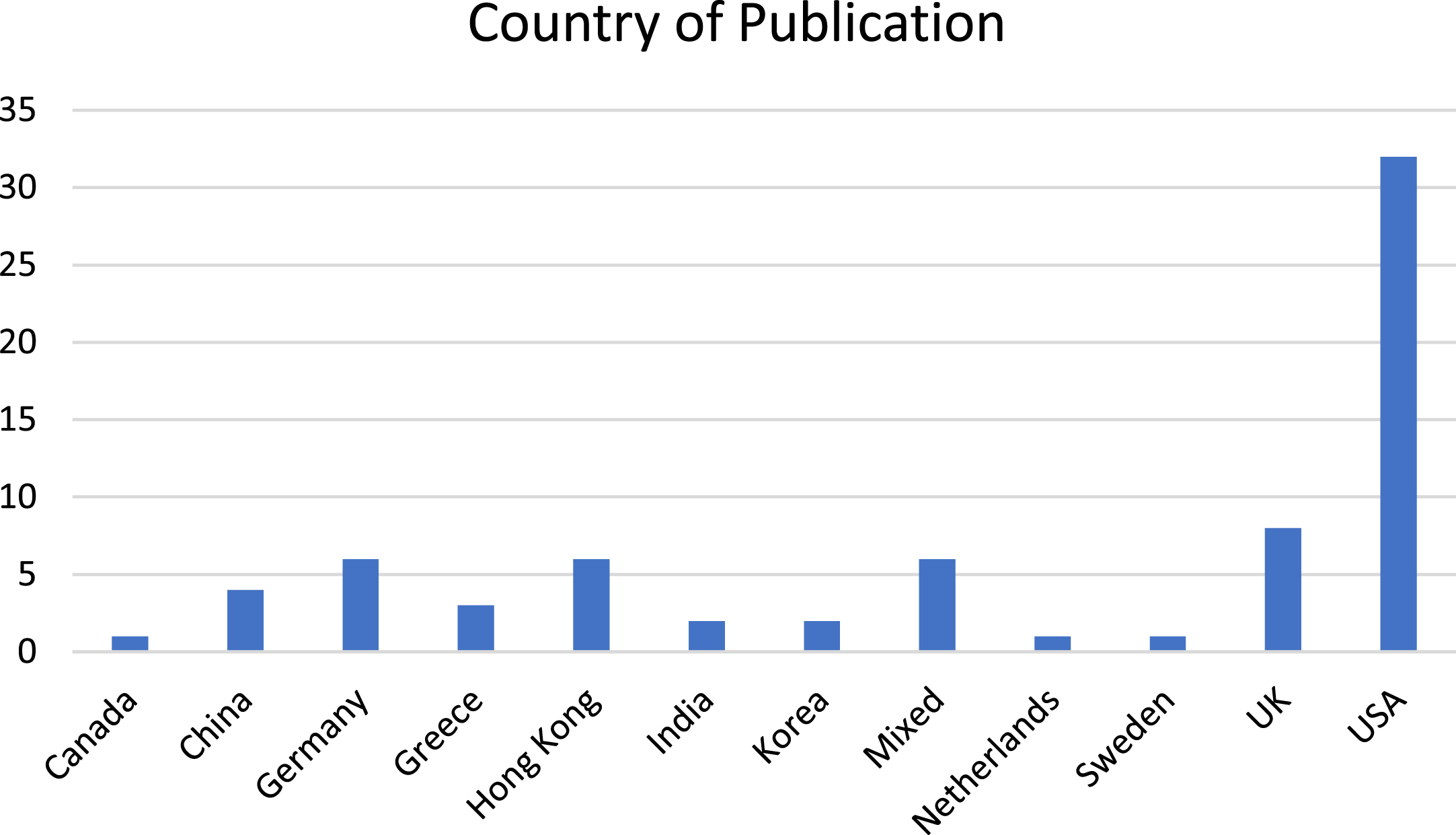
The earliest study was published in 2000 and this was followed by a twelve-year gap until 2012, although it is worth nothing that five articles from this period were excluded, as no participant data was included in the papers. Figure 3 provides an overview of the publications since 2000.
Fig. 3
Publications since 2000.
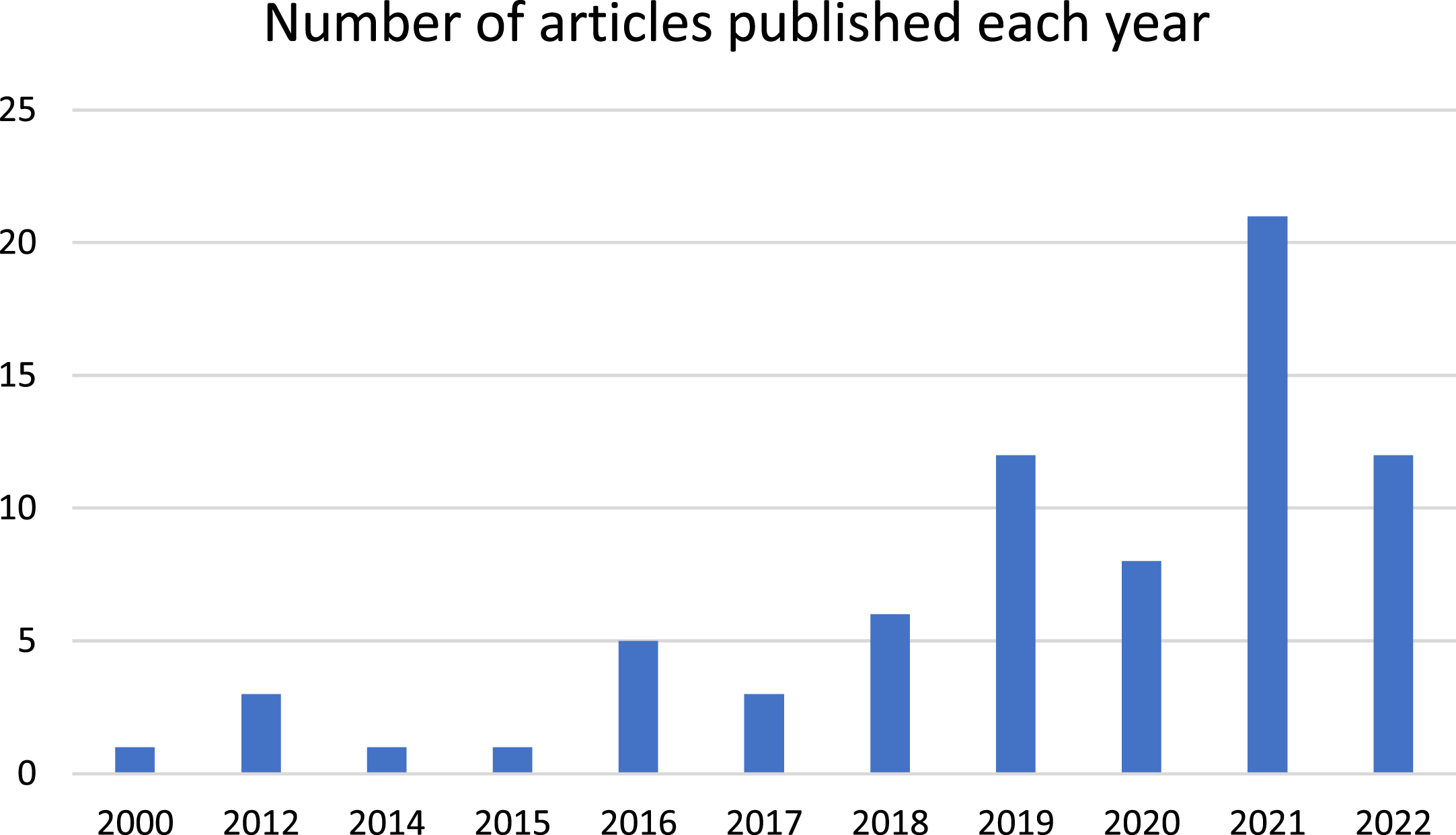
The studies appeared in publications and conference proceedings across a range of academic fields. The majority of studies appeared in neurology (24), stroke (11) and computing (14) publications, and the remaining in speech technology (9), multidisciplinary (7), speech and language (4) and health technology (2) publications. Fifty of the included studies were journal articles published in peer-review journals, 14 were conference proceeding and seven were conference abstracts. In addition, one preprint article was included in the review.
There was significant variation within the study aims, methods and data types. All studies included data from participants with aphasia and sixteen studies also included data from matched controls. The type of data varied across studies and included a range of language assessments such as Aachen Aphasia Test, Boston Naming Test, Cantonese Aphasia Battery, Comprehensive Assessment of Aphasia, North Western Naming Batter, North Western Assessment of Verbs and Sentences, Psycholinguistic Assessment of Language Processing in Aphasia, Philadelphia Naming Test, Philadelphia Repetition Test, Pyramids and Palm Tests, Token Test and the Western Aphasia Battery, along with other non-standardised language assessments. Other data gathered included audio recordings as well as CT scans, electroencephalogram and structural MRI and fMRI, in addition data collected from mobile therapy applications was also used in analysis. The wide range and variation in reporting of participant and assessment data prevents synthesis of findings at a population level. Instead, the areas of research are mapped and described below in keeping with the methodology of the mapping review.
3.2Areas of research within big data in aphasia
The 72 studies spanned a range of subject matter and different fields within big data and AI research in aphasia. These subject categories are outlined in Table 2 and are described further below. The inductively developed framework with subject categories and sub-categories is also visually represented in Fig. 4. A sample study from each category and subcategory is presented in Table 3.
Table 2
Framework of big data, AI and ML research in post-stroke aphasia
Category | Subcategory | Number of papers |
Prediction and Association | Lesion-symptom mapping | 21 |
Meta-analysis of Big Data | 9 | |
Associations and predictions using AI or ML | 5 | |
Tailoring therapy application | 2 | |
Automation | Assessment | 2 |
Automatic Speech recognition | 19 | |
Diagnosis | 10 | |
Hypothesis/theory testing | 3 | |
Aphasia classification model | 1 |
Fig. 4
Framework of Big Data and AI research in Post-Stroke Aphasia.
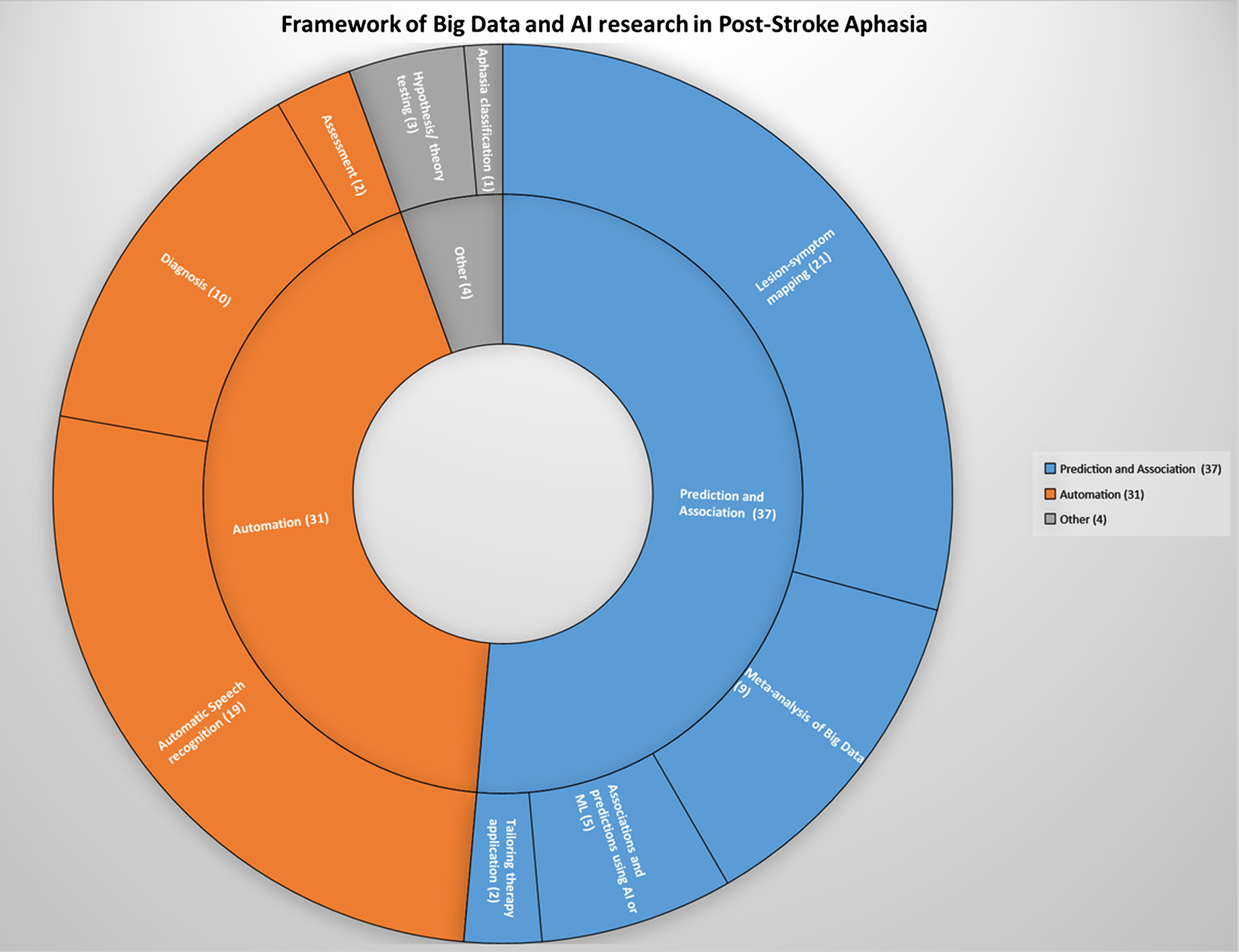
3.2.1Prediction and association
Thirty-seven studies were categorized under prediction and association. These included lesion-symptom mapping studies, meta-analyses - which applied statistical methods to large datasets - as well as studies that used AI or machine learning algorithms to explore associations and predictive models with respect to symptoms and outcomes, or to tailor and personalise therapy apps for individuals. These studies sought to understand relationships between variables and outcomes for specific phenomenon in aphasia, for example the degree of recovery in aphasia, or to use variables to create predictors through the application of machine learning techniques.
3.2.1.1Lesion-symptom mapping.
Twenty-one studies were categorized as lesion-symptom mapping studies. These studies used machine learning methods including support vector machines, decision tree analysis, random forest classifiers, Chi square automatic interaction detection, dynamic causal modelling, Pearson correlation and regression modelling to gain predictive insight from large amounts of neuroimaging data. All studies used lesion data from neuroimaging and there was variation in other data collected for analysis within the studies. Such data was dependent on the research aim and included standardized and non-standardized (cognitive and language) assessment results, demographic and medical information as well as aphasia type. Examples of research aims within these studies included the development of a predictive model of aphasia recovery from language test scores and neuroimaging (Lai et al.; Loughnan et al., 2019) and examination of the relationship between Health-Related Quality of Life and demographic factors, impairment-based measures, and lesion characteristics in chronic aphasia (Dvorak et al., 2021).
3.2.1.2Meta-analyses.
Nine studies were categorized as meta-analyses. These studies included data collated from completed aphasia studies. Numbers of subjects in these studies ranged from 2,500 to 5,928 as well as one study which collated data from 149,560 individuals (although not all were individuals with aphasia). Six of these meta-analyses aimed to gain insight into the evolution of and degree of recovery from aphasia. All except one article was produced by authors involved in the CATs REhabilitation and recovery of peopLE with Aphasia after StrokE (RELEASE) initiative (see https://www.aphasiatrials.org/release/ for more information).
3.2.1.3AI or machine learning algorithms.
Five studies were categorized under predicting outcomes and associations using AI and machine learning techniques. Four of these studies used machine-learning algorithms to develop prediction models from symptom patterns and severity and compared the models with actual outcomes. One study investigated the classification of symptom clusters based on language task performance using machine learning algorithms in order to identify features strongly associated with the clusters (Fromm et al., 2022).
Table 3
Framework and sample study per category
Category | Subcategory | Sample study | Title | Data set | Goal | Outcome |
Prediction and association | Lesion-symptom mapping | Loughnan et. al 2019 | Generalizing post-stroke prognoses from research data to clinical data | 828 British Stroke Patients (training set) | This study aimed to produce a predictive model of aphasia recovery from language test scores and neuroimaging that generalised across different languages, aphasias, research settings and neuroimaging technologies. | Results suggest that models trained on English, chronic aphasia patients in a research setting with MRI images did generalise to spanish speaking acute aphasia patients in a clinical setting with CT images. |
Meta-analysis of Big Data | Williams et al. 2022 | Utilising a systematic review-based approach to create a database of individual participant data for meta- and network meta-analyses: the RELEASE database of aphasia after stroke | 5928 individual participant data from people with post-stroke aphasia | This study aims to report the development, preparation and establishment of an internationally agreed aphasia after stroke research database of individual participant data (IPD) to facilitate planned aphasia research analyses | The application of big data principles in the context of aphasia research; the rigorous methodology for data acquisition and cleaning can serve as a template for the establishment of similar databases in other research areas | |
Associations and predictions using AI or ML | Fromm et al. 2022 | Enhancing the classification of aphasia: A statistical analysis using connected speech | 306 People with aphasia | This study aims to identify coherent clusters of PWA based on language output using unsupervised statistical algorithms and to identify features that are most strongly associated with those clusters | Seven distinct clusters of PWA were identified by the K-means algorithm. Using the random forest algorithm, a classification tree was proposed and validated, showing 91% agreement with the cluster assignments. This representative tree used only two variables to divide the data into distinct groups: total words from free speech tasks and total closed-class words from the Cinderella storytelling task | |
Tailoring therapy application | Musso et al. 2022 | Aphasia recovery by language training using a brain-computer interface: A proof-of-concept study | 10 stroke patients with aphasia | This paper presents a new language training approach for the rehabilitation of patients with aphasia based on a brain–computer interface system. The system utilizes machine learning to decode individual brain states in a single trial based on task-informative features, e.g. evoked potentials. | Results obtained from this proof-of-concept study found that the high-intensity training (30 h, 4 days per week) was feasible, despite a high-word presentation speed and unfavourable stroke-induced EEG signal characteristics. Second, the training induced a sustained recovery of aphasia, which generalized to multiple language aspects beyond the trained task. | |
Automation | Assessment | Issa et al. 2021 | An objective tool for classification of language deficits in adults | Profiles of 49 participants diagnosed with aphasia was loaded onto MATLAB software as part of training for an Artificial Neural Network | The aim of the study was to build an objective tool that provides assistive objective evaluation along with confidence index on aphasic individuals and possible rehabilitation domains. | Positive agreement between the developed objective tool and traditional subjective evaluation. Hence this tool can help guide novice clinicians in decision making as well as planning appropriate intervention strategies |
Automatic Speech recognition | Le et al. 2018 | Automatic quantitative analysis of spontaneous aphasic speech | 401 People with aphasia and 187 control speakers without aphasia, spanning 19 sub-datasets and 130.9 h of speech. | This study aims to perform one of the first large-scale quantitative analysis of spontaneous aphasic speech based on automatic speech recognition (ASR) output. | Study results demonstrate that these measures can be used to accurately predict the revised Western Aphasia Battery (WAB-R) Aphasia Quotient (AQ) without the need for manual transcripts. | |
Diagnosis | Axer et al. 2000 | An aphasia database on the internet: A model for computer-assisted analysis in aphasiology | 254 people with aphasia | This study describes a web-based software model developed as an example for data mining in aphasiology | The classifier produced correct results in 92% of the test cases. The neural network approach is similar to grouping performed in group studies, while the nearest-neighbor method shows a design more similar to case studies. | |
Hypothesis/theory testing | Dickens et al. 2021 | Two types of phonological reading impairment in stroke aphasia | 30 adults with left-hemisphere stroke and 37 age- and education-matched controls | This study utilizes behavioural and neuroanatomical evidence to determine the explanatory value of integrating the architectural details of contemporary neurocognitive models of speech processing into a model of reading aloud. The authors tested competing predictions of the undifferentiated and differentiated phonological reading hypotheses. | The results clarify that at least two dissociable phonological processes contribute to the pattern of reading impairment in aphasia - these results motivate a revised cognitive model of reading aloud that incorporates a sensory-motor phonological circuit | |
Aphasia classification model | Landrigan et al. 2021 | A data-driven approach to post-stroke aphasia classification and lesion-based prediction | 296 participants between the ages of 18 and 80 and primarily monolingual English speakers (55% reported speaking a second language). Most participants had chronic aphasia (i.e. 46 months post stroke). | This study proposes an alternative classification method to the traditional Wernicke Lichtein model for people with aphasia. The study aimed to identify clusters of individuals with post-stroke aphasia who have similar deficit profiles. | The results of the (community detection analysis) CDA algorithm did not align with the traditional model of aphasia in either behavioural or neuroanatomical patterns. Instead, the results suggested that the primary distinction in aphasia (after severity) is between phonological and semantic processing rather than between production and comprehension. Further, lesion-based classification reached 75% accuracy for the CDA-based categories and only 60% for categories based on the traditional fluent/non-fluent aphasia distinction. |
3.2.1.4Tailoring therapy applications.
Two papers examine how big data can be used to tailor therapy tasks to individuals. Kiran et al. (2017) describe a method in which a large datasets gathered from the Constant Therapy app facilitates decision-making and is used to personalise neurorehabilitation. While Musso et al. (2022) describe the use of machine learning to decode signals from a brain-computer interface to generate immediate feedback for participants as part of a novel therapeutic approach.
3.2.2Automation
Thirty one studies were categorized under automation, encompassing a range of machine learning methods including classical machine learning, neural networks, dynamic time warping, class activation mapping, Kullback-Leibler divergence, decision trees, random forest, logistic regression, naïve Bayes classifiers, support vector machines, Gaussian mixture models and hidden Markov models. These studies sought to perform a process where human input is minimised, for example to process human speech into written text or to make a decision regarding diagnosis, without human involvement in the process.
3.2.2.1Automatic speech recognition.
Nineteen studies investigated automatic speech recognition (ASR). Nine studies used voice recordings from aphasic speech corpora such as AphasiaBank while the other 10 made their own recordings for analysis. As well as straightforward speech-to-text mechanisms, the studies also included methods of automating articulation, fluency and tone scores and measuring satisfaction levels, stress and depression from patient’s speech.
3.2.2.2Diagnosis and assessment.
Ten studies presented methods of automating the diagnostic process. Assessment measures included binary yes/no aphasia classification, predicting Western Aphasia Battery –Aphasia Quotient scores from recordings, semantic relatedness measures and detecting FAST symptoms from patient’s speech. Five of these studies used recordings from aphasic speech corpora such as AphasiaBank while four used their own recordings and transcriptions.
One study, which did not make use of recorded speech, suggested a method of reducing the high cognitive load on patients required by fMRI neuroimaging procedures (Lorenz et al., 2021). This was achieved by combining real-time fMRI with machine-learning to create a more targeted process which was quicker to administer and required the subject to complete fewer tasks.
3.2.3Hypothesis or theory testing
Three studies were categorized under hypothesis or theory testing. The studies used machine learning to test the application of models of language processing. Lissón et al. (2021) investigated whether sentence comprehension impairments in aphasia can be explained by difficulties arising from dependency completion processes in parsing. The study evaluated the activation-based model and the direct-access model of dependency completion difficulty. The authors used Bayesian machine learning methods to simulate the two models and compared their performance to real data from aphasic subjects completing a picture selection task. Arslan et al. (2017) compared the comprehension of subject and object questions in individuals with aphasia speaking German and Turkish (respectively, a head-initial and head-final language). Subjects’ responses to a picture selection task were analysed using logistic regression and random forest methods. The third study built on their findings from a lesion-symptom mapping study, by utilising machine learning, in order to investigate the contribution of phonological processes within cognitive models of reading in aphasia (Dickens et al., 2021).
3.2.4Aphasia classification model
One study was categorized under aphasia classification model (Landrigan et al., 2021). The authors proposed an alternative classification method to the traditional Wernicke-Lichtheim model for people with aphasia. The study combined statistical, machine learning, and MRI lesion mapping methods to examine behavioural deficit profiles and their lesion correlates and predictors in a cohort of 296 individuals with post-stroke aphasia. Individuals with aphasia were clustered based on their behavioural deficit profiles using a community detection analysis (CDA) algorithm and these clusters were compared with the traditional aphasia subtypes.
4Discussion
This mapping review examines the extent, range and nature of available literature on big data and AI research in aphasia. Seventy-two articles were eligible for inclusion in the review. These studies were published in a range of journals covering different academic disciplines and with the exception of a small number of meta-analyses, were primarily quasi-experimental studies. Research into big data and AI in aphasia is a growing area of interest, with an increasing number of articles published in recent years. The bulk of research in this field concerned automation in the form of automatic speech recognition as well as automated assessment and diagnostic processes. In addition, there is also a growing body of research on predicting outcomes through lesion-symptom mapping, meta-analyses of existing studies and prognostic modelling. Finally, a small number of studies investigate techniques for tailoring rehabilitation using AI and/or big data analysis as well as examining hypothesis/theory testing and new methods of aphasia classification.
While previous articles have focused on particular aspects of big data and AI in aphasia, these have not attempted to map the full extent of research in this field. Kiran (2016) discusses the utility of big data analysis for understanding how individual responsiveness to rehabilitation is influenced by a patient’s severity of language and cognitive impairment via data gathered from an app, whereas Faroqi-Shah (2016) discussed neuroimaging methods in neurorehabilitation, meta-analyses involving large aphasic databases garnered from clinical trials and corpora such as AphasiaBank. Our review provides the first map of the current evidence and research in this field and allows for the categorisation of research into big data and AI in aphasia (See Fig. 4).
This is a relatively new and divergent area of research with dissemination spanning a range of academic fields including, but not limited to speech and health technology, computing and neurology.
4.1Clinical and policy implications
Practitioners and researchers working with people with aphasia should be aware of the contribution of big data and AI to aphasia research. This is not a straightforward task as the research is relatively recent, growing exponentially and appears across a range of academic disciplines. This mapping review is a first step towards a holistic understanding of big data and AI in aphasia research. The advances in automating assessment and diagnostic processes outlined in this review, while still in their early stages, show promise for clinical practice (Fergadiotis et al., 2016; Issa et al., 2021). In the future, these may contribute to increased accuracy and efficiency in assessment and diagnosis. While also currently at an early stage, continued advances in automatic speech recognition are likely to become more feasible for use in real-world clinical applications (Le et al., 2018) as well as potentially improve the utility and effectiveness of augmentative and alternative communication devices in the future. Developments in predictive models offer insights into aphasia prognoses and response to rehabilitation (Kiran et al., 2017; Kristinsson et al., 2021) and this may carry further clinical and policy implications in the future. One of the key promises of big data has been the potential to personalise healthcare and rehabilitation (Sagiroglu & Sinanc, 2013). While researchers have explored the potential use of AI in order to generate computer-based speech and language therapy sessions, that can be tailored to the needs and interests of a person with aphasia (Higgins et al., 2016), only a small number of studies have examined the outcome of such an approach (Kiran et al., 2017; Musso et al., 2022). This is surprising considering research on the use of genetic algorithms to tailor and personalise self-administered therapy sessions (Higgins et al., 2014) and the recognition that self-administered aphasia rehabilitation is both acceptable to people with post-stroke aphasia (Kearns et al., 2021) and enables more intensive and extended treatment (Macoir et al., 2019). However, it is likely that growth in the study of signal processing, and specifically automatic speech recognition, has facilitated the advancement of this research in the context of aphasia. The use of open source software and authors’ wishes to make applications available through open source platforms e.g. GitHub will likely result in continued growth in both experimental and clinical applications.
4.2Areas for further research
This mapping review took a broad approach, as it was necessary to map the current body of research in big data and AI in aphasia research and therefore this task did not warrant a systematic review. More specific research should be carried out to further examine and evaluate the categories of research identified within big data and AI aphasia research in this review. The two categories with the largest number of studies are prediction and association, and automation; it would be appropriate to explore each further. Further research questions should evaluate the efficacy of automated assessment and diagnosis, automatic speech recognition and the accuracy of predicting outcomes through lesion-symptom mapping and through meta-analysis. Moreover, big data and AI have the potential to contribute to an improved evidence synthesis process in aphasia research generally. The CATs had described research into aphasia as having limited efficiency, strength and utility due to being language, region and discipline specific (COSTAction-IS1208, 2013-2017). In their Cochrane Systematic Review, Brady et al. (2016) identified that aphasia trials were suffering from a lack of consistency in methodology and reporting and this hampers evidence synthesis. On the one hand, the rise in big data and AI in aphasia research has contributed to these challenges by ‘adding to the pile’ of disparate forms of research. At the same time, it is contributing valuable new knowledge, applications, and insight into aphasia.
The term big data belies the fact that its value lies not just in processing and synthesising vast amounts of data but numerous types of data too. Ninety-five percent of what is termed big data is described as unstructured, which means it lacks the structural organisation of a spreadsheet or database required for simple analysis (Gandomi & Haider, 2015). For example, lesion-symptom mapping studies gain insights from processing and synthesising large numbers of diverse data including images alongside demographic and medical information. Similarly, meta-analyses collate, categorise and integrate data from thousands of participants in hundreds of studies with differing methodologies, levels of data reporting and outcome measures. It is possible that wider adoption of big data and AI such as classifier algorithms and regression analysis could go some way to support advancement in aphasia research.
4.3Strengths and weaknesses
Mapping reviews are a useful and valuable exercise in understanding a disparate body of work and this approach was an appropriate choice of review design for big data and AI in aphasia due to the broad nature of the subject. However, there are weaknesses implicit in this type of review. Firstly, while published, peer-reviewed mapping reviews were used to guide and inform this methods undertaken in this review, there is no standardized process for conduct and reporting mapping reviews. This is likely due to the relatively recent emergence of mapping reviews (Miake-Lye et al., 2016). Protocols such as PRISMA-P allow for planning and documentation of review methods, act as a guard against arbitrary decision-making during review conduct, enable readers to assess for the presence of selective reporting against completed reviews and reduce duplication of efforts (Shamseer et al., 2015). This guidance informed our review process. Both of the authors are speech and language therapists and while one author was studying for a diploma in computer science at the time of the review their professional backgrounds will have influenced the analysis process. The approach used in this review required the characterisation of included studies at a broad descriptive level and lacks the synthesis and analysis of other review methods. This approach results in a risk of oversimplification of findings. There is also a high level of subjectivity in this characterisation process (Grant & Booth, 2009). Lastly, in keeping with mapping review methods, a quality appraisal process was not carried out. While appropriate for this review method (Grant & Booth, 2009) it limits the analysis to descriptive synthesis of the included studies.
5Conclusion
This research aimed to examine the extent, range and nature of available literature on big data and AI research relating to aphasia. The findings from this mapping exercise, which categorised, described and mapped available evidence into an inductively developed framework, indicate that big data and AI is making a growing contribution to aphasia research. This contribution is largely that of automating assessment and diagnostic processes, automatic speech recognition and predicting outcomes for aphasic patients through lesion-symptom mapping and meta-analysis. The majority of the research is in the form of quasi-experimental studies. This is not unusual for early-stage research, particularly when clinical data is available and randomisation is not feasible due to methodological or funding constraints (Harris et al., 2006). The coming years may see increasing adoption of big data as well as the use of AI in larger scale and higher quality studies such as RCTs. Additionally, big data and artificial intelligence techniques provide a capacity to process large amounts of data, including unstructured data. Applied more widely and with transparency, these techniques may contribute towards solving issues of inconsistency in methodology and reporting between aphasia studies, which hampers evidence synthesis.
Acknowledgments
The authors would like to acknowledge the contribution of Elaine Geoghegan during the initial planning and data base searches.
Conflict of interest
Dr Áine Kearns is a guest editor on this Special Issue. However, she was blinded to the reviewing process for this paper, which was undertaken by a second Guest Editor.
Supplementary material
[1] The supplementary material is available in the electronic version of this article: https://dx.doi.org/10.3233/ACS-230005.
References
1 | Ali, M. , Vandenberg, K. , Williams, L. J. , Williams, L. R. , Abo, M. , Becker, F. , Bowen, A. , Brandenburg, C. , Breitenstein, C. , Bruehl, S. , Copland, D. A. , Cranfill, T. B. , Pietro-Bachmann, M. D. , Enderby, P. , Fillingham, J. , Lucia Galli, F. , Gandolfi, M. , Glize, B. , Godecke, E. , ... Brady, M. C. Predictors of Poststroke Aphasia Recovery, Stroke ((2021) ) 52: (5), 1778–1787. https://doi.org/10.1161/strokeaha.120.031162 |
2 | Altbach, P. G. , & De Wit, H. ((2018) ) Too Much Academic Research Is Being Published, International Higher Education (96), 2–3. https://doi.org/10.6017/ihe.2019.96.10767 |
3 | Arslan, S. , Gür, E. , & Felser, C. ((2017) ) Predicting the sources of impaired wh-question comprehension in non-fluent aphasia: A cross-linguistic machine learning study on Turkish and German, Cogn Neuropsychol 34: (5), 312–331. https://doi.org/10.1080/02643294.2017.1394284 |
4 | Beeson, P. M. , & Robey, R. R. ((2006) ) Evaluating single-subject treatment research: Lessons learned from the aphasia literature, Neuropsychology Review 16: , 161–169. |
5 | Brady, M. C. , Ali, M. , Vandenberg, K. , Williams, L. J. , Williams, L. R. , Abo, M. , Becker, F. , Bowen, A. , Brandenburg, C. , Breitenstein, C. , Bruehl, S. , Copland, D. A. , Cranfill, T. B. , Di Pietro-Bachmann, M. , Enderby, P. , Fillingham, J. , Galli, F. L. , Gandolfi, M. , Glize, B. , ... Wright, H. H. ((2022) ) Complex speech-language therapy interventions for stroke-related aphasia: the RELEASE study incorporating a systematic review and individual participant data network meta-analysis, Health and Social Care Delivery Research 10: (28), 1–272. https://doi.org/10.3310/rtlh7522 |
6 | Brady, M. C. , Kelly, H. , Godwin, J. , Enderby, P. , & Campbell, P. ((2016) ) Speech and language therapy for aphasia following stroke, Cochrane Database of Systematic Reviews (6). |
7 | COSTAction-IS1208. (2013-2017). Collaboration of Aphasia Trialists (CATs). Retrieved 03/11/2022 from https://www.cost.eu/actions/IS1208/ |
8 | Dash, S. , Shakyawar, S. K. , Sharma, M. , & Kaushik, S. ((2019) ) Big data in healthcare: management, analysis and future prospects, Journal of Big Data 6: (1). https://doi.org/10.1186/s40537-019-0217-0. |
9 | Dickens, J. V. , DeMarco, A. T. , van der Stelt, C. M. , Snider, S. F. , Lacey, E. H. , Medaglia, J. D. , Friedman, R. B. , & Turkeltaub, P. E. ((2021) ) Two types of phonological reading impairment in stroke aphasia, Brain Communications 3: (3). https://doi.org/10.1093/braincomms/fcab194 |
10 | Dvorak, E. L. , Gadson, D. S. , Lacey, E. H. , DeMarco, A. T. , & Turkeltaub, P. E. ((2021) ) Domains of Health-Related Quality of Life Are Associated With Specific Deficits and Lesion Locations in Chronic Aphasia, Neurorehabil Neural Repair 35: (7), 634–643. https://doi.org/10.1177/15459683211017507 |
11 | Faroqi-Shah, Y. ((2016) ) The Rise of Big Data in Neurorehabilitation, Seminars in Speech and Language 37: (01), 003–009. https://doi.org/10.1055/s-0036-1572385 |
12 | Fergadiotis, G. , Gorman, K. , & Bedrick, S. ((2016) ) Algorithmic Classification of Five Characteristic Types of Paraphasias, Am J Speech Lang Pathol 25: (4s), S776–s787. https://doi.org/10.1044/2016_ajslp-15-0147 |
13 | Fromm, D. , Greenhouse, J. , Pudil, M. , Shi, Y. , & MacWhinney, B. ((2022) ) Enhancing the classification of aphasia: a statistical analysis using connected speech, Aphasiology 36: (12), 1492–1519. |
14 | Gandomi, A. , & Haider, M. ((2015) ) Beyond the hype: Big data concepts, methods, and analytics, International Journal of Information Management 35: (2), 137–144. https://doi.org/10.1016/j.ijinfomgt.2014.10.007 |
15 | Grant, M. J. , & Booth, A. ((2009) ) A typology of reviews: ananalysis of 14 review types and associated methodologies, Health Information & Libraries Journal 26: (2), 91–108. https://doi.org/10.1111/j.1471-1842.2009.00848.x |
16 | Harris, A. D. , McGregor, J. C. , Perencevich, E. N. , Furuno, J. P. , Zhu, J. , Peterson, D. E. , & Finkelstein, J. ((2006) ) The use and interpretation of quasi-experimental studies in medical informatics, J Am Med Inform Assoc 13: (1), 16–23. https://doi.org/10.1197/jamia.M1749 |
17 | Higgins, C. , Kearns, Á. , Ryan, C. , Fernstrom, M. (2016). The role of gamification and evolutionary computation in the provision of self-guided speech therapy. In Handbook of Research on Holistic Perspectives in Gamification for Clinical Practice (pp. 158-182). IGI Global. |
18 | Higgins, C. , Ryan, C. , Kearns, A. , Fernstrom, M. (2014). The creation and facilitation of speech and language therapy sessions for individuals with aphasia. |
19 | Issa, S. H. , Ahmed, H. R. , Alwan, E. E. , Mutahar, A. A. , Bajiri, M. E. , & BP, A. ((2021) ) An objective tool for classification of language deficits in adults, Review of International Geographical Education Online 11: (5). |
20 | James, K. L. , Randall, N. P. , & Haddaway, N. R. ((2016) ) A methodology for systematic mapping in environmental sciences, Environmental Evidence 5: (1). https://doi.org/10.1186/s13750-016-0059-6 |
21 | Kearns, Á. , Kelly, H. , & Pitt, I. ((2021) ) Self-reportedfeedback in ICT-delivered aphasia rehabilitation: a literaturereview, Disabil Rehabil 43: (9), 1193–1207. https://doi.org/10.1080/09638288.2019.1655803 |
22 | Kiran, S. ((2016) ) How Does Severity of Aphasia Influence Individual Responsiveness to Rehabilitation? Using Big Data to Understand Theories of Aphasia Rehabilitation, Semin Speech Lang 37: (1), 48–60. https://doi.org/10.1055/s-0036-1571358 |
23 | Kiran, S. , Godlove, J. , Advani, M. , & Anantha, V. ((2017) ) Abstract TMP: Personalizing Rehabilitation for Stroke Survivors- A Big Data Approach, Stroke 48: (suppl_1), ATMP44-ATMP44. https://doi.org/10.1161/str.48.suppl_1.tmp44 |
24 | Kristinsson, S. , Zhang, W. , Rorden, C. , Newman-Norlund, R. , Basilakos, A. , Bonilha, L. , Yourganov, G. , Xiao, F. , Hillis, A. , & Fridriksson, J. ((2021) ) Machine learning-based multimodal prediction of language outcomes in chronic aphasia, Human Brain Mapping 42: (6), 1682–1698. https://doi.org/10.1002/hbm.25321 |
25 | Lai, S. , Billot, A. , Varkanitsa, M. , Braun, E. , Rapp, B. , Parrish, T. , Kurani, A. , Higgins, J. , Caplan, D. , Thompson, C. , Kiran, S. , Betke, M. , Ishwar, P. (2021). An Exploration of Machine Learning Methods for Predicting Post-stroke Aphasia Recovery. |
26 | Landrigan, J.-F. , Zhang, F. , & Mirman, D. ((2021) ) A data-driven approach to post-stroke aphasia classification and lesion-based prediction, Brain 144: (5), 1372–1383. https://doi.org/10.1093/brain/awab010 |
27 | Le, D. , Licata, K. , & Mower Provost, E. ((2018) ) Automatic quantitative analysis of spontaneous aphasic speech, Speech Communication 100: , 1–12. https://doi.org/10.1016/j.specom.2018.04.001 |
28 | Lissón, P. , Pregla, D. , Nicenboim, B. , Paape, D. , Het Nederend, M. L. , Burchert, F. , Stadie, N. , Caplan, D. , & Vasishth, S. ((2021) ) A Computational Evaluation of Two Models of Retrieval Processes in Sentence Processing in Aphasia, Cognitive Science 45: (4). https://doi.org/10.1111/cogs.12956 |
29 | Lorenz, R. , Johal, M. , Dick, F. , Hampshire, A. , Leech, R. , & Geranmayeh, F. ((2021) ) A Bayesian optimization approach for rapidly mapping residual network function in stroke, Brain 144: (7), 2120–2134. https://doi.org/10.1093/brain/awab109 |
30 | Loughnan, R. , Lorca-Puls, D. L. , Gajardo-Vidal, A. , Espejo-Videla, V. , Gillebert, C. R. , Mantini, D. , Price, C. J. , & Hope, T. M. H. ((2019) ) Generalizing post-stroke prognoses from research data to clinical data, Neuroimage Clin 24: , 102005. https://doi.org/10.1016/j.nicl.2019.102005. |
31 | Macoir, J. , Lavoie, M. , Routhier, S. , & Bier, N. ((2019) ) Key Factors for the Success of Self-Administered Treatments of Poststroke Aphasia Using Technologies, Telemedicine and E-Health 25: (8), 663–670. https://doi.org/10.1089/tmj.2018.0116 |
32 | MacWhinney, B. , & Fromm, D. ((2016) ) AphasiaBank as BigData, Semin Speech Lang 37: (1), 10–22. https://doi.org/10.1055/s-0036-1571357 |
33 | Miake-Lye, I. M. , Hempel, S. , Shanman, R. , & Shekelle, P. G. ((2016) ) What is an evidence map? A systematic review of published evidence maps and their definitions, methods, and products, Systematic reviews 5: (1). https://doi.org/10.1186/s13643-016-0204-x |
34 | Musso, M. , Hübner, D. , Schwarzkopf, S. , Bernodusson, M. , LeVan, P. , Weiller, C. , & Tangermann, M. ((2022) ) Aphasia recovery by language training using a brain-computer interface: a proof-of-concept study, Brain Commun 4: (1), fcac008. https://doi.org/10.1093/braincomms/fcac008 |
35 | NOCA. (2021). Irish National Audit of Stroke National Report 2020. N. O. o. C. Audit. https://s3-euwest-1.amazonaws.com/noca-uploads/general/Irish_National_Audit_of_Stroke_National_Report_2020_FINAL.pdf |
36 | O’Cathain, A. , Thomas, K. J. , Drabble, S. J. , Rudolph, A. , & Hewison, J. ((2013) ) What can qualitative research do for randomised controlled trials? A systematic mapping review, BMJ open 3: (6), e002889. https://doi.org/10.1136/bmjopen-2013-002889. |
37 | Page, M. J. , McKenzie, J. E. , Bossuyt, P. M. , Boutron, I. , Hoffmann, T. C. , Mulrow, C. D. , Shamseer, L. , Tetzlaff, J. M. , Akl, E. A. , Brennan, S. E. , Chou, R. , Glanville, J. , Grimshaw, J. M. , Hróbjartsson, A. , Lalu, M. M. , Li, T. , Loder, E. W. , Mayo-Wilson, E. , McDonald, S. , ... Moher, D. (2021). The PRISMA 2020 statement: an updated guideline for reporting systematic reviews. Bmj, n71. doi:10.1136/bmj.n71 |
38 | Sagiroglu S. , Sinanc D. (2013, 2013). Big data: A review. |
39 | Senders, J. T. , Staples, P. C. , Karhade, A. V. , Zaki, M. M. , Gormley, W. B. , Broekman, M. L. D. , Smith, T. R. , & Arnaout, O. ((2018) ) Machine Learning and Neurosurgical Outcome Prediction: A Systematic Review, World Neurosurg 109: , e471. https://doi.org/10.1016/j.wneu.2017.09.149 |
40 | Shamseer, L. , Moher, D. , Clarke, M. , Ghersi, D. , Liberati, A. , Petticrew, M. , Shekelle, P. , & Stewart, L. A. ((2015) ) Preferred reporting items for systematic review and meta-analysis protocols (PRISMA-P) 2015: elaboration and explanation, BMJ: British Medical Journal 349: , g7647. https://doi.org/10.1136/bmj.g7647. |
41 | Wallace, S. J. , Worrall, L. , Rose, T. , Le Dorze, G. , Breitenstein, C. , Hilari, K. , Babbitt, E. , Bose, A. , Brady, M. , Cherney, L. R. , Copland, D. , Cruice, M. , Enderby, P. , Hersh, D. , Howe, T. , Kelly, H. , Kiran, S. , Laska, A.-C. , Marshall, J. , ... Webster, J. ((2019) ) A core outcome set for aphasia treatment research: The ROMA consensus statement, International Journal of Stroke 14: (2), 180–185. https://doi.org/10.1177/1747493018806200 |
42 | Whittemore, R. , & Knafl, K. ((2005) ) The integrative review: updated methodology, Journal of Advanced Nursing 52: (5), 546–553. https://doi.org/10.1111/j.1365-2648.2005.03621.x |