Precompetitive Data Sharing as a Catalyst to Address Unmet Needs in Parkinson’s Disease1
Abstract
Parkinson’s disease is a complex heterogeneous disorder with urgent need for disease-modifying therapies. Progress in successful therapeutic approaches for PD will require an unprecedented level of collaboration. At a workshop hosted by Parkinson’s UK and co-organized by Critical Path Institute’s (C-Path) Coalition Against Major Diseases (CAMD) Consortiums, investigators from industry, academia, government and regulatory agencies agreed on the need for sharing of data to enable future success. Government agencies included EMA, FDA, NINDS/NIH and IMI (Innovative Medicines Initiative). Emerging discoveries in new biomarkers and genetic endophenotypes are contributing to our understanding of the underlying pathophysiology of PD. In parallel there is growing recognition that early intervention will be key for successful treatments aimed at disease modification. At present, there is a lack of a comprehensive understanding of disease progression and the many factors that contribute to disease progression heterogeneity. Novel therapeutic targets and trial designs that incorporate existing and new biomarkers to evaluate drug effects independently and in combination are required. The integration of robust clinical data sets is viewed as a powerful approach to hasten medical discovery and therapies, as is being realized across diverse disease conditions employing big data analytics for healthcare. The application of lessons learned from parallel efforts is critical to identify barriers and enable a viable path forward. A roadmap is presented for a regulatory, academic, industry and advocacy driven integrated initiative that aims to facilitate and streamline new drug trials and registrations in Parkinson’s disease.
ABBREVIATIONS
CamPaIGN | Cambridgeshire Parkinson’s Incidence from GP to Neurologist |
OxfordPD | The Oxford Parkinson’s Disease Centre (OPDC) Discovery cohort |
ProBaND | Parkinson’s Repository of Biosamples and Networked Datasets |
PPMI | Parkinson’s Progression Marker Initiative |
ADAGIO | Attenuation of Disease progression with Azilect Given Once Daily |
DATATOP | Deprenyl and tocopherol antioxidative therapy of parkinsonism |
PRECEPT | Parkinson Research Examination of CEP-1347 Trial |
SWEDD | Scans without evidence of dopamine deficiency |
Glossary of terms
PD | Parkinson’s disease |
ACE-R | Addenbrooke’s Cognitive Examination Revised |
AD | Alzheimer’s disease |
ADAGIO | Attenuation of Disease Progression with Azilect Given Once-daily |
ADNI | Alzheimer’s Disease Neuroimaging Initiative |
ALS | Amyotrophic Lateral Sclerosis |
CAMD | Coalition Against Major Diseases |
CamPaIGN | Cambridgeshire Parkinson’s Incidence from GP to Neurologist |
CDE | Common Data Elements |
CDISC | Clinical Data Interchange Standards Consortium |
CHET | Center for Human Experimental Therapeutics |
CODR | C-Path Online Data Repository |
C-Path | Critical Path Institute |
CSF | Cerebrospinal Fluid |
DESCRIPA | Development of Screening Guidelines and Criteria for Predementia Alzheimer’s disease |
DMR | Data Management Resource |
DPUK | Dementias Platform UK |
DTI/RS MRI | Diffusion Tensor Imaging/Resting State MRI |
EMA | European Medicines Agency |
EMIF | European Medical Information Framework |
ET | Essential Tremor |
FDA | US Food and Drug Administration |
GAAIN | Global Alzheimer’s Association Interactive Network |
ICICLE-PD | Incidence of Cognitive Impairment in Cohorts with Longitudinal Evaluation PD |
IDA | Imaging Data Archive |
IMI | Innovative Medicines Initiative |
IRB | Institutional Review Board |
LONI | Laboratory of Neuro Imaging |
MDS-UPDRS | Movement Disorders Society-UPDRS |
MMSE | Mini-Mental State Examination |
MRI | Magnetic Resonance Imaging |
MSA | Multisystem Atrophy |
MSOAC | Multiple Sclerosis Outcomes Assessment Consortium |
INTRODUCTION
Parkinson’s disease (PD) is the second most common neurodegenerative disease after Alzheimer’s disease (AD) [1]. Primarily a disease of adults over the age of 60 yrs who may also have other comorbidities, about 4% of cases begin prior to the age of 50 [2].
Therapeutic goals for PD are based on symptomatic relief, but halting or slowing the neurodegenerative process and the prevention of long-term adverse outcomes represent urgent unmet needs. Available dopamine-based therapeutic strategies achieve the reduction of motor symptoms, but do not significantly impact on the numerous non-motor manifestations of PD [3]. Fluctuation phenomena and dyskinesia remain a challenge, particularly in long-term treatment [4]. It is now recognized that the first signs of motor impairment in PD (early motor PD) is preceded for many years by pathologic changes (reduced dopamine nerve terminal function) and a variety of other biomarker changes including rapid eye movement (REM) sleep behavioral disturbances, GI disturbances and olfactory deficits.
A major challenge is our lack of understanding of disease progression and heterogeneity. Despite the many candidate disease modifying therapies tested to date, there is a lack of retrospective learning from these costly clinical trials. For example, analysis of the failed PRECEPT study [5] illustrates that many subjects who were enrolled did not have evidence of dopaminergic deficit or disease progression and thus were unlikely to have PD. At present, the field lacks a comprehensive, standardized and integrated database of relevant longitudinal studies and clinical trials in PD.
Big data analytics in healthcare has evolved significantly as an innovative approach for providing insight from very large data sets with the goal of improving patient outcomes in clinical practice and drug development. Other diseases have undergone efforts to standardize and integrate relevant data, which have advanced therapeutic trial designs and enabled model-based drug development and personalized medicine strategies. Examples include: from the oncology field, DataSphere [6], from Amyotrophic Lateral Sclerosis (ALS), the PRO-ACT database [7, 8], from Alzheimer’s disease, Critical Path Institute (C-Path) Online Data Repository (CODR) [9]; and from Multiple Sclerosis, the Multiple Sclerosis Outcomes Assessment Consortium (MSOAC) database [10]. PD stands to benefit from applying such learnings, particularly given the advances in our understanding of these diseases and vast amounts of data not presently available for broad use.
The future of open science is catalyzed by the expanding landscape of precompetitive collaborative consortia. The term ‘precompetitive’ refers to collaboration on projects of mutual benefit between diverse stakeholders, including industry partners that may produce competing goods or commercial products later in the R&D process. Precompetitive collaboration improves the prospects of all stakeholders relative to common challenges they face. It can also benefit the public by reducing duplication of effort, increasing the effectiveness of R&D investments, and establishing common standards and resources. Consortia and precompetitive collaborations are highlighted in the recent Nature Reviews Drug Discovery issue dedicated to US precompetitive consortia [11] (http://www.nature.com/nrd/focus/consortia/index.html) and are embraced in Europe through the Innovative Medicines Initiative (IMI) [12, 13] with endorsement of public-private partnerships [14].
To begin developing a strategic framework focused on data sharing as the path towards successful drug development in Parkinson’s disease, Parkinson’s United Kingdom (Parkinson’s UK) convened a meeting in London in May, 2014. Diverse stakeholders included representatives from academia, patient advocacy and charitable organizations, government agencies, regulatory agencies and precompetitive consortia. The goals of this meeting were to identify questions that, if answered, could tangibly impact PD drug development, explore how existing data might provide answers to those questions, and identify the barriers to using those data, with the goal of mapping out a path forward. In parallel with the above efforts, notably, government agencies have also initiated development of strategic recommendations that are desperately needed to fill the gaps in PD research and drug development. For example, in January, 2014 the National Institute on Neurologic Disorders and Stroke (NINDS) convened an expert meeting aimed at building consensus on research priorities for PD across basic, clinical, and translational domains [15]. Big data was a key area of focus and a strategic priority for the future. Recognizing that pooling and sharing data is a costly endeavor that requires collaboration from diverse stakeholders {e.g., [16]}, workshop participants addressed impediments to data sharing and potential solutions that have gained traction in other fields. They also considered new data sources including data collected from personal- and home-monitoring devices, which may provide relevant measures of the functional impairments associated with PD. Finally, they mapped out plans for using these data to build quantitative drug development tools through regulatory paths to increase the efficiency of clinical trials. The following themes were identified as gaps in the field and areas of focus during the Parkinson’s UK/Coalition Against Major Diseases (CAMD) two-day workshop:
• The need for regulatory approved endpoints, trial designs, and modeling tools.
• Identification of indicators of very early disease state markers to foster development of pre-symptomatic and potential neuroprotective or neuromodulatory treatments.
• Development of reliable biomarkers to monitor disease progression, particularly to assess agents that may modify the course of the disease.
• Understanding disease subtypes to enable the stratification of patients to allow for more efficient clinical trials.
REVIEW OF DATA SOURCES (SEE TABLE 1)
Information that could provide greater understanding of how PD progresses across the trajectory of the disease lies in large datasets collected in clinical and observational studies over the past decades. Unfortunately, these datasets are inherently complex in nature and have been largely inaccessible to researchers, thereby limiting innovative analyses and generation of new knowledge. Workshop participants thusprioritized efforts to obtain access to and develop a means of aggregating, comparing, and analyzing such data. Existing datasets held by pharmaceutical companies as well as academic research groups were targeted for inclusion with the aim of enabling data-sharing of clinical, imaging, and biomarker data. Other potential data sources include electronic medical records and claims data from public and private payers. Notably, the intent of this meeting was not to catalogue and inventory all possible sources of PD data globally. Rather, datasets owned by meeting participants that may be available for sharing were described and discussed collectively, with the common goal of integration for the future.
Pharmaceutical clinical data, Teva Pharmaceuticals
Teva Pharmaceuticals plans to release treatment and control arm data from two clinical trials: PRECEPT, a trial of the kinase inhibitor CEP-1347, which enrolled 806 patients with early PD [17]; and ADAGIO (Attenuation of Disease progression with Azilect Given Once Daily), a trial of rasagiline in early idiopathic PD that enrolled 1176 participants [18]. Outcome measures in PRECEPT included the Unified Parkinson’s Disease Rating Scale (UPDRS) and β-CIT SPECT imaging of the dopamine transporter [5]. The trial terminated early when pre-specified endpoints for futility were reached; but the sponsor continued to follow subjects with biomarker studies in the PostCEPT observational study, now the largest cohort of PD patients actively followed in North America. PostCEPT provided blood samples for genetic studies and cell line repository and conducted a substudy of α-synuclein and RNA biomarkers. The ADAGIO study is a large randomized clinical trial evaluating the effects of rasagaline in a delayed start design. These data, available to the CAMD Consortium, will support development of biomarkers and innovative clinical trial modeling tools for paving the regulatory route for Parkinson’s treatment.
The Oxford Parkinson’s Disease Centre (OPDC) Discovery cohort (http://opdc.medsci.ox.ac.uk) was established in 2009 with funding from Parkinson’s UK as a longitudinal study of 1000 early stage, population-ascertained PD subjects recruited from the Thames Valley, UK. Its primary goal is to investigate the earliest genetic, molecular, cellular, and neuronal pathways affected in PD, and to identify novel biomarkers for early diagnosis and prognostication. Data and tissue collected from OPDC subjects include a wide range of clinical motor, non-motor, and cognitive assessments, serum, plasma, DNA and CSF samples, skin biopsies with induced pluripotent stem cell generation, and MRI brain studies [19– 21]. Three hundred control and 170 PD at-risk subjects have been recruited to the Discovery cohort thus far, with 18-month longitudinal follow-up now ongoing and guaranteed for a 10-year observation period in the PD and PD at-risk groups.
The Tracking Parkinson’s Study - Parkinson’s Repository of Biosamples and Networked Datasets (PRoBaND)
PRoBaND has enrolled 2000 subjects with recent onset, including 240 young onset patients, and is enrolling 840 unaffected siblings from 60 active recruiting centers (http://proband.org.uk/). In addition to clinical, demographic, and genetic data, PRoBaND has collected data on cognition, olfactory function, sleep, autonomic function, quality of life, and environmental exposures. Investigators will be asking multiple research questions, including comparing young and recent onset PD (e.g., progression, response to therapy), definition of subtypes (including genetics), and the relationship between non-motor and motor features.
Cambridgeshire Parkinson’s Incidence from GP to Neurologist (CamPaIGN)
The CamPaIGN study collected a population-based cohort of newly diagnosed PD cases in the county of Cambridgeshire, UK over two-year period (Dec 2000-Dec 2002), in order to estimate the incidence of PD and parkinsonism in this region, and to characterize the frequency and pattern of cognitive impairment in a population-representative incident PD cohort [22]. The cohort has since been prospectively followed, with 10-year data recently published [23]. Longitudinal assessments have included standardized motor assessments such as the UPDRS and Hoehn and Yahr scale, neuropsychological assessments, and standardized measures of mood, function and quality of life. DNA samples have also been collected. The cohort comprises 142 cases meeting UKPDS Brain Bank criteria for PD. Following attrition due to death and loss to follow-up, 49 remained at the 10-year timepoint. Follow-up is ongoing, with 12-year data currently being collected.
Parkinsonism: Incidence and Cognitive Heterogeneity in Cambridgeshire (PICNICS)
PICNICS is a sequential comparative incidence study in the same Cambridgeshire population. During an extended recruitment period (2008– 2013), 286 cases meeting UKPDS Brain Bank criteria idiopathic PD were enrolled. Patients are being followed up at 18-month intervals with a more extended panel of assessments than the CamPaIGN cohort, including the revised Movement Disorders Society-UPDRS (MDS-UPDRS) [24] and incorporating the Addenbrooke’s Cognitive Examination Revised (ACE-R) into the cognitive battery [25]. A subgroup of PICNICS subjects are also enrolled in the Incidence of Cognitive Impairment in Cohorts with Longitudinal Evaluation PD (ICICLE-PD) study, which incorporates a number of additional non-motor questionnaires, more extensive neuropsychological testing, saccadometry, and biomarker analysis [26]. The maximum duration of follow-up of the PICNICS cohort is 72 months at the time of this meeting.
The Parkinson Progression Marker Initiative (PPMI) biomarker study
PPMI mimics the landmark Alzheimer’s Disease Neuroimaging Initiative (ADNI) in terms of a focus on standardizing protocols and providing the research community with open access to data and biosamples. At the time of the London meeting, over 180,000 data downloads and over 40 biological specimens had been requested through the ADNI. Data collected through the PPMI include clinical (motor and non-motor, neurobehavioral/cognitive, autonomic, olfaction, sleep), imaging (DaTscanTM, AV133, amyloid, DTI/RS MRI), and corresponding biological samples (DNA, blood, CSF). The PPMI study population originally included 400 newly diagnosed and unmedicated PD subjects as well as 200 age- and gender-matched healthy controls, and 70 subjects with a clinical diagnosis of PD but without evidence of dopaminergic deficiency (SWEDD) by dopamine transporter SPECT imaging [27]. Subsequently, three other subgroups were added: 100 pre-motor, 500 subjects with LRRK2 mutations, and 100 with α-synuclein mutations (50 with PD and 50 unaffected family members). There are also future plans to incorporate novel data sources that include wearable sensors in PPMI.
Parkinson’s Study Group (PSG) and the University of Rochester Center for Human Therapeutics (CHET)
PSG is a network of 132 Parkinson centers in the United States, Canada and Puerto Rico, created to conduct clinical trials in a consistently rigorous manner. PSG conducted the clinical trials that led to the approval of five PD marketed drugs, as well as many other trials conducted through the NIH Exploratory Trials in PD (NET-PD). Data from PSG, NET-PD, and the PPMI studies are managed by the Center for Human Experimental Therapeutics (CHET) at the University of Rochester. The CHET coordinating center currently houses data from over 40 PD clinical studies enrolling 7000 PD participants as well as from observational studies, including data from physician-rated clinical scales such as the UPDRS, Mini-Mental State Examination (MMSE) and the Beck Depression Inventory, as well as patient-reported outcomes data, imaging, laboratory and biomarkers, genetics, and demographics.
The PSG hosts a list of data on the website, a short narrative about what the study covers, and guidance on how to access the data (http://www.parkinson-study-group.org/). The review process is coordinated by the Michael J Fox Foundation and any researcher can apply. There have been over 200 publications resulting from the use of these data to date and future use is encouraged, especially for modeling disease progression Data used in modeling is only about 20% of the data available through the PSG, but additional data sources are relevant for this purpose (Table 1). Modeling and simulation are a key part of learning and confirming drugs, doses and outcomes and should be used to improve the design of clinical trials.
The National Institute of Neurological Disorders and Stroke (NINDS) Parkinson’s Disease Biomarkers Program (PDBP).
In November of 2012, NINDS launched a comprehensive PD biomarker initiative that focuses on standardized protocols for biospecimen collection (DNA, RNA, blood, and CSF) and longitudinal clinical assessments (motor/non-motor, neurobehavioral, cognitive, sleep, olfaction, family history and medications) for PD participants across the disease spectrum. A standard set of clinical assessments and biosample collections are made at six- and 12-month intervals. As of May 2014, clinical, genetic and biomarker data from 618 cases and 310 age- and gender-matched healthy controls were broadly available to the research community through the PDPB data management resource (DMR). A subset of participants (290) will also undergo MRI and 150 participants will undergo DTI and fMRI analysis. Unique to the PDBP program is a subset of participants who have been diagnosed with Multisystem Atrophy (50 MSA), Progressive Supranuclear Palsy (50 PSP), and Essential Tremor (30 ET). The overall target enrollment for the PDBP initiative is 1500.
The PDBP DMR is the first database for Parkinson’s disease that brings together disparate types of datasets (clinical, genetic, imaging and biomarker) and biorepository information under one data resource for querying and downloading of data and requesting of related biosamples. Like ADNI and PPMI, the PDBP also shares data internationally with academic and industry investigators. As of May 2014, more than 1000 data downloads and over 1500 biosamples had been requested.
Other potential data sources
Understanding PD progression will require integration of data not just globally, but also from more heterogeneous sources, including asymptomatic cohorts such as PREDICT-PD in the UK [28], EPIPARK in Germany [29], and the Honolulu Aging Study [30]; primary and secondary care datasets (e.g., electronic medical records); and collections of biological specimens. In the UK, the Dementias Platform UK is aggregating data from up to two million participants from existing cohort studies, is establishing a network of access to electronic medical records, and is enhancing UK Biobank with very large programs of neuroimaging and biomarker studies together with outcome data collected from medical records. As the primary purpose of the Dementias Platform UK (DPUK) will be to identify risk factors and biomarkers and to enable and conduct experimental medicine in primary dementia conditions, diseases with dementia as a component such as PD and other neurodegenerative disorders will be within sight of the DPUK objectives. Data from consumer technologies such as smartphones and wearable devices can capture information about motor symptoms, sleep patterns, and other functional behavioral aspects that may reflect early signs of PD. Such data includes that recorded in the John Hopkins pilot study [31], the Oxford OPDC cohort, and as part of UK Biobank. Other datasets from investigations of exposure to pesticides or other environmental riskfactors could also be useful.
DATA TRANSFERABILITY, STANDARDIZATION AND INTEGRATION
While data sharing is widely acknowledged across stakeholders as essential to scientific progress including the development of new treatments for diseases such as PD, technical and cultural roadblocks have limited our ability to exploit the potential of these vast and growing data resources. The main roadblocks discussed were: data transferability, remote data accessibility and privacy/consent issues, data remapping to comprehensive standards, and data integration. Over the past decade, data sharing models have emerged that have begun to break down some of the barriers [16].
• ADNI, a public-private partnership created in 2003 to expedite drug development by standardizing and validating imaging and other biomarkers for Alzheimer’s disease. ADNI provides researchers with open access to raw and processed imaging, clinical, genomic, and biomarker data through the Laboratory of Neuro Imaging (LONI) Imaging Data Archive (IDA) at the University of Southern California. Open access has resulted in seven million data downloads and 500 peer-reviewed articles from researchers around the world [32]. However, with the increasing number of sophisticated imaging protocols and the increased use of genome sequencing, the amount of data collected has increased exponentially, such that it has become impractical to house all data at one site. The Global Alzheimer’s Association Interactive Network (GAAIN) is a federated cloud-enabled platform for sharing and providing access to data analytic tools (www.gaain.org). GAAIN now has many contributors, including ADNI.
Lessons from ADNI relevant to the PD initiative include the need to define the key scientific questions to be answered, standardize data collection protocols, build tools that are sophisticated and matched to the data collected, maintain open communication with the research community about their needs to predict future needs; and anticipate what informatics tools/resources will be needed to address new research questions. Several platforms and networks recently established or in development are positioned to address some of these issues:
• The MRC Dementias Platform UK (DPUK) plans to bring together 22 cohorts from across the UK, including the UK Biobank, integrated into a single informatics platform. DPUK also plans to develop a readiness cohort with baseline imaging data as well as amyloid, genetics discovery, and -omics discovery cohorts.
• The European Medical Information Framework (EMIF) is a five-year IMI with 56 partners in 14 European countries, established to make datasets visible to researchers, to integrate research cohorts for combined analysis, and to enable re-use of medical and other data for research. IMI-EMIF is establishing three broad approaches to data reutilizing and sharing. Firstly, to make data visible and potentially utilizable by researchers, it has established a browser for meta-data or descriptions of data types and rules of engagement across cohorts with up to 50,000 or more participants in Europe. Secondly, the program has begun to harmonize data across diverse cohorts to generate meta-cohorts for combined analysis. Thirdly, EMIF is establishing safe and secure platforms, respecting local legislative, ethical and other data-governance models, which enable networked or distributed analysis of very large datasets including a total of more than 50 million persons. The primary datasets include deliberately different types of studies and data sources to establish scalable solutions for data access and analysis. These data sources include made-for-purpose, ADNI-like biomarker studies such as AddNeuroMed and DESCRIPA, large population-based epidemiological cohorts now re-purposed or enhanced for neurodegenerative or metabolic disease purposes, and very large electronic medical records databases such as The Health Improvement Network (THIN), a UK dataset covering a total of nearly four million active patients from more than 500 primary care physicians.
• Sage Bionetworks, a nonprofit biomedical research organization, aims to create globally integrated, open-source systems that will enable investigators to leverage multi-dimensional data to distill meaningful information related to human health and disease. Sage is a partner in the Common Mind Consortium, which generates disease models for neuropsychiatric disease by bringing together large, well curated brain samples and data management and analysis expertise to enable integrated analysis of molecular, genomic, and disease data. In partnership with the Global CEO Initiative on Alzheimer’s disease, Sage has also launched big data challenges to the AD research community, seeking to leverage open source biomarker, cognitive, genetic, and demographic data from ADNI, Rush University Medical Center, and the European AddNeuroMed study to create a roadmap of AD predictive biomarkers. In the PD arena, Sage is partnering with the patient network PatientsLikeMe (http://www.patientslikeme.com) to combine patient-reported information with data collected from the phone-based voice recordings. The goal is to identify PD-related voice impairments that may be useful in tracking disease progression and response to therapy.
Integrating data across diverse domains and data generators requires the application of commonly used data standards. In support of the PDBP, NINDS developed a set of common data elements (CDEs) in 2010 for use in the PDBP data repository, along with a data dictionary and analytic tools, to enable data comparison and meta-analyses across studies. NINDS plans to capture legacy data from the Morris K. Udall Centers of Excellence for Parkinson’s Disease and NINDS-sponsored PD clinical trials.
C-Path and NINDS worked with industry partners and the Clinical Data Interchange Standards Consortium (CDISC, www.cdisc.org) to transform these CDEs into global standards for collection of PD clinical trials data. The FDA has recognized that CDISC standards will be required, beginning in 2017, for regulatory submissions to facilitate efficient review of medical products [33]. The CDISC standards will be updated as new concepts or information important to PD drug development are identified.
BARRIERS TO DATA SHARING AND INTEGRATION (SEE TABLE 2)
Some of the challenges to data sharing and integration include lack of patient consent, diversity of data formats in legacy data, legal concerns, patient privacy issues, protecting the interests of junior researchers and PhD students who have contributed to the data collection, given the current academic reward systems that may not reward collaboration and sharing of data, and possible conflict between individual and consortium study group goals and achievements [6]. However, data sharing between study groups has become routine in large scale genetics studies, e.g., the Structural Genomics Consortium, a public-private partnership that supports the discovery of new medicines through open access research (http://www.thesgc.org). Different data-sharing models have been successfully pursued, e.g., a common data repository with controlled access or collaborative access with specific summary data being released by specific study investigators that are then incorporated into a meta-analytical framework. It is important to differentiate between studies that have been designed from their outset to be open-access and for which participants were clearly informed, and those that are led by a specific academic group or company with the potential for external collaboration. An additional consideration is whether studies are historical and no further follow-up is envisaged (closed) or whether data are from prospective cohorts with future collection rounds where it is essential that no actions are taken that could harm future cohort retention (open).
A survey of Parkinson’s UK members reported that the vast majority of people with PD were eager for their data to be accessed and made available to advance research and therapeutic development. Barriers such as informed consent will be addressed in diverse fora e.g., universal Institutional Review Board (IRB) for neurodegeneration, Sage Bionetworks portable consent. Focused attention on issues of data sharing has been the subject of numerous fora. This is clearly an evolving concern in research, industry and regulatorysettings.
QUANTITATIVE DRUG DEVELOPMENT PLATFORMS ENABLE MODELING OF DISEASE PROGRESSION
There is growing recognition of the heterogeneity of PD based on advances in genetics, biomarkers, pathology and diversity of clinical phenotypes. A quantitative, data-driven understanding of PD progression is key to advancing a personalized medicine approach to successful treatments. Modeling multiple sources of variability in heterogeneous populations could provide a valuable platform to support improved clinical trials, including developing enrichment strategies and simulating different trial designs. Indeed, modeling and simulation tools have been endorsed by the FDA as a means of assessing the value of different trial designs to detect disease modifying effects of treatments in early stage PD [34].
CAMD developed and gained regulatory insight and endorsement from both FDA and EMA for such a model-based drug development tool for AD [9]. The CAMD AD clinical trial simulation tool was designed to understand and optimize clinical trial design for mild to moderate AD based on a disease-drug model that incorporates a quantitative understanding of disease progression, drug effects, dropout rates, placebo rates, and sources of variability [9]. Data used to develop this tool came from patient-level data from clinical trials and observational studies, and summary-level data from the literature. The CAMD AD clinical trial database consists of placebo arm data from 6500 AD patients from global clinical trials and can be accessed by researchers for broad applications [33]. Regulatory endorsement of the AD clinical trial simulation tool [35] demonstrates regulators’ support for such tools to optimize trial design.
From a regulatory perspective, data sharing is important for transparency, reproducibility, and identification of new information via analysis with the purpose of answering broad ranging drug development questions. FDA recognizes that analysis of multiple clinical and/or pre-clinical data sets provides an opportunity to advance drug development [36]. EMA and FDA are aiming to align on regulatory processes and guidance, where possible. At present, there is no regulatory-defined concept of prodromal PD as a target population for drug approvals yet data in the future may impact this path, as has been the case with AD.
PROPOSED ROADMAP FOR A MODEL-BASED CLINICAL TRIAL ENRICHMENT PLATFORM FOR EARLY PD
Personalized medicine strategies enable treatments that target the right therapy to the right patient at the right time. Enriching trials for subjects likely to show clinical benefit has been endorsed by both the FDA and EMA as a means of increasing the efficiency of those trials [37, 38]. To date, trials in PD have typically recruited all subjects that meet historically defined diagnostic criteria for the disease. Yet at present, diagnostic criteria for PD are being redefined [24, 39, 40] and there is an urgent need to identify subsets of PD patients with defined disease trajectories. Regulatory endorsement of modeling and simulation tools in a defined context of use, serves to de-risk drug development in design of trials and streamline the review of new drug candidates through a regulatory endorsed model that applies across therapeutic targets and can be utilized by multiple sponsors. Figure 1 illustrates how available data might be applied to the development of a model-based clinical trial enrichment strategy with regulatory focus. Observational data from both the literature and at least seven individual datasets, including biomarker data from several cohort studies (OPDC, ProBaND, CamPaIGN, and PPMI) and clinical trials will be used to model disease progression similarly to the way ADNI and other data were used to build and then confirm a hypothetical model of disease progression in AD [9, 41, 42]. Success of the proposed PD regulatory pathway will require the implementation of PD data standards and the construction of a data sharing mechanism of an integrated database to effectively utilize those data. The model will enable simulation of PD progression trajectories of different subpopulations, thus indicating particular endophenotypes, which can then be used to optimize entry criteria for clinical trials, improve the statistical power and increase chances of success. The proposed roadmap follows a path to enable regulatory decisions with broad application to clinical trials. Furthermore, enhanced data-sharing will catalyze novel discoveries in PD research.
CONCLUSIONS
The need for a global database that integrates large amounts of diverse data was identified as essential to fuel progress in identifying indicators of pre-clinical and early motor PD, which would enable the development of pre-symptomatic disease modifying treatments and improved symptomatic treatments.
The workshop aligned on interim steps toward the eventual goal of building and achieving consensus on precompetitive data sharing as a catalyst to advancing research for PD. One proposal is the regulatory endorsement of new drug development tools, including a clinical trial enrichment platform and a trial modeling and simulation tool. This will require:
• Cataloging existing clinical and observational datasets and the types of data within those datasets that are relevant to the research question(s) being posed.
• Developing and applying data standards that will enable integration of data across multiple datasets, including novel types of data such as that collected from remote monitoring devices andbiosensors.
• Maximizing the use of existing data by establishing acceptable guidelines for data sharing.
• Supporting the further development of new biomarkers and assessment tools that will provide a better understanding of the phenotypic variations of PD.
• Promote the implementation of new technologies such as wearable devices into PD for enabling personalized medicine.
• Promoting further collaboration across all stakeholders.
ACKNOWLEDGMENTS
The authors thank the following colleagues for their participation and contributions to the success of the meeting: Sue Dubman (UCSF), Karl Kieburtz (Univ Rochester), Madeline Kelly (GSK), Katie Le Blond, Arthur Roach, and Bunia Gorelick (Parkinson’s UK), Vincent Low (UCB), Annette Schrag (UCL), Richard Moscicki (FDA), Jan Johannessen (FDA), Nancy B Sager (FDA), and Vikram Sinha (FDA).
Disclaimer: The views expressed in this article are those of the authors and do not necessarily reflect the official views of FDA, NOR of NINDS. The manuscript meets all requirements for authorship as per The International Committee of Medical Journal Editors (ICMJE) guidelines. The roadmap outlined in Fig. 1 and the summary of workshop is a consensual agreement of the authors.
REFERENCES
1 | Dorsey ER, Constantinescu R, Thompson JP, Biglan KM, Holloway RG, Kieburtz K, Marshall FJ, Ravina BM, Schifitto G, Siderowf A, Tanner CM(2007) Projected number of people with Parkinsondisease in the most populous nations, 2005 through 2030Neurology68: 384386 |
2 | Van Den Eeden SK, Tanner CM, Bernstein AL, Fross RD, Leimpeter A, Bloch DA, Nelson LM(2003) Incidence of Parkinson’s disease: Variation by age, gender, and race/ethnicityAm J Epidemiol157: 10151022 |
3 | Breen KC, Drutyte G(2013) Non-motor symptoms of Parkinson’s disease: The patient’s perspectiveJ Neural Transm120: 531535 |
4 | Jankovic J(2005) Motor fluctuations and dyskinesias in Parkinson’s disease: Clinical manifestationsMov Disord20: Suppl 11S11S16 |
5 | Marek K, Seibyl J, Eberly S, Oakes D, Shoulson I, Lang AE, Hyson C, Jennings DParkinson Study Group PRECEPT, Investigators(2014) Longitudinal follow-up of SWEDD subjects in the PRECEPT StudyNeurology Ahead of Print Apri23: 2014 |
6 | Institute of Medicine(2013) Sharing Clinical Research Data: Workshop summaryWashington, D.C.The National Academies Press |
7 | Atassi N, Berry J, Shui A, Zach N, Sherman A, Sinani E, Walker J, Katsovskiy I, Schoenfeld D, Cudkowicz M, Leitner M(2014) ThePRO-ACT database: Design, initial analyses, and predictivefeaturesNeurology83: 17191725 |
8 | Kuffner R, Zach N, Norel R, Hawe J, Schoenfeld D, Wang L, Li G, Fang L, Mackey L, Hardiman O, Cudkowicz M, Sherman A, Ertaylan G, Grosse-Wentrup M, Hothorn T, van Ligtenberg J, Macke JH, Meyer T, Scholkopf B, Tran L, Vaughan R, Stolovitzky G, Leitner ML(2015) Crowdsourced analysis of clinical trial data to predictamyotrophic lateral sclerosis progressionNat Biotechnol33: 5157 |
9 | Romero K, Ito K, Rogers JA, Polhamus D, Qiu R, Stephenson D, Mohs R, Lalonde R, Sinha V, Wang Y, Brown D, Isaac M, Vamvakas S, Hemmings R, Pani L, Bain LJ, Corrigan BW(2014) The future is now: Model-based clinical trial design forAlzheimer’s diseaseClin Pharmacol Ther97: 3210214 |
10 | Rudick RA, Larocca N, Hudson LD(2013) Multiple SclerosisOutcome Assessments Consortium: Genesis and initial project planMult Scler20: 1217 |
11 | Woodcock J, Brumfield M, Gill D, Zerhouni E(2014) The drivingrole of consortia on the critical path to innovative therapiesNat Rev Drug Discov13: 781 |
12 | Goldman M(2012) The innovative medicines initiative: A Europeanresponse to the innovation challengeClin Pharmacol Ther91: 418425 |
13 | Goldman M(2013) New frontiers for collaborative research. 216edSci Transl Med5: 222 |
14 | Goldman M, Compton C, Mittleman BB(2013) Public-private partnerships as driving forces in the quest for innovative medicinesClin Transl Med2: 2 |
15 | Sieber BA, Landis S, Koroshetz W, Bateman R, Siderowf A, Galpern WR, Dunlop J, Finkbeiner S, Sutherland M, Wang H, Lee VM, Orr HT, Gwinn K, Ludwig K, Taylor A, Torborg C, Montine TJParkinson’s Disease: Advancing Research ILCOC(2014) Prioritized research recommendations from the National Institute of Neurological Disorders and Stroke Parkinson’s Disease 2014 conferenceAnn Neurol76: 469472 |
16 | Wilhelm EE, Oster E, Shoulson I(2014) Approaches and costs for sharing clinical research dataJAMA311: 12011202 |
17 | The Parkinson Study Group PRECEPT, Investigators(2007) Multiple lineage kinase inhibitor CEP-1347 fails to delaydisability in early Parkinson diseaseNeurology69: 14801490 |
18 | Olanow CW, Rascol O, Hauser R, Feigin PD, Jankovic J, Lang A, Langston W, Melamed E, Poewe W, Stocchi F, Tolosa EInvestigators AS(2009) A double-blind, delayed-start trial of rasagiline in Parkinson’s diseaseN Engl J Med361: 12681278 |
19 | Szewczyk-Krolikowski K, Tomlinson P, Nithi K, Wade-Martins R, Talbot K, Ben-Shlomo Y, Hu MT(2014) The influence of age and gender on motor and non-motor features of early Parkinson’s disease: Initial findings from the Oxford Parkinson Disease Center (OPDC) discovery cohortParkinsonism Relat Disord20: 99105 |
20 | Rolinski M, Szewczyk-Krolikowski K, Tomlinson PR, Nithi K, TalbotK , Ben-Shlomo Y, Hu MT(2014) REM sleep behaviour disorder isassociated with worse quality of life and other non-motor featuresin early Parkinson’s diseaseJ Neurol Neurosurg Psychiatry85: 560566 |
21 | Szewczyk-Krolikowski K, Menke RA, Rolinski M, Duff E, Salimi-Khorshidi G, Filippini N, Zamboni G, Hu MT, Mackay CE(2014) Functional connectivity in the basal ganglia network differentiates PD patients from controlsNeurology83: 208214 |
22 | Foltynie T, Brayne CE, Robbins TW, Barker RA(2004) The cognitive ability of an incident cohort of Parkinson’s patients in the UK. The CamPaIGN studyBrain127: 550560 |
23 | Williams-Gray CH, Mason SL, Evans JR, Foltynie T, Brayne C, Robbins TW, Barker RA(2013) The CamPaIGN study of Parkinson’s disease: 10-year outlook in an incident population-based cohortJ Neurol Neurosurg Psychiatry84: 12581264 |
24 | Goetz CG, Tilley BC, Shaftman SR, Stebbins GT, Fahn S, Martinez-Martin P, Poewe W, Sampaio C, Stern MB, Dodel R, Dubois B, Holloway R, Jankovic J, Kulisevsky J, Lang AE, Lees A, Leurgans S, LeWitt PA, Nyenhuis D, Olanow CW, Rascol O, Schrag A, Teresi JA, van Hilten JJ, LaPelle NMovement Disorder Society URTF(2008) Movement Disorder Society-sponsored revision of the Unified Parkinson’s Disease Rating Scale (MDS-UPDRS): Scale presentation and clinimetric testing resultsMov Disord23: 21292170 |
25 | Mioshi E, Dawson K, Mitchell J, Arnold R, Hodges JR(2006) The Addenbrooke’s Cognitive Examination Revised (ACE-R): A brief cognitive test battery for dementia screeningInt J Geriatr Psychiatry21: 10781085 |
26 | Yarnall AJ, Breen DP, Duncan GW, Khoo TK, Coleman SY, Firbank MJ, Nombela C, Winder-Rhodes S, Evans JR, Rowe JB, Mollenhauer B, Kruse N, Hudson G, Chinnery PF, O’Brien JT, Robbins TW, Wesnes K, Brooks DJ, Barker RA, Burn DJGroup I-PS(2014) Characterizing mild cognitive impairment in incident Parkinson disease: The ICICLE-PD studyNeurology82: 308316 |
27 | Schneider SA, Edwards MJ, Mir P, Cordivari C, Hooker J, Dickson J, Quinn N, Bhatia KP(2007) Patients with adult-onset dystonic tremor resembling parkinsonian tremor have scans without evidence of dopaminergic deficit (SWEDDs)Mov Disord22: 22102215 |
28 | Noyce AJ, Bestwick JP, Silveira-Moriyama L, Hawkes CH, Knowles CH, Hardy J, Giovannoni G, Nageshwaran S, Osborne C, Lees AJ, Schrag A(2014) PREDICT-PD: Identifying risk of Parkinson’s disease in the community: Methods and baseline resultsJ Neurol Neurosurg Psychiatry85: 3137 |
29 | Kasten M, Hagenah J, Graf J, Lorwin A, Vollstedt EJ, Peters E, Katalinic A, Raspe H, Klein C(2013) Cohort Profile: A population-based cohort to study non-motor symptoms in parkinsonism (EPIPARK)Int J Epidemiol42: 128-128k |
30 | Gelber RP, Launer LJ, White LR(2012) The Honolulu-Asia Aging Study: Epidemiologic and neuropathologic research on cognitive impairmentCurr Alzheimer Res9: 664672 |
31 | Arora S, Little MA, Venkataraman V, Donohue S, Biglan K, Dorsey ER(2013) High accuracy discrimination of Parkinson’s disease participants from healthy controls using smarphonesMov Disord28: e12 |
32 | Alzheimer’s Disease Neuroimaging Initiative, ADNI, http://adni.loni.usc.edu/ |
33 | Neville J, Kopko S, Broadbent S, Aviles E, Stafford R, Solinsky C, Bain LJ, Cisneroz M, Romero K, Stephenson D(2015) Development of a unified clinical database for Alzheimer’s diseaseAlzheimers Dement110Feb 9 |
34 | Bhattaram VA, Siddiqui O, Kapcala LP, Gobburu JV(2009) Endpoints and analyses to discern disease-modifying drug effects in early Parkinson’s diseaseAAPS J11: 456464 |
35 | European Medicines Agency. Draft qualification opinion of a novel data-driven model of disease progression and trial evaluation in mild and moderate Alzheimer’s disease. http://www.ema.europe.eu/docs/en_GB/document_library/Regulatory_and_procedural_guideline/2013/10/WC500151309.pdf |
36 | Woodcock J(2014) Paving the critical path of drug development: The CDER perspectiveNat Rev Drug Discov13: 783784 |
37 | European Medicine Agency. Qualification Opinion of Alzheimer’s Disease Novel Methodologies/biomarkers for BMS-708163. EMA/CHMP/SAWP/102001/2011. Accessed online 11-7-11 athttp://www.ema.europa.eu/docs/en_GB/document_library/Regulatory_and_procedural_guideline/2011/02/WC500102018.pdf |
38 | Food and Drug Administration (2012) accessed online 7/18/2013 at http://www.fda.gov/downloads/Drugs/GuidanceComplianceRegulatoryInformation/Guidances/UCM332181.pdf |
39 | Berardelli A, Wenning GK, Antonini A, Berg D, Bloem BR, Bonifati V, Brooks D, Burn DJ, Colosimo C, Fanciulli A, Ferreira J, Gasser T, Grandas F, Kanovsky P, Kostic V, Kulisevsky J, Oertel W, Poewe W, Reese JP, Relja M, Ruzicka E, Schrag A, Seppi K, Taba P, Vidailhet M(2013) EFNS/MDS-ES/ENS [corrected] recommendations for the diagnosis of Parkinson’s diseaseEurJ Neurol20: 1634 |
40 | Litvan I, Goldman JG, Troster AI, Schmand BA, Weintraub D, Petersen RC, Mollenhauer B, Adler CH, Marder K, Williams-Gray CH, Aarsland D, Kulisevsky J, Rodriguez-Oroz MC, Burn DJ, Barker RA, Emre M(2012) Diagnostic criteria for mild cognitive impairment in Parkinson’s disease: Movement Disorder Society Task Force guidelinesMov Disord27: 349356 |
41 | Jack CRJr, Knopman DS, Jagust WJ, Shaw LM, Aisen PS, Weiner MW, Petersen RC, Trojanowski JQ(2010) Hypothetical model of dynamic biomarkers of the Alzheimer’s pathological cascadeLancet Neurol9: 119128 |
42 | Rogers JA, Polhamus D, Gillespie WR, Ito K, Romero K, Qiu R, Stephenson D, Gastonguay MR, Corrigan B(2012) Combining patient-level and summary-level data for Alzheimer’s disease modeling and simulation: A beta regression meta-analysisJ Pharmacokinet Pharmacodyn39: 479498 |
Figures and Tables
Fig.1
Proposed Roadmap for building PD drug development tools with existing data. Proposed roadmap outlining a potential future path for integrating global data from PD observational and clinical trials targeting early stages. Integration of diverse data from at least seven independent clinical studies into a unified database will enable a regulatory path for use of biomarkers and quantitative disease progression models that serve to streamline and derisk drug development of new therapies.
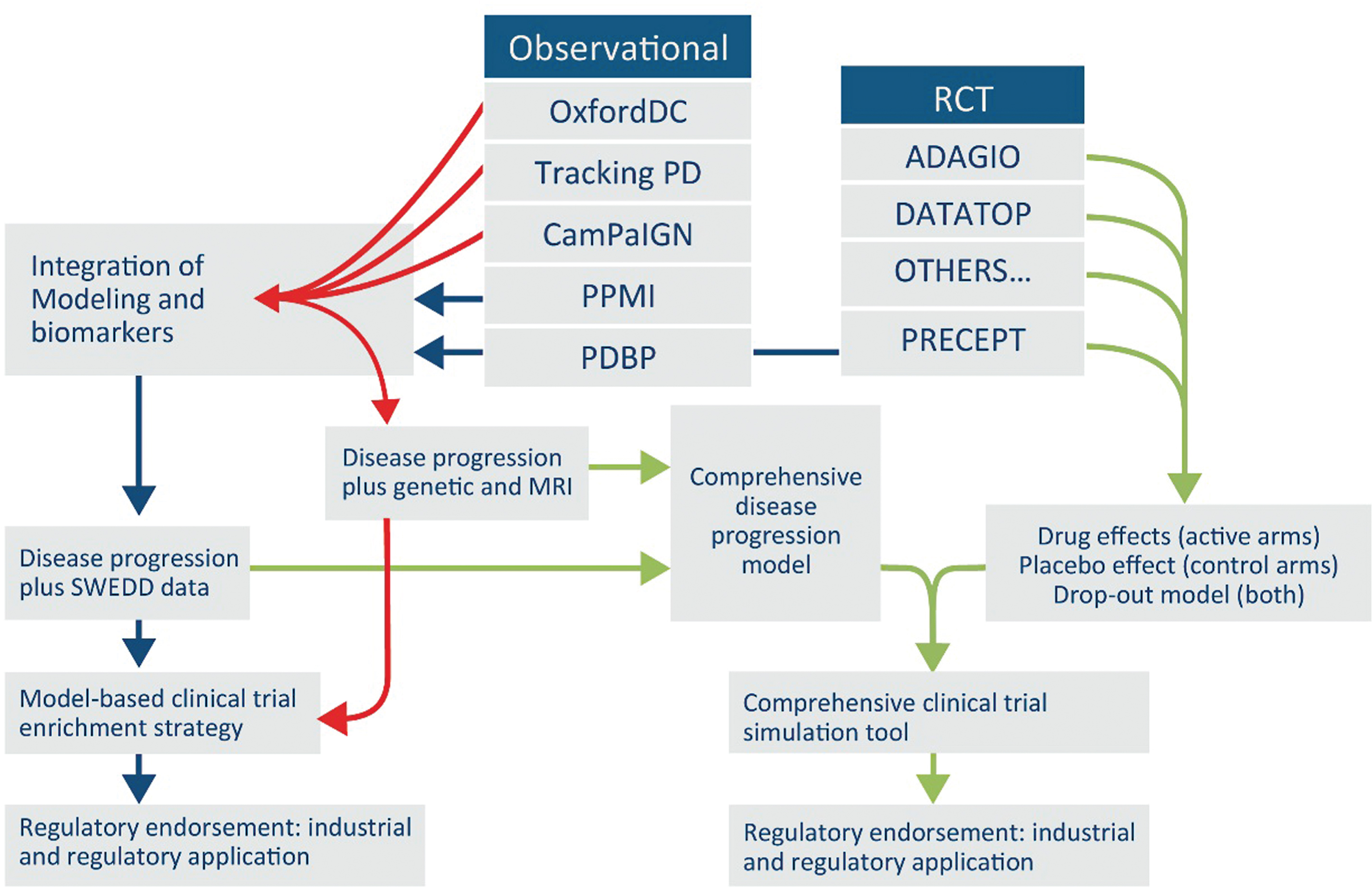
Table 1
Sources of Parkinson’s disease clinical data for integration and future analyses
Study | Type of study | Number of patients | Duration of study (if longitudinal) | Reason for cohort (drugtrial/cohort study/other) | Study ongoing (yes/no) | Assessments | Tissue sample available (serum, plasma, CSF etc.) | Genotyped | Scanning (MRI, PET etc.) | Other |
ICICLE | Longitudinal (predicting dementia) | 160 | 8 years | Predictingdementia | Yes | UPDRS, motor, non-motor, cognitivedecline | Serum, CSF,DNA, RNA | Yes | MRI baseline &18mo &FDGPET in ∼45 | Gait &sleepdata |
CamPaIGN | Longitudinal(from time of diagnosis) | 142 (diagnosed between 2000– 2002) | 13– 15 years | Community-based incidencecohort | Yes | UPDRS, motor, non-motor, cognitivedecline | No | Yes (n = 129)(MAPT H1vs H2, COMT val(158)met, SNCA, APOE, MAOA), DNA stored | No | Neuropsychologic, mood, function, quality of life |
PICNICS | Longitudinal(from time of diagnosis) | 286 (diagnosedDec 2007– June 2013) | 2– 7 years | Community-basedcohort study | Yes | UPDRS, motor, non-motor, cognitivedecline | Plasma andserum (n = 98), CSF (n = 11) | Yes (n = 276)(MAPT H1vs H2, COMT Val158Met, SNCA, BuChE, ApoE), DNA stored | Yes (n = 48) | Neuropsychologic, mood, function, quality of life |
PRoBaND | Longitudinal(from time of diagnosisfor PD) | 3000 (2000patients within3 yrs of diagnosis, 240 young onsetand 760 relatives) | 3– 5 years | Community-basedcohort study | Yes | UPDRS, motor, non-motor, cognitivedecline | Serum | Yes, LRRK2 and GBA (all subjects) and Parkin and PINK1 (young onset) | Sub-study in4-5 centres | Olfactory function, Sleep, Autonomic function, Quality of life, Environmental exposures |
OPDCDiscoverycohort | Longitudinal(within 3years ofdiagnosis) | 1650 (1100 PD patients within3 yrs of diagnosis; 100 PD relative early stage; 150 prodromal RBD; 300 control | 10 years | Community-basedcohort study | Yes | UPDRS I-IV,motor, non-motor, cognitive decline | Serum and DNSin all. Plasma, CSF, G.I biopsy tissue, skin in subgroup | Yes (n = 869) SNP analysis, DNA stored. 250 whole exome analysis. | MRI (structuraland functional) in 80 PD, 30 controls,25 RBDsubjects | Olfactory function, Objective motor testing (android phone app test, saccadometry) |
TEVA-PRECEPT | Longitudinal | 806 early PD | Terminatedearly (averageof 21.4 months follow-up) | Clinical trial | No | UPDRS,cognition, depression,quality of life | No | No | Beta-CIT SPECT imaging | |
TEVA-ADAGIO | Longitudinal | 1176 early PD | 72 weeks | Delayed startclinical trial | No | UPDRS | No | No | Beta-CIT SPECT imaging | Rasagiline as a disease-modifyingtherapy in PD |
PostCept (and LABS-PD) | Longitudinal | 709 subjectsfrom PRECEPT enrolled into PostCEPT and LABS-PD | Ongoing since2008 | Population-basedstudy | Yes | UPDRS, qualityof life,cognition | Serum, blood biomarkers(alpha-synuclein, proteomics) | yes (DNAbanking) | Beta-CIT SPECT imaging, DAT imaging | PostCEPT rolledinto LABS-PD |
(Continued) | ||||||||||
Parkinson Progression Marker Initiative (PPMI) Biomarker Study | Longitudinal (from time of diagnosis) | 400 newly diagnosed PD, 200 controls, 64 SWEDD, 100 prodromal, 600 genetic registry participants | Ongoing since 2010 | Community-based cohort study | Yes | UPDRS-III,motor, non-motor, cognitive decline MDS-UPDRS | DNA, RNA, serum, plasma, urine, CSF | Yes (ApoE and selected SNPs) | MRI, DAT, PET ([18F] florbetaben) CT (some sites) | |
DATATOP | Longitudinal | 800 | 8 years | Clinical trial | No | UPDRS, cognition, depression, quality of life | Serum, urine,CSF, DNA | Yes by requesting for access to biospecimen repository | No | Video repository |
CALM-PD | Longitudinal | 301 | 2– 4 years | Clinical trial | No | dopaminergicmotor complication, UPDRS, quality of life, MMSE | No | No | Beta-CIT SPECT imaging | Health care utilization |
TEMPO | Longitudinal | 404 (early PD) | 1 year | Clinical trial | No | UPDRS, qualityof life, MMSE, depression | No | No | No | Rasagiline pharmacokinetics, platelet MAO-B activity |
ELLDOPA | Longitudinal | 361 | 42– 44 weeks | Clinical trial | No | UPDRS, qualityof life, MMSE, Hamilton depression scale | No | No | Beta-CIT Spect imaging (select subjects) | Video repository |
PRESTO | Longitudinal | 472 (advancedPD) | 6 months | Clinical trial | No | UPDRS, “on-off” diaries, quality of life, MMSE | No | No | No | Rasagiline pharmacokinetics, platelet MAO-B activity |
The National Institute of Neurological Disorders and Stroke (NINDS) Parkinson’s Disease Biomarker Program(PDBP) | 439 Cross-sectional,825 Longitudinal(3– 5 years) | 748 PD, 386 control, 50 Multisystem Atrophy, 50 Progressive Supranuclear Palsy, 30 Essential Tremor | 3-5 years | Community-basedcohort study | Yes | MDS-UPDRS,motor, non-motor, cognitivedecline | CSF (269), plasma (674), serum (775), RNA (1,234), DNA (1,191) | Yes, NeuroXchip | MRI (290), DTI (440), fMRI (150) | Gait (120), biosample QC(hemoglobin analysis for plasma, serumand CSF), qualityof life |
The studies in this table represent the candidate PDclinical studies that were described at the PD data sharingconsensus conference as potential sources of data forstandardization, integration and future analyses by principleinvestigators and meeting participants. This is not acomprehensive list of all possible PD studies yet provided aframework for the stakeholders and potentialroadmap (Fig. 1).
Table 2
Issues and potential paths to enable data sharing in PD
Issues/challenges | Possible Solutions |
Different formats of data | Implementation of data standards |
Country focused initiatives at present | Implementation of global PPPs and consortia |
Regulatory landscape– need for biomarkers | Regulatory endorsement of drug development tools |
Need for reliable longitudinal data | Funding streams for high quality observational studies |
Approval to access varied patient level datasets | Data sharing initiatives through global PPPs and consortia |
Cost for establishing and especially | Business case for funding streams from government, |
maintaining global database | non-profit and private sectors |
Privacy protection | Adherence to patient privacy regulations and de-identification of patient-level data |
Patient consent for sharing | Implementation of broad informed consent documents in line with national guidelines |
Incentives for data contributors | Immediate access to integrated databases to further research |
Recognition for data contributors | Coauthorship and widespread dissemination |
Data access and sharing | Publication strategy and dissemination mechanism |
Infrastructure needed for future sustainability | Self sustained consortia based models and infrastructure |
Alignment across consortia | Focus on synergistic research areas and regulatory alignment |
Define incentives for industry | Derisking of drug development programs through impact on regulatory science |
Improved drug safety | Reporting and monitoring of drug adverse effects |
Impact on patients and families | Rewarding in advancing the cause for all, altruistic to others and for self |
Young investigators to benefit | Accelerate pathway for advanced degrees and training |