Closed-Loop Adaptive Deep Brain Stimulation in Parkinson’s Disease: Procedures to Achieve It and Future Perspectives
Abstract
Parkinson’s disease (PD) is a neurodegenerative disease with a heavy burden on patients, families, and society. Deep brain stimulation (DBS) can improve the symptoms of PD patients for whom medication is insufficient. However, current open-loop uninterrupted conventional DBS (cDBS) has inherent limitations, such as adverse effects, rapid battery consumption, and a need for frequent parameter adjustment. To overcome these shortcomings, adaptive DBS (aDBS) was proposed to provide responsive optimized stimulation for PD. This topic has attracted scientific interest, and a growing body of preclinical and clinical evidence has shown its benefits. However, both achievements and challenges have emerged in this novel field. To date, only limited reviews comprehensively analyzed the full framework and procedures for aDBS implementation. Herein, we review current preclinical and clinical data on aDBS for PD to discuss the full procedures for its achievement and to provide future perspectives on this treatment.
INTRODUCTION
Parkinson’s disease (PD) is the second most common neurodegenerative disease [1, 2]. In 2019, the global PD prevalence was estimated at over 8.5 million individuals, and 106.3 per 100,000 people had been affected with PD [3]. Moreover, the incidence and prevalence of the disease as well as disability and death due to PD rise even more rapidly than for other neurological disorders, which places a heavy burden on patients, families, and society [3, 4]. Based on its main neuropathology, which is the loss of dopaminergic neurons and depletion of dopamine in the nigrostriatal pathway [5, 6], PD is initially managed with dopaminergic medications [7]. However, as the disease progresses, many PD patients have a poor quality of life because of fluctuations in medication response, levodopa-unresponsive tremor, and medication-related complications (such as levodopa-induced dyskinesia) [7, 8]. For these patients, deep brain stimulation (DBS) can provide better improvement in function and quality of life [9].
As a minimally invasive procedure, DBS shows high effectiveness and safety for several neurological diseases [10, 11]. Concerning PD, multiple clinical studies have demonstrated that DBS was more effective than best medical therapy for moderate-to-severe PD [9] even after a 10-year follow-up and was accompanied by limited adverse events [12], suggesting its acceptable long-term benefits and safety. Most previous studies have applied open-loop conventional DBS (cDBS) for PD, which delivers continuous, uninterrupted stimulations with constant amplitude, frequency, and pulse width and shape, regardless of the patient’s functional status or needs [13, 14]. However, the design of cDBS has inherent limitations, including various stimulation-induced adverse effects, such as dysarthria and imbalance [12, 15, 16], increased battery consumption [13, 14], and frequent parameter adjustments during follow-up to address symptom changes [17].
To overcome these shortcomings of cDBS, the strategy of closed-loop adaptive DBS (aDBS) has been proposed [18–23]; scientific interest in this strategy has increased in recent years [11, 24, 25]. For this design, aDBS recognizes input signals such as brain or motor signals that correlate to a patient’s changed symptoms to provide responsive optimized stimulation in real time [13, 14]. In theory, this on-demand aDBS design can improve therapeutic efficacy and generate fewer side effects with increased battery longevity [13, 14, 26]. Encouragingly, several animal models [21, 27] and clinical studies of aDBS have shown its benefits [28–31]. This topic has attracted scientific interest while both achievements and challenges appear [32]. Although several previous reviews discussed different aspects of aDBS for various neurological disorders [11, 14, 24, 26, 33–40], few of them analyzed the full framework to achieve aDBS for PD [14, 26, 38]; the literature still needs a comprehensive, up-to-date summary of advances in the detailed procedures for aDBS. Herein, we review current preclinical and clinical data on aDBS for PD to discuss a full framework for its implementation in terms of its optimal phenotypes, targets, and patients; its input signals; and its adaptive strategy and stimulation parameters; we also aim to provide a comprehensive review of its implementation procedures, directions of development, and future perspectives and ultimately to promote its future development for the practical benefit of PD patients.
PROCEDURES TO ACHIEVE aDBS FOR PD
Overall, to achieve aDBS for PD, the first step is to identify optimal symptoms, targets, and PD patients. After that, the aDBS is designed with three main modules for sensing, control, and stimulation with associated functions of detecting input signals that reflect symptoms, determining adaptive strategy and adjusting parameters according to the input signal, and providing stimulation, respectively [13]. These procedures are discussed in the following sections, and a diagrammatic sketch of the implementation is shown in Fig. 1.
Fig. 1
A diagrammatic sketch of the current adaptive deep brain stimulation (aDBS) design for Parkinson’s disease (PD). No. 1: input signals as cortical signals; No. 2: input signals as subcortical signals; No. 3: input signals as surface electromyography (sEMG) and other wearable devices; No. 4: input signals as future potential signals; lines in blue show the signals line and lines in yellow show the stimulation line; the figure shows three main modules for sensing, control, and stimulation with the function of detecting input signals reflecting symptoms, processing signals and selecting adaptive strategy and adjusting parameters, and providing stimulation, respectively.
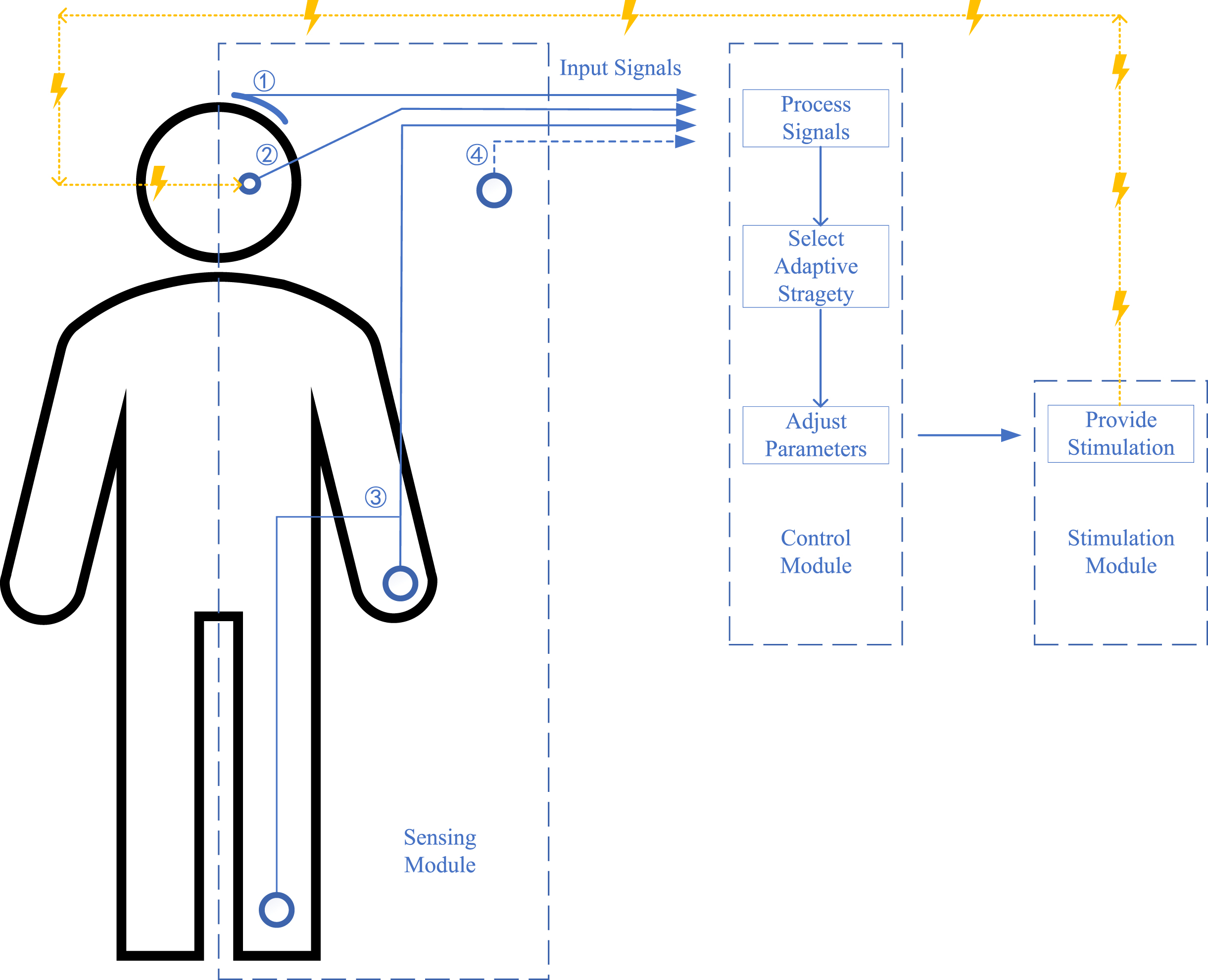
Optimal symptoms, targets, and PD patients
Essentially, aDBS only changes the way it delivers stimulation to make it more appropriately correlated with the clinical condition of the patient [13], which means its main indications do not overstep the boundaries of cDBS [41]. Thus, the general eligibility principle for aDBS candidates should be consistent with cDBS in theory [42]. However, available studies and devices on aDBS for PD are still limited, especially on its input signals for specific symptoms, which means aDBS only covers several optimal phenotypes, targets, and groups of patients under the current design [14]. A table summarizing current animal models and clinical studies on aDBS in PD is presented as Table 1 to provide more information for readers.
Table 1
Current animal models and clinical studies of adaptive deep brain stimulation (aDBS) in Parkinson’s disease (PD)
Study | Samples | Size (n) | Groups | Stimulation target | Input signals | Adaptive strategy | Stimulation time | Main results |
Animal Models | ||||||||
Rosin et al., 2011 [21] | MPTP Monkeys | 2 | 1 aDBS, 1 cDBS | Unilateral GPi | Gpi and M1 spikes | ON/OFF | Short-term | 1. aDBS suppresses pallidal discharge rate and oscillations and meliorates akinesia; 2. cDBS reduces pallidal discharge rate, oscillations and akinesia but to a lesser degree than aDBS; 3. aDBS has lower stimulus frequency and increased stimulus irregularity than cDBS. |
Johnson et al., 2016 [27] | MPTP Monkey | 1 | Self-control | Unilateral STN | STN LFP (β band power) | ON/OFF | 2–3 minutes | 1. aDBS and cDBS significantly reduced rigidity scores compared to Off-DBS, aDBS (52.6% of the time) significantly lower than cDBS in elbow rigidity score and trended lower in mean total rigidity scores (n.s.); 2. Reaching task improved bradykinesia only during cDBS; peak return speed during aDBS was slower compared to Off-DBS. |
Clinical Studies | ||||||||
Little et al., 2013 [23] | Advanced PD patients with motor fluctuations and/or dyskinesias | 8 | Self-control | Unilateral STN | STN LFP (β band power) | ON/OFF | 5 minutes | 1. Both aDBS (66.2% and 49.7%) and cDBS (54.3% and 30.5%) improved motor scores; the improvement of cDBS was significantly less than aDBS; maintained if excluded rigidity scores; 2. Mean total energy delivered with aDBS was significantly less than that with cDBS. |
Rosa et al., 2015 [48] | A PD patient with dyskinesias, bradykinesia, and slight hypophonia | 1 | Self-control | Bilateral STN | STN LFP (β band power) | Continuous | 100 minutes | 1. aDBS had better control of main symptom of bradykinesia and reduced dyskinesias during gait and at rest over time than cDBS; 2. aDBS and cDBS improved patient’s axial symptoms to a similar extent; 3. aDBS did not elicit side effects and was well tolerated. |
Malekmohammadi et al., 2016 [58] | Tremor-dominant PD patients | 5 | Self-control | Bilateral STN | Wearable watch (4–8 Hz tremor power) | Gradual | 30 minutes | 1. Average tremor during aDBS was significantly lower (36.6%) than average tremor during calibration; 2. Mean aDBS voltage was lower (76.35%) than that used for clinical cDBS and was delivered for only 51.5% duration |
Little, Tripoliti, et al., 2016 [65] | Advanced PD patients with tremor/motor fluctuations/ dystonia/dyskinesias | 8 | Self-control | Bilateral STN | STN LFP (β band power) | ON/OFF | 15 minutes | 1. aDBS delivered 42.6±3.7% of stimulation time; 2. Better speech intelligibility with aDBS (70.4±6.4%) than with cDBS (60.5±8.2%); aDBS was no different to Off-DBS; but cDBS was worse than Off-DBS; 3. significant improvement of motor score on aDBS compared to cDBS (19.7±1.0 vs. 31.6±4.3). |
Little, Beudel, et al., 2016 [28] | Advanced PD patients with tremor/motor fluctuations/dystonia | 4 | Self-control | Bilateral STN | STN LFP (β band power) | ON/OFF | 15 minutes | 1. aDBS had substantial improvement (reduction 43% for motor score, 37±10% and 39±5% for limb bradykinesia and axial symptoms, 55±12% for tremor, speech, facial expression and freezing; no comparison with cDBS); 2. aDBS had 45±4.8% time on stimulation |
Piña-Fuentes et al., 2017 [49] | A PD patient had STN cDBS before | 1 | Self-control | Unilateral STN* | STN LFP (β band power) | ON/OFF | 12 minutes | 1. aDBS was well tolerated and only induced transient contralateral paresthesia in the contralateral hand; 2. aDBS was better in bradykinesia than cDBS and Off-DBS |
Rosa et al., 2017 [64] | PD patients | 10 | 5 aDBS, 5 cDBS | Unilateral STN | STN LFP (β band power) | Continuous | 2 hours | 1. aDBS (–46.1% ±10.5%) and cDBS (–40.1% ±17.5%) had similar improvement on global motor symptoms; 2. aDBS (11.7±67) was more effective on dyskinesias than cDBS (11.7±67 vs. 15.0±8.7); 3. average power saving of 73.6% ±22.9% in aDBS compared with cDBS. |
Arlotti et al., 2018 [46] | Rigid-akinetic advanced PD patients | 11 | Self-control | Unilateral STN** | STN LFP (β band power) | Continuous | 8 hours | 1. aDBS reduced motor scores by 30%, “off” medication; and 45%, “on” medication (no comparison with cDBS); 2. levodopa and aDBS did not elicit dyskinesias, no adverse effects. |
Swann et al., 2018 [31] | PD patients had previous STN cDBS with dyskinesia | 2 | Self-control | Unilateral STN | Cortical ECoG (γ oscillation) | Gradual | 30–62 minutes | 1. Patient 1 had similar bradykinesia and dyskinesia scores for aDBS and cDBS, and Patient 2 had no overt clinical difference; 2. Energy savings of aDBS were substantial (38% –45%). |
Velisar et al., 2019 [29] | 6 akinetic rigid and 7 tremor PD patients had STN cDBS before | 13 | Self-control | Unilateral STN | STN LFP (β band power) | Gradual | Median 21.67 minutes | 1. aDBS improved bradykinesia and tremor more than Off-DBS (no comparison with cDBS); 2. aDBS delivered 56.86% of the total energy than cDBS; 3. Neural classifier and control policy algorithms achieved for aDBS in tremor dominant and akinetic rigid subjects. |
Arlotti et al., 2019 [47] | An akinetic-rigid PD patient had STN cDBS before | 1 | Self-control | Bilateral STN | STN LFP (β band power) | Continuous | 24 hours | 1. aDBS has stable improvement ranging between 30–37%, which was similar to cDBS (36%); 2. No adverse events or complaints by the patient were reported. |
Petrucci et al., 2020 [63] | A PD patient with FoG | 1 | Self-control | Bilateral STN | STN LFP (β band power) | Gradual | 48 hours | 1. FoG percent: 68.7% Off-DBS, 2.3% /23.5% cDBS, 1.5% aDBS; 2. Stepping arrhythmicity: 54.9% Off-DBS, 18.2% /27.4% cDBS, 5.2% aDBS; 3. Energy: aDBS 2% higher than cDBS. |
Molina et al., 2021 [62] | PD patients with FoG | 5 | Self-control | Bilateral PPN*** | PPN LFP (1–8 Hz band power) | ON/OFF | 5–15 months | 1. 40% device explantation rate due to infection, 14 related adverse events, 7 severe; 2. aDBS 3/5 greater than 40% improvement in the number of FoG episode, no significant with cDBS. |
Bocci et al., 2021 [42] | Advanced PD patients | 8 | Self-control | Unilateral STN | STN LFP (β band power) | Continuous | 8 hours | 1. Relative score was significantly lower in the aDBS than cDBS; 2. Rest tremor and bradykinesia did not differ in aDBS and cDBS; cDBS had higher rigidity; dyskinesia score was significantly lower in aDBS; 3. Energy was significantly higher in cDBS. |
Gilron et al., 2021 [30] | Rigidity and bradykinesia PD patients | 5 | Self-control | Bilateral STN | STN and M1 oscillation | Gradual | 14 months | 1. An implantable two-way neural interface for wireless was designed and applied; 2. Increased on time without dyskinesia during aDBS than clinically optimized cDBS at home. |
MPTP, 1-methyl-4-phenyl-1,2,3,6-tetrahydropyridine (primate model of Parkinson’s disease); DBS, deep brain stimulation; aDBS, adaptive DBS; cDBS, conventional DBS; GPi, globus pallidus internus; M1, primary motor cortex; PD, Parkinson’s disease; STN, subthalamic nucleus; LFP, local field potentials; n.s, not significant; FoG, freezing of gait. *This patient accepted bilateral cDBS initially and transferred to unilateral aDBS; **Patients were implanted bilaterally but under aDBS unilaterally; ***Patients applied bilateral aDBS on PPN and bilateral cDBS on STN.
Predominant Parkinsonian symptoms mainly include bradykinesia/rigidity, tremor, freezing of gait (FoG)/imbalance, dyskinesia, and nonmotor symptoms [1, 2, 43]. Bradykinesia-rigidity PD is the most studied phenotype for aDBS [13, 14]. Both subcortical and cortical signals show an ability to represent bradykinesia and rigidity [44] and can be input signals for aDBS [22, 45]. Most previous studies on aDBS also focused on bradykinesia-rigidity PD [29, 30, 46, 47] or reported significant improvement in akinesia and rigidity fluctuations [21, 23, 27, 28, 42, 48, 49], suggesting an optimal phenotype for aDBS. In tremor-dominant PD, wearable devices show advantages in detecting and predicting tremor [50–52], while the correlation of subcortical and cortical signals with tremor remains controversial [53–57]. Not only did the study of Malekmohammadi et al. achieve aDBS for tremor-dominant PD based on wearable device signals [58], but some aDBS efforts for other PD phenotypes based on subcortical and cortical signals also provided improvement in tremor [28, 29, 42, 49]. In addition, Cernera et al. [59], He et al. [60], and Opri et al. [61] applied closed-loop aDBS for essential tremor (ET) based on wearable-sensor-driven signals, subcortical (thalamic) LFPs, and cortical (M1) activity. These studies in ET also revealed the potential of different biomarkers for tremor-dominant PD. However, only a limited number of studies with small sample sizes have focused on FoG/imbalance [62, 63], dyskinesia [64], and speech side effects [65] of aDBS for PD, and its benefits are still debated, requiring more studies. Moreover, aDBS targeting nonmotor symptoms of PD is still lacking. For PD combined with psychiatric symptoms, the progress of aDBS for psychiatric disorders [66, 67] can be a paradigm to modulate both motor and psychiatric pathological brain circuit activities together [25, 68]. Future development of aDBS for PD requires further understanding of the pathophysiological mechanisms of different symptoms to cover more symptoms [25].
Another concern relates to the stimulation target. Commonly used targets for cDBS of PD include the subthalamic nucleus (STN) and the globus pallidus internus (GPi) [69] while these two targets are believed to have similar motor benefits [70, 71]. STN cDBS may allow a greater reduction of medication, while GPi cDBS exerts a direct anti-dyskinetic effect [70, 71]. In addition to these common targets, recent cDBS studies have suggested that stimulation of the ventral intermediate nucleus (Vim) has long-term benefit for tremor control and that the pedunculopontine nucleus (PPN) cDBS improves axial motor deficits, particularly FoG and falls [71, 72]. As stimulation of the Vim and PPN addressed other motor features of PD insufficiently, they only applied for patients with particular severe symptoms [71, 72]. Almost all previous studies on aDBS of PD were applied for the STN and showed favorable efficacy [23, 27–31, 42, 46–49, 58, 64, 65]. The STN featured subcortical signal (local field potentials, LFP) correlates with several clinical symptoms [73, 74], making it a sufficient input signal during delivery stimulation [14]. Only a clinical study on PPN (targets PD patients with FoG) based on PPN-LFP [62] and a nonhuman study on GPi based on Gpi and primary motor cortex (M1) spikes [27] performed aDBS on other targets. Furthermore, limited by devices and algorithms, most prior clinical studies only applied unilateral aDBS [23, 29, 31, 42, 64] (or bilaterally implanted but unilaterally turned on aDBS [46, 49]), while bilateral aDBS is becoming available in recent aDBS studies for PD [28, 30, 47, 48, 58, 65]. Because bilateral stimulation shows greater improvement in motor symptoms and gait [75], unilateral stimulation is now only proposed for asymmetric PD patients [71]. Thus, aDBS should be studied more on bilateral targets. Synchronous stimulation on multiple targets may provide additional efficacy for wider symptom coverage [72, 76]. However, a bilateral GPi cDBS plus bilateral PPN aDBS study for PD patients with medication-refractory FoG did not reveal more benefit but reported more adverse events [62]. Studies exploring the efficacy and safety of bilateral aDBS on different targets for PD are still needed, especially comparisons of different targets.
In general, current studies have provided initial experience with optimal symptoms, targets, and PD patients receiving aDBS. Available data suggest that phenotypes such as bradykinesia/rigidity and tremor and targets such as bilateral STN could be adequate choices, while further studies on FoG/imbalance, dyskinesia, nonmotor symptoms, and side effects based on bilateral aDBS of different targets need more exploration. Furthermore, since it involves a novel brain signal process and neuromodulate strategy, data safety and ethics concerns can also make acceptance challenging for potential PD patients on aDBS, which means that more detailed preoperative communication with patients is needed.
Input signals of aDBS for PD
A sensing module that measures the symptoms and status of PD patients is the first step in developing a valid aDBS system [14]. Thus, it is necessary to determine robust input signals of aDBS for PD [25]. To date, an increasing number of studies on electrophysiological and brain circuits have provided several biomarkers correlating to PD patients’ symptoms and status, which are considered promising input signals of aDBS [73]. These input signals mainly included subcortical signals, cortical signals, signals from surface electromyographic (sEMG) and other wearable devices, and future potential signals [77]. In the following, we review each of these input signals.
Subcortical signals
Concerning the input signal of aDBS for PD, most of the current subcortical signals refer to the recoding of the basal ganglia LFP, which reflects the sum of the extracellular electrical activity of a group of neurons in the vicinity of the recording electrode [73]. LFP can be captured through microelectrodes during DBS implantation [78], through cables connecting DBS leads during the temporary lead externalization period (3–4 days after implantation and before the final connection to the implanted pulse generator) [79], and through new generation chronic bidirectional sensing and stimulation electrodes [24, 80]. In this aspect, STN-LFP is tested the most as a biomarker, and it has been tested with clinical relevance and stable consistency [81]. Numerous studies have demonstrated that the beta band power (13–30 Hz) of STN-LFP is abnormally high in PD patients [73, 74, 82–85]. During movement preparation and execution, this abnormal high beta activity decreased and then rebounded after movement, suggesting a correlation between beta activity of STN-LFP and movement status and symptoms [86–88]. Dopaminergic intake and/or DBS can suppress this beta activity, and the degree of suppression of oscillatory power correlates with improved Parkinsonian symptoms such as bradykinesia, rigidity, and akinesia [22, 45, 89]. In addition, tremor [90, 91] and FoG/imbalance [92, 93] symptoms as well as sleep stage [94, 95], speaking behavior [96–98], and emotional symptoms [99] can also be detected by different frequency bands of STN-LFP. A number of proof-of-concept clinical studies have applied STN beta activity as an input signal of aDBS [23, 28, 29, 46, 64, 65]. These studies suggested that beta-LFP-based STN aDBS provided greater or at least comparable motor improvement [23, 28, 29, 46, 64, 65] with less energy delivered [23, 29, 64] than cDBS for PD. A recent meta-analysis comparing aDBS with cDBS (mainly in the beta band) for PD suggested that aDBS might outperform cDBS in overall motor improvement with a reduction in energy delivery (55% less than cDBS), while rigidity-bradykinesia, levodopa-induced dyskinesia, and speech intelligibility in aDBS and cDBS were comparable, and beta-based aDBS might not be as efficient as cDBS for tremor control [100]. However, most of the current studies were acute trials with limited sample sizes; thus, there is still a need for future long-term class I evidence such as that which will result from the ongoing randomized multicenter clinical trial NCT04547712 [101]. It is also important to note a recent study suggesting beta-driven aDBS can compromise human motor behavior in PD, especially impairing movement termination [102], which merits further research and attention. As mentioned before, LFP can be recoded chronically through new generation electrodes, making it available for safe long-term recording without additional devices [79, 80]. Another advantage is that the correlation between the beta power of STN-LFP and motor symptom severity shows stable consistency for PD [103] even after long-term STN-DBS [104]. These findings suggest that STN-LFP may be an available input and feedback signal for aDBS that can stably reflect Parkinsonian symptoms [24, 30].
In addition to beta activity of STN-LFP, other STN-LFP-based electrophysiological biomarkers may also be suitable as input signals for aDBS targeting different symptoms, such as low-frequency band (< 12 Hz) [105], gamma band (31–100 Hz) [106], high-frequency band (> 200 Hz) [107] activity and single-unit activity [108]. However, only limited studies on these biomarkers and their correlations to different symptoms are still under further exploration. Furthermore, an animal model using LFP of Gpi [21] and a clinically used LFP of PPN [62] also showed that LFP of other targets also had the potential to become input signals of aDBS. Another promising source of input signals for aDBS could be evoked potentials elicited by applied stimuli (recorded from local stimulation sites such as the STN and GPi as subcortical signals or from remote areas of the cortex as cortical signals), which reveal information about neural connectivity and function [109–111]. A recent study by Sanabria et al. suggested that evoked potentials generated in the brain by GPi-DBS could be recorded to suppress or amplify frequency-specific (16–22 Hz, resonating in the beta band) neural activity in a PD patient implanted with a directional DBS lead [112]. These studies describe encouraging attempts, but their effectiveness and applicability still need to be confirmed.
Although subcortical signals may be regarded as the most studied and potentially suitable input signals of aDBS for PD [24], they still have some limitations. First, movement artifacts and stimulation artifacts make the detection and recognition of LFP a challenge [113, 114], and some patients may not easily be recorded with all bands of LFP [45]. Progress with more sensitive sensors and real-time noise removal algorithms can be helpful [113, 114]. Second, the ability of subcortical signals to capture all different symptoms and statuses remains unclear [53–57]. For example, the abilities of LFP to reflect tremor, FoG/imbalance and nonmotor symptoms of PD are still under debate [28, 29, 42, 49, 62]; machine learning applied to multiple frequency bands and the addition of other input signals may be required [55, 92, 93, 115].
Cortical signals
Cortical signals are recorded through noninvasive scalp electroencephalography (EEG) [116] or through invasive subdural electrocorticography (ECoG), which requires an implanted grid with contacts [117]. However, disturbance of the scalp makes the stability and consistency of EEG unable to fit the sufficiency requirement of input signal [118]. Thus, ECoG oscillations instead of EEG are utilized as input cortical signals of aDBS for PD [118, 119]. ECoG studies on PD patients revealed that high beta (20–30 Hz) power and gamma power are increased in M1 during movement tasks [120]. These abnormal oscillations in sensorimotor cortices can be suppressed and normalized by DBS [121, 122]. A recent study suggested that ECoG might be superior to LFP for movement decoding in PD [123]. Moreover, ECoG can also be recorded to monitor dyskinesia [124] and to recognize speech [125]. Recent studies on phase-amplitude coupling (PAC), whereby the amplitude of one frequency signal is coupled to the phase of another frequency signal (such as beta-phase/broadband-gamma amplitude coupling) either within an area or between areas [126], also show its role in reflecting Parkinsonian symptoms. M1-beta-gamma-PAC is exaggerated in PD patients with dystonia compared to humans without movement disorders, and peaks in M1 gamma amplitude are coupled to, and precede, the STN beta-trough, suggesting a model of the basal ganglia-cortical circuit in PD [126]. This M1-beta-gamma-PAC decreases after STN-DBS or GPi-DBS, and the change is parallel to a decrease in bradykinesia-rigidity [126–128], which can be observed even after chronic stimulation [126, 128]. In contrast, M1-beta-gamma-PAC decreases during resting tremor [53]. Our team also revealed that the intensity of M1-beta-gamma-PAC predicted FoG severity and that STN-DBS alleviated FOG by reducing cortical PAC and increasing cortical resilience [129]. In addition, several studies have confirmed that ECoG technology produces chronic recordings safely [126, 128]. These findings provide a further foundation for ECoG as an appropriate input signal in aDBS for PD.
In developing cortical signals as input signals of aDBS for PD, a monkey 1-methyl-4-phenyl-1,2,3,6-tetrahydropyridine (MPTP) PD model used Gpi and cortical ECoG oscillatory burst as input signals for GPi aDBS and suggested that aDBS could better suppress pallidal discharge rate and oscillations and better ameliorate akinesia than cDBS [21]. Swann et al. applied cortical signals as input signals of aDBS for two PD patients [31]. They adjusted the stimulation voltage according to ECoG narrowband gamma (60–90 Hz) oscillations and suggested similar bradykinesia and dyskinesia scores for aDBS and cDBS, while aDBS had substantial energy savings (38% –45%) [31]. A recent long-term study applied STN-LFP and cortical ECoG as input signals of STN aDBS for 5 PD patients with rigidity and bradykinesia and revealed an increased time without dyskinesia during aDBS compared with clinically optimized cDBS [30]. These studies showed the ability to use cortical signals as input signals, but their limitations on sample size, follow-up periods, and efficacy assessments still indicate the need for more studies.
The use of cortical signals as the input signal of aDBS for PD also has some challenges. A major concern is that the recording of ECoG requires an invasively implanted subdural grid. Although this grid can be placed through the same burr hole as DBS electrodes, the additional implant may still increase the risk of complications, such as infection and hemorrhage [57]. Long-term studies with large sample sizes on the safety of the chronically implanted ECoG grid should be conducted. Another question is whether cortical oscillations have some limitations as an input signal of aDBS for PD. During movement preparation and execution [128] and stimulation [130], cortical beta-PAC may be altered, which has implications for feedback signal analysis. Additionally, the ability of cortical signals to represent all motor and nonmotor symptoms is still unclear [44, 117, 130]. Generally, the potential abilities of reflecting and predicting Parkinsonian symptoms (especially on FoG) and decoding movement and behavior make cortical signals a promising input signal of aDBS for PD. Further steps to be taken are developing cortical signal algorithms for different motor and nonmotor symptoms and integrating other potential signals for the whole PD spectrum.
sEMG and other wearable devices
Noninvasive sEMG and other wearable devices (gyroscopes, accelerometers, or magnetometers) provide useful information for movement status and symptoms, especially for tremor detection and prediction [51, 131–134]. Thus, for tremor-dominant PD, these devices could be considered as a potential input signal of aDBS. Studies based on sEMG and other wearable devices as input signals of aDBS for ET revealed sufficient efficacy [135]. Malekmohammadi et al. innovatively used 4–8 Hz tremor power measured by a wearable smartwatch as an input signal for STN aDBS in 8 tremor-dominant PD patients [58]. The researchers observed a significantly lower (36.6%) resting tremor and a lower (76.35%) voltage used than cDBS [58]. This study expands insight regarding these wearable devices as a source of input signals for tremor-dominant PD. In addition, several studies have demonstrated the potential of wearable devices for monitoring postural instability and gait in PD [136]; such devices include inertial measurement units (IMUs) [137–139]. Although these wearable technologies provide an opportunity to monitor PD symptoms chronically [140], research is still needed to standardize them and improve their resolution [136].
However, evidence on the use of sEMG and other wearable devices to detect bradykinesia-rigidity symptoms is limited [132, 141, 142]. This makes it necessary for these devices to combine other input signals for akinetic-rigid PD patients and patients with multiple symptoms. Another concern is the consistency. Patients are required to wear and manage these devices by themselves, which may lead to position shifting and data quality loss [132]. Subcutaneous implantation of sensors may increase stability. However, an implanted device may also face complications. These shortcomings make sEMG and other wearable devices more suitable for combining other stable signals to cover more symptoms or to identify symptoms and verify efficacy than providing a single input signal of aDBS for PD [30, 61].
Future potential signals
Patient-dependent mobile health using telemonitoring or video monitoring is proposed for PD patients’ care at home [143]. This design allows patients to adjust or choose parameters and even turn ON/OFF according to their individual needs [144]. This flexible method may make the simulation more suitable for the needs of patients [144]. Our team also revealed that simple and flexible voltage-based programming can be effective for remote self-administration [145]. However, when the patient’s symptoms or adverse effects cannot be resolved by self-control programing, a comprehensive evaluation and adjustment is still needed at the hospital [145]. Furthermore, the development of smartphones and remote sensors increases the ability to capture symptom fluctuation when patients are at home [146, 147]. These remote information technologies provide complementary support for input signals of aDBS [146, 147], especially when aDBS is applied in chronic daily use at home.
Mechanism-based neurochemical recordings of dopamine may be a future input signal for aDBS [148]. An important step is to develop real-time measurement of neurochemical substances using biocompatible devices and to verify its applicability for aDBS in PD [149]. Recently, optogenetic studies have uncovered unique functionality in stimulating specific groups of neurons to control the basal ganglia circuitry [150]. This novel optogenetic technology may be helpful in locating individual circuits [150] and new targets [151]. For instance, Zhang et al. suggested that targeting parafascicular thalamic circuits may be helpful for motor and nonmotor deficits in PD [151]. Fougère et al. identified the glutamatergic neurons of the cuneiform nucleus as a potential stimulation group to improve locomotor activity in PD [150]. These optogenetic works show great potential in the future development of aDBS for PD.
Although the development of aDBS based on these signals is in an early phase, these studies have proposed new paths or complementary supports and provided insights that may enhance methods in aDBS for PD. However, it is still a long way to develop, verify, put in use, and realize “from bench to bedside.”
Adaptive strategy and stimulation parameters
After determining and sensing input signals, a sensing module can be realized. The next step is to establish a control and stimulation module. Together, these three technological components can establish an available aDBS for PD [13]. In the following sections, we discuss the current progress and remaining challenges regarding adaptive strategies and stimulation parameters.
First of all, neural recordings of brain signals may encode various clinical states at different time scales, requiring fast or slow adaptation strategies according to different temporal dynamics [152]. A common example is the fast and slow modulation of beta-LFP-based aDBS. Previous studies on PD revealed that beta-LFP is not consistently elevated but rather comes in bursts [23, 65, 153]; and these beta bursts have prolonged duration (pathological 200–1,000 ms vs. physiological 100–200 ms) and increased amplitudes when Parkinsonian motor impairment is present [153–155]. Thus, a fast adaptive strategy is required to capture these fast-changing dynamics in the range of milliseconds; to date, timescales of less than 400 ms [23, 153] and 800 ms [29] have been achieved. Another slow adaptive strategy is to process beta activity in the range of minutes without detecting beta bursts but considering medication-related OFF/ON Parkinsonian symptom fluctuations; this strategy has been tested with a 50 s smoothing time [46, 64]. Moreover, gamma-ECoG-based aDBS applies a design with 30 s evaluating averaged power followed by a 600 ms decision, which is another example of a slow adaptive strategy [31]. Methods to capture and integrate distinct biomarkers under different temporal dynamics still need to be explored [152]. Readers are encouraged to review the study of Tinkhauser et al. regarding adaptive strategies with different temporal dynamics [152].
Next, amplitude (either of voltage or of current) serves as a major automatically adjusted parameter to improve symptoms for PD patients in existing treatments [24]. Regarding amplitude modulation approaches, three adaptive strategies are proposed: the ON/OFF design, the gradual design, and the continuous design [14]. The ON/OFF design decides a fixed setting on amplitude, frequency, pulse width, and stimulation period before the adaptive mode, and a certain threshold (for example, exceeding a given beta amplitude of the LFP) is determined [27]. After the adaptive mode of aDBS is turned on, the input signal is continuously recorded. When the input signal reaches and exceeds a certain threshold, stimulation is turned on instantly and is turned off after the signal returns to the given range [27]. This design has been widely used in aDBS using beta-LFP as an input signal for PD patients [23, 27, 28, 49, 65]. The ON/OFF design is a simple and stable adaptive strategy for aDBS. However, some details should be considered. First, to reduce paresthesia, the amplitude should ramp up gradually from zero to the predefined setting [23, 153]. Next, stimulation can be locked to specific pathological neurophysiological phases in PD as a phase-dependent stimulation strategy [23, 153], which may be useful in enhancing long-lasting therapeutic efficacy and minimizing stimulation-related side effects [156]. Cagnan et al. designed the phase-dependent strategy aDBS for ET with locked tremor phase, which reduced tremor severity while also mitigating energy consumption and potentially the risk of tolerance and rebound [157, 158]. Their latest results further supported phase specificity in achieving postural and kinetic tremor control and suggested that ET patients with more stable tremor characteristics might benefit more from this strategy [159]. These findings suggest potential insights for phase-dependent strategy of aDBS for PD. Moreover, to reduce influences on patients’ daily activities, machine-learning algorithms may be applied to predict the occurrence of symptoms and turn on stimulation before the symptoms [160]. However, the ON/OFF design does not sufficiently correspond to the input signal, which makes it necessary to propose gradual and continuous designs [14]. Gradual design applies multiple thresholds and increases or decreases the amplitude stepwise when the input signal is above or below these thresholds, respectively [29, 30]. For instance, Velisar et al. applied a dual threshold algorithm on beta-LFP of STN aDBS for PD based on patients’ specific therapeutic voltage windows [29]. Continuous design links the amplitude to input signals, which makes the output amplitude incline toward a line parallel to input signals [42, 46–48, 64]. Gradual and continuous designs are believed to have better consistency with input signals as well as symptom severity; in theory, they may have better efficacy and be associated with fewer adverse effects [24]. However, the increased stimulation time introduces more battery consumption [79], and a stable feedback signal is required [42]. Additionally, no studies have compared these different designs of amplitude adaptive strategies; they deserve further research, especially for PD patients with different phenotypes and aDBS with different input signals. Concerning other stimulation parameters, such as frequency and pulse width, most current aDBSs for PD apply a preset fixed setting during stimulation [29, 42]. However, reprogramming these parameters is also an important step toward realizing the best compromise between therapeutic response and adverse effects [17]. In particular, high (130 Hz), low (60–80 Hz), and varied frequencies may have different efficacies in Parkinsonian symptoms and adverse events [17, 161]. The ultimate goal is to develop reliable semiautomated [162] or even fully automated [163] approaches to optimize multiple parameters of aDBS for PD; this will require the building of machine learning models [164].
In summary, the adaptive strategy and stimulation parameters are also important for the development of an available aDBS for PD. Most of the current studies only adjusted the amplitude with several designs. Future research directions should include comparison of different adaptive designs and development of a reliable automated module to adjust multiple parameters and to realize better efficacy and fewer side effects with less battery consumption.
FUTURE PERSPECTIVES OF aDBS FOR PD
From unimodal to multimodal inputs
As mentioned above, a single input signal has inherent limitations. For example, subcortical signals are featured in reflecting bradykinesia/rigidity [22, 45, 89] but are debated in tremor and FoG/imbalance [55, 92, 93, 115]. Cortical signals and sEMG and wearable devices show advantages in detecting FoG/imbalance [127, 129, 165] and tremor [58], respectively. Patient-dependent mobile health provides complementary support for input signals of aDBS [146, 147] when chronically used at home. Thus, combinations of different input signals may be more helpful to cover different symptoms. The FDA-approved Activa PC C + S and Summit RC C + S platforms for experimental platforms have chronic neural sensing from both subcortical and cortical signals and can wirelessly stream neural recordings, providing a perspective on multimodal inputs [24, 30]. With these devices, Arlotti et al. used Activa PC C + S in aDBS mode for 24 hours, and Golron et al. applied Summit RC C + S during normal daily activities for 14 months, showing its great potential in chronic applicability [30]. Additionally, an automatic and validated Parkinsonian symptom identification system using these multimodal inputs can be developed to choose individual-specific inputs and provide symptom-guided stimulation. Furthermore, multimodal inputs have advantages in identifying activity status (walking, resting, sleeping, etc.) [86–88, 94, 95, 123], which makes on-demand stimulation possible. These state-based on-demand aDBSs are particularly useful for the future application of chronically implanted aDBSs [24]. For instance, tremor-dominant PD patients can reduce stimulation during sleeping and increase stimulation after awaking. Despite these insights, more energy consumption because of multiple signals and complex algorithm calculations as well as increased risk of adverse events because of increased implants should be considered for multimodal input design.
On nonmotor symptoms and side effects
Apart from motor symptoms, a wide variety of nonmotor complications, including psychiatric symptoms, cognitive impairment, and somatosensory changes, are usually observed in PD patients [2, 43]. Previous studies suggested discordant or even conflicting results in mood effects [166] and cognitive outcomes [167] of cDBS for PD. A meta-analysis suggested that DBS improved depression and anxiety symptoms, which might be associated with the improvement of motor symptoms [166]. In general, DBS has no severe impact on cognitive functions in PD patients [167]. However, some patients experienced cognitive decline [167, 168], which might be related to exceptional brain network connectivity and could be relieved by stimulation reprogramming [168]. However, the current study on cDBS targeting motor and nonmotor symptoms together is still lacking. The advantage of aDBS from its ability to detect neurophysiological signals and circuit activities makes it available to modulate motor and nonmotor circuits together. The progress of identifying state-dependent responses and applying circuit-based aDBS for psychiatric disorders such as depression [66, 67] and brain stimulation for cognitive defect diseases such as Alzheimer’s disease by tackling circuit dysfunction [169] could provide helpful foundations. Future aDBS can combine these available circuits with motor circuits to develop treatments for PD patients with both motor and nonmotor symptoms. Furthermore, continuous cDBS could induce side effects such as dysarthria and imbalance, which might be related to excessive stimulation and exceptional circuits [12, 15, 16]. Research on aDBS for PD should be conducted to identify these side effects and provide sufficient stimulation to reduce their occurrence.
A future next-generation fully adaptive aDBS
In summary, a future next generation aDBS for PD should be “fully adaptive” with the following features. First, the sensing module identifies the patient’s symptoms, phenotype, circuit, and biomarkers to choose the best combination of stimulation targets and multimodal input signals for symptom detection. Next, the control module processes these input signals, analyzes the optimal adaptive strategy and determines the stimulation parameters. After that, the stimulation module provides the best optimized therapeutic effects. The sensing module continuously monitors efficacy, side effects, activity status, and multimodal inputs as feedback signals and then sends them to the control module for further adaptive strategy and parameter adjustments. After patients return home for chronic use, remote mobile health provides patients with some self-control parameter choices, and patients can make adjustments according to their individual needs. This remote mobile health system also monitors patients’ symptoms and needs and sends them to clinicians for guidance. When problems cannot be resolved remotely, the monitoring system alerts a hospital revisit.
Clearly, more efforts are still needed to achieve this fully adaptive “one-time implant for long-term use” aDBS and achieve better outcomes for PD patients. With the development of new devices and technologies, the proceedings can be accelerated, predictably. A diagrammatic sketch of this future next-generation fully adaptive aDBS and future key perspectives is shown in Fig. 2.
Fig. 2
A diagrammatic sketch of a future next-generation “fully adaptive” deep brain stimulation (aDBS) design for Parkinson’s disease (PD) and its future key perspectives. Words and lines in red show the improvement perspectives, lines in blue show the signals line, and lines in yellow show the stimulation line; the figure shows the main procedures in monitoring multimodal inputs for identifying motor and nonmotor symptoms, analyzing optimal adaptive strategy and stimulation parameters, providing best optimal stimulation according to the input and feedback signals, and monitoring efficacy and side effects for further adaptation.
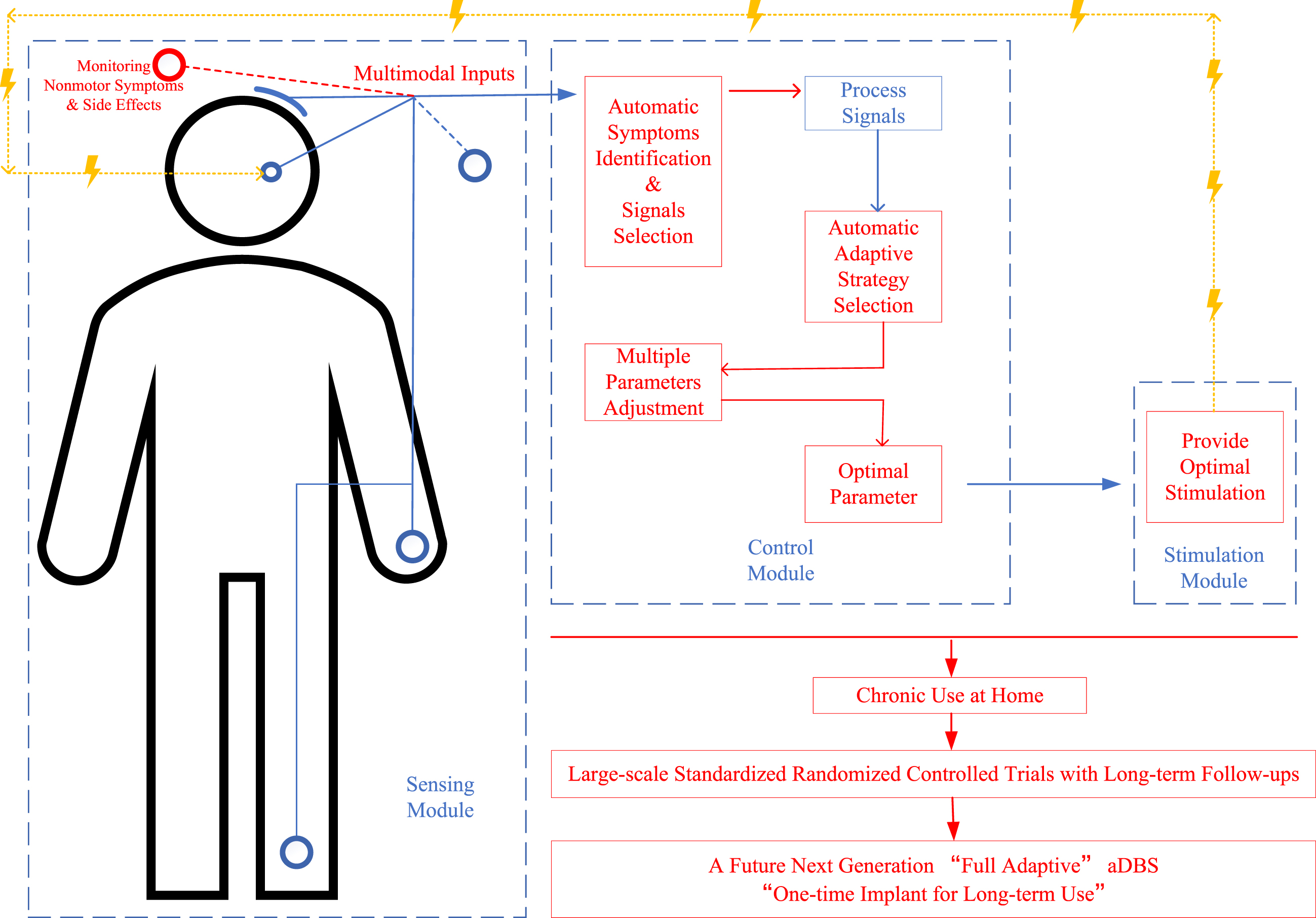
Toward standardized clinical trials
Performing well-designed standardized clinical trials is an important step to verify the efficacy and safety of aDBS for PD. Several papers have reported the application of aDBS in PD patients, and a summary is shown in Table 1. Although previous studies have provided beneficial explorations, most of these studies are proof-of-concept studies with limited sample sizes [28, 47–49, 80]. To date, the largest cohort was the study of Arlotti et al., which enrolled 11 PD patients with aDBS in 2018 [46]. A sufficient sample size is needed to meet the requirement of test power for providing convincing results. Another concern is about the stimulation time and follow-up period. Prior studies tested aDBS during the lead externalization period, which lasted only several minutes during the perioperative window period [23, 28, 49, 65]. These studies only tested the availability of aDBS design, but the “stun” or “microlesion” effect might make it not accurately represent the real efficacy of aDBS and restrict its long-term use [14]. The development of new chronically implanted devices provides opportunities to explore their long-term efficacy and applicability for home use [30, 42], regarding which further studies are required to analyze benefits and safety. Furthermore, most previous study designs only applied self-control (switched from cDBS to aDBS [23, 30, 42, 65] or only tested aDBS with baseline [29, 46]), while few studies directly compared cDBS and aDBS in separate groups [64]. Additionally, comparisons of aDBS with different designs in input signals and adaptive strategies are lacking. In general, the crucial next step for upcoming aDBS for PD trials will be to formally compare the clinical benefits and safety of aDBS with cDBS using different designs for future clinical application. Well-designed standardized clinical trials with sufficient sample sizes, adequate follow-up and stimulation periods, and appropriate analytical procedures are recommended.
CONCLUSIONS
Growing evidence has suggested that aDBS, as an emerging field, may have potential to outstrip traditional cDBS as a therapeutic choice for PD. Important steps in successful attempts to achieve aDBS include identifying optimal phenotypes, targets, patients, input signals, and adaptive strategy and stimulation parameters. However, further studies exploring multimodal inputs covering more Parkinsonian symptoms and targeting nonmotor symptoms and side effects are needed to realize a future next-generation fully adaptive “one-time implant for long-term use” aDBS. Additionally, large-scale standardized clinical trials with long-term follow-ups and high quality are required to better verify the clinical efficacy and limitations of aDBS. Together, these findings will shed light on the future clinical use of aDBS for PD.
ACKNOWLEDGMENTS
This work was supported by the National Natural Science Foundation of China (81830033).
CONFLICT OF INTEREST
The authors have no conflicts of interest to report.
DATA AVAILABILITY
All data generated or analyzed during this study are included in this published article.
REFERENCES
[1] | Bloem BR , Okun MS , Klein C ((2021) ) Parkinson’s disease. Lancet 397: , 2284–2303. |
[2] | Tolosa E , Garrido A , Scholz SW , Poewe W ((2021) ) Challenges in the diagnosis of Parkinson’s disease. Lancet Neurol 20: , 385–397. |
[3] | ((2020) ) Global burden of 87 risk factors in 204 countries and territories, 1990-2019: A systematic analysis for the Global Burden of Disease Study 2019. Lancet 396: , 1223–1249. |
[4] | Dorsey ER , Sherer T , Okun MS , Bloem BR ((2018) ) The emerging evidence of the Parkinson pandemic. J Parkinsons Dis 8: , S3–s8. |
[5] | Trist BG , Hare DJ , Double KL ((2019) ) Oxidative stress in the aging substantia nigra and the etiology of Parkinson’s disease. Aging Cell 18: , e13031. |
[6] | Schweitzer JS , Song B , Herrington TM , Park TY , Lee N , Ko S , Jeon J , Cha Y , Kim K , Li Q , Henchcliffe C , Kaplitt M , Neff C , Rapalino O , Seo H , Lee IH , Kim J , Kim T , Petsko GA , Ritz J , Cohen BM , Kong SW , Leblanc P , Carter BS , Kim KS ((2020) ) Personalized iPSC-derived dopamine progenitor cells for Parkinson’s disease. N Engl J Med 382: , 1926–1932. |
[7] | Verschuur CVM , Suwijn SR , Boel JA , Post B , Bloem BR , van Hilten JJ , van Laar T , Tissingh G , Munts AG , Deuschl G , Lang AE , Dijkgraaf MGW , de Haan RJ , de Bie RMA ((2019) ) Randomized delayed-start trial of levodopa in Parkinson’s disease. N Engl J Med 380: , 315–324. |
[8] | Turcano P , Mielke MM , Bower JH , Parisi JE , Cutsforth-Gregory JK , Ahlskog JE , Savica R ((2018) ) Levodopa-induced dyskinesia in Parkinson disease: A population-based cohort study. Neurology 91: , e2238–e2243. |
[9] | Weaver FM , Follett K , Stern M , Hur K , Harris C , Marks WJ Jr., Rothlind J , Sagher O , Reda D , Moy CS , Pahwa R , Burchiel K , Hogarth P , Lai EC , Duda JE , Holloway K , Samii A , Horn S , Bronstein J , Stoner G , Heemskerk J , Huang GD ((2009) ) Bilateral deep brain stimulation vs best medical therapy for patients with advanced Parkinson disease: A randomized controlled trial. JAMA 301: , 63–73. |
[10] | Wang S , Zhao M , Li T , Zhang C , Zhou J , Wang M , Wang X , Ma K , Luan G , Guan Y ((2021) ) Long-term efficacy and cognitive effects of bilateral hippocampal deep brain stimulation in patients with drug-resistant temporal lobe epilepsy. Neurol Sci 42: , 225–233. |
[11] | Krauss JK , Lipsman N , Aziz T , Boutet A , Brown P , Chang JW , Davidson B , Grill WM , Hariz MI , Horn A , Schulder M , Mammis A , Tass PA , Volkmann J , Lozano AM ((2021) ) Technology of deep brain stimulation: Current status and future directions. Nat Rev Neurol 17: , 75–87. |
[12] | Limousin P , Foltynie T ((2019) ) Long-term outcomes of deep brain stimulation in Parkinson disease. Nat Rev Neurol 15: , 234–242. |
[13] | Guidetti M , Marceglia S , Loh A , Harmsen IE , Meoni S , Foffani G , Lozano AM , Moro E , Volkmann J , Priori A ((2021) ) Clinical perspectives of adaptive deep brain stimulation. Brain Stimul 14: , 1238–1247. |
[14] | Habets JGV , Heijmans M , Kuijf ML , Janssen MLF , Temel Y , Kubben PL ((2018) ) An update on adaptive deep brain stimulation in Parkinson’s disease. Mov Disord 33: , 1834–1843. |
[15] | Tanaka Y , Tsuboi T , Watanabe H , Torii J , Nakatsubo D , Maesawa S , Sato M , Hiraga K , Satake Y , Yokoi K , Hattori M , Kawabata K , Hara K , Yamamoto M , Sobue G , Katsuno M ((2021) ) Instability of speech in Parkinson disease patients with subthalamic nucleus deep brain stimulation. Parkinsonism Relat Disord 93: , 8–11. |
[16] | Collomb-Clerc A , Welter ML ((2015) ) Effects of deep brain stimulation on balance and gait in patients with Parkinson’s disease: A systematic neurophysiological review. Neurophysiol Clin 45: , 371–388. |
[17] | Dayal V , Limousin P , Foltynie T ((2017) ) Subthalamic nucleus deep brain stimulation in Parkinson’s disease: The effect of varying stimulation parameters. J Parkinsons Dis 7: , 235–245. |
[18] | Marceglia S , Rossi L , Foffani G , Bianchi A , Cerutti S , Priori A ((2007) ) Basal ganglia local field potentials: Applications in the development of new deep brain stimulation devices for movement disorders. Expert Rev Med Devices 4: , 605–614. |
[19] | Santaniello S , Fiengo G , Glielmo L , Grill WM ((2011) ) Closed-loop control of deep brain stimulation: A simulation study. IEEE Trans Neural Syst Rehabil Eng 19: , 15–24. |
[20] | Rosa M , Giannicola G , Servello D , Marceglia S , Pacchetti C , Porta M , Sassi M , Scelzo E , Barbieri S , Priori A ((2011) ) Subthalamic local field beta oscillations during ongoing deep brain stimulation in Parkinson’s disease inhyperacute and chronic phases. Neurosignals 19: , 151–162. |
[21] | Rosin B , Slovik M , Mitelman R , Rivlin-Etzion M , Haber SN , Israel Z , Vaadia E , Bergman H ((2011) ) Closed-loop deep brain stimulation is superior in ameliorating parkinsonism. Neuron 72: , 370–384. |
[22] | Little S , Pogosyan A , Kuhn AA , Brown P ((2012) ) β band stability over time correlates with Parkinsonian rigidity and bradykinesia. Exp Neurol 236: , 383–388. |
[23] | Little S , Pogosyan A , Neal S , Zavala B , Zrinzo L , Hariz M , Foltynie T , Limousin P , Ashkan K , FitzGerald J , Green AL , Aziz TZ , Brown P ((2013) ) Adaptive deep brain stimulation in advanced Parkinson disease. Ann Neurol 74: , 449–457. |
[24] | Vedam-Mai V , Deisseroth K , Giordano J , Lazaro-Munoz G , Chiong W , Suthana N , Langevin JP , Gill J , Goodman W , Provenza NR , Halpern CH , Shivacharan RS , Cunningham TN , Sheth SA , Pouratian N , Scangos KW , Mayberg HS , Horn A , Johnson KA , Butson CR , Gilron R , de Hemptinne C , Wilt R , Yaroshinsky M , Little S , Starr P , Worrell G , Shirvalkar P , Chang E , Volkmann J , Muthuraman M , Groppa S , Kühn AA , Li L , Johnson M , Otto KJ , Raike R , Goetz S , Wu C , Silburn P , Cheeran B , Pathak YJ , Malekmohammadi M , Gunduz A , Wong JK , Cernera S , Hu W , Wagle Shukla A , Ramirez-Zamora A , Deeb W , Patterson A , Foote KD , Okun MS ((2021) ) Proceedings of the Eighth Annual Deep Brain Stimulation Think Tank: Advances in optogenetics, ethical issues affecting DBS research, neuromodulatory approaches for depression, adaptive neurostimulation, and emerging DBS technologies. Front Hum Neurosci 15: , 644593. |
[25] | Bouthour W , Mégevand P , Donoghue J , Lüscher C , Birbaumer N , Krack P ((2019) ) Biomarkers for closed-loopdeep brain stimulation in Parkinson disease and beyond. Nat Rev Neurol 15: , 343–352. |
[26] | Beudel M , Brown P ((2016) ) Adaptive deep brain stimulation in Parkinson’s disease. Parkinsonism Relat Disord 22: (Suppl 1), S123–126. |
[27] | Johnson LA , Nebeck SD , Muralidharan A , Johnson MD , Baker KB , Vitek JL ((2016) ) Closed-loop deep brain stimulationeffects on parkinsonian motor symptoms in a non-human primate - is beta enough? Brain Stimul 9: , 892–896. |
[28] | Little S , Beudel M , Zrinzo L , Foltynie T , Limousin P , Hariz M , Neal S , Cheeran B , Cagnan H , Gratwicke J , Aziz TZ , Pogosyan A , Brown P ((2016) ) Bilateral adaptive deep brain stimulation is effective in Parkinson’s disease. J Neurol Neurosurg Psychiatry 87: , 717–721. |
[29] | Velisar A , Syrkin-Nikolau J , Blumenfeld Z , Trager MH , Afzal MF , Prabhakar V , Bronte-Stewart H ((2019) ) Dual threshold neural closed loop deep brain stimulation in Parkinson disease patients. Brain Stimul 12: , 868–876. |
[30] | Gilron R , Little S , Perrone R , Wilt R , de Hemptinne C , Yaroshinsky MS , Racine CA , Wang SS , Ostrem JL , Larson PS , Wang DD , Galifianakis NB , Bledsoe IO , San Luciano M , Dawes HE , Worrell GA , Kremen V , Borton DA , Denison T , Starr PA ((2021) ) Long-term wireless streaming of neural recordings for circuit discovery and adaptive stimulation in individuals with Parkinson’s disease. Nat Biotechnol 39: , 1078–1085. |
[31] | Swann NC , de Hemptinne C , Thompson MC , Miocinovic S , Miller AM , Gilron R , Ostrem JL , Chizeck HJ , Starr PA ((2018) ) Adaptive deep brain stimulation for Parkinson’s disease using motor cortex sensing. J Neural Eng 15: , 046006. |
[32] | Little S , Brown P ((2020) ) Debugging adaptive deep brain stimulation for Parkinson’s disease. Mov Disord 35: , 555–561. |
[33] | Cuschieri A , Borg N , Zammit C ((2022) ) Closed loop deep brain stimulation: A systematic scoping review. Clin Neurol Neurosurg 223: , 107516. |
[34] | van Wijk BCM , de Bie RMA , Beudel M ((2023) ) A systematic review of local field potential physiomarkers in Parkinson’s disease: From clinical correlations to adaptive deep brain stimulation algorithms. J Neurol 270: , 1162–1177. |
[35] | Merk T , Peterson V , Köhler R , Haufe S , Richardson RM , Neumann WJ ((2022) ) Machine learning based brain signal decoding for intelligent adaptive deep brain stimulation. Exp Neurol 351: , 113993. |
[36] | Frey J , Cagle J , Johnson KA , Wong JK , Hilliard JD , Butson CR , Okun MS , de Hemptinne C ((2022) ) Past, present, and future of deep brain stimulation: Hardware, software, imaging, physiology and novel approaches. Front Neurol 13: , 825178. |
[37] | Potel SR , Marceglia S , Meoni S , Kalia SK , Cury RG , Moro E ((2022) ) Advances in DBS technology and novel applications: Focus on movement disorders. Curr Neurol Neurosci Rep 22: , 577–588. |
[38] | Pozzi NG , Isaias IU ((2022) ) Adaptive deep brain stimulation: Retuning Parkinson’s disease. Handb Clin Neurol 184: , 273–284. |
[39] | di Biase L , Tinkhauser G , Martin Moraud E , Caminiti ML , Pecoraro PM , Di Lazzaro V ((2021) ) Adaptive, personalized closed-loop therapy for Parkinson’s disease: Biochemical, neurophysiological, and wearable sensing systems. Expert Rev Neurother 21: , 1371–1388. |
[40] | Priori A , Foffani G , Rossi L , Marceglia S ((2013) ) Adaptive deep brain stimulation (aDBS) controlled by local field potential oscillations. Exp Neurol 245: , 77–86. |
[41] | Eisinger RS , Cernera S , Gittis A , Gunduz A , Okun MS ((2019) ) A review of basal ganglia circuits and physiology: Application to deep brain stimulation. Parkinsonism Relat Disord 59: , 9–20. |
[42] | Bocci T , Prenassi M , Arlotti M , Cogiamanian FM , Borellini L , Moro E , Lozano AM , Volkmann J , Barbieri S , Priori A , Marceglia S ((2021) ) Eight-hours conventional versus adaptive deep brain stimulation of the subthalamic nucleus in Parkinson’s disease. NPJ Parkinsons Dis 7: , 88. |
[43] | Jankovic J , Tan EK ((2020) ) Parkinson’s disease: Etiopathogenesis and treatment. J Neurol Neurosurg Psychiatry 91: , 795–808. |
[44] | Cagnan H , Mallet N , Moll CKE , Gulberti A , Holt AB , Westphal M , Gerloff C , Engel AK , Hamel W , Magill PJ , Brown P , Sharott A ((2019) ) Temporal evolution of beta bursts in the parkinsonian cortical and basal ganglia network. Proc Natl Acad Sci U S A 116: , 16095–16104. |
[45] | Giannicola G , Marceglia S , Rossi L , Mrakic-Sposta S , Rampini P , Tamma F , Cogiamanian F , Barbieri S , Priori A ((2010) ) The effects of levodopa and ongoing deep brain stimulation on subthalamic beta oscillations in Parkinson’s disease. Exp Neurol 226: , 120–127. |
[46] | Arlotti M , Marceglia S , Foffani G , Volkmann J , Lozano AM , Moro E , Cogiamanian F , Prenassi M , Bocci T , Cortese F , Rampini P , Barbieri S , Priori A ((2018) ) Eight-hours adaptive deep brain stimulation in patients with Parkinson disease. Neurology 90: , e971–e976. |
[47] | Arlotti M , Palmisano C , Minafra B , Todisco M , Pacchetti C , Canessa A , Pozzi NG , Cilia R , Prenassi M , Marceglia S , Priori A , Rampini P , Barbieri S , Servello D , Volkmann J , Pezzoli G , Isaias IU ((2019) ) Monitoring subthalamic oscillations for 24 hours in a freely moving Parkinson’s disease patient. Mov Disord 34: , 757–759. |
[48] | Rosa M , Arlotti M , Ardolino G , Cogiamanian F , Marceglia S , Di Fonzo A , Cortese F , Rampini PM , Priori A ((2015) ) Adaptive deep brain stimulation in a freely moving Parkinsonian patient. Mov Disord 30: , 1003–1005. |
[49] | Piña-Fuentes D , Little S , Oterdoom M , Neal S , Pogosyan A , Tijssen MAJ , van Laar T , Brown P , van Dijk JMC , Beudel M ((2017) ) Adaptive DBS in a Parkinson’s patient with chronically implanted DBS: A proof of principle. Mov Disord 32: , 1253–1254. |
[50] | Basu I , Graupe D , Tuninetti D , Shukla P , Slavin KV , Metman LV , Corcos DM ((2013) ) Pathological tremor prediction using surface electromyogram and acceleration: Potential use in ‘ON-OFF’ demand driven deep brain stimulator design. J Neural Eng 10: , 036019. |
[51] | Khobragade N , Graupe D , Tuninetti D ((2015) ) Towards fully automated closed-loop Deep Brain Stimulation in Parkinson’s disease patients: A LAMSTAR-based tremor predictor. Annu Int Conf IEEE Eng Med Biol Soc 2015: , 2616–2619. |
[52] | Delrobaei M , Memar S , Pieterman M , Stratton TW , McIsaac K , Jog M ((2018) ) Towards remote monitoring of Parkinson’s disease tremor using wearable motion capture systems. J Neurol Sci 384: , 38–45. |
[53] | Qasim SE , de Hemptinne C , Swann NC , Miocinovic S , Ostrem JL , Starr PA ((2016) ) Electrocorticography reveals beta desynchronization in the basal ganglia-cortical loop during rest tremor in Parkinson’s disease. Neurobiol Dis 86: , 177–186. |
[54] | Hirschmann J , Baillet S , Woolrich M , Schnitzler A , Vidaurre D , Florin E ((2020) ) Spontaneous network activity<35 Hz accounts for variability in stimulus-induced gamma responses. Neuroimage 207: , 116374. |
[55] | Hirschmann J , Abbasi O , Storzer L , Butz M , Hartmann CJ , Wojtecki L , Schnitzler A ((2019) ) Longitudinal recordings reveal transient increase of alpha/low-beta power in the subthalamic nucleus associated with the onset of parkinsonian rest tremor. Front Neurol 10: , 145. |
[56] | Shreve LA , Velisar A , Malekmohammadi M , Koop MM , Trager M , Quinn EJ , Hill BC , Blumenfeld Z , Kilbane C , Mantovani A , Henderson JM , Brontë-Stewart H ((2017) ) Subthalamic oscillations and phase amplitude coupling are greater in the more affected hemisphere in Parkinson’s disease. Clin Neurophysiol 128: , 128–137. |
[57] | Meidahl AC , Tinkhauser G , Herz DM , Cagnan H , Debarros J , Brown P ((2017) ) Adaptive deep brain stimulation for movement disorders: The long road to clinical therapy. Mov Disord 32: , 810–819. |
[58] | Malekmohammadi M , Herron J , Velisar A , Blumenfeld Z , Trager MH , Chizeck HJ , Brontë-Stewart H ((2016) ) Kinematic adaptive deep brain stimulation for resting tremor in Parkinson’s disease. Mov Disord 31: , 426–428. |
[59] | Cernera S , Alcantara JD , Opri E , Cagle JN , Eisinger RS , Boogaart Z , Pramanik L , Kelberman M , Patel B , Foote KD , Okun MS , Gunduz A ((2021) ) Wearable sensor-driven responsive deep brain stimulation for essential tremor. Brain Stimul 14: , 1434–1443. |
[60] | He S , Baig F , Mostofi A , Pogosyan A , Debarros J , Green AL , Aziz TZ , Pereira E , Brown P , Tan H ((2021) ) Closed-loop deep brain stimulation for essential tremor based on thalamic local field potentials. Mov Disord 36: , 863–873. |
[61] | Opri E , Cernera S , Molina R , Eisinger RS , Cagle JN , Almeida L , Denison T , Okun MS , Foote KD , Gunduz A ((2020) ) Chronic embedded cortico-thalamic closed-loop deep brain stimulation for the treatment of essential tremor. Sci Transl Med 12. |
[62] | Molina R , Hass CJ , Cernera S , Sowalsky K , Schmitt AC , Roper JA , Martinez-Ramirez D , Opri E , Hess CW , Eisinger RS , Foote KD , Gunduz A , Okun MS ((2021) ) Closed-loop deep brain stimulation to treat medication-refractory freezing of gait in Parkinson’s disease. Front Hum Neurosci 15: , 633655. |
[63] | Petrucci MN , Neuville RS , Afzal MF , Velisar A , Anidi CM , Anderson RW , Parker JE , O’Day JJ , Wilkins KB , Bronte-Stewart HM ((2020) ) Neural closed-loop deep brain stimulation for freezing of gait. Brain Stimul 13: , 1320–1322. |
[64] | Rosa M , Arlotti M , Marceglia S , Cogiamanian F , Ardolino G , Fonzo AD , Lopiano L , Scelzo E , Merola A , Locatelli M , Rampini PM , Priori A ((2017) ) Adaptive deep brain stimulation controls levodopa-induced side effects in Parkinsonian patients. Mov Disord 32: , 628–629. |
[65] | Little S , Tripoliti E , Beudel M , Pogosyan A , Cagnan H , Herz D , Bestmann S , Aziz T , Cheeran B , Zrinzo L , Hariz M , Hyam J , Limousin P , Foltynie T , Brown P ((2016) ) Adaptive deep brain stimulation for Parkinson’s disease demonstrates reduced speech side effects compared to conventional stimulation in the acute setting. J Neurol Neurosurg Psychiatry 87: , 1388–1389. |
[66] | Scangos KW , Makhoul GS , Sugrue LP , Chang EF , Krystal AD ((2021) ) State-dependent responses to intracranial brain stimulation in a patient with depression. Nat Med 27: , 229–231. |
[67] | Scangos KW , Khambhati AN , Daly PM , Makhoul GS , Sugrue LP , Zamanian H , Liu TX , Rao VR , Sellers KK , Dawes HE , Starr PA , Krystal AD , Chang EF ((2021) ) Closed-loop neuromodulation in an individual with treatment-resistant depression. Nat Med 27: , 1696–1700. |
[68] | Tinaz S , Kamel S , Aravala SS , Sezgin M , Elfil M , Sinha R ((2021) ) Distinct neural circuits are associated with subclinical neuropsychiatric symptoms in Parkinson’s disease. J Neurol Sci 423: , 117365. |
[69] | Fox SH , Katzenschlager R , Lim SY , Barton B , de Bie RMA , Seppi K , Coelho M , Sampaio C ((2018) ) International Parkinson and movement disorder society evidence-based medicine review: Update on treatments for the motor symptoms of Parkinson’s disease. Mov Disord 33: , 1248–1266. |
[70] | Mansouri A , Taslimi S , Badhiwala JH , Witiw CD , Nassiri F , Odekerken VJJ , De Bie RMA , Kalia SK , Hodaie M , Munhoz RP , Fasano A , Lozano AM ((2018) ) Deep brain stimulation for Parkinson’s disease: Meta-analysis of results ofrandomized trials at varying lengths of follow-up. J Neurosurg 128: , 1199–1213. |
[71] | Honey CR , Hamani C , Kalia SK , Sankar T , Picillo M , Munhoz RP , Fasano A , Panisset M ((2017) ) Deep brain stimulation target selection for Parkinson’s disease. Can J Neurol Sci 44: , 3–8. |
[72] | Thevathasan W , Debu B , Aziz T , Bloem BR , Blahak C , Butson C , Czernecki V , Foltynie T , Fraix V , Grabli D , Joint C , Lozano AM , Okun MS , Ostrem J , Pavese N , Schrader C , Tai CH , Krauss JK , Moro E ((2018) ) Pedunculopontine nucleus deep brain stimulation in Parkinson’s disease: A clinical review. Mov Disord 33: , 10–20. |
[73] | Lee LN , Huang CS , Chuang HH , Lai HJ , Yang CK , Yang YC , Kuo CC ((2021) ) An electrophysiological perspective on Parkinson’s disease: Symptomatic pathogenesis and therapeutic approaches. J Biomed Sci 28: , 85. |
[74] | Neumann WJ , Degen K , Schneider GH , Brücke C , Huebl J , Brown P , Kühn AA ((2016) ) Subthalamic synchronized oscillatory activity correlates with motor impairment in patients with Parkinson’s disease. Mov Disord 31: , 1748–1751. |
[75] | Lizarraga KJ , Jagid JR , Luca CC ((2016) ) Comparative effects of unilateral and bilateral subthalamic nucleus deep brain stimulation on gait kinematics in Parkinson’s disease: A randomized, blinded study. J Neurol 263: , 1652–1656. |
[76] | Zhang C , Wang L , Hu W , Wang T , Zhao Y , Pan Y , Almeida L , Ramirez-Zamora A , Sun B , Li D ((2020) ) Combined unilateral subthalamic nucleus and contralateral globus pallidus interna deep brain stimulation for treatment of Parkinson disease: A pilot study of symptom-tailored stimulation. Neurosurgery 87: , 1139–1147. |
[77] | Arlotti M , Rosa M , Marceglia S , Barbieri S , Priori A ((2016) ) The adaptive deep brain stimulation challenge. Parkinsonism Relat Disord 28: , 12–17. |
[78] | Weber I , Florin E , von Papen M , Visser-Vandewalle V , Timmermann L ((2020) ) Characterization of information processing in the subthalamic area of Parkinson’s patients. Neuroimage 209: , 116518. |
[79] | Rosa M , Scelzo E , Locatelli M , Carrabba G , Levi V , Arlotti M , Barbieri S , Rampini P , Priori A ((2017) ) Risk of infection after local field potential recording from externalized deep brain stimulation leads in Parkinson’s disease. World Neurosurg 97: , 64–69. |
[80] | Swann NC , de Hemptinne C , Miocinovic S , Qasim S , Ostrem JL , Galifianakis NB , Luciano MS , Wang SS , Ziman N , Taylor R , Starr PA ((2018) ) Chronic multisite brain recordings from a totally implantable bidirectional neural interface: Experience in 5 patients with Parkinson’s disease. J Neurosurg 128: , 605–616. |
[81] | Godinho F , Fim Neto A , Bianqueti BL , de Luccas JB , Varjão E , Terzian Filho PR , Figueiredo EG , Almeida TP , Yoneyama T , Takahata AK , Rocha MS , Soriano DC ((2021) ) Spectral characteristics of subthalamic nucleus local field potentials in Parkinson’s disease: Phenotype and movement matter. Eur J Neurosci 53: , 2804–2818. |
[82] | Litvak V , Eusebio A , Jha A , Oostenveld R , Barnes G , Foltynie T , Limousin P , Zrinzo L , Hariz MI , Friston K , Brown P ((2012) ) Movement-related changes in local and long-range synchronization in Parkinson’s disease revealed bysimultaneous magnetoencephalography and intracranial recordings. J Neurosci 32: , 10541–10553. |
[83] | Khawaldeh S , Tinkhauser G , Shah SA , Peterman K , Debove I , Nguyen TAK , Nowacki A , Lachenmayer ML , Schuepbach M , Pollo C , Krack P , Woolrich M , Brown P ((2020) ) Subthalamic nucleus activity dynamics and limb movement prediction in Parkinson’s disease. Brain 143: , 582–596. |
[84] | Wingeier B , Tcheng T , Koop MM , Hill BC , Heit G , Bronte-Stewart HM ((2006) ) Intra-operative STN DBS attenuates the prominent beta rhythm in the STN in Parkinson’s disease. Exp Neurol 197: , 244–251. |
[85] | Tamir I , Wang D , Chen W , Ostrem JL , Starr PA , de Hemptinne C ((2020) ) Eight cylindrical contact lead recordings in the subthalamic region localize beta oscillations source to the dorsal STN. Neurobiol Dis 146: , 105090. |
[86] | Canessa A , Pozzi NG , Arnulfo G , Brumberg J , Reich MM , Pezzoli G , Ghilardi MF , Matthies C , Steigerwald F , Volkmann J , Isaias IU ((2016) ) Striatal dopaminergic innervation regulates subthalamic beta-oscillations and cortical-subcortical coupling during movements: Preliminary evidence in subjects with Parkinson’s disease. Front Hum Neurosci 10: , 611. |
[87] | Joundi RA , Brittain JS , Green AL , Aziz TZ , Brown P , Jenkinson N ((2013) ) Persistent suppression of subthalamic beta-band activity during rhythmic finger tapping in Parkinson’s disease. Clin Neurophysiol 124: , 565–573. |
[88] | He S , Everest-Phillips C , Clouter A , Brown P , Tan H ((2020) ) Neurofeedback-linked suppression of cortical β bursts speeds up movement initiation in healthy motor control: A double-blind sham-controlled study. J Neurosci 40: , 4021–4032. |
[89] | Brittain JS , Brown P ((2014) ) Oscillations and the basal ganglia: Motor control and beyond. Neuroimage 85: (Pt 2), 637–647. |
[90] | Hirschmann J , Schoffelen JM , Schnitzler A , van Gerven MAJ ((2017) ) Parkinsonian rest tremor can be detected accurately based on neuronal oscillations recorded from the subthalamic nucleus. Clin Neurophysiol 128: , 2029–2036. |
[91] | Yao L , Brown P , Shoaran M (2019) Resting tremor detection in Parkinson’s disease with machine learning and kalman filtering. IEEE Biomed Circuits Syst Conf 2018, BIOCAS.2018.8584721. |
[92] | Syrkin-Nikolau J , Koop MM , Prieto T , Anidi C , Afzal MF , Velisar A , Blumenfeld Z , Martin T , Trager M , Bronte-Stewart H ((2017) ) Subthalamic neural entropy is a feature of freezing of gait in freely moving people with Parkinson’s disease. Neurobiol Dis 108: , 288–297. |
[93] | Fim Neto A , de Luccas JB , Bianqueti BL , da Silva LR , Almeida TP , Takahata AK , Teixeira MJ , Figueiredo EG , Nasuto SJ , Rocha MSG , Soriano DC , Godinho F ((2022) ) Subthalamic low beta bursts differ in Parkinson’s disease phenotypes. Clin Neurophysiol 140: , 45–58. |
[94] | Chen Y , Gong C , Hao H , Guo Y , Xu S , Zhang Y , Yin G , Cao X , Yang A , Meng F , Ye J , Liu H , Zhang J , Sui Y , Li L ((2019) ) Automatic sleep stage classification based on subthalamic local field potentials. IEEE Trans Neural Syst Rehabil Eng 27: , 118–128. |
[95] | Christensen E , Abosch A , Thompson JA , Zylberberg J ((2019) ) Inferring sleep stage from local field potentials recorded in the subthalamic nucleus of Parkinson’s patients. J Sleep Res 28: , e12806. |
[96] | Golshan HM , Hebb AO , Hanrahan SJ , Nedrud J , Mahoor MH ((2018) ) A hierarchical structure for human behavior classification using STN local field potentials. J Neurosci Methods 293: , 254–263. |
[97] | Chrabaszcz A , Neumann WJ , Stretcu O , Lipski WJ , Bush A , Dastolfo-Hromack CA , Wang D , Crammond DJ , Shaiman S , Dickey MW , Holt LL , Turner RS , Fiez JA , Richardson RM ((2019) ) Subthalamic nucleus and sensorimotor cortex activity during speech production. J Neurosci 39: , 2698–2708. |
[98] | Golshan HM , Hebb AO , Mahoor MH ((2020) ) LFP-Net: A deep learning framework to recognize human behavioral activities using brain STN-LFP signals. J Neurosci Methods 335: , 108621. |
[99] | Sun Y , Wang Z , Hu K , Mo Y , Cao P , Hou X , He X , Zhang S , Xue S ((2021) ) α and θ oscillations in thesubthalamic nucleus are potential biomarkers for Parkinson’s disease with depressive symptoms. ParkinsonismRelat Disord 90: , 98–104. |
[100] | An Q , Yin Z , Ma R , Fan H , Xu Y , Gan Y , Gao Y , Meng F , Yang A , Jiang Y , Zhu G , Zhang J ((2023) ) Adaptive deep brain stimulation for Parkinson’s disease: Looking back at the past decade on motor outcomes. J Neurol 270: , 1371–1387. |
[101] | ClinicalTrails NCT04547712, Adaptive DBS algorithm for personalized therapy in Parkinson’s Disease, https://ClinicalTrials.gov/show/NCT04547712, Accessed March 14, 2023. |
[102] | Iturrate I , Martin S , Chavarriaga R , Orset B , Leeb R , Sobolewski A , Pralong E , Castro-Jiménez M , Benninger D , Bloch J , Millán JdR (2019) Beta-driven closed-loop deep brain stimulation can compromise human motor behavior in Parkinson’s Disease. bioRxiv, 696385. |
[103] | Neumann WJ , Staub-Bartelt F , Horn A , Schanda J , Schneider GH , Brown P , Kühn AA ((2017) ) Long term correlation of subthalamic beta band activity with motor impairment in patients with Parkinson’s disease. Clin Neurophysiol 128: , 2286–2291. |
[104] | Anderson RW , Wilkins KB , Parker JE , Petrucci MN , Kehnemouyi Y , Neuville RS , Cassini D , Trager MH , Koop MM , Velisar A , Blumenfeld Z , Quinn EJ , Henderson J , Bronte-Stewart HM ((2021) ) Lack of progression of beta dynamics after long-term subthalamic neurostimulation. Ann Clin Transl Neurol 8: , 2110–2120. |
[105] | Benis D , Haegelen C , Voruz P , Pierce J , Milesi V , Houvenaghel JF , Vérin M , Sauleau P , Grandjean D , Péron J ((2020) ) Subthalamic nucleus oscillations during vocal emotion processing are dependent of the motor asymmetry of Parkinson’s disease. Neuroimage 222: , 117215. |
[106] | Fischer P , Pogosyan A , Herz DM , Cheeran B , Green AL , Fitzgerald J , Aziz TZ , Hyam J , Little S , Foltynie T , Limousin P , Zrinzo L , Brown P , Tan H ((2017) ) Subthalamic nucleus gamma activity increases not only during movement but also during movement inhibition. Elife 6: , e23947. |
[107] | Johnson LA , Aman JE , Yu Y , Escobar Sanabria D , Wang J , Hill M , Dharnipragada R , Patriat R , Fiecas M , Li L , Schrock LE , Cooper SE , Johnson MD , Park MC , Harel N , Vitek JL ((2021) ) High-frequency oscillations in the pallidum: A pathophysiological biomarker in Parkinson’s disease? Mov Disord 36: , 1332–1341. |
[108] | Micheli F , Vissani M , Pecchioli G , Terenzi F , Ramat S , Mazzoni A ((2021) ) Impulsivity markers in parkinsonian subthalamic single-unit activity. Mov Disord 36: , 1435–1440. |
[109] | Thevathasan W , Sinclair NC , Bulluss KJ , McDermott HJ ((2020) ) Tailoring subthalamic nucleus deep brain stimulation for Parkinson’s disease using evoked resonant neural activity. Front Hum Neurosci 14: , 71. |
[110] | Dale J , Schmidt SL , Mitchell K , Turner DA , Grill WM ((2022) ) Evoked potentials generated by deep brain stimulation for Parkinson’s disease. Brain Stimul 15: , 1040–1047. |
[111] | Schmidt SL , Brocker DT , Swan BD , Turner DA , Grill WM ((2020) ) Evoked potentials reveal neural circuits engaged by human deep brain stimulation. Brain Stimul 13: , 1706–1718. |
[112] | Escobar Sanabria D , Aman JE , Zapata Amaya V , Johnson LA , Farooqi H , Wang J , Hill M , Patriat R , Sovell-Brown K , Molnar GF , Darrow D , McGovern R , Cooper SE , Harel N , MacKinnon CD , Park MC , Vitek JL ((2022) ) Controlling pallidal oscillations in real-time in Parkinson’s disease using evoked interference deep brain stimulation (eiDBS): Proof of concept in the human. Brain Stimul 15: , 1111–1119. |
[113] | Ahmadi N , Constandinou TG , Bouganis CS ((2021) ) Impact of referencing scheme on decoding performance of LFP-based brain-machine interface. J Neural Eng 18: , 016028. |
[114] | Nie Y , Guo X , Li X , Geng X , Li Y , Quan Z , Zhu G , Yin Z , Zhang J , Wang S ((2021) ) Real-time removal of stimulation artifacts in closed-loop deep brain stimulation. J Neural Eng 18: , 066031. |
[115] | Yao L , Brown P , Shoaran M ((2020) ) Improved detection of Parkinsonian resting tremor with feature engineering and Kalman filtering. Clin Neurophysiol 131: , 274–284. |
[116] | Gong R , Wegscheider M , Mühlberg C , Gast R , Fricke C , Rumpf JJ , Nikulin VV , Knösche TR , Classen J ((2021) ) Spatiotemporal features of β-γ phase-amplitude coupling in Parkinson’s disease derived from scalp EEG. Brain 144: , 487–503. |
[117] | Kondylis ED , Randazzo MJ , Alhourani A , Lipski WJ , Wozny TA , Pandya Y , Ghuman AS , Turner RS , Crammond DJ , Richardson RM ((2016) ) Movement-related dynamics of cortical oscillations in Parkinson’s disease and essential tremor. Brain 139: , 2211–2223. |
[118] | Buril J , Burilova P , Pokorna A , Balaz M ((2020) ) Use of high-density EEG in patients with Parkinson’s disease treated with deep brain stimulation. Biomed Pap Med Fac Univ Palacky Olomouc Czech Repub 164: , 366–370. |
[119] | Chen R , Berardelli A , Bhattacharya A , Bologna M , Chen KS , Fasano A , Helmich RC , Hutchison WD , Kamble N , Kühn AA , Macerollo A , Neumann WJ , Pal PK , Paparella G , Suppa A , Udupa K ((2022) ) Clinical neurophysiology of Parkinson’s disease and parkinsonism. Clin Neurophysiol Pract 7: , 201–227. |
[120] | Crowell AL , Ryapolova-Webb ES , Ostrem JL , Galifianakis NB , Shimamoto S , Lim DA , Starr PA ((2012) ) Oscillations in sensorimotor cortex in movement disorders: An electrocorticography study. Brain 135: , 615–630. |
[121] | Abbasi O , Hirschmann J , Storzer L , Özkurt TE , Elben S , Vesper J , Wojtecki L , Schmitz G , Schnitzler A , Butz M ((2018) ) Unilateral deep brain stimulation suppresses alpha and beta oscillations in sensorimotor cortices. Neuroimage 174: , 201–207. |
[122] | Pauls KAM , Korsun O , Nenonen J , Nurminen J , Liljeström M , Kujala J , Pekkonen E , Renvall H ((2022) ) Cortical beta burst dynamics are altered in Parkinson’s disease but normalized by deep brain stimulation. Neuroimage 257: , 119308. |
[123] | Merk T , Peterson V , Lipski WJ , Blankertz B , Turner RS , Li N , Horn A , Richardson RM , Neumann WJ ((2022) ) Electrocorticography is superior to subthalamic local field potentials for movement decoding in Parkinson’s disease. Elife 11: , e75126. |
[124] | Swann NC , de Hemptinne C , Miocinovic S , Qasim S , Wang SS , Ziman N , Ostrem JL , San Luciano M , Galifianakis NB , Starr PA ((2016) ) Gamma oscillations in the hyperkinetic state detected with chronic human brain recordings in Parkinson’s disease. J Neurosci 36: , 6445–6458. |
[125] | Herff C , Schultz T ((2016) ) Automatic speech recognition from neural signals: A focused review. Front Neurosci 10: , 429. |
[126] | de Hemptinne C , Ryapolova-Webb ES , Air EL , Garcia PA , Miller KJ , Ojemann JG , Ostrem JL , Galifianakis NB , Starr PA ((2013) ) Exaggerated phase-amplitude coupling in the primary motor cortex in Parkinson disease. Proc Natl Acad Sci U S A 110: , 4780–4785. |
[127] | Malekmohammadi M , AuYong N , Ricks-Oddie J , Bordelon Y , Pouratian N ((2018) ) Pallidal deep brain stimulationmodulates excessive cortical high β phase amplitude coupling in Parkinson disease. Brain Stimul 11: , 607–617. |
[128] | de Hemptinne C , Swann NC , Ostrem JL , Ryapolova-Webb ES , San Luciano M , Galifianakis NB , Starr PA ((2015) ) Therapeutic deep brain stimulation reduces cortical phase-amplitude coupling in Parkinson’s disease. Nat Neurosci 18: , 779–786. |
[129] | Yin Z , Zhu G , Liu Y , Zhao B , Liu D , Bai Y , Zhang Q , Shi L , Feng T , Yang A , Liu H , Meng F , Neumann WJ , Kühn AA , Jiang Y , Zhang J ((2022) ) Cortical phase-amplitude coupling is key to the occurrence and treatment of freezing of gait. Brain 145: , 2407–2421. |
[130] | Cole SR , van der Meij R , Peterson EJ , de Hemptinne C , Starr PA , Voytek B ((2017) ) Nonsinusoidal beta oscillations reflect cortical pathophysiology in Parkinson’s disease. J Neurosci 37: , 4830–4840. |
[131] | Wang X , Hao M , Chou CH , Zhang X , Pan Y , Sun B , Bai M , Dai C , Lan N ((2022) ) The effects of deep brain stimulation on motor unit activities in Parkinson’s disease based on high-density surface EMG analysis. Annu Int Conf IEEE Eng Med Biol Soc 2022: , 682–685. |
[132] | Lu R , Xu Y , Li X , Fan Y , Zeng W , Tan Y , Ren K , Chen W , Cao X ((2020) ) Evaluation of wearable sensor devices in Parkinson’s disease: A review of current status and future prospects. Parkinsons Dis 2020: , 4693019. |
[133] | Vescio B , Quattrone A , Nisticò R , Crasá M , Quattrone A ((2021) ) Wearable devices for assessment of tremor. Front Neurol 12: , 680011. |
[134] | Mancini M , Bloem BR , Horak FB , Lewis SJG , Nieuwboer A , Nonnekes J ((2019) ) Clinical and methodological challenges for assessing freezing of gait: Future perspectives. Mov Disord 34: , 783–790. |
[135] | Herron JA , Thompson MC , Brown T , Chizeck HJ , Ojemann JG , Ko AL ((2017) ) Chronic electrocorticography for sensing movement intention and closed-loop deep brain stimulation with wearable sensors in an essential tremor patient. J Neurosurg 127: , 580–587. |
[136] | Keogh A , Argent R , Anderson A , Caulfield B , Johnston W ((2021) ) Assessing the usability of wearable devices to measure gait and physical activity in chronic conditions: A systematic review. J Neuroeng Rehabil 18: , 138. |
[137] | O’Day J , Lee M , Seagers K , Hoffman S , Jih-Schiff A , Kidziński Ł , Delp S , Bronte-Stewart H ((2022) ) Assessinginertial measurement unit locations for freezing of gait detection and patient preference. J NeuroengRehabil 19: , 20. |
[138] | Lim ACY , Natarajan P , Fonseka RD , Maharaj M , Mobbs RJ ((2022) ) The application of artificial intelligence and custom algorithms with inertial wearable devices for gait analysis and detection of gait-altering pathologies in adults: A scoping review of literature. Digit Health 8: , 20552076221074128. |
[139] | Mancini M , Shah VV , Stuart S , Curtze C , Horak FB , Safarpour D , Nutt JG ((2021) ) Measuring freezing of gait during daily-life: An open-source, wearable sensors approach. J Neuroeng Rehabil 18: , 1. |
[140] | Erb MK , Karlin DR , Ho BK , Thomas KC , Parisi F , Vergara-Diaz GP , Daneault JF , Wacnik PW , Zhang H , Kangarloo T , Demanuele C , Brooks CR , Detheridge CN , Shaafi Kabiri N , Bhangu JS , Bonato P ((2020) ) mHealth and wearable technology should replace motor diaries to track motor fluctuations in Parkinson’s disease. NPJ Digit Med 3: , 6. |
[141] | di Biase L , Summa S , Tosi J , Taffoni F , Marano M , Cascio Rizzo A , Vecchio F , Formica D , Di Lazzaro V , Di Pino G , Tombini M ((2018) ) Quantitative analysis of bradykinesia and rigidity in Parkinson’s disease. Front Neurol 9: , 121. |
[142] | Ferreira-Sánchez MDR , Moreno-Verdú M , Cano-de-la-Cuerda R ((2020) ) Quantitative measurement of rigidity inParkinson’s disease: A systematic review. Sensors (Basel) 20: , 880. |
[143] | Fabbri M , Caldas AC , Ramos JB , Sanchez-Ferro Á , Antonini A , Ružička E , Lynch T , Rascol O , Grimes D , Eggers C , Mestre TA , Ferreira JJ ((2020) ) Moving towards home-based community-centred integrated care inParkinson’s disease. Parkinsonism Relat Disord 78: , 21–26. |
[144] | Li D , Zhang C , Gault J , Wang W , Liu J , Shao M , Zhao Y , Zeljic K , Gao G , Sun B ((2017) ) Remotely programmed deep brain stimulation of the bilateral subthalamic nucleus for the treatment of primary Parkinson disease: A randomized controlled trial investigating the safety and efficacy of a novel deep brain stimulation system. Stereotact Funct Neurosurg 95: , 174–182. |
[145] | Wang S , Wang X , Zhao M , Li T , Zhang C , Wang M , Luan G , Guan Y ((2020) ) Long-term efficacy and cognitive effects of voltage-based deep brain stimulation for drug-resistant essential tremor. Clin Neurol Neurosurg 194: , 105940. |
[146] | Zhan A , Mohan S , Tarolli C , Schneider RB , Adams JL , Sharma S , Elson MJ , Spear KL , Glidden AM , Little MA , Terzis A , Dorsey ER , Saria S ((2018) ) Using smartphones and machine learning to quantify Parkinson disease severity: The Mobile Parkinson Disease Score. JAMA Neurol 75: , 876–880. |
[147] | Angeles P , Tai Y , Pavese N , Wilson S , Vaidyanathan R ((2017) ) Automated assessment of symptom severity changes during deep brain stimulation (DBS) therapy for Parkinson’s disease. IEEE Int Conf Rehabil Robot 2017: , 1512–1517. |
[148] | Elkouzi A , Vedam-Mai V , Eisinger RS , Okun MS ((2019) ) Emerging therapies in Parkinson disease - repurposed drugsand new approaches. Nat Rev Neurol 15: , 204–223. |
[149] | Price JB , Rusheen AE , Barath AS , Rojas Cabrera JM , Shin H , Chang SY , Kimble CJ , Bennet KE , Blaha CD , Lee KH , Oh Y ((2020) ) Clinical applications of neurochemical and electrophysiological measurements for closed-loop neurostimulation. Neurosurg Focus 49: , E6. |
[150] | Fougère M , van der Zouwen CI , Boutin J , Neszvecsko K , Sarret P , Ryczko D (2021) Optogenetic stimulation of glutamatergic neurons in the cuneiform nucleus controls locomotion in a mouse model of Parkinson’s disease. Proc Natl Acad Sci U S A 118. |
[151] | Zhang Y , Roy DS , Zhu Y , Chen Y , Aida T , Hou Y , Shen C , Lea NE , Schroeder ME , Skaggs KM , Sullivan HA , Fischer KB , Callaway EM , Wickersham IR , Dai J , Li XM , Lu Z , Feng G ((2022) ) Targeting thalamic circuits rescues motor and mooddeficits in PD mice. Nature 607: , 321–329. |
[152] | Tinkhauser G , Moraud EM ((2021) ) Controlling clinical states governed by different temporal dynamics with closed-loop deep brain stimulation: A principled framework. Front Neurosci 15: , 734186. |
[153] | Tinkhauser G , Pogosyan A , Little S , Beudel M , Herz DM , Tan H , Brown P ((2017) ) The modulatory effect of adaptive deep brain stimulation on beta bursts in Parkinson’s disease. Brain 140: , 1053–1067. |
[154] | Tinkhauser G , Pogosyan A , Tan H , Herz DM , Kühn AA , Brown P ((2017) ) Beta burst dynamics in Parkinson’s disease OFF and ON dopaminergic medication. Brain 140: , 2968–2981. |
[155] | Lofredi R , Okudzhava L , Irmen F , Brücke C , Huebl J , Krauss JK , Schneider GH , Faust K , Neumann WJ , Kühn AA ((2023) ) Subthalamic beta bursts correlate with dopamine-dependent motor symptoms in 106 Parkinson’s patients. NPJ Parkinsons Dis 9: , 2. |
[156] | Kumari LS , Kouzani AZ ((2021) ) Phase-dependent deep brain stimulation: A review. Brain Sci 11: , 414. |
[157] | Cagnan H , Brittain JS , Little S , Foltynie T , Limousin P , Zrinzo L , Hariz M , Joint C , Fitzgerald J , Green AL , Aziz T , Brown P ((2013) ) Phase dependent modulation of tremor amplitude in essential tremor through thalamic stimulation. Brain 136: , 3062–3075. |
[158] | Cagnan H , Pedrosa D , Little S , Pogosyan A , Cheeran B , Aziz T , Green A , Fitzgerald J , Foltynie T , Limousin P , Zrinzo L , Hariz M , Friston KJ , Denison T , Brown P ((2017) ) Stimulating at the right time: Phase-specific deep brain stimulation. Brain 140: , 132–145. |
[159] | Reis C , He S , Pogosyan A , Haliasos N , Low HL , Misbahuddin A , Aziz T , Fitzgerald J , Green AL , Denison T , Cagnan H (2022) Phase-specific deep brain stimulation revisited: Effects of stimulation on postural and kinetic tremor. medRxiv, 2022.2006.2016.22276451. |
[160] | Ibrahim A , Zhou Y , Jenkins ME , Luisa Trejos A , Naish MD ((2020) ) The design of a Parkinson’s tremor predictor and estimator using a hybrid convolutional-multilayer perceptron neural network. Annu Int Conf IEEE Eng Med Biol Soc 2020: , 5996–6000. |
[161] | Romagnolo A , Zibetti M , Lenzi M , Vighetti S , Pongmala C , Artusi CA , Montanaro E , Imbalzano G , Rizzone MG , Lopiano L ((2021) ) Low frequency subthalamic stimulation and event-related potentials in Parkinson disease. Parkinsonism Relat Disord 82: , 123–127. |
[162] | Louie KH , Petrucci MN , Grado LL , Lu C , Tuite PJ , Lamperski AG , MacKinnon CD , Cooper SE , Netoff TI ((2021) ) Semi-automated approaches to optimize deep brain stimulation parameters in Parkinson’s disease. J Neuroeng Rehabil 18: , 83. |
[163] | Sasaki F , Oyama G , Sekimoto S , Nuermaimaiti M , Iwamuro H , Shimo Y , Umemura A , Hattori N ((2021) ) Closed-loop programming using external responses for deep brain stimulation in Parkinson’s disease. Parkinsonism Relat Disord 84: , 47–51. |
[164] | Boutet A , Madhavan R , Elias GJB , Joel SE , Gramer R , Ranjan M , Paramanandam V , Xu D , Germann J , Loh A , Kalia SK , Hodaie M , Li B , Prasad S , Coblentz A , Munhoz RP , Ashe J , Kucharczyk W , Fasano A , Lozano AM ((2021) ) Predicting optimal deep brain stimulation parameters for Parkinson’s disease using functional MRI and machine learning. Nat Commun 12: , 3043. |
[165] | Eguchi K , Shirai S , Matsushima M , Kano T , Ichikawa T , Yamazaki K , Hamauchi S , Sasamori T , Seki T , Kitagawa M , Shiraishi H , Houkin K , Sasaki H , Yabe I ((2021) ) Chronic deep brain stimulation reduces cortical β-γ phase amplitude-coupling in patients with Parkinson’s disease. Parkinsonism Relat Disord 89: , 148–150. |
[166] | Cartmill T , Skvarc D , Bittar R , McGillivray J , Berk M , Byrne LK ((2021) ) Deep brain stimulation of the subthalamic nucleus in Parkinson’s disease: A meta-analysis of mood effects. Neuropsychol Rev 31: , 385–401. |
[167] | Mehanna R , Bajwa JA , Fernandez H , Wagle Shukla AA ((2017) ) Cognitive impact of deep brain stimulation on Parkinson’s disease patients. Parkinsons Dis 2017: , 3085140. |
[168] | Reich MM , Hsu J , Ferguson M , Schaper F , Joutsa J , Roothans J , Nickl RC , Frankemolle-Gilbert A , Alberts J , Volkmann J , Fox MD ((2022) ) A brain network for deep brain stimulation induced cognitive decline in Parkinson’s disease. Brain 145: , 1410–1421. |
[169] | Lam J , Lee J , Liu CY , Lozano AM , Lee DJ ((2021) ) Deep brain stimulation for Alzheimer’s disease: Tackling circuit dysfunction. Neuromodulation 24: , 171–186. |