Identifying research gaps: A review of virtual patient education and self-management
Abstract
BACKGROUND:
Avatars in Virtual Reality (VR) can not only represent humans, but also embody intelligent software agents that communicate with humans, thus enabling a new paradigm of human-machine interaction.
OBJECTIVE:
The research agenda proposed in this paper by an interdisciplinary team is motivated by the premise that a conversation with a smart agent avatar in VR means more than giving a face and body to a chatbot. Using the concrete communication task of patient education, this research agenda is rather intended to explore which patterns and practices must be constructed visually, verbally, para- and nonverbally between humans and embodied machines in a counselling context so that humans can integrate counselling by an embodied VR smart agent into their thinking and acting in one way or another.
METHODS:
The scientific literature in different bibliographical databases was reviewed. A qualitative narrative approach was applied for analysis.
RESULTS:
A research agenda is proposed which investigates how recurring consultations of patients with healthcare professionals are currently conducted and how they could be conducted with an embodied smart agent in immersive VR.
CONCLUSIONS:
Interdisciplinary teams consisting of linguists, computer scientists, visual designers and health care professionals are required which need to go beyond a technology-centric solution design approach. Linguists’ insights from discourse analysis drive the explorative experiments to identify test and discover what capabilities and attributes the smart agent in VR must have, in order to communicate effectively with a human being.
1.Introduction
Chatbots and conversational intelligent software agents, so called smart agents, implemented as text- or speech-based dialog systems are used in many domains and are becoming more and more part of our everyday life. They substitute communication with a human being and act as a natural language interface to more or less intelligent machines. For purely functional dialogues such as making an appointment or reserving a table in a restaurant, they are very efficient. But what about a complex communication task, such as advising a chronically ill patient who has to learn to live with diabetes?
In the current situation we have probably become particularly aware of how valuable and rich personal face-to-face conversations are. Social co-presence, the use of nonverbal and paraverbal communication as well as the possibility to interpret and react to the counterparts’ state of mind make face-to-face communication for many situations highly effective and pleasant. Multimedia chats pick up on this by giving conversational smart agents a human appearance in the form of avatars. Immersive Virtual Reality (VR) goes one step further and allows us to interact with smart agents in a three-dimensional world that creates a feeling of social co-presence. However, such a realistic setting, close to a real-life face-to-face communication raises numerous design challenges. A smart agent who talks to a diabetes patient may be given a humanoid shape, facial expressions and gestures, but this does not mean that the smart agent can interpret the mental state, body language and linguistic nuances such as irony or pauses of his human counterpart and react adequately. As human beings how do we want to communicate with an artificially intelligent being in virtual space? To what extent is communication different when a person communicates with a smart agent in VR? Do we act according to the same pattern of language behavior with a smart agent as with a human counterpart? Do we expect a simulation of an interpersonal face-to-face communication or will new practices for verbal and nonverbal communication with artificially intelligent “beings” emerge?
2.Relevance
To capture the full complexity of a conversation as a social interaction, research and design on embodied conversational smart agents have to be interdisciplinary and linked to a relevant and challenging communication task. The use case of patient education is predestined to investigate the effectiveness of communication. Healthcare professionals not only convey knowledge and skills in interventions, but also aim to initiate and recognize behavioral changes. One case, where patient education is of great importance is diabetes. The management of patients with diabetes belongs to one of the major challenges for healthcare worldwide. In 2000, the global estimate of diabetes prevalence was 151 million. According to the International Diabetes Federation the estimates have since shown alarming increases, tripling to the 2019 estimate of 463 million [1]. The prevalence of diabetes in men lies by 6.9% and 4.4% in women [2]. Improved patient self-management support and increased access to expertise are considered highly effective interventions for patients suffering from a chronical disease like diabetes [3]. Researching and prototyping technology-enabled interventions based on immersive VR and smart agents is therefore in line with the efforts to search for new ways to prevent and treat diabetes more successfully [2]. If we succeed in developing and validating a design approach for this challenging communication task, the basis for further conversational applications based on embodied smart agents would be created.
3.State of research
Immersive VR and conversational smart agents embodied by avatars enable new possibilities for humans to communicate with intelligent machines. This research agenda deals with the design space of this novel form of human-machine interaction from four perspectives. This is done with the intention of supplementing the predominantly technologically oriented work with empirical, applied, interdisciplinary aspects:
1. Linguistics (social interaction and language);
2. Design (visualization and perception);
3. Technology (systems behavior); and
4. Healthcare (the chosen use case: patient education for patients with diabetes).
In the following section the four views on this challenge and the respective state of research are described.
3.1Linguistics: Social interactions and language in (diabetes) consultations
Diabetes consultations can be seen as a specific type of the interaction type “consultation” [4]. As a communicative practice with specific patterns in a very limited time frame with few participants, it requires actors who have been socialized into the specific cultural practice and language pattern for a smooth process [5]; in this case a healthcare professional and a patient with diabetes is involved. A systematic examination of the communication between healthcare professionals and patients with diabetes has not yet taken place in linguistics. The most recent study was conducted by Bührig, Fienemann and Schlickau [6] and deals with the link between negative quality of diabetes consultations as perceived by patients on the one hand and the lack of patient action following medical advice (called compliance, adherence or empowerment) on the other hand. They also tried to measure the communicative quality of the diabetes consultations. In particular, they pointed out that the patient’s integrity zone was often infringed by the utterances of the healthcare professionals and that the communication was affected by different stocks of knowledge [6]. The transfer of knowledge from healthcare professional to patient, which can be understood in a broader sense as patient education [7, 8] seems to make up a large part of the diabetes consultation. Like doctor-patient communication, diabetes consultations are therefore located somewhere between shared-decision-making and informed-choice [9, 10, 11] in which healthcare professionals appear as consultants, who make [12].
The choice of the use case of patient education for this proposed research agenda is motivated by the fact that the willingness of patients to change their behavior accordingly (therapy adherence) can be positively influenced by successful communication between medical consultants and patients, which is supported by various studies [13, 14, 15, 16].
So far, the relevant literature on diabetes counselling has only examined communication between people. However, in our proposed agenda one of the conversation participants is an intelligent machine a smart agent operating in a virtual reality. Nevertheless we can still call it an interaction. The human participants of the conversation perceive their counterpart. Through the avatar’s reactions, the human partners construct a conversation situation, they recognize in a certain sense that they themselves are perceived. To a certain degree, a conversation situation is constructed in this way as an interaction [17, 18]. It is not surprising that this condition alters communication radically. (Effective) communication is made up of different units: verbal, para- and nonverbal behavior like mimicry, gestures, speech expression or eye contact are equally important and can even contribute to a better understanding of utterances [19]. The theory practices and patterns in linguistic also emphasizes, that grammatical and lexical structures are significant, interact with voice, gaze, facial expressions, gestures, movements in space and handling objects [5] and far more. It is often presumed that the acceptance of technically simulated, nonverbal behavior by viewers depends on the look and behavior of the avatar (e.g. gender, posture, figure, skin color, age and size or degree of realism and anthropomorphism) [20, 21, 22]. Some studies have shown that the more human a smart agent looks and acts, the more human traits are attributed to him [23]. Realistic-looking avatars provide stronger social presence [24] and participants show the similar language behavior as if they were talking to a human being [25]. So far, this humanoid appearance and behavior was interpreted in such a way that it has a positive effect on communication efficiency [26]. Previous linguistic research on human-machine communication, especially in the field of computational linguistics, has therefore focused primarily on the imitation of face-to-face communication between humans [27].
In the field of discourse analysis research which focuses on verbal data, also focuses on this optimization behavior on the human side and less on optimization principles for voice-controlled dialog systems [28]. In general, conversations, which take place between people and computers additionally, are preceded in each case by specific media and their affordances. They frame specific conversation situations and their requirements [29, 30]. These settings (e.g., a phone call), are decisive and limit the choice of communicative-linguistic possibilities and their stylistic realization. On the other hand, however, they also open up new possibilities for use. For example, in a conversation with an artificially generated interlocutor, metacommunicative information and units are used in a different strategic sense than in a situation in which metacommunicative units can be captured and processed.
3.2Design: Visualization and perception of avatars
User acceptance of smart agents depends on behavioral realism as well as on visual and auditive realism. Research in this area dates back to the early days of VR [20], but results regarding user efficiency and acceptance in dependence of visual realism are inconclusive [31, 32]. However, studies have shown that an avatar’s behavioral fidelity should be coherent with its visual fidelity as well as the visual fidelity of its surroundings in order to avoid eery and alienating effects [33]. Published studies in the field VR are almost exclusively conducted in the fields of computer sciences, engineering and psychology and use the term “visual realism” to describe the fidelity of avatar appearance [34]. In this research agenda, a design perspective is applied and therefore, the term “visual abstraction” is used to emphasize the conscious approach of creating a coherent look and feel and reducing human appearance to a level which is best accepted by patients and most likely to guarantee successful communication.
3.3Technology: Implementation of affective smart agent avatars in VR
We are witnessing a continuously growing prevalence of Augmented and Virtual Reality (mixed reality) technologies in both, private and business application domains. One factor fueling this development has been the gaming industry, which is producing ever cheaper and more powerful hardware as well as ever more advanced runtime environments and development tools [35]. These enable the design and development of novel mixed reality applications for other purposes than gaming. Meeting and running a conversation in virtual space represents such a field of application. In contrast to conversations mediated by video or telephone Virtual Reality creates a feeling of social presence and provides for a digital space in which the participants can do more than just see and talk to each other [35, 36, 37]. Essential for social presence in VR are avatars, who can talk, move, manipulate objects and thus engage in more complex forms of interaction [38]. Avatars in VR cannot only represent human participants, but they can embody intelligent software agents, and thereby enable a new paradigm of human-machine interaction. Embodied conversational agents could become a part of people’s everyday life, but to date they are not fully capable of understanding and realizing the seemingly “natural” and socialized aspects of human face-to-face communications [39]. One reason for this is the fact that communication not only consists in successfully conveying content verbally. Effective communication also includes non- and paraverbal signals expressed by means of body language such as gestures, facial expressions and tone of voice. Avatars in Virtual Reality are able to convey non- and paraverbal signals and have the potential to raise the effectiveness of virtual communication [40]. However due to technical limitations animated motions of current embodied conversational agents still have little variations and quickly become repetitive [41]. Furthermore, a smart agent also needs to receive and interpret the nonverbal and paraverbal signals of its human counterpart. But in today’s immersive Virtual Reality environments the transmission of non- and paraverbal information of the human participant is only partially supported. One reason for this is the fact that users need to wear a Head Mounted Display (HMD), which covers part of the face. Motion capturing of body and hands is realized by commercial products, but sensing and interpreting facial expressions relies on experimental hardware solutions like electromyography (EMG) sensors attached to the HMD to track facial muscle movements [42]. The key message of the technology-related state of research in this field is that contributions from different research areas and functionality of existing development tools (SDKs) can be combined, transferred, applied and tested to realize the novel idea of an experimental system to analyze and design dialogues with an avatar in immersive VR. Key building blocks include sentiment, tone and voice analysis: voice analysis [43, 44, 45, 46, 47, 48, 49], sentiment analysis [50, 51, 52, 53]; gesture recognition by vision and hand tracking [54, 55, 56, 57, 58, 59] and speech recognition and analysis [60, 61]. The overall envisioned architecture in the context of this research agenda is inspired by existing work on virtual human communication architectures and embodied conversational agents [62, 63, 64, 65, 66].
3.4Health: Educational interventions for diabetes patients by healthcare professionals
Improved patient self-management support and increased access to expertise are considered highly effective interventions for patients suffering from a chronical condition like diabetes [3, 4, 5, 6, 7, 8, 9, 10, 11, 12, 13, 14, 15, 16, 17, 18, 19, 20, 21, 22, 23, 24, 25, 26, 27, 28, 29, 30, 31, 32, 33, 34, 35, 36, 37, 38, 39, 40, 41, 42, 43, 44, 45, 46, 47, 48, 49, 50, 51, 52, 53, 54, 55, 56, 57, 58, 59, 60, 61, 62, 63, 64, 65, 66, 67]. Self-management is understood as a collective term for patient-centered intervention strategies [68]. It comprises symptom management, the concrete embedding of this in daily life of the patient and their family and finally the development of implementation strategies for successful symptom management in order to recognize disease crises in time and to get help [68]. The American Association of Diabetes Educators describes seven self-care behaviors in patients with diabetes [69]. At the core of these are lifestyle-influencing behaviors in the use of food and daily (sport) activities. Adherence to prescribed medication is just as central. Healthcare professionals support the patients and their families through appropriate self-management support. In doing so the focus is not only on knowledge transfer [70]. Patient education is also about initiating behavioral change and positively influencing clinical and health outcomes [70]. For this purpose a person-centred attitude and supportive activities are needed, among other things, to help the patient solve problems [71, 72, 73]. Antonovsky described such self-management support to increase the patient’s resilience with “sense of coherence” as early as 1979 [74, 75]. According to Antonovsky, supportive activities must be comprehensible to the patient, make sense in their everyday life and be applicable in their everyday practice. These requirements are considered as critical constraints in the proposed research agenda.
4.Research goal and proposed research agenda
In essence, this research agenda focuses on the question of how humans speak to machines and, related to this, what the machine must be able to do to advise humans in repetitive contexts, so that the overall goal can be achieved, that communication does not fail. For decades natural language processing research has concentrated on anticipating and reproducing human speech as faithfully as possible [76]. Significant progress has been made in recent years through quantitative approaches (e.g., IBM Project Debater [77]), but it is still only an inadequate approximation of the highly complex reality. Conversational agents mimicking human voices and paraverbals perfectly, such as Google Duplex, pass the Turing test for purely functional dialogues, such as making an appointment without people realizing they talk to a machine [78]. Immersive Virtual Reality now allows us to go one level further in this “imitation game”. It is obvious and tempting to perfect a conversation with an embodied smart agent in VR towards an imitation of a real face-to-face conversation. But on the one hand, there are still some technical challenges in the way of such an endeavor [41] and on the other hand, we ask ourselves whether and to what extent imitation is necessary and desirable in order to make a machine avatar an effective counterpart in complex dialogues. We are therefore pursuing a different approach: the point for us is not that avatars of smart agents look, speak and behave “like humans”, but rather the question of which patterns and practices must be constructed verbally, para- and nonverbally between humans and embodied machines in a counselling context so that humans can integrate counselling by the machine into their thinking and acting in one way or another. This may not require “all” aspects of a social interaction to be fulfilled in communication with a smart agent in VR, at best reduced patterns are sufficient to prevent failure. If one avoids failures in consulting sequences, depending on one’s perspective, it is also possible to become a success, so to speak. However, the judgement about “success” and “failure” can only be made in the context of a specific communication task. Educational interventions for diabetes patients with diabetes mellitus type 2 are a highly complex communication task that nevertheless have some potential of standardization. Healthcare professionals apply communication strategies, such as motivational interviewing to initiate behavioral changes of patients [79, 80]. They have frameworks to evaluate complex interventions [81] and are trained to recognize such changes based on the nonverbal and verbal utterances of the patient during a consultation. Furthermore, they can relate these observations to measurable health outcomes, such as blood sugar levels or number of steps as an indicator for physical activity.
Figure 1 outlines the interplay of the four involved disciplines and their research contributions. The goal is to identify, create and validate the linguistic, visual and functional prerequisites for effective communication with smart agents in VR using the case of patient education for diabetes. To this end, the following research questions are to be addressed.
Figure 1.
Scientific content and focus by discipline aligned to research questions (RQ).
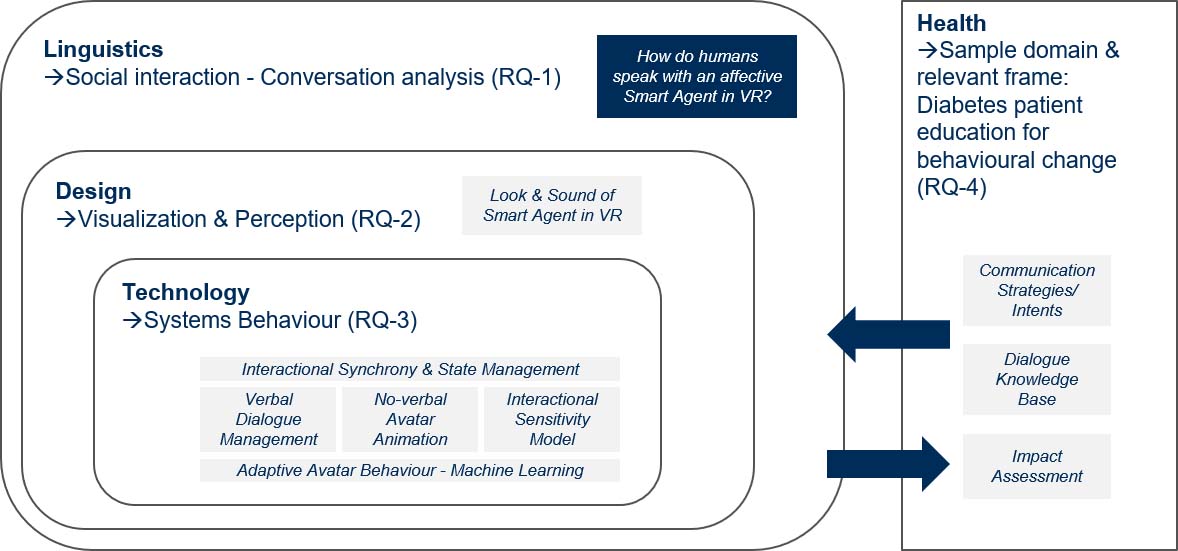
4.1RQ-1: Linguistics (social interaction and language)
4.1.1Research questions
To what extent is communication different when a person communicates with a smart agent in VR? Do we exhibit the same language behavior interacting with a smart agent as with a human counterpart? How do we as human beings want to communicate with an artificially intelligent being in virtual space? Do we expect a simulation of an interpersonal face-to-face communication or will new practices for verbal and nonverbal communication with artificially intelligent beings emerge?
4.1.2Corresponding approach
Linguists’ insights from ethnomethodologically oriented conversation analysis drive the explorative experiments by identifying the discourse structures, language patterns and social practices of co-present conversations between healthcare professionals and diabetes patients: I. human
4.1.3Expected generation of knowledge
Generation of a corpus for future conversation-analytical examinations of the conversation-type diabetes consultations.
4.1.4Scientific impact
Testing the suitability of VR as an experimental environment for linguistic analyses.
4.2RQ-2: Design (visualization and perception)
4.2.1Research questions
How does the degree of visual abstraction influence user acceptance? How close does nonverbal communication behavior (mimics, gestures, posture) have to match that of a real human in order to clearly express a smart agent’s simulated emotions?
4.2.2Corresponding approach
A morphological analysis frames the experiments to explore various possible constellations of appearance and capabilities of the smart agent, its environment and interaction with the patient in a systematic manner [82]. A morphological approach supports the required multi-dimensional view of and provides a structure to explore the non-quantifiable possible solutions [83].
4.2.3Expected generation of knowledge
Validated set of variables for the visual design space of a conversational smart agent avatar.
4.2.4Scientific impact
Parameterizable environment to test the impact of avatars with varying degrees of abstractions on users in any context.
4.3RQ-3: Technology (systems behavior)
4.3.1Research questions
What are the relevant nonverbal verbal, affective and cognitive capabilities the smart agent must have, in order to communicate with a human being in a way so that the conversation is effective and desirable, or at least does not fail?
4.3.2Corresponding approach
Starting from the premise that enabling a dialogue with a smart agent in VR does not mean to imitate a human-to-human conversation, we cannot formulate relevant scenarios of a target state or requirements for a solution, because we can only speculate how the solution could be and how humans may behave when they communicate with increasingly intelligent embodied machines in virtual spaces. Therefore “classical” deterministic requirements, which are tested in terms of hypotheses based on a prototype, are not useful. Explorative experiments, on the other hand, are more suitable to test and discover what capabilities and attributes the smart agent in VR must have, in order to communicate with a human being in a way so that the conversation does not fail. Explorative experiments are particularly helpful in the context of human-machine interaction whenever we introduce new technologies into society, but we cannot yet assess their impact as socio-technical system [84, 85]. To ensure desirability a participatory human-centered design approach framed by self-determination theory [86] is applied: What does the patient but also the healthcare professional win and lose, in terms of autonomy, competence, relatedness by transferring communication to VR and by involving a smart agent? What motivates a human being to communicate with an intelligent machine?
4.3.3Expected generation of knowledge
Validated functional capabilities for dialogues with affective smart agents in VR.
4.3.4Scientific impact
Experimental system for dialogue analysis and design in immersive VR, usable and to be developed on a continuous basis
4.4RQ-4: Health
4.4.1Research question
What is the required interaction with a smart agent avatar in immersive VR during an initial consultation to promote physical activity in patients with diabetes mellitus type 2, non-insulin-dependent, with grade 1 obesity? How is behavioral change talked about in consultations?
4.4.2Corresponding approach
Evaluating the outcome of complex interventions [81]: instructing the patient on lifestyle-changing lifestyles in patients with type 2 diabetes on being active based on the seven self-care behaviors used by the American Association of Diabetes Educators (AADE) [69, 70] for assessing patients and outcomes
4.4.3Expected generation of knowledge
Generation of a corpus for future patient-centered self-management diabetes chronic-care lifestyle instructions in a smart agent VR; Smart agent VR-instructions on lifestyle-changing lifestyles in patients with type 2 diabetes on being active and taking medication based on the seven self-care behaviors of the AADE [69, 70]; Smart agent VR-interventions taking into account four critical time points to assess, to provide and to adjust self-management support and education. Thus patterns of complex interventions and corresponding patient responses in consultations of chronically ill patients can be developed.
4.4.4Scientific impact
Assessing the value and the usability of immersive VR as an environment to evaluate complex interventions for behavioral change in diabetes chronic care management.
5.Conclusions
This research agenda investigates how recurring consultations of patients with healthcare professionals are currently conducted and how they could be conducted with an embodied smart agent in immersive VR. The interdisciplinary team consisting of linguists, computer scientists, visual designers and health care professionals will go beyond a technology-centric solution design approach. Linguists’ insights from discourse analysis drive the explorative experiments to identify test and discover what capabilities and attributes the smart agent in VR must have, in order to communicate effectively with a human being. This is done with the aim of developing a prototype for patient education in VR. The social added value and potential cost savings are evident given the current increase in diabetes. The need to standardize the process of managing the care of people with diabetes while still allowing for individualized care could not be timelier. Researching and prototyping technology-enabled educational interventions based on immersive VR and smart agents is, therefore, highly relevant and future-oriented.
Conflict of interest
None to report.
References
[1] | IDF, International Diabetes Federation. IDF Diabetes Atlas 9th edition 2019. (2019) ; [cited April 11, 2020]. Available from: https://www.diabetesatlas.org/en/. |
[2] | WHO, World Health Organisation. WHO |
[3] | Wagner EH, Austin BT, Von Korff M. Organizing care for patients with chronic illness. The Milbank Quarterly. (1996) ; 74: : 511–544. doi: 10.2307/3350391. |
[4] | Pick I. Beraten in Interaktion: eine gesprächslinguistische Typologie des Beratens. Frankfurt am Main: Peter Lang Edition, (2017) . |
[5] | Deppermann A, Feilke H, Linke A. Sprachliche und kommunikative Praktiken: Eine Annäherung aus linguistischer Sicht. (2016) . |
[6] | Bührig K, Fienemann J, Schlickau S. On Certain Characteristics of “Diabetes Consultations.” In Hohenstein C, Lévy-Tödter M, eds., Multilingual Healthcare: A Global View on Communicative Challenges. Wiesbaden: Springer Fachmedien Wiesbaden, (2020) . doi: 10.1007/978-3-658-27120-6. |
[7] | Duke S-AS, Colagiuri S, Colagiuri R. Individual patient education for people with type 2 diabetes mellitus. Cochrane Database of Systematic Reviews. (2009) . doi: 10.1002/14651858.CD005268.pub2. |
[8] | Swedish Council on Health Technology Assessment. Patient Education in Managing Diabetes: A Systematic Review. Stockholm: Swedish Council on Health Technology Assessment (SBU), (2009) . |
[9] | Koerfer A, Obliers R, Köhle K. Entscheidungsdialog zwischen Arzt und Patient. Modelle der Beziehungsgestaltung in der Medizin. In Neies M, Spranz-Fogasy T, Ditz S, eds., Psychosomatische Gesprächsführung in der Frauenheilkunde. Ein interdisziplinärer Ansatz zu verbalen Intervention. 1st ed. Stuttgart: Wissenschaftliche Verlagsgesellschaft, (2005) , pp. 137–157. |
[10] | Mathijssen EGE, van den Bemt BJF, van den Hoogen FHJ, Popa CD, Vriezekolk JE. Interventions to support shared decision making for medication therapy in long term conditions: a systematic review. Patient Education and Counseling. (2020) ; 103: : 254–265. doi: 10.1016/j.pec.2019.08.034. |
[11] | Tamhane S, Rodriguez-Gutierrez R, Hargraves I, Montori VM. Shared decision-making in diabetes care. Current Diabetes Reports. (2015) ; 15: : 112. doi: 10.1007/s11892-015-0688-0. |
[12] | Becker M, Spranz-Fogasy T. Empfehlen und Beraten: Ärztliche Empfehlungen im Therapieplanungsprozess. In Pick I, ed., Beraten in Interaktion. Eine gesprächslinguistische Typologie des Beratens. Frankfurt am Main: Lang, (2017) , pp. 163–184. |
[13] | Cvengros JA, Christensen AJ, Cunningham C, Hillis SL, Kaboli PJ. Patient preference for and reports of provider behavior: impact of symmetry on patient outcomes. Health Psychology: Official Journal of the Division of Health Psychology, American Psychological Association. (2009) ; 28: : 660–667. doi: 10.1037/a0016087. |
[14] | Naik AD, Kallen MA, Walder A, Street RL. Improving hypertension control in diabetes mellitus the effects of collaborative and proactive health communication. Circulation. (2008) ; 117: : 1361–1368. doi: 10.1161/CIRCULATIONAHA.107.724005. |
[15] | Parchman ML, Flannagan D, Ferrer RL, Matamoras M. Communication competence, self-care behaviors and glucose control in patients with type 2 diabetes. Patient Education and Counseling. (2009) ; 77: : 55–59. doi: 10.1016/j.pec.2009.03.006. |
[16] | Zolnierek KBH, Dimatteo MR. Physician communication and patient adherence to treatment: a meta-analysis. Medical Care. (2009) ; 47: : 826–834. doi: 10.1097/MLR.0b013e31819a5acc. |
[17] | Goffman E. The neglected situation. American Anthropologist. (1964) ; 66: : 133–136. |
[18] | Hausendorf H. Interaktionslinguistik. In Eichinger L, ed., Sprachwissenschaft im Fokus. Positionsbestimmungen und Perspektiven. Berlin, Boston: De Gruyter, (2015) , pp. 43–69. |
[19] | Pabst-Weinschenk M. “… und was sagt die Stimme?”: Sprechwissenschaftliche Analysen zur Wirkung para-und extraverbalen Ebenen der Arzt-Patienten-Kommunikation. In Bechmann S, ed., Sprache und Medizin: Interdisziplinäre Beiträge zur medizinischen Sprache und Kommunikation. Berlin: Frank & Timme GmbH, (2017) , pp. 181–212. |
[20] | Garau M, Slater M, Vinayagamoorthy V, Brogni A, Steed A, Sasse MA. The Impact of Avatar Realism and Eye Gaze Control on Perceived Quality of Communication in a Shared Immersive Virtual Environment. In Proceedings of the SIGCHI Conference on Human Factors in Computing Systems. New York, NY, USA: ACM, (2003) , pp. 529–536. doi: 10.1145/642611.642703. |
[21] | Roth D, Lugrin J-L, Galakhov D, Hofmann A, Bente G, Latoschik ME, Fuhrmann A. Avatar realism and social interaction quality in virtual reality. 2016 IEEE Virtual Reality (VR). (2016) , 277–278. doi: 10.1109/VR.2016.7504761. |
[22] | Waltemate T, Gall D, Roth D, Botsch M, Latoschik ME. The impact of avatar personalization and immersion on virtual body ownership, presence, and emotional response. IEEE Transactions on Visualization and Computer Graphics. (2018) ; 24: : 1643–1652. doi: 10.1109/TVCG.2018.2794629. |
[23] | Krämer NC, Bente G. Virtuelle Helfer: Embodied Conversational Agents in der Mensch-Computer-Interaktion. In Krämer NC, Bente G, Petersen A, eds., Virtuelle Realitäten. Göttingen: Hogrefe-Verlag GmbH & Co. KG, (2002) , pp. 203–226. |
[24] | Kwon JH, Powell J, Chalmers A. How level of realism influences anxiety in virtual reality environments for a job interview. International Journal of Human-Computer Studies. (2013) ; 71: : 978–987. doi: 10.1016/j.ijhcs.2013.07.003. |
[25] | Heyselaar E, Hagoort P, Segaert K. In dialogue with an avatar, language behavior is identical to dialogue with a human partner. Behavior Research Methods. (2017) ; 49: : 46–60. doi: 10.3758/s13428-015-0688-7. |
[26] | Bente G, Krämer NC. Virtuelle Gesten: VR-Einsatz in der nonverbalen Kommunikationsforschung. In Bente G, Krämer NC, Petersen A, eds., Virtuelle Realitäten. Göttingen: Hogrefe-Verlag GmbH & Co. KG, (2002) , pp. 81–108. |
[27] | Hausser RR. Grundlagen der Computerlinguistik: Mensch-Maschine-Kommunikation in natürlicher Sprache. Springer-Verlag, (2013) . |
[28] | Thar E. “Ich habe Sie leider nicht verstanden”: linguistische Optimierungsprinzipien für die mündliche Mensch-Maschine-Interaktion. (2015) . |
[29] | Faraj S, Azad B. The Materiality of Technology: An Affordance Perspective. In Leonardi PM, Nardi BA, Kallinikos J, eds., Materiality and Organizing: Social Interaction in a Technological World. Oxford: Oxford University Press, (2012) , pp. 237–258. |
[30] | Evans SK, Pearce KE, Vitak J, Treem JW. Explicating affordances: a conceptual framework for understanding affordances in communication research. Journal of Computer-Mediated Communication. (2017) ; 22: : 35–52. doi: 10.1111/jcc4.12180. |
[31] | Catrambone R. Anthropomorphic Agents as a User Interface Paradigm? Experimental Findings and a Framework for Research. (2002) . |
[32] | Lugrin J-L, Wiedemann M, Bieberstein D, Latoschik ME. Influence of avatar realism on stressful situation in VR. 2015 IEEE Virtual Reality (VR). (2015) , pp. 227–228. doi: 10.1109/VR.2015.7223378. |
[33] | Schwind V. Implications of the uncanny valley of avatars and virtual characters for human-computer interaction, Dissertation, Universität Stuttgart, (2018) . |
[34] | Hvass J, Larsen O, Vendelbo K, Nilsson N, Nordahl R, Serafin S. Visual realism and presence in a virtual reality game. In 2017 3DTV Conference: The True Vision – Capture, Transmission and Display of 3D Video (3DTV-CON). (2017) , pp. 1–4. doi: 10.1109/3DTV.2017.8280421. |
[35] | Anthes C, García-Hernández RJ, Wiedemann M, Kranzlmüller D. State of the art of virtual reality technology. In 2016 IEEE Aerospace Conference. (2016) , pp. 1–19. doi: 10.1109/AERO.2016.7500674. |
[36] | Greenwald SW, Wang Z, Funk M, Maes P. Investigating Social Presence and Communication with Embodied Avatars in Room-Scale Virtual Reality. In Beck D, Allison C, Morgado L, Pirker J, Khosmood F, Richter J, Gütl C, eds., Immersive Learning Research Network. Springer International Publishing, (2017) , pp. 75–90. |
[37] | Lombard M, Ditton T. At the heart of it all: the concept of presence. Journal of Computer-Mediated Communication. (1997) ; 3: . doi: 10.1111/j.1083-6101.1997.tb00072.x. |
[38] | Campbell AG, Holz T, Cosgrove J, Harlick M, O’Sullivan T. Uses of Virtual Reality for Communication in Financial Services: A Case Study on Comparing Different Telepresence Interfaces: Virtual Reality Compared to Video Conferencing. In Arai K, Bhatia R, eds., Advances in Information and Communication. Cham: Springer International Publishing, (2020) , pp. 463–481. doi: 10.1007/978-3-030-12388-8_33. |
[39] | Geraci F. Design and implementation of embodied conversational agents, Rutgers University – School of Graduate Studies, (2019) . doi: 10.7282/t3-3wbz-9431. |
[40] | Dimatteo MR, Taranta A. Nonverbal communication and physician-patient rapport: an empirical study. Professional Psychology. (1979) ; 10: : 540–547. doi: 10.1037/0735-7028.10.4.540. |
[41] | Borer D, Lutz D, Guay M. Animating an Autonomous 3D Talking Avatar. arXiv:1903.05448 [cs]. (2019) . |
[42] | Lou J, Wang Y, Nduka C, Hamedi M, Mavridou I, Wang F-Y, Yu H. Realistic facial expression reconstruction for VR HMD users. IEEE Transactions on Multimedia. (2020) ; 22: : 730–743. doi: 10.1109/TMM.2019.2933338. |
[43] | Benba A, Jilbab A, Hammouch A. Voice assessments for detecting patients with neurological diseases using PCA and NPCA. International Journal of Speech Technology. (2017) ; 20: : 673–683. doi: 10.1007/s10772-017-9438-9. |
[44] | Dasgupta PB. Detection and analysis of human emotions through voice and speech pattern processing. arXiv preprint arXiv:1710.10198. (2017) . |
[45] | Eyben F, Scherer KR, Schuller BW, Sundberg J, André E, Busso C, Devillers LY, Epps J, Laukka P, Narayanan SS, Truong KP. The geneva minimalistic acoustic parameter set (GeMAPS) for voice research and affective computing. IEEE Transactions on Affective Computing. (2016) ; 7: : 190–202. doi: 10.1109/TAFFC.2015.2457417. |
[46] | Gómez-García JA, Moro-Velázquez L, Godino-Llorente JI. On the design of automatic voice condition analysis systems. Part II: Review of speaker recognition techniques and study on the effects of different variability factors. Biomedical Signal Processing and Control. (2019) ; 48: : 128–143. doi: 10.1016/j.bspc.2018.09.003. |
[47] | Swetha BC, Divya S, Kavipriya J, Kavya R, Rasheed AA. A novel voice based sentimental analysis technique to mine the user driven reviews. (2017) . |
[48] | Vocal Emotion Recognition Test by Empath. Vocal Emotion Recognition Test by Empath. n.d.; [cited February 19, 2020]. Available from: https://webempath.net/lp-eng/. |
[49] | Watson Tone Analyzer. (2016) ; [cited February 19, 2020]. Available from: https://www.ibm.com/watson/services/tone-analyzer/. |
[50] | Cambria E. Affective computing and sentiment analysis. IEEE Intelligent Systems. (2016) ; 31: : 102–107. doi: 10.1109/MIS.2016.31. |
[51] | Sentiment Analysis. DeepAI. n.d.; [cited February 19, 2020]. Available from: https://deepai.org/machine-learning-model/sentiment-analysis. |
[52] | Soleymani M, Garcia D, Jou B, Schuller B, Chang S-F, Pantic M. A survey of multimodal sentiment analysis. Image and Vision Computing. (2017) ; 65: : 3–14. doi: 10.1016/j.imavis.2017.08.003. |
[53] | Zhang L, Wang S, Liu B. Deep learning for sentiment analysis: a survey. WIREs Data Mining and Knowledge Discovery. (2018) ; 8: : e1253. doi: 10.1002/widm.1253. |
[54] | Chiu P, Takashima K, Fujita K, Kitamura Y. Pursuit Sensing: Extending Hand Tracking Space in Mobile VR Applications. Symposium on Spatial User Interaction. New Orleans, LA, USA: Association for Computing Machinery, (2019) , pp. 1–5. doi: 10.1145/3357251.3357578. |
[55] | Diliberti N, Peng C, Kaufman C, Dong Y, Hansberger JT. Real-Time Gesture Recognition Using 3D Sensory Data and a Light Convolutional Neural Network. In Proceedings of the 27th ACM International Conference on Multimedia. Nice, France: Association for Computing Machinery, (2019) , pp. 401–410. doi: 10.1145/3343031.3350958. |
[56] | Liu H, Wang L. Gesture recognition for human-robot collaboration: a review. International Journal of Industrial Ergonomics. (2018) ; 68: : 355–367. doi: 10.1016/j.ergon.2017.02.004. |
[57] | Mitra S, Acharya T. Gesture recognition: a survey. IEEE Transactions on Systems, Man, and Cybernetics, Part C (Applications and Reviews). (2007) ; 37: : 311–324. doi: 10.1109/TSMCC.2007.893280. |
[58] | VIVE Hand Tracking SDK. VIVE Developer Resources. n.d.; [cited February 18, 2020]. Available from: https://developer.vive.com/resources/knowledgebase/vive-hand-tracking-sdk/. |
[59] | Zhu G, Zhang L, Shen P, Song J. Multimodal gesture recognition using 3-D convolution and convolutional LSTM. IEEE Access. (2017) ; 5: : 4517–4524. doi: 10.1109/ACCESS.2017.2684186. |
[60] | Abdul-Kader SA, Woods JC. Survey on chatbot design techniques in speech conversation systems. International Journal of Advanced Computer Science and Applications. (2015) ; 6: . |
[61] | Graves A, Mohamed A, Hinton G. Speech recognition with deep recurrent neural networks. In 2013 IEEE International Conference on Acoustics, Speech and Signal Processing. (2013) , pp. 6645–6649. doi: 10.1109/ICASSP.2013.6638947. |
[62] | Hartholt A, Fast E, Reilly A, Whitcup W, Liewer M, Mozgai S. Ubiquitous Virtual Humans: A Multi-platform Framework for Embodied AI Agents in XR. In 2019 IEEE International Conference on Artificial Intelligence and Virtual Reality (AIVR). (2019) , pp. 308–3084. doi: 10.1109/AIVR46125.2019.00072. |
[63] | Kolkmeier J, Bruijnes M, Reidsma D, Heylen D. An ASAP Realizer-Unity3D Bridge for Virtual and Mixed Reality Applications. In Beskow J, Peters C, Castellano G, O’Sullivan C, Leite I, Kopp S, eds., Intelligent Virtual Agents. Cham: Springer International Publishing, (2017) , pp. 227–230. doi: 10.1007/978-3-319-67401-8_27. |
[64] | Kopp S, Krenn B, Marsella S, Marshall AN, Pelachaud C, Pirker H, Thórisson KR, Vilhjálmsson H. Towards a Common Framework for Multimodal Generation: The Behavior Markup Language. In Gratch J, Young M, Aylett R, Ballin D, Olivier P, eds., Intelligent Virtual Agents. Berlin, Heidelberg: Springer, (2006) , pp. 205–217. doi: 10.1007/11821830_17. |
[65] | van Welbergen H, Bergmann K, Buschmeier H, Kahl S, de Kok I, Sadeghipour A, Yaghoubzadeh R, Kopp S. Architectures and Standards for IVAs at the Social Cognitive Systems Group. In Workshop on Architectures and Standards for IVAs, held at the “14th International Conference on Intelligent Virtual Agents (IVA 2014)”: Proceedings. (2014) . |
[66] | Cafaro A, Vilhjálmsson HH, Bickmore T, Heylen D, Pelachaud C. Representing Communicative Functions in SAIBA with a Unified Function Markup Language. In Bickmore T, Marsella S, Sidner C, eds., Intelligent Virtual Agents. Cham: Springer International Publishing, (2014) , pp. 81–94. doi: 10.1007/978-3-319-09767-1_11. |
[67] | Wagner EH. Chronic disease management: what will it take to improve care for chronic illness? Effective Clinical Practice: ECP. (1998) ; 1: : 2–4. |
[68] | Kickbusch I, Maag I. Health Literacy. In Heggenhougen K, Quah S, eds., International Encyclopedia of Public Health, 1st ed,. San Diego: Elsevier, (2008) , pp. 204–211. doi: 10.1016/B978-012373960-5.00633-X. |
[69] | American Association of Diabetes Educators, AADE. AADE 7 |
[70] | American Association of Diabetes Educators. An Effective Model of Diabetes Care and Education: Revising the AADE 7 Self-Care Behaviors. The Diabetes Educator. (2020) ; 46: : 139–160. doi: 10.1177/0145721719894903. |
[71] | Coulter A. Engaging patients in healthcare. Maidenhead: Open University Press, (2011) . |
[72] | Lundahl BW, Kunz C, Brownell C, Tollefson D, Burke BL. A meta-analysis of motivational interviewing: twenty-five years of empirical studies. Research on Social Work Practice. (2010) ; 20: : 137–160. doi: 10.1177/1049731509347850. |
[73] | Miller W, Rollnick S. Motivierende Gesprächsführung, 3rd ed. Freiburg/Breisgau: Lambertus, (2015) . |
[74] | Antonovsky A. Health, stress, and coping. San Francisco: Jossey-Bass, (1979) . |
[75] | Eriksson M, Lindström B. Validity of Antonovsky’s sense of coherence scale: a systematic review. Journal of Epidemiology & Community Health. (2005) ; 59: : 460–466. doi: 10.1136/jech.2003.018085. |
[76] | Hirschberg J, Manning CD. Advances in natural language processing. Science. (2015) ; 349: : 261–266. doi: 10.1126/science.aaa8685. |
[77] | IBM. IBM Research AI – Project Debater. IBM Research AI. (2018) ; [cited May 9, 2020]. Available from: https://www.research.ibm.com/artificial-intelligence/project-debater//. |
[78] | Nebel B. Turing-Test. In Liggieri K, Müller O, eds., Mensch-Maschine-Interaktion: Handbuch zu Geschichte – Kultur – Ethik. Stuttgart: J.B. Metzler, (2019) , pp. 304–306. doi: 10.1007/978-3-476-05604-7_57. |
[79] | Christie D, Channon S. The potential for motivational interviewing to improve outcomes in the management of diabetes and obesity in paediatric and adult populations: a clinical review. Diabetes, Obesity and Metabolism. (2014) ; 16: : 381–387. doi: 10.1111/dom.12195. |
[80] | Kröger C, Velten-Schurian K, Batra A. Motivierende gesprächsführung zur aktivierung von verhaltensänderungen. DNP – Der Neurologe und Psychiater. (2016) ; 17: : 50–58. doi: 10.1007/s15202-016-1377-9. |
[81] | Craig P, Dieppe P, Macintyre S, Michie S, Nazareth I, Petticrew M. Developing and evaluating complex interventions: the new Medical Research Council guidance. The BMJ. (2008) ; 337: . doi: 10.1136/bmj.a1655. |
[82] | Zwicky F. The Morphological Approach to Discovery, Invention, Research and Construction. In Zwicky F, Wilson AG, eds., New Methods of Thought and Procedure. Berlin, Heidelberg: Springer, (1967) , pp. 273–297. doi: 10.1007/978-3-642-87617-2_14. |
[83] | Rakov DL, Sinyev AV. The structural analysis of new technical systems based on a morphological approach under uncertainty conditions. Journal of Machinery Manufacture and Reliability. (2015) ; 44: : 650–657. doi: 10.3103/S1052618815070110. |
[84] | Kroes P. Experiments on socio-technical systems: the problem of control. Science and Engineering Ethics. (2016) ; 22: : 633–645. doi: 10.1007/s11948-015-9634-4. |
[85] | Steinle F. Entering new fields: exploratory uses of experimentation. Philosophy of Science. (1997) ; 64: : S65–S74. doi: 10.1086/392587. |
[86] | Ryan RM, Deci EL. A Self-Determination Theory Perspective on Social, Institutional, Cultural, and Economic Supports for Autonomy and Their Importance for Well-Being. In Chirkov VI, Ryan RM, Sheldon KM, eds., Human Autonomy in Cross-Cultural Context: Perspectives on the Psychology of Agency, Freedom, and Well-Being. Dordrecht: Springer Netherlands, (2011) , pp. 45–64. doi: 10.1007/978-90-481-9667-8_3. |