Association between serum magnesium and common complications of diabetes mellitus
Abstract
BACKGROUND:
Magnesium ion, as important cation in the human body, involved in various enzymatic reactions, glucose transport and insulin release. Now diabetes mellitus and diabetic complications have become important public health problems around the world.
OBJECTIVE:
This paper explores the association between concentration levels of serum magnesium and common complications and comorbidities of diabetes mellitus and other biochemical indexes.
METHODS:
There are 1217 eligible patients selected from 14,317 cases of diabetic hospitalization patients from January 2010 to December 2011. Random forest algorithm was applied to assess the importance of various biochemical indexes and to perform diabetic complications prediction.
RESULTS:
The research results showed that low concentration of serum magnesium and four common diabetic complications – diabetic retinopathy, diabetic nephropathy, diabetic neuropathy and diabetic macroangiopathy – exists association, but no obvious correlation with other comorbidities like hypertension.
CONCLUSIONS:
The specific factors of four common diabetic complications were selected from the biochemical indexes to provide a reference direction for further research.
1.Introduction
Diabetes mellitus and its complications have become important public health problems around the world, and the incidence of diabetes mellitus has reached the rate of epidemic. The International Diabetes Federation estimates that an additional 7 million diabetics will increase each year over the next decade [1]. Type 2 diabetes is characterized by insulin resistance and beta cell dysfunction, affecting 5.9% of adults worldwide, covering 80% of developing countries [1]. Diabetes is a chronic disease that requires continuous medical care to prevent acute complications and reduce chronic complications. Whether suffering from complications or the extent of illness varies from person to person. Although the clinical manifestations of diabetes complications are diverse, but there are some common pathophysiological characteristics exist in these syndromes, trace elements are one of the important factors. There are currently studies on the potential prevention or treatment of trace elements for types 1 and 2 diabetes mellitus and their common complications. However, the level of magnesium in patients with diabetes mellitus and its complications, research results are not consistent [2, 3, 4]. Magnesium ion is the second most important intracellular cation, after potassium ion. There are about 21 to 28 g of magnesium in adults, of which 99% are present in the cells, and only 1% is present in the extracellular fluid. The distribution is subdivided, then the presence of magnesium in the three parts of the body: about 65% in the mineral phase of the bone, 34% in the cell space, only 1% distributed in the extracellular fluid. The serum magnesium contains three forms of presence: with the protein binding (of which 25% binding to albumin and 8% binding to globulin), chelate (12%), and the ionized portion of the metabolic response (i.e. magnesium ion: 55%). Magnesium is mainly absorbed in the small intestine and is excreted through the kidney [5]. Kidney can help the plasma magnesium concentration to maintain a normal level at 1.7–2.4 mg/dl. Magnesium ion plays an important role in carbohydrate metabolism and insulin response, as a cofactor involved in transmembrane transport of glucose and release of insulin. Studies have found that magnesium deficiency is usually associated with endocrine and metabolic disorders, especially type 2 diabetes mellitus [6].
In recent years, big data analysis and data mining are gradually aroused attention, especially in the field of medical and health care, the use of data mining is extremely extensive. Since the adoption of the Hospital Information System (HIS) in the hospitals, a large number of patient medical data stored by the HIS can be analyzed by data mining methods. This study is based on the data of inpatients in the general hospital of the PLA from 2010–2011, and patients with diabetes mellitus (both types 1 and 2) were selected for cross-sectional study. The related factors of diabetes mellitus and common complications of diabetes mellitus were analyzed by data mining method, and the patients with common complications of diabetes mellitus were predicted according to the extracted characteristic variables. The results of this study can be used to explore the related factors of common complications of diabetes mellitus, to provide new ideas for further research.
2.Materials and methods
2.1Data sources
The research data are based on the hospitalization data sets from the clinical data center from January 2010 to December 2011, with “diabetes” as keywords to select inpatient with a history of diabetes. Medical records of these patients during hospitalization generated the research database. The data items provided by the database include patient ID, sex, age, weight, pulse, and blood pressure, frequency of medication, urine routine, biochemical examination, and pathological diagnosis and so on.
2.2Inclusion criteria and data extraction
There are 14,317 cases of diabetic hospitalization patients in the database from January 2010 to December 2011, with a total of 82,017 hospital medical records. We established the following exclusion criteria: (1) records without making a full set of biochemical examination; (2) doctor clinical diagnosis contains uncertain terms or symbols; (3) non-first-time inspection records after hospitalization (to reduce regulatory intervention for index value during hospitalization); (4) patients with multiple diabetic complications or comorbidities (in order to analyze the specificity factors of single certain diabetic complication). According to the above exclusion criteria, 1217 patients were selected, including 774 males and 443 females, aged 7–92 years, with an average age of 60.54
Table 1
Various parameters in the research data set
Type | Parameter |
---|---|
Biochemistry | Alanine transaminase, total protein, aspartate transaminase, serum albumin, |
Demography | Age, gender |
Diagnosis | Diabetes mellitus, diabetic retinopathy, diabetic nephropathy, diabetic neuropathy, diabetic ketoacidosis, diabetic macroangiopathy, diabetic foot, hypertension, other diseases |
2.3Conventional measurement and principle of serum magnesium
Methyl thymol blue colorimetric method is widely used in conventional magnesium measurement, the basic principle is that serum magnesium, calcium ions can bind with methyl thymol blue dye in a basic solution to generate a blue-violet complex, addition of ethylene glycol diethyl ether diamine tetraacetic acid (EGTA) can mask the interference of calcium ion, compared with the same standard solution, the content of magnesium can be calculated [7].
2.4Statistical methods
The mean
In this paper, R version 3.2.2 was used for data analysis, with 0.01 as a significant standard for statistical significance.
2.5Random forest
Random forest is an ensemble method that can be used for many tasks such as classification, regression, and anomaly detection. During the training phase, the decision trees are constructed with different random variables. Through the learning of training set data, each decision tree obtains the respective results. For classification problems, the output of random forest uses the majority voting method, taking the most categories of all decision tree results as the final predictive classification [8].
For the kth tree in the random forest, a random vector
Wherein
In a random forest,
The above equation explains why random forest will not overfit as the increase of the decision tree, but will produce a certain range of generalization error.
The random forest uses the bagging algorithm to generate different training sets, and uses stochastic feature selection method to generate a new decision tree for each new training set without pruning. In each new training set, about one-third samples of the original training set does not appear, and these data are referred to as out-of-bag (OOB) data [10]. As the decision tree increases the error rate decreases, the OOB value tends toward the current error rate and is unbiased.
Random forest gives two methods, an average reduction in accuracy and an average reduction of the Gini index, to assess the importance of variables. The more the accuracy or the Gini index decreases, the more important the variables are [11, 12]. In this study, the two methods were used to judge the importance of variables, and then the associated factors of each research item were ranked according to the importance of the variables.
3.Results
3.1Preliminary statistics
In this study, preliminary statistics on hospital records of 1217 patients were conducted. The serum magnesium distribution of patients with diabetes mellitus is shown in Fig. 1.
As can be seen from Fig. 1, both male and female patients with diabetes, the distribution of serum magnesium levels in male and female patients with diabetes are roughly the same, serum magnesium value of female diabetic patients ranges slightly larger than the male span. By Shapiro-Wilk test, serum magnesium values of both male and female diabetic patients are subject to normal distribution. The kurtosis is 3.67 and 5.01, respectively, and the skewness is 0.71 and 0.82, respectively. According to analysis of variance, there was no significant difference in serum magnesium between male and female, the F-value was 0.7829 and the p-value was 0.003304. Serum magnesium distribution of male and female patients was calculated and is shown in Table 2.
Table 2
Statistics of serum magnesium concentration
Range (mmol/L) | Number of patients | |
---|---|---|
Male (N | Female (N | |
Mg | 0 | 0 |
0.6 | 91 | 77 |
0.8 | 627 | 330 |
1.0 | 50 | 30 |
1.2 | 4 | 2 |
Mg | 1 | 1 |
Mg: serum magnesium.
Figure 1.
Serum magnesium distribution of different gender diabetic patients.
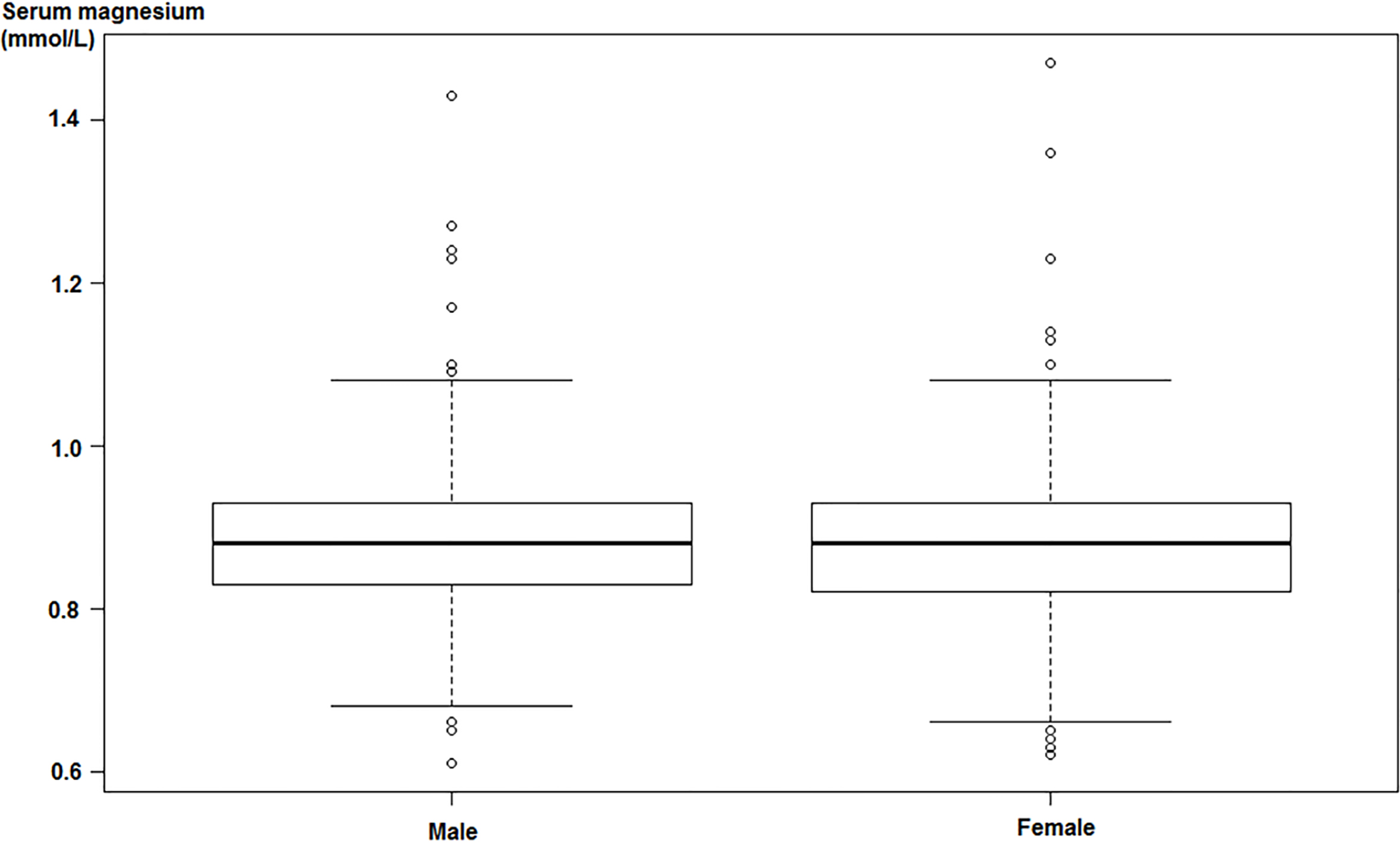
3.2Associated factors of serum magnesium
According to the current biochemical examination indices and the pathological diagnosis, the clas- sification of serum magnesium concentration was learned by establishing random forest, and the importance of each index was estimated. Prediction results showed that for all patients, age was strongly correlated with serum magnesium concentration. The mean decrease Gini value of age is 3.3003. For male patients, the relevant biochemical indicators of serum magnesium are glucose, urea, potassium, creatinine, total protein and direct bilirubin (sorted by variable importance, the same below). Male diabetic patients, whose serum magnesium concentration in the normal but low range (that is, the range 0.6–0.8 mmol/L, the same below), showed stronger association with three types of diabetic complications – diabetic nephropathy, diabetic retinopathy and diabetic neuropathy, than other comorbidities. The mean decrease Gini value for the abovementioned three complications were more than twice those for other complications and comorbidities. That is, compare to other diseases, male patients are more susceptible to the above three complications. For female patients, the relevant biochemical indicators of serum magnesium are lactate dehydrogenase, creatinine, urea, glucose, sodium and high density lipoprotein cholesterol. Female diabetic patients, whose serum magnesium concentration in the normal but low range showed stronger association with diabetic retinopathy, diabetic nephropathy and diabetic macroangiopathy. The mean decrease Gini value for the three complications were at least more than double those of other complications and comorbidities.
3.3Specific factors analysis of common diabetic complications
The results show that serum magnesium is the associated factor of male and female diabetic patients suffering from diabetic retinopathy, diabetic nephropathy. For diabetic neuropathy, serum magnesium is only associated with male diabetic patients. For diabetic macroangiopathy, serum magnesium is only associated with female diabetic patients. In this study, the risk factors of the above four common diabetic complications were studied by establishing the random forest model. According to the variable importance obtained from the random forest model, biochemical indices have strong correlation with the disease were regarded as the specific factors of the disease. The results are shown in Table 3. Combining serum magnesium and the specific factors, the patients with diabetes complications were predicted, so as to complete the reliability test of specific factors.
Table 3
Specific factors of common diabetic complications
Complication | Specific factors |
---|---|
Diabetic retinopathy (Male) | Creatinine, urea, total cholesterol and direct bilirubin |
Diabetic retinopathy (Female) | Total bilirubin, Serum lipoprotein, total protein and chloride |
Diabetic nephropathy (Male) | Creatinine, serum uric acid, serum albumin, urea, homocysteine, total protein, direct bilirubin and lactate dehydrogenase |
Diabetic nephropathy (Female) | Creatinine, total protein, alkaline phosphatase, creatinine and chloride |
Diabetic neuropathy (Male) | Serum sialic acid, high density lipoprotein cholesterol, glucose, urea, glycated serum protein and low density lipoprotein cholesterol |
Diabetic macroangiopathy (Female) |
Table 4
Evaluation of prediction model
Complications | Accuracy | Sensitivity | Specificity | Youden index |
---|---|---|---|---|
Diabetic retinopathy (Male) | 0.886 | 1.000 | 0.878 | 0.878 |
Diabetic retinopathy (Female) | 0.843 | 1.000 | 0.826 | 0.826 |
Diabetic nephropathy (Male) | 0.901 | 0.900 | 0.901 | 0.801 |
Diabetic nephropathy (Female) | 0.853 | 0.833 | 0.854 | 0.687 |
Diabetic neuropathy (Male) | 0.896 | 1.000 | 0.887 | 0.887 |
Diabetic macroangiopathy (Female) | 0.784 | 0.571 | 0.800 | 0.371 |
In this study, stratified sampling and under sample were conducted within 566 male and 335 female inpatient records in 2011 to obtain a training data set. These sampling methods are beneficial to enhance the stability and accuracy of classification prediction under the circumstance of ensuring the full learning of the current training set. Different classification prediction models of diabetic complications in male and female were constructed by random forest algorithm, the models were verified on the test data set, which consists of 202 male cases and 102 females recorded in 2010.
This research adopts the sensitivity, specificity and accuracy and Youden index to evaluate prediction effect. Sensitivity is the likelihood that the patient is correctly identified as the person with the disease. Specificity refers to the likelihood that a non-complication patient is correctly identified as non-ill. Accuracy means the percentage of accurate results in all prediction results. Youden index can be calculated by Youden index
4.Discussion
4.1Associated factors of serum magnesium
Magnesium is the fourth highest cation in the human body, the level of serum magnesium is usually maintained in a very stable state in healthy individuals. As a second messenger of insulin action, magnesium plays an important role in insulin sensitivity and glucose metabolism. Magnesium deficiency can lead to decreased insulin sensitivity. Although serum magnesium value does not always reflect the overall state of magnesium metabolism, it is closely related to the intracellular free magnesium status.
The results of this study showed that serum magnesium level was strongly related to the age of patients with diabetes. Guerrero-Romero and Rodríguez-Morán [13] found that age, alcohol consumption and use of diuretics were risk factors for low serum magnesium levels in diabetic patients. It is similar to the results of this study. As the results of this study show, serum magnesium of the men with diabetes is related to glucose, urea, potassium, creatinine, total protein and direct bilirubin. Ma et al. [14] found that fasting insulin, glucose, systolic pressure, smoking were related to serum magnesium among diabetic patients, which is similar to the results of this study. However, the results of Randadass et al. [6] showed that there was no statistically significant correlation between serum magnesium and fasting blood glucose and postprandial blood glucose in patients with type 2 diabetes mellitus, which was contradictory to this result, this may be related to the fact that serum magnesium cannot fully reflect the magnesium content in the body.
The results of this study show that the serum magnesium of the female diabetic patients is related to lactate dehydrogenase, creatinine, urea, glucose, sodium and high density lipoprotein cholesterol. Takagi et al. [3] found that magnesium intake increased the creatinine and uric acid levels in diabetic patients, and decreased the percentage of body fat. Sales et al. [15] also showed that creatinine was associated with serum magnesium, which is similar to the result of this study. Barbagallo et al. [5] found that after oral administration of magnesium, total cholesterol and low-density lipoprotein may increase while high-density lipoprotein cholesterol decrease in patients with type 2 diabetes, which is similar to the results of this study. The research results of Guerrero-Romero et al. [16] also indicated that after oral administration of MgCl
4.2Association between common diabetic complications and serum magnesium
The deficiency of magnesium can lead to a variety of diseases, such as impaired glucose tolerance, insulin resistance, abnormal lipid metabolism and so on. Some studies have revealed that abnormal magnesium metabolism may lead to the occurrence and development of diabetes, diabetic microvascular and macroangiopathy complications [15].
The results of this study show that serum magnesium is an associated factor of diabetic nephropathy and diabetic retinopathy complications. Previous studies [17, 18, 19] found that lower serum magnesium level can affect the development and progression of diabetic retinopathy and diabetic nephropathy, which is similar to the results of this study.
The results also show that serum magnesium is a risk factor for male diabetic patients suffering from diabetic neuropathy and female diabetic patients suffering from diabetic macrovascular disease. The study of Agrawal et al. [1] found that low concentrations of magnesium can oxidize low-density lipoprotein cholesterol, and then promote the occurrence of atherosclerosis and diabetic macrovascular, which verifies the possibility of our findings. However, Ma et al. [14] found that low concentrations of serum magnesium promoted the occurrence of cardiovascular disease, hypertension, atherosclerosis and diabetes, which contradicted against part of the results of this study (the results of this study show that there is no significant association between hypertension and concentration of serum magnesium). This may be due to the number of cases with hypertension is small in this study, resulting in strong correlation not be detected. But Floege [20] found that the relationship between serum magnesium concentration and hypertension is weak.
Sales and Pedrosa [21] found that magnesium concentration in erythrocytes was associated with diabetic neuropathy. Barbagallo et al. [22] found that insulin response and diabetes were related to the concentration of magnesium in erythrocytes. Because the decrease of serum magnesium concentration presents after severe deficiency of magnesium in the body, and the reduction of serum magnesium is closely related to intracellular magnesium concentration, serum magnesium can be used as a specific indicator of magnesium metabolism. But the sensitivity of serum magnesium is low. Therefore, the concentration of magnesium in erythrocytes and the value of urine magnesium can be combined to investigate the general condition of magnesium in vivo.
4.3Specific factors of common diabetic complications
As can be seen from Table 4, the prediction results of diabetic retinopathy, diabetic nephropathy and male diabetic neuropathy are better. For imbalance data, the evaluation indices are relatively high, the prediction accuracy and sensitivity is high, which means the rate of missed diagnosis is lower. But for the prediction of diabetic macrovascular complication in women, the sensitivity was relatively poor, only 0.571. The reason may be that smaller training samples resulted in insufficient feature learning. Because the data used in this article is cross-sectional record of hospitalized patients, the effect of specific factors to various types of diabetic complications is still not clear. Detailed plan for collecting efficient data should be designed for further study.
Serum magnesium concentration as a specific marker of magnesium metabolic status, is associated with three common microvascular complications – diabetic retinopathy, diabetic nephropathy, diabetic neuropathy and diabetic macrovascular disease. But magnesium in the human body is more distributed in the cell space, so magnesium concentration in red blood cells and urine should be combined to consider the overall situation of magnesium, so as to provide a reference for the disease screen or treatment, decision or adjustment of medication regimen, and help to improve prognosis of diabetic patients. Due to the limitation of data resources, this paper only makes a preliminary study on the association factors and classification predictions of serum magnesium and common diabetic complications based on retrospective data. There are still many aspects need further study and exploration.
5.Conclusion
Diabetic retinopathy, diabetic nephropathy, diabetic neuropathy and diabetic macrovascular disease are associated with serum magnesium concentration. We should pay more attention to diabetic patients with low serum magnesium level but still in the normal range. The impact of serum magnesium level on the process and prediction of diabetes mellitus worthy of further study.
Acknowledgments
The authors would like to express our enormous appreciation and gratitude to Clinical Data Center. Work described in this paper is financially supported by the National Natural Science Foundation of China (61701028).
Conflict of interest
None to report.
References
[1] | Agrawal, P., Arora, S., Singh, B., Manamalli, A., Dolia, P.B. Association of macrovascular complications of type 2 diabetes mellitus with serum magnesium levels. Diabetes & Metabolic Syndrome. (2011) ; 5: (1): 41-44. |
[2] | Tu, Y.F., Yu, H.Y., Li, L.X. Association between serum magnesium level and 24-hour urinary albumin level in patients with type 2 diabetes mellitus. Chinese General Practice. (2012) ; 15: (7): 751-754. |
[3] | Takagi, H., Kobayashi, Y., Taguchi, O., Takei, Y., Sumida, Y. Influence of dietary intake of fish oil, magnesium and zinc on metabolic parameters among individuals tested for diabetes. Nutrition. (2015) ; 31: (7-8): 988-993. |
[4] | Fu, Y.Y., Chen, X.Y., Zhang, H., Huang, X.C., Yu, Z.Y. Relationship between different levels of blood glucose with lipid and different levels of nutrients in patients with type 2 diabetics. Academic Journal of Guangzhou Medical College. (2012) ; 40: (3): 5-8. |
[5] | Barbagallo, M., Dominguez, L.J. Magnesium metabolism in type 2 diabetes mellitus, metabolic syndrome and insulin resistance. Archives of Biochemistry & Biophysics. (2007) ; 458: (1): 40-47. |
[6] | Ramadass, S., Basu, S., Srinivasan, A.R. Serum magnesium levels as an indicator of status of diabetes mellitus type 2. Diabetes & Metabolic Syndrome. (2015) ; 9: (1): 42-45. |
[7] | Medical Administration Department of Ministry of Public Health, P. R. C. National Guide to Clinical Laboratory Procedures. Southeast University Press: Nanjing; (2004) . |
[8] | Dong, S.S., Huang, Z.X. A Brief Theoretical Overview of Random Forests. Journal of Integration Technology. (2013) ; 2: (1): 1-7. |
[9] | Breiman, L. Random Forests. Machine Learning. (2001) ; 45: : 5-32. |
[10] | Qi, Y. Random forest for bioinformatics. Ensemble Machine Learning. (2012) ; 307-323. |
[11] | Kälsch, J., Bechmann, L.P., Heider, D., Best, J., Manka, P., Kälsch, H., et al. Normal liver enzymes are correlated with severity of metabolic syndrome in a large population based cohort. Scientific Reports. (2015) ; 5: : 13058. |
[12] | Janitza, S., Tutz, G., Boulesteix, A.L. Random forest for ordinal responses: prediction and variable selection. Computational Statistics & Data Analysis. (2016) ; 96: : 57-73. |
[13] | Guerrero-Romero, F., Rodríguez-Morán, M. Complementary therapies for diabetes: the case for chromium, magnesium, and antioxidants. Archives of Medical Research. (2005) ; 36: (3): 250-257. |
[14] | Ma, J., Folsom, A.R., Melnick, S.L., Eckfeldt, J.H., Sharrett, A.R., Nabulsi, A.A., Hutchinson, R.G., et al. Associations of serum and dietary magnesium with cardiovascular disease, hypertension, diabetes, insulin, and carotid arterial wall thickness: the ARIC study. Journal of Clinical Epidemiology. (1995) ; 48: (7): 927-940. |
[15] | Sales, C.H., Pedrosa, L.F., Lima, J.G., Lemos, T.M., Colli, C. Influence of magnesium status and magnesium intake on the blood glucose control in patients with type 2 diabetes. Clinical Nutrition. (2011) ; 30: (3): 359-364. |
[16] | Guerrero-Romero, F., Simental-Mendía, L.E., Hernández-Ronquillo, G., Rodriguez-Morán, M. Oral magnesium supplementation improves glycaemic status in subjects with prediabetes and hypomagnesaemia: a double-blind placebo-controlled randomized trial. Diabetes & Metabolism. (2015) ; 41: (3): 202-207. |
[17] | Xiang, M., Yang, H., Ye, C.F., Xu, X.R., Zhang, C. Level of blood trace elements in female patients with type 2 diabetic retinopathy and its related factors analysis. China Medical Herald. (2014) ; 11: (13): 9-11. |
[18] | Zhu, H., Zhu, Y.C., Wang, W.Q., Shi, C.H., Sun, X.D. A joint diagnostic test to assess the clinical risk factors of diabetic retinopathy. Chinese Journal of Ocular Fundus Diseases. (2012) ; 28: (3): 219-223. |
[19] | Sun, R., Pan, B.B., Ma, J.H. Study on the Relationships Between Serum Magnesium, Blood Glucose and Diabetic Complications. Guide of China Medicine. (2012) ; (36): 9-10. |
[20] | Floege, J. Magnesium in CKD: more than a calcification inhibitor? J Nephrol. (2015) ; 28: (3): 269-277. |
[21] | Sales, C.H., Pedrosa, L.F. Magnesium and diabetes mellitus: their relation. Clinical Nutrition. (2006) ; 25: (4), 554-562. |
[22] | Barbagallo, M., Dominguez, L.J., Galioto, A., Ferlisi, A., Cani, C., Malfa, L., et al. Role of magnesium in insulin action, diabetes and cardio-metabolic syndrome x. Molecular Aspects of Medicine. (2003) ; 24: (1-3): 39-52. |