Building trust and facilitating use of data
Abstract
Multiple crises, including the COVID-19 pandemic and increased frequency and intensity of disasters related to climate change, have demonstrated the critical importance of timely and open access to trusted data. Open data principles and practices that facilitate data access and use, relevance to policy needs, and increase the impact and value of data are central to building trust in data. The paper outlines four trends that present opportunities for expanding adoption and use of open data principles and practices and building data trust: the modernization of data governance; increased attention to the role of citizens in building trust and increasing the relevance of data and citizens’ contribution to data throughout the data value chain; the adoption of open data principles; and the work of watchdog organizations monitoring the progress of countries and agencies and identifying areas of data governance that still need attention.
1.Introduction
The need for data to implement the 2030 Agenda for Sustainable Development has drawn attention to the role of robust data and statistical systems in serving the common good and supporting well-informed decisions. In addition, over the last few years, a cascade of crises including the COVID-19 pandemic, conflicts, and the increased frequency and intensity of disasters related to climate change have demonstrated how timely and open access to reliable data can make a difference in how actions are prioritized, and crises are managed. On the other hand, the response to the pandemic revealed a breakdown in trust for data and for data-driven policies. This highlights the need to rebuild trust in official statistics by improving data governance, encouraging the participation of citizens in governing and producing statistics; making statistical systems more transparent and data more accessible and usable through implementation of open data principles; and welcoming independent monitoring of the performance of statistical systems.
Figure 1.
The data value chain.
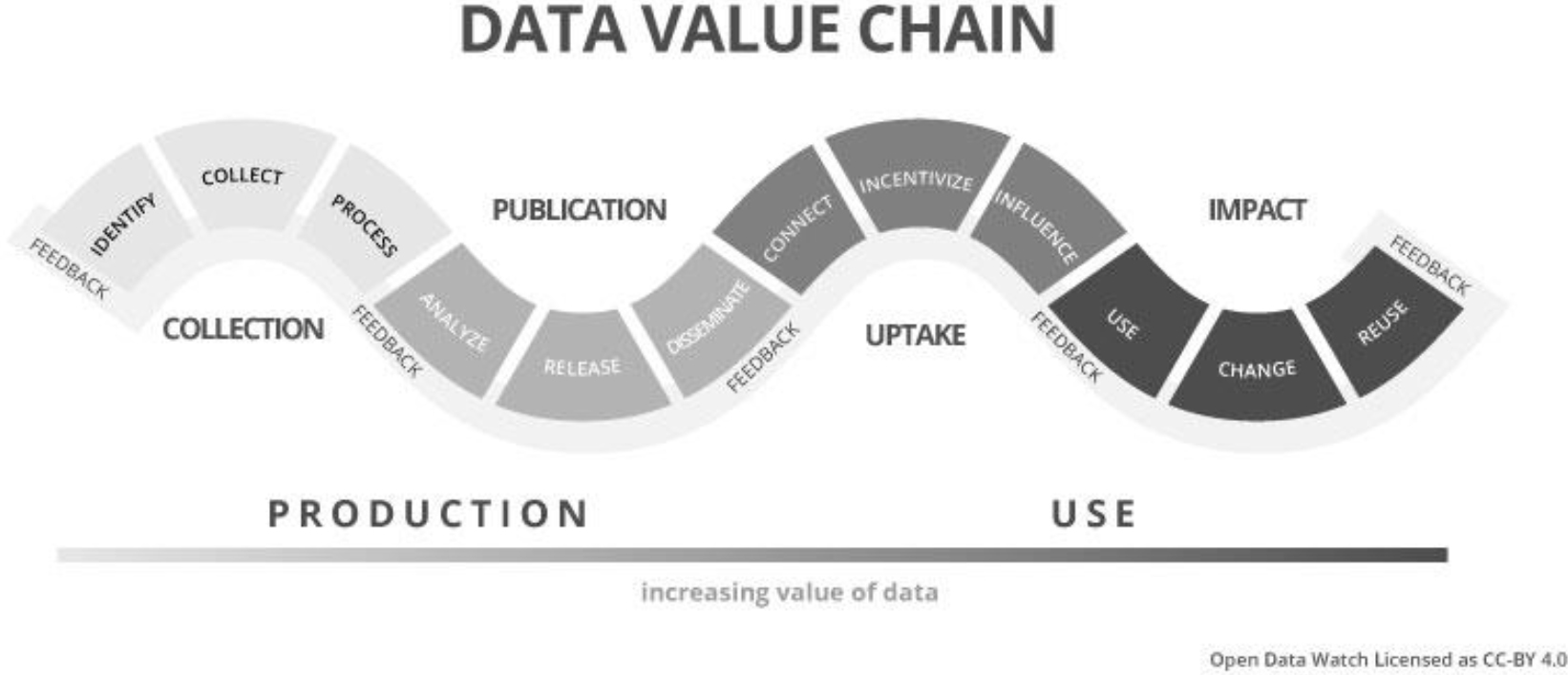
As the need for quality, timely, inclusive data is more pressing than ever, open data principles and practices are central in facilitating better data access and use, data relevance to policy needs, and increased impact and value of data, ultimately contributing to increasing data trust. There are multiple examples of how data in recent years have played a pivotal role in making policies effective and driving changes, advancing for instance progress towards some of the SDGs. Countries have increasingly adopted innovative tools and established partnerships across various data producers to overcome data challenges and create more inclusive data systems [1]. Increased data openness combined with more extensive use of innovative data solutions has improved data impact and contributed to a more effective use of data in shaping policies. For instance, in Chile, poverty estimates obtained at a more granular level through integration of survey data with administrative data have led to better allocation of funds at the municipal level [2]. In Moldova, government interventions to assist households affected by the energy crisis were driven by Household Budget Survey data [1]. In the Gambia, a national survey on SDG 16 (Peace, justice, and strong institutions), measured citizens’ views on government services and led to the establishment of a new ministry to oversee service delivery [3]. And citizen generated data are increasingly demonstrating how citizens’ bigger role in data production and use leads to a more inclusive and effective approach to development.
The paper discusses four major trends that present opportunities to increase trust in data: advances in data governance; wider recognition of the central role of citizens in ensuring data are trusted, inclusive, and relevant to policy needs and decision making; increased adoption of open data principles; and the increased role of watchdog organizations in monitoring progress of countries and agencies and highlighting areas that need attention.
2.Trust is needed across the data value chain
Trusted data are data that create value. When addressing issues of data trust it is important to look at data holistically through the different stages of the data value chain (Fig. 1) [4], highlighting how trust is needed at all stages – from the start, when identifying data needs, to data release and uptake, and finally to data use and impact.
For data to be trusted they must adhere to important ethical and quality principles at every stage of the data value chain. Below are some of the essential requirements for trust in data as one moves along the stages of the data value chain.
Data should be:
• Free of political interference
• Representative of all individuals in society
• Relevant and responsive directly to users’ needs
• Free to access, use, and re-use by anyone
• Used properly and effectively for maximum value and impact
2.1Free of political interference
Political independence must be ensured throughout the DVC, from the identification and conceptualization of what should be measured, and the design of the data collection approaches and tools, to the last phase of the DVC of data uptake and use, ensuring that data are used appropriately to make decisions and to inform policies and change, so that all can benefit.
Political interference takes many forms, from the extreme case of actual manipulation of data released by various agencies or direct interference with how data collection tools are designed, to less visible and evident forms of interference. For instance, the imposition of certain frameworks for measurement or restrictions on topics may influence the scope and content of data that limit their usability and impact, for a politically motived reason. To avoid even the appearance of political interference, data should be released simultaneously to all interested parties [5, p. 63].
2.2Representative of all individuals
To be trusted, data must be inclusive and represent all individuals in society, including the experiences of those who are harder to reach and often misrepresented or underrepresented in data [6]. The key is to involve citizens in the decision of what is measured and how, as there is always a risk of introducing biases and excluding parts of society in the process. Citizens and communities should have agency throughout the value chain, which is particularly important for empowering marginalized groups.
In addition, data may also disguise important differences when the data collection methods do not allow for the necessary level of granularity and coverage. Data collection and analysis must proceed at a level that produces information of value to all segments of the population. This may require increasing the sample sizes of surveys or more finely disaggregating census and administrative data.
2.3Relevant and responsive to users’ needs
Trusted data are data that respond directly to users’ needs and that answer questions from citizens, government agencies, non-governmental organizations, and decision-makers at all levels. Therefore, it is important to engage all data users in deciding what to measure, how to measure, and how to make the data available. Feedback mechanisms and active engagement with citizens are needed to ensure a continuous cycle of improvement and relevance.
2.4Free to access, use and re-use
Free access and use are the core principles of open data. It is important for data to be free from regulations that limit their use or reuse and for data producers to make their data discoverable and provide all necessary guidance for proper and effective use, including adequate metadata and paradata, options for downloading data in machine readable formats, and tools to facilitate data use and maximize impact. A critical aspect is also the role citizens can play in decisions on improving accessibility and usability.
The misuse of data is often cited as a reason for restricting access, regulating use, or imposing penalties on data users. There needs to be a clear distinction between regulations that prevent violation of privacy and rights and ensure transparency on data practices, and regulations that ultimately restrict access. The best corrective measure to misuse or misrepresentation of statistics is public debate and citizen engagement. In addition, the Fundamental Principles of Official Statistics [7] offer important guidance on how the official statistical agencies can comment on erroneous interpretations and correct misuse of statistics. In addition, data protection regulations are needed in the context of privately held data.
2.5Used properly and effectively
Trusted data are also data that have value and are used to their full potential by all target groups. Data may be misconstrued or misused deliberately or inadvertently. Statistical agencies have a responsibility to identify and correct misstatements [7]. They can also demonstrate the appropriate and effective use of statistics and provide guidance to others. Efforts to increase data literacy are important for increasing understanding and encouraging the use of statistics. Tools to facilitate data visualization and analysis can go a long way to maximize data use. Everyone must gain confidence to engage with and use data. Wide-spread data confidence is a building block of a fair data future.
3.Emerging trends in building trust in data
Improving data governance, giving citizens and communities agency, and fostering and increasing data transparency are needed to rebuild trust in data. In this section, we highlight four major trends that have emerged in recent years:
• Modernization and advancements of data governance practices
• Increasing demand for citizens’ data agency
• Increasing efforts to improve data transparency
• Increasing the role of watchdog organizations to monitor data transparency and openness to maintain trust
3.1Modernization of data governance practices
Efforts to modernize data governance have focused on: developing a better understanding of data stewardship functions and roles within the broader data ecosystem; increasing investments to improve data security and privacy practices; promoting responsible AI and ethical data practices; and building data partnerships to foster sharing and learning and bridging different data communities from both the public and the private sector.
3.1.1Growing emphasis on data stewardship
Defining and promoting data stewardship for better coordination, coherence, and data quality assurance across the data system helps ensure trust in data and increases data inclusivity and effectiveness. National statistical offices (NSOs) can take the important role of data stewards and help foster inclusive data systems and more effective use of data. In the new data ecosystem, NSOs are broadening their mandate and the scope of their work to ensure the efficient use of all data sources while safeguarding data quality, confidentiality, and security.
As data stewards, NSOs can also foster data equity and inclusion by operationalizing data equity and inclusion within data governance; developing a better understanding of users’expectations and needs to increase trust in (and the value, use, and impact of) data for the public good; and providing overall data governance and legal frameworks to facilitate the involvement of all stakeholders and the integration of new data sources.
The Data Stewardship working group of theUNSC [8], established in 2021, provides guidance to national statistical offices on approaches to data stewardship. The group has focused on developing a common understanding across countries of the functions of data stewardship and providing guidance to countries on how to achieve better governance, ensure adherence to important principles such as trust and ethics across the data ecosystem and promote data equity and inclusion.
3.1.2Improving data security and privacy practices
Data governance begins by ensuring fair and ethical data practices. As the data ecosystem becomes increasingly complex so do the ethical aspects of data production and use. While we do have well-established principles to promote ethical data practices including protection of confidentiality within the official statistical system – the Fundamental Principles for Official Statistics – those alone cannot guarantee proper use of the larger part of the data available today, as they cover mainly statistical production within the boundaries of the official statistical system. There is a growing need for legal and regulatory frameworks, such as for example the GDPR (General Data Protection Regulation) of the European Union [30], or the California Consumer Privacy Act [31], or Brazil’s General Data Protection Law [32], to make sense of the broader flow of data within and outside the boundaries of the official statistical system.
In the literature, there are several examples of core principles to guide the ethical production, dissemination, and use of data in the broader data ecosystem. Below, we highlight a few:
• Informed consent: Individuals have the right to understand how their data will be used and grant informed and voluntary consent before their data are collected, processed, or used in any way. Individuals must consent to the use of their data. They should have control over their data and understand the purpose and use of the data collected.
• Data ownership and control: Control refers to individuals’ control over the data value chain, including over how data are used to ensure data are not used in ways that harm individuals or discriminate or stigmatize individuals or groups. In the broader data ecosystem, privately held data should be regulated to prevent misuse of personal data and allow individuals to have the ability to access, review, understand how data are used and even erase data under certain circumstances [9].
• Data security and protection of privacy: Data collection should be done safely and securely to protect respondents and to prevent data access from unauthorized uses or breaches. Data collected through censuses, surveys, and administrative records often contain confidential information that must be protected from unauthorized access or use. Statistics computed from these data must be compiled so that the identity of individuals is not disclosed. In recent years, there has been wider recognition of the critical importance of statistical disclosure control [10] and investments have been made to improve data security and increase privacy practices.
• Transparency: Data producers must be transparent about their data practices. These include data collection methods, storage procedures, intended uses, and safeguards against misuse. Data collectors should provide clear, openly accessible metadata and paradata about their operations, including research design, data collection methods and description on how data will be used and shared.
• Openness, and accessibility: Data should be made accessible and publicly available to all, including persons with disabilities and those with limited access to technologies, and where possible, results should be published in an open access format for use and re-use. A core principle of open data is non-discrimination among data users.
• Equity and social good: All groups should be included in data production, including those that are vulnerable and marginalized, and participation should be free, open, equitable, accessible, and transparent. Data practices should not discriminate against individuals or groups.
• Accountability: Data producers are accountable for upholding human rights in their operations and for the impact of their data collection operations.
The recent rapid and remarkable progress in the development and use of AI (artificial intelligence) systems brings new and more challenging ethical issues, especially in relation to privacy, data protection, the potential use of discriminatory data and algorithms, and the difficulty of ensuring inclusivity and equity in representation [11, 12]. The principles of ethical data collection, dissemination and use should be upheld to ensure ethical AI, especially privacy concerns and data protection. However, when vast amounts of data are collected for machine learning, individuals are not normally informed of the collection and use of their personal data. In addition, there are no easy ways to ensure transparency and accountability and to protect individuals from potential negative outcomes.
3.1.3Building data partnerships
Data partnerships that foster sharing and learning and increase data interoperability and integration can improve data governance and make data more relevant and trusted. A survey to national statistical offices undertaken in 2022 by the United Nations and the World Bank [13], reveals that 45 percent of responding offices had established partnerships with the private sector, 56 percent with academia, 66 percent with international organizations and 81 percent with other government entities. Partnerships have been instrumental in improving data coverage and creating new data solutions that better respond to users’needs. The recent development of the Google Data Commons [14] and the UN Data Commons for SDGs [15] are clear examples of how powerful tools by the private sector – in this case advanced natural language data search functionality – can make data more easily accessible and discoverable, promoting a culture of data transparency and trust. However, those benefits exist only to the extent that data are comparable and interoperable, and good data governance is in place.
3.2Increasing demand for citizens’agency in data
There is wider recognition of the central role of citizens in ensuring data are trusted, inclusive and relevant to policy needs and decision making at all levels, and increasing demand for citizens’data agency, control over personal data, and intersectional data practices. The emergence of innovative approaches to engaging citizens throughout the data value chain, initiated by various parts of the data and statistical system including national statistical offices, has increased the scope of citizen engagement. These range from designing and implementing respondent-centric data collection practices to placing citizens at the center of data production, with citizens playing an active role in designing and implementing data collection initiatives. Engaging people and educating them on how to control the data flow, making them aware and giving them agency, is increasingly seen as critical to avoid attacks on democratic values and to promote trust.
A recent initiative on citizen data was endorsed by the UN Statistical Commission [16] to develop a framework that will encourage responsible production and curation of citizen data and enable CSOs, communities and citizens in countries to use the data they produce more broadly and more effectively. In that context, citizen-generated data are seen as an important tool to empower citizens to be agents of change, while enhancing data availability, timeliness, quality, openness, and inclusiveness.
Under the new initiative a framework is being developed that defines possible types of citizen data and offers a common understanding of relevant concepts and definitions as a foundation for citizen data [17]. The framework will encourage responsible production and curation of citizen data, support citizens to develop a sense of agency and ensure representation and increase the relevance and responsiveness of the NSO as the steward of the national data ecosystem by fostering an enabling environment to citizens, communities and civil society organizations as data producers.
The range of citizens’contributions to data is very wide, encompassing all stages of the data value chain and taking different forms. In developing the conceptual framework, the focus has been on data produced by citizens, communities, and civil society organizations, with the aim of informing decision making, responding to specific needs of the community, challenging existing data from the national statistical system when not reflecting the realities of a particular group or community, and filling data gaps. Other forms of citizens’contributions to regular data collection processes conducted by national statistical offices, such as censuses or surveys, in terms of engagements to increase the response rate or for other purposes, have not been included in the framework. Similarly, citizens’contributions to the analysis, dissemination and communication of data produced by government agencies, where citizens do not play a role in the production, were also excluded from what is currently covered by the framework. However, they are also important advancements in those areas, including as it relates to taking a user-centric approach in traditional data collection programmes, such as surveys, and involving citizens in efforts by National Statistical Offices to make data more relevant and accessible to users.
Figure 2.
ODIN Scores 2016—2022. Source: Authors’ calculations from https://odin.opendatawatch.com/Data/download.
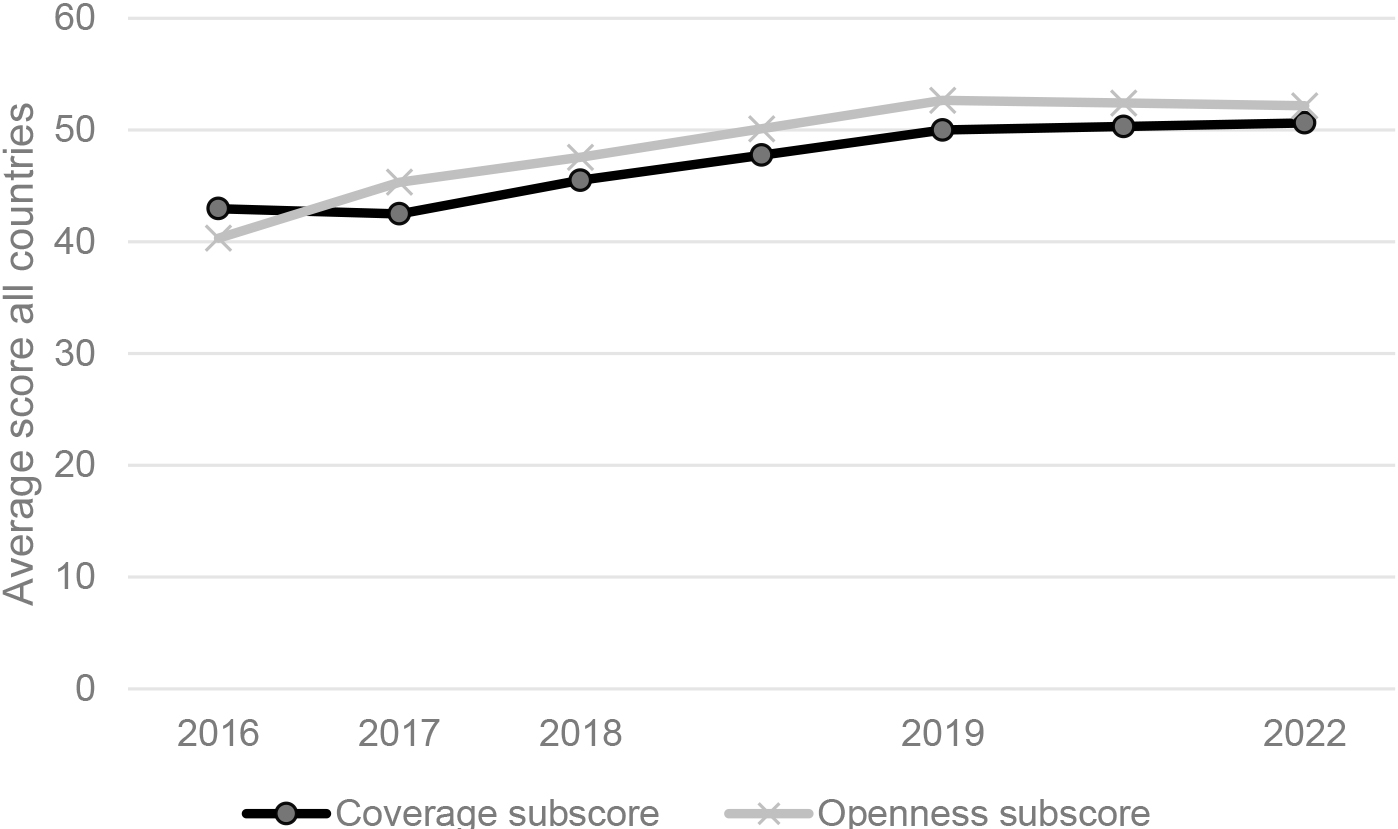
3.3Increasing data transparency
Data transparency makes visible each step of the data value chain. The core requirements for data transparency are summarized by the Open Definition [18]. This requires making data freely available to the public and publishing the data, metadata, and paradata in readily accessible formats without restrictions on who can use them or how they can be used. By removing barriers to access and use, open data facilitates data integration and linkage to policy, increasing the impact and value of data.
The origins of the movement for making data open can be traced back to 1995 when the US National Research Council called for making environmental data available to the public to allow researchers to use data that transcended borders [19]. There are strong arguments for making data open, both political and ethical. For example, people have a right to see how their government is working and to use that information to demand accountability. There are also significant benefits, both economic and social, including allowing for more rapid uptake of innovative solutions, and to save lives when data are open and accessible in crises and emergencies.
The open data movement for official statistics achieved its first prominent international success in 2010 with the open release of the World Bank’s World Development Indicators [20]. However, its antecedents can be found in Principle 1 of the Fundamental Principles of Official Statistics, first adopted in 1994.11 The International Open Data Charter, adopted at the Open Government Partnership Global Summit in 2015, extends the concept of open data by incorporating principles of transparency based on comparable and interoperable data; the use of data for improved governance and citizen engagement; and inclusive development and innovation [21].
As the concept of open data has evolved, it has been an amazing enabler for moving data up the data value chain towards greater value and impact. During the COVID-19 pandemic we saw the power of agencies with open data capacity to stand out in helping with data to manage the pandemics. The same will be true of the increasing demand for climate change data and gender equality and other sectors that are still suffering from serious data gaps. Overall, open data has proved to be fundamental to building robust and sustainable data systems, promoting ethical data practices, and maintaining trust in data.
In 2019 the UN Statistical Commission established a working group on open data composed of NSOs and other partners such as Open Data Watch [22]. The working group has addressed issues of data interoperability, and implementation of open data practices and offered guidance to NSOs for the assessment and practical application of open data in the context of official statistics.
There is evidence of increasing accessibility and openness of official statistics. The Open Data Inventory (ODIN) has found slow improvement over time in the availability and openness of official statistics. In 2023 the average coverage score, measuring the availability of time-series data in 22 statistical categories, improved from 43.0 (out of 100) in 2016 to 50.6 in 2022. (See Fig. 2) Over the same period, openness scores improved from 40.3 to 52.2 [23]. For many countries, large improvements are possible by publishing more disaggregated data with longer time series and adopting fully open terms of use, but much work remains to be done.
Figure 3.
Indicators for SDG 17.18.1. Source: Authors’ calculations from https://odin.opendatawatch.com/Data/download and https://github.com/ worldbank/SPI.
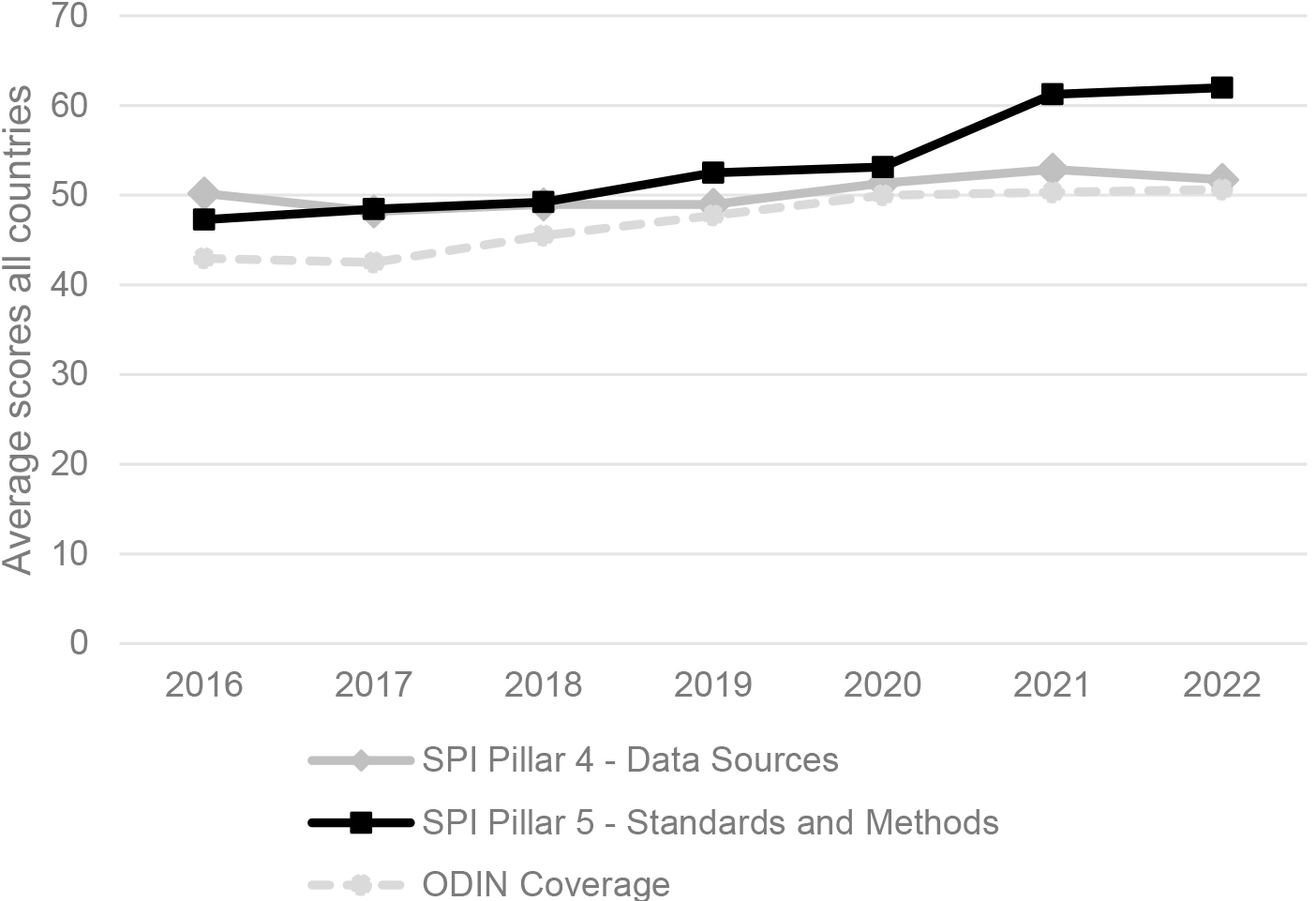
3.4Monitoring data transparency and openness to maintain trust
There is an increasing interest in monitoring the statistical and data capacity of countries along the data value chain from production to use and links to policy. The agencies and organizations involved in these monitoring exercises play a crucial role in promoting transparency, accountability, and building trust. They create incentives for improved governance by collecting information on the availability of government data, including official statistics, and their adherence to legal and professional standards. The results are typically summarized as indexes that provide comparable ratings across countries.
One of the earliest examples of a monitoring program was the International Monetary Fund’s Special Data Dissemination Standard and General Data Dissemination System [5], both of which were primarily concerned with the production standards and publication schedules of economic and financial data as reported by IMF member states. Summaries of the results are available through the Data Dissemination Standards Bulletin Board [24]. The self-reported results are subject to review by the IMF.
The Open Data Inventory (ODIN) produced by Open Data Watch has measured the coverage and openness of official statistics over 195 countries since 2016. ODIN is often described as a measure of the “revealed capacity” of national statistical systems because it measures what countries choose to produce with the resources available to them. ODIN assessments are made at arm’s length, based on what countries publish on official websites. Data are collected biennially by trained assessors. Subscores measuring data coverage and openness are available for 22 topical categories of statistics.
The Statistical Capacity Index (SCI) produced by the World Bank from 2004 to 2020 and now superseded by the Statistical Performance Indicators (SPI) provides a general assessment of the capacity of official statistical systems [25]. The SPI compiles information from online sources and other assessment programs, including the Open Data Index. Results are presented for five topical “pillars”: Data use; Data services; Data products; Data sources; Data infrastructure. Information to fully specify some pillars are still under development.
Based on their broad country coverage and focus on the capacity of national statistical systems, the coverage subscore of the Open Data Inventory and the SPI pillars for data sources and standards and methods were adopted as indicators for SDG 17.18.1, measuring the capacity of countries to monitor progress toward the Sustainable Development Goals [26].
Other monitoring programs look across a broader array of government information. The Global Data Barometer (GBD), the successor to the Open Data Barometer [27], is an example. The GDB is a multidimensional measure structured in four “pillars”: Governance; Capability; Data availability; Use and impact. GB also includes thematic modules of company information; land; political integrity; public finance; and public procurement. Data for the GDB are collected through a network of independent experts, complemented by government surveys and secondary data. The GDB currently includes 109 countries.
Many independent assessments of the functioning of governments include information about the performance of statistical systems. Two high-profile measures are the Mo Ibrahim Index of African Governance [28] and the Worldwide Governance Indicators (WGI) produced by the World Bank [29]. Although neither has the statistical system as its principal focus, they include inputs that reflect the production and use of official statistics that, in turn, affect their overall ratings.
More than just “league tables” conferring bragging rights on winners, objective assessments of countries’capacity to produce and use open data can be used to promote a dialogue with national government agencies and other partners on the importance of open data and on guidance on how to make their data open. They help to Identify opportunities for further improvement; raise awareness on capacity needs; and inform advocacy to increase the necessary funding.
4.Conclusions
In this paper, we argue for an implicit theory of change that proposes that open data principles and practices facilitate better access and use, improve relevance to policy needs, and increase the impact and value of data and as a result contribute to increasing trust in data. There is a strong link between the changing nature of trust in data and the evolving data ecosystem. So, we also highlight four major trends that have emerged in recent years that present important opportunities as data and statistical systems continue to evolve to respond to ever changing data demands and to integrate an increasing variety of data sources.
As national statistical offices become data stewards with increased emphasis on users and the use and impact of trusted data, they will play a significant role in facilitating the integration between the national statistical system and other data providers, while providing coordination, coherence, and data quality assurance. They can also broaden their mandate to effectively integrate all data sources while safeguarding data quality, confidentiality, and security. Most importantly, they can foster a culture of data equity and inclusion that are essential for building and maintaining trust in data.
We also argue that citizens and communities should play a leading role at all stages of the data value chain and that the increasing demands for citizens’ data agency is a welcome trend that ensures citizens have control over their personal data and that data are trusted, inclusive, and relevant to decision making.
Critical to a continuous progress towards better trust in data is the implementation of open data principles and the monitoring of openness and data coverage to ensure that shortcomings are visible and can be addressed. The transparency benefits gained from open data not only help with building trust but strengthen the role and reputation of institutions behind the data and lead to more reliable and meaningful use of the investments made and create a virtuous cycle creating a well-resourced, used, and trusted data ecosystem.
Notes
1 “…official statistics that meet the test of practical utility are to be compiled and made available on an impartial basis by official statistical agencies to honour citizens’ entitlement to public information.: [2].
References
[1] | United Nations Department of Economic and Social Affairs. The Sustainable Development Goals Report 2023: Special Edition. New York: UN DESA, July (2023) . |
[2] | United Nations Statistics Division. Chile. UN Statistics Wiki. [Online] [Cited: January 10, 2024.] https://unstats.un.org/wiki/display/SAE4SDG/Chile. |
[3] | Republic of the Gambia. THE GAMBIA: Voluntary National Review Report on the Implementation of the 2030 Agenda for Sustainable Development. (2022) . |
[4] | Open Data Watch. The Data Value Chain: Moving from Production to Impact. Open Data Watch. [Online] [Cited: January 10, 2024.] https://opendatawatch.com/publications/the-data-value-chain-moving-from-production-to-impact/. |
[5] | International Monetary Fund. The special data dissemination standard: guide for subscribers and users. Washington, DC: International Monetary Fund, (2013) . p. 63. |
[6] | D’Ignazio C, Klein LF. Data Feminism. Cambridge, MA: The MIT Press, (2020) . |
[7] | United Nations. Fundamental Principles of Official Statistics. United Nations Statistics Division, (2014) . A/RES/68/261. |
[8] | United Nations Statistical Commission. Report of the Working Group on Data Stewardship. (2022) . E/CN.3/2023/20. |
[9] | Cote C. Five principles of data ethics for business. Harvard Business School Online. [Online] March 16, (2021) . [Cited: January 10, 2024.] https://online.hbs.edu/blog/post/data-ethics. |
[10] | Templ M, Meindl B, Kowarik A, Chen S. Statistical Disclosure Control. Washington, DC: International Household Survey Network, (2014) . WP007. |
[11] | Ghosh P. Top-ethical-issues-with-ai-and-machine-learning. Dataversity. [Online] October 12, (2023) . https://www.dataversity.net/top-ethical-issues-with-ai-and-machine-learning/. |
[12] | Floridi L, Cowls J, Beltrametti M, et al. AI4People – An Ethical Framework for a Good AI Society: Opportunities, Risks, Principles, and Recommendations. Minds & Machines, December (2018) , Vol. 28: , pp. 689-707. |
[13] | United Nations Statistics Division and the World Bank. One year into the pandemic: Monitoring the state of statistical operations under COVID-19. [Online] [Cited: January 22, 2024] https://documents1.worldbank.org/curated/en/911901627637432764/pdf/One-Year-Into-the-Pandemic-Monitoring-the-State-of-Statistical-Operations-Under-COVID-19.pdf]. |
[14] | Google. About Data Commons. Data Commons. [Online] [Cited: January 10, 2024.] https://www.datacommons.org/about. |
[15] | United Nations Statistics Division. UN Data Commons for the SDGs. [Online] [Cited: January 10, 2024.] https://unstats.un.org/UNSDWebsite/undatacommons/sdgs. |
[16] | United Nations Statistical Commission. Report on the 54th session. United Nations. p. Decision 54/102 paragraph (h). E/2023/24-E/CN.3/2023/37. |
[17] | United Nations. The Copenhagen Framework on Citizen Data. forthcoming. |
[18] | Open Knowledge Foundation. The Open Definition. opendefinition.org. [Online] [Cited: January 8, 2024.] https://opendefinition.org/. |
[19] | Badiee S, Crowell J, Noe L, Pittman A, Rudow C, Swanson E. Open Data for Official Statistics: History, Principles, and Implementation. Statistical Journal of the IAOS, December (2020) , Vol. 37: (2), pp. 1-21. |
[20] | The World Bank Group. World Bank Group Opens Data to All. Washington, DC: The World Bank Group, (2010) . Press release. |
[21] | Open Data Charter. ODC Principles. Open Data Charter. [Online] [Cited: January 8, 2024.] https://opendatacharter.org/principles/. |
[22] | United Nations Statistical Commission. Report of the fiftieth session (5–8 March 2019). Economic and Social Council, (2019) . p. 31. E/2019/24-E/CN.3/2019/34. |
[23] | Open Data Watch. Open Data Inventory 2022/23 Biennial Report. Open Data Inventory. [Online] July (2023) . [Cited: January 8, 2024.] https://odin.opendatawatch.com/Report/biennialReport2022. |
[24] | International Monetary Fund. Dissemination Standards Bulletin Board (DSBB). [Online] [Cited: January 14, 2024.] https://dsbb.imf.org/. |
[25] | The World Bank. Statistical Performance Indicators (SPI). The World Bank. [Online] [Cited: January 10, 2024.] https://www.worldbank.org/en/programs/statistical-performance-indicators. |
[26] | United Nations Statistics Division. SDG Indicators Metadata repository. Sustainable Development Goals. [Online] [Cited: January 10, 2024.] https://unstats.un.org/sdgs/metadata/?Text=&Goal=17&Target=17.18. |
[27] | Global Data Barometer. Global Data Barometer. [Online] [Cited: January 10, 2024.] https://globaldatabarometer.org/. |
[28] | Mo Ibrahim Foundation. Ibrahim Index of African Governance (IIAG). [Online] [Cited: January 10, 2024.] https://mo.ibrahim.foundation/iiag. |
[29] | The World Bank. Worldwide Governance Indicators. [Online] [Cited: January 14, 2024.] https://www.worldbank.org/en/publication/worldwide-governance-indicators. |
[30] | European Commission. Data Protection. [Online] [Cited January 18, 2024] https://commission.europa.eu/law/law-topic/data-protection_en. |
[31] | State of California Department of Justice. California Consumer Privacy Act (CCPA). [Online] [Cited January 19, 2024]. https://www.oag.ca.gov/privacy/ccpa. |
[32] | Isaza JJ, Katshir H. Brazil Passes Landmark Privacy Law: The General Law for the Protection of Privacy. [Online] American Bar Association. May (2020) . [Cited January 19, 2024]. https://www.americanbar.org/groups/business_law/resources/business-law-today/2020-may/brazil-passes-landmark-privacy-law/. |