Perception of insecurity in municipalities in Mexico: A small area estimation approach
Abstract
In this paper, the percentage of the population aged 18 years and over with perception of insecurity during March and April 2021 is estimated for each municipality in Mexico using small area estimation techniques. Two methods are considered: the Empirical Best Linear Unbiased Predictor (EBLUP) and the Spatial Empirical Best Linear Unbiased Predictor (SEBLUP), both based on the Fay-Herriot area-level model. The National Survey of Victimization and Perception of Public Safety 2021 (ENVIPE 2021, for its acronym in Spanish) is the base survey from which the variable object of estimation is obtained; the auxiliary variables that allow to establish the considered models are obtained from other information sources, such as the population and housing census and administrative records. The results are adjusted to satisfy the benchmarking property and are contrasted with direct estimates given by the same survey, ENVIPE 2021, to compare their reliability level.
1.Introduction
A growing concern in both local governments and societies is to understand the general situation that exists in their environment in terms of insecurity and, to address this information need, the National Statistical Offices develop surveys on criminal victimization and the perception of insecurity that support the design of public policies and the knowledge of the national scene on these issues. In Mexico, the National Survey of Victimization and Perception of Public Safety (ENVIPE, Encuesta Nacional de Victimización y Percepción sobre la Seguridad Pública) [1] is an annual survey conducted by the National Subsystem of Information on Government, Public Safety and Law Enforcement (SNIGSPIJ, Subsistema Nacional de Información de Gobierno, Seguridad Pública e Impartición de Justicia) coordinated by the National Institute of Statistics and Geography (INEGI, Instituto Nacional de Estadística y Geografía). This survey is aimed to collect information that allows the estimation of victimization and public safety levels in the place of residence at both national and state levels among people 18 years of age and over who permanently reside in private homes [2]. The perception of insecurity is a paramount element in the study of crime, it measures the number of people who experience fear of being a victim of a crime; it is an important measure in decision-making involving public safety policies that allow the design, monitoring and evaluation of these programs since this fear arises from loss of control situations, social cohesion, political carelessness and distrust in the local police system, generating mistrust in authorities and inhibiting citizen participation as a complainant or witness, increasing the black figure [3]. As a result of the aforementioned facts, local governments have increased their demand for reliable and official information at local levels, such as the municipal level. This disaggregation level is not considered in the survey design, which implies that in some municipalities the sample is null, or insufficient to provide estimates with acceptable coefficients of variation according to the reliability criteria considered by the INEGI. This, coupled with the lack of other information sources that satisfy this demand, leads to the implementation of methods to obtain this reliable information in such a way that the planned costs and resources are not altered, as would happen if the sample were expanded or another survey were designed. An approach to address these conditions are the Small Area Estimation (SAE) techniques, they have proven to be an important tool in the production of official statistics [4] on several social issues. This is reflected in the range of applications that can be found on poverty, labor, health and more [5, 6, 7, 8, 9, 10, 11]. The use of SAE techniques has increased in public security issues as these can provide essential official information on the effects and crime perceptions, Buil-Gil, Medina and Shlomo in [12] analyze the dark figure at local and neighborhood levels in England and Wales; Buelens and Benschop in [13] estimate violent crime incidence at regional level in Netherlands; D’Alò, Di Consiglio and Corazziari estimate violence rate against women at regional level in Italy in [14]; Fay, Planty and Diallo in [15] estimate rates of different crimes in US states; among others. This work widens these study cases; here the perception of insecurity estimates in each Mexican municipality were obtained using SAE techniques, in order to have reliable estimates at this disaggregation level.
A municipality is defined as the political and administrative territorial division of a state; to obtain the estimates, 2,469 municipalities registered in the Census of Population and Housing 2020 (CPV 2020, Censo de Población y Vivienda 2020) were considered, of which 1,347 did not have a sample in ENVIPE 2021. The estimates were obtained using SAE techniques applying the Empirical Best Linear Unbiased Predictor and the Spatial Empirical Best Linear Unbiased Predictor, both based on the Fay-Herriot area-level model. The proportion of the population aged 18 and older who feel unsafe in their municipality of residence is considered to be the target variable and all the required direct estimates of this variable were obtained from ENVIPE 2021. Female population, employed population, population density and criminal incidence were considered as auxiliary variables. These variables were obtained from CPV 2020 and administrative records. Using the established models, figures were obtained and adjusted by an Iterative Proportional Fitting (IPF) to satisfy the benchmarking property and obtain consistent results with the data given by ENVIPE 2021 at the state level. Finally, the reliability levels of the results were compared with the results obtained from the same survey and with data obtained from the National Survey of Urban Public Safety (ENSU, Encuesta Nacional de Seguridad Pública Urbana), which is designed to provide quarterly estimates of perceived insecurity in certain Mexican cities considered to be of interest.
2.Small area estimation
Small Area Estimation is a set of statistical techniques used to generate estimates of subpopulation parameters using sample data obtained through a survey that did not consider those subpopulations in its sample design, such is the case of ENVIPE 2021 where municipalities are considered small areas, since the survey is not designed to obtain estimates for these. SAE methods are divided into two types: direct and indirect methods; direct methods only use available information in the survey related or pertaining to each area, whereas indirect methods use information related to other areas assuming some degree of homogeneity between them [16]. A particular class of indirect methods consists of model-based estimators which incorporate heterogeneity not explained by the considered auxiliary information. Owing to the available information, in this work two area-level estimators based on the Fay-Herriot model were considered: the Empirical Best Linear Unbiased Predictor and, its spatial version, the Spatial Empirical Best Linear Unbiased Predictor.
The Fay-Herriot model is a linear mixed model which incorporates area-specific random effects in addition to the fixed effects given by the auxiliary variables. The first element is the sampling model which relates, for each area
(1)
and the second element is the linking model which relates the parameter of interest to area-level auxiliary variables
(2)
with
(3)
where
(4)
It can be seen from this expression that the EBLUP is a convex linear combination of the direct estimator
To obtain a spatial version of the EBLUP, the same sampling model is considered, however the addition of spatially correlated area random effects in the linking model are taken into account. The vector
(5)
where
(6)
with
(7)
with
3.Methodology
In order to have the required data for the execution of EBLUP and SEBLUP, some processes were carried out to obtain the direct estimates, to model the sampling variance, and to set the auxiliary variables.
3.1Direct estimates
To adjust the considered models, it is necessary to know the direct estimates of the proportion of the population aged 18 years and over that feels insecure in their municipality of residence. These estimates are obtained for the 1,122 municipalities that had a sample in ENVIPE 2021 considering the same primary sampling units (PSUs) and the same strata. A factor adjustment is implemented to expand the sampled population aged 18 years and over to the corresponding population given by the population census CPV 2020 [20]: the original expansion factor for the selected element
(8)
Using this adjustment, direct estimates of the variable of interest for each municipality that had a sample, along with their variances, errors and coefficients of variation are obtained. This information is also used to validate the resulting estimates
The criteria considered by the INEGI to interpret the reliability of the data are in terms of the following acceptance limits [2]: if the coefficient of variation is between 0% and 15%, the data is considered to have a high degree of reliability; if the coefficient of variation is higher than or equal to 15% and less than 30%, the data is considered to have a tolerable degree of reliability; and if the coefficient of variation is higher than or equal to 30%, the data must be greeted with certain reservations due to its low reliability. In this way, direct estimates result in 520 municipalities that have estimates with high degree of reliability, 107 with a tolerable degree of reliability and 27 with low reliability
3.2Sampling variance modeling
Within the set of 1,122 municipalities that had a sample in ENVIPE 2021, there are 468 with a single PSU, therefore in these municipalities it would not be possible to calculate the sampling variance, and this could interfere with the efficiency and precision of the estimates obtained through the models. To avoid this, a common practice in SAE is to implement a sampling variance modeling [17, 21]. The considered model is the one proposed by You and Hidiroglou [22] which performs a logarithmic linear regression on the variance of the direct estimator
(9)
where
(10)
where
3.3Auxiliary variables
A thorough research was carried out in different administrative records from government agencies with the goal of having a set of potential auxiliary variables, and initially 13 of them were considered which are listed below:
• Proportion of female population aged 18 years and over (FP).
• Proportion of male population aged 18 years and over (MP).
• Proportion of employed population aged 12 years and over (EMP).
• Proportion of population aged 60 years and over (OAP).
• Proportion of the population aged 18 years and over with post-basic education (PBE).
• Proportion of population aged 15 years and over that migrated due to crime or insecurity (MIP).
• Population density (PD).
• Crime incidence in 2020 and the first quarter of 2021 (CINC).
• Criminal incidence in 2020 (CINC_20).
• Marginalization index (MI).
• Gini coefficient (GC).
• Proportion of population aged 18 years and over living in the urban area (UAP).
• Proportion of population aged 18 years and over living in the rural area (RAP).
Since the data of the variable corresponding to the Gini coefficient is not available for all the municipalities, it was discarded. Is also observed that the FP-MP, UAP-RAP and CINC-CINC_20 variables have a perfect correlation (Pearson coefficient
(11)
(12)
where
4.Model construction
Figure 1.
Frequency distribution and normal Q-Q plots of the residuals and random effects of the 449 selected municipalities obtained by EBLUP.
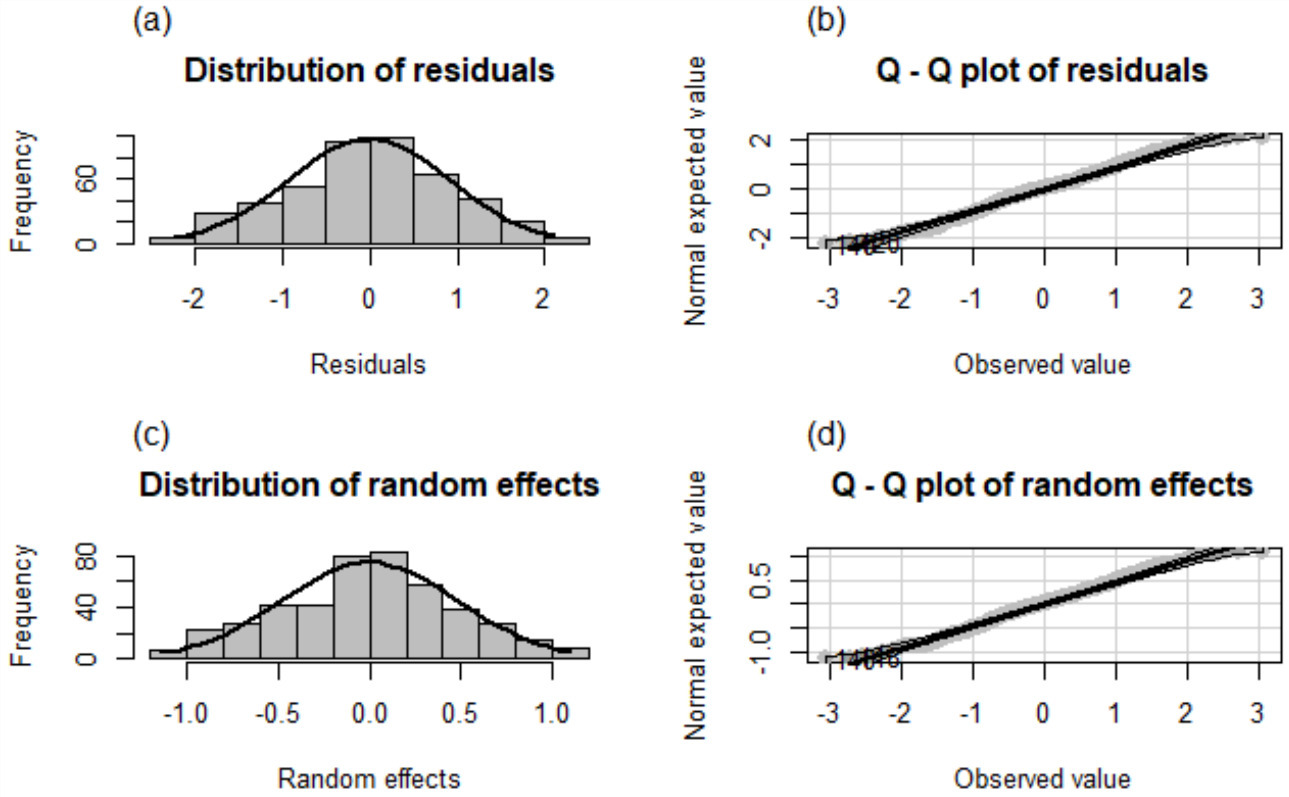
Figure 2.
Frequency distribution and normal Q-Q plots of the residuals and random effects of the 449 selected municipalities obtained by SEBLUP.
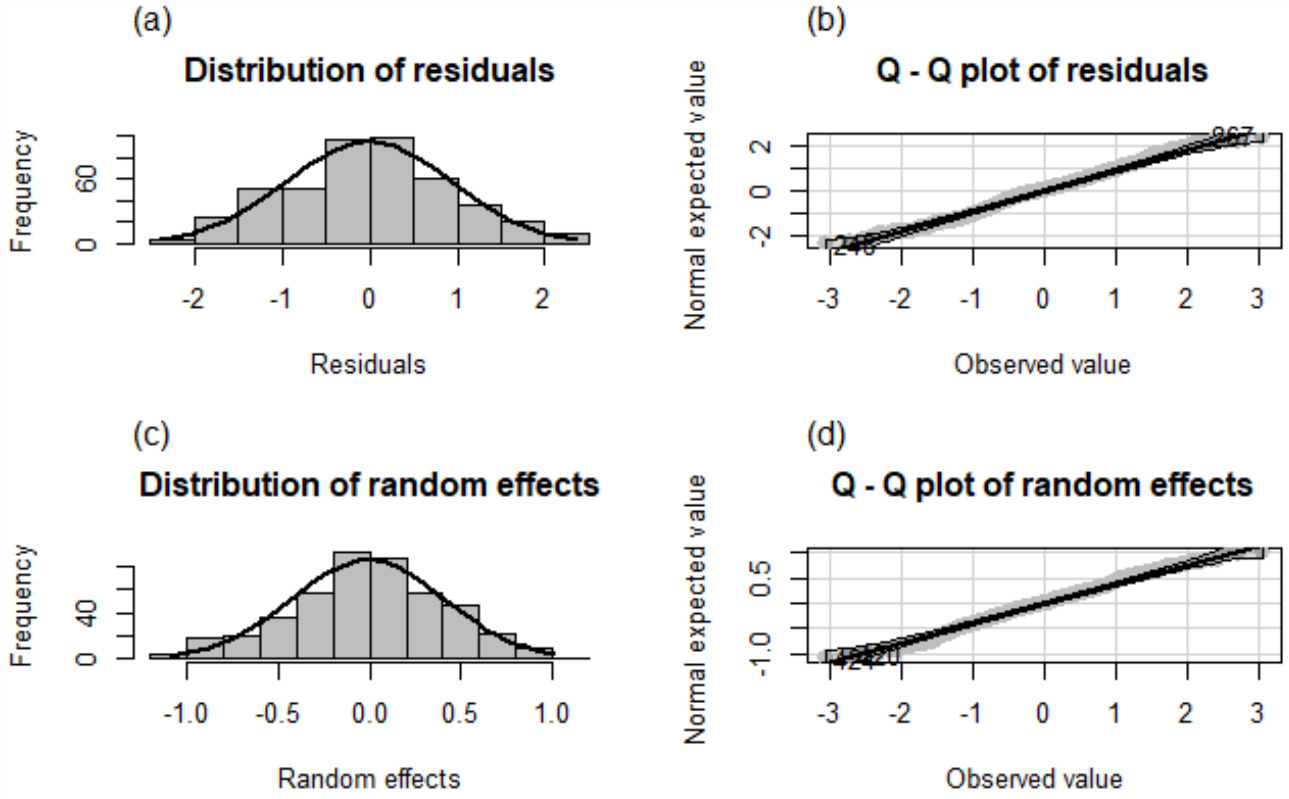
All the 1,122 municipalities that had a sample in ENVIPE 2021 were taken as an input to obtain a first EBLUP under the Fay-Herriot model using the eblupFH function in the sae library from R [31]. Outliers were detected by calculating the robust Mahalanobis distances [32, 33] of the residuals and the random effects of the model. Graphical and maximum likelihood fitting tests for different probability distributions [34] were conducted with the obtained distribution of the robust distances; these tests were made with the normal, gamma, Pareto, Cauchy, chi-square, Student
Table 1
Verification of the statistical assumptions of the models
Test | EBLUP | SEBLUP | |
---|---|---|---|
Normality | Shaphiro-Wilks | 0.008 | 0.016 |
Residuals | Kolmogorov-Smirnov | 0.042 | 0.010 |
Jarque-Bera | 0.248 | 0.273 | |
Normality | Shaphiro-Wilks | 0.016 | 0.033 |
Random effects | Kolmogorov-Smirnov | 0.315 | 0.111 |
Jarque-Bera | 0.181 | 0.217 | |
Homoscedasticity | Breusch-Pagan | 0.021 | 0.213 |
Residuals | Harrison-McCabe | 0.155 | 0.118 |
Goldfeld-Quandt | 0.150 | 0.109 | |
Multicollinearity | CI | 11.099 | |
Condition index | |||
Spatial correlation | Moran’s index | 0.272 | |
( |
Table 2
Descriptive statistics of the estimates and CV of direct estimation, EBLUP and SEBLUP
Direct estimation | EBLUP | SEBLUP | ||||
---|---|---|---|---|---|---|
Descriptive statistics | Estimate | CV | Estimate | CV | Estimate | CV |
Minimum | 16.4 | 0.1 | 10.2 | 0.1 | 10.8 | 0.1 |
Lower quartile | 45.4 | 4.1 | 39.6 | 7.8 | 39.7 | 5.8 |
Median | 62.0 | 7.8 | 50.1 | 9.7 | 50.8 | 7.1 |
Mean | 60.3 | 10.4 | 50.8 | 9.9 | 51.0 | 7.3 |
Upper quartile | 75.1 | 13.5 | 60.5 | 11.9 | 61.1 | 8.5 |
Maximum | 98.5 | 63.9 | 97.7 | 52.7 | 96.8 | 36.8 |
Figure 3.
Residuals against fitted values.
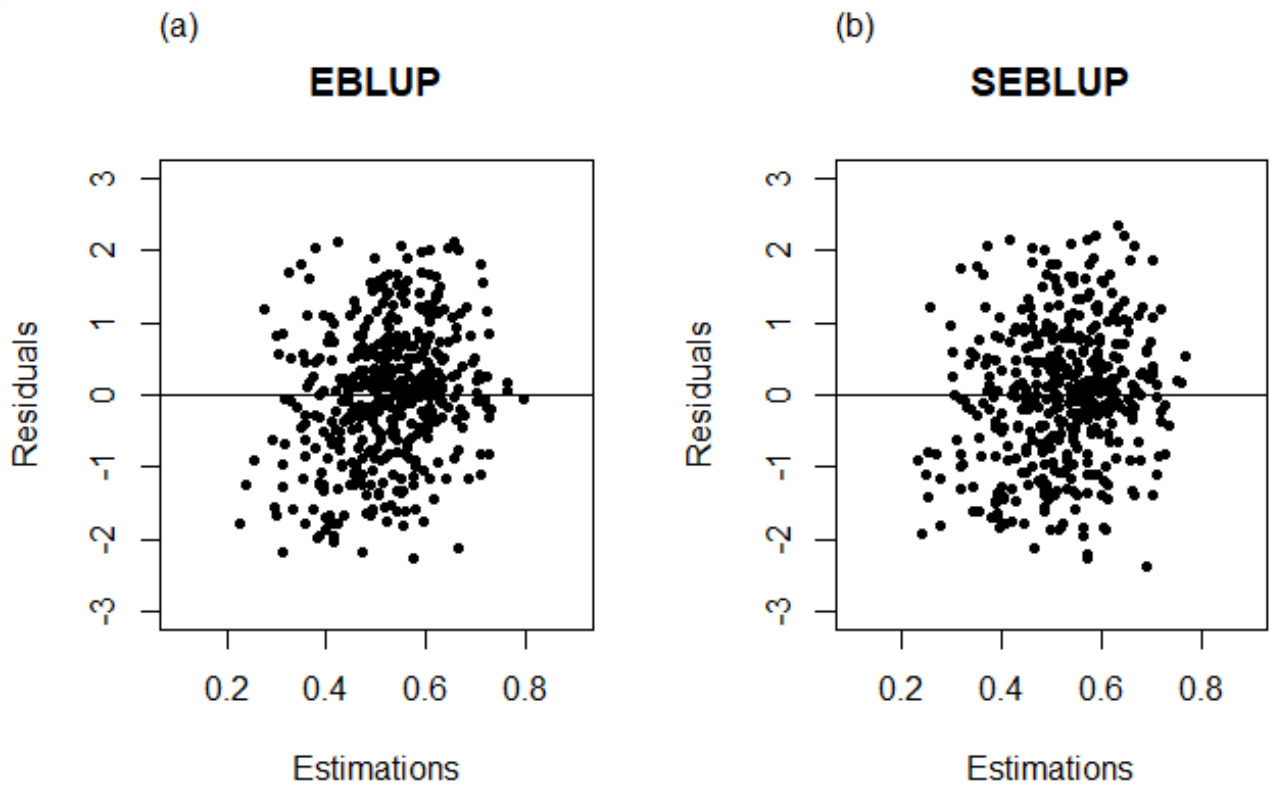
The results were assessed verifying the statistical assumptions that the model must comply with. For the assumption of normality of residuals and random effects, their histograms and Q-Q plots were inspected graphically, and the analytical tests of Shapiro-Wilks, Kolmogorov-Smirnov, and Jarque-Bera were implemented. To verify the assumption of homoscedasticity of the residuals, Breusch-Pagan, Harrison-McCabe and Goldfeld-Quandt tests were considered; for the tests of normality and homoscedasticity, it is expected to obtain
Table 3
Municipalities according to the reliability level of the estimates
Direct estimation | EBLUP | SEBLUP | |
---|---|---|---|
High reliability (0% | 520 | 2276 | 2439 |
Tolerable reliability (15% | 107 | 192 | 29 |
Low reliability (CV | 27 | 1 | 1 |
It is possible to conclude that these same 449 municipalities also meet the assumptions of the spatially correlated linear mixed model, in addition, a positive spatial correlation is observed between them, obtained through the Moran’s index, which indicates that the perception of insecurity through these municipalities is not a random phenomenon but tends to cluster spatially. The corresponding graphical and analytical tests are presented in Figs 2 and 3b, and in Table 1, respectively.
Once the estimation in the 449 selected municipalities has been carried out, there are still 673 municipalities that had a sample and with atypical values, and 1,347 that did not have a sample. In the case of EBLUP, to provide an estimate in the set of 673 municipalities, it can be assumed that the variance of the random effects,
Now, for SEBLUP, the proximity matrix W must be constructed first. To that end, the coordinates of each municipality center are extracted with the help of the shape files. Then, the Lambert conformal conic projection for Mexico ITRF2008 is applied to find the Euclidean distances between each municipality center and its
Figure 4.
Distribution of absolute differences between: (a) the estimates of EBLUP and ENSU I-2021, and (b) the estimates of SEBLUP and ENSU I-2021.
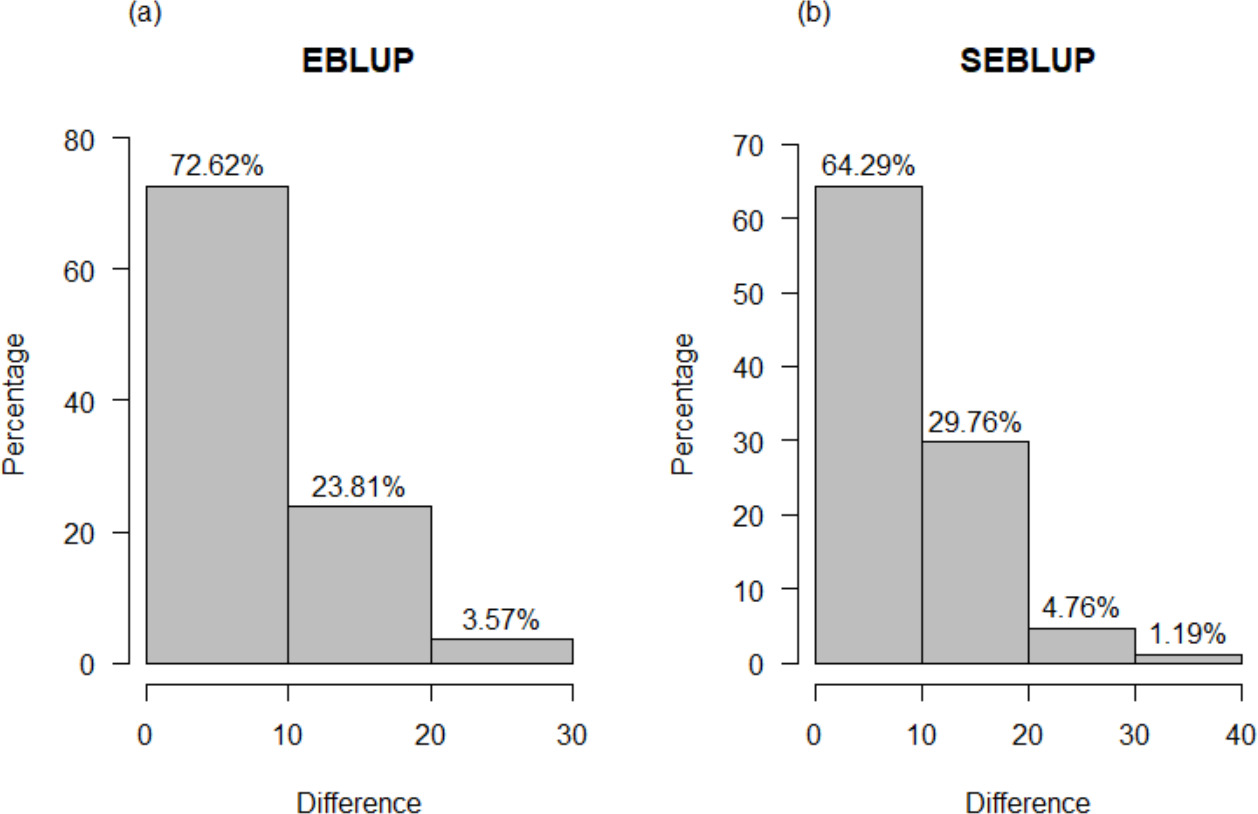
Figure 5.
Perception of insecurity as given by SEBLUP.
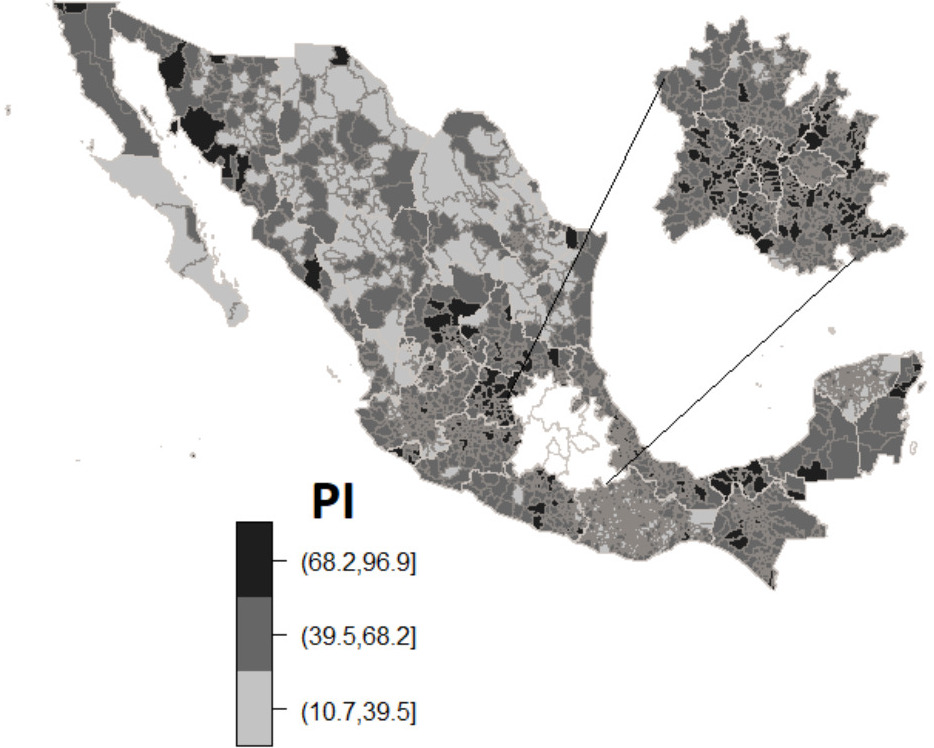
The proximity matrix is built using the national mean, median and mode (
Finally, it must be noted that EBLUP and SEBLUP estimators do not comply with the benchmarking property. In this case, benchmarking requires that the total sum of people aged 18 years and older who feel insecure in all the municipalities of each state, add up to the state total provided by ENVIPE 2021. To guarantee this, EBLUP and SEBLUP estimated totals are adjusted by performing the IPF. The resulting percentages are shown in the next section.
5.Results
The results from EBLUP and SEBLUP were first compared with the direct estimates obtained from ENVIPE 2021. In Table 2 some descriptive statistics of estimations and coefficients of variation are presented; an improvement can be observed in the coefficients of variation of model-based estimates, especially in those obtained by SEBLUP. Table 3 shows how the municipalities are distributed according to the reliability level. Initially the direct estimation provides 520 municipalities with high reliability level estimates, and with EBLUP and SEBLUP this number increases to 2,276 and 2,439, respectively; in addition, EBLUP and SEBLUP estimates for all 2,469 municipalities are obtained, unlike direct estimates.
Now, a comparison is made between the model-based estimates and the estimates of the perception of insecurity provided by ENSU-I 2021. ENSU is a survey conducted quarterly with the purpose of obtaining relevant information to generate estimates with representativeness at an urban national level on the public’s perception of public safety in their city, considering only urban areas of 84 municipalities of interest [37]. This survey is independent of ENVIPE 2021 and has a different geographic coverage since ENSU emphasizes the urban reality as the main source of victimization cases. Figure 4a shows the absolute differences between the 84 estimates of EBLUP and ENSU. It can be appreciated that 72.62% of the differences are between 0% and 10%, while the remaining 27.38% are greater than 10%, with a maximum difference of 28.71%. On the other hand, Fig. 4b shows the comparison between SEBLUP and ENSU. In this case, 64.29% of the absolute differences are between 0% and 10%, whereas the other 35.71% exceed 10%, with a maximum difference of 32.00%. Despite ENSU was designed to give estimates only at urban areas of municipalities, a certain agreement with the model-based estimates can still be observed.
The map of the perception of insecurity resulting from the SEBLUP is shown in Fig. 5. In a large part of the country there is a moderate to high perception of insecurity, furthermore, a high perception of insecurity can be observed in the northern municipalities of Baja California and northwestern Sonora as well as several other municipalities in the center of the country belonging to Zacatecas, San Luis Potosí, and Guanajuato. Likewise, in the densely populated areas of the Valley of Mexico, Puebla, Tlaxcala, and Morelos there are also municipalities with high perception of insecurity, as well as in the southeastern part of the country, for example Tabasco.
6.Conclusions
Using two SAE estimators, EBLUP and SEBLUP based on Fay Herriot area-level models, the percentage of the population aged 18 years and older who felt unsafe during March and April 2021 was estimated for the 2,469 municipalities in Mexico registered in the population census CPV 2020. The results and their reliability level, in both cases, were compared with those obtained from ENVIPE 2021 concluding that EBLUP and SEBLUP provide estimates with higher levels of reliability. As a result, the methods employed produce acceptable results. Overall, the results presented here suggest that SEBLUP is the most appropriate tool for producing high-reliability estimates relative to EBLUP.
Within the framework of the models considered, this work contributes to the identification and analysis of possible patterns of the perception of insecurity based on population characteristics at the municipal level. The obtained results are complementary to the surveys on victimization and public safety in Mexico and can contribute to the monitoring and design of local public policies.
Acknowledgments
Our gratitude to Alain Charrez Quiterio, Dionicio Ibarias Jiménez and Luis Reyes Torres for the comments received to improve the work. The points of view expressed in this work are those of the authors and do not necessarily reflect the opinion of the National Institute of Statistics and Geography.
References
[1] | National Institute of Statistics and Geography (INEGI) [www.inegi.org.mx]. Encuesta Nacional de Victimización y Percepción sobre Seguridad Pública (ENVIPE). Available from: www.inegi.org.mx/programas/envipe/2021/. |
[2] | National Institute of Statistics and Geography (INEGI) [www.inegi.org.mx]. Diseño muestral, Encuesta Nacional de Victimización y Percepción sobre Seguridad Pública (ENVIPE). Available from: www.inegi.org.mx/contenidos/productos/prod_serv/contenidos/espanol/bvinegi/productos/nueva_estruc/889463902454.pdf. |
[3] | Jiménez Ornelas R. Percepciones sobre la inseguridad y la violencia en México: Análisis de encuestas y alternativas de política. In: Alvarado A, Arzt S, editors. El desafío democrático de México: seguridad y estado de derecho. México, El Colegio de México; (2001) . pp. 145-172. |
[4] | Kordos J. Development of small area estimation in official statistics. SiT. (2016) ; 17: (1): 105-32. doi: 10.21307/stattrans-2016-008. |
[5] | Molina I, Rao JNK. Small area estimation of poverty indicators. Can J Stat. (2010) ; 38: (3): 369-85. doi: 10.1002/cjs.10051. |
[6] | Pratesi M, Salvati N. Introduction on measuring poverty at local level using small area estimation methods. In: Analysis of Poverty Data by Small Area Estimation. Chichester, UK: John Wiley & Sons, Ltd; (2016) . pp. 1-18. |
[7] | Wawrowski Ł. The spatial Fay-Herriot model in poverty estimation. FOS. (2016) ; 16: (2): 191-202. doi: 10.1515/foli-2016-0034. |
[8] | Gonzalez ME, Hoza C. Small-area estimation with application to unemployment and housing estimates. J Am Stat Assoc. (1978) ; 73: (361): 7-15. doi: 10.1080/01621459.1978.10479991. |
[9] | Orozco EV, Rivera JV, Mata GA. Labor figures for Mexico’s municipalities: Small Area Estimation. Stat J IAOS. (2021) ; 37: (2): 629-40. doi: 10.3233/SJI-200780. |
[10] | Gutreuter S, Igumbor E, Wabiri N, Desai M, Durand L. Improving estimates of district HIV prevalence and burden in South Africa using small area estimation techniques. PLoS One. (2019) ; 14: (2): e0212445. doi: 10.1371/journal.pone.0212445. |
[11] | Li W, Kelsey JL, Zhang Z. Small Area Estimation and Prioritizing Communities for Obesity Control in Massachusetts. Am J Public Health. (2009) ; 99: (3): 511-19. doi: 10.2105/AJPH.2008.137364. |
[12] | Buil-Gil D, Medina J, Shlomo N. Measuring the dark figure of crime in geographic areas: Small area estimation from the Crime Survey for England and Wales. Br J Criminol. (2021) ; 61: (2): 364-88. doi: 10.1093/bjc/azaa067. |
[13] | Buelens B, Benschop T. Small area estimation of violent crime victim rates in the Netherland. In: Proceedings of NTTS seminar. (2009) . |
[14] | D’Alò M, Di Consiglio L, Corazziari I. Small area estimation for victimization data: Case study on the violence against women. In: Proceedings of NTTS seminar. (2012) . |
[15] | Fay RE, Planty M, Diallo MS. Small area estimates from the national crime victimization survey. In: Proceedings of the Section on Survey Research Methods. American Statistical Association; (2013) . pp. 1544-1557. |
[16] | Molina I. Desagregación de datos en encuestas de hogares: metodologías de estimación en áreas pequeñas. Series Estudios Estadísticos, No 97, (LC/TS.2018/82/Rev.1). Santiago: Comisión Económica para América Latina y el Caribe (CEPAL); (2019) . |
[17] | Rao JNK, Molina I. Small Area Estimation. 2nd ed. Hoboken: John Wiley & Sons Inc; (2015) . |
[18] | Prasad NGN, Rao JNK. The Estimation of the Mean Squared Error of Small-Area Estimators. J Am Stat Assoc. (1990) ; 85: (409): 163-171. doi: 10.2307/2289539. |
[19] | Molina I, Salvati N, Pratesi M. Bootstrap for estimating the MSE of the spatial EBLUP. Comput Stat. (2009) ; 24: (3): 441-458. doi: 10.1007/s00180-008-0138-4. |
[20] | National Institute of Statistics and Geography (INEGI) [www.inegi.org.mx]. Censo de Población y Vivienda. Available from: www.inegi.org.mx/programas/ccpv/2020/. |
[21] | You Y. Small area estimation using Fay-Herriot area level model with sampling variance smoothing and modeling. Surv Methodol. (2021) ; 47: (2): 361-371. |
[22] | Estevao V, Hidiroglou M, You Y. Small-area estimation unit-level model with eblup and pseudo-eblup estimation methodology specifications. Technical report, Statistics Canada, Ottawa, ON: (2012) . |
[23] | Rivest LP, Vandal N. Mean squared error estimation for small areas when the small area variances are estimated. In: Proceedings of the International Conference on Recent Advances in Survey Sampling. Laboratory for Research in Statistics and Probability, Carleton University; (2003) . pp. 197-206. |
[24] | Gujarati DN, Porter DC. Basic Econometrics. 5th ed. New York: McGraw-Hill; (2009) . |
[25] | Mansfield ER, Helms BP. Detecting multicollinearity. Am Stat. (1982) ; 36: (3a): 158-160. doi: 10.2307/2683167. |
[26] | Box GE, Cox DR. An analysis of transformations. J R Stat Soc B. (1964) ; 26: (2): 211-243. |
[27] | Olsrr: Tools for Building OLS Regression Models, R-Packages. Available from https://CRAN.R-project.org/package=olsrr. |
[28] | Tzavidis N, Zhang LC, Luna A, Schmid T, Rojas-Perilla N. From start to finish: a framework for the production of small area official statistics. J R Stat Soc A. (2018) ; 181: (4): 927-979. doi: 10.1111/rssa.12364. |
[29] | Cavanaugh JE, Neath AA. The Akaike information criterion: Background, derivation, properties, application, interpretation, and refinements. Wiley Interdiscip Rev Comput Stat. (2019) ; 11: (3): e1460. doi: 10.1002/wics.1460. |
[30] | Secretariado Ejecutivo del Sistema Nacional de Seguridad Pública. Available from: www.gob.mx/sesnsp/acciones-y-programas/incidencia-delictiva-del-fuero-comun-nueva-metodologia?state=published. |
[31] | Marhuenda Y, Molina I, Morales D. SAE: An R package for Small Area Estimation. R J. (2015) ; 7: (1): 81-98. doi: 10.32614/RJ-2015-007. |
[32] | Ghorbani H. Mahalanobis distance and its application for detecting multivariate outliers. FU Math Inform. (2019) ; 34: (3): 583-595. doi: 10.22190/FUMI1903583G. |
[33] | Todorov V, Filzmoser P. An object-oriented framework for robust multivariate analysis. J Stat Softw. (2010) ; 32: (3): 1-47. doi: 10.18637/jss.v032.i03. |
[34] | Gan FF, Koehler KJ. Goodness-of-Fit Tests Based on P-P Probability Plots. Technometrics. (1990) ; 32: (3): 289-303. doi: 10.2307/1269106. |
[35] | Belsley DA, Kuh E, Welsch RE. Regression diagnostics: Identifying influential data and sources of collinearity. John Wiley & Sons; (2005) . |
[36] | Asfar AK, Sadik K. Optimum spatial weighted in small area estimation. Glob J Pure Appl Math. (2016) ; 12: (5): 3977-3989. |
[37] | National Institute of Statistics and Geography (INEGI). Encuesta Nacional de Seguridad Pública Urbana (ENSU). Available from: www.inegi.org.mx/programas/ensu/#Tabulados. |