A data ethics framework for responsible responsive organizations in the digital world
Abstract
Data are an integral part of the normative world and therefore ethics. With the advent of big data and data science, increased attention has been given to the ethics of artificial intelligence. However, data ethics is broader than that and must now be considered on its own as a field of ethics. In this paper, we make the case for the importance of data ethics and propose a general framework to support organizations in adopting ethical data practices. We provide the examples of statistics and standards as two contexts within which data ethics can be advanced and where advancements have already been made.
1.Introduction
Data come in many forms including “facts, figures, observations, or recordings that can take the form of image, sound, text or physical measurements” [1]. The availability and magnitude of data have made them a key part of the decision mechanism and management toolboxes of organizations, as they can be used in a variety of ways. Due to the central role and link between data and decision making, unethical use of data leads to distrust in organizations and institutions. Therefore, in an era where data are considered to be of critical value to organizations, their ethical use within and across organizations is key to meet legal and compliance requirements, manage reputational risk, and most importantly, ensure that through the use of these data, organizations are not causing harm (e.g. social, environmental, financial, or political) to their stakeholders and society at large. We argue that private, public, and not-for-profit organizations need to systematically integrate data ethics into their governance practices, operational processes and management systems to enable trust, accountability and continuous deliberation on changing legal and social licences. To this end we propose a framework to leverage standardization tools to enable and integrate data ethics accountability mechanisms within organizations. We also use the case study of Statistics Canada’s development of its data ethics framework and practices and derive insights on broader principles and tools that could inform the establishment of data ethics functions across organizations.
As society has been changing through more massive uses of technologies, so has our understanding of it and the forms of recording information about it. During the last decade profound changes in how data are created, collected, produced, stored, analysed and consumed have reshaped our relation to data and even to the world [2, 3]. In 2014, the Secretary General of the United Nations asked an expert advisory group to analyse, what he termed, the data revolution, and produce a report with recommendations [4]. This report in itself marked a transformation point in how we understand the role and policy implications of data in society. Data are now a key component of the everyday world, with numerous producers and users of data interacting with a wide range of methods, tools and applications. Organizations across the globe have been attempting to measure and quantify the value of data and activities in the digital economy. For instance, in 2019, Statistics Canada produced the first experimental set of estimates of the value of digital economic activities. While the study provided a range estimate and recommended additional exploration to validate assumptions, it nonetheless identified the growing significance of data across the Canadian economy. Investment in various data products, such as data themselves, databases and data science, has been growing since the 1990s [5].
As data are becoming an increasingly important asset to private, public and not-for-profit organizations [6], different organizations are becoming custodians of data and require expertise on how to handle such resources. An organization’s ability (or inability) to demonstrate that its data practices are ethical constitutes a key asset (or liability), in particular if it can show how they are implemented beyond legal compliance [7]. In an era when data and information flow very quickly, reputational risk is also high. For example, unethical data practices can heavily damage the reputation of an organization, could lead to decreased customer trust, and loss of business [7]. In the context of democratic public institutions, mismanagement or unethical use of data could lead to citizens’ distrust; data ethics has now become fundamental to democratic institutions. This increasingly important need by organizations has created an opportunity as well as an obligation to manage data responsibly, which has been manifested by the proliferation of Chief Data Officer positions and the development and implementation of data strategies. For example, the Government of Canada developed its own data strategy to guide its transformation of “a more transparent, collaborative, citizen-centred and digitally enabled public service” [8]. This data strategy will support a change in operations, decision making, and delivery of services for Canadians.
2.Data ethics and the data supply chain
2.1Definition
Much as ethics or business ethics had been in place (or at least considered) in organizations, the data revolution, and the COVID-19 experience (and the resulting needs to exploit data way beyond past uses) have brought to the forefront the importance and need for data ethics. Data ethics is now seen as a branch of ethics [9] and can be defined as the study of moral problems related to the data themselves, to the computer programs and algorithms that use such data and to the methods and approaches that are employed to use the data. Further, this applies throughout the data lifecycle process from collection and ingestion through decision-making and all the way to publication and/or sharing of information produced from the data. It applies to data generated by humans such as when responding to questionnaires, filling online forms to receive a service or a product but also all the non-human generated data produced by sensors, cell phones, satellites and all electronic devices that produce data. It also applies to any form of synthetic data produced through models for any purpose. Data ethics is also about respect and truth. To increase the insurance that fake data does not enter a process, there is a strong requirement to assess quality of the data, including the reliability and credibility of data sources prior to any analysis.
As society builds itself, new development avenues and opportunities constantly emerge, leading to new and more complex ramifications. In this digital age, all actors, whether they be people, businesses or public sector organisations are now both producing and consuming data in a myriad of forms. And as the appetite for more data grows, new issues are arising. When people order goods or services on-line, they will provide (and obtain, but rarely keep!) information. Similarly, as citizens interact with governments, there is an exchange of information and services. So, for businesses and public organizations to function, they require information that will enable them to operate in this complex society.
Manoeuvring in this context raises new issues. How are the data obtained? Is it in a legitimate fashion? What is happening to the data once obtained and along the data life-cycle? Who can and who does modify them, how and for which purpose? How are the data being used? Who has access to them and are they being shared? Who is the responsible custodian of the data? Who owns the data and can sell it? How securely are they kept? These are examples of questions that arise as more actors are playing on the data world stage. Data ethics then comes in to ensure that people’s data are ethically managed and to help organizations to have the appropriate interactions with data in such a manner that is both beneficial and acceptable.
2.1.1Data ethics 1: The data themselves
In terms of the actual data points, data ethics provides a questioning framework for a number of issues. First, what is the purpose for which the data will be obtained and used? Is it for public good, for profit or both? What if the data have been obtained in ways that are not ethically correct? What if data were stolen or hacked? Or wrongfully obtained without consent? What if data are produced or modified intentionally with values or trends that are not true in order to influence decisions or make money? Should data collected for one purpose be used for another to avoid running a new data collection activity? These are the types of questions that need to be answered before data are acquired, as they are being acquired/produced and during the complete stewardship, preservation and disposition cycle.
2.1.2Data ethics 2: Algorithms
Once data have been gathered through collection, acquisition or production, the ethical issues relate to how the data are going to be used and the manner in which they will be made into information and how decisions will be made. This is the realm of algorithms. Programmed algorithms can range from simple queries and models to very modern machine learning algorithms and complex artificial intelligence systems. Algorithms are varied and should not be used in isolation. The whole data lifecycle needs to be taken into account, particularly as it relates to preparing data. This includes clarifying the purpose for which an algorithm is used, assessing data quality, curating data and then validating models and results.
Algorithms have been in place for several centuries; “An algorithm is an effective procedure, a way of getting something done in a finite number of discrete steps.” [10]. According to this reference, the first computer programming algorithm is considered to have been written by Ada Lovelace in the 1800s. Numerous types and version of computer algorithms were developed since the middle of the 19
2.1.3Data ethics 3: Behaviour
Data and algorithms are virtual objects but still they are objects. Just like a pen/keyboard, a knife or a car, they can be extremely useful to people and society when put to good use. However, when their users behave unethically, all benefits go away and serious harm can be caused. There is a parallel with data and algorithms. The critical issue is how people will use them. Unfortunately, with data, it is not only ill-intended use that can be harmful but also inadvertent uses. It is therefore very important in the new data world with algorithms and AI to ensure that there is a solidly implemented culture of data ethics. The behaviour of employees, researchers and managers could well be the most underestimated, yet the most important part of the development and implementation of data ethics. One has to remember that ultimately it is people who design the algorithms, people who determine how the data will be collected, processed and used, and people who make decisions based on these. They are subjected to unconscious biases. Therefore, creating the systems, processes and culture to support the desired behaviours is key.
2.2Data ethics throughout the data lifecycle and supply chain
Similar to other types of ethical dilemmas [14], data ethics concerns all aspects of an organization’s interaction with data. In fact, it may even transcend the scope of a single organization, as the data are often created by one organization and then passed on to other ones. This is what we refer to as the data supply chain. Girard presents how the supply chain has evolved from the traditional one to a big data supply chain [15]. He explains that in the new context of big data there is a need to create new data value chains. For this to happen, it is crucial that the various steps in the chain be created and organized in an ethical manner so that society can find legitimacy in data, in their creation and in their processing. With the appropriate data ethics practices, trust can be maintained and/or increased, which results in an increase of the value of data.
As the data progress through the supply chain, one can see the data lifecycle from one organization’s viewpoints. Rancourt introduced a simple four-step description of such a lifecycle. Data are gathered, guarded, grown and given [16]. These steps apply to researchers, businesses and public sector organizations and ethical dilemmas may occur during each step and throughout the transition between steps. Considering each of these steps, those responsible for data in the organization, along with all managers and analysts who will have any involvement with data - need to be concerned with data ethics.
2.3Data ethics in practice: Towards a data ethics framework
Since data can have impacts on every element of an organization’s operations and many stakeholder groups, data governance needs to be dealt with on various levels, first and foremost at the legal compliance level. For example, the European Union developed the most comprehensive data protection regulation to date [17]. Canada has developed two versions of privacy legislations: one to protect the privacy of individuals (The Privacy Act) [18] and one to establish the rules to govern the collection use and disclosure of personal information (The Personal Information Protection and Electronic Documents Act – PIPEDA) [19]. Such laws are key to governance as can be argued: “the key to sustainable data science may lie in institutional governance” [20].
Second, since often a country’s legal framework is not sufficient to fully address the scope of data ethics challenges, it needs to be complemented with an ethics framework at the organization level, to ensure that an organization’s operations address both the legal license and the social license. Often, the legal license may not provide for all that the social licence may call for, and grey areas will point decision making related to data to the realm of ethics [7]. Therefore, in this paper we propose that data ethics be considered as an essential element of organizations’ governance and operations, while leveraging standardization tools to level the playing field, create consistency, transparency and comparability across organizations operating along the data supply chain (see Fig. 1). Having a method for organizations to describe, assess the quality, continuously improve, and disclose their practices related to data ethics is important. In both practices and the literature, we identify a gap in the ability to measure and ensure consistent data ethics practices. We propose a framework that accounts for the necessary components an organization will need in order to ensure ethical data practices. Our proposed approach to data ethics aligns and complements the work of other professional bodies who published guidelines (e.g. American Statistical Association’s Ethical Guidelines for Statistical Practice [21]. These measures include:
Figure 1.
Framework for enabling data ethics in organizations – responsive organizations.
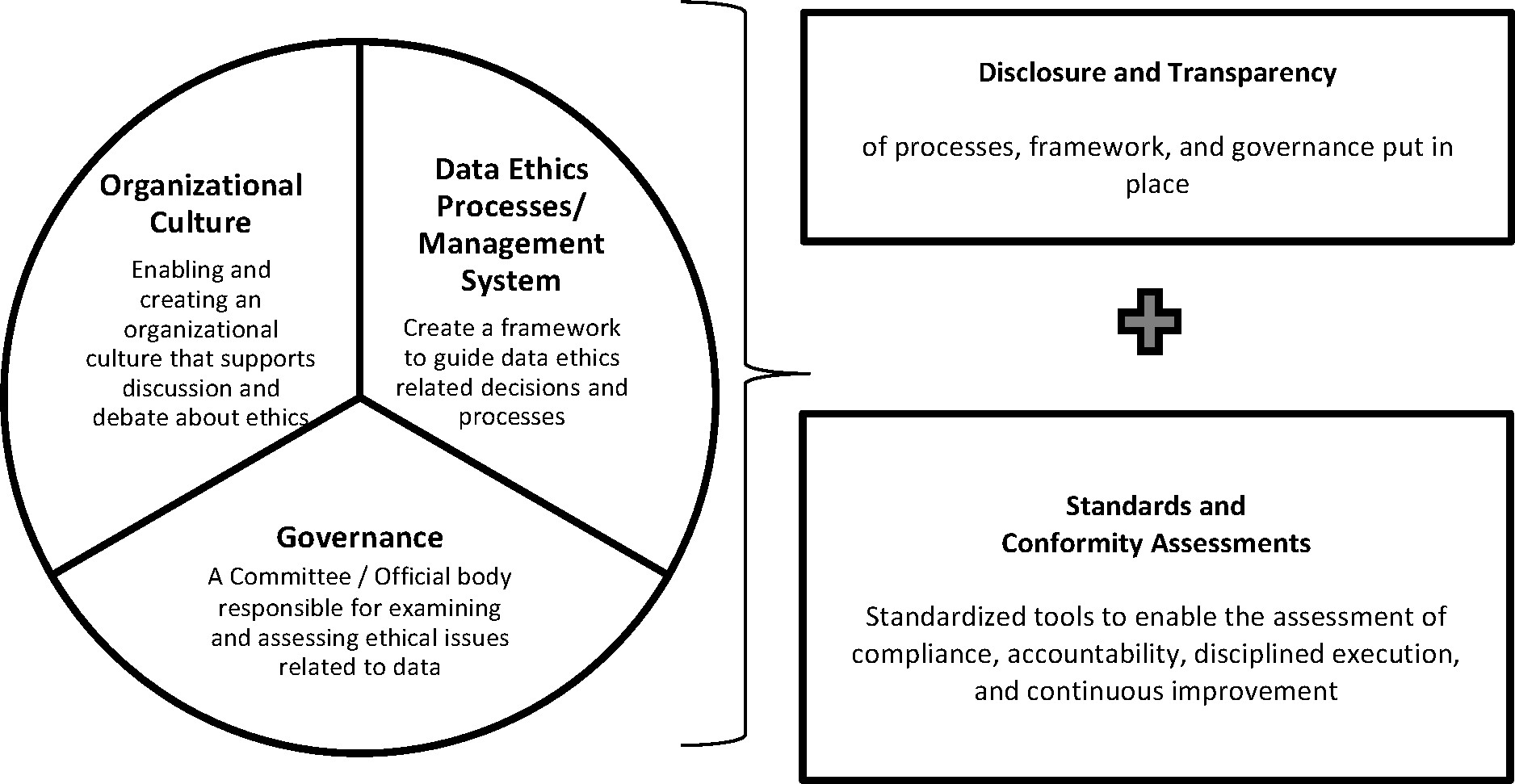
1. Integration of data ethics into the broader organizational culture and ethics programmes;
2. Presence of a data ethics processes or management system;
3. Existence of a governance structure to oversee the processes and/or management system. It can be in the form of a committee or an official body in charge of ethical issues related to data;
4. Disclosure and transparency of how organizations make ethical decisions along the data supply chain; and,
5. A standardized approach to demonstrating and verifying ethical data practices.
The potential impact of such practices would be to reduce information asymmetries and ultimately increase trust, transparency, and accountability, while supporting important pillars of democracy.
2.3.1Organizational culture
Enabling and creating an organizational culture that supports learning, discussion and debate about ethics, and data ethics, is key to ensuring ethical handling of data. It has long been argued that the organization’s culture is an essential component determining the performance of an organization [22]. As such, organizational culture may also be a limiting factor in an organization’s strategy [23]. Organizational culture is also an enabler for an organization to be accountable for its data [24]. Aligning organizational incentives and habits with best practices that consider data ethics is a key foundation of a resilient organization.
2.3.2Data ethics processes/management system
Embedding data ethics across the organization and ensuring the right questions are asked along the data supply chain is key to ensuring that best practices are disseminated systematically across an organization. Developing and implementing a framework to guide decisions and processes related to data ethics can provide a consistent and systematic approach to addressing and exploring ethical dilemmas as they arise within an organization. Such a framework can be tied to the various data governance processes and practices, legal and compliance as well as human resources. A holistic view of an organization would be useful when developing the framework due to the scope and opportunities for ethical dilemmas and challenges related to data ethics. The framework could provide employees with the tools, mechanisms and training to use when they encounter an ethical dilemma.
2.3.3Governance
Governance of data ethics can be divided into two parts. The first is internal governance of data ethics. Such a function includes internal oversight, coordination and a centre of expertise and leadership related to data ethics, and oversees the internal implementation of processes and systems related to data ethics. The second function is related to the overarching governance of the organization by its governing body. Such a function and its responsibilities in relations to data ethics are described in ISO 37000:2021 Governance of Organizations which addresses data and decision making as a key element to be considered by governing bodies of organizations. Moreover, the standard specifically states that “the governing body should, in particular, ensure that the organization recognizes data as a strategic resource and ensures that the organization uses data responsibly and ethically.” [25].
2.3.4Disclosure and transparency
While disclosure is not sufficient in driving ethical behaviour in organizations, it has been promoted as a tool that could drive more transparency and increase knowledge of non-financial aspects of an organization. Since data are considered to be a significant strategic asset for organizations, risks or liabilities related to this asset must be considered in the various financial and non-financial disclosures or other reporting tools used by companies. Furthermore, legal statutes may actually require organizations to disclose and report on data breaches. For example, in Canada, the Personal Information Protection and Electronic Documents Act (PIPEDA) [19] requires an organization to report “any breaches of security safeguards involving personal information that pose a real risk of significant harm to individuals”. Since the reporting became mandatory in 2019, the number of reports has increased by six-fold compared to the previous year [26].
In the area of Environmental, Social, and Governance (ESG) disclosure, organizations are often required to disclosure information about privacy data breaches, cyberattacks, as a way to signal investors of material impacts on the organization’s financial performance. However, ethical dilemmas related to data may occur in contexts other than privacy or cyberattacks and may have implications that go beyond material financial performance. Hence, there is value in disclosure and transparency in relation to the full realm of data and its governance with a particulate attention to data ethics.
2.3.5Standards and conformity assessments
Standardization tools, which include standards and conformity assessment, could be useful as a way to capture and embed best practices of systems and processes related to data ethics in organizations. Standards are documents developed by a group of experts that codify and diffuse knowledge and best practices within and across industries [27]. Conformity assessment is a process that provides the assurance that the requirements set in a standard have been met. Standardization enables organizations to communicate and provide greater transparency about their practices, in our case data ethics.
In the ICT ecosystem there are a wide variety of organizations developing standards, including public institutions (e.g. National Statistical Offices), consortia (IEEE), International Standards Organizations (ISO, IEC, and ITU), National Standard Development Organizations [e.g. BSI, Deutsches Institut für Normung (DIN), CSA]. These organizations differ in their reach, connection to regulators and markets, mandate, process, and accountability of the standard development process. There are already standards in place that capture best practices related to data governance, and such standards might be a natural place to discuss data ethics. However, due to the broad scope of data ethics and implications for organizations, capturing of best practices may require additional standards that go beyond the governance of data. For example, in the areas of governance of organizations or how to manage data ethics in organizations.
Figure 2.
Interplay between standards and ethics.
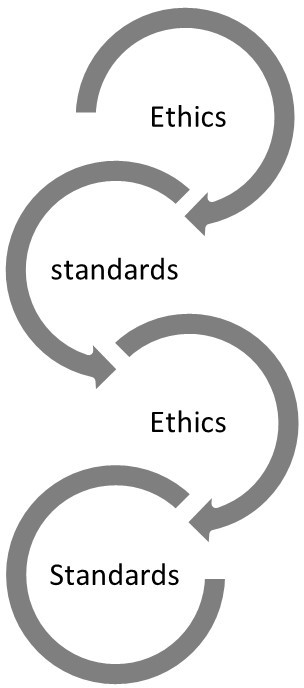
Another useful feature of standards, particularly national standards or International Standards,11 is their continual revision and update. This creates an important feedback loop over time between ethics and the standards (see Fig. 2). Just like the continuous evolution of technologies, ethics could evolve over time. Some things that are considered ethically acceptable today may not be so in the future and new questions may need to be asked to cover such evolution. As new norms and ethical practices are becoming mainstream in society, there might be a need to adapt and evolve the associated standardization tools. These changes can impact the frameworks, processes, and organizational culture of organizations. Any standard that is developed to inform ethical practices will reflect the ethical approach of the present. Therefore, the continual revision, reflection, and improvement of both the standards and their implementation allows standards to evolve and adjust to the evolution of new ethics, norms, and cultures.
Depending on the evolution of the standardization of data ethics frameworks, continuous improvement can fall in the national or international realm. It can also develop in private consortia-led standard development organizations or various parts of the national and international standardization system that support the corresponding quality infrastructure. At a system level, for example, a data ethics framework tailored to an organization, such as the one developed at Statistics Canada and described in Section 3, can become a seed document and developed into a National Standards of Canada or an International Standard. If such rout were taken, the document would become part of the national or international voluntary standardization system. The benefits of creating a national or international standards on data ethics means that the practices of data ethics would be updated at a minimum every 5 years by a committee of diverse and balanced stakeholders by consensus. At the organizational level, standardized approach to data ethics could embed a requirement to continuously evaluate the organizational data ethics practices. The benefits of such an approach are, first, the linkages to potential accredited conformity assessment to verify practices within organizations, where accredited third-party certification would provide the highest level of trust. Second, the connection to a broad set of other standards across sectors, which can create coherence and interoperability.
Presently, standardization tools in the realm of data ethics are rapidly evolving and are gaining momentum. Currently there is a number of documents and standards published by the international standards community that deal with ethics. For example, at the international level, the International Organization for Standardization (ISO) and International Electrotechnical Commission (IEC) have been developing various International Standards that currently address, or could address in the future, data ethics. They include the following:
• ISO 37000:2021 Governance of organizations – Guidance – this standard “lays the foundation for the fulfilment of the purpose of the organization in an ethical, effective and responsible manner in line with stakeholder expectations.” [25]. The standard also includes a section with guidance related to data and decision making by the governing body to ensure effective performance, responsible stewardship and ethical behaviour.
• ISO/IEC 38507 Information technology – Governance of IT – Governance implications of the use of artificial intelligence by organizations (currently under development at time of submission) – will address key considerations for governing the use of AI.
• ISO/IEC TR 24028:2020 Information technology – Artificial intelligence – Overview of trustworthiness in artificial intelligence – A technical report designed to support trust in AI systems through a variety of methods.
• ISO/IEC TR 24027 Information technology – Artificial Intelligence (AI) – Bias in AI systems and AI aided decision making – a technical report to support organizations in addressing bias in AI systems.
• ISO/TS 17033:2019 Ethical claims and supporting information – Principles and requirements – this standard lays out the “principles and requirements for developing and declaring ethical claims and for providing supporting information. It is intended for use by all types of organizations and is applicable to all types of ethical claims relating to a product, process, service or organization.” [28] helps organizations to substantiate their claims related to ethics.
• ISO 22222:2005 Personal financial planning – Requirements for personal financial planners – this standard includes a Section 5 that lays down the ethical principles financial planners are ought to follow [29].
Other international organizations such as IEEE have also developed comprehensive documents to help organizations address the ethical dilemmas arising from emerging technologies, where data is at their core [30]. In Canada, a national standard, CAN/CIOSC 101:2019 Ethical design and use of automated decision systems was published in 2019 [31].
These standards are illustrative examples to help imagine the possibilities that a set of standards in data ethics could contain. For example, ISO/TS 17033:2019 Ethical claims and supporting information – Principles and requirements, could be used to verify the reliability of organizations who are claiming to treat data ethically. ISO 22222:2005 Personal financial planning – Requirements for personal financial planners, could serve as an inspiration to develop a set of ethical principles to be followed by the data science professions or more broadly by those who work with data along the data value chain. Since many employees are working along the data value chain, data ethics has vast implications on how organizations work and are not reserved to those handling data directly. Therefore, an internationally recognized set of principles, that crosses disciplines, might be a way to further embed the desired behaviours in organizational cultures, and a way for organizations to communicate about what data ethics mean. A first steps was taken with the publishing of ISO 37000:2021 Governance of organizations – Guidance’s section on Data and Decisions. However, there is a standardization gap in how to manage and integrate data ethics across the organization. The integration of data ethics into existing or future management system-type of standards will provide the overarching guidance and mechanism to ensure continual improvement is integrated into organizations’ management of data ethics, that data ethics is done consistently in a verifiable way across organizations and industries.
3.Use case: Data ethics in official statistics – the case of Statistics Canada
The use case of Statistics Canada provides an example of an organization, whose mission is to “provide trusted data, statistical services and insights required to support decisions, while continuing to build and maintain the trust of Canadians,” [32] and who governs and manages its data ethics practices in a systematic manner. While many organizations around the world manage data, national statistical agencies are a fundamental part of democratic institutions. The data entrusted in them requires them to abide by the highest ethical standards and operate in the public interest. With technological advances along the data supply chain, statistical agencies have been exploring ways to provide more timely and relevant data to support policy, decision making and mostly the public good. In looking for new ways to deliver on its mission, Statistics Canada has developed unique capabilities in integrating data ethics throughout its operations. This experience is shared in this section.
3.1Statistical context
National statistical organizations produce information for society as a public good. In doing so, data ethics has always been a concern, however, it has not been an explicit concern (perhaps except for some health programs that have involved taking direct measures on people’s bodies). For example, confidentiality protection and data security have been strong foundational principles of statistical systems. Similarly, the concept of bias and efforts towards its avoidance have guided much of the activities to produce sound statistics. This work to avoid statistical bias has the consequence of directly contributing to producing representative statistics which enable in turn decision-makers to avoid favoring and disfavoring population groups. These concepts of confidentiality, security and bias avoidance are related to ethical issues and it is in that sense data ethics has been implicitly taken into account by national statistical organizations. For example, the United Nations have adopted fundamental principles to guide the work of National Statistical Offices (NSOs) [33] where there is very little reference to ethics but where many of the elements are actually ethical dimensions.
With the advent of new data needs, new questions and new data available, new issues have surfaced. It is no longer desirable nor possible to only implicitly deal with data ethics. At Statistics Canada, modernization of activities has shown that data ethics now needs to be organized explicitly, systematically, and considered in all steps of the data lifecycle.
3.1.1Organizational culture
Thanks to clear senior management support, the data ethics activities introduced within Statistics Canada have benefited from a solid anchoring point. As with most organizations, some reticence was expressed early on in various parts of the organization, but with a constant and renewed message from the top that data ethics is important, the idea was gradually incorporated into normal activities across the organization. In particular, data ethics reviews through a Necessity and Proportionality Framework (NPF) were made mandatory for all new data gathering activities and were embedded into existing processes through simple tools. To support staff, a number of data ethics courses were prepared and offered to employees by a university ethics/philosophy professor and supported by a dedicated internal team.
Data ethics has led to clear benefits for the organization, but more importantly for Canadians. Using the model presented in Section 2, a number of benefits can be identified. Firstly, the implementation of the NPF has strengthened the corporate ethics culture. Nowadays, managers identify much more often topics that merit more in-depth ethical reviews and are more effective at providing explanations and justifications for their projects. The framework has also contributed to foster a service mindset that is more centered on Canadians. This has materialized and can be seen by the more complete and systematic considerations of potential impacts of data decisions.
The data ethics framework and processes that were put in place have contributed to making new and redesigned projects ethically sound by design. This is due to a more explicit and systematic implementation of data ethics that includes a clearer sense of data accountability. Also, the mechanism is in continuous state of improvement to be in line with current data ethics practices and attuned to the context.
3.1.2Data ethics processes/management system
In 2019, Statistics Canada developed and implemented a NPF initially to make privacy protection of data gathering more systematic and integrated within statistical processes [34]. The NPF serves an internal standard which outlines the process by which data ethics are implemented and managed. It serves as the backbone management system to ensure all data operations across the statistical agency consider the ethical aspects involved, that issues are appropriately elevated, and dilemmas are discussed from the onset of any project. To concretely illustrate the type of issues raised, the following are examples of questions raised as part of the ethical reviews:
• What is the public benefit for gathering theses data?
• How is the project linked to the identified need?
• Which specific information is needed?
• What level of precision is needed?
• How transparent will the project managers be?
• How is confidentiality protected?
• What are the efforts made to ensure fairness and prevent harm?
• Are there alternative approaches?
The NPF implemented at Statistics Canada has evolved into one that is solidly anchored in the scientific approach and that explicitly includes ethical reviews. This constitutes a means to ensure that data ethics is considered in all phases of the data lifecycle. Data acquisitions contemplated by program managers are assessed to identify ethical considerations which are then provided to managers for them to take into account. This process acts both as a checkpoint before processes are further advanced but also (and perhaps more so) as a culture enabling process that increases data ethics awareness and supports ethical actions among managers and employees. The NPF is also fully imbedded into the corporate data acquisition process.
Data ethics goes beyond the process of gathering data. For example, when there is a need to link two files for a purpose that is different from that for which data were obtained in the first place, then this raises a number of issues and the NPF is applied. In the case of modeling and using machine learning techniques and various forms of algorithms that can be categorized as artificial intelligence, Statistics Canada has equipped itself with a directive on responsible use of machine learning. Further, in terms of avoiding possible biases, quality guidelines have been in place for many decades. More generally, a quality assurance framework is in place and under review to account for the modern data context.
3.1.3Governance
To support data ethics activities, a well-established process and data ethics decision structure is now in place with internal and external data ethics committees supported by a very active secretariat. To this end, a corporate (internal) data ethics committee was set-up and is supported by an ethics secretariat staffed by experts in ethics/philosophy. The data ethics activities are led by a senior manager with a title of Principal Data Ethics and Scientific Integrity Officer who reports in this capacity to the Chief Statistician of Canada. This officer is responsible for all data ethics reviews and is responsible for critical review of projects, which includes commenting, questioning, enforcing amendments and in some cases preventing projects from going forward in case of ethical challenges that cannot be resolved. Further, Statistics Canada is advised by an external body, the Advisory Council on Ethics and Modernization of Micro-data Access who has, as part of its mandate, to provide advice in the matters of privacy and data ethics.
3.1.4Disclosure and transparency
National Statistical Organizations such as Statistics Canada are very well equipped with strong legal instruments to ensure the confidentiality of data for which they exercise stewardship. The Canadian Statistics Act has explicit provisions related to the protection of confidentiality. In terms of practice, this goes beyond the legal aspect as it has become a hallmark of all employees to be profoundly interested and active in protecting confidentiality. As a result, disclosure of statistics and information in general is subject to rigorous attention in terms of quality but also fairness, and privacy principles.
In terms of transparency, a trust center was created and is visible to the public on Statistics Canada’s website. The trust center contains information on all administrative data acquisitions that Statistics Canada has made and is planning to make. Further the web site provides information on how the data will be used. Furthermore, in terms of transparency, all projects planning to gather data in one way or another (surveys, administrative data, other data sources) must prepare a Privacy Impact Assessment (PIA). The NPF has been embedded into the PIAs and therefore, all data ethics considerations are impacting the PIAs which are made available to the Office of the Privacy Commissioner of Canada. Finally, transparency is further advocated by means of a policy on informing users of data quality and methodology. With the efforts that have been deployed to make transparency a more pro-active and explicit activity, Canadians are kept well informed of data developments taking place at Statistics Canada.
3.1.5Standards and conformity assessments
Currently, Statistics Canada’s NPF is an internal standard and practice that has turned to provide great value within the institution. In order to elevate and increase the impact of this approach, codifying Statistics Canada’s NPF standard into a National Standard of Canada could provide great value to Canadians and other organizations who confront data ethics challenges in their operations. Mobilizing the knowledge and best practices through the standardization system could facilitate accredited conformity assessment and provide the tools to ensure that data are treated ethically across organizations and that organizations have the right systems, governance and culture to respond to the evolving ethical considerations around data.
4.Implementing data ethics across sectors
The implementation of a data ethics framework, culture, and governance can differ, depending on the type of organization. The incentives to comply or use the proposed approach might be different across organizations. One could think about the difference between a public institution whose goal is to serve the public good and a for-profit enterprise. While their incentives may differ, there is an increased pressure on private enterprises to “do more for the good of society” [35, p. 6] and ensure its operations generate sustainable long-term value for its shareholders. Given the increased scrutiny, social and political pressures, not only public institutions are required to consider ethics, but also more broadly any enterprise dealing with data along the data supply chain [35].
How might we create system-wide incentives to ensure organizations incorporate data ethics into their governance and day-to-day operations? First, there could be a legal obligation (however, currently, this is not the case, except for the area of privacy). Second, there might be a set of desired ethical behaviors that go beyond those required by law. Below is a list of a few ideas for further exploration.
Standardization tools can help create agreed-upon frameworks, systems, and processes that govern data and ensure ethical considerations are an integral part of data governance along the data supply chain. Such tools can be used on a voluntary basis or be adopted by regulators and become a mandatory requirement. Implementation of standardization tools along with disclosure can assist organizations to become more accountable and systematic in how dilemmas related to data ethics are handled.
In the public sphere, one can consider the Canadian federal government as an example. There are various places where data ethics are considered. For example, a Scientific Integrity Policy was developed to provide a policy model that is to be applied by Deputy Heads across the government “for the management of research, science and related activities in their organization” [36] This policy could serve as a constructive example of how ethics can be considered across the public sector. To ensure that data ethics be considered across departments and agencies, there would be value in considering a systematic approach to data ethics, such as the proposed framework as part of the centralized agencies management frameworks. This would allow a consistent and decentralized dissemination approach to data ethics across departments.
In the private sphere, the implementation of data ethics operates not only within organizations, but also between organizations, and between organizations and their various stakeholders. Non-financial disclosure frameworks, if/when adopted, could become useful benchmarking mechanisms to incentivize companies to comply with data ethics standards. For instance, companies often disclose ESG information to demonstrate to investors and other stakeholders their performance on environmental, social, and governance issues. As data become a key asset, issues around data ethics should be disclosed.
Currently, ESG disclosure and reporting tools such as indices [37], standards [38]; [39] and frameworks [40] explicitly require the disclosure of practices related to data security and data privacy. However, there is no explicit consideration of data ethics or data governance as indicators in those tools. Presumably, data ethics could be disclosed in a catch all categories under governance, business ethics, and/or issue identification that can be found in various ESG tools [40]. The lack of explicit consideration, could lead to overlooking such challenges and lead to reporting inconsistencies across organizations. Since data are becoming increasingly more central to an organization’s operations, governance, reputation and long-term competitiveness [2], there is a need to consider a more explicit approach to the disclosure of practices related to data ethics and data governance in ESG metrics and associated rating instruments. Since 2021, the space of ESG disclosure and reporting has been evolving rapidly. As the field is shaping, there is an opportunity to ensure that disclosure and reporting align with performance, including in the area of data ethics.
Disclosure is not sufficient. If such a system of incentives is to be complete, there is a need to create a link between the disclosure standards and framework, and the actual implementation of data ethics within organizations. Acting as the glue for such activities are the standardization tools proposed in this paper. Standardization tools can create the link between disclosure and actual operations. Data ethics standards will provide the guidance on how to integrate data ethics in systems and processes, they can also outline ethical principles for professionals working with data. Such standards can then be accompanied by certification programs, to provide assurance that organizations indeed are following the best practices as related to data ethics.
Integrating data ethics framework with culture, governance and complementing those by disclosure and standards as well as conformity assessments can be beneficial on several levels and to several stakeholder groups involved. For examples, consumers of data will be able to identify and trust data they use or purchase from organizations that get certified to a data ethics standard or a set of standards. Second, once verified, claims about ethical use of data can be easily communicated and added to ESG types of indices and serve as a signal to investors. Third, companies involved in the collection and production of data will be able to signal investors and consumers of their efforts to ensure ethical data practices along the data supply chain. This can result in building trust and reducing reputational risks. Fourth, regulators may choose to reference such standards or certification requirements as those align with policy objectives and legal requirements. Principles of data ethics could also be integrated with quality management systems, which could then have an impact on organizational processes, habits, and culture.
Finally, organizations could benefit from a common language around data ethics, a system to manage and improve data ethics practices. Standardization tools can be used to help organizations ensure they have the right framework, systems, and processes in place to allow for trustworthy decision-making related to data ethics, while ensuring a systematic execution of those framework, systems and processes.
5.Conclusions
Since data ethics is increasingly playing an important role in organizations, leaders need to consider the ways in which they develop their organizational culture and systems that support an open dialogue and debate about data ethics with an action mechanism to resolve ethical dilemmas. To this end, we have proposed a general framework to support and guide the implementation of data ethics both in the private and public sectors. The example of Statistics Canada and the context of standards have illustrated the potential and real benefits that a well-established set of data ethics principles, approaches and tools can bring to an organization. Such a structure, once in place, can lead to increased trust for those who adopt data ethics policies and frameworks.
Notes
1 International Standards are standards that are published by the following organizations: ISO, IEC, ITU.
Acknowledgments
The authors would like to thank Alain Auger, Martin Beaulieu, Cory Chobanik, Miguel DaCosta e Silva, Cloé Gratton, Marta Janczarski, André Loranger, Guillaume Maranda, Michelle Parkouda, for their helpful comments and suggestions.
References
[1] | Statistics Canada. Statistics: Power from Data! (2021) . Available at: https://www150.statcan.gc.ca/n1/edu/power-pouvoir/ch1/definitions/5214853-eng.htm. |
[2] | OECD. Digital Transformation in the Age of COVID-19: Building Resilience and Bridging Divides. Digital Economy Outlook 2020 Supplement. OECD Publishing: Paris. Available at: https://www.oecd.org/digital/digital-economy-outlook-covid.pdf. |
[3] | OECD. OECD Digital Economy Outlook 2020. OECD Publishing. Paris. (2020) ; doi: 10.1787/bb167041-en. |
[4] | Independent Expert Advisory Group (IEAG). A world that counts: Mobilizing the data revolution for sustainable development. Report, the Independent Expert Advisory Group on a Data Revolution for Sustainable Development, United Nations, New York, (2014) . Available at: https://www.undatarevolution.org/wp-content/uploads/2014/11/A-World-That-Counts.pdf |
[5] | Statistics Canada. The value of data in Canada: Experimental estimates. Latest Developments in the CanadianEconomic Accounts. Her Majesty the Queen in Right of Canada, (2019) . Available at: https://www150.statcan.gc.ca/n1/en/catalogue/13-605-X201900100009. |
[6] | Hagiu A, Wright J. When data creates competitive advantage. Harvard Business Review. January–February (2021) ; Available at: https://hbr.org/2020/01/when-data-creates-competitive-advantage. |
[7] | Hirsch D, Bartley T, Chandrasekaran A, Norris D, Parthasarathy S, Turner PN. Business Data Ethics: Emerging Trends in the Governance of Advanced Analytics and AI. Ohio State Legal Studies Research Paper No. 628. (2020) ; Available at: https://papers.ssrn.com/sol3/papers.cfm?abstract_id=3828239. |
[8] | Government of Canada. A data strategy roadmap for the federal public service. (2018) ; Available at: https://www.canada.ca/content/dam/pco-bcp/documents/clk/Data_Strategy_Roadmap_ENG.pdf. |
[9] | Floridi L, Taddeo M. What is data ethics? Philosophical Transactions of the Royal Society A, (2016) ; 374: (2083): doi: 10.1098/rsta.2016.0360. |
[10] | Belinski D. The advent of the algorithm – The idea that rules the world. Harcourt, Int., (2000) . |
[11] | Angwin J, Larson J, Surya M, Kirchner L. Machine Bias. ProPublica. (2016) ; Available at: https://www.propublica.org/article/machine-bias-risk-assessments-in-criminal-sentencing. |
[12] | Dilhac MA, Abrassart C, Voarino N. Montréal Declaration Responsible AI: Montreal declaration for a responsible development of artificial intelligence. (2018) ; Available at: https:// www.montrealdeclaration-responsibleai.com/_files/ugd/ebc 3a3_506ea08298cd4f8196635545a16b071d.pdf. |
[13] | Government of Canada. Directive on Automated Decision-Making. (2019) ; Available at: https://www.tbs-sct.gc.ca/pol/doc-eng.aspx?id=32592. |
[14] | Taylor A. The Five Levels of an Ethical Culture: How to Build and Sustain Organizations with Integrity. Business Corporate Responsibility. (2017) . |
[15] | Girard M. Standards for the Digital Economy: Creating an Architecture for Data Collection, Access and Analytics. Centre for International Governance Innovation. Policy Brief No. 155, (2019) ; Available at: https://issuu.com/cigi/docs/pb_no.155web. |
[16] | Rancourt E. The scientific approach as a transparency enabler throughout the data lifecycle. Statistical Journal of the IAOS. (2019) ; 53: (4): 549–558. doi: 10.3233/SJI-190581. |
[17] | European Union. Regulation (EU) 2016/679 of the European Parliament and of the Council of 27 April 2016 on the protection of natural persons with regard to the processing of personal data and on the free movement of such data, and repealing Directive 95/46/EC (General Data Protection Regulation). (2016) ; Available at: https://eur-lex.europa.eu/eli/reg/2016/679/oj. |
[18] | Government of Canada. Privacy Act. (1985) . Availabel at: https://laws-lois.justice.gc.ca/eng/ACTS/P-21/index.html. |
[19] | Government of Canada. Personal Information Protection and Electronic Document Act. (2000) ; Available at: https://laws-lois.justice.gc.ca/ENG/ACTS/P-8.6/index.html. |
[20] | Taylor L, Purtova N. What is responsible and sustainable data science? Big Data & Society. (2019) ; 6: (2): 1–6. doi: 10.1177/2053951719858114. |
[21] | America Statistical Association. Ethical Guidelines for Statistical Practice. (2022) ; Available at: https://www.amstat.org/docs/default-source/amstat-documents/ethicalguidelines.pdf?Status=Master&sfvrsn=bdeeafdd_6/. |
[22] | Warrick DD. What leaders need to know about organizational culture. Business Horizons. (2017) ; 60: (3): 395–404. doi: 10.1016/j.bushor.2017.01.011. |
[23] | Schien EH. Organizational Culture and Leadership. Jossey-Bass: USA, (2004) . |
[24] | Sebastian-Coleman L. The culture challenge: Organizational accountability for data. In: Sebastian-Coleman L (ed), Meeting the Challenges of Data Quality Management. Academic Press, (2022) , pp. 165–184. doi: 10.1016/B978-0-12-821737-5.00008-0. |
[25] | ISO 37000:2021. Governance of organizations. |
[26] | Government of Canada. A full year of mandatory data breach reporting: What we’ve learned and what businesses need to know. (2019) ; Available at: https://www.priv.gc.ca/en/blog/20191031/. |
[27] | Blind K. The economic functions of standards in the innovation process. In: Hawkins R, Blind K, Page R (eds.) Handbook Standards and Innovation. Edward Elgar Publishing, (2017) , pp. 38–62. |
[28] | ISO/TS 17033:2019. Ethical claims and supporting information – Principles and requirements. |
[29] | ISO 22222:2005. Personal financial planning – Requirements for personal financial planners. |
[30] | IEEE. Ethically Aligned Design: A Vision for Prioritizing Human Well-Being with Autonomous and Intelligent Systems. (2019) ; Available at: https://ethicsinaction.ieee.org/wp-content/uploads/ead1e.pdf. |
[31] | CIO Strategy Council. CAN/CIOSC 101:2019 Ethical design and use of automated decision systems. |
[32] | Statistics Canada. Raison d’être, mandate and role: who we are and what we do. Available at: https://www.statcan.gc.ca/en/about/mandate. |
[33] | United Nations. Fundamental Principles of Official Statistics. Directive E/RES/2013/21, Economic and Social Council. Available at: https://unstats.un.org/unsd/dnss/gp/FP-Rev2013-E.pdf. |
[34] | Statistics Canada. Principles of Necessity and Proportionality. (2020) ; Available at: https://www.statcan.gc.ca/en/trust/address. |
[35] | Kurznack L, Schoenmaker D, Schramade W, A model of long-term value creation. Journal of Sustainable Finance & Investment. (2021) ; doi: 10.1080/20430795.2021.1920231. |
[36] | Government of Canada. Scientific Integrity Policies. (2018) ; Available at: https://www.canada.ca/en/treasury-board-secretariat/services/information-notice/scientific-integrity-policies.html. |
[37] | MSCI. MSCI ESG Rating Methodology. (2020) ; Available at: https://www.msci.com/documents/1296102/21901542/MSCI+ESG+Ratings+Methodology+-+Exec+Summary+Nov+2020.pdf. |
[38] | Sustainability Accounting Standards Board. E-commerce: Sustainability accounting standard. (2018) ; Available at: https://www.sasb.org/wp-content/uploads/2018/11/E_Commerce_Standard_2018.pdf. |
[39] | Global Reporting Initiative. GRI 418: Customer Privacy 2016. (2018) ; Available at: https://www.globalreporting.org/standards/media/1033/gri-418-customer-privacy-2016.pdf. |
[40] | International Integrated Reporting Council. International <IR> Framework. (2021) ; Available at: https://www.integratedreporting.org/wp-content/uploads/2021/01/InternationalIntegratedReportingFramework.pdf. |