Leveraging on big data and advanced technologies to enhance domestic revenue mobilization
Abstract
Businesses are getting smarter with analytics. Tax agencies across the globe are increasingly relying on digital methods to mobilize revenue. This has resulted in an unprecedented amount of citizens’ Data flowing between systems, businesses enterprises, institutions, and governments. This Data has the potential to help increase revenue collections through targeted compliance initiatives, expanding the tax base, and improving overall operational efficiency. Tax administrations, therefore have an opportunity to deliver value in this new era of digital tax by embracing enterprise initiatives and transformations that facilitate enhanced Data utilization. To enhance the use of Data analytics there is a need for a shift in mind-set around how Data is managed and analyzed. Kenya Revenue Authority implemented a transformation agenda titled ‘Towards being an insight driven Tax Authority’, aimed at drastically enhancing the Authority’s performance in a rapidly changing environment. This would see the enterprise leverage on Data warehousing, business intelligence and advanced analytical skills and tools to transform Data management and utilization. To optimize the value of analytics there has been initiatives towards integrating with big Data sources including Data sourced from third parties. This transformation can only happen if there is a deliberate initiative to build Data literacy including skills in Data mining, modelling, artificial intelligence; among others. This paper aims at exploring how a government revenue mobilization authority is transforming and is leveraging on its digital systems, big Data, and advanced analytics to enhance revenue mobilization for the government.
1.Introduction
The modern-day market place is characterized by businesses adopting high-end technologies that facilitate the virtual transaction and real-time transfer and exchange of Data. Digitization, both in the public sector and in society in general, is completely changing how Data is moving around. There is a lot of Data available including that produced from digital tools of service delivery as well as Data from third-party sources and social networks.
The global tax landscape is changing dramatically, with tax authorities and taxpayers looking for more innovation in tax services. Tax administration must of essence adopt new strategies in order to secure their due share of taxes. The Kenya Revenue Authority (KRA) has over the past decade adopted automated systems of revenue mobilization which have been producing large volumes of Data. As the volume increased by the day, the need to manage and utilize Data for purposes of enhancing the operational efficiency in revenue mobilization became apparent. However, Data existed in silos without proper governance structures which resulted in poor quality and low accessibility, eventually resulting in low Data utilization.
Another challenge KRA faced was a weak Data-sharing framework among government entities. Without proper governance, Data quality issues regularly surfaced. The timing of when the information was produced and differing Data definitions led to discrepancies which resulted in low trust in Data as a tool of decision making. It became necessary to work towards availing the right Data to the right decision-makers, timely, and thus the establishment of structures that would ensure that Data is available, reliable, secure, and trusted. Towards achieving goals of deriving optimum value from Data, the following programs are under implementation; Data Governance, Data Cleaning, Data Warehouse & Business Intelligence (DWBI) project, Risk Profiling and Case Management tools and Data Literacy and Culture.
2.Problem statement
2.1Implementation of Data transformation agenda
KRA implemented a transformation agenda titled ‘Towards being an insight driven Revenue mobilization enterprise’, aimed at drastically enhancing the Authority’s performance in a rapidly changing environment characterized by rapid technological advances and changing stakeholder expectations. In transforming into a Data driven organization, it was necessary to put in place processes and tools that support Data management and utilization. This was achieved through the establishment of the Corporate Data Office (CDO) in December 2017 whose role was to centralize the Data management and utilization function, provide oversight of all Data quality activities and access control. These areas encompass around Data integration, Data architecture, Data security, Data quality, Data migration, business intelligence and 3
2.2Key Data issues
KRA had digitized most of the tax payer services with all registrations, returns and payments being done electronically with large volumes of data being produced from the digital systems. This data can be used to derive more insights about taxpayers’ behavior and their operating environment, for purposes of enhancing tax compliance. However, data utilization remained low. Some of the challenges that hindered KRA from realizing full value from data and that led to the establishment of a Corporate Data governance function include poor Data quality, Data systems that existed in silos, unstandardized Data definitions, poor Data literacy and culture, poor access, low data availability and poor Data utilization, Priority areas of focus for the CDO function include;
• Oversee development of Data Governance structures (processes, standards, tools, skills).
• Enhance Data quality manage and oversee the Data cleaning project.
• Driving Data utilization for insight driven enterprise – Business Intelligence, risk profiling and advanced analytics.
• Enhancing Data literacy and driving the Data culture.
• Employ emerging trends and technologies in Data analytics to drive transformation through automation.
2.3Business case for improved Data governance
A Data governance framework was developed to guide the management and utilization of Data through defined rules, regulations, and controls that ensure security, accountability, and integrity of Data in-flowing from internal systems, external systems, and 3rd party Data sources. The Data governance function is also responsible for development of Data policies, standards, and practices, both at the business and corporate levels, to achieve the required level of consistency, quality and protection to meet overall business needs.
Enhanced Data governance would improve revenue forecasting and planning as the Data provided will be accurate and the cost of compliance will be reduced. With improved Data governance KRA would be able to achieve the following outcomes which are key in enhancing revenue mobilization
• Obtain a 360-degree view of a taxpayer by accessing insights from large Datasets.
• Detect fraud by integrating current Data with social network analysis.
• Provide unsupervised learning methods to identify new, unknown types of risk and interesting/ anomalous patterns in Data.
• Automatically detect and remedy errors for filing and payment compliance (via Master Data management tool and Data quality end to end solution).
• Offer risk modelling and controlled experimentations to identify cases and/or customers likely to fail and the interventions that can remedy the situation.
• Expand the use of our predictive models to better understand taxpayer decisions.
3.Literature review
3.1Why Data Governance
Data Governance includes the people, processes and technologies needed to manage and protect the company’s Data assets in order to guarantee generally understandable, correct, complete, trustworthy, secure and discoverable corporate Data (https://bi-survey.com/ Data-governance/ 2020). A Data Governance framework seeks to guide the management of Data through defined rules, regulations and controls that ensure security, accountability and integrity of Data in-flowing from internal systems, external systems and 3rd party Data sources and out-flowing for use in business and decision making.
Data governance function focuses on management of Data throughout the it’s lifecycle including Data acquisition quality, security, protection, access, utilization, architecture and integration, sharing and archival. Seiner [2] identified the following as core principles that of Data governance;
• Data must be recognized as a valued & strategic enterprise asset.
• Data must have clearly defined accountability.
• Data must be managed to follow internal & external rules.
• Data quality must be defined & managed consistently across the Data life cycle.
3.2Data Management Body of Knowledge DMBOK
The DAMA International’s Guide to the Data Management Body of Knowledge (DAMA-DMBOK) is a Data management guide for concepts and capability maturity models for the standardization of activities, processes, roles and responsibilities and best practices. DAMA International has created definitions for eleven (11) knowledge areas which are defined in the DMBOK2 Guide, as shown by the Data Management knowledge area wheel (Fig. 1). The DMBOK Data manageent knowledge areas as follows; Data Governance; Data Architecture Data Modelling & Design; Data Storage & Operations; Data Security; Data Integration & Interoperability; Documents & Content; Reference & Master Data; Data Warehousing & Business Intelligence; MetaData; and Data Quality [8].
Figure 1.
The DAMA-DMBOK2 guide knowledge area wheel.
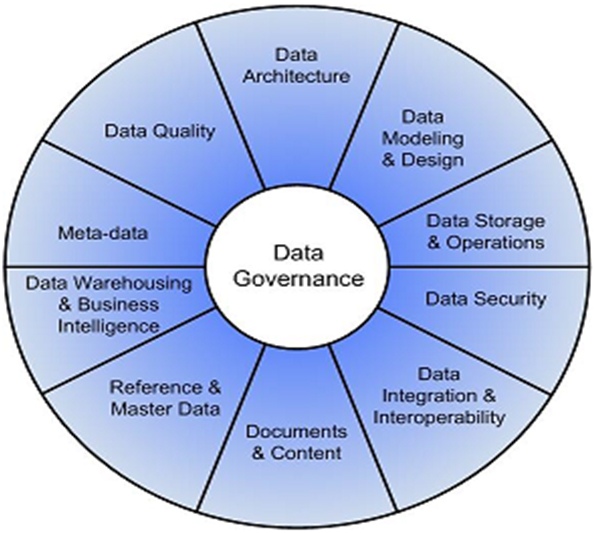
3.3Data quality
Of the biggest hindrances to optimal utilization of Data is poor quality. Data quality is synonymous with information quality since poor Data quality results in inaccurate information and poor business performance. The goals of Data quality management are to measurably improve the quality of defined business expectation, define requirements and specifications for integrating Data quality control into the system development life cycle and to provide defined processes for measuring, monitoring, and reporting.
3.4Data security and Privacy
Data Privacy describes the practices which ensure that the Data shared by customers is only used for its intended purpose. In a world with ever-growing mountains of big Data, privacy is an increasing topic of scrutiny.
Data security is focused on protecting personal Data from any unauthorized third-party access or malicious attacks and exploitation of Data. It is set up to protect personal Data using different methods and techniques to ensure Data privacy. Data security ensures the integrity of the Data, meaning Data is accurate, reliable and available to authorized parties https://Dataprivacymana ger.net/ Principals of Data privacy and security are defined by policies and procedures governing who may access the Data as well as how the Data is accessed. This includes people within the organization or third parties who may be granted access to the Data.
3.5Data warehouse and business intelligence (DWBI) solution
A Data warehouse also is known as an enterprise Data warehouse (EDW), is a system used for reporting and Data analysis, and is considered a core component of business intelligence [5]. Business intelligence (BI) comprises the strategies and technologies used by enterprises for the Data analysis of business information [4].
The DWBI solution enhances precision in decision making by giving access to the right information by the right people at the right time. BI and Analytics help in decision making by giving customized and timely reports on key performance indicators and identify gaps that require operational and strategic focus. The Business Intelligence and analytics value of the solution can only be as good as the quality of the Data.
3.6Data literacy and culture
As the organization works towards being Data-driven, it is of the essence to design and implement initiatives that enhance Data culture and literacy. Data literacy is the ability to read, understand, create, and communicate Data as information. Data culture is the principle established in the process of social practice in both public and private sectors which requires all staffs and decision-makers to focus on the information conveyed by the existing Data and make decisions and changes according to these results instead of leading the development of the company based on experience in the particular field [6].
An important driver of Data literacy and culture are Data stewards. Data stewards gather the business’s needs and requirements related to Data fitness for use and translate them into methodical steps necessary to implement Data corrections and adjustments. They are also responsible for collecting and analyzing Data in support of business activities [7]. Data stewards have a specialist role that incorporates processes, policies, guidelines and responsibilities for administering organizations’ entire Data in compliance with policy and/or regulatory obligations. All stakeholders should be aware of organizational investments in Data management projects, and the objectives and expectations for these projects as well as understand whatever responsibilities they have to conform to Data standards and comply with external regulations.
4.Methodology
4.1Data management maturity baseline and annual monitoring
To ensure effective Data management practice, the DAMA International’s Guide to the Data Management Body of Knowledge (DAMA-DMBOK) was adopted. The first step was to assess Data management maturity in the organization with a focus on the main tax administration system. Based on the findings recommendations were made and a roadmap proposed for the resolution of a majority of identified gaps. The roadmap lists a number of activities that can be completed by the KRA in the short-, medium-and long-term. The output of the assessment was a report that ranked Data maturity levels and indicated specific areas that required improvement.
4.2Data management maturity baseline
In 2018 an initial Data management maturity level assessment was done using DMBOK standards. The assessment focused on five (5) key aspects of Data management which were important to the institution at the time; quality, availability usability, access controls, and privacy & security. The assessment result was rated on a scale of 1 (Improvement needed) to 4 (Adopted best practice).
4.3Data utilization survey baseline
A Data utilization survey was also conducted in 2018 to establish a baseline for the corporate Data utilization level. The survey is designed to measure users’ experience in utilization Data including Data availability, fit for use, usability as well as Data literacy and culture. The survey measure of various aspects is expressed as a percentage.
4.4Annual assessment
An assessment of the Data management maturity levels is done annually to monitor the effectiveness of Data governance initiatives. A repeat survey is Data utilization survey is administered annually to monitor effectiveness of the Data utilization strategy
5.Key transformation initiatives
5.1Enhancing Data quality
5.1.1Data quality gaps
The data quality assessment established a baseline score of 2 out of 4. It was evident that there was need to focus on improving Data quality. Data quality issues posed a potential risk with regards to the realization of the revenue mobilization and customer service delivery outcomes. Data quality issues were characterized by missing and erroneous customers’ bio information, missing and erroneous transactions, duplicate identities, shared identities and existence multiple legacy system that contained critical customers’ information,
There was a need to ensure that the Data quality issues were addressed sustainably by implementing the following strategies;
• Stopping dirt from the start.
• Cleaning existing gaps in Data to ensure accuracy and completeness of the customer attributes.
• Address system gaps that led to poor Data quality.
• Entrench Data quality considerations in future system requirements.
• Simplify the tax administration process to make it easy for customers to provide the right information.
5.1.2Data cleaning and migration
Data cleaning and migration were prioritized as critical in enhancing the Data quality and its improve accuracy, completeness, validity, relevance, and integrity; thus making Data useful in enhancing compliance and in identifying the gaps in domestic revenue mobilization. Poor Data quality was characterized by inaccurate or incomplete customers register, missing or inaccurate transactional information, an incomplete view of taxpayer’s information since Data existed in multiple and parallel platforms. An analysis was done to determine the source of Data gaps which were identified as follows;
• System gaps; Some systems gaps introduced Data quality issues.
• Process gaps; Some administrative processes were not aligned to existing laws which led to gaps in Data.
• Lack of Data validation; Taxpayer self-registration that is not verified.
• Poor linkage with third-party Data sources for purposes of verifying taxpayer’s registration and transaction information.
A Data cleaning project was set up with the mandate to address existing Data quality gaps as well as recommend strategies for sustainable Data quality improvement gaps. This would enable KRA to have trusted and dependable Data which is critical in actualizing the goal of being a Data-driven organization. The Data cleaning team comprised of officers drawn from core business departments, Data analysts, and ICT experts. The team commenced this task by developing work plans, business rules for Data cleaning, and identifying system changes that will be critical in sealing the gaps that compromise Data quality. Two teams were formed to clean the master and transactional Data respectively. Master Data cleaning team focused on registration Data (duplicate identity, wrong Tax obligations, erroneous dormancy, erroneous and missing contacts, updating, and economic and professional categories). The transaction Data cleaning team focused on transactional records (missing payments, missing returns erroneous credits, and erroneous penalties and interests). This task required members to have sufficient technical knowledge on tax administration and were therefore mostly drawn from business. The Data cleaning process would culminate in the migration of records from legacy systems to the current Tax systems for purposes of gaining a complete view of customers’ activities.
5.1.3Towards sustainable Data quality
Learning from historical Data quality issues, it became necessary to establish a Data quality assurance function responsible for assuring sustainable Data quality. A Data quality assurance function was established to coordinate the development and implementation of strategies, policies, and standards for sustainable Data quality going forward. Data quality management provides solutions to improved Data quality and integrity. Data quality policies were developed and institutionalized processes for oversight, management, and improvement through continuous identification of the business needs for quality Data and determining the best ways to measure, monitor, control and report on the quality of the Data.
5.2Data privacy, protection and security
The Data security maturity assessment established a baseline score of 2 out of 4 which indicated that there was need to enhance Data security. Data security issues were characterized by gaps in Data security and privacy policies. Towards addressing these gaps Data security and privacy policies and procedures have been developed to ensure that the right people access or update Data in the right way and that all inappropriate access and update is restricted. The policies have been aligned to global and national regulations as well as good practice.
5.3Gaps in Data availability – acquisition of Data from third party sources
The Data availability maturity assessment established a baseline score of 2 out of 4. One of the greatest challenges faced by revenue administrations in developing countries is the inability to effectively widen the tax base using their internal Data sources. KRA has in the past couple of years invested widely in systems that have yielded results in terms of providing the authority with Data sources that enhanced compliance. However, with the business environment getting more and more complex and customers devising smart methods of staying away from the tax brackets, it has become necessary for tax administrations to source Data from other entities to increase the visibility of taxpayer’s economic activities. Data from third party sources is cross-matched against internal Data to assist in identifying gaps in compliance which will then yield in enhancing compliance and widening the tax base. The overall objective of the third party accusation function is to ensure the provision of timely and quality third party Data for decision making and policy formulation by adopting best practice in third party management including;
• Acquisition, utilization, and management of third party Data for enhancing compliance and risk management.
• Developing protocols for sharing Data with third parties.
• Outlining third-party Data reporting framework and manage Data security and access.
• Leverage on predictive analytics that provides information on likely future outcomes and process.
The goal is to integrate with all critical third party Data systems for purposes of achieving operational efficiency and simplification tax administration process.
5.4Data utilization
5.4.1Data utilization strategy
The Data utilization survey established a baseline score of 62%. The major gaps identified include customers’ Data existed in digital parallel systems, lack of customized dashboards for critical functions, inaccurate information and incomplete customer profiles. A Data strategy was developed to guide the implementation of strategies for improving Data management and utilization including Data integration and architecture, Data security, Data quality, Data migration, business intelligence, and 3rd party Data management. Implementation of the Data strategy will assist KRA to achieve consistent, trustworthy, accurate, and reliable Data as well as manage all organization-wide Data Governance activities and have responsibility for improving the quality and managing the protection of sensitive Data and information assets. The Data strategy has a 3-year implementation period.
5.4.2Data Warehouse and Business Intelligence (DWBI) solution
To be able to derive value from the vast amounts of Data available from the highly digitized tax administration system in KRA a Data Warehouse and Business Intelligence (DWBI) solution was developed and rolled out. The expected outcome of the DWBI initiative was to develop Business intelligence and taxpayer profiles by analyzing Data from different sources, thus increasing revenue yields and expand the tax base and in so doing enhance the level of compliance with tax regulations. Data stored in the Data warehouse is sourced from the different operating systems internally as well as from third-party sources, and stored for purposes of deriving business intelligence and profiling customers. Before the implementation of the DWBI solution, Data was disjointed and was sourced from the different revenue department systems. The Data variables lacked standardized definitions which made the task of collection, collating, reporting, and revenue projection tedious, inaccurate, and time-consuming. The base for the development of a unified taxpayer Database is to gain knowledge on the Data in scope. The DWBI solution has a foundational purpose for subsequent initiatives and includes the following tasks:
• Provide a complete view of customer activities using information from multiple Data sources.
• Provide a centralized source of truth for the corporate decision-making process.
• Provide timely and customized dashboards and reports for Data users.
• Reduce amount of time used gathering and analyzing and disseminating Data.
• Better use of Data by employing analytical methods that can address risks that are common to many revenue administrations such as tax fraud, identity theft, illicit trade, and tax evasion.
• Improve the efficiency and effectiveness of revenue administration, with a particular emphasis on developing capacity within organizations to derive value from Data.
• Improved Data quality.
5.4.3Business intelligence dashboards and reports
BI, analytics and reporting have been key in transforming KRA to be a Data-driven revenue administration since it helps in decision making by giving customized and timely reports on key performance indicators and identify gaps that require operational and strategic focus. This level of visibility is very useful in decision making across revenue departments, research, risk management, investigations, marketing, and tax base expansion among other operational functions. But is also valuable as a means of communicating performance and achievements to senior management, highlighting problem areas to justify further focus, as well as to customers, auditors, and government. With the capabilities of the tools in the Data warehouse, KRA can get a detailed, transparent, and meaningful representation of the complex relationships of customers as well as to conduct tax and customs risk assessments. Statistical information is disseminated in the form of dashboards and reports which are customized to meet the specific needs of the users. The dashboards are refreshed daily to ensure that information is up to date. A total of 60 dashboards have been created for different users and new requirements keep emerging with the ever-changing business environment. The challenge is to build capacity for customers for self-service BI and reduce reliance on the analytical team for BI development.
5.4.4Risk profiling and case management
As the KRA works towards enhancing compliance in paying taxes it is of necessity to increase the visibility of citizens’ economic activities and identify gaps in compliance. Towards achieving this mandate, the authority is leveraging on Data to drive a risk-based compliance agenda. Risk profiling entails developing profiles based on predefined parameters used to identify and rank non-compliant tax-paying entities. The DWBI has profiling tools and leverages on Data collected from multiple systems including third party Data. Through this approach, customers are profiled based on their history of compliance as either high, medium or low risk through the risk profiling tool. This profiling enables KRA detect tax evasion through monitoring of fraudulent behavior and hidden patterns in customers. In the long run, this goes a long way in enhancing revenue mobilization by broadening the tax base and fostering tax compliance.
The case management solution monitors workflow of segmented cases assigned to revenue officers. These cases range from a taxpayer audit, debt, a disputed assessment, an outstanding return, and responding to taxpayer correspondence. These cases are prioritized based on the risk assessment and electronically assigned to officers. This makes it easy for top management to make Data driven and timely decisions as a result of the information availed. It also helps maintain an audit trail of any access or adjustments made. The end goal is transparency in the audit process and saves on the time spent on case approvals and follow-ups.
5.4.5Data literacy and culture
Towards improving the institutional capacity to utilize Data, a Data literacy and culture strategy was developed and implemented. Data stewards were identified at all levels to communicate, educate, and promote the importance and value of Data and information assets as well as articulate the responsibility of various departments in Data management. This is gradually raising stakeholder and user’s awareness and appreciation of the value of Data in decision making as well as proper management of the same.
All Data producers and information consumers must understand Data policies and the organization ‘s commitment to enhancing Data quality, security, protection, utilization, and communication. All stakeholders are made be aware of Data stewardship and governance programs, organizations, roles, and responsibilities.
5.5Developing partnerships in implementation
Technological investments are expensive and may take longer to be prioritized for funding. Realizing this KRA developed bilateral and multilateral partnerships in implementing this transformation agenda. Partners institution have given support in acquisition of tools, developing technical skills, building Data literacy and culture, and consultancy in critical areas of program delivery. Currently the programme is working with three development partners.
6.Key outcomes
The following outcomes have been realized;
• Improved Data quality; Data quality maturity levels improved from 2 to 3 within one year of implementation. Over 90% of all customers’ bio Data have been cleaned, thereby improving traceability as well as enhanced our knowledge on our customers. Duplicate pins have been removed, Strategies for clean-up and migration of customer’s transactional Data from legacy systems are currently being implemented. Data has been migrated from one of the legacy systems. Connection to critical third party Data sources for purposes of validating registration details has been established.
• Improved Data security; Data Security maturity level improved from 2 to level 3. This has been achieved through the implementation of Data protection and security policies. The implementation of polices is monitored quarterly.
• Improved Data utilization; Data utilization index improved from 63% to 72%. Data warehouse solution has been implemented. The Data warehouse is the central repository of Data from all internal sources as well as Data from third parties, thus creating complete view of customers’ activities. Sixty (60) BI dashboards and reports have been created and customized to meet the needs of users.
• Risk profiling function has now been operationalized. Tax compliance initiatives are now largely risk and intelligence driven resulting to higher revenue yield per officer.
• The BI dashboard had reduced the time revenue officers spend analyzing Data. Dashboards are available at a click of a button and are refreshed daily.
• Integration with several third-party Data system sources for real-time and seamless flow of Data has also been achieved.
• A Data stewardship programme has been established to spearhead Data literacy towards a Data driven culture within the enterprise.
7.Challenges and lessons learnt
Despite the gains made, KRA Data journey has had its good share of challenges.
• Prioritize Data Quality; Data quality has been the biggest impediment to realization KRA goal of being insight-driven Revenue administration. It is of essence that Data quality issues are identified and addressed early since if left unaddressed they can create huge inefficiencies in revenue administration as well as create opportunities costs in revenue collection.
• Culture Change; Culture charge is critical for an organization that is transforming to be Data-driven and it must be driven from the top. There is a need for leaders to believe in the Data agenda and influence their people to influence people to change their mind-set on the value of Data. It helps if everyone has visibility on how Data adds value to their day to day operations.
• Start small demonstrate value and grow gradually; People will buy into new ideas when there is evidence of value.
• Leverage on open sources; Technological investments are expensive and may take longer to be prioritized for funding. It is the responsibility of the Data teams to identify technologies that are within the allocated budgets. Consider the use of open source technologies where possible.
8.Next steps – adoption of emerging technologies
KRA is working towards using Data in real-time and adopting advanced analytics in economic analysis, extracting all Data of economic relevance for insight to help improve revenue management and compliance with tax policies. Machine Learning and Advanced Analytics can be leveraged as a tool for improving efficiency in the administration of taxes. This entails the extensive use of Data, statistical and quantitative analysis, explanatory and predictive models, and fact-based management to drive decisions and actions. Advanced analytics can positively impact tax administration in many scenarios:
• Auditing; Tax administrations can extract insights from large Datasets, assess the next-best alternative, analyze social networks, or predict unreported income.
• Taxpayer Supervision; Maximizing risk detection and intelligent audits – Areas that can benefit from this include VAT refunds payment, taxpayer education, taxpayer services, customs declaration fraud detection, debt management, and taxpayer base expansion.
• Policy evaluation; Tax administrations can better measure tax gaps or assess the impact of policy changes.
• Taxpayer segmentation; Tax administrations can identify groups based upon similarities on set criteria or predict response to intervention.
• Fraud Detection; KRA will be able to analyze big Data sets thus able to detect specific taxpayer patterns based on historical Data analysis.
• Integrating with third-party systems will enhance efficiency in service delivery, simplify the process of filing returns by prepopulating forms, and enhance Data quality by validating Data with third-party Data sources. KRA plans to invest in API technology investing to assist in cloud migration. APIs are also key in Data sharing initiatives.
9.Conclusion
KRA has made progress towards achieving its goal of being an insight-driven enterprise through Implementation of the Data governance and Data utilization strategies. This entailed leveraging on Data and advanced digital systems, as well as adopting good practice in Data management and utilization. The last one year of has seen remarkable improvements in Data quality, security, privacy, access, availability and utilization. This has positively impacted on operational efficiency and customer experience.
KRA continues to work towards achieving best practice in Data management and optimum Data utilization. This will be achieved when quality Data flows real-time seamlessly between systems and returns forms are pre-populated using intelligently mined Data processes, optimum compliance to tax laws is achieved and there is realization of the full value of Data. This is necessary to not only ensure adequate flows of exchequer revenues but also meet the changing needs and expectations of the 21st Century.
References
[1] | https://bi-survey.com/Data-governance/ accessed om 08/07/ 2020. |
[2] | Seiner RS. 2014 Non-Invasive Data Governance. |
[3] | https://Dataprivacymanager.net/ accessed om 08/07/2020. |
[4] | Dedić N, Stanier C. “Measuring the Success of Changes to Existing Business Intelligence Solutions to Improve Business Intelligence Reporting” Lecture Notes in Business Information Processing. 268. Springer International Publishing. (2016) . 225–236. |
[5] | Davenport TH, Harris JG. Competing on Analytics: The New Science of Winning. (2007) . Harvard Business School Press. ISBN 978-1-4221-0332-6. |
[6] | Ramaswamy P. How to Create a Data Culture (PDF). Cognizant. June 2015. Retrieved 29 November 2017. |
[7] | Mark A, Dalton C. Multi-Domain Master Data Management. (2015) . |
[8] | Zachman ZI. Thoughts on the Transition from the “T” in IT to the “I” in IT. Originally written as a Foreword to Data Management Body Of Knowledge Book 2 (DMBOK2). (2019) . |