Semantic models and services for conservation and restoration of cultural heritage: A comprehensive survey
Abstract
Over the last decade, the Cultural Heritage (CH) domain has gradually adopted Semantic Web (SW) technologies for organizing information and for tackling interoperability issues. Several semantic models have been proposed which accommodate essential aspects of information management: retrieval, integration, reuse and sharing. In this context, the CH subdomain of Conservation and Restoration (CnR) exhibits an increasing interest in SW technologies, in an attempt to effectively handle the highly heterogeneous and often secluded CnR information. This paper investigates semantic models relevant to the CnR knowledge domain. The scope, development methodology and coverage of CnR aspects are described and discussed. Furthermore, the evaluation, deployment and current exploitation of each model are examined, with focus on the types and variety of services provided to support the CnR professional. Through this study, the following research questions are investigated: To what extent the various aspects of CnR are covered by existing CnR models? To what extent existing CnR models incorporate models of the broader CH domain and of relevant disciplines (e.g., Chemistry)? In what ways and to what extent services built upon the reviewed models facilitate CnR professionals in their various tasks? Finally, based on the findings, fields of interest that merit further investigation are suggested.
1.Introduction
Over the past decade, the Cultural Heritage (CH) domain has gradually adopted knowledge representation methods and tools of the Semantic Web (SW) for providing a common base for structuring and managing cultural data [16]. This practice initially emerged as an efficient way to address interoperability issues of (commonly heterogeneous and scattered) cultural data, addressing the need for unified collection, management, and exchange of data between different CH Institutions [77,100]. Primarily, web ontologies have been widely adopted by the CH community for representing the domain, in order to achieve data interoperability, as well as to provide platforms and services to efficiently discover and share domain knowledge between remote interconnected sources [100]. In this context, web ontologies have attracted particular attention within the Conservation and Restoration (CnR) of the tangible CH research community, as a means for representing and sharing knowledge and data.
The primary aim of CnR of tangible CH lies in the maintenance of the physical, aesthetic, and historical integrity of conservation objects,11 ensuring preservation and access for present and future generations [68,83]. Tangible CH encompasses i) movable CH, which refers to portable objects such as paintings, coins, and manuscripts, ii) immovable CH, which refers to built works such as monuments and sites and iii) underwater CH, which refers to objects or whole sites that are under water such as shipwrecks, ruins, cities [8,107]. CnR professionals seek to understand the original and present condition of conservation objects and to determine the actions required in order to manage the change and sustain the values22 of the conservation objects [39]. In doing so, CnR professionals follow a certain process in order to decide if and how to act, which generally may comprise up to six stages [39,68]:
i. initiation of the CnR project, during which the problems of the conservation object and the requirements and objectives of the project are specified,
ii. risk evaluation, during which potential risks regarding health and safety, potential hazards of CnR actions (or inactions), compatibility of actions etc. are identified,
iii. consideration of options and selection of CnR actions, during which potential remedial, preserving or restoring actions are considered for suitability, according to specifications recorded in previous stages,
iv. design of the CnR plan, during which an action plan is specified and agreed between all involved parts,
v. implementation of the agreed plan, during which the agreed actions are carried out,
vi. completion of the CnR project, during which the outcome of the actions is recorded and evaluated, and guidelines for future maintenance and handling of the conservation objects are proposed.
Documentation,33 as well as management of documented information, constitutes an integral part of CnR which occurs during all the different stages of the CnR process [64]. CnR professionals and scientists collect and disseminate information from and to the wider CH knowledge domain [64]. Practically, they need to accumulate and exchange a wide range of information regarding material and immaterial aspects of the conservation object, and of similar conservation objects, as well as general knowledge and implementation examples about diagnosis methods and results, conservation interventions and preventive measures [6]. The combination of these pieces of information is critical to the overall CnR process, since professionals reach conclusions, make decisions, and eventually conduct the appropriate intervention based on this information [34,84]. For this purpose, CnR professionals need to access, compare, combine, analyze, and often visualize the relevant information (either retrieved or produced).
However, up to now, CnR actors face the problem of limited means for retrieving and linking information, mainly due to the fact that CnR-related data are usually heterogeneous and often fragmented, for a number of reasons. First, CnR laboratories record their data in databases isolated from each other, each one developed according to different requirements which stem from different specializations [81,83,110]. Second, CnR data can be found in various forms, structured (e.g., in the form of relational databases), semi-structured (XML annotated documents) or unstructured (free texts), and, as such, are not semantically interoperable [84,110]. Lastly, the CnR domain heavily suffers from terminology inconsistency, since domain specialists tend to use specialized terms in diverse ways44 [84,115]. Even though difficult to achieve, data interoperability (syntactic as well as semantic) and exchange is vital for the CnR domain, and the SW provides very promising means to tackle the aforementioned issues, paving the way to effective and efficient organization and management of information [84,110]. Information integration from different sources, efficient retrieval, and juxtaposition of information, as well as identification of conservation issues and recommendation of solutions are some services which SW technologies may provide, thereby significantly facilitating the various stages of the CnR process in terms of information management and exploitation [14,19,84]. In this context, the CnR community has made ample attempts to develop semantic models for representing aspects of CnR knowledge and to deploy those models in various data modelling and management tasks, proposing interesting solutions that could dramatically change the way conservation experts acquire and utilize CnR information for supporting the CnR process.
In response to the increasing interest of the CnR domain in semantic representation methods, this paper reviews semantic models that have been developed and deployed in the context of the CnR domain. The gathered works propose mainly (but not exclusively) formal ontologies. The scope and development methodology of each model are described, while the fundamental aspects of the underlying conceptualization are highlighted. Furthermore, the evaluation and deployment (if any) of each model as part of a SW system is examined, with focus on the types and variety of services provided to support the CnR professional. Based on the study, the following research questions are investigated: (a) To what extent the various aspects of CnR are covered by existing CnR models? (b) To what extent existing CnR models incorporate models of the broader CH domain and of relevant disciplines (e.g., Chemistry)? and (c) In what ways and to what extent services built upon the reviewed models facilitate CnR professionals in their various tasks?
The remainder of the paper is structured as follows. In Section 2, the methodology of the survey is discussed. In Section 3, each reviewed work is presented, based on a set of predefined axes (scope, development, coverage, deployment, evaluation, exploitation). The paper concludes with a discussion that summarizes interesting observations over the reviewed models as well as paths that merit further research, towards a more active and well-rounded support of the CnR process.
2.Methodology
As mentioned, the current survey reviews knowledge models in the context of the CnR domain that have been developed using SW technologies and methods. The research of the literature was conducted using the data sources of Semantic Scholar, Springer Link, ScienceDirect and AATA Online, and searching for topics related to Conservation, Cultural Heritage, Ontology(ies), Semantic Web and CIDOC CRM. It should be noted that more general or top-level models that have also been used for CnR data modelling are reported at the introduction of Section 3 for completeness. However, they are not discussed extensively since the current survey is meant to focus on specialized models developed in the context of the CnR domain.
In terms of coverage, the models were reviewed according to the basic aspects of CnR information [75]:
– administration, which refers to general information about the conservation object, such as identification information (accession number, title, creator, dimensions etc.), ownership, preservation, and management (date of action, location of action, involved stakeholders etc.),
– materials & technology, which refers to information about i) production materials and techniques and ii) structural layers and components of the conservation object,
– alteration, which refers to information on the causes (e.g., radiation), processes (e.g., photooxidation) and effects (e.g., peeling) of the conservation object’s deterioration,
– investigation, which refers to a variety of activities aiming to the acquisition of information either directly from the conservation object and its environment or from other sources (e.g., bibliography, experiments), and the processing of this information for the purpose of condition assessment, alteration diagnosis and eventually determining intervention requirements,
– intervention, which refers to information about planning and implementation of actions in order to i) prevent future deterioration of the conservation object (preventive conservation), ii) ensure the long-term safekeeping of conservation objects (remedial conservation) or iii) make changes to the conservation object so that it optimally approximates its original state or other previous state (restoration).
In Section 3, along with the presentation of each model a table is provided that explicitly states which CnR aspects the model covers, by mapping the model’s thematic clusters or top-level classes to specific CnR aspects. This mapping provides an essential terminology harmonization in cases where different models use diverse terms to represent the same CnR aspects. For example, the thematic cluster Paint of one model and the thematic cluster Component and Construction Description of another model may use completely different terminology, but according to their description they both correspond to the CnR aspect Materials & Technology.
It is important to clarify that the different CnR information aspects may be collected and recorded during different stages throughout the CnR process. For example, information regarding a CnR intervention may be collected and recorded during the stages of risk evaluation, identification, evaluation, and selection of CnR options (general information on interventions), as well as during the stages of development and agreement of a plan and implementation of the agreed plan (specific information on implemented intervention cases).
3.Models review
CnR is a multidisciplinary domain, which lies within the wider CH domain. As such, formal ontologies which have been developed within the CH domain may cover, at different abstraction levels, CnR requirements regarding data modelling. For example, the International Committee of Documentation Conceptual Reference Model (CIDOC CRM) of the International Council of Museums (ICOM) is a widely used top-level ontology for the representation of CH data which includes classes and relations that represent at some extent some CnR aspects [25,36]. CIDOC CRM provides the basic classes and relations that represent the various CH disciplines and is extended by ten modular models which cover documentation requirements of specific disciplines of the CH domain (FRBRoo, PRESSoo, CRMinf, CRMarchaeo, CRMsci, CRMgeo, CRMdig, CRMba, CRMtex, CRMsoc) [26]. The different versions of both the CIDOC CRM55 and the CRM official extensions66,77,88,991010,1111,1212,1313,1414,1515 are available online. The models are provided in RDF form – although partially – supporting exploitation of SW technologies [32]. The CIDOC CRM and its official extensions have been used for CnR data modelling through the years [66,82,120].
Another, analogous example is the more recent Architecture of Knowledge (ArCo) ontology network, which is the result of a collaboration between the Institute of Catalogue and Documentation (ICCD), of the Italian Ministry of Cultural Heritage, and the Institute of Cognitive Sciences and Technologies of CNR (Italian National Research Council) [21,22]. The project aims at modelling the domain of Italian CH using a network of formal ontologies in order to publish ICCD data as LOD.1616 ArCo ontology network reuses other ontologies, such as OntoPiA [88] and Cultural-ON [67] and it is aligned to existing upper-level ontologies of the CH domain, such as Europeana Data Model (EDM) [24] and CIDOC CRM [25]. ArCo consists of seven ontology modules in order to cover the various CH aspects, including -at some level- the aspects of the CnR domain [22]. The ArCo ontology network (owl files and relevant documentation) is available online.1717
In addition to the use of CH related ontologies for the representation of the various aspects of CnR information, the CnR community has developed specialized models, which in some cases integrate and/or extend existing models of the CH domain. After a thorough bibliographic research, 16 works were identified, which were gathered, studied, and are presented below. The presentation of the reviewed works follows a chronological order (oldest first). The bibliographic research spans from 2011, when the first endeavors are dated, up to today. In case of multiple publications on the same work, the initial publication is taken into account in the ordering. Each work has been reviewed and is presented here according to six study axes:1818 i) scope and context of the project, ii) model development methodology, iii) coverage (in terms of CnR information aspects – see Section 2), iv) deployment of the model as part of a SW system or service, v) evaluation of the model and its deployment as a SW system or service, vi) current exploitation of the model and system/service for the development of ontologies within the CnR domain.
3.1.Ontology of paintings and preservation of art
Twentieth Century Paint Project1919,2020 (20thCPaint) explored the preservation of 20th C. paintings in Asia and the Pacific [52]. Key objective of the project was the development of an online knowledge base that allows painting conservators to access integrated and structured information about conservation of 20th c. paintings and facilitates information exchange between painting conservators and other preservation experts.2121
In this context, [52] proposes the Ontology of Paintings and Preservation of Art (OPPRA), a semantic model specialized in CnR of paintings. The ontology was developed in order to: i) capture CnR documentation data, ii) capture data from published papers and iii) integrate the aforementioned data that derive from both internal and external data sources so that reasoning can be performed over them.
The development of OPPRA was organized in five stages [87]: i) conceptual modelling of information relevant to conservation of paintings, ii) reuse of existing models, iii) reuse of existing controlled vocabularies, iv) extension and refinement of the reused classes and relationships, v) evaluation of ontology applicability to services developed in the context of the 20th CPaint Project. The ontology is implemented in Web Ontology Language Description Logics2222 (OWL DL) using the Protégé2323 software (for further information see [80]). Also, logical rules were defined using OWL 2 RL2424 (implemented in OWLIM -currently GraphDB-2525 OpenRDF Sesame triple store2626).
OPPRA combines and reuses the CIDOC CRM [25], the Open Archives Initiative Object Re-use and Exchange standard (OAI-ORE) [61] and the OreChem model [60]. Certain classes of OPPRA extend classes from CIDOC CRM [25] and OreChem (see [87]). Furthermore, OPPRA reuses the following controlled vocabularies: Getty Art and Architecture Thesaurus (AAT) [47], Visual Glossary of the Australian Institute for the Conservation of Cultural Material (AICCM) [4], International Network for the Conservation of Contemporary Art Database for Artists’ Archive (INCCA) [87], RUG Spectral Database (from now on RUG) [53], Conservation and Art Materials Encyclopedia Online (CAMEO) [72] and US National Institute of Standards and Technology Chemistry WebBook (from now on NIST) [86]. OPPRA consists of 2325 classes and 181 relations (either new or reused) [87].
OPPRA classes and relations model information related to the following thematic clusters [52,87]: i) Painting, which represents the identity of the conservation object (e.g., title, artist, period, technique, genre, condition, owner, custodian, provenance), ii) Paint, which represents the production material(s) and technique(s) of the conservation object (e.g., paint name, type, chemical property, physical property, pigment, additive), iii) Paint Decomposition, which represents alterations of the conservation object (e.g., type, cause, physical/chemical process/reaction), iv) Paint Analysis Method, which represents the analysis of the conservation object (e.g., SEM, TEM, FTIR, Raman), v) Paint Conservation/Preservation Treatment, which represents the remedial or preventive conservation of the conservation object (e.g., cleaning, environmental conditions control), vi) Experiment, which represents experiments that either simulate a damaging process or test a potential conservation treatment, vii) Publications, which represents published works related to CnR processes, experiments, and case studies. Table 1 depicts the correspondence between the CnR aspects (see Section 2), and the thematic clusters covered by OPPRA.
Table 1
Correspondence between the CnR aspects and the thematic clusters covered by OPPRA
CnR Aspect | OPPRA thematic cluster |
Administration | Painting |
Materials & technology | Paint |
Alteration | Paint Decomposition |
Investigation | Paint Analysis Method, Publications |
Intervention | Paint Conservation/Preservation Treatment |
Based on OPPRA, a system that consists of an online knowledge base and intelligent services, specialized in CnR and Conservation Science (CS)2727 was developed [87]. The system allows the import of documentation and description of experiments conducted by conservators and material scientists, and the uploading of the experimental data to the knowledge base. Furthermore, it allows automatic extraction of structured data about past research and experiments from publications and websites that are relevant to art conservation. The extracted data from published papers are integrated into the knowledge base, together with data from external databases, allowing their linking to the imported user data and consequently a seamless unified search over critical information about conservation, art history and materials science. The knowledge base and services do not appear to be open for access, while it is not clear if they are still in use.
OPPRA was evaluated by assessing its applicability to the aforementioned services [87]. The evaluation showed that conservators and scientists were able to document and link data using OPPRA, thereby achieving more precise data retrieval. Additionally, the automatic extraction of structured data from relevant publications achieved high accuracy. Finally, the semantic search of integrated cross-disciplinary data hosted in the knowledge base allowed more complex queries, compared to traditional data integration tools.
OPPRA used to be online available, though the url is no longer accessible.2828 The bibliographic search showed no evidence of the model’s re-use in later projects for the development of ontologies within the CnR domain.
3.2.Monument damage ontology
The main objective of the Monument Damage Information System (MONDIS) project2929 was the development of the MONDIS system. MONDIS is a knowledge-based system dedicated to damage diagnosis and possible conservation interventions of historical buildings [20].
MONDIS project developed Monument Damage Ontology (MDO) in order to efficiently integrate, organize and process diverse information related to the domain of interest, and eventually support documentation and monitoring of historical buildings damages, as well as potential intervention planning/application [11,20].
The development of MDO was organized in three phases [20]: i) distinguishing the requirements of damage documentation according to literature and international standards, ii) establishment of the relations among damage factors based on CnR methodologies and workflows, iii) validation of the ontology with experts. MDO was implemented in OWL 2 using OpenRDF Sesame triple store.
MDO is divided in two parts: i) the core, which represents knowledge about damages of immovable CH and ii) special taxonomies which provide particular vocabularies for the documentation and analysis of damages and interventions [11]. MDO integrates (either partially or fully) the following taxonomies, thesauri, and glossaries: International Database and Gallery of Structures (from now on IDGS) [103], Material and component classification from Strufail (MCCS) [37], Taxonomy of Building Components for Performance-Based Earthquake Engineering (TBCPBEE) [94], RTS: Trıdnık stavebnıch konstrukcı a pracı (RTS) [11], ICOMOS illustrated glossary on stone deterioration patterns (ICOMOS) [111], On Site For Masonry Standard Damage Catalogue and List of Structural Typologies and Related Requirements (OSM) [11]. The core ontology consists of 59 classes and 119 relations,3030 while the version of the ontology with the special taxonomies consists of 1258 classes and 121 relations.3131
The core part of MDO is divided in five thematic clusters [11,19]: i) Component and Construction Description, which represents the physical and functional characteristics of a conservation object ii) Events, which represents occurrences that can influence the condition of a conservation object, iii) Damage Diagnosis and Intervention, which represents alteration processes that affect a conservation object, as well as remedial or preventive conservation and restoration activities that confront the alteration processes and their effects, iv) Risk Assessment, which represents the analysis of a potential event or effect that can potentially harm a conservation object, v) Measurement Assessment, which represents the measurement of the magnitude of alteration factors and effects. Table 2 depicts the correspondence between the CnR aspects (see Section 2) and the thematic clusters covered by MDO.
Table 2
Correspondence between the CnR aspects and the thematic clusters covered by MDO
CnR Aspect | MDO thematic cluster |
Administration | – |
Materials & technology | Component and Construction Description |
Alteration | Events, Damage Diagnosis and Intervention |
Investigation | Risk Assessment, Measurement Assessment |
Intervention | Damage Diagnosis and Intervention |
Based on the MDO, MONDIS knowledge-based system provides a set of tools for data import, editing, integration, processing, and visualization [18]. More specifically MONDIS includes the inputting applications i) MONDIS mobile/desktop app and ii) Ontomind profile, as well as the visualizing and supporting tools iii) MONDIS explorer, iv) knowledge matrix and v) terminology editor. MONDIS application allows the documentation (on-site for the mobile version) of the condition of historical buildings based on measurements and observations about examined damages [18,19]. The data are uploaded to the MONDIS server, and after being validated by the users -in order to verify the quality of the record- they become accessible through the MONDIS explorer. Once shown in the MONDIS explorer records can be integrated with extra information which was not collected and documented during the on-site examination of a building, via Ontomind profile. Ontomind visualizes the ontological mapping of records to the MDO as a simple tree-like structure. The records available in the MONDIS are semantically linked to their diagnosis and possible interventions which are visualized and presented to the user through the knowledge-matrix web-based application. Finally, the MONDIS terminology editor facilitates the browsing or editing of the taxonomies and term lists used in MONDIS software tools. The software tools are available online.3232
Each section of MDO was validated by conducting public workshops and internal meetings [20]. Furthermore, MDO has been populated with records which were used for the presentation of MONDIS software tools functionality [18].
Both the core MDO ontology and the MDO version with the taxonomies are available online. According to the bibliographic search, MDO has been re-used by [118] for the development of a new ontology within the CnR domain (see Section 3.9).
3.3.Color and space in cultural heritage knowledge representation
The Color and Space in Cultural Heritage (COSCH)3333 project aimed to enhance the mutual understanding of tangible CH documentation between the various experts of CnR and Preservation3434 of the CH domain [14].
An important outcome of the COSCH community research is COSCH Knowledge Representation (COSCHKR), a semantic model which encodes experts’ knowledge on good practices for visual documentation and analysis (with non-invasive techniques) of tangible CH.
COSCHKR was developed through an iterative process where the gathered knowledge was first verified by groups of experts [55,114]. The experts participated in the specification of relevant terms and vocabularies as well as in discussions over three representative case studies, contributing to the development of COSCHKR class structure and dependencies as well as the specification of inference rules [114]. The ontology was implemented in OWL 2.
The top-level structure of COSCHKR consists of five classes interrelated through five properties [14]. In general, the COSCHKR ontology contains more than 750 classes, while its taxonomic hierarchy has on average five levels [114]. COSCHKR subclasses are associated with inference rules, which cut across the top-level classes (e.g., Revelation of Underdrawing (subclass of CHApplications) has Requirement 2D_Data (subclass of Data)). While the COSCHKR does not reuse some existing ontology, in the context of its development there was the intention to keep it in line with CIDOC CRM in order to maintain information interoperability.
The core of COSCHKR consists of five top-level classes [14]: i) Physical Thing, which represents a conservation object to be measured in terms of its physical and visual characteristics, ii) CH Applications, which represents CH research questions applying to spectral or spatial data, iii) Data, which represents digital/analogue data and document types that are either generated or used to process existing/generated data, iv) Technologies, which represents technical processes, measurement principles, tools/instruments and the way they are set up to generate or process data, and v) External Influences, which represents limitations of a documentation/analysis project (in terms of location, budget, environmental conditions etc.). Table 3 depicts the correspondence between the CnR aspects (see Section 2) and the top-level classes of COSCHKR.
Table 3
Correspondence between the CnR aspects and the top-level classes of COSCHKR
CnR Aspect | COSCHKR top-level classes |
Administration | – |
Materials & technology | Physical Thing |
Alteration | Physical Thing |
Investigation | CH Applications, Data, Technologies, External Influences |
Intervention | – |
Based on COSCHKR, [114] describes a recommender system that enables experts from different subdomains of CnR and preservation of tangible CH to put forward their queries and get answers related to documentation/analysis strategies for CH objects and applications without worrying about the complexity of the backend model [55]. Particularly, the proposed system would allow seeking answers to queries of varying complexity and invokes the model to infer underlying facts and heuristics. First of all, the system aims to help users to identify useful factors for different documentation/analysis actions as well as factors that cannot be satisfied, using implicit reasoning. Afterwards, based on those factors, the proposed system would be able to recommend solutions, providing experts with an overview of optimal spectral and spatial recording strategies according to their needs.
The three representative case studies were not only used to enrich the knowledge model but also to evaluate the inference mechanism and results [114]. The evaluation results [114] showed that the inferences of the model are satisfying, though it is highlighted that COSCHKR is still under development, and therefore the CH applications, inference rules and corresponding recommendations that are to be added in the future will improve the model and its performance.
The ontology is not yet available online, but it is intended to be documented and made publicly available [114]. Additionally, the OWL file may be provided (as noted in the project website3535). The bibliographic search showed no evidence of the model’s re-use in later projects for the development of ontologies within the CnR domain.
3.4.DOC-CULTURE
The Development of an integrated information environment for assessment and documentation of conservation interventions to cultural works/objects with nondestructive testing techniques (DOC-CULTURE)3636 project explored non-destructive testing and evaluation techniques (NDT&E) in three axes: i) the NDT&E usage in assessment of conservation object condition and conservation interventions effects, ii) the documentation of NDT&E data through metadata and conceptual frameworks and iii) the implementation of an information system for the recording and storage of NDT&E data [57,58].
In the context of the DOC-CULTURE project a model which is based on CIDOC CRM [25] and Dublin Core (DC) [38] models together with a CIDOC CRM extension are proposed for facilitating the complete representation of CnR processes and stages, including NDT&E techniques [58,109].
For developing the CIDOC CRM extension, the intended user groups, the documentation requirements and the different CnR processes and stages were defined, and the main entities and properties of NDT&E were specified [58,109]. Next, different standards for modelling data related to the CH domain (namely Metadata Object Description Schema (MODS) [71], Resource Description and Access (RDA) [99], PREMIS [95] and Muse Meta [59]) were studied and employed for the representation of DOC-CULTURE entities and properties. Furthermore, extra classes required in order to better represent the NDT&E field were added. Particularly, based on the study conducted in the context of the DOC-CULTURE project, the extension of three CIDOC CRM classes (namely E7_Activity, E11_Modification and E4_Period) were proposed [58].
The five main entities of DOC-CULTURE model are [109]: i) Artifact, which represents the identity, materials and technique and preservation state of the conservation object, ii) Conservation, which represents CnR interventions, iii) Measurement, which represents measurement actions, iv) Equipment, which represents equipment used for CnR and measurement actions and v) Digital Documentation, which represents both the digital representation of the conservation object (e.g., digital images) and any digital file produced during CnR or measurement actions. Table 4 depicts the correspondence between the CnR aspects (see Section 2) and the main entities of DOC-CULTURE.
Table 4
Correspondence between CnR aspects and the main entities of DOC-CULTURE
CnR Aspect | DOC-CULTURE main entities |
Administration | Artifact, Digital Documentation |
Materials & technology | Artifact |
Alteration | Artifact |
Investigation | Measurement, Equipment, Digital Documentation |
Intervention | Conservation, Equipment, Digital Documentation |
The proposed model has been used for modelling data related to conservation interventions provided by the National Archaeological Museum of Athens [59]. Additionally, the model has been deployed in an information system built for the purposes of the DOC-CULTURE project. The system consists of the following subsystems, which provide the respective services [59]: i) image processing, which allows the application of filters on images of conservation objects, ii) numerical analysis, which provides functions for the identification of past conservation processes and detects lesions on the surface of a conservation object, iii) metadata management, which allows the matching of metadata elements to database columns, iv) image annotation, which enables the addition of annotation marks on an image together with information about previous CnR processes and v) documentation management functionality, which implements the proposed metadata scheme as well as the documentation process conducted by CnR experts.
The model and CIDOC CRM extensions are not available online. The bibliographic search showed no evidence of the model’s re-use in later projects for the development of ontologies within the CnR domain.
3.5.Ontology for degradation phenomena and annotation on 3D reconstructions
In [69] a correlation pipeline is proposed for the integration of the three dimensions of a masonry structure: i) semantic dimension, which refers to concepts used by experts in order to describe conservation state, ii) spatial dimension, which refers to spatialized annotations on 3D representations, iii) morphological dimension, which refers to morphological descriptors (e.g., occlusion, curvature, roughness) of annotated regions of 3D representations.
The pipeline uses an ontology (ODPA-3DR) for recording and integrating multidisciplinary observations of the conservation state of masonry structures, spatialized into a reality-based 3D representation3737 [69].
ODPA-3DR was developed based on Lassila’s method [63] and was implemented using Protégé [70,80]. The development of the ontology had to meet the requirements of semantic digital acquisition of data related to the morphology of a masonry structure (e.g., photography), the annotation of 2D or 3D representations of a masonry structure, as well as the four concepts for describing a masonry structure and its conservation state [70]: i) Material, ii) Building technique, ii) Architectural component and iv) Alteration. For each of these concepts specialized thesauri were built, based on the terms collected from experts and existing glossaries (namely ICOMOS [111] and Architecture: méthode et vocabulaire (from now on ARC) [89]). In order to align the proposed ontology with the wider CH domain, certain classes were mapped to CIDOC CRM [25], while CIDOC CRM compatible models (namely CRMsci [31], CRMdig [29] and CRMinf [30]) were re-used.
Based on the presentation of ODPA-3DR ontology in [70], five main thematic clusters can be identified: i) Argumentation, which represents the scientific justification of the observed damages, materials and techniques of a conservation object, ii) Information, which represents documents and digital files about a conservation object, iii) Region, which represents a specific area on a conservation object, iv) Description Concepts, which represents material(s), building technique(s), architectural components and degradation phenomena of an area under study, v) Digital Acquisition, which represents the digitization of a conservation object. Table 5 depicts the correspondence between the CnR aspects (see Section 2) and the thematic clusters covered by ODPA-3DR.
Table 5
Correspondence between the CnR aspects and the thematic clusters covered by ODPA-3DR
CnR Aspect | ODPA-3DR main thematic clusters |
Administration | Information |
Materials & technology | Description Concepts |
Alteration | Argumentation, Description Concepts |
Investigation | Argumentation, Information, Digital Acquisition |
Intervention | – |
ODPA-3DR is deployed in a system for reality-based 3D semantic annotations of masonry structures’ conservation states [69,70]. The basic functionality of the system is as follows: the user inputs a set of digital images of a physical object, and marks 2D regions on these images. Based on the marks, the system extracts spatial relationships (by 2D-to-3D-to-2D projection), as well as morphological features (by morphological analysis). Then, the user annotates the marked regions with description concepts of different thematic layers. Finally, the user indicates references and resources that justify the annotation. The system is not available online.
In order to be populated for testing purposes, ODPA-3DR has been mapped to a database holding data generated by experts during digital acquisition and observation of a masonry structure (3D point cloud, scientific imagery, documents, etc.). The first results showed the possibility to calculate the overlapping degree between different annotations (associated with different description concept types) of the same structure (e.g., a wall) [70].
The ontology is not available online. The bibliographic search showed no evidence of the model’s re-use in later projects for the development of ontologies within the CnR domain.
3.6.PARCOURS semantic model
The Patrimoine culturel et Restauration-Conservation: Ontologies pour l’ Usage d’ un Referentiel commun aux differentes Sources de donnees (PARCOURS) project followed an ontology-driven approach in order to address the interoperability problem of CnR data provided by different CH Institutes and develop a common infrastructure for the semantic retrieval of integrated CnR data [84].
In this context, [84] proposed a semantic model of CnR of tangible CH, the PARCOURS semantic model, which aims to integrate CnR data from different sources, in order to enable the querying of data in a unified way.
The development of PARCOURS semantic model started with the definition of a core structure and the main CnR requirements of the ontology [10]. During this process scientists and domain experts of the CnR field were involved. Next, a set of sample data structures and example data related to the CnR processes was mapped to existing CH domain ontologies (EDM [24], ABC [36] and CIDOC CRM [25]). CIDOC CRM was considered as the most appropriate choice for CnR data modelling, and it was used and extended -where necessary- for the representation of the domain of interest. Finally, the developed model integrated a set of thesauri in order to tackle the problem of inconsistency among different CnR terms, at both the syntactic and semantic level. The PARCOURS semantic model uses OWL and RDF technology [83,85].
PARCOURS semantic model adopts a layered ontology architecture: i) a top-level ontology, ii) extensions of the top-level ontology with specialized classes, iii) specialized thesauri [83]. The PARCOURS semantic model reuses CIDOC CRM [25] and its official compatible model CRMsci [31]. Additionally, it introduces CRMcr, an extension of CIDOC CRM and CRMsci, developed in the context of the PARCOURS project [10]. The PARCOURS semantic model consists of i) 93 concepts and 82 relationships of CIDOC CRM ontology, ii) 22 concepts and 24 relationships of CRMsci and iii) 63 new concepts and 27 new relationships. Regarding the use of specialized thesauri, most of them were built during the PARCOURS project. They were managed by the Thesaurus Management System (TMS) Gestion Informatisée de Nomenclatures Collaboratives et Ouvertes3838 (GINCO) and were integrated into the CRMcr model [83].
The classes and relations of the PARCOURS semantic model represent knowledge related to five main thematic clusters [10]: i) Conservation object, which represents the identification, production and physical features of a conservation object, ii) Location and owners, which represents information about the conservation object’s locations and ownership during its lifecycle, iii) Degrading events, which represents alterations of a conservation object, iv) Non degrading events, which represent scientific studies and their results, as well as intervention activities, v) Instruments, which represents the equipment used during the various non degrading events. Table 6 depicts the correspondence between the CnR aspects (see Section 2) and the thematic clusters covered by PARCOURS semantic model thematic clusters.
Table 6
Correspondence between the CnR aspects and the thematic clusters covered by PARCOURS semantic model
CnR Aspect | PARCOURS semantic model thematic clusters |
Administration | Conservation Object, Location and owners |
Materials & technology | Conservation Object |
Alteration | Degrading events |
Investigation | Non degrading events, Instruments |
Intervention | Non degrading events, Instruments |
In the context of the PARCOURS project, a data integration and querying system for the CnR domain was developed based on PARCOURS semantic model, providing search and retrieval services [83,85]. The system has the form of a web portal that allows users to retrieve CnR data from multiple datasets. In order to achieve unified access to multiple datasets, the system follows a mediator approach which tackles restrictions imposed by CH institutions, allowing them to keep managing their repositories autonomously. In particular, all data sources involved in the integration process (namely Research Laboratory for Historical Monuments (LRMH) and French Museum’s Research and Restoration Center (C2RMF)) refer to the proposed model, which is used as the mediator. To process a given query, the system interacts simultaneously with the different databases and retrieves data concerning both the physical characteristics of the queried object (e.g., location, authors) and related events (e.g., type of event, used techniques, measurements, actors). Moreover, the system provides a key-word-querying interface, which allows the user to choose specific keywords from the thesauri linked with the PARCOURS semantic model. The system does not appear to be open for public use [83].
The ontology is not available online. According to the bibliographic research the extension CRMcr has been re-used by [121] for the development of a new ontology within the CnR domain (see Section 3.16).
3.7.Cultural heritage artefact partonomy
The GRAVITATE project3939 developed the GRAVITATE platform which provides tools for reconstructing, annotating, and analyzing 3D models of artefacts, as well as for retrieving information about artefacts [90]. The platform is particularly useful for archaeological and conservation study of CH artefacts that are not easily accessible.
A basic outcome of the GRAVITATE project is the Cultural Heritage Artefact Partonomy (CHAP), a SKOS vocabulary developed to describe the relationships between the parts and the overall context of two archaeological collections: the terracotta statues from the port of Salamis, attributed to the Neo-Cypriote style (ca. 600-500 BC), and the small clay statuettes from the Ayia Irini sanctuary, mostly attributed to the Cypro-Archaic period (700-500 BC).
The main classes of CHAP were defined based on an archaeological corpus of texts (i.e., archaeological publications, catalogues, excavation reports) as well as fundamental archeological knowledge [23]. First, the main hierarchy was developed. Next, the hierarchy was aligned to a semantic scheme suitable for representing knowledge about both artefacts and their digital counterparts. The CHAP model was edited in Protégé software [80].
The CHAP meronomy has been modelled as a SKOS hierarchy4040,4141 and has been aligned to a semantic scheme formed by a combination of CIDOC CRM [25], CRMdig [29], Common Shape Ontology (CSO) [108] and extra classes defined based on requirements of the GRAVITATE project. The extra classes and object properties were introduced as extensions of CIDOC CRM and CRMdig.
The semantic scheme can be divided in two main conceptualization aspects [23]: i) the Physical artefact, which represents statues and figurines, their features and dimensions and ii) the Digital artefact, which represents the digital counterpart of statues and figurines, the type of its geometric representation, its areas and areas’ dimensions. The CHAP meronomy is included in the first aspect and it is organized in five central concepts all related to statues and figurines [23]: i) Body part, which represents anatomical constituents and related characteristics (e.g., hairstyle), ii) Attire, which represents accessories (e.g., necklace), iii) Decoration, which represents figurative decorations and geometric decorations (e.g., flower), iv) Colour, which represents coloring, and v) Technique, which represents manufacturing techniques. Table 7 depicts the correspondence between the CnR aspects (see Section 2) and the conceptualization aspects covered by the CHAP semantic model.
Table 7
Correspondence between the CnR aspects and the conceptualization aspects of CHAP semantic model
CnR Aspect | CHAP semantic model conceptualization aspects |
Administration | Physical artefact, Digital artefact |
Materials & technology | Physical artefact |
Alteration | – |
Investigation | Digital artefact |
Intervention | – |
CHAP is deployed in the knowledge base of the GRAVITATE platform and it is exploited by the tools provided by the platform for analysis and annotation of 3D models of artefacts [23,90]. In particular, the GRAVITATE platform’s tools are i) inspection view, which facilitates the parallel visualization of different 3D models and geometric properties, ii) feature recognition, which provides automatic identification of features on 3D models of artefacts and iii) annotation mode, which allows the annotation of areas on 3D models of artefacts. The user can input qualitative and quantitative data about annotated areas, which are stored in the knowledge base of the platform. Data and metadata about artefacts and artefacts’ 3D models are stored in the knowledge base and can be retrieved using queries.
CHAP has been used for modelling the characteristics and production techniques of statues/figurines in three case studies [23]. CHAP has also been used for semantic annotation (automatic or manual), of 3D reconstructions of objects (whether whole artefacts or fragments of artefacts). The annotation of features observed on objects allows experts to search, retrieve and examine objects in juxtaposition (e.g., based on the morphological analysis of their decoration) in order to validate hypotheses regarding their production or original form (e.g., fragments that belong to the same statue) [23].
The ontology is not available online. The bibliographic search showed no evidence of the model’s re-use in later projects for the development of ontologies within the CnR domain.
3.8.Conservation process model
The Built heritage information modelling and management (BHIMM) project4242 explored building information modelling (BIM) techniques and solutions implemented in the CH domain for management and preservation of historical buildings [1]. In this context, an ontology-based deployment is proposed [1] which exploits Autodesk Revit,4343 a building information modelling (BIM) software.4444 The deployment aims to provide integration of geometrical and non-geometrical information related to conservation of architectural heritage.
For the purposes of the BHIMM project the Conservation Process Model (CPM) was developed, a semantic model that specializes in CnR of historical buildings. CPM has two main objectives: i) to represent knowledge about the related CnR processes and ii) to facilitate integration, mediation, and interchange of heterogeneous CnR data, at both the academic and the professional level [1].
For the development of CPM, the main thematic clusters of built heritage were defined. For the formalization of CPM, existing models were taken into account, namely CIDOC CRM [25], FRBRoo [35] and AR model [45]. CPM incorporates classes from CIDOC CRM since the former covers concepts and relations which are also required for the CPM domain of interest. Specialized terms of the ICOMOS [111] were mapped to CPM classes related to decay phenomena, in order to provide standardized terminology for decay specification. CPM was developed in OWL using the Protégé software [80]. Additionally, several rules were developed in Semantic Web Rule Language4545 (SWRL) in order to compare data resulting from different analyses so as to further validate the results of investigation processes [42].
Classes and relations of CPM are organized in five thematic clusters [42]: i) Artefact, which represents the architectural structure of buildings, ii) Investigation Process, which represents the examination of buildings with non-destructive/destructive methods, iii) Actors, which represents people related to the building’s history or study, iv) Lifecycle 1, which represents the description and analysis of alterations of materials and structure and v) Lifecycle 2, which represents conservation planning, interventions and general managing processes. Table 8 depicts the correspondence between the CnR aspects (see Section 2) and the thematic clusters covered by CPM.
Table 8
Correspondence between the CnR aspects and the thematic clusters covered by CPM
CnR Aspect | CPM thematic clusters |
Administration | Artefact, Actors |
Materials & technology | Artefact, Lifecycle 1 |
Alteration | Lifecycle 1 |
Investigation | Investigation Process |
Intervention | Lifecycle 2 |
Based on CPM, the authors of [1,101] present BIM Semantic Bridge, an ontology-based module that provides a connection between a BIM-based database and a knowledge base of CnR data. Particularly, BIM Semantic Bridge reconstructs the class hierarchies of the two databases, as well as assigned properties and derived instances. In this way, the representations of the two databases are homogenized, allowing the generation of correspondences between similar classes and data stored in the databases, in order to perform data comparison and transfer. BIM-based software Autodesk Revit has been used for the annotation of specific areas of decay or intervention on building models. The combination of Autodesk Revit and CPM facilitated geometrical representation of CnR data.
CPM has been used to represent and manage CnR information related to the 6th-century San Sabaoratory, in Rome, and its components [1,101]. Furthermore, the SWRL rules that had been formulated based on CPM were tested for information inconsistencies which had been provided by the same or different investigation activities and operators. As mentioned in [101], the achieved correlation, querying and inference making over the knowledge captured by CPM enhances the awareness of experts over any interpretation inconsistencies and proposed solution implications, thereby supporting them to choose the most suitable solution.
The CPM model file is not available online. According to the bibliographic search CPM has been re-used for the representation of conservation management of urban buildings, including their main features together with vulnerability and transformation index, using the Risk Map of Cultural Heritage system of Culture Ministry of Italian Republic (MiBAC) [2]. Additionally, [33] presents the development and use of an ontology which adopts the four thematic clusters of CPM (Artefact, Lifecycle 1, Investigation Process and Actors).
3.9.Built cultural heritage ontology
The authors of [118], present the Built Cultural Heritage ontology (BCHO), a semantic model for the preventive conservation of built CH. BCHO was developed in order to facilitate integration and exchange of heterogeneous information related to built CH.
BCHO was developed using On-To-Knowledge methodology [118], which consists of two processes, i) the Knowledge Meta process, which led to the construction of the ontology and ii) the Knowledge process, which specified activities for populating and testing the ontology [102]. Furthermore, BCHO was verified using OntOlogy Pitfall Scanner4646 a tool that detects anomalies in the ontology, improving representation quality [117]. The ontology was developed in OWL using Protégé [80].
BCHO reuses three external ontologies: i) Geneva CityGML, an ontology of the University of Geneva which is based on CityGML [56], ii) Monument Damage Ontology [11] and iii) Erlagen CRM/OWL, the OWL implementation of the CIDOC CRM top-level CH model [27]. Additionally, BCH ontology introduces a number of extra classes and properties in order to provide a complete semantic framework for management and preventive conservation of built CH [119]. In total, BCHO consists of 148 classes and 102 relations [117].
The basic classes of BCHO are organized in four thematic clusters [119]: i) Analysis, which covers the collection of information about the conservation object, ii) Diagnosis, which covers the assessment of current condition and potential risks of the conservation object, iii) Therapy, which covers preventive or remedial interventions (suggested or implemented), and iv) Control, which covers the assessment of the implemented intervention. Table 9 depicts the correspondence between the CnR aspects (see Section 2) and the thematic clusters covered by BCHO.
Table 9
Correspondence between the CnR aspects and the thematic clusters covered by BCHO
CnR Aspect | BCHO thematic clusters |
Administration | Analysis |
Materials & technology | Analysis |
Alteration | Diagnosis |
Investigation | Diagnosis |
Intervention | Therapy, Control |
So far there has been no deployment of BCHO in a particular SW system or service supporting the CnR process, though the possibility of using BCHO for data integration, 3D representation of buildings, inference making etc. is discussed [117].
In order to be evaluated, BCHO was tested in terms of its ability to represent information regarding the preventive conservation cycle of the San Luis seminary, a historical building in the city of Cuenca, Ecuador [117]. The evaluation showed that BCHO is able to represent the information required in each phase. However, the following needs are highlighted which arose during the evaluation [117]: i) guidelines for reference names, ii) user manual with examples of ontology usage, iii) addition of methodologies for risk assessment, intervention assignments, etc.
BCHO is available online,4747 though the creators mention that the use, change, or distribution of this product is forbidden, since it is still in a testing stage. The bibliographic search showed no evidence of the model’s re-use in later projects for the development of ontologies within the CnR domain.
3.10.Polygnosis thesaurus
In the context of the Politismos-Technologia, New Technologies in the Research, Study, Documentation and Access to the Information for Cultural Heritage Objects and Monuments (POLITEIA) project the Polygnosis educational knowledge web platform (Polygnosis platform) was developed [91]. Polygnosis platform can be used to improve access to information about the state-of-the-art of laser applications and methods on conservation, analysis and diagnosis of CH objects [93].
Polygnosis platform deploys an ontological model which is based on CIDOC CRM [25] and its compatible models, CRMsci [31] and CRMdig [29]. Furthermore, the platform deploys Polygnosis Thesaurus which is not an ontology per se, but has been built based on the ontological model of Polygnosis platform. Polygnosis Thesaurus aims to facilitate the collaboration of interdisciplinary working groups, in order to retrieve information about optical and laser-based techniques for advanced imaging, analysis and diagnosis of CH objects (movable and immovable CH), an aspect that falls within the CnR domain [91].
The development of the Polygnosis Thesaurus commenced with the collection and analysis of scientific sources, terminology and thesauri related to i) conservation and diagnosis of CH objects and ii) laser-based examination techniques [91]. Afterwards, the Polygnosis Thesaurus structure was specified developed in three steps: i) definition of semantic categories and facets of the thesaurus according to the main concepts of the Polygnosis platform model, ii) formulation of terms hierarchies and finally iii) definition of semantic relationships between the terms. Polygnosis Thesaurus was developed with TMS THeMaS.4848
The thesauri and vocabularies which were studied, include i) AAT [47], ii) Network of Research Computer Image SystemS in Europe (NARCISSE) [62], iii) Conservation & Restoration Institutions for Scientific Terminology dedicated to Art Learning Network (CRISTAL) [43] and iv) European Illustrated Glossary of Conservation terms for Wall-Paintings & Architectural Surfaces (EwaGlos) [115]. Polygnosis Thesaurus is structured in four main facets which are organized in hierarchies (IS-A relations). Apart from the organization of IS-A hierarchies, terms are interconnected through additional (non-hierarchical) binary relations. Furthermore, the four facets of Polygnosis have been mapped to CIDOC CRM classes (e.g., Material Objects facet maps to cidoc-crm: E70_Thing) [91]. The mapping was conducted to ensure a common understanding of terms and concepts by the different scientific communities of CH.
The main concepts of the Polygnosis platform model are: i) Method Application, which refers to the application techniques of investigation methods, ii) Example, which refers to measurement events, examination and diagnosis procedures, iii) Technical Examination, which refers to investigation methods, iv) Objects, which refers to the object under investigation and its features, v) Data, which refers to data derived from object investigation, vi) Glossary Terms, which refers to relevant terminology and vii) Publications, which refers to relevant bibliographic documentation. The Polygnosis Thesaurus is included in the Glossary Terms and is organized in four facets [91]: i) Material Objects, which represents things with physical substance that constitute complete units and have a relatively stable form with identifiable boundaries in at least one dimension, ii) Investigation Methods, which represents systematic procedures designed to detect, identify and demonstrate Identifiable Features of Material Objects, iii) Identifiable Features, which represents features that are inextricably linked with Material Objects on which they are found (e.g., construction features, deterioration phenomena etc.), and iv) Data, which represents digital informational material related to the documentation of Material Objects or information objects and other processes of information acquisition and/or production (by recording, by digitization, through study, during intervention etc.). Table 10 depicts the correspondence between the CnR aspects (see Section 2) and the main concepts of the Polygnosis platform model.
Table 10
Correspondence between the CnR aspects and the main concepts of the polygnosis platform model
CnR Aspect | Polygnosis platform model main concepts |
Administration | Objects, Glossary Terms |
Materials & technology | Objects |
Alteration | Objects |
Investigation | Method Application, Examples, Technical Examination, Data, Publications |
Intervention | – |
Polygnosis Thesaurus supports the educational role of the Polygnosis platform, by providing relevant terms that the user might not have thought of, thereby facilitating the exploration and disambiguation of information [91]. The Polygnosis platform stores and integrates data, collected from scientific examination methodologies and applications that have been conducted in the laboratory of Photonics for Cultural Heritage of Institute of Electronic Structure and Laser – Foundation of Research and Technology Hellas (IESL-FORTH). It captures accumulated knowledge and data regarding diagnostic tools and methodologies, and display cases (the use of tools and application of methodologies to CH objects). In practice, the user (conservator, heritage researcher or practitioner) can select the case that interests him/her either by examination type or evidence type and get descriptive texts regarding the examination type and evidence selected, as well as a list of related cases on different CH objects. Polygnosis platform and thesaurus has been evaluated by users4949 twice [91]: during the workshop ‘Laser SYNTHESIS and POLYGNOSIS; The POLITEIA Documentation System and Knowledge Platform’, and, two years later, during a workshop that was conducted in the Department of Conservation of Antiquities and Works of Arts in TEI of Athens. According to the findings, semantic presentation forms and design were considered a key issue for an effective learning platform, while the whole idea of a system which supports a common language in the field of interest was appreciated.
Both the Polygnosis platform model and thesaurus are not available online. The bibliographic search showed no evidence of the model’s re-use in later projects for the development of ontologies within the CnR domain.
3.11.Heritage resilience against climate events on site
The European project HERACLES (HEritage Resilience Against CLimate Events on Site) aimed to develop an ontology-based platform, which provides a knowledge base for the efficient storage and management of data related to the impact of climate change on immovable CH and the mitigation of potentially harmful effects [48].
An important output of this research was the HERACLES application ontology, a semantic model which covers the preservation of immovable CH. As such, the primary object of the HERACLES ontology is the efficient integration, exchange and retrieval of data related to climate change impact, which are often unstructured, incompatible or in some cases partial.
The HERACLES ontology was developed following a workshop-based approach, while the WebGenesis software was used for development [48]. During the workshop, the participants determined requirements for the conceptual representation according to the methodology of [44]. Next, competency questions (CQs) were formulated in order to specify the area of interest. The participants identified the main subdomains of the ontology. Next, classes and their relationships were defined, while descriptions of the classes were added to facilitate human comprehension of the data model. Other ontologies, related to the domain of interest have been studied and used as reference material for the ontology.
The sources used as reference material for the HERACLES ontology, include: i) SWEET ontologies [96], ii) the Materials Ontology [9], iii) Open Geospatial Consortium (OGC) standards including the Sensor Things Application Programming Interface (from now on ST-API) [65] and the Internet of Things Tasking Capability (from now on ITTC) [51]. HERACLES consists of 109 classes, 204 object properties (102 properties plus their inverse), 49 data properties and 141 individuals [48].
HERACLES core classes are [48,49]: i) Cultural Heritage, which represents monumental art and buildings, ii) Cultural Heritage Properties, which represents characteristics of monumental art and buildings, iii) Cultural Heritage Element, which represents components of monumental art and buildings, iv) Damage and Effect, which represents changes that occurred on a component of monumental art and buildings due to an event, as well as abiotic factors that induce climate events that in turn cause damages, v) Material, which represents materials used in CH management, vi) Action, which represents measurements for monitoring, analyzing or preserving monumental art and buildings, vii) Stakeholders, which represents actors in CH preservation, viii) Risk, which represents the risk of harm of monumental art and buildings and ix) Location, which represents spatial information (e.g., the position of a measurement sensor). Table 11 depicts the correspondence between the CnR aspects (see Section 2) and the core classes of HERACLES.
Table 11
Correspondence between the CnR aspects and the core classes of HERACLES
CnR Aspect | HERACLES core classes |
Administration | Cultural Heritage, Stakeholders, Location |
Materials & technology | Cultural Heritage Properties, Cultural Heritage Element, Material |
Alteration | Damage and Effect |
Investigation | Material, Action, Risk |
Intervention | Material, Action |
The HERACLES ontology serves as the backbone of the HERACLES knowledge base: every entry in the knowledge base is an instance of the ontology [49]. HERACLES knowledge base collects and integrates multisource information in order to effectively i) provide complete and up-to-date awareness about the conditions occurring in a CH site and ii) support retrieval and decision making for innovative measurements improving CH resilience. Particularly, HERACLES platform provides input forms, through which data are semantically integrated. The input form contains several text fields (e.g., for textual descriptions), while links to other instances can be created through selecting elements from lists. Additionally, an online endpoint is provided to facilitate instance creation/deletion. Regarding presentation of data, for each entry the system provides images and quick links to useful related information (e.g., damages, reports, sensor data). This endpoint is also used by the HERACLES mobile application, which allows reporting of damages on site, by delivering information such as location coordinates and description, as well as pictures, video footage etc. to the HERACLES knowledge base, in order to be presented to the back-end user.
Both the HERACLES platform and the HERACLES ontology have been evaluated in the context of four use-cases [49]. The use-cases included: i) Minoan Palace of Knossos in Heraklion, Crete, ii) Venetian Sea Fortress of Koules in Crete, iii) Consoli Palace in Gubbio, Italy and iv) the town walls in Gubbio, Italy. These test sites represent key case-studies for the impact of climate change on European CH assets. Using the HERACLES ontology made possible the semantic integration of collected data, while the platform effectively supported the retrieval of data required by experts in order to prepare reports for monuments condition state and environmental conditions [49].
The ontology is available online.5050 The bibliographic search showed no evidence of the model’s re-use in later projects for the development of ontologies within the CnR domain.
3.12.Heritage building core ontology
The COST action Innovation in Intelligent Management of Heritage Buildings5151 (COST-TD1406) aims to create a European network in order to promote synergies between different specialists and stakeholders of the Heritage Building (HB) domain, as well as to achieve a unified common understanding and operation within the domain.
In the context of COST-TD1406, an ontology for the Heritage Building (HB) domain, namely the HB Core Ontology (HBCO), has been developed [105,106]. The ontology aims to support HB experts to efficiently collect and use data, produced by the different specialists of the domain in the context of the various HB lifecycle activities (including analysis, documentation, preventive conservation, restoration, economic aspects of HB, use and management).
HBCO was developed using METHONTOLOGY methodology [41], while it was encoded in OWL, using Protégé [80].
The following terminologies and ontologies were reused for the development of HBCO: buildingSMART Data Dictionary (buildingSMART) [17], FOAF Vocabulary Specification (FOAF) [15], ISA Programme Location Core Vocabulary (Location) [40], ISA Programme Person Core Vocabulary (Person) [54], Time Ontology in OWL (Time) [28] and CIDOC CRM [25]. Additionally, HBCO introduces a number of extra classes and properties in order to represent HB related data [104]. The ontology consists of 87 classes, 43 object properties and 18 data properties [104].
The main subgroups of the HBCO concepts are [104]: i) Location, which refers to building location, ii) Time, which refers to the building’s history and related events, iii) Geometry, which refers to the building’s geometry, expressed using some coordinate reference system, v) Management, which refers to activities related to the management of the building, v) FOAF related concepts, which refer to actors related to the building and their relations and vi) CH related concepts, which refer to the description of the building in terms of materials, construction etc. and activities related to building preservation. Table 12 depicts the correspondence between the CnR aspects (see Section 2) and the main concepts’ subgroups of HBCO.
Table 12
Correspondence between the CnR aspects and the main concepts’ subgroups of HBCO
CnR Aspect | HBCO main concepts’ subgroups |
Administration | Location, Geometry, Time Management, FOAF related concepts, CH related concepts |
Materials & technology | CH related concepts |
Alteration | CH related concepts |
Investigation | Management, CH related concepts |
Intervention | Management, CH related concepts |
HBCO was populated with HB data about 12 building projects [105,106]. Furthermore, [104] theoretically describes a platform for storage and management of HB data.
The ontology is not available online. The bibliographic search showed no evidence of the model’s re-use in later projects for the development of ontologies within the CnR domain.
3.13.Conservation reasoning ontology
The work presented in [78] proposes the Conservation Reasoning ontology (CORE), a semantic model which was developed with the purpose of facilitating integration and querying of data related to conservation of byzantine icons.
The CORE ontology extends certain classes from CIDOC CRM [25], in order to facilitate CnR data modelling. The extension of CIDOC CRM was conducted in a bottom-up manner based on empirical analysis, scientific knowledge and existing CnR vocabularies [78]. Finally, inference rules were formulated in order to facilitate semantic querying [78].
For the development of CORE, a number of CIDOC CRM classes and relations were reused in combination with existing CnR vocabularies (namely AAT [47], CAMEO [72] and AIC wiki [116]). The ontology includes general concepts that refer broadly to CnR of artwork, as well as concepts specific to CnR of byzantine icons. The ontology was developed in OWL using Protégé [78,80].
The main classes of the CORE ontology are [78]: i) Actor, which refers to humans and groups involved in the CnR, ii) Condition State, which refers to the categorization of the condition of a conservation object based on visual examination, iii) Date, which refers to the dates of events (e.g., production year), iv) Dimension, which refers to the measurable sizes of an object or its environment, v) Equipment, which refers to investigation, intervention or production instruments and tools, vi) Event, which refers to processes or activities related to a conservation object or its environment, vii) Information Object, which refers to inscriptions, publications and documents related to conservation objects, viii) Man-Made Object, which refers to conservation objects, object components (e.g., structural layer) or production materials, ix) Physical Feature, which refers to features of a conservation object (e.g., a damage), x) Place, which refers to the environment of the conservation object (interior or exterior), xi) Timespan, which refers to the duration of an event. Table 13 depicts the correspondence between the CnR aspects (see Section 2) and the main classes of CORE.
Table 13
Correspondence between the CnR aspects and the main classes of CORE
CnR Aspect | CORE ontology main classes |
Administration | Actor, Date, Timespan, Dimension, Information Object, Place |
Materials & technology | Man-Made Object, Equipment |
Alteration | Condition State, Event, Physical Feature |
Investigation | Event, Equipment |
Intervention | Event, Equipment |
In order to be evaluated, CORE was populated with selected data from conservation reports, while CQs were formed as SPARQL and SPARQL DL queries. According to the evaluation results [78], modelling CnR data using CORE allowed for the formulation of queries such as “Which are the structural layers that have ever been recorded about an object?” and “Which are the structural layers of an object since the last conservation treatment?”. Such queries can assist the CnR professional during the collection of information about the history and condition of a conservation object.
The ontology is not available online. The model has been re-used for the representation of data related to preventive conservation and sensor data [76].
3.14.Chinese ancient buildings damages ontology
An ontology for the CnR of ancient buildings (CABD) is proposed in [113]. CABD was developed in order to facilitate querying and case-based reasoning of CH information related to ancient Chinese building damages and repairs.
For the development of CABD, information related to damages in ancient Chinese buildings was analyzed, focusing on the process of selecting the appropriate CnR method for damage repair. The mapping of specific repair methods to specific damage cases was implemented in the form of SWRL rules [113]. The ontology was developed in Protégé [80].
The main classes of CABD are [113]: i) Ancient building, which refers to the conservation object, ii) Damage body, which refers to the damaged part of the conservation object, iii) Damage object, which refers to the damage, iv) Damage feature, which refers to quantitative characteristics of the damage (e.g., width), v) Repair craft, which refers to the CnR intervention. Table 14 depicts the correspondence between the CnR aspects (see Section 2) and the main classes of CABD.
Table 14
Correspondence between the CnR aspects and the main classes of CABD
CnR Aspect | CABD ontology main classes |
Administration | – |
Materials & technology | Ancient building, Damage body |
Alteration | Damage object, Damage feature |
Investigation | – |
Intervention | Repair craft |
To be tested, the proposed model was populated with data describing cracks on the surfaces of buildings of WuDian type, together with the appropriate repair method. Based on these data, the ontology was used for retrieving cases of damages and corresponding repair methods that present similarities with a given case (target case), by formulating SPARQL queries [113].
The ontology is not available online. The bibliographic search showed no evidence of the model’s re-use in later projects for the development of ontologies within the CnR domain.
3.15.Network of modular ontologies for heritage buildings
A framework for a Linked Data-based Heritage BIM is proposed in [13], in order to improve the modelling of structured building data and its exchange over the Web. In this context of the framework a network of modular ontologies (Network of Modular Ontologies for Heritage Buildings – NMOHB) is proposed for obtaining rich and interoperable heritage building descriptions. The development of NMOHB was based on the benchmark requirements for the Linked Data-based Heritage BIM.
NMOHB adopts a layered architecture that incorporates: i) core ontologies, which provide the basic concepts related to construction, ii) specialized taxonomies which extend the core ontologies, and iii) metadata ontologies, which are used for data exchange.
In particular, a number of complementary ontologies were developed in the context of the research (namely Construction Tasks Ontology (CTO), ConTax Ontology (ConTax), Construction Dataset Context Ontology (CDC), Construction Properties (CP), Built Heritage Properties (BHP), Ontology for Geometry Formats (FOG), Geometry Metadata Ontology (GOM), MDCS Damage Atlas Ontology (MDCS-O), MWV Damage Ontology (MWV-D), MWV Tasks (MWV-T) and AAT-Buildings), while the Building Topology Ontology (BOT) [98] and Data Catalogue Vocabulary (DCAT) [5] ontologies were reused. In addition, the following ontologies were developed in collaboration with other projects and were included in NMOHB: Ontology for Managing Geometry (OMG) [112], Ontology for Property Management (OPM) [97] and Damage Topology Ontology (DOT) [46]. The core layer of NMOHB is formed by the BOT, DOT, CTO, OMG and OPM ontologies.
The concepts of the core ontologies of the NMOHB are organized in the following groups [13]: i) Building topology, which refers to the building, its structure and materials, ii) Construction damages, which refers to the observable damages or damaged areas of the building, as well as the inspection of those damages, iii) Geometry descriptions, which refers to 2D and 3D representations of a building and their metadata, iv) Construction tasks, which refers to CnR interventions. Table 15 depicts the correspondence between the CnR aspects (see Section 2) and the groups of concepts of the core ontologies of NMOHB.
Table 15
Correspondence between the CnR aspects and the main concepts’ groups of NMOHB
CnR Aspect | NMOHB main concepts’ groups |
Administration | Buildings |
Materials & technology | Buildings |
Alteration | Construction damages |
Investigation | Construction damages, Geometry descriptions |
Intervention | Construction tasks |
To be tested, the developed ontology network was deployed in five example cases, inspired by two built heritage projects in Ghent [13]. The example cases demonstrate the key functionalities of NMOHB and underlying technologies, which are: i) the possibility of linking and using geometry from a variety of common geometry formats, ii) the use of flexible and integrated classification systems, iii) the provision of combined views on previously disparate datasets, iv) the provision of feedback mechanisms for construction damages and tasks based on structured data and v) the provision of metadata about construction datasets to manage the flow of internal and exchanged datasets. As discussed in [13] the outcome of the evaluation phase indicated that most requirements related to the data structure and management workflows (e.g., data exchange) have been addressed successfully by the proposed modular ontology network. However, it is highlighted that more contributions of domain experts are considered necessary to maintain, extend and verify the content of the NMOHB taxonomies. Especially the property taxonomies, damage and task classification are currently not extensive enough for use in real projects.
All the different ontologies of the network (BOT,5252 DCAT,5353 OMG,5454 OPM,5555 DOT,5656 CTO,5757 ConTax,5858 CDC,5959 FOG,6060 GOM,6161 MDCS-O,6262 MWV-D,6363 MWV-T,6464 AAT-Buildings6565) used in the scheme are available online. The bibliographic search showed no evidence of the model’s re-use in later projects for the development of ontologies within the CnR domain.
3.16.CRMBnF
The Data mining and ALGOrithms for predicting the condition of COLlections (DALGOCOL) research project6666 is a collaboration between the French National Library (BnF) and the Heritage Sciences Foundation. The project aims to develop a decision support system for conservation experts for analyzing the conservation history of documents in order to enable reliable predictions about their physical state.
In this context, [121] proposes the Conceptual Reference Model of BnF (CRMBnF). The proposed model aims to i) represent the conservation history of a document as a sequence of events (conservation trajectories) providing a unified vocabulary for the events and ii) be used for the pairwise comparison of events in two conservation trajectories overcoming terminological heterogeneity of events.
CRMBnF was developed in close collaboration with domain experts at the BnF and is encoded in RDF/S 2 and OWL. For developing the ontology three external ontologies were re-used [121]: CIDOC CRM [25], CRMsci [31] and CRMcr [10].
CRMBnF includes two main classes [121]: i) Conservation Process, which represent CnR interventions, ii) Degradation, which represent deterioration events that occur on a conservation object. Table 16 depicts the correspondence between the CnR aspects (see Section 2) and the main classes of CRMBnF.
Table 16
Correspondence between the CnR aspects and the main classes of CRMBnF
CnR Aspect | CRMBnF main classes |
Administration | – |
Materials & technology | – |
Alteration | Degradation |
Investigation | – |
Intervention | Conservation Process |
CRMBnF has been used for the comparison of the events between conservation trajectories, a process which serves as a base to build an adequate predictive model for the decision support system [121]. The comparison is performed by computing a similarity score using the ontology, considering the relative position of the concepts corresponding to the names of the events in the ontology.
Three experiments were conducted, using real conservation and communication datasets from the BnF. The first two experiments aimed to assess the effectiveness of using the ontology for finding matching events and for evaluating the similarity of trajectories. The third experiment aimed to show the effectiveness of using the ontology and the LCESS measure by comparing the computed similarity to a gold standard [121]. According to the results, the proposed ontological approach improves the precision of the matching process [121].
The ontology is not available online. The bibliographic search showed no evidence of the model’s re-use in later projects for the development of ontologies within the CnR domain.
4.Discussion and conclusions
The study of the different representations of CnR knowledge and their deployment in systems and services that exploit SW technologies and methods revealed some interesting points of convergence or divergence, which are discussed in the following sections. The study findings are overviewed in Table 17 and are organized and discussed according to three axes: i) content, ii) re-use of existing models, and iii) deployment.
Table 17
Overview of the reviewed works according to i) content, ii) re-use of existing models and iii) deployment
Model’s name | Content | Re-use of existing models | Deployment | ||||
Object type | CnR aspectsa | Ontologies | Metadata standards | Terms lists/Thesauri | Related system | Servicesb | |
OPPRA | Movable CH | ADM, MAT, ALT, INV, INT | CIDOC CRM, OreChem | OAI-ORE | AAT, AICCM, INCCA, RUG, CAMEO, NIST | 20thCPaint Platform | INTEG, SEA, ANN |
MDO | Immovable CH | MAT, ALT, INV, INT | – | – | IDGS, TBCPBEE, MCCS, RTS, ICOMOS, OSM | MONDIS | INTEG, SEA, VIS |
COSCHKR | Movable & Immovable CH | MAT, ALT, INV | – | – | – | COSCHKR Platform | REC |
DOC-CULTURE | Movable CH | ADM, MAT, ALT, INV, INT | CIDOC CRM | DC, MODS, RDS, PREMIS, Muse Meta | – | DOC-CULTURE Information System | VIS, ANN |
ODPA-3DR | Immovable CH | ADM, MAT, ALT, INV | CIDOC CRM, CRMsci, CRMdig, CRMinf | – | ICOMOS, ARC | Reality-based 3D semantic annotation of masonry structures’ conservation state | SEA, VIS, ANN |
PARCOURS semantic model | Movable & Immovable CH | ADM, MAT, ALT, INV, INT | CIDOC CRM, CRMsci | – | – | PARCOURS System | INTEG, SEA |
CHAP | Movable CH | ADM, MAT, INV | CIDOC CRM, CRMdig, CSO | – | – | GRAVITATE Platform | SEA, VIS, ANN, FEA |
CPM | Immovable CH | ADM, MAT, ALT, INV, INT | CIDOC CRM | – | ICOMOS | Ontology-based BIM Semantic Bridge | INTEG, VIS, ANN |
BCHO | Immovable CH | ADM, MAT, ALT, INV, INT | Geneva CityGML, MDO Erlagen CRM/OWL | – | – | – | – |
Polygnosis Thesaurus | Movable & Immovable CH | ADM, MAT, ALT, INV | CIDOC CRM | – | AAT, NARCISSE, CRISTAL, EwaGlos | Polygnosis Platform | INTEG, SEA |
HERACLES | Immovable CH | ADM, MAT, ALT, INV, INT | SWEET, Materials Ontology | OGC, ST-API, ITTC | – | HERACLES Platform | INTEG, SEA, VIS |
HBCO | Immovable CH | ADM, MAT, ALT, INV, INT | FOAF, Location, Person, Time, CIDOC CRM | buildingSMART | – | – | – |
CORE | Movable CH | ADM, MAT, ALT, INV, INT | CIDOC CRM | – | AAT, CAMEO, AIC | – | – |
Table 17
(Continued)
Model’s name | Content | Re-use of existing models | Deployment | ||||
Object type | CnR aspectsa | Ontologies | Metadata standards | Terms lists/Thesauri | Related system | Servicesb | |
CABD | Immovable CH | MAT, ALT, INT | – | – | – | – | – |
NMOHB | Immovable CH | ADM, MAT, ALT, INV, INT | BOT, DCAT, DOT, OMG, OPM | – | AAT | Linked Data Heritage BIM | INTEG, SEA |
CRMBnF | Movable CH | ALT, INT | CIDOC CRM, CRMsci, CRMcr | – | – | – | – |
a ADM: administration, MAT: materials & technology, ALT: alteration, INV: investigation, INT: intervention.
b INTEG: data integration, SEA: semantic search, VIS: visualization, ANN: semantic annotation, FEA: feature recognition, REC: recommendation of digitization and analysis methods.
4.1.Content
Obviously, a common requirement is the modelling of the conservation object per se. The majority of the reviewed models specialize in a specific type of conservation objects: in particular, 8 out of the 16 reviewed models specialize in immovable CH, while 5 of them specialize in movable CH (with only 3 models covering tangible CH in general). Those numbers suggest a significant interest in SW technologies exploitation in the CnR of the immovable CH community.
Figure 1 depicts the degree to which the CnR aspects defined in Section 2 are covered by the reviewed models. The materials & technology aspect is covered by the great majority (15 out of 16) of the reviewed models, which is expected since the conservation object in itself, its structure, materials and features are in the center of the initial stage of the CnR process since they are used for establishing the identity and condition of the conservation project (see Section 1). The alteration and investigation aspects are also covered by 15 and 14 out of 16 models respectively, which is similarly expected since the main objective of all the reviewed works is the organization and management of CnR data, and the investigation stage constitutes the primary means of collecting such data. The expert conducts investigation in the first stage of the CnR process but also in later stages for evaluating risk and for identifying the appropriate solutions. The main objective of the investigation is to enable the expert in identifying and recording the attributes of a conservation object, both material (e.g., structural layers) and non- material (e.g., historic value), as well as to deduce current and potential preservation issues that may occur due to inaction or action (e.g., deterioration due to environmental conditions, deterioration due to an intervention). The coverage of the aforementioned aspects by the great majority of the models indicates their importance and necessity, while it constitutes a solid base for data modelling endeavors. On the other hand, while the intervention aspect is of apparent interest for the CnR domain and it also constitutes an important source for acquiring CnR information, it is covered only by 12 models, which is less than expected, especially compared to the high coverage of the investigation aspect.
Fig. 1.
Coverage of CnR aspects.
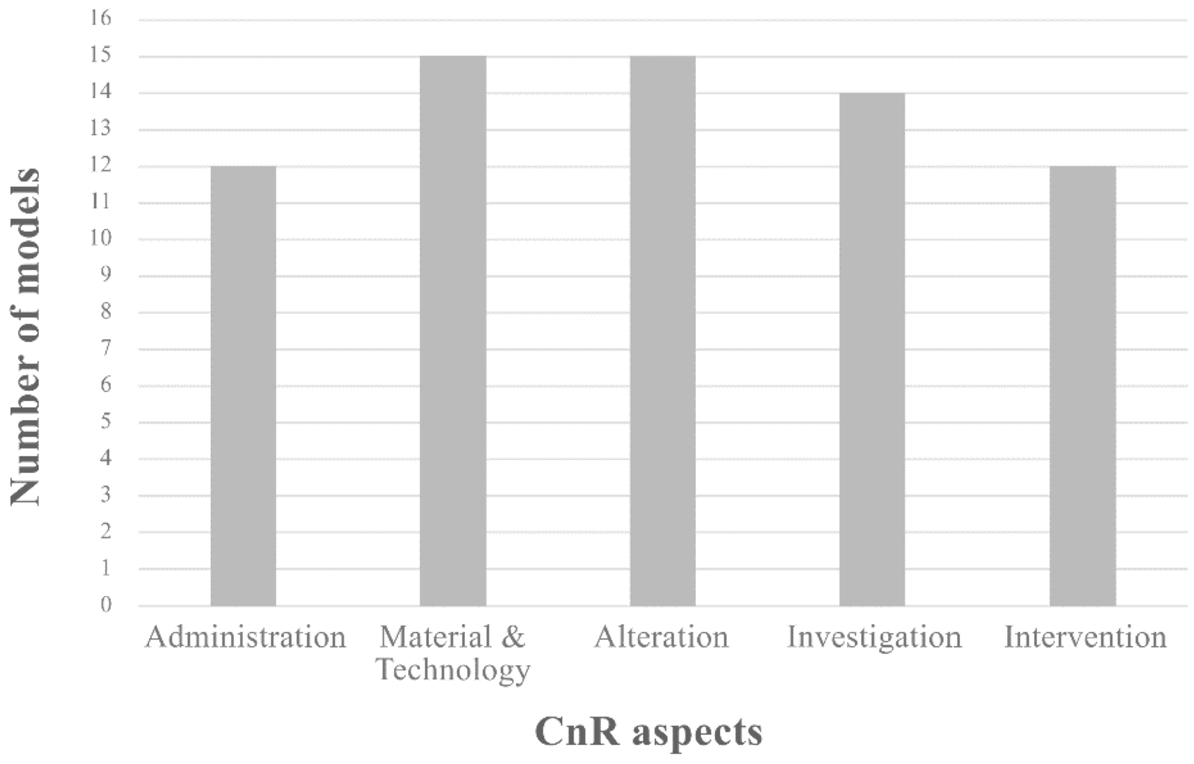
An interesting observation regarding the content is that different models allow different granularities for categorization of the various concepts. For example, the CPM model represents a building (i.e., the conservation object) using classes of specific building types (EcpmA36_Oratory), while the COSCHKR model represents a building at a more abstract level, using the class coschkr:CompositeObject. In another example, MDO represents the concept of material using a number of specialized classes (mdo:CementMortar, mdo:SodaGlass, etc.) whereas HERACLES provides only the general class heracles:Material for the same purpose. Different granularities of representation may be handy in different use cases (e.g., we may not be certain about the use of a building in order to categorize it as Oratory) or different reasoning requirements.
4.2.Re-use of existing models
While the scope and context of the reviewed works may differ, there is a common interest for providing interoperability of CnR data. Towards that direction, most of the reviewed models were developed either from scratch and were then mapped/aligned to existing ontologies, or they were built entirely by extending existing ontologies (with the exception of MDO, COSCHKR, and CABD).
The CIDOC CRM ontology, as well as its compatible models, were largely adopted by the majority of the projects. Additionally, specialized ontologies from other knowledge domains relevant to CnR were adopted for the development of the models. Figure 2 depicts the origin domain of the various ontologies, metadata standards, term lists and thesauri that are re-used by the reviewed models.
Most of the works took into account and adopted ontologies from other fields such as the field of Architecture & Construction (BOT was adopted by NMOHB), the field of Chemistry and Material Science (OreChem was adopted by OPPRA, MO was adopted by HERACLES), the field of Earth Science (SWEET ontologies were adopted by HERACLES) and the field of Digital Media (CSO was adopted by CHAP). Furthermore, thesauri, glossaries and controlled vocabularies, specialized either in CnR or in other related domains, were similarly employed.
Fig. 2.
The origin domain of the ontologies, metadata standards, terms lists and thesauri that are reused by the reviewed models.
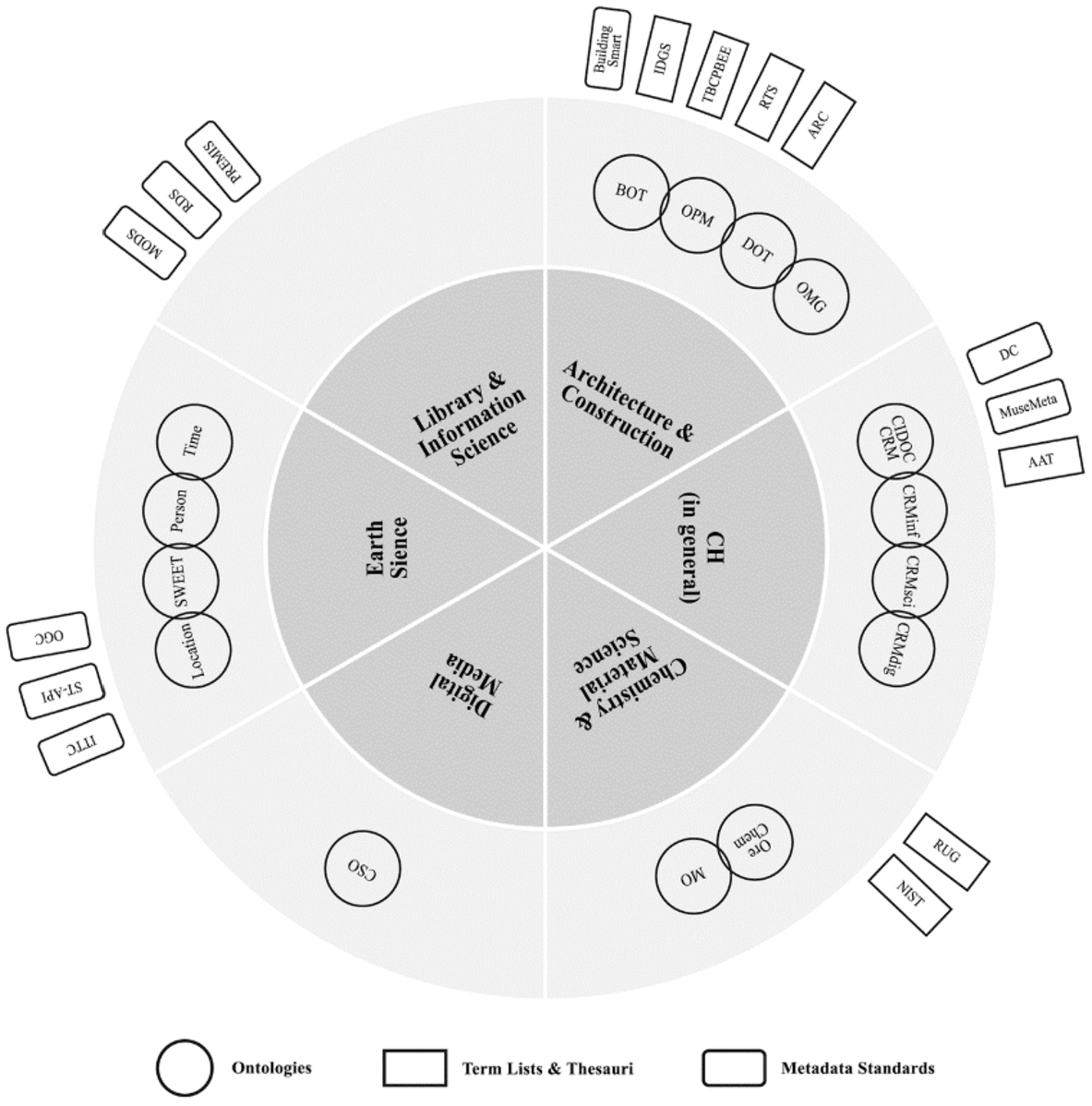
4.3.Deployment
All the models included in the current survey have been employed for developing SW systems that offer various domain-specific services. In the course of the survey, we identified a number of services that are common among those systems. In particular:
– semantic search, which refers to retrieval of CnR data based on the meaning of the search query,
– data integration of conservation-related data, derived from remote and possibly heterogeneous sources, into a unified form,
– visualization of CnR data (e.g., 2D or 3D visualization of alterations, mind maps),
– semantic annotation of i) text with conservation-related content (e.g., scientific papers) or ii) 2D/3D models of conservation objects with semantically structured machine-processable data,
– feature recognition (e.g., decorative element, structural element) of conservation objects,
– recommendation of digitization and analysis methods based on i) object characteristics, ii) CnR expert’s requirements.
Figure 3 depicts the degree to which the identified services are provided by the systems built upon the reviewed models. It should be mentioned that 5 out of 16 reviewed models (namely BHO, HBCO, CORE, CABD and CRMBnF) have not been deployed in some system, though they have been used for data modelling, testing SPARQL queries and comparison of annotated data. It should be mentioned that CRMBnF is supposed to be used in a decision-support system, for conservation objects classification based on their history.
Apparently, semantic search is the most popular among provided services, while data integration, visualization and semantic annotation follow. The popularity of these services is expected considering that they intend to support the CnR process (see Section 1) and by extension CnR decision-making.
Decision-making plays a central role in the day-to-day work of the CnR professional [50,64]. In broad terms, decision making in CnR can be thought of as equivalent to the conservation process itself [64]. Conservators participate in decision-making processes by i) contributing their expertise to a broader, multi-disciplinary group of CH experts, and ii) making their own CnR decisions [50]. In this context, data integration and semantic search provide unified access to the required knowledge/information (in order to make an effective intervention recommendation), in this way reducing information retrieval time and improving quality of search results (e.g., information completeness), consequently supporting the different stages of the CnR process, from the problem identification to the potential risks and the selection of an efficient solution. Similarly, visualization contributes to CnR decision-making, at different stages of the CnR process, by providing a more articulate and meaningful documentation as well as correlation of the requested information (e.g., the visualization of extent and severity of an alteration phenomenon gives a thorough view of the conservation object’s condition). Finally, semantic annotation, either text-based or visual (2D/3D), is used alongside semantic search and visualization contributing to the production of context which can be retrieved and displayed.
Fig. 3.
Provision of SW services by systems built upon the reviewed models.
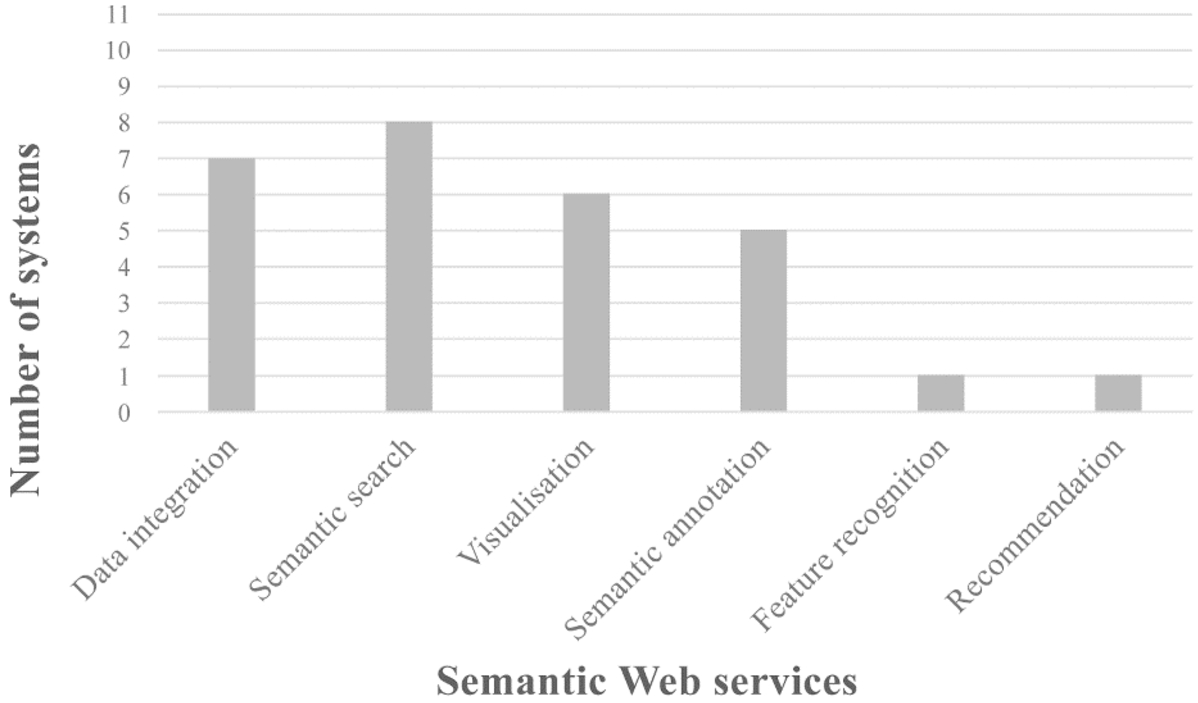
On the other hand, actual recommendation of digitization and analysis methods (as an explicit service), is proposed by only one system (the COSCHKR platform). And even in that case, the recommendation merely covers a certain step of the CnR decision-making process, that is selecting the appropriate digitization and analysis method for identifying the technology and condition of a conservation object in order to determine intervention requirements.
4.4.Conclusions and further research: Towards an ontology of CnR decision-making
As discussed in the previous section, decision-making constitutes the backbone of the CnR task. Through decision-making the expert transforms various chunks of possibly diverse information relevant to a conservation object, such as scientific information (e.g., material ageing), administrative (e.g., loaning preconditions) or even cultural information (e.g., historical value), into concrete and specific intervention decisions. The current survey showed that all the reviewed models more or less represent knowledge relevant to intervention decision-making, and that they have actually been employed for implementing SW services that support intervention decision-making; although merely in an assistive way (for instance, by offering semantic retrieval of data related to a conservation diagnosis). In other words, the decision-making process per se, i.e., including all the parameters, criteria, intervention options etc. potentially involved, and more importantly their complex interdependence, which often generates restrictions that can dramatically affect the decision-making outcome, has not been modelled as of yet. For example, the MDO, OPPRA, DOC-CULTURE, PARCOURS semantic model, CPM, BCHO, HERACLES, HBCO, CORE, CABD, CRMBnF models, as well as some of the models of NMOHB provide the relations that are necessary to correlate an intervention with i) a conservation object and ii) one or more alteration phenomena. However, as [42] highlights, a simple correlation between conservation interventions and alteration phenomena does not adequately represent the potential complexity of interplay between the various parameters that need to be taken into account in order to come to a valid intervention recommendation.
Drawing on the above, it is strongly suggested that further research should be conducted in order to analyze and conceptualize intervention decision-making at a granularity that will allow a more thorough representation, suitable to drive the implementation of services that will deliver intervention recommendations, as an explicit decision-support service. For example, a semantic model of intervention decision-making knowledge by means of a formal ontology can serve as the basis for the development of decision-support systems, with the objective of recommending specific, case-based intervention options. Eventually, such systems will actively assist the CnR expert i) to better organize their thoughts and determine requirements over a decision-making task, and most importantly ii) to retrieve and assess valid intervention options more quickly and effectively. Furthermore, a formal ontology will enable experts to share decision-making knowledge and exchange data with the wider community in a unified way [12]. Dissemination and exchange of know-how about intervention decision- making among CnR experts is bound to benefit them in multiple ways, for example, in training new professionals or effectively communicating information with clients, stakeholders or other (often interdisciplinary) professional groups [73,74], thus, elevating their work to the next level.
Notes
1 Conservation object refers to “the object which is worthy of conservation, and not only repair, maintenance, cleaning, or care” [79].
3 Documentation refers to the information collected, created and maintained for the purpose of present and future CnR of the conservation objects and for reference [6,39].
4 As [115] mentions the term fresco may refer to both i) wall paintings in general and ii) wall paintings produced by painting on fresh lime plaster, in different European countries.
16 Though the project is still ongoing, a significant amount of ICCD data has been published and is available via a SPARQL endpoint (see https://dati.beniculturali.it/sparql).
18 In cases where the published documentation of a model does not reveal details regarding a certain study axis, the corresponding sub-section is omitted.
19 Collaborative project between the Asia Pacific Twentieth Century Conservation Art Research Network (APTCCARN) and the eResearch Lab at the University of Queensland.
20 The website of the project is no longer available: http://www.20thcpaint.org/index.jsp.
21 Other preservation experts are curators, materials scientists, chemists, characterization experts and information scientists.
27 CS is defined as the “interdisciplinary study of the maintenance, care, and protection of art, architecture, and other cultural works” [7].
28 No longer accessible url: http://www.20thcpaint.org/oppra.owl.
33 COSCH is the COST-Action TD 1201.
34 Preservation is defined as “the protection of cultural property through activities that minimize chemical and physical deterioration and damage and that prevent loss of informational content” [3].
37 Reality-based 3D is the technique used to create a three-dimensional representation of a real object [69].
39 The website of the project is no longer available: http://www.gravitate-project.eu/.
41 SKOS uses RDF in order to provide a standard way to represent knowledge organization systems.
44 BIM is an environment that allows the creation of virtual building models, which can be linked to numerical data, texts, images, and other types of information. It is used in the fields of Architecture, Engineering and Construction (for further information see [92]).
49 In the first workshop the users were young researchers, scholars, graduate students, and professionals, while in the second the users were conservation students, graduates and professionals.
Acknowledgements
The research work was supported by the Hellenic Foundation for Research and Innovation (HFRI) under the HFRI PhD Fellowship grants (Fellowship Number: 115).
References
[1] | M. Acierno, S. Cursi, D. Simeone and D. Fiorani, Architectural heritage knowledge modelling: An ontology-based framework for conservation process, Journal of Cultural Heritage 24: ((2017) ), 124–133. doi:10.1016/j.culher.2016.09.010. |
[2] | M. Acierno and D. Fiorani, Innovative tools for managing historical buildings: The use of geographic information system and ontologies for historical centers, in: ISPRS – International Archives of the Photogrammetry, Remote Sensing and Spatial Information Sciences, XLII-2/W11, (2019) , pp. 21–27. doi:10.5194/isprs-archives-XLII-2-W11-21-2019. |
[3] | AIC, Conservation Terminology, (2022). https://www.culturalheritage.org/about-conservation/what-is-conservation/definitions (accessed June 2, 2022). |
[4] | AICCM, Visual Glossary, (2022). https://aiccm.org.au/conservation/visual-glossary/ (accessed June 2, 2022). |
[5] | R. Albertoni, D. Browning, S. Cox, A. Gonzalez Beltran, A. Perego and P. Winstanley, Data Catalog Vocabulary (DCAT) – Version 2, 2020, https://www.w3.org/TR/vocab-dcat-2/, (accessed June 2, 2022). |
[6] | B. Appelbaum, Conservation Treatment Methodology, Routledge, London, (2007) . |
[7] | Art & Architecture Thesaurus Online Full Record Display, Conservation Science (Cultural Heritage Discipline), (2022). https://www.getty.edu/vow/AATFullDisplay?find=conservation+science&logic=AND¬e=&english=N&prev_page=1&subjectid=300379510 (accessed June 2, 2022). |
[8] | Art & Architecture Thesaurus Online Full Record Display, Movable Works, (2022). https://www.getty.edu/vow/AATFullDisplay?find=movable+works&logic=AND¬e=&english=N&prev_page=1&subjectid=300310093 (accessed June 2, 2022). |
[9] | T. Ashino, Materials ontology: An infrastructure for exchanging materials information and knowledge, Data Science Journal 9: ((2010) ), 54–61. doi:10.2481/dsj.008-041. |
[10] | I. Bannour, C. Marinica, L. Bouiller, R. Pillay, C. Darrieumerlou, O. Malavergne, D. Kotzinos and C. Niang, CRMCR – a CIDOC-CRM extension for supporting semantic interoperability in the conservation and restoration domain, in: 2018 3rd Digital Heritage International Congress (DigitalHERITAGE) Held Jointly with 2018 24th International Conference on Virtual Systems & Multimedia (VSMM 2018), IEEE, (2018) , pp. 1–8. doi:10.1109/DigitalHeritage.2018.8810098. |
[11] | M. Blaško, R. Cacciotti, P. Křemen and Z. Kouba, Monument damage ontology, in: Progress in Cultural Heritage Preservation, EuroMed 2012, M. Ioannides, D. Fritsch, J. Leissner, R. Davies, F. Remondino and R. Caffo, eds, Lecture Notes in Computer Science, Vol. 7616: , Springer, Berlin, Heidelberg, (2012) , pp. 221–230. doi:10.1007/978-3-642-34234-9_22. |
[12] | E. Blomqvist, The use of semantic web technologies for decision support – a survey, Semantic Web Journal 5: ((2014) ), 177–201. doi:10.3233/SW-2012-0084. |
[13] | M. Bonduel, A Framework for a Linked Data-based Heritage BIM, Doctoral thesis, KU Leuven – Faculty of Engineering Technology, 2021. |
[14] | F. Boochs, A. Trémeau, Ó. Murphy, M. Gerke, J.L. Lerma, A. Karmacharya and M. Karaszewski, Towards a knowledge model bridging technologies and applications in cultural heritage documentation, ISPRS Annals of the Photogrammetry, Remote Sensing and Spatial Information Sciences II-5: ((2014) ), 81–88, (accessed June 2, 2022). doi:10.5194/isprsannals-II-5-81-2014. |
[15] | D. Brickley and L. Miller, FOAF Vocabulary Specification 0.99, (2014) , http://xmlns.com/foaf/spec/#:~:text=The%20FOAF%20speci-fication%20is%20produced,known%20informally%20as%20’FOAF’. |
[16] | G. Bruseker, N. Carboni and A. Guillem, Cultural heritage data management: The role of formal ontology and CIDOC CRM, in: Heritage and Archaeology in the Digital Age. Quantitative Methods in the Humanities and Social Sciences, M. Vincent, V. López-Menchero Bendicho, M. Ioannides and T. Levy, eds, Springer, Cham, (2017) , pp. 93–131. doi:10.1007/978-3-319-65370-9_6. |
[17] | buildingSmart, buildingSmart Data Dictionary (2022). https://www.buildingsmart.org/users/services/buildingsmart-data-dictionary/ (accessed June 2, 2022). |
[18] | R. Cacciotti, Integrated knowledge-based tools for documenting and monitoring damages to built heritage, in: ISPRS – International Archives of the Photogrammetry, Remote Sensing and Spatial Information Sciences, Vols XL-5/W7: , (2015) , pp. 57–63. doi:10.5194/isprsarchives-XL-5-W7-57-2015. |
[19] | R. Cacciotti, M. Blasko and J. Valach, A diagnostic ontological model for damages to historical constructions, Journal of Cultural Heritage. 16: (1) ((2014) ), 40–48. doi:10.1016/j.culher.2014.02.002. |
[20] | R. Cacciotti, J. Valach, P. Kuneš, M. Cernanský, M. Blasko and P. Kremen, Monument damage information system (MONDIS): An ontological approach to cultural heritage documentation, ISPRS Annals of the Photogrammetry, Remote Sensing and Spatial Information Sciences II-5: ((2013) ), 55–60. doi:10.5194/isprsannals-II-5-W1-55-2013. |
[21] | V.A. Carriero, A. Gangemi, M.L. Mancinelli, L. Marinucci, A.G. Nuzzolese, V. Presutti and C. Veninata, ArCo: The Italian cultural heritage knowledge graph, in: The Semantic Web – ISWC 2019, C. Ghidini, O. Hartig, M. Maleshkova, V. Svátek, I. Cruz, A. Hogan and J. Song, eds, Lecture Notes in Computer Science, Vol. 11779: , Springer, Cham, (2019) , pp. 36–52. doi:10.1007/978-3-030-30796-7_3. |
[22] | V.A. Carriero, A. Gangemi, M.L. Mancinelli, A.G. Nuzzolese, V. Presutti and C. Veninata, Pattern-based design applied to cultural heritage knowledge graphs, Semantic Web Journal 12: (2) ((2021) ), 313–357. doi:10.3233/SW-200422. |
[23] | C.E. Catalano, V. Vassallo, S. Hermon and M. Spagnuolo, A cultural heritage partonomy for the documentation of 3D digital artefacts of cypriot coroplastic art, in: 6th Annual CIDOC – ICOM Conference in 2018 (CIDOC 2018), (2018) . doi:10.5281/zenodo.2536814. |
[24] | V. Charles, A. Isaac, V. Tzouvaras and S. Hennicke, Mapping cross-domain metadata to the Europeana data model (EDM), in: Research and Advanced Technology for Digital Libraries, TPDL 2013, T. Aalberg, C. Papatheodorou, M. Dobreva, G. Tsakonas and C.J. Farrugia, eds, Lecture Notes in Computer Science, Vol. 8092: , Springer, Berlin, Heidelberg, (2013) , pp. 484–485. doi:10.1007/978-3-642-40501-3_68. |
[25] | CIDOC CRM, CIDOC CRM (2022) http://www.cidoc-crm.org/ (accessed June 2, 2022). |
[26] | CIDOC CRM, Compatible models & Collaborations (2022) http://www.cidoc-crm.org/collaborations (accessed June 2, 2022). |
[27] | CIDOC CRM, Erlangen CRM/OWL (2022) http://www.cidoc-crm.org/erlangen-crm-owl (accessed June 2, 2022). |
[28] | S. Cox and C. Little, Time Ontology in OWL, (2022) , https://www.w3.org/TR/owl-time/, (accessed June 2, 2022). |
[29] | CRMdig, CRMdig (2022) http://www.cidoc-crm.org/crmdig/ (accessed June 2, 2022). |
[30] | CRMinf, CRMinf (2022) http://www.cidoc-crm.org/crminf/ (accessed June 2, 2022). |
[31] | CRMsci, CRMsci (2022) http://www.cidoc-crm.org/crmsci/ (accessed June 2, 2022). |
[32] | R. Cyganiak, D. Wood and M. Lanthaler, RDF 1.1 Concepts and Abstract Syntax, (2014) , https://www.w3.org/TR/rdf11-concepts/#section-Graph-Literal, (accessed June 2, 2022). |
[33] | F. Di Stefano, A. Gorreja, E. Malinverni and C. Mariotti, Knowledge modeling for heritage conservation process: from survey to HBIM implementation, ISPRS – International Archives of the Photogrammetry, Remote Sensing and Spatial Information Sciences, XLIV–4/W1-2020, 2020: pp. 19–26. https://doi.org/10.5194/isprs-archives-XLIV-4-W1-2020-19-2020. |
[34] | M. Doerr, Ontologies for cultural heritage, in: Handbook on Ontologies, International Handbooks on Information Systems, S. Staab and R. Studer, eds, Springer, Berlin, (2009) , pp. 463–486. doi:10.1007/978-3-540-92673-3_21. |
[35] | M. Doerr, C. Bekiari and P. Le Boeuf, FRBRoo, a conceptual model for performing arts, in: 2008 Annual Conference of CIDOC, Athens, (2008) . |
[36] | M. Doerr, J. Hunter and C. Lagoze, Towards a core ontology for information integration, Journal of Digital Information 4: ((2003) ), 1. |
[37] | M. Drdácký, J. Valach, P. Křemen and J. Abrahamčík, Cultural Heritage Protection Against Flooding, In: Damage Database, Institute of Theoretical and Applied Mechanics (2011), 185–195. |
[38] | Dublin Core Metadata Initiative, Dublin Core Metadata Element Set, Version 1.1: Reference Description (2012) http://dublincore.org/documents/dces/ (accessed June 2, 2022). |
[39] | EN 16853:2017 Irish Standard, Conservation of Cultural Heritage – Conservation Process – Decision making, planning and implementation, NSAI Standards (2017). |
[40] | EU ISA Programme Core Vocabularies Working Group (Location Task Force), ISA Programme Location Core Vocabulary (2015) https://www.w3.org/ns/locn (accessed June 2, 2022). |
[41] | M. Fernandez-Lopez, A. Gomez-Perez and N. Juristo, METHONTOLOGY: From ontological art towards ontological engineering, in: Engineering Workshop on Ontological Engineering, AAAI 1997, Spring Symposium Series, Vols SS-97-06: , (1997) , pp. 33–40. |
[42] | D. Fiorani and M. Acierno, Conservation process model (CPM): A twofold scientific research scope in the information modelling for cultural heritage, in: ISPRS – the International Archives of the Photogrammetry, Remote Sensing and Spatial Information Sciences, Vols XLII-5/W1: , (2017) , pp. 283–290. doi:10.5194/isprs-archives-XLII-5-W1-283-2017. |
[43] | FORTH Institute of Computer Science, CRISTAL Project (2022) https://www.ics.forth.gr/isl/project/2020 (accessed June 2, 2022). |
[44] | M. Grüninger and M.S. Fox, Methodology for the design and evaluation of ontologies, in: Workshop on Basic Ontological Issues in Knowledge Sharing, IJCAI-95, Montreal, (1995) . |
[45] | A. Guillem, G. Bruseker and P. Ronzino, Process, concept or thing? Some initial considerations in the ontological modelling of architecture, International Journal on Digital Libraries 18: ((2017) ), 289–299. doi:10.1007/s00799-016-0188-0. |
[46] | A.H. Hamdan and M. Bonduel, Damage Topology Ontology, (2017) , https://alhakam.github.io/dot/, (accessed June 2, 2022). |
[47] | P. Harping, in: Introduction to Controlled Vocabularies, Getty Publications, Los Angeles, (2010) . |
[48] | T. Hellmund, P. Hertweck, D. Hilbring, J. Mossgraber, G. Alexandrakis, P. Pouli, A. Siatou and G. Padeletti, Introducing the HERACLES ontology semantics for cultural heritage management, Heritage 1: (2) ((2018) ), 377–391. doi:10.3390/heritage1020026. |
[49] | T. Hellmund, P. Hertweck, J. Mossgraber, D. Hilbring, P. Pouli and G. Padeletti, Implementing the HERACLES ontology – an ontology as backbone for a knowledge base in the cultural heritage protection domain, International Journal on Advances in Intelligent Systems 12: (3&4) ((2019) ), 158–169. |
[50] | J. Henderson and R. Waller, Effective preservation decision strategies, Studies in Conservation 61: (6) ((2016) ), 308–323. doi:10.1179/2047058415Y.0000000019. |
[51] | C.Y. Huang and C.H. Wu, A WebService protocol realizing interoperable Internet of things tasking capability, Sensors 16: (6) ((2016) ), 1395. doi:10.3390/s16091395. |
[52] | J. Hunter and S. Odat, Building a semantic knowledge base for painting conservators, in: 2011 7th IEEE International Conference on EScience, IEEE, Stockholm, (2011) , pp. 173–180. doi:10.1109/eScience.2011.32. |
[53] | IRUG, Keyword Search IRUG Spectral Database (2022) http://www.irug.org/search-spectral-database (accessed June 2, 2022). |
[54] | ISA Programme of the European Union, ISA Program Person Core Vocabulary (2012) https://www.w3.org/ns/person (accessed June 2, 2022). |
[55] | A. Karmacharya, S. Wefers and F. Boochs, Knowledge based recommendation on optimal spectral and spatial recording strategy of physical cultural heritage objects, in: 10th International Conference on Advances in Semantic Processing, IARIA, XPS Press, Venice, (2016) , pp. 49–58. |
[56] | T.H. Kolbe, Representing and exchanging 3D city models with CityGML, in: 3D Geo-Information Sciences, J. Lee and S. Zlatanova, eds, Springer, Berlin, Heidelberg, (2009) , pp. 15–31. doi:10.1007/978-3-540-87395-2_2. |
[57] | D. Kouis and G. Giannakopoulos, Incorporate cultural artifacts conservation documentation to information exchange standards – the DOC-CULTURE case, in: Procedia – Social and Behavioral Sciences, Vol. 147: , (2014) , pp. 495–504. doi:10.1016/j.sbspro.2014.07.144. |
[58] | D. Kouis, D. Kyriaki-Manesi, S. Zervos, G. Giannakopoulos, E. Cheilakou and M. Koui, Integrating non destructive testing techniques data for cultural heritage monuments to CIDOC conceptual reference model, in: 9th International Symposium on the Conservation of Monuments in the Mediterranean Basin, Ankara, (2014) . |
[59] | D. Kouis, E. Vassilakaki, E. Vraimaki, E. Cheilakou, A.C. Saint, E. Sakkopoulos, E. Viennas, E.V. Pikoulis, N. Nodarakis, N. Achilleopoulos, S. Zervos, G. Giannakopoulos, D. Kyriaki-Manessi, A. Tsakalidis and M. Koui, Standardizing NDT&e techniques and conservation metadata for cultural artifacts, in: Metadata and Semantics Research, MTSR 2015, E. Garoufallou, R. Hartley and P. Gaitanou, eds, Communications in Computer and Information Science, Vol. 544: , Springer, Cham, (2014) , pp. 1–12. doi:10.1007/978-3-319-24129-6_38. |
[60] | C. Lagoze, The oreChem project: Integrating chemistry scholarship with the semantic web, in: WebSci’09, Society On-Line, Athens, (2009) . |
[61] | C. Lagoze, H. Van De Sompel, M.L. Nelson, S. Warner, R. Sanderson and P. Johnston, Object Re-Use & Exchange: A Resource-Centric Approach, ArXiv. (2008). |
[62] | C. Lahanier and M. Aubert, Network of art research computer image systems in Europe (NARCISSE), in: Museums and Interactive Multimedia: Selected Papers from the Second International Conference on Hypermedia and Interactivity in Museums, Archives and Museum Informatics, D. Lees, eds, Cambridge, (1993) , pp. 298–304. |
[63] | O. Lassila and R. Swick, Resource Description Framework (RDF) Model and Syntax Specification W3C Recommendation, 1999, http://www.w3.org/TR/1999/REC-rdf-syntax-19990222/, (accessed June 2, 2022). |
[64] | R. Letellier, W. Schmid and F. LeBlanc, Recording, Documentation, and Information Management for the Conservation of Heritage Places: Guiding Principles, Getty Conservation Institute, CA, Los Angeles, 2007. |
[65] | S. Liang, C.Y. Huang and T. Khalafbeigi, OGC SensorThings API, Part 1: Sensing, Version 1.0, Wayland, MA, Open Geospatial Consortium, (2016) . doi:10.25607/OBP-455. |
[66] | Linked Conservation Data, Linked Conservation Data (2022). https://www.ligatus.org.uk/lcd/ (accessed June 2, 2022). |
[67] | G. Lodi, L. Asprino, A.G. Nuzzolese, V. Presutti, A. Gangemi, D.R. Recupero, C. Veninata and A. Orsini, Semantic web for cultural heritage valorisation, in: Data Analytics in Digital Humanities, Multimedia Systems and Applications, S. HaiJew, ed., Springer, Cham, (2017) , pp. 3–37. doi:10.1007/978-3-319-54499-1_1. |
[68] | H. Marçal, R. Macedo, A. Nogueira and A. Duarte, Whose decision is it? Reflections about a decision making model based on qualitative methodologies, CeROArt ((2013) ). doi:10.4000/ceroart.3597. |
[69] | T. Messaoudi, L. De Luca and P. Véron, Towards an ontology for annotating degradation phenomena, in: Digital Heritage International Congress DH’15, IEEE, Granada, (2015) , pp. 1–4. doi:10.1109/DigitalHeritage.2015.7419528. |
[70] | T. Messaoudi, P. Véron, G. Halin and L. De Luca, An ontological model for the reality-based 3D annotation of heritage building conservation state, Journal of Cultural Heritage 29: ((2017) ), 100–112. doi:10.1016/j.culher.2017.05.017. |
[71] | Metadata Object Description Schema – Library of Congress, Metadata Object Description Schema (2022). https://www.loc.gov/standards/mods/design-principles-mods-mads.html (accessed June 2, 2022). |
[72] | MFA-BOSTON, CAMEO Materials Database Search (2022). http://cameo.mfa.org/wiki/Category:Materials_database (accessed June 2, 2022). |
[73] | S. Michalski and M. Rossi-Doria, Using decision diagrams to explore, document, and teach treatment decisions, with an example of their application to a difficult painting consolidation treatment, in: ICOM-CC 16th Triennial Conference, J. Bridgland, ed., Lisbon, (2011) , pp. 1–8. |
[74] | S.W. Michalski, Sharing conservation decisions: Tools, tactics, and ideas, in: Sharing Conservation Decisions: Current Issues and Future Strategies, International Centre for the Study of the Preservation and Restoration of Cultural Property (ICCROM), A. Heritage and J. Copithorne, eds, Rome, (2018) , pp. 183–202. |
[75] | M. Moore, Conservation documentation and the implications of digitisation, Journal of Conservation and Museum Studies. 7 (2001). doi:10.5334/jcms.7012. |
[76] | E. Moraitou, J. Aliprantis and G. Caridakis, Semantic preventive conservation of cultural heritage collections, in: Third International Workshop on Semantic Web for Cultural Heritage in Conjunction with 15th Extended Semantic Web Conference (ESWC 2018), Heraklion, Crete, (2018) . |
[77] | E. Moraitou, J. Aliprantis, Y. Christodoulou, A. Teneketzis and G. Caridakis, Semantic bridging of cultural heritage disciplines and tasks, Heritage 2: ((2019) ), 611–630. doi:10.3390/heritage2010040. |
[78] | E. Moraitou and E. Kavakli, Knowledge management using ontology on the domain of artworks conservation, in: Digital Cultural Heritage, M. Ioannides, ed., Lecture Notes in Computer Science, Vol. 10605: , Springer, Cham, (2018) . doi:10.1007/978-3-319-75826-8_5. |
[79] | S. Muñoz-Vi nas, Contemporary theory of conservation, Studies in Conservation 47: (1) ((2012) ), 25–34. |
[80] | M.A. Musen, The protégé project: A look back and a look forward, AI Matters 1: (4) ((2015) ), 4–12. doi:10.1145/2757001.2757003. |
[81] | R. Mustalish and D. Green, Digital Technologies and the Management of Conservation Documentation: A Survey Commissioned by the Andrew W. Mellon Foundation, 2009. |
[82] | N. Naoumidou, M. Chatzidaki and A. Alexopoulou, ARIADNE conservation documentation system: Conceptual design and projection on the CIDOC CRM framework and limits, in: Annual Conference of CIDOC, Athens, (2008) . |
[83] | C. Niang, C. Marinica, B. Bouchou-Markhoff, E. Leboucher, O. Malavergne, L. Bouiller, C. Darrieumerlou and F. Laissus, Supporting semantic interoperability in conservation-restoration domain: The PARCOURS project, Journal on Computing and Cultural Heritage 10: (3) ((2017) ), 16:1–16:20. doi:10.1145/3097571. |
[84] | C. Niang, C. Marinica, E. Leboucher, L. Bouiller and C. Capderou, An ontological model for conservation-restoration of cultural objects, Digital Heritage 2: ((2015) ), 157–160. doi:10.1109/DigitalHeritage.2015.7419476. |
[85] | C. Niang, C. Marinica, E. Leboucher, L. Bouiller, C. Capderou and B. Bouchou-Markhoff, Ontology-based data integration system for conservation-restoration data, in: 20th International Database Engineering & Applications Symposium, IDEAS 2016, E. Desai, B.C. Desai, M. Toyama and J. Bernardino, eds, ACM, (2016) , pp. 218–223. doi:10.1145/2938503.2938545. |
[86] | NIST, NIST Chemistry WebBook (2011). http://webbook.nist.gov/ (accessed June 2, 2022). |
[87] | S. Odat, A Semantic e-Science Platform for 20th Century Paint Conservation, Doctoral thesis, The University of Queensland, School of Information Technology and Electrical Engineering, 2011. |
[88] | OntoPia Ontology Network, OntoPia Ontology Network (2022) https://github.com/italia/daf-ontologie-vocabolari-controllati/tree/master/Ontologie (accessed June 2, 2022). |
[89] | J.M. Pérouse de Montclos, Architecture: Méthode et Vocabulaire, Éditions du Patrimoine, (2004) . |
[90] | S. Phillips, P. Walland, S. Modafferi, L. Dorst, M. Spagnuolo, C. Catalano, D. Oldman, A. Tal, I. Shimshoni and S. Hermon, Gravitate: Geometric and semantic matching for cultural heritage artefacts, in: Eurographics Workshop on Graphics and Cultural Heritage, the Eurographics Association, C.E. Catalano and L. De Luca, eds, (2016) . doi:10.2312/gch.20161407. |
[91] | N. Platia, M. Chatzidakis, C. Doerr, L. Charami, C. Bekiari, K. Melessanaki, K. Hatzigiannakis and P. Pouli, ‘POLYGNOSIS’: The development of a thesaurus in an ed- ucational web platform on optical and laser-based investigation methods for cultural heritage analysis and diagnosis, Heritage Science 5: ((2017) ), 50. doi:10.1186/s40494-017-0163-0. |
[92] | D.P. Pocobelli, J. Boehm, P. Bryan, J. Still and J. Grau-Bové, BIM for Heritage Science: A Review, Heritage Science 6: ((2018) ), 30. doi:10.1186/s40494-018-0191-4. |
[93] | Polygnosis User Manual (2015). https://projects.ics.forth.gr/isl/KRHPIS-POLITEIA/educational-material/PolygnosisUserManualVersion1.0September2015.pdf (accessed June 2, 2022). |
[94] | K.A. Porter, A Taxonomy of Building Components for Performance-Based Earthquake Engineering, PEER Report 2005/3, Pacific Earthquake Engineering Research Center College of Engineering University of California, Berkeley, 2005, (accessed June 2, 2022). |
[95] | PREMIS: Preservation Metadata Maintenance Activity, Preservation Metadata Maintenance Activity (2022). http://www.loc.gov/standards/premis/ (accessed June 2, 2022). |
[96] | R.G. Raskin and M.J. Pan, Knowledge representation in the semantic web for Earth and environmental terminology (SWEET), Computers & Geosciences 31: (9) ((2005) ), 1119–1125. doi:10.1016/j.cageo.2004.12.004. |
[97] | M.H. Rasmussen and M. Lefrançois, Ontology for Property Management, (2018) , https://w3c-lbd-cg.github.io/opm/, (accessed June 2, 2022). |
[98] | M.H. Rasmussen, P. Pauwels, M. Lefrançois and G.F. Schneider, Building Topology Ontology, (2021) , https://w3c-lbd-cg.github.io/bot/, (accessed June 2, 2022). |
[99] | Resource Description and Access (RDA), Library of Congress, Resource Description and Access, 2022, https://www.loc.gov/aba/rda/, (accessed June 2, 2022). |
[100] | O. Signore, The semantic web and cultural heritage: Ontologies and technologies help in accessing museum information, in: Information Technology for the Virtual Museum, (2006) . |
[101] | D. Simeone, S. Cursi and M. Acierno, BIM semantic-enrichment for built heritage representation, Automation in Construction 97: ((2019) ), 122–137. doi:10.1016/j.autcon.2018.11.004. |
[102] | S. Staab and R. Studer, Handbook on Ontologies, Springer, Berlin, Heidelberg, (2009) . doi:10.1007/978-3-540-92673-3. |
[103] | Stracturae, International Database and Gallery of Structures, (2022) , https://structurae.net/en/, (accessed June 2, 2022). |
[104] | P. Tiano, A. Tibaut, B. Kaucic, R. Jardim-Goncalves and H. Panetto, Knowledge based IT platform for Heritage Buildings, Innovation in Intelligent Management of Heritage Buildings (i2MHB), 2019, pp. 41–55. doi:10.1484/M.DEM-EB.5.118097. |
[105] | A. Tibaut, B. Kaucic and D. Dvornik Perhavec, Ontology-based data collection for heritage buildings, in: Digital Cultural Heritage, M. Ioannides, ed., Lecture Notes in Computer Science, Vol. 10605: , Springer, Cham, (2018) . doi:10.1007/978-3-319-75826-8_6. |
[106] | A. Tibaut, B. Kaučič, D. Dvornik Perhavec, P. Tiano and J. Martins, Ontologizing the heritage building domain, in: Advances in Digital Cultural Heritage, M. Ioannides, J. Martins, R. Žarnić and V. Lim, eds, Lecture Notes in Computer Science, Vol. 10754: , Springer, Cham, (2018) . doi:10.1007/978-3-319-75789-6_11. |
[107] | UNESCO, Cultural Heritage, (2022). http://uis.unesco.org/en/glossary-term/cultural-heritage (accessed June 2, 2022). |
[108] | G. Vasilakis, A. Garcia-Rojas, L. Papaleo, C.E. Catalano, F. Robbiano, M. Spagnuolo, M. Vavalis and M. Pitikakis, Knowledge-based representation of 3D media, International Journal of Software Engineering and Knowledge Engineering 20: (5) ((2010) ), 739–760. doi:10.1142/S0218194010004773. |
[109] | E. Vassilakaki, S. Zervos and G. Giannakopoulos, CIDOC-CRM extensions for conservation processes: A methodological approach, in: AIP Conference Proceedings, Vol. 1644: , (2015) , pp. 185–192. doi:10.1063/1.4907835. |
[110] | A. Velios, Online event-based conservation documentation: A case study from the IIC website, Studies in Conservation. 61 (2015). |
[111] | V. Vergès-Belmin, Illustrated Glossary on Stone Deterioration Patterns, Monuments and Sites, ICOMOS, France, (2008) . |
[112] | A. Wagner, M. Bonduel and P. Pauwels, Ontology for Managing Geometry, (2019) , https://www.projekt-scope.de/ontologies/omg/archive/0-3/, (accessed June 2, 2022). |
[113] | R. Wang and W. Chen, Case-based reasoning of damaged ancient buildings based on ontology, in: E3S Web Conf, Vol. 165: , (2020) , p. 04007. doi:10.1051/e3sconf/202016504007. |
[114] | S. Wefers and A. Karmacharya, Ontology-based structuring of spectral and spatial recording strategies for cultural heritage assets: Background, state of affairs, and future perspectives, in: Digital Techniques for Documenting and Preserving Cultural Heritage, Collection Development, Cultural Heritage and Digital Humanities, A. Bentkowska-Kafel and L. MacDonald, eds, ARC Humanities Press, Leeds, (2017) , pp. 157–172. doi:10.1515/9781942401353-013. |
[115] | A. Weyer, P. Roig Picazo, D. Pop, J. Cassar, A. Özköse, J.M. Vallet and I. Srša, EwaGlos-European Illustrated Glossary of Conservation Terms for Wall Paintings and Architectural Surfaces, (2015) , http://www.ewaglos.eu/pages/download.php, (accessed June 2, 2022). |
[116] | A.I.C. Wiki and A.I.C. Wiki, 2022, https://www.conservation-wiki.com/wiki/Main_Page, (accessed June 2, 2022). |
[117] | O. Zalamea Patiño and G. García, Validation of the BCH-ontology, in: The International Archives of the Photogrammetry, Remote Sensing and Spatial Information Sciences, (2020) , pp. 497–504, XLIV-M-1-2020, 2020. doi:10.5194/isprs-archives-XLIV-M-1-2020-497. |
[118] | O. Zalamea Patiño, J. Van Orshoven and T. Steenberghen, From a CityGML to an ontology-based approach to support preventive conservation of built cultural heritage, in: Proceedings of the 19th AGILE International Conference on Geographic Information Science, Springer, Belgium, (2016) . |
[119] | O. Zalamea Patiño, J. Van Orshoven and T. Steenberghen, Merging and expanding existing ontologies to cover the built cultural heritage domain, Journal of Cultural Heritage Management and Sustainable Development 8: (2) ((2018) ), 162–178. doi:10.1108/JCHMSD-05-2017-0028. |
[120] | A. Zerbini, Developing a heritage database for the middle East and North Africa, Journal of Field Archaeology 43: (1) ((2018) ), 9–18. doi:10.1080/00934690.2018.1514722. |
[121] | A. Zreik and Z. Kedad, Matching conservation-restoration trajectories: An ontology-based approach, in: Research Challenges in Information Science, RCIS 2021, S. Cherfi, A. Perini and S. Nurcan, eds, Lecture Notes in Business Information Processing, Vol. 415: , Springer, Cham, (2021) . doi:10.1007/978-3-030-75018-3_15. |