Analyzing biography collections historiographically as Linked Data: Case National Biography of Finland
Abstract
Biographical collections are available on the Web for close reading. However, the underlying texts can also be used for data analysis and distant reading, if the documents are available as data. Such data is usable for creating intelligent user interfaces to biographical data, including Digital Humanities tooling for visualizations, data analysis, and knowledge discovery in biographical and prosopographical research. In this paper, we re-use biographical collection data from a historiographical perspective for analyzing the underlying collection. For example: What kind of people have been included in the collection? Does the language used for describing female biographees differ from that for men? As a case study, the Finnish National Biography, available as part of the Linked Open Data service and semantic portal BiographySampo – Finnish Biographies on the Semantic Web is used. The analyses show interesting results related to, e.g., how specific prosopographical groups, such as women or professional groups are represented and portrayed. Various novel statistics and network analyses of the biographees are presented. Our analyses give new insights to the editors of the National Biography as well as to researchers in biography, prosopography, and historiography. The presented approach can be applied also to similar biography collections in other countries.
1.Introduction
Biographical dictionaries are scholarly resources used by the public and by the academic community alike. Most national biographical dictionaries follow the traditional form of combining a lengthy non-structured text, often written with authorial individuality and personal insight, with a structured synopsis of basic biographical facts, such as family relations, education, works, career events, and so on. Biographies are an invaluable information source for researchers across various disciplines with an interest in the past [30]. A well-known example of a biographical dictionary is the Oxford Dictionary of National Biography (ODNB)11 with more than 60000 lives. It was published in print and online in 2004, and since then many dictionaries have opened their editions on the Web. These include USA’s American National Biography,22 Austrian Prosopographical Information System,33 Germany’s Neue Deutsche Biographie,44 Biography Portal of the Netherlands,55 The Dictionary of Swedish National Biography,66 and the National Biography of Finland77 (NBF). There are also many “who is who” services online, and Wikipedia contains lots of short biographies.
In this paper, we use the BiographySampo portal and its data, based on the National Biography of Finland, to study and analyze biographees, their lives, and the source material with two goals in mind. Firstly, our goal is to argue and show that using biographies as Linked Data opens up unprecedented new possibilities for the study by distant reading [41,42]. Secondly, the analyses present novel insights into the nature and contents of the NBF. Here, our focus is on the historiographical analysis of biographies. We anticipate that comparative results can be expected, if the methodology and tools introduced are applied to similar national biographical dictionaries. Our approach can also be applied to other domains of Cultural Heritage data, such as museum collections, library catalogs, manuscripts in archives, archaeological finds, etc., as demonstrated by the Sampo series of semantic portals88 [17].
1.1.National Biography of Finland
In Finland, the National Biography collection and several other collections of biographical and prosopographical data have been compiled and are maintained by the Finnish Literature Society (SKS)99 established in 1831. The work has been carried out by the Biographical Centre of the SKS, now part of the society’s scholarly publishing house, in collaboration with several Finnish learned societies and researchers in different fields.
The kernel of the collection is the National Biography of Finland (Suomen kansallisbiografia in Finnish), based on the biographies written in collaboration with the Finnish Historical Society in 1993–2001. The NBF was created for an educated reader, who is not an expert in history. Historical terms and concepts are explained, and the biographees are presented within the frame of national history. The articles have been written with a critical attitude and in accordance with sound historiographical methods. The facts and the emphasis of the articles must derive from recent research and be well argued. The NBF strives to be enjoyable and interesting reading as well as to bring new insights into the impact of individuals in history. In addition to the general reader, the NBF is also a useful handbook for researchers from all fields who are seeking reliable biographical information. The articles have been peer reviewed and contain reference to archival sources and literature.
The NBF contains 6500 lives and goes back a thousand years in history. The National Biography of Finland was one of the largest projects ever carried out in the field of history in Finland: it involved twenty historians serving in the three editorial boards (Swedish era, Russian era, and Independence era) and over 900 other scholars who wrote the biographies. The writing of the articles began in 1993 and the first articles were published online in 1997 when Finland celebrated her 80 years of independence. The majority of the biographies were written before the year 2000. Some 6000 articles were published in print in 2003–2007 (Suomen kansallisbiografia 1–10 [31]) by the Finnish Literature Society.
Early on in the project, half of the 6000 lives to be commissioned were allocated to the period of independence from 1917 onward. The Swedish era from the earliest decades to 1809 and the Russian era from 1809 to 1917 were each given a 25 percent of the entries.
Contrary to most national biographical dictionaries, the NBF includes people who are still alive, although most of them are already past the peak of their career and activity. The reason was the emphasis on the period of independence in the work of the editorial board. Had only deceased Finns been included, the big picture of the independence era created by the lives would have been incomplete and distorted.
In addition to the NBF, the Finnish Literature Society has also published other biographical collections, e.g., the Finnish Clergy 1554–1721 and 1800–1920, the Finnish Generals and Admirals in the Russian armed forces 1809–1917, and the Finnish Business Leaders, totaling today over 13100 biographies. The biographies have been made available also as a web service.1010 In 2018, the collections were re-published as the semantic portal BiographySampo – Finnish biographies on the Semantic Web [21] and it has had approximately some 40000, end-users on the Web.
1.2.A paradigm shift in publishing biography collections
BiographySampo1111 [21] is a semantic portal that is based on a knowledge graph that has been extracted automatically from textual biographies to its additional metadata. The portal has been built to help historians and scholars in biographical [45] and prosopographical research [10,53].1212 A major novelty of BiographySampo is to provide the user with data-analytic and visualization tools for solving research problems in Digital Humanities (DH), based on Linked Data [12,16]. The idea of publishing biographies as structured Linked Data for machines with ready-to-use tooling for humans to use in Digital Humanities research can be seen as a paradigm shift in the field of biographical publishing [18,21]. Traditionally, biographies have been published as printed texts, in our case as a series of ten volumes [31] of nearly 10000 pages. Then, the Web emerged as a publication channel for biographies for human consumption. In the case of the NBF, this happened already in 1997. BiographySampo demonstrates the next step ahead where the biographies are published not only as texts for close reading but also as machine “understandable” Linked Data for distant reading. This facilitates data analysis and tooling to be used for DH research, and even application of Artificial Intelligence to knowledge discovery, where the machine can help the user in finding research problems, in solving them, and in explaining the results [18].
BiographySampo is based on the Sampo model [17] that formulates the idea of aggregating and publishing distributed, heterogeneous local data sources in a global linked data service. In this way, the data of all data providers can be enriched with each other’s content, by reasoning based on Semantic Web standards, and the global data can be used easily across original local data silo boundaries. This arguably creates a sustainable “business model” where every data provider wins through collaboration, and of course the end users in particular. Data alignment and linking in this approach is based on a shared global data model and a set of shared domain ontologies (places, people, etc.) that are used for describing the contents of the different data sources for semantic interoperability.
The data is searched, explored, and analyzed in a kind of standardized way with the following way. Firstly, the landing page of the portal provides the user with multiple “perspectives” for searching and exploring the underlying data. In our case, biographical data can be accessed from seven search perspectives [21]: Persons, Places, Lives on maps, Statistics, Networks, Relations, and Linguistics. Secondly, each perspective provides the end-user with a semantic faceted search engine, where the results can be filtered and found flexibly by making selections using a set of orthogonal facets (e.g., place, time, person, etc.). Thirdly, after filtering down a target set of entities of interest, the set can be analyzed and visualized using a variety of ready-to-use data-analytic tools. For example, various map- and network-based visualizations and statistics are available. Furthermore, the SPARQL endpoint of the underlying Linked Open Data service can be used for querying, analyzing, and visualizing the data in flexible ways using tools, such as Yasgui [44] for SPARQL, or Jupyter1313 and Google Colab1414 by Python scripting. In this paper, analyses by both the ready-to-use tools of the portal and by using Google Colab on the underlying SPARQL endpoint will be presented. The portal interface was developed by using the SPARQL Faceter tool [32] that has later on been developed into the full stack Sampo-UI framework [26].
1.3.Related work
Biographical collections can be used to study the underlying historical world. However, the texts, the language used, and the biographical collection as a whole can also be studied from a different, historiographical perspective as an artifact reflecting its own time, the editorial values and biases in selecting the biographees, the authors’ perspectives, and also from a linguistic points of view. Such analyses have been already made for some national dictionaries of biography, e.g., for the ODNB [55] and the Irish Ainm [2].
Christopher N. Warren claims [55] that national dictionaries of biography, such as the ODNB, speak with a double voice: they give us information about things as they happened, but are at the same time a testimony about how a key piece of historiographical infrastructure was made. He sees the ODNB as data and, at the same time, as a historical artifact. There are also related studies using, e.g., Wikipedia articles as the data source [29,39]. This paper presents, in the same vein, a study of the National Biography of Finland. The methods and tools created in our work for the analysis are generic and can be re-used for similar tasks based on Linked Data standards. The data and SPARQL endpoint used are available at the Linked Data Finland platform1515 [25]. The work presented is novel in its way of using Linked Data for historiographical analysis of textual biographies. It is also arguably the first historiographical analysis of the NBF collection. The data is open for further analyses for anyone on the Web.
Aside publishing biographical dictionaries in print and on the Web, representing and analyzing biographical data has grown into a new research and application field. In 2015, the first Biographical Data in Digital World workshop BD2015 was held presenting several works on studying and analyzing biographies as data [51], and the proceedings of BD2017 contain more similar works [7]. In [34], analytic visualizations were created based on U.S. Legislator registry data. The idea of biographical network analysis is related to the Six Degrees of Francis Bacon system1616 [33,54] that utilizes data of the Oxford Dictionary of National Biography. However, a novelty of our approach is to use faceted search for filtering out target groups for studying. The work was influenced by the early Semantic NBF demonstrator [19] and its follow-up prototype [23], whose software has been applied also to a historical register of students [20] and to the U.S. Legislator data [40]. However, BiographySampo extends these systems into several new directions in terms of the DH tooling provided, such as faceted network analysis views, relational search, and text analysis views for studying the language of the biographies. Also, more heterogeneous datasets are used.
Extracting Linked Data from texts has been studied in several works, cf. e.g. [8,43]. In [6] language technology was applied for extracting entities and relations in RDF using Dutch biographies in the BiographyNet.1717 This work was part of the larger NewsReader project1818 extracting data from news [46]. This line of research is similar to ours, based on the idea of extracting RDF data from unstructured biographical texts. However, BiographyNet focuses more on the challenges of natural language processing and managing the provenance information of data from multiple sources, while our focus is on providing the end user with intelligent search and browsing facilities, enriched reading experience, and easy to use data-analytic tooling for biography and prosopography. The Austrian Prosopographical Information System (APIS) [1,9,47] is a virtual research environment that transforms text collections to machine readable formats and enables the use of natural language processing based methods to enrich the documents by extracting and linking information in them. The system has been used to transform and to study the collection of Austrian Biographical Dictionary 1815–1950 (ÖBL). Similarly to BiographySampo, the APIS can be used to analyze and visualize datasets using for example network analysis methods.
This paper is structured as follows. First, an overview of the NBF data and its transformation into Linked Open Data is described. After this, various data analyses are presented and discussed using the tools of the portal as well as Google Colab scripting. Finally, issues related to data quality and interpretation of the analyses are discussed, and directions for further research are outlined.
2.Transforming biographies into linked open data
This section explains contents of the NBF data to be used in our analyses, and how the source data was transformed into Linked Data and published in a SPARQL endpoint on the Semantic Web.
Fig. 1.
Amount of biographies by biographee’s birth decade; screenshot from the BiographySampo portal.
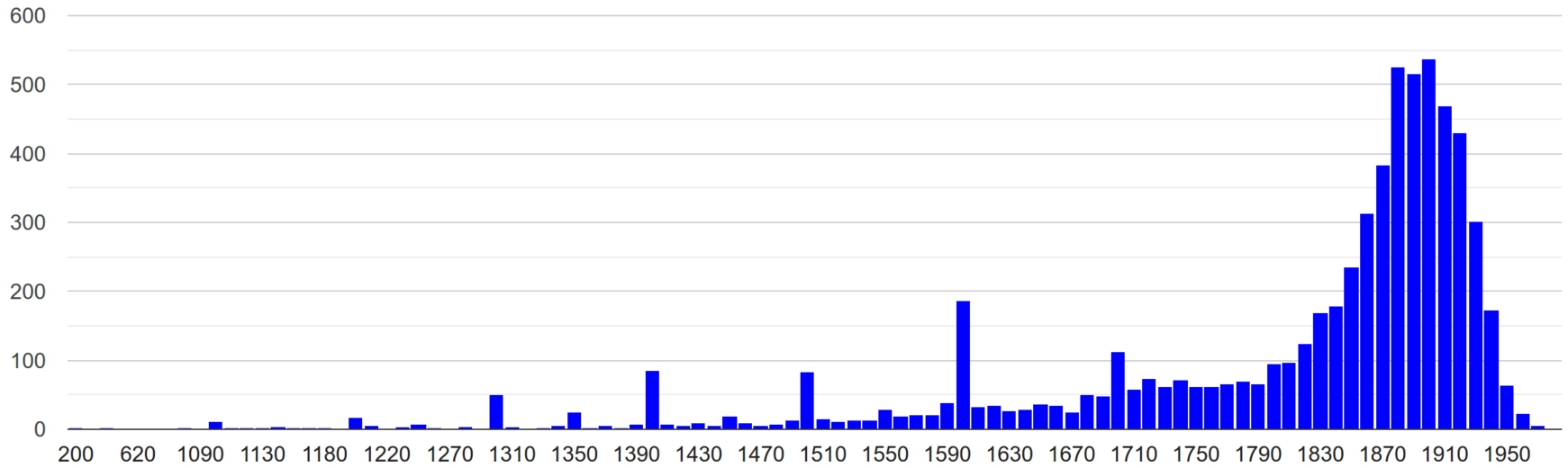
2.1.Source data
BiographySampo contains some 13100 biographies including the core NBF and four supplement datasets: Finnish Clergy 1554–1721, Finnish Clergy 1800–1920, Finnish Generals and Admirals 1809–1917, and Business Leaders. The NBF alone contains 6478 entries, 5268 men, 929 women, 11 couples, and 268 families [22]. In the NBF dataset, there were also two individual biographees whose gender is missing in the data. The earliest biographee is a saint approximately from the year 200, whereas there are also many biographies about living persons in the collection, such as Jenni Haukio, the current First Lady of Finland. The distribution of the biographical texts by decade can be seen in Fig. 1. In this paper, only men and women in the core NBF dataset are considered; the couples and the families are left out as well as the other four supplement datasets mentioned above.
A biography text in the NBF is represented in two major parts: First, there is a narrative text on the life of the biographee, including a lead section. This text is written in ordinary natural Finnish. The text is used in the online version of the NBF and includes hand coded HTML links to related biographies in the collection; this is the only semantic markup in the text. After the free text section, a summary of the person’s life is presented including basic data about the biographee (name, birth, death etc.) and information about family relations, life events, and career achievements [56]. In the NBF, the summary is unstructured text, too, but written in a semi-formal language using different section headings and notations for separating, e.g., information about family relations from career achievements. The sentences in the semi-formal part are shortened, use specific short hand notations, and do not, e.g., have predicates.
In addition to the biographical text, the NBF data includes structured metadata about the biographies and the biographees available as a spreadsheet in CSV format. The metadata contains the basic biographical information of the biographee, i.e., person names with possible variations like maiden or altered names, places and times of birth and death, vocational/occupational group of the person (Politics, Economics, Science, etc.), and a link to the photo of the person. The metadata is used as the basis for searching biographies in the online version of the NBF. In addition to biographical metadata, the dataset included information about the authors of the biographies, their gender and birth year.
In addition to the biographies, BiographySampo also makes use of several external data sources for enriching the data. For example, the biographees are linked with same as links to 16 additional data sources on the Web. One application perspective in BiographySampo, Relational Search for knowledge discovery [24], makes use of additional datasets extracted from collections of museums, libraries, and archives. This supplementary data is not considered or used in the analyses of this paper.
2.2.Transformation into Linked Data
In BiographySampo, the metadata CSV as well as the textual biographies were analyzed and transformed automatically into linked data, and links to external data sources were established. The modeling choices, transformation, and enriching of the data have been described in various articles throughout the project [22,24,35,48,49]. The result was published as a SPARQL endpoint that was used as the basis for the semantic portal and the analyses presented in this paper. The data in the service can be divided into the following conceptual categories:
Basic information about the biographees This data is based on the metadata CSV. A custom NBF namespace is used in addition with Dublin Core Metadata Initiative (DCMI) Metadata Terms1919 and Schema.org.2020 During the data transformation, the literal property values of persons, such as variations of family and given names, lifetime dates, and URLs for person images where transformed into data resources according to the data schema while some data values, such as vocations, vocational groups, and places of birth and death, were aligned with the domain ontologies of BiographySampo. This data is reliable as it is hand coded by the editors and authors of the NBF, and the terminology used, such as vocational groups, is controlled and unambiguous.
Metadata about biography documents The author and publishing date data was extracted from the hand coded CSV metadata. Here, the NBF namespace is supplemented with the Dublin Core (DC) Metadata Element Set,2121 DCMI Metadata Terms, and Schema.org. The free text and semi-formal summary paragraphs were categorized based on content to be able to target different categories for different data analytical applications and knowledge extraction. The content types included free text paragraphs such as the lead paragraph and the narrative text whereas the semi-formal was typed to summary of person’s life, family relations, life events, and career achievements. This was done to distinguish the content type for automatic annotation processes. The lead paragraph was found from 6500 biographies, narrative text from 6500 and family relations from 6220, and career events or achievements from 6430 biographies. The accuracy of the classification of the text paragraphs was 98.5%. It was estimated for 200 randomly picked paragraphs and the most common error was mixing lead paragraph and narrative text paragraph in biographies that had unusual document structure. In addition, the subject matter of biography texts, based on the free text parts, was analyzed using automatic annotation and represented using keywords taken from the Finnish General Ontology YSO.2222
Reference network to other biographees within the NBF The data about the biographee resources was enriched with internal links to other biographees. The links were extracted in two different ways: (1) Linkage based on the hand coded directed HTML reference links between the biographies. (2) Linkage based on mentions of persons in the free text parts of the biographies. The HTML links were extracted while transforming the text to RDF [49] with 99.4% accuracy that was estimated for randomly selected 36 documents containing 176 links. The mentioned people were extracted computationally using Named Entity Linking [38,48]. The accuracy of named entity linking succeeded with 74.0% accuracy. The networks based on link types 1 and 2 can be used independently from each other in analyses; the choice can be made, e.g., in the portal user interface. The modeling choices are described in more detail in [48,49].
Linkage network to persons in external data sources Data about the person resources was enriched with “same as” links to 16 external biographical data sources, such as Wikidata,2323 Getty Union List of Artist Names (ULAN),2424 The Virtual International Authority File (VIAF),2525 Finnish databases providing biographical information, and other Sampo portals on the Semantic Web. In most cases, this linking could be made accurately using names and dates of birth and death. In addition, most of the biographees have an entry in Wikidata, especially those who lived after the 18th century. However, for people of medieval times the available information about his/her years of living might be inadequate. Different databases often use different name variations of the same person. For example, the names of notable medieval Swedish people are translated to Finnish in the NBF.
Personal life events The life of each biographee was described semantically in terms of spatio-temporal events which they participated in. The event data was extracted from the semi-formal summaries of the biographies using regular expressions. However, the events of birth and death are based on the CSV metadata. The life event data has been modelled using an actor-event schema based on the CIDOC CRM standard.2626 Here life events fall in different subclasses and are characterized by properties that tell the place, time, and participants of the event. According to our evaluation 97.5% of the expressions of time were correctly extracted and interpreted from the texts. The main disambiguation and linking challenge here were the historical place names used in descriptions, but this could also be performed fairly reliably with a precision of 98.4% and a recall of 85.7%.
Genealogical network A separate genealogical network was created automatically based on the mentions of different family relations, mother, father, child, or spouse in the semi-formal part of the biographies. This data was enriched by reasoning the gender of mentioned persons if needed [50] and by inferring additional relations, such as grandfather or cousin. The genealogical network includes lots of historical persons that do not have a biography in the NBF. Generally, according to our evaluation 93.9% of the mentioned person names were correctly interpreted in the conversion process.
Family relations are modelled using the Bio CRM model [52], an extension of the CIDOC CRM standard. The method and process of extracting the family relations is described and the results are evaluated in [35].
Linguistic descriptions of biography texts A linguistic knowledge extraction pipeline was created for analyzing the free text parts of the biographies. It identifies text structures, such as paragraphs, sentences, and words, including morphological analysis data (e.g., part-of-speech tags (POS), lemmas, and dependency grammar information). The results were described using mainly the NLP Interchange Format (NIF) [13–15] and the CoNLL namespace by using the CoNLL-RDF [5] tool. The model was extended with the DC Metadata Element Set, DCMI Metadata Terms, and the NBF namespace for describing, for example, relations between text structures (e.g., documents and its paragraphs, sentences, and words) to facilitate querying the linguistic data in detail. The linguistic knowledge graph was also enriched with additional precalculated relations that are used for making SPARQL queries simpler and more efficient in the BiographySampo portal. According to our evaluation the linguistic graph for the NBF extraction succeeded with 100% for paragraphs, 99.5% for sentences, 99.0% for words, and 95.6% for POS tags. The results were calculated for 200 randomly selected entities in each category. Sometimes initials (e.g., J. A. von Essen) caused issues with sentence splitting and for POS tagging (the tags for initials varied between SYM and PROPN), while sometimes timespans (e.g., 2008–2009 was occasionally split to two word tokens as hyphen was included in either of the numbers) caused issues for token classification.
The quality of the data in these categories in terms of uncertainty, incompleteness, and errors is different depending on the data source and the knowledge extraction process used. This matter will be discussed later in chapter 3 when presenting and interpreting the analyses made using these data.
Fig. 2.
Amounts of extracted biographical and linguistic data.
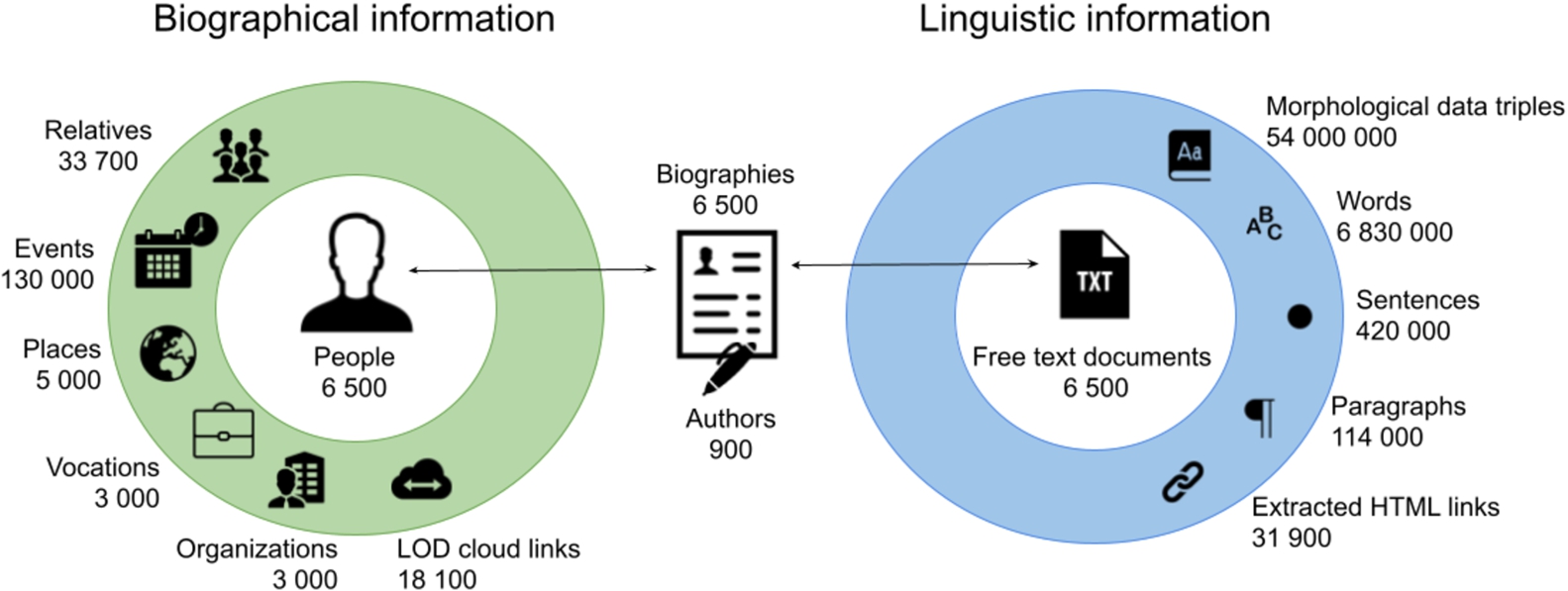
The final outcome of the knowledge extraction process is illustrated in Fig. 2. The linked data is divided into mutually related biographical and linguistic knowledge graphs. The size on the knowledge graphs is documented in terms of the number of instances in different classes, except for the values of LOD cloud links and Morphological data, which are amounts of triples. For example, the biographees were involved in all together 117000 events during their lives, and the free text parts contain nearly 7 million words.
2.3.Linked open data service
Finally, the transformed knowledge graphs were published openly (under the CC BY 4.0 license,2727 excluding data about the biographical texts and living people) on the Linked Data Finland platform LDF.fi2828 [25]. LDF.fi provides the user with a standard SPARQL endpoint for querying the data,2929 on top of which the online BiographySampo portal was implemented. In addition, the data service supports best practices on W3C for publishing Linked Data [12]. A URI identifier resolving mechanism is provided. This means, for example, that if a URI is typed in a browser, a HTML protocol is returned that shows the corresponding data as a human readable HTML page that can be examined further by linked data browsing. In the same vein, the data in RDF form can be accessed by applications by using the HTML protocol. It is also possible to download the data in textual form for off-line processing. The LDF.fi platform also includes additional tools that aim at helping the user to re-use the data. For example, schemas are documented automatically for the human user by a schema documentation generator, the LODE Documentation Environment3030 service. The data model for the NBF is documented for people and biography metadata in [21], linguistic knowledge graph in [49], and for enrichment with named entities in [48].
3.Analyzing and visualizing the National Biography of Finland
In this chapter, we present analyses based on the NBF data service. In BiographySampo there are ready-to-use tools [35,36,49] for general statistics and more conceptual categories such as linguistic analysis, network analysis, and map visualizations. This chapter starts with general statistics. After this more detailed analyses based on the conceptual categories of data are presented and interpreted. Some analyses can be tested online in BiographySampo as part of the tool set available there. For others, the SPARQL endpoint has been used with Google Colab, and a variety of Python data analysis and visualization tools such as Matplotlib.3131
3.1.General collection statistics
The general statistics of the NBF can be created and visualized in BiographySampo with versatile options. The statistics tell about the demographic nature of the people included in the dataset. The statistical tools are available online through a “Statistics” application perspective,3232 with separate tabs for histograms, pie chars, and a Sankey chart for analyzing the family relations of the biographees. In all tabs it is possible to focus the statistical analyses prosopographically to subsets of biographees, such as women or people born on a certain time period in Helsinki, by using a faceted search/filtering engine. Filtering the data is also possible using non-demographic metadata, such as authorship of the biographies and the inclusion of the biographee in other data sources, such as Wikipedia/Wikidata or ULAN. In addition, there are separate tabs available for making comparisons between subsets of the biographees, like between two vocational groups.
In Fig. 1, the number of biographies have been plotted by decade. The plot is taken from the BiographySampo portal’s statistical analysis page. In the plot, the decade has been selected based on the birth year of the biographee. The distribution shows a peak of biographies that have been written about people born between the end of 19th century and the beginning of the 20th century and they have been active when the Finnish identity as a sovereign nation was established. There are also a few peaks earlier in history that are in general less well-known in Finnish history. In some cases, the data is not accurate enough and the birth year of a biographee is not known. In these cases it has been set to the beginning of a century, which explains the earlier peeks in the beginning of each century.
Fig. 3.
Number of male and female biographees alive on a timeline.
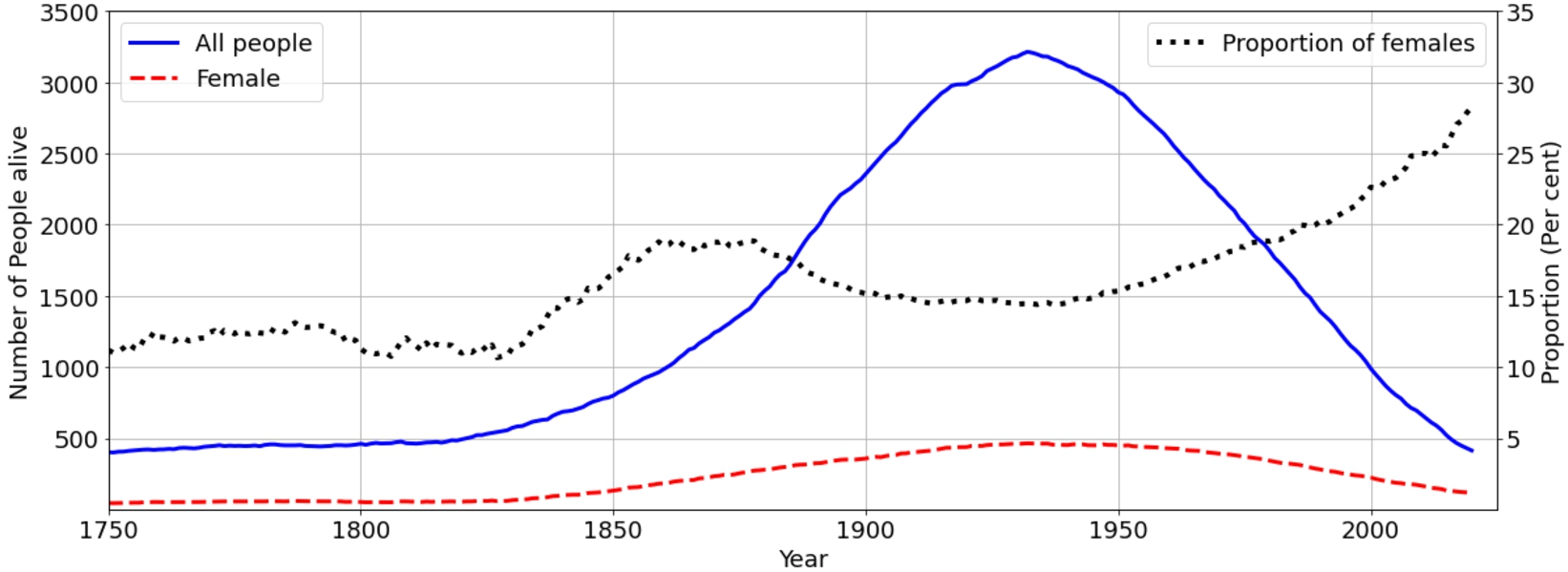
Similarly to [55] we have plotted the distribution of people alive on a timeline based on biographee’s birth and death data. Figure 3 depicts the number of biographees alive in different times but due to lack of total population information in Finland before 1900s we do not have comparison between biographees and general population but we wanted to look at women in contrast to all biographees. The blue curve is the total amount, the dashed red curve the amount of females, and the dotted line is the proportion of females. The curve indicates that the largest number of biographees lived during the first half of the 20th century. The total curve appears smooth and does not show sudden changes due to historical events, e.g., the Second World War. The female percentage reaches a local maximum during the late 19th century and is growing constantly from 1950.
Fig. 4.
Average lifespan of the biographee’s; screenshot from the BiographySampo portal.
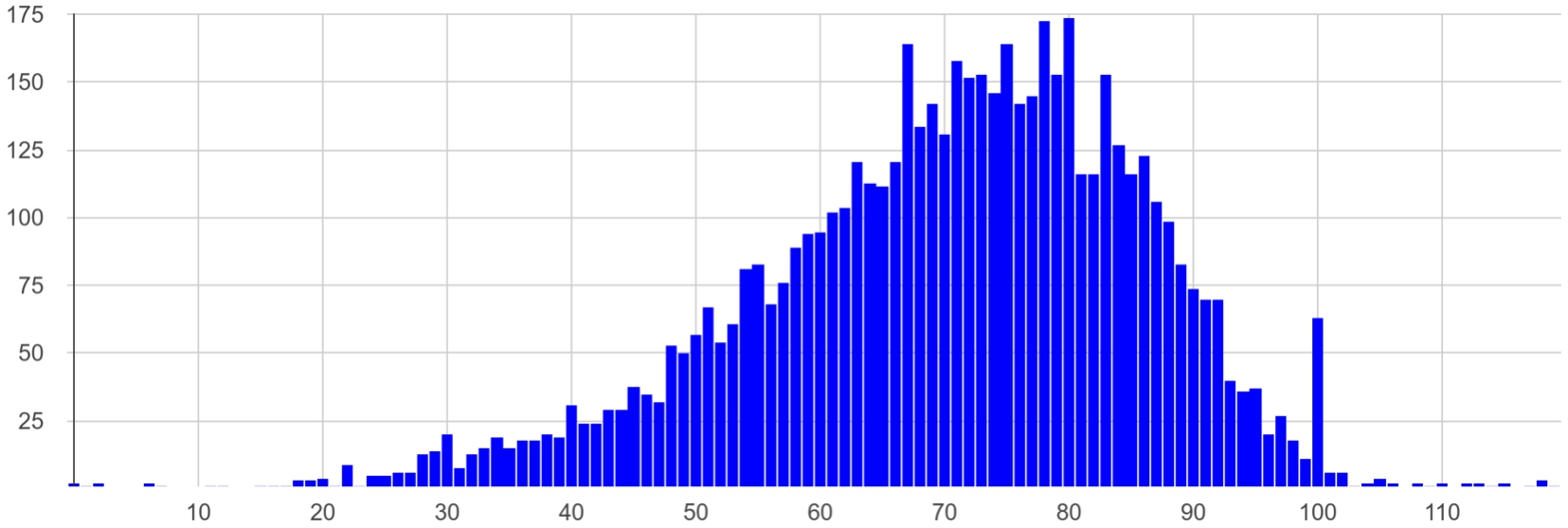
BiographySampo portal also allows one to look at the properties of the biographees, such as their average lifespan depicted in Fig. 4. The average life span for all biographees is 70.2 years. When comparing the male and female biographees, women on average live up to 72.2 years and men 69.8 years of age. Most biographees have died during their adulthood, but there are a few exceptions. For example, Sigfrid Jusélius (1887–1898),3333 who died at the age of 11, was included in the collection because her father, the well-known tycoon Fritz Arthur Jusélius (1855–1930)3434 founded with his will the Sigfrid Jusélius Foundation3535 to promote medical research. Another example is soldier Yrjö Saarenpuu (1901–1919)3636 who was executed in a peculiar situation at the age of 19 instead of another person. There also seems to be quite a few biographees who lived 100 years old. However, the peek at 100 years is not a fact but results from the underlying data. At the moment, the underlying data does not tell whether a year, such as 1100 is rounded, or actually is a precise value.
Fig. 5.
Average age of marriage; screenshot from the BiographySampo portal.
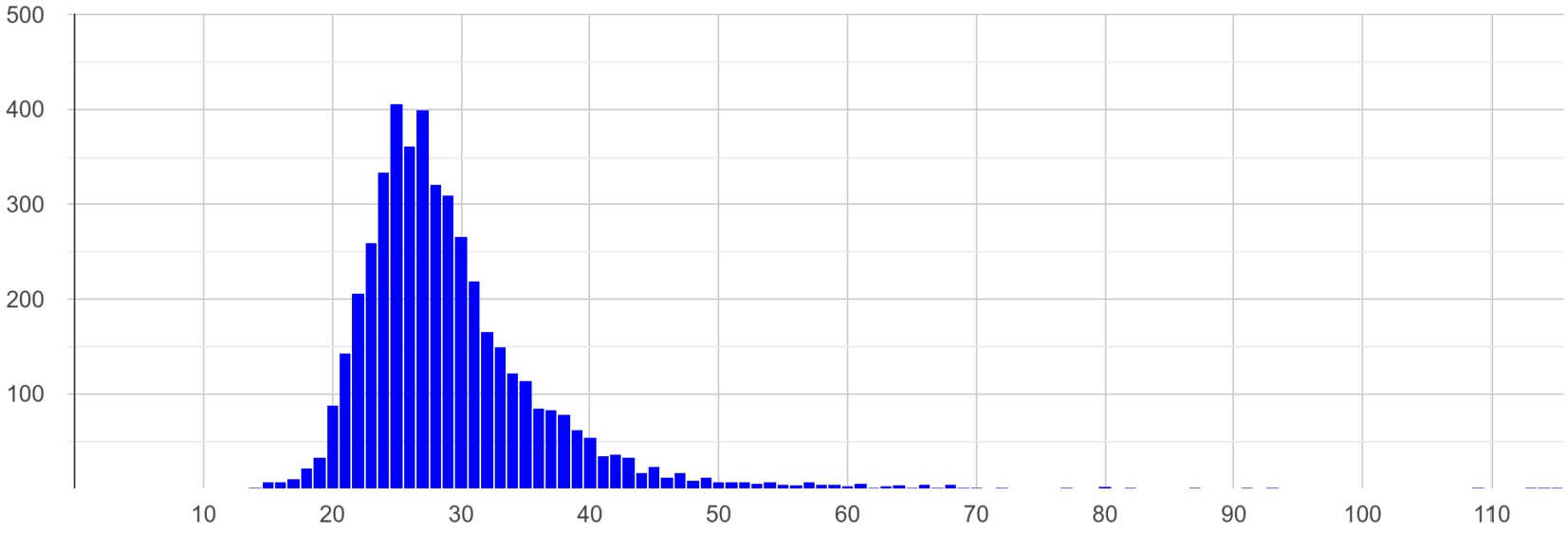
The statistics application perspective of BiographySampo gives also insight into the life events of the biographies, such as getting married or having children. For example, Fig. 5 shows that the biographees got married on average at the age of 29 but there are also a few teen marriages and some older couples. A comparison of male and female biographees shows that women marry younger at the age of 26 than men at the age of 30 years. Men also marry more often after the age of 60 years.
Fig. 6.
Average number of spouses for female and male biographees; screenshots from the BiographySampo portal.
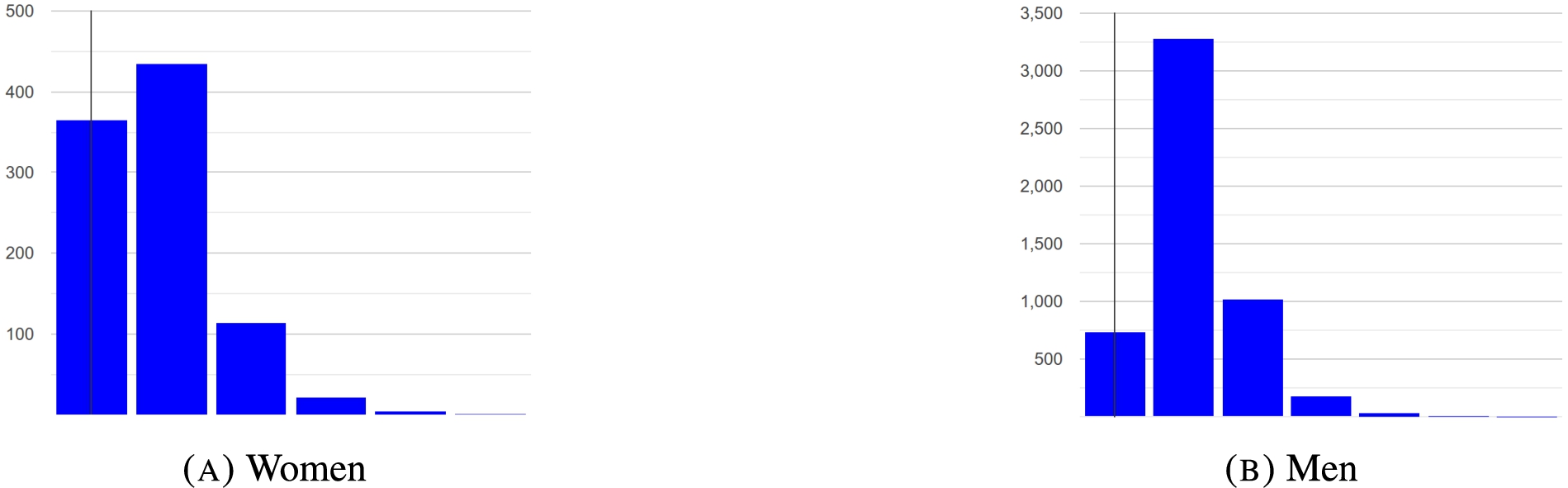
Fig. 7.
Average number of children for female and male biographees; screenshots from the BiographySampo portal.
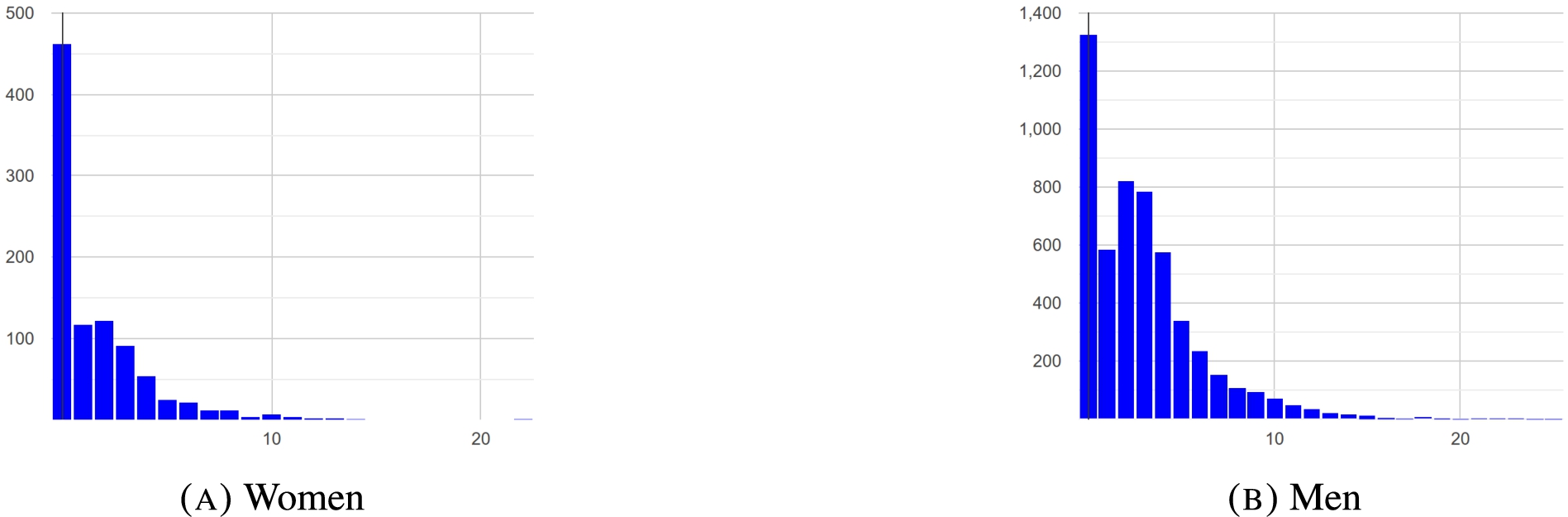
There are also statistics about the number of children and spouses in the portal. The Fig. 6 the number of spouses for women and men and the Fig. 7 represents the amount of children. These plots are taken from the BiographySampo’s statistics comparison view. Women’s statistics are on the left hand side whereas the men’s statistics are on the right hand side. Based on the statistics most women are married but have no children whereas men are mostly married to one partner and have no children. On average men have more children than women. Based on further data analysis using SPARQL queries,3737 there are approximately 30.3% (286) of women and 9.32% (493) of men who are unmarried and childless. Using a different SPARQL query3838 it can be noted that the most common vocation for these childless and unmarried women is a teacher whereas for men it’s a professor.
Fig. 8.
Sankey diagram depicting the correlations between the vocations of husbands and wifes; screenshot from the BiographySampo portal with English translations in red text.
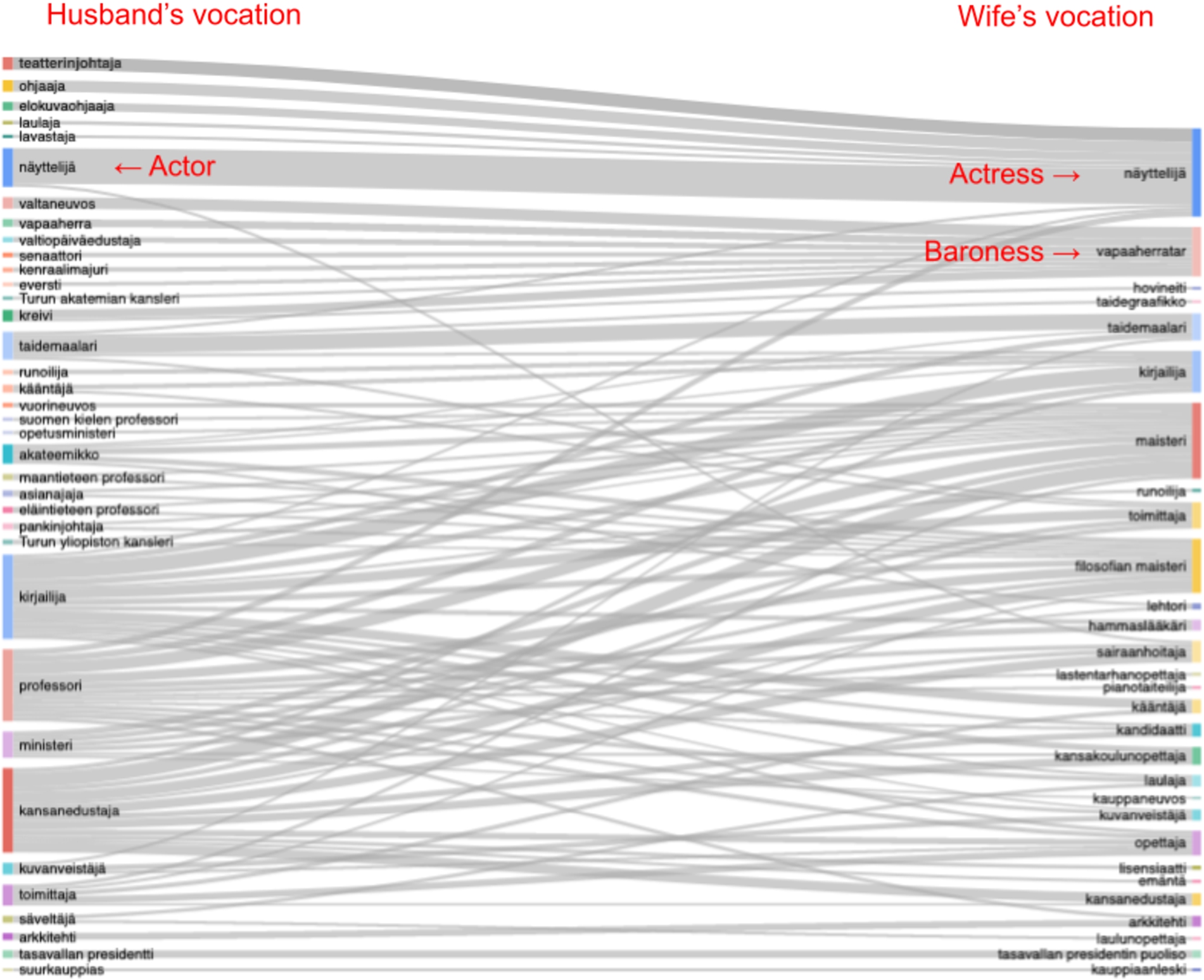
The BiographySampo portal allows users to generate statistical visualizations of correlations between, e.g., vocations or places of birth or death between biographees and their relatives. The Sankey diagram in Fig. 8 visualizes correlations between the vocations of spouses so that husbands’ vocations are on the left and their wives’ on the right. The visualization suggests, for example, that men having a vocation related to theater often have an actress (näyttelijä in Finnish) as a wife. However, a wife of men of nobility gets a title of a baroness (vapaaherratar in Finnish). On the other hand, in cases like a farmer the vocation of a wife is not mentioned in the data at all.
3.1.1.Vocations
The NBF dataset also contains the vocations of each biographee except for 116 people. In this article the terms vocation and vocational group are used instead of terms occupation and occupational group. The vocation term is used because the person data contains in addition to occupational titles also, for example, honorary titles, academic degrees, and ranks of the peerage.
The biographees were distributed into vocational groups already at the stage when the collection was being mapped out by the editorial board. They chose to use a fairly standardized vocational classification previously used by other research projects in the 1980’s, which was slightly modified to include all vocational groups in the NBF.
The use of vocational groups has a dual goal. On one hand they gave the editorial board a means to compose a diverse collection of biographies, and on the other hand they give the reader one more possibility to search the biographies. The vocational groups made it possible to take into account the different sectors and periods of Finnish history in selecting the biographees. The vocational groups are also useful as a search feature since they categorize the different titles (e.g., prime minister) to domains (e.g., politics).
Table 1 lists the 10 most common vocations for all, female and male biographees. The number in parentheses after the vocation indicates the number of occurrences. The list of the most common vocations for all and for men are similar but may have a different order of titles. The most common ones of these vocations appear for both female and male biographees. However, there are vocations which are more related to only one gender, like Lutheran minister and merchant for males, or actress and queen for females. The queen appears in the female vocations because the dataset contains all the historical rulers of Finland with their spouses.
Table 1
Most common vocations by gender
Rank | Female | Male | All |
1 | Author (139) | Professor (1106) | Director (1182) |
2 | Director (125) | Director (1057) | Professor (1169) |
3 | Teacher (95) | Minister (443) | Author (501) |
4 | Professor (63) | Author (362) | Minister (481) |
5 | Painter (54) | Reporter (306) | Reporter (355) |
6 | Reporter (49) | Painter (203) | Painter (257) |
7 | Actress (46) | Lutheran minister (154) | Teacher (234) |
8 | Queen (45) | Merchant (144) | Scholar (159) |
9 | Unknown (40) | Scholar (140) | Merchant (158) |
10 | Minister (38) | Teacher (139) | Lutheran minister (154) |
In addition to vocations, there are also vocational groups for each biographee in the data. The vocational groups categorize the different titles, such as director, to different domains. Figure 9 depicts the distribution of the most common vocational groups in the NBF. In this figure, the vocational domains have been grouped based on the vocational grouping in the data. For example, musicians, authors, and artists are considered to be in the group Culture whereas lawyers and judges are grouped to Juridiciary. However, many biographees have more than one vocation, and instead of selecting just one, they are all included in the visualization. The biographees have a maximum of 4 vocational groups and on average have 1.7 groups. For example, a person can be a judge and an author and is then included in both groups Juridiciary and Culture. The group Charitable and NGO consists of people working for charitable and non-governmental organizations (NGO) whereas Other contains marginal vocations, such as a member of the nobility, criminals, lovers, muses, fictional characters, and celebrities. The group Unknown is the proportion of biographees whose vocational group is unknown. The group of Rewarded is a heterogeneous group of people who have received a notable recognition for their work. This group was added into the list of vocational groups because it was a significant group of approximately 900 biographies. With all this in mind, based on the chart, the largest vocational groups within the NBF are Culture, Politics, Science, and Economics. From all the biographees, 50% of vocations belong to the four most popular groups. Similar visualization can be found from the ODNB [55] but vocational categories (areas of renown) differ.
Fig. 9.
Most common vocational groups in the NBF.
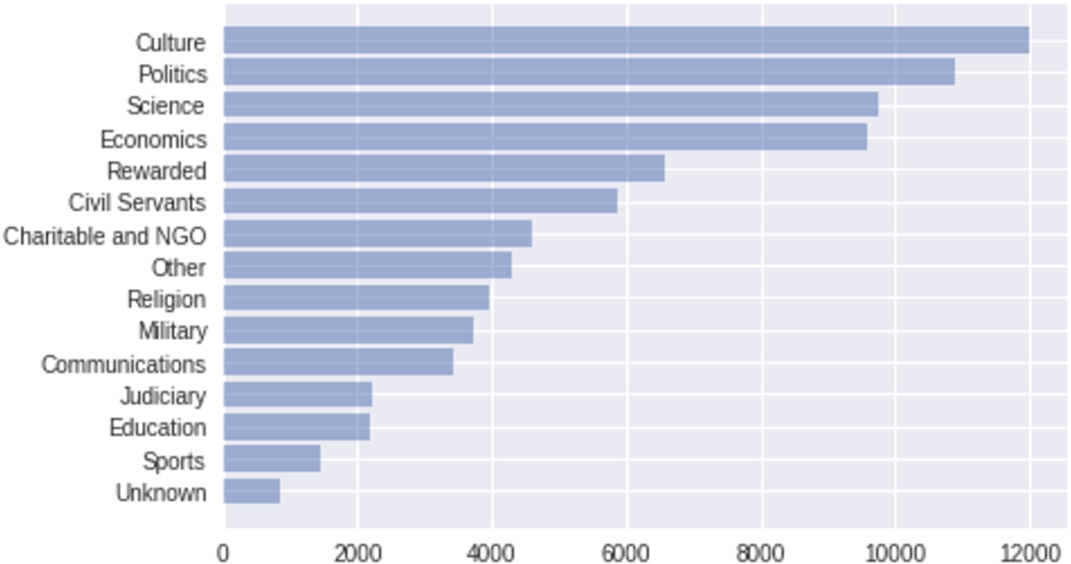
Fig. 10.
Correlations of the most common vocational groups.
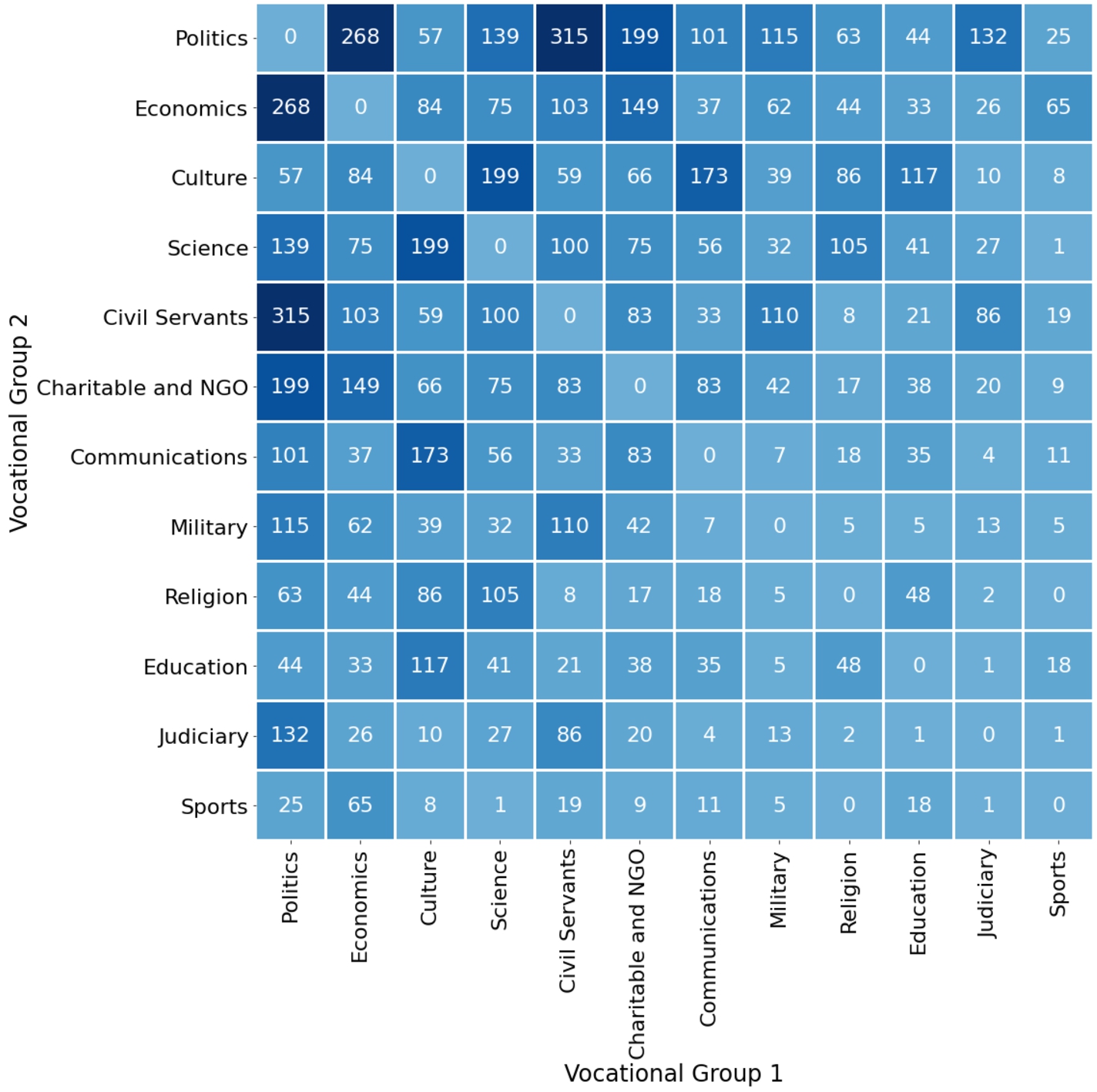
Fig. 11.
The most common vocations ranked on a timeline.
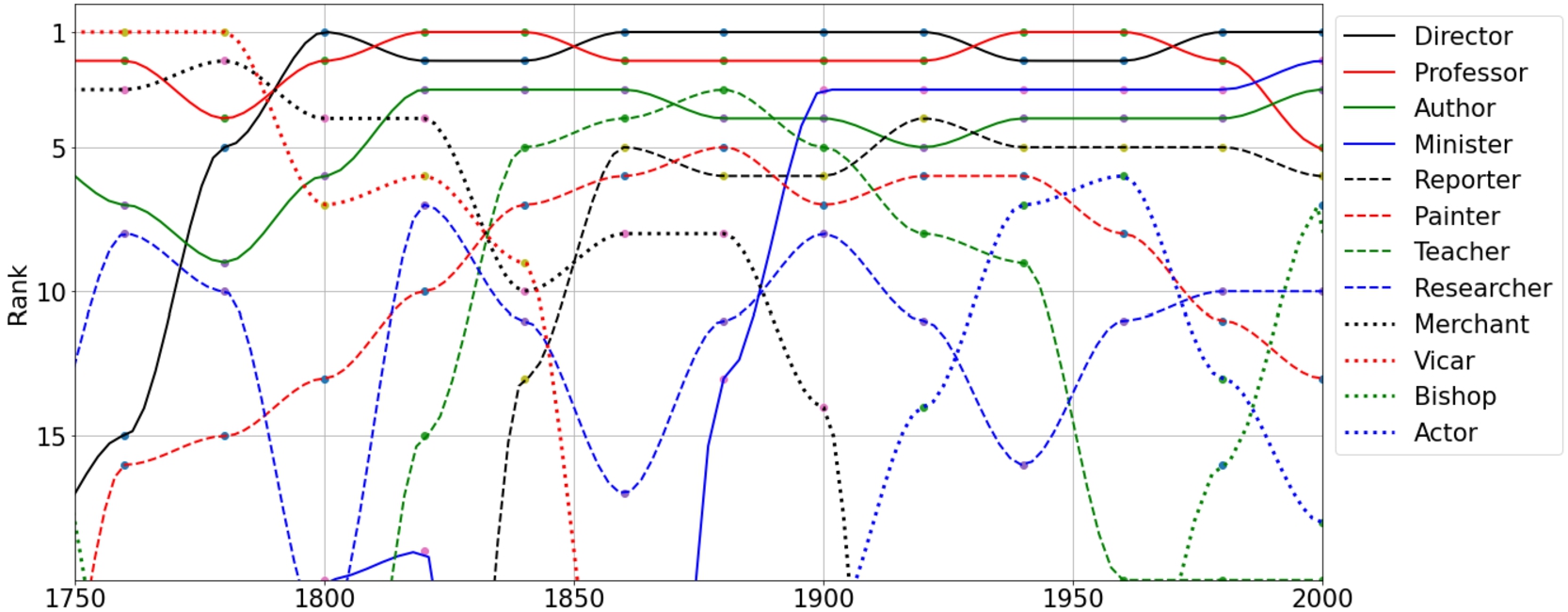
Fig. 12.
The most common vocations on a timeline.
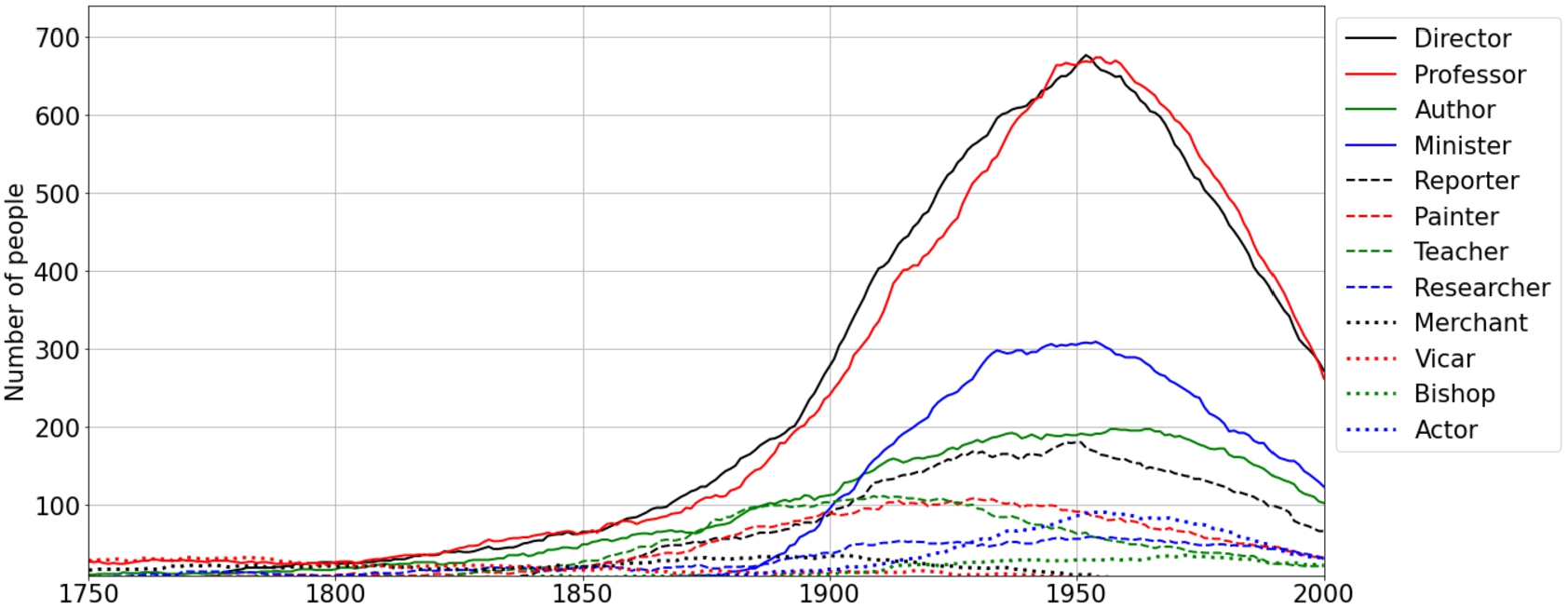
As mentioned earlier, a biographee can belong to more than one vocational group. The Fig. 10 depicts the most common intersecting vocational groups for a biographee who has more than one vocational group. For example, Field Marshal, president Gustaf Mannerheim (1867–1951)3939 was active in the military and politics. In this diagram the diagonal consists of zeros because one biography cannot have one vocation more than once. When looking at the other vocational combinations, it can be seen that the people grouped into the group Rewarded are often also in the field of business and economic life or culture. Similarly, politicians are also often civil servants or working in economics. However, athletes have a very low correlation with the fields of science, religion, and the judiciary.
In addition to looking at the most common vocations and vocational groups, there is also a difference in most common vocations as a function of time which is depicted in Fig. 11 and 12. Figure 11 shows the ranking of 12 of the most common vocations and Fig. 12 the total amount of people with these vocations. The figures show that some vocations, e.g., director, professor, or author have a constantly high rank throughout the timeline. On the other hand, vocations like minister or reporter start gaining a higher rank during the late 19th century. Actor gains its highest rank in the years 1930–50 and naturally there are no movie actors before the cinema was invented and brought to Finland. Furthermore, some vocations such as merchant or Lutheran minister descend in the rank in the 19th century.
3.1.2.Relatives and vocations
The biographies have 5410 mentions of a father and 5310 mentions of a mother. In 619 cases the father also has a biographical entry, 94 of the mothers have biographies. Generally, especially with earlier biographees it is common that the vocation of a mother is not mentioned. There are approx. 5850 mothers whose vocation remains unknown, while 1130 fathers are missing this information. As an observation, there are, e.g., 340 cases where the father is a farmer, and 256 cases where he is a Lutheran minister. In cases like this, one could assume that the mother has been a farmer’s wife, although it is not mentioned in the data entries.
Table 2 shows the 10 most common vocations of the biographees’ parents. Six different columns where chosen similarly as in [55]. In the table teacher, farmer’s wife, and nurse appear as the most common vocations of a mother, while farmer, director, and merchant as the most common of a father. On the other hand, some vocations of the biographees (Table 1) like minister, painter, or scholar do not appear in the parent data at all. Baroness and queen appear in the list of men’s mothers, indicating that among nobility, the mother often has a biography entry in the dataset in her own right. The bottom row shows the number of cases where the information about a parent’s vocation was not available.
Table 2
Most common vocations of parents by gender
Rank | Women’s Mothers | Men’s Mothers | Women’s Fathers | Men’s Fathers | Women’s Parents | Men’s Parents |
1 | Teacher (23) | Teacher (89) | Farmer (52) | Farmer (378) | Director (57) | Farmer (380) |
2 | Farmer’s wife (20) | Farmer’s wife (59) | Director (51) | Merchant (250) | Farmer (53) | Merchant (263) |
3 | Nurse (9) | Nurse (25) | Merchant (44) | Director (236) | Merchant (44) | Director (245) |
4 | Seamstress (8) | Master of Art/Science …(22) | Professor (35) | Lutheran minister (212) | Teacher (37) | Lutheran minister (214) |
5 | Director (6) | Baroness (21) | Lutheran minister (28) | Professor (161) | Professor (36) | Teacher (180) |
6 | Author (6) | Queen (16) | Proprietor (17) | Provost (124) | Lutheran minister (28) | Professor (164) |
7 | Master of Art/Science …(5) | Lecturer (teacher) (14) | Provost (16) | Landed Peasant (113) | Farmer’s wife (20) | Provost (124) |
8 | Actress (4) | Merchant (13) | Sea captain (14) | Teacher (91) | Proprietor (17) | Landed Peasant (123) |
9 | Servant (4) | Author (13) | Teacher (14) | Chaplain (88) | Reporter (17) | Chaplain (88) |
10 | Reporter (4) | Seamstress (12) | Blacksmith (13) | Blacksmith (83) | Nurse (16) | Blacksmith (83) |
Unknown | 655 | 3910 | 225 | 1195 | 880 | 5105 |
Fig. 13.
Correlations between the vocational groups of parents and children.
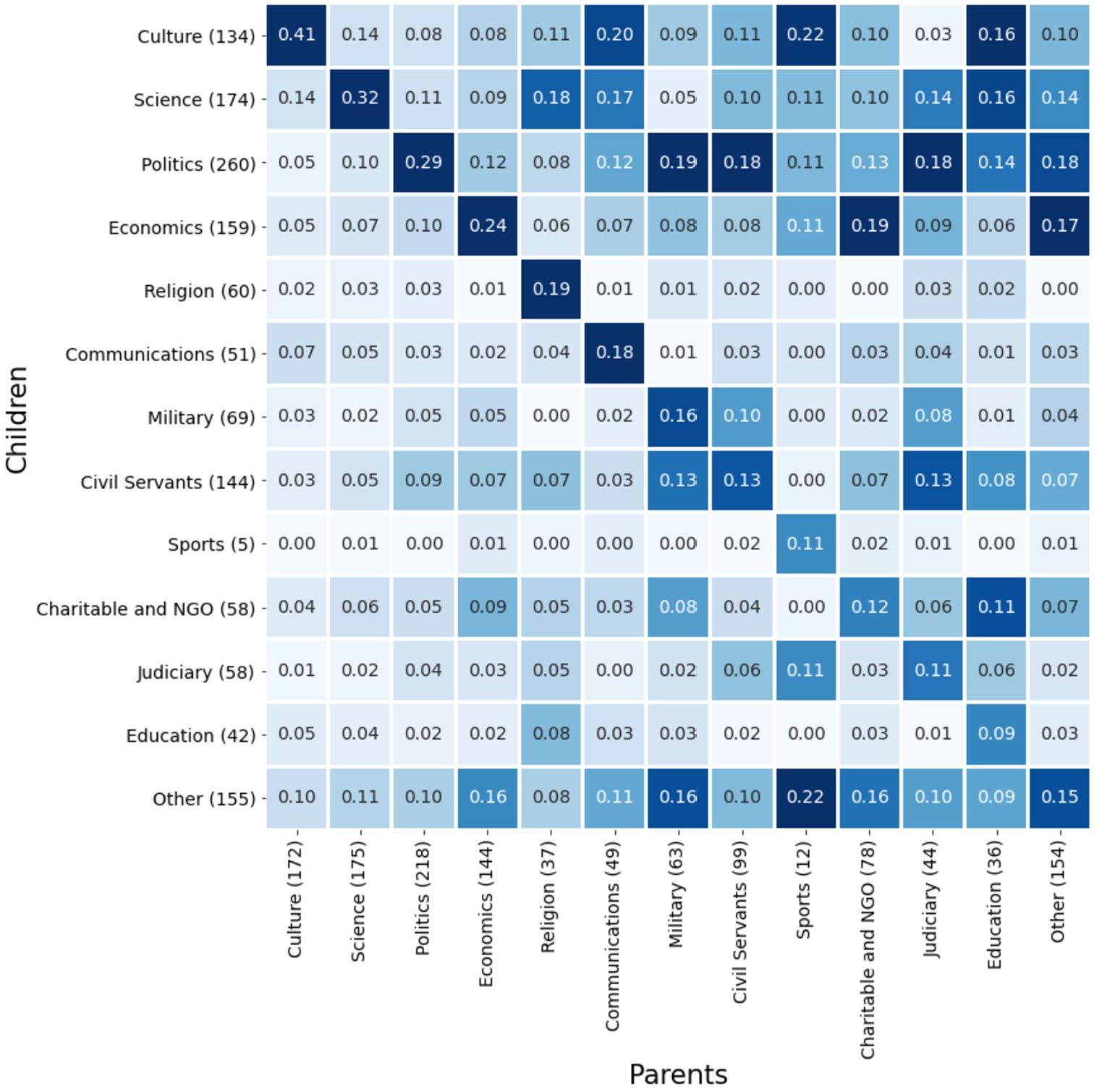
Figure 13 depicts the correlation between the vocational groups of a child and his/her parents. The horizontal rows correspond to the groups of a child while the vertical columns to the groups of a parent. The number of biographees in each group is in the parenthesis after the group label. The values in the cells are normalized so that the values in each column sum up to one. To wit, the cell indicates the conditional probability for the group of child when the group of parent is known. Due to the dominant values at the diagonal of the matrix, there is an obvious correlation between the groups of a parent and of a child. The strongest correlations are found in the groups of Culture, Politics, and Science. Notice also how the off-diagonal values within the three groups are relatively low indicating a low intercorrelation and that they remain separated from each other. It can also be noticed that although Agriculture was a significant source of livelihood in Finland until the 1960’s, the selection of biographies does not reflect that fact although many of the biographees came from farmer families.
3.2.Events
Events include the births and deaths converted from the structured CSV data, added with the lifetime events extracted from the semi-formal descriptions. An event usually contains a timespan and a possible reference to a place; we have extracted these mentions so that the event data can be illustrated on maps and timelines. The birth information was available for 6210 and death for 5800 out of the total of 6230 people. The semi-formal chapter of lifetime events was split into paragraphs describing the career, achievements (works, acknowledgments etc.), and a list of references. 5080 biographies contained a description of career and 3450 of achievements. Many of the people without a career description were historical figures of whom the records of education or vocations are not available. The data extraction generated 69400 events of career, 29900 events of achievement, and 18000 mentions of honor.
Fig. 14.
Timeline with the number of events.
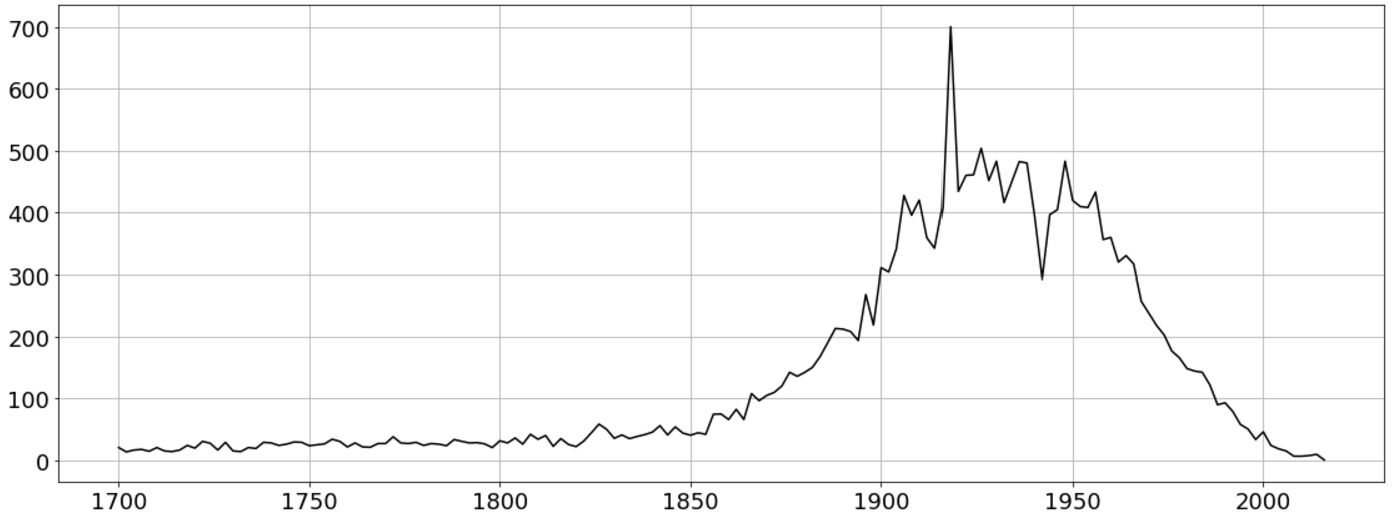
The timeline in Fig. 14 depicts the number of events by year, e.g., births, deaths, and events related to a person’s career. Generally the curve clearly follows the distribution of people alive shown in Fig. 3. The curve reaches the highest count around 1918, the time of the Russian revolution, of the beginning of Finland’s independence and the Finnish Civil War. On the other hand, the curve shows a downwards peak in 1942, during the Second World War. This decrease is explained by the missing events in people’s civil careers, although there are military personnel in the people data. Furthermore, before the decade 1850 the data is so sparse and major events of that time, e.g., wars or plague pandemics, do not form distinct peaks to the figure.
3.3.Lives on maps
Similarly to [55] we have ranked the ten most often mentioned places on a timeline in Fig. 15 but the illustration also contains names of towns and cities. The data was binned to intervals of 20 years. Helsinki became the capital of Finland in 1812 and has a constant highest ranking from the 1840’s onward. The chart also shows a strong connection to Sweden with even more events than with the former capital Turku. Paris has had a high ranking during the latter half of the 19th century when it was a popular location for, e.g., university studies. The United States started to gain attention in the early 20th century. This attraction peaked during the decades 1940–1960. The old Finnish city of Vyborg lost its significance after the Second World War when it was annexed by the Soviet Union.
Fig. 15.
Top 10 places on a timeline.
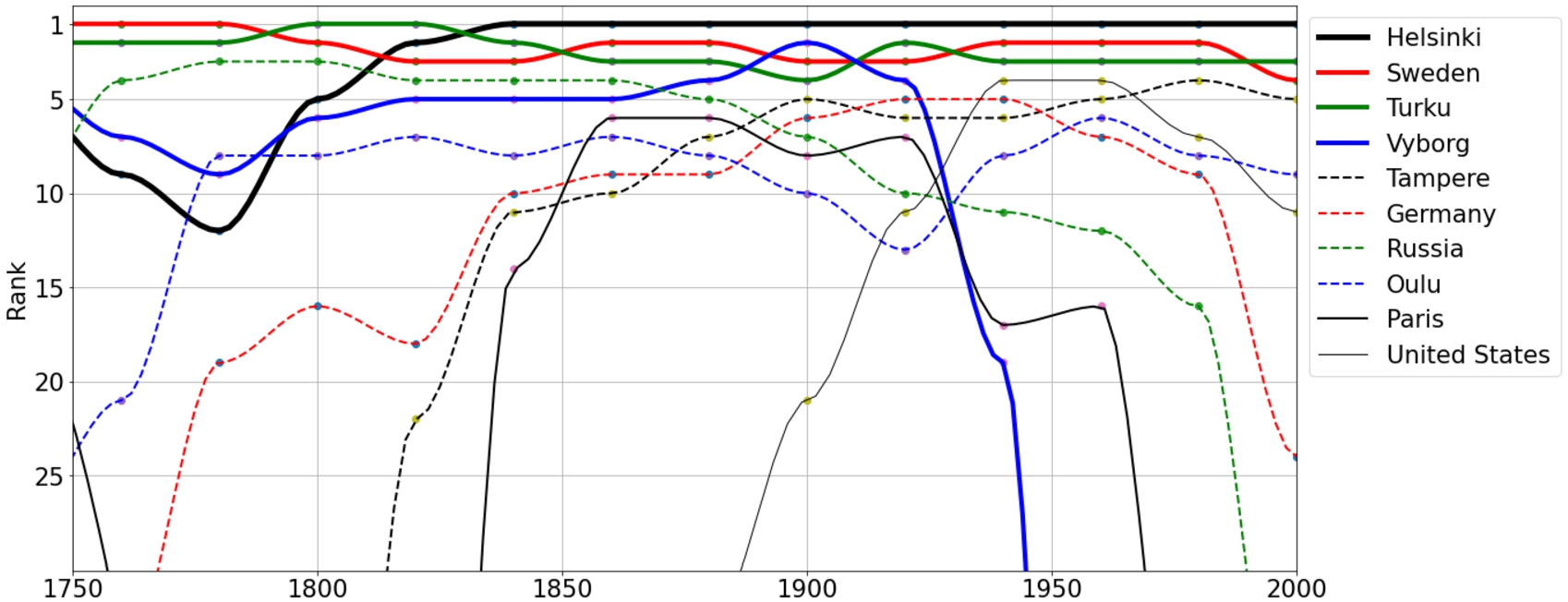
Figure 16 depicts a simplified illustration showing the referenced countries or continents. Generally biographees have had close connections to Sweden and Germany, and historically also to Russia, although it’s significance has decreased during the 20th century. The Baltic Countries have increased their ranking after gaining independence from the Soviet Union. The third position of the United States after the 1940’s is explained by, e.g., international studies. Africa has gained an increasing rank after 1960’s due to, e.g., activities of development aid organized by the United Nations.
Fig. 16.
Top 15 countries on a timeline.
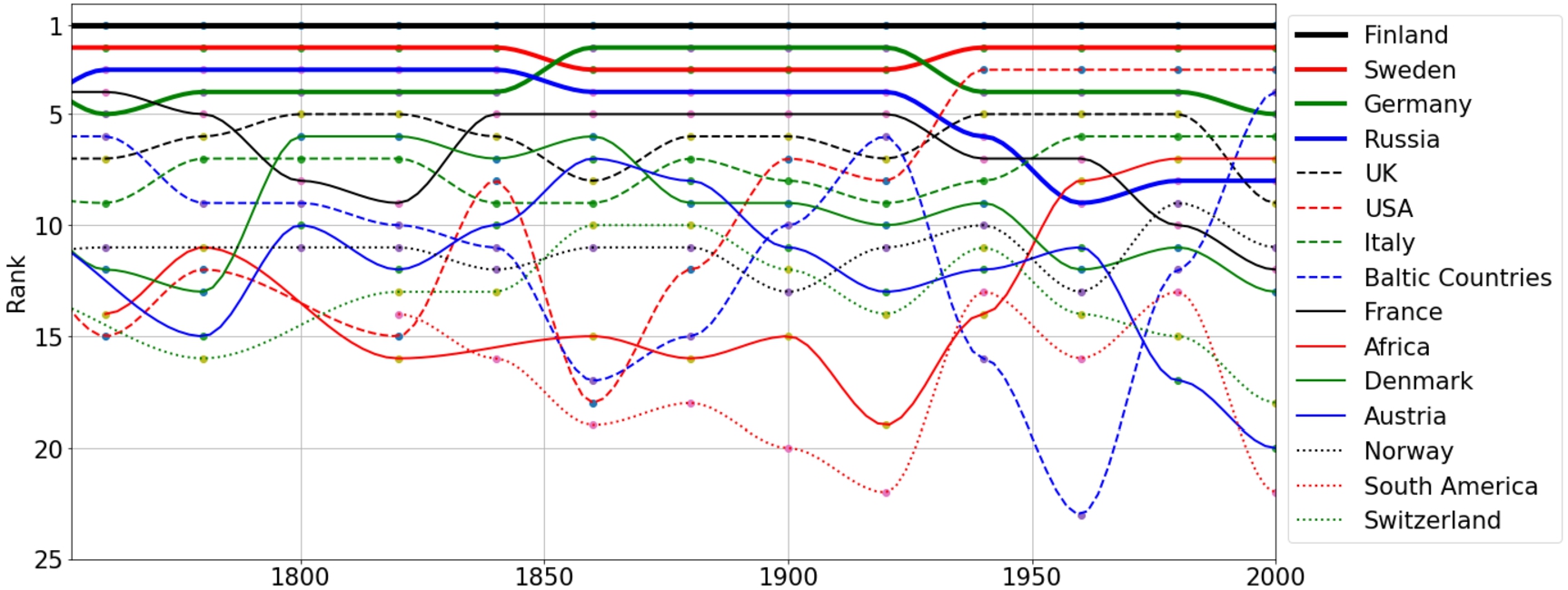
Fig. 17.
Comparing life maps of male (left) and female (right) biographees in the NBF in the BiographySampo portal.
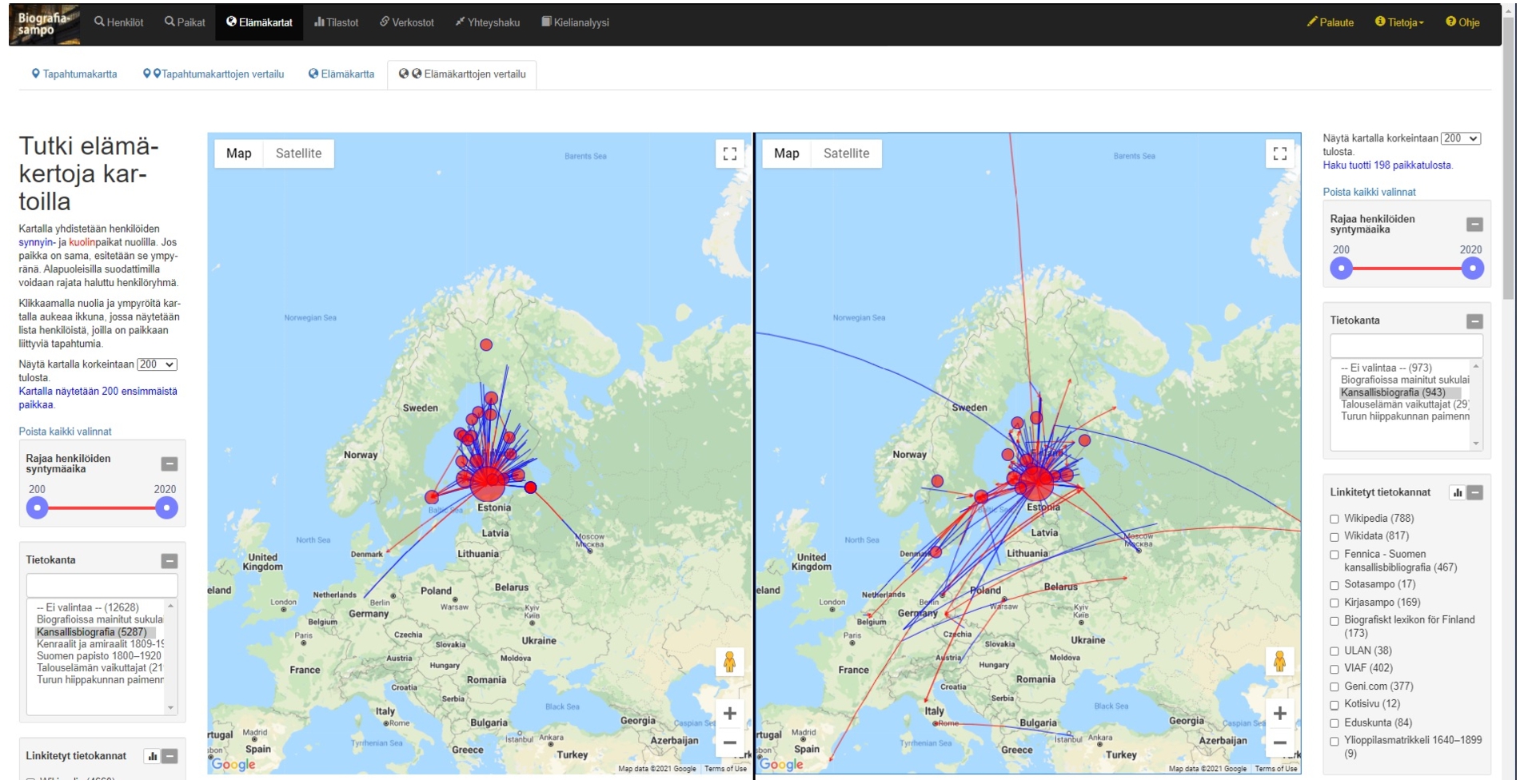
BiographySampo also provides the user with a map search view4040 in which the events extracted from the biographies are projected on the places where they occurred. After finding a place on the map, the place can be clicked. This opens a window showing the events with links to biographies. The maps in this view are not only contemporary ones but also historical maps served by the Finnish Ontology Service of Historical Places and Maps4141 [27], using a historical map service4242 based on geo-rectification and warping application Map Warper.4343 Many events of Finnish history took place in the eastern parts of the country that was annexed to the Soviet Union after the Second World War. Old Finnish places there may have been destroyed, place names have been changed, and are now written in Russian. Using semi-transparent digitized historical maps on top of contemporary maps solves the problem by giving a better historical context for the events.
There is also a Life Maps application perspective in the portal. This perspective contains two kinds of prosopographical tools: (1) Event maps show how different events (births, deaths, career events, artistic creation events, and accolades) that a target group of people participated in are distributed on maps. (2) Life charts summarize the lives of persons from a transitional perspective as blue-red arrows from the birth places (blue end) to the places of death (red end). The prosopographical tools and visualizations in BiographySampo can be applied not only to one target group but also to two parallel groups in order to compare them. For example, Fig. 17 compares the life charts of male (on the left) and female (on the right) biographees in the NBF. This visualization suggests, perhaps surprisingly, higher international mobility of the female biographees. The arrows are interactive for close reading. For example, by clicking on the peculiar arrow to the north on the right, one sees that the feminist, activist and politician Annie Furuhjelm (1859–1937) was born in Alaska. Both Finland and Alaska belonged to the Russian empire, and Annie Furuhjelms’s father Hampus Furuhjelm was the governor of Alaska.
3.4.Reference analysis and networks
Fig. 18.
Extract from the reference network.
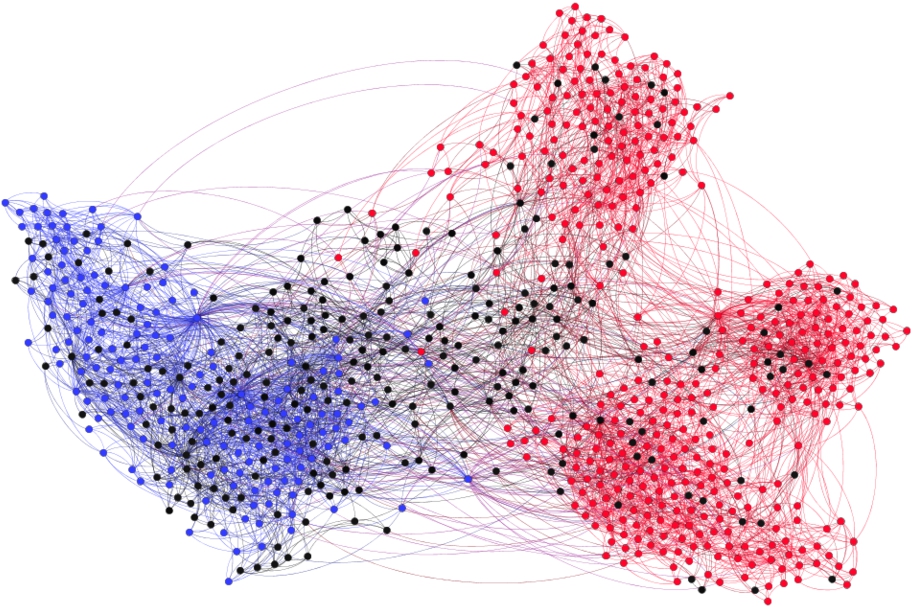
Based on the person data and extracted person references, the BiographySampo portal also contains network visualizations of people and how they are referenced in biographies. The networks enable the study of egocentric and socio-centric networks. In addition to using the BiographySampo portal, it is also possible to study the networks by using SPARQL queries to get the data. As an example, Fig. 18 depicts an extract around the vocational categories culture (marked with red) and politics (marked with blue) and black for other groups. The network is generated using the HTML links because of the coverage; currently the person references are extracted for people born in the 1900s. HTML links referenced people in different datasets of SKS and were made only for the first occurrence of a biographee’s name. The graph shows that the politicians form one solid cluster while the people who are grouped by their vocation to culture vocational group are divided into three smaller clusters, one representing literature, one classical music, and one popular culture, when the corresponding biographies are analyzed by close reading.
3.4.1.Reference analysis
Fig. 19.
Sentences that reference people.
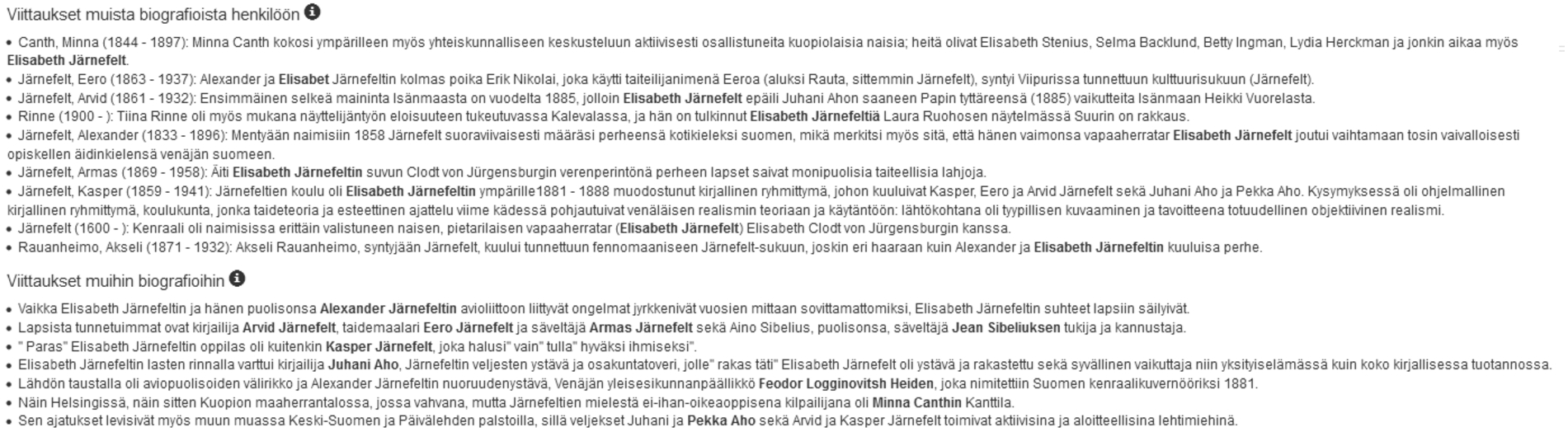
In addition to enabling browsing of the data via networks, the tools in BiographySampo also enable link analysis currently only for biographies with HTML links. For each person, there is a view4444 where one can browse the references made to the biographee and to other biographies. The sentences containing the references are available from the linguistic RDF data and can be viewed in BiographySampo. For example, Fig. 19 shows the sentences that mention (a) the biographee, here baroness Elisabeth Järnefelt (1839–1929),4545 in the other biographies, and (b) the other biographees who are mentioned in her biography. These references show how a biographee is discussed in other biography texts, and how biographees are referenced in this biography. This is useful, for example, when studying the links in the egocentric networks. For example, in the egocentric network of the poet Aale Tynni (1913–1997)4646 there is a reference to the javelin thrower and film actor Tapio Rautavaara (1915–1979),4747 which seems odd. However, in this case the link analysis view explains the serendipitous connection: Aale Tynni and Tapio Rautavaara won gold medals in the 1948 Summer Olympics of London and they traveled together to receive their rewards.
Fig. 20.
Plotting number of references by decade using the BiographySampo portal.
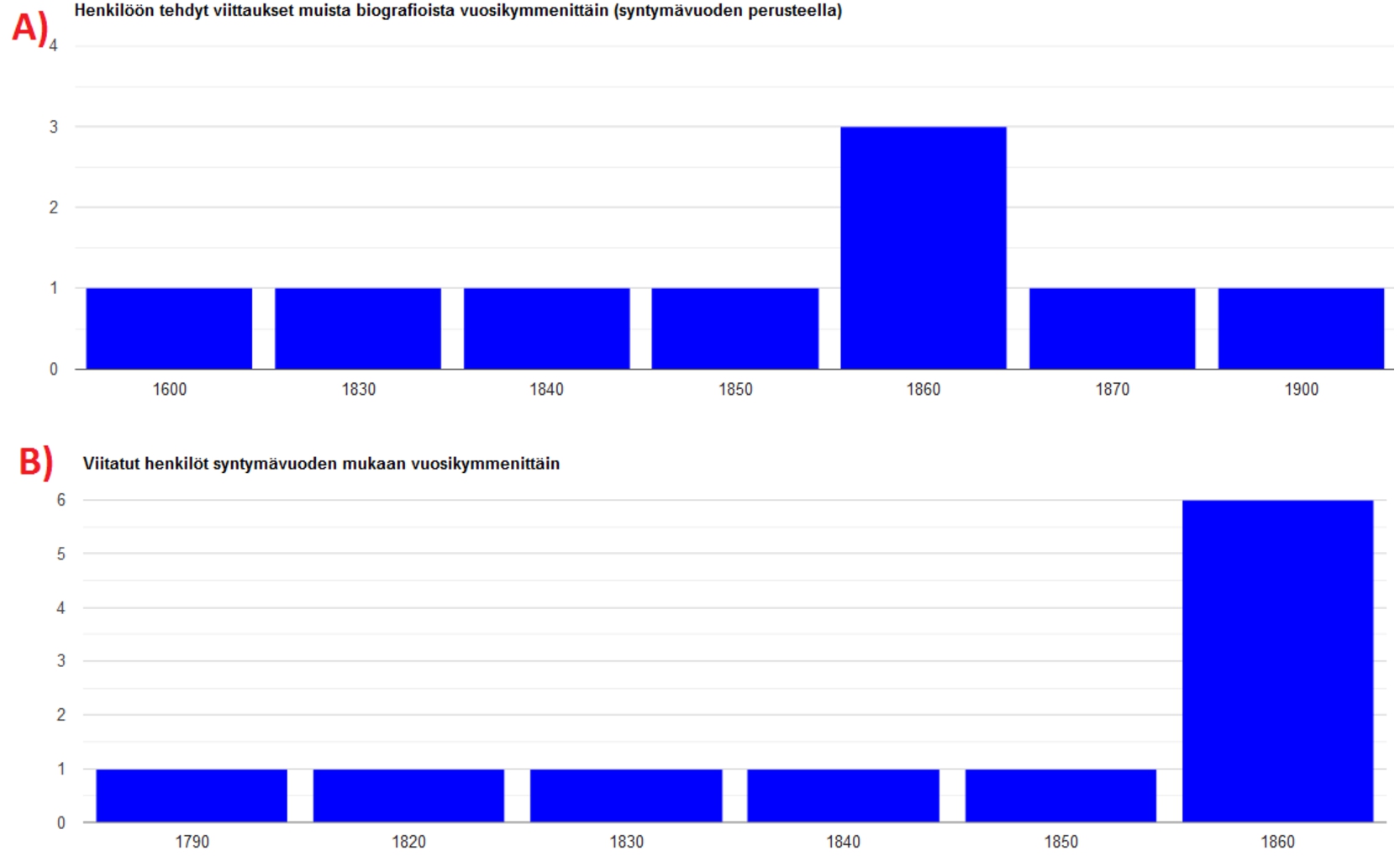
BiographySampo also contains a chart for each biography, where the links from the source biography to other target biographies are calculated based on the birth decade of the target. This is illustrated in Fig. 20, where the references of a source biographee and people referenced in the source’s biography are plotted by their decade of birth. These plots show (a) the influence of the source biographee by decade4848 and (b) the prominent figures4949 mentioned in the biography of the biographee. This chart shows when the biographee influenced others the most or vice versa when people influencing the biographee were born. For example, a notable playwright can be mentioned frequently throughout history if the person’s works are used by directors to recreate the scripts on stage or in movies.
In the BiographySampo portal there are no ready-to-use tools for counting references between biographies. In situations like this, one can use the data service SPARQL API directly to find out, for example, based on the HTML links who are the most often referred or “important” biographees. In Table 3 is the list of the top 10 people most commonly referred in the biographies of women. Whereas Table 4 is based on counting the references from the biographies of men. In addition to counting the references, the tables contain corresponding listings in the right column based on the PageRank centrality measure of the reference network. The PageRank measure and algorithm [3,4] was developed in Google to sort search results in a relevance order: the idea is to calculate the web pages’ importance recursively based on the number of times the page is referred to and the PageRank of the referencing nodes, which emphasizes the value of references from highly ranked pages. Using the PageRank method leads to quite different ranking orders from the counting based rankings.
Table 3
Top 10 referenced people in female biographies
Count | PageRank | |
1 | Author Zachris Topelius (1818–1898) | Author Zachris Topelius (1818–1898) |
2 | Author Johan Ludvig Runeberg (1804–1877) | Author Minna Canth (1844–1897) |
3 | President Urho Kekkonen (1900–1986) | Singer Laila Kinnunen (1939–2000) |
4 | Author Fredrika Runeberg (1807–1879) | Politician Miina Sillanpää (1866–1952) |
5 | Author Minna Canth (1844–1897) | Author Fredrika Runeberg (1807–1879) |
6 | Author Hilda Käkikoski (1864–1912) | Author Marja-Liisa Vartio (1924–1966) |
7 | President Gustaf Mannerheim (1867–1951) | President Urho Kekkonen (1900–1986) |
8 | Composer Jean Sibelius (1865–1957) | Sculptor Essi Renvall (1911–1979) |
9 | Painter Helene Schjerfbeck (1863–1946) | Author Annikki Kariniemi (1913–1984) |
10 | Painter Adolf von Becker (1831–1909) | Painter Venny Soldan-Brofeldt (1863–1945) |
The PageRank measures have been calculated using the NetworkX Python library5050 after extracting the group of biographies from the SPARQL endpoint. A weighted network of biographies was created and was used for calculating the weight of the edges based on how many times there was a reference to a particular biographee. The PageRank algorithm produces similar results to counting but the rank of a person changes. Women and therefore their networks are scarce causing the results between PageRank and counting the references to differ more. Women’s list consists mainly of cultural influencers while men’s have more politicians and rulers.
Table 4
Top 10 referenced people in male biographies
Count | PageRank | |
1 | President Gustaf Mannerheim (1867–1951) | President Urho Kekkonen (1900–1986) |
2 | President Urho Kekkonen (1900–1986) | President Gustaf Mannerheim (1867–1951) |
3 | President Juho Kusti Paasikivi (1870–1956) | King Gustav III of Sweden (1746–1792) |
4 | King Gustav III of Sweden (1746–1792) | President Juho Kusti Paasikivi (1870–1956) |
5 | Author Johan Ludvig Runeberg (1804–1877) | Author Johan Ludvig Runeberg (1804–1877) |
6 | Author Zachris Topelius (1818–1898) | Author Zachris Topelius (1818–1898) |
7 | Prime minister Väinö Tanner (1881–1966) | King Charles XII of Sweden (1682–1718) |
8 | King Charles XII of Sweden (1682–1718) | Prime minister Väinö Tanner (1881–1966) |
9 | Composer Jean Sibelius (1865–1957) | Composer Jean Sibelius (1865–1957) |
10 | President Kaarlo Juho Ståhlberg (1865–1952) | President Kaarlo Juho Ståhlberg (1865–1952) |
Table 5 depicts the people with the highest centrality measures during chosen periods in the history of Finland. The data was generated by first constructing the entire graph, and then filtering people related to each period and picking the ten people with the highest PageRank measures. The first column describes the years (–1809) when Finland was a part of Sweden. The first row under the header has the number of people during each period. Most of the people in the first column are monarchs of Russia or Sweden with Peter the Great, Emperor of Russian, on the first place and Empress Elizabeth on the second. Next, during the time in the second column (1809–1917) the Grand Duchy of Finland was an autonomous part of the Russian Empire. In contrast to the first column, the highly ranked people are not monarchs but prominent figures in Finnish culture and politics, such as the politician J.V. Snellman, and the poets and writers J. L. Runeberg and Z. Topelius. The third column covering the early years of the Finnish independence 1918–1944 contains mostly presidents and significant politicians of the era like the fourth column of years 1945–1994 between the Second War World and joining the European Union. One can, e.g., notice that presidents Paasikivi and Kekkonen as well as Field Marshal, president Mannerheim are present in both columns. In general, all the columns during the Finnish independence (1918–) are dominated by politicians.
Table 5
People with highest PageRank values during five historical periods
–1808 | 1809–1917 | 1918–1944 | 1945–1994 | 1995– | |
# of people | 1270 | 2519 | 2682 | 2623 | 910 |
1 | Emperor Peter the Great | Senator Johan V. Snellman | President Gustaf Mannerheim | President Urho Kekkonen | President Mauno Koivisto |
2 | Empress Elizabeth of Russia | Governor-general Nikolai I. Bobrikov | President Juho K. Paasikivi | President Juho K. Paasikivi | Politician Jörn Donner |
3 | King Gustav III of Sweden | Author Johan L. Runeberg | President Pehr E. Svinhufvud | Prime minister Väinö Tanner | Prime minister Paavo Lipponen |
4 | Empress Catherine the Great | Author Zachris Topelius | President Urho Kekkonen | President Mauno Koivisto | Prime minister Kalevi Sorsa |
5 | Emperor Peter III of Russia | Professor Elias Lönnrot | President Kaarlo J. Ståhlberg | President Gustaf Mannerheim | Politician Elisabeth Rehn |
6 | King Gustav I of Sweden | Politician Georg Z. Yrjö-Koskinen | Prime minister Väinö Tanner | Attorney general Olavi Honka | President Tarja Halonen |
7 | King Charles IX of Sweden | Politician Alexander Armfelt | Composer Jean Sibelius | Prime minister Karl-August Fagerholm | President Martti Ahtisaari |
8 | King Frederick I of Sweden | President Gustaf Mannerheim | Prime minister Aimo K. Cajander | Composer Jean Sibelius | Prime minister Harri Holkeri |
9 | Governor-general Per Brahe | Emperor Nikolai I of Russia | President Kyösti Kallio | Prime minister Vieno J. Sukselainen | Politician Paavo Väyrynen |
10 | Professor Henrik G. Porthan | Statesman Arseni A. Zakrewsky | Painter Akseli Gallen-Kallela | Prime minister Rafael Paasio | Author Bo Carpelan |
3.4.2.References by gender and between relatives
Out of the references from male biographies 93.3% refer to a male biography, whereas only 6.7% to a female biography. On the other hand, from the female biographies 28.2% refer to a female biography. The average amount of links in a biography is 4.18 and there is no significant difference between the genders.
The difference between the ages of linked biographees was also studied with the observation that on average the mentioned person is 6.18 years older than the biographee. However, for females the average is 8.93 years while for men 5.73. A histogram of age differences is depicted in Fig. 21, where the negative values refer to an older person. The histogram shows that the modes of female and male distributions are both around zero, indicating that all people have plenty of links to people of nearly the same age. On the other hand, females have more links to people who are 20–75 years older while men have more links to people who are 10–50 years older than they. These statistics where calculated by picking random samples of the same size from both genders in order to avoid the male dominating bias in the data. This observation may be partly explained by the more frequent mentions of relatives in female biographies.
Fig. 21.
Histogram of differences in age of linked biographees.
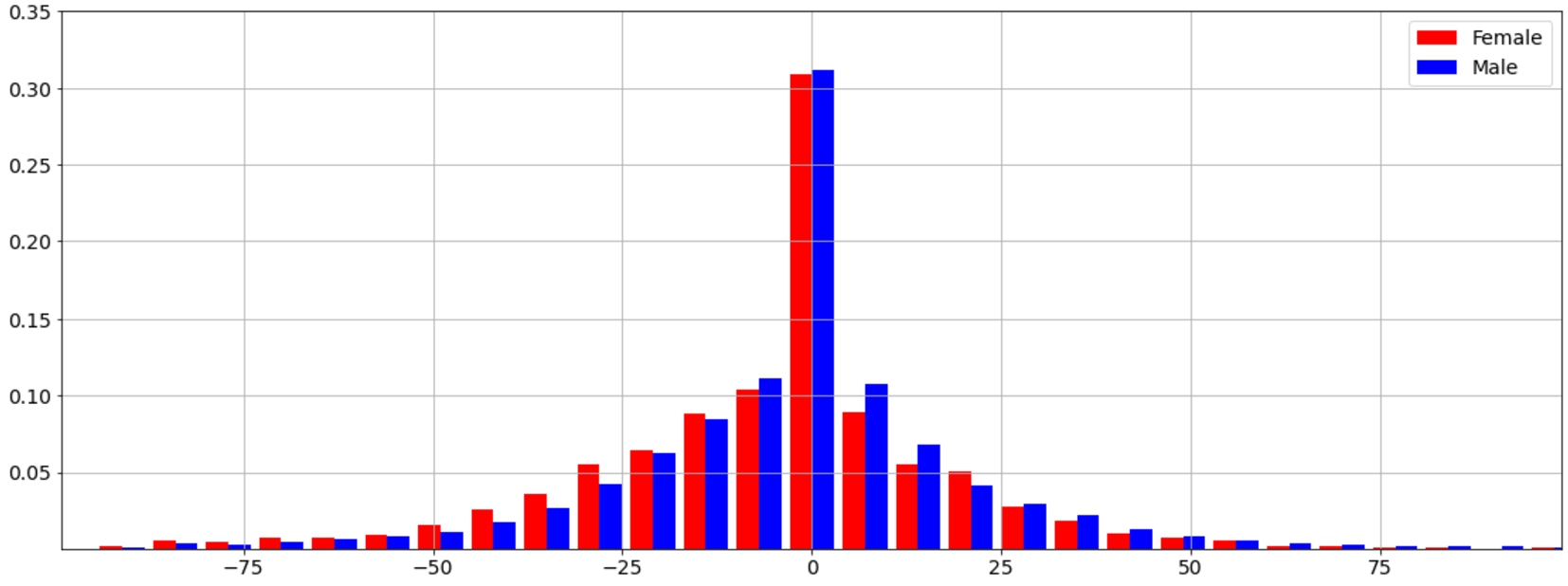
Table 6
Percentages of references to relatives by gender
Parent | Spouse | Child | Sibling | Other older relative | Other younger relative | Total | |
Female | 0.41 | 0.74 | 0.20 | 0.31 | 0.32 | 0.14 | 2.11% |
Male | 0.29 | 0.11 | 0.17 | 0.27 | 0.24 | 0.10 | 1.17% |
Table 6 shows the percentage of references between a biographee and his/her relative who is also a biographee. The studied relations are parents, spouses, children, siblings, and other relatives, e.g., cousins, grandparents and -children, or in-law-relatives. The table clearly indicates that females have in general more relatives in the dataset. Females have in average 2.11% of relatives mentioned in their biographies, while the corresponding value for men is 1.17%. Especially the spouse is mentioned in 0.74% of female biographies, while only in 0.11% of male biographies.
Figure 22 depicts the correlation between the vocational groups of two linked biographees. The numeric values of rows, columns, and cells follow the same principle as in Fig. 13. The strongest correlations are found in the groups of culture, politics, and science. These three major dominant groups also appear as separated from each other due to their low correlation. Groups like religion and athletes have plenty of references not only to these three major groups but also to themselves. On the other hand, these groups are rarely referenced from any other groups.
Fig. 22.
Correlations between the vocational groups of linked biographees.
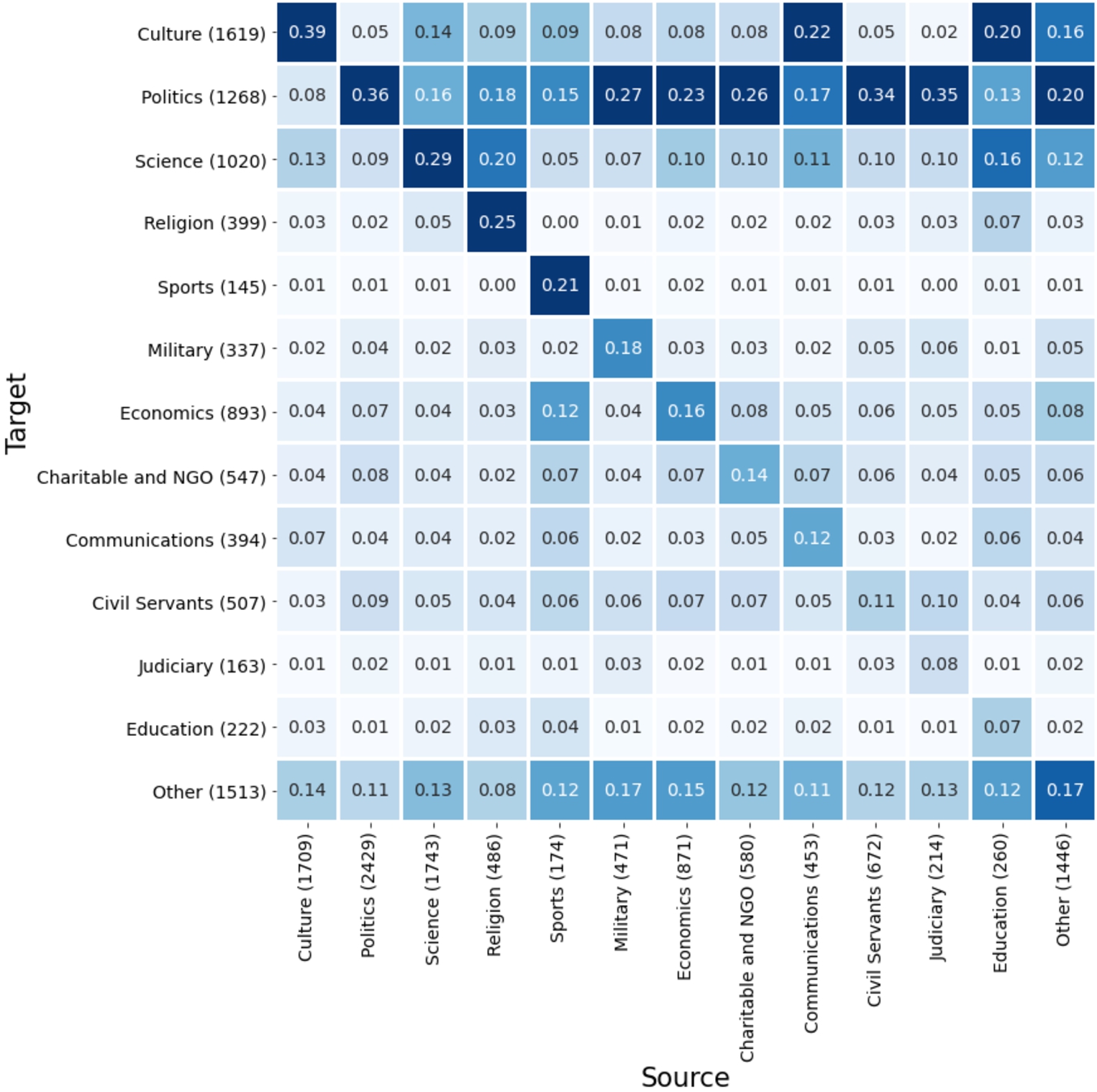
3.5.Network metrics
The data has been enriched by linking mentions of people in the biographies, complementing the existing HTML links in the source data. The F-score of the HTML links in the source dataset is 97.3%. The result was calculated for 181 links from 35 biographies sampled randomly from the dataset. In few cases some biographies had not linked people who had a biography (mainly because they were written before the linking could be made), and in a couple cases the links pointed to wrong people. Some biographies had no links to other biographies. Typically, the biographies of athletes had no links because they only mentioned people such as team mates or coaches. The biographies are rarely written about coaches or lesser known athletes. In 75.5% of the biographies of athletes contained links while other vocational groups had links in over 81% of biographies, 88.2% of female and 89.8% of male biographees had links.The automatically extracted links add missing relations between biographees in addition to mentions of people who don’t have biographies in the dataset. These automatically created links are used alongside the HTML links in the BiographySampo portal in a contextual reader application for the biographies and in reference networks.5151
Table 7 contains general metrics of the four networks, (1) manually linked HTML network, (2) automatically linked network, (3) the network linked both manually and automatically, and (4) the genealogical network. This table contains first the numbers of nodes and edges in the network. Average degree indicates the average amount of links for a single node and highest degree (HD) is the highest node degree in the network. Max clique size is the largest size of a clique, e.g., a value 8 indicates that there exists a subgroup of 8 people who all are linked to one another. The table shows the number of separated components in the network, and the size of the largest connected component. It is to be observed that the genealogical network is scattered into numerous separated components, while the three reference networks are all more connected having giant components connecting most of the data points. The Diameter is the number of edges along the longest path between any two nodes in the network. Alpha
Table 7
Comparison between the four networks in the BiographySampo data using standard network metrics
HTML links | Automatic | HTML + Automatic | Genealogical | |
Nodes | 5729 | 3247 | 5820 | 2487 |
Edges | 25013 | 12865 | 29464 | 3672 |
Average degree | 8.73 | 11.08 | 14.53 | 2.95 |
HD | 430 | 557 | 986 | 19 |
Max clique size | 8 | 9 | 9 | 10 |
Giant component | 5664 | 3170 | 5779 | 428 |
Number of components | 30 | 35 | 20 | 585 |
Diameter | 11 | 12 | 11 | 30 |
When comparing the results shown in Table 7 one has to remember how the automatic references complete the graph of HTML links which is clearly shown by the measures of nodes and edge counts, average and highest degree, and giant component size. The last example network, the genealogical network is completely different by its nature where the people are linked by family relations.
Table 8
Comparison between five example networks and reference networks of BiographySampo
Epinions | Wikipedia | Author | HTML | Automatic | HTML + Automatic | |||
Edges | 3099 | 13739 | 11672 | 2396 | 2404 | 2200 | 2678 | 2741 |
Density | 6.18 | 27.47 | 23.34 | 4.79 | 4.80 | 4.40 | 5.36 | 5.48 |
HD | 237 | 278 | 281 | 499 | 102 | 159 | 403 | 323 |
Diameter | 11 | 7 | 12 | 7 | 10 | 5 | 5 | 5 |
CCG | 0.19 | 0.43 | 0.35 | 0.54 | 0.60 | 0.36 | 0.34 | 0.35 |
APL | 2.60 | 1.93 | 2.10 | 1.98 | 2.87 | 2.88 | 2.74 | 2.76 |
α | 1.57 | 1.20 | 1.21 | 1.87 | 1.66 | 1.45 | 1.42 | 1.43 |
Hashmi et al. [11] used a random sampling strategy for calculating the network measures in their study for structural similarity of social, communication, or collaboration networks. The example networks in their study are Twitter Friendship Network, Epinions Social Network, Wikipedia Vote Network, EU Email Communication Network, and Author Network. Their sampling strategy was to sample subgraphs of the size of 500 nodes with a breadth-first search and then calculate the values as average of ten such samples. Table 8 shows our reference networks in comparison with the five example networks analysed by Hashmi et al. where we used the same strategy to calculate the metrics. Comparing the values to their results shows that, e.g., the number of edges and therefore also the densities in our reference networks are in the same range as in Email and Author networks. Also the values indicating a small world or scale free behavior, e.g., CCG and α are in the same range as in the comparison networks. The smaller diameter in networks of BiographySampo can be explain by the degree distribution, approx. 75% of the nodes have a degree in the range 1 to 10.
3.6.Text analysis
Fig. 23.
Amount of words in biographies by decade; screenshot from the BiographySampo portal.
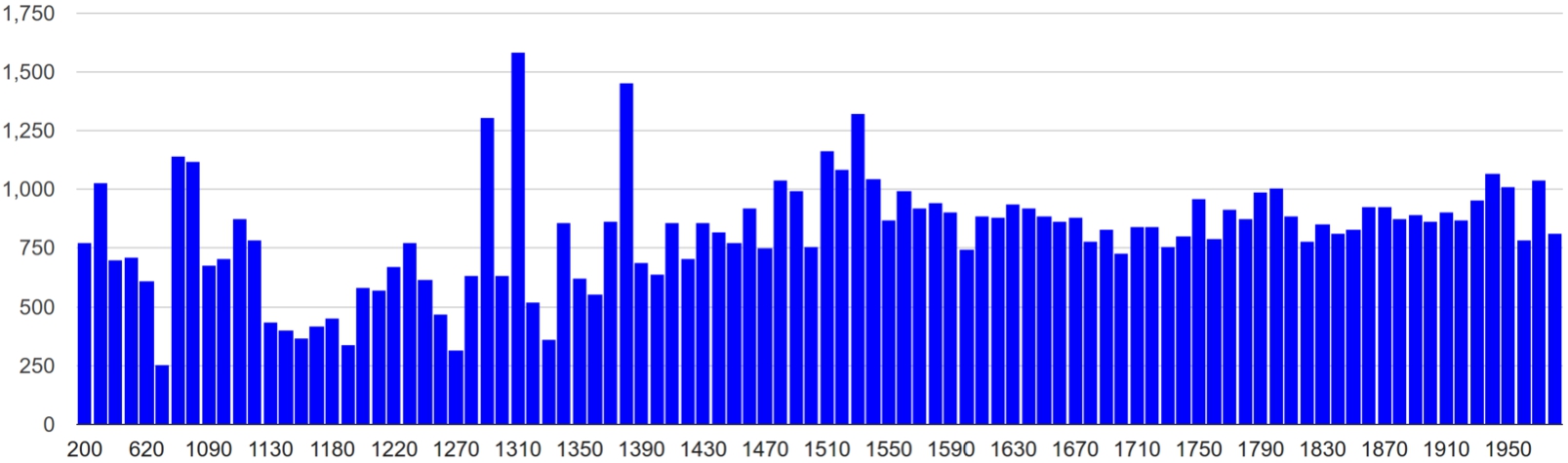
The biographies in BiographySampo can also be studied from a linguistic perspective in the Language Analysis view5252 of the portal. The Language view uses the linguistic knowledge graph to enable quantitative analysis of the biographical texts. Figure 23 shows in one of the plots in BiographySampo’s Language view the average word count of biographies by decade. The histogram tells the typical length of biographies in different times based on the decade when the biographees were alive. This plot shows that the biographies of earlier people are somewhat shorter than the biographies concerning the 15th century, often due to the lack of data sources. However, when comparing this plot to the earlier distribution of the number of biographies by decade in Fig. 1, it can be seen that until the 19th century there are fewer biographies. This indicates that there may be a few longer biographies that distort the distribution of Fig. 23. For example, in the 16th century the biography of Mikael Agricola (1510–1557), a bishop who translated the New Testament into Finnish and developed Finnish into a written language, is several pages long whereas typical biographies of that time were only a page or two long, and in total there are approximately a little over 80 biographies. When looking at the number of biographies concerning the late 19th century, there are typically 500 biographies at the peak of the top decades.
In addition to the general statistics about the word count by decade, the user can get a list of the biographies with highest and lowest word counts. In Table 9, the top 10 of the longest and shortest biographies are listed based on their word counts. In the Table 9(a) of the longest biographies, the list mainly consists of politicians, presidents, and regents of Finland with one exception, Mikael Agricola. In Table 9(b) of the shortest biographies, there are people with different vocations, such as a local government official, two artists, a lesser known ruler, an athlete, and a priest. Most of the people in the list of the longest biographies are people who were in power or active during and after the World War II, such as president Urho Kekkonen. In the list of the shortest biographies, there are people who have been active in the Middle Ages or in the 18th and early 19th century.
Table 9
Longest and shortest biographies
(a) Longest texts | (b) Shortest texts | ||
Biography | Words | Biography | Words |
President Mauno Koivisto (1923–2017) | 5369 | Castle overseer Bengt Mårteninpoika (1442–1451) | 174 |
President Gustaf Mannerheim (1867–1951) | 4855 | Lutheran minister Georg Stolpe (1778–1852) | 174 |
Politician Otto Wille Kuusinen (1881–1964) | 4717 | Bear hunter Per Huuskoinen (1732–1823) | 174 |
Senator Johan Vilhelm Snellman (1806–1881) | 4656 | Lithographer Johan Henric Strömer (1807–1904) | 177 |
Prime minister Kalevi Sorsa (1930–2004) | 4579 | Painter Fridolf Weurlander (1851–1900) | 177 |
Prime minister Edwin Linkomies (1894–1963) | 4543 | Writer Carl Fredrik von Burghausen (1811–1844) | 180 |
Prime minister Rafael Paasio (1903–1980) | 4462 | King Kol of Sweden (?–1173) | 197 |
Bishop Mikael Agricola (1510–1557) | 4171 | Mason master Petrus Murator de Kymitto (1466) | 199 |
Queen Christina of Sweden (1626–1689) | 4130 | Athlete Albin Stenroos (1889–1971) | 201 |
President Urho Kekkonen (1900–1986) | 4075 | Demagogue Filippus (mentioned 1438) | 205 |
In Table 10 the top 10 vocations that have the highest and lowest average word count in biographies are listed based on their word counts and on the number of biographies in the group. In Table 10(a) of vocations with the highest average word count, the list consists mainly of vocations that dominated also the list of biographees with the longest biographies by word count. The list’s first group of the longest biographies has only 7 biographies by different authors and is about the lovers, muses, and favorites of politicians, artists, nobility, and military personnel who lived before the Finnish Independence. The other groups contain more biographies and have lower average word counts. In contrast, in the Table 9(b) lists the vocations with the shortest biographies (the lowest average word count). There are vocations, such as artisans, athletes, families, clergy, and government administrative officials. Some of these were found also on the list of the shortest biographies. The vocational group with the shortest biographies is athletes followed by artisans and judicial authorities.
Table 10
Top 10 longest and shortest texts by vocation
(a) Longest texts: average word count by vocation | (b) Shortest texts: average word count by vocation | ||||
Vocational group | Word count | Count | Vocational group | Word count | Count |
Favourites, muses, lovers | 1377 | 7 | Athletes | 684 | 153 |
Rulers and heads-of-state | 1245 | 155 | Artisans | 696 | 80 |
Administration (scientific communities) | 1218 | 154 | Judicial authorities | 702 | 264 |
Theology | 1088 | 87 | Lawyers | 728 | 59 |
Organizations, institutions | 1081 | 30 | Families | 734 | 269 |
Social sciences | 1052 | 73 | Local governments | 746 | 151 |
Politicians, activists | 1049 | 308 | Catholics | 761 | 93 |
Humanistic sciences | 1048 | 396 | Agriculture and forestry | 774 | 248 |
Education and Cultural Work | 1041 | 27 | Regional administration | 776 | 277 |
Nobility | 1007 | 141 | Trade, transport | 786 | 384 |
In addition to word counts, the actual words and their frequencies can be listed for a filtered set of biographies. Table 11 lists the most common words (nouns, adjectives, and proper nouns) and the most common keywords for the whole NBF. The list of adjectives (Table 11(c)) contains common adjectives such as Finnish, new, first, great. These lists become more descriptive after the most common stop words are ignored. In the Table 11(a), the most common keywords are listed for the biographies and the number of times they appear (in column Count) in different biographies. The keywords have been extracted using the basic TF-IDF method from the nouns in the biographies. As can be seen from the table, this method typically picks up titles and other attributes related to the people described in the biographical texts, such as professors, kings, or women. In comparison, Table 11(b) lists the most common nouns in the biographies, containing similar words as in the keyword listing but in singular form (e.g., university and professor). However, these nouns constitute roughly 0.6% or less of the nouns and 0.2% or less of all the words in the dataset. All the keywords in the top 10 list can be found by looking at the top 50 nouns list.
Table 11
Top 10 words and keywords in BiographySampo
(a) Top keywords | (b) Top nouns | (c) Top adjectives | ||||||
Keyword | English | Count | Noun | English | Count | Adjective | English | Count |
Professorit | Professors | 536 | Vuosi | Year | 30770 | Suomalainen | Finnish | 13381 |
Kuninkaat | Kings | 427 | Aika | Time | 19328 | Uusi | New | 11405 |
Yliopistot | Universities | 371 | Puheenjohtaja | Chairman | 12655 | Ensimmäinen | First | 11344 |
Puolueet | Political parties | 370 | Jäsen | Member | 11577 | Suuri | Great | 10112 |
Teokset | Works | 312 | Yliopisto | University | 11391 | Oma | Own | 8410 |
Naiset | Women | 283 | Lapsi | Child | 9709 | Vanha | Old | 5939 |
Sukulaiset | Relatives | 267 | Professori | Professor | 8709 | Nuori | Young | 5614 |
Piispat | Bishops | 256 | Hallitus | Government | 8345 | Merkittävä | Notable | 4912 |
Kirjailijat | Writers | 246 | Poika | Boy | 8216 | Hyvä | Good | 4888 |
Tutkimus | Research | 240 | Historia | History | 7250 | Usea | Several | 4590 |
Table 12
Top ten words used in the biographies of female politicians
NOUN | ADJ | |||||
Finnish | English | Count | Finnish | English | Count | |
1 | Nainen | Woman | 557 | Poliittinen | Political | 303 |
2 | Kuningatar | Queen | 459 | Vanha | Old | 169 |
3 | Puolue | Political party | 456 | Nuori | Young | 162 |
4 | Kuningas | King | 422 | Seuraava | Next | 156 |
5 | Lapsi | Child | 378 | Suomalainen | Finnish | 154 |
6 | Puoliso | Spouse | 317 | Yhteiskunnallinen | Societal | 122 |
7 | Eduskunta | Parliament | 314 | Merkittävä | Significant | 109 |
8 | Poika | Son | 283 | Sosiaalidemokraattinen | Socialdemokratic | 100 |
9 | Äiti | Mother | 283 | Tärkeä | Important | 97 |
10 | Puheenjohtaja | Chairperson | 278 | Kansainvälinen | International | 94 |
As mentioned earlier, the user can select using facets any selection of the given data for inspection. As an example, we have selected the most common words used in the biographies of male and female politicians (e.g., MPs, presidents, ministers, rulers, and other political influencers in Finnish history). In Table 12 and Table 13 are the lists of the top ten nouns and adjectives for female and male politicians in BiographySampo. The table contains list of words for each group and the word count for the given word. Both lists have been created by querying from the biographical texts the top words of each part-of-speech group and filtering out most common words using a Finnish stop word list.5353 Both lists consist of mainly the same words but with some differences. In the female politician’s list of nouns, the words for family life, such as spouse, son, daughter, and mother occur much more often whereas in the list of male politician’s, nouns related to career, such as chairperson, post, and president are emphasized. The list of adjectives have similar words but with slight differences in order. However, when looking at lists generated to contain words that only exist in either biographies of male or female politicians, for example, in lists of nouns and adjectives, themes are highlighted. Both groups have many terms that describe politics and career. But female politicians have a significant amount of nouns and adjectives that are related to family themes. Respectively, male politicians have a higher number of nouns and adjectives that describe economics, war, and religion.
Table 13
Top ten words used in the biographies of male politicians
NOUN | ADJ | |||||
Finnish | English | Count | Finnish | English | Count | |
1 | Hallitus | Government | 4066 | Poliittinen | Political | 2493 |
2 | Puolue | Political party | 3766 | Suomalainen | Finnish | 1453 |
3 | Tehtävä | Task | 2725 | Merkittävä | Significant | 1108 |
4 | Puheenjohtaja | Chairperson | 2649 | Tärkeä | Important | 1093 |
5 | Jäsen | Member | 2460 | Vanha | Old | 1078 |
6 | Kuningas | King | 1845 | Keskeinen | Central | 995 |
7 | Toiminta | Action | 1840 | Nuori | Young | 985 |
8 | Eduskunta | Parliament | 1786 | Seuraava | Next | 983 |
9 | Sota | War | 1742 | Sanottu | So called or said | 693 |
10 | Presidentti | President | 1718 | Yhteiskunnallinen | Societal | 646 |
3.7.Author analysis
In BiographySampo’s dataset there are not only data about the biographees and their relatives but also about the authors of the biographical texts and their publishing dates. In this section statistics about the articles and their authors presented based on SPARQL queries to the data service.
The authors were chosen by the editorial board based on their expertise and previous research. Precedence was given to researchers who had recently published on the person in question or who had a deep knowledge of a specific field or period of history. The whole group of authors, more than 900 Finnish scholars, is so large and varied that it is very difficult to scrutinize them, especially because they come from so many fields of research. In addition to historians, they are specialists in various fields, e.g., art studies, jurisprudence, and medicine. The majority had a doctoral degree and a university affiliation. It is a group that can’t be easily analyzed, since the information in the editorial database only includes their title and date of birth but not the affiliation or the field of study.
The authors had to undertake to follow the guidelines and goals of the NBF, set by the editorial board. All articles were peer reviewed before being accepted for publication.
Fig. 24.
Number of articles written yearly in total.
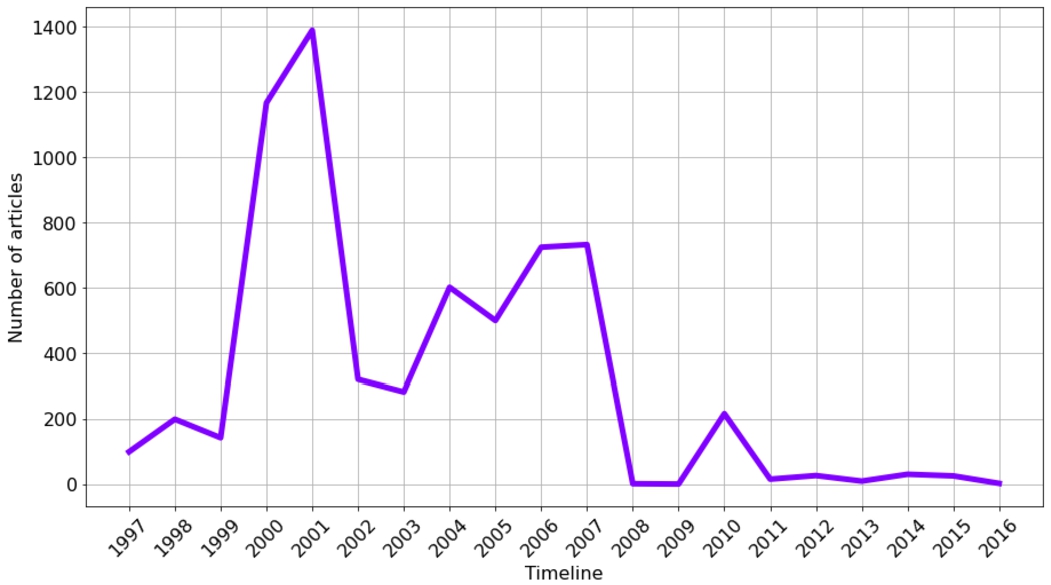
Since the publication of the NBF in print from 2003 to 2007, only 400 new biographies have been published. These newer articles were written thematically including biographies or people in different minorities, politicians, authors, actors and actresses, movie makers, theater directors, music educators, circus performers, and cartoonists.
The distribution of the number of articles published yearly can be seen in Fig. 24. The figure shows how the articles have been published from 1997 onward until 2016 (the most recent articles are not included in the BiographySampo). The figure has peaks before 2008 (the end of the publishing in print) and afterwards a minor peak in 2010 when a collection of new articles called the Multifaceted Finland was published online. Figure 25 depicts the distribution of how old the authors were when publishing biographies. The distribution also shows the difference between male and female authors.
Fig. 25.
Author age distribution.
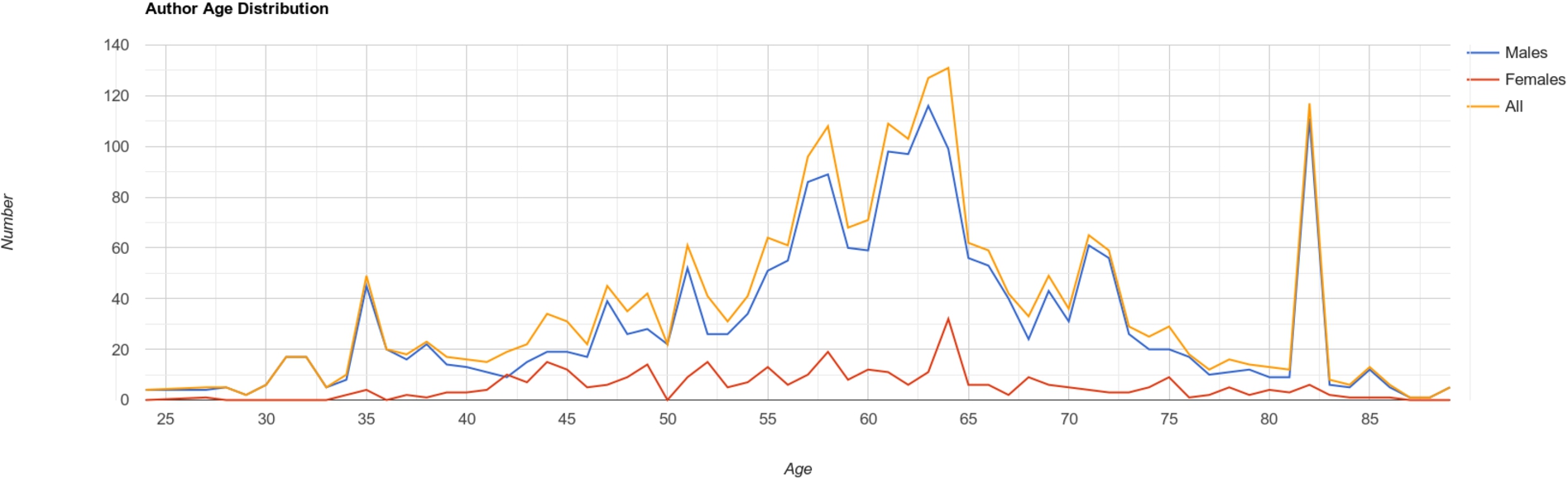
Statistics about male and female authors of the biographies can be seen in Table 14, indicating also the gender of biographees they write about. The fraction of female writers is 32% of all writers in the dataset; the male writers dominate (68%) this dataset. There are three authors whose gender is unclear in the data, but they have written only 90 articles (approximately 1% of the articles). On closer inspection on whom the authors write about, it can be seen that men write mainly about men (94%) and women write about both genders. 41% of the female authors have so far written only about men and 26% about only women, while 5.7% of male authors write only about women.
Table 14
Breakdown of articles written by men and women
Gender | Women | Men |
Writers | 31.7% | 68.0% |
Articles | 29.5% | 69.1% |
Write about women | 39.1% | 5.68% |
Write about men | 60.9% | 94.3% |
Only write about women | 25.6% | 4.52% |
Only write about men | 41.2% | 79.5% |
Write about both | 33.2% | 16.0% |
Table 15 indicates that the female authors have written more often about people who are known influencers of culture, rewarded individuals, or people active in charitable or non-governmental organizations. In contrast to this, the male writers have mainly written about prominent politicians, scientists, or economical influencers. According to the editorial policies of the NBF, the authors have not chosen their target biographees freely but were asked by the editors to write about particular people. The authors were selected based on what was known to be their areas of expertise.
Table 15
Most popular vocational groups of biographees for female and male authors
Women | Men | |||||
Vocational group | Percentage | Count | Vocational group | Percentage | Count | |
1 | Culture | 42.6% | 766 | Politics | 75.5% | 1232 |
2 | Politics | 24.4% | 398 | Science | 72.8% | 1065 |
3 | Economics | 25.4% | 365 | Economics | 73.3% | 1053 |
4 | Science | 24.8% | 363 | Culture | 54.1% | 972 |
5 | Rewarded | 27.3% | 269 | Civil servants | 81.7% | 720 |
6 | Charitable and NGO | 27.3% | 188 | Rewarded | 72.0% | 710 |
7 | Education | 55.3% | 183 | Other | 80.6% | 518 |
8 | Religion | 28.3% | 168 | Military | 90.0% | 505 |
9 | Civil servants | 17.6% | 155 | Charitable and NGO | 72.3% | 498 |
10 | Communications | 23.8% | 122 | Religion | 71.6% | 425 |
4.Discussion
BiographySampo offers historians and the public data analytic tools that can be used for biographical and prosopographical research without experience in computer science by using the portal. With a little experience in formulating SPARQL queries and/or Python programming, the underlying SPARQL endpoint can be used for custom-made complex data analyses. In this paper, both approaches were used for creating historiographical analyses of the core part of the BiographySampo data, the National Biography of Finland. In addition, we have evaluated our methods to estimate the reliability of our results. Our approach gives scholars novel biographical and prosopographical tools for analyzing individual persons and their groups. The tools combine the quantitative approach and distant reading methods [28] with the qualitative approach, often based on close reading, typical to biographical research. The portal contains numerous views that enable the users to study the lives of the biographees as well as prosopographical groups in terms of statistics, maps, language usage, and networks based on references made in the biographies or based on the family relations extracted from the biographical descriptions.
The key findings of this paper give insight to the editors of the National Biography as well as to researchers in biography, prosopography, and historiography. They also highlight the possibilities and issues in modeling historical data related to, e.g, editorial choices, modeling uncertainty, serendipitous knowledge discovery, and data literacy.
Using automatically structured linked data in research needs new kind data literacy from the end user. As discussed above, in BiographySampo some parts (subgraphs) in the NBF dataset are based on reliable hand coded metadata while others were created by the machine. In big datasets like this it is not possible to check and correct the generated data manually, so more errors are expected to be encountered than in manually curated datasets. Furthermore, the linked data approach is based on using explicit classifications and ontologies for which different opinions may arise. In many cases, the underlying real world is too complex to be modelled fully in practice. For example, the historical place ontology underlying BiographySampo covers centuries of places that in reality change in time. For example, Finland was part of Sweden until 1809, then part of Russia until becoming independent in 1917, and after that some parts of her were annexed to the Soviet Union that became later the modern Russia.
The gaps in describing the lives of historical figures caused also challenges for analytics and data modeling. There are irregularities in describing biographees, their relatives, and vocations due to lack of reliable historical sources. This makes knowledge extraction somewhat challenging at times and the possibility for errors can increase, as the algorithms may misinterpret the original data and skip or mislabel data resulting in, for example, mislabeled family relations and anomalies in statistical or network visualizations. For example, similarly to what is mentioned by [28], the exact birth and death years of some people who lived in the early days of history are not known precisely, and heavily rounded inexact dates, such as 1100, appear in the data. The source data does not tell whether a year, such as 1100, is rounded or actually is a precise value. Without better knowledge, the system now assumes that all dates are accurate, resulting,e.g., in a peak of 100-year-old people in statistical visualizations. This phenomenon indicates how source criticism and understanding the underlying data is needed when interpreting quantitative results. A mechanism for representing uncertainty in a machine understandable way would be needed to address the problem, but it remains a topic for future research.
In our work, the data was transformed from the CSV format to RDF and used as an input for further enrichment and transformation. Modelling the person and document metadata as RDF facilitated to creating the visualizations and performing the analyses depicted in this article. The transformation, extraction, and linking of the data was performed with satisfactory results (cf. Section 2.2). This data was used to enable distant reading by building data analytical applications and visualizations into BiographySampo. Unlike in [2,54,55], the data is in RDF format stored as knowledge graphs.
The Linked Data infrastructure created for BiographySampo also enables serendipitous knowledge discovery. The user can not only learn about the demographics through the statistical lens but also the connections between individual biographees through the network visualizations and reference analysis tools. The transformed knowledge graphs are published openly and can be queried with SPARQL to learn more about the data and the demographics.
Based on the analytics presented in this paper we have shown how to use Linked Data and SPARQL to create statistical, linguistic, and network analytics and visualizations to study a biographical data collection and its demographic features. These applications are related to the analytics represented in [2,54,55] but extend these analytics to describe the NBF dataset and also consider how the data has been created and used [37]. The data quality is not only impacted by its modeling and transformation process but also by its biases and sometimes historical uncertainty that exists in the source data. In comparison to the Ainm [2], the NBF is also biased towards the period from the mid 19th century onward whereas the ODNB [55] covers a wider span of time between the 16th century and current times.
Similarly to the Ainm and the ODNB, the visualizations tell the history of both the nation and of the collection itself. The place visualizations in this paper conform mainly to Finnish historical narratives that are tied to its neighbouring and European countries. Similar themes are present in the visualizations regarding relatives and vocations. The social structures are different in different countries, and cannot be used easily for transnational comparisons. As in Ainm and ODNB, the demographic of our dataset consists mainly of men while women are a minority. Furthermore, the networks are also influenced by the authors’ decisions as each reference to another person is based on a choice. This has also become evident through the language analysis, as the lists of most common words in biographies of women contain more words to describe families than in the biographies of men. However, the language usage requires closer inspection to sort out the influence of the authors and it remains as a future work.
The Linked Data approach presented in this paper helps one to describe and analyze a biography collection with its strengths and weaknesses for further research, and to find out points of interest for close reading. The methods, results, and insights presented for the NBF can be utilized in DH research for other similar collections to learn more about the demographics of the collection itself, the underlying history, and to evaluate the reliability of the results.
Notes
11 Online at www.biografiasampo.fi; see project homepage https://seco.cs.aalto.fi/projects/biografiasampo/en/ for further info and publications.
12 Prosopography is a method that is used to study groups of people through their biographical data. The goal of prosopography is to find connections, trends, and patterns from these groups.
29 See the dataset home page at https://www.ldf.fi/dataset/nbf for more details.
37 Query amount of unmarried and childless men and women: https://api.triplydb.com/s/oc6bZUcvp.
38 Query most common jobs for unmarried and childless persons: https://api.triplydb.com/s/Wtj8eUkhZ.
48 I.e. by the birth year of the person whose biography references the source biographee.
49 By their decade of birth.
Acknowledgements
Thanks to Mikko Kivelä, Jouni Tuominen, and other members of the Semantic Computing Research Group (SeCo) for inspirational discussions related to network analyses and Linked Data services. We would also like to thank Werner Scheltjens and the anonymous reviewers for valuable feedback and comments of the earlier version of the article. Our research was part of the project Texts as Data Services (Severi),5454 funded mainly by Business Finland, and the EU project In/Tangible European Heritage – Visual Analysis, Curation and Communication (InTaVia).5555 CSC – IT Center for Science has provided computational resources for our projects.
References
[1] | Á.Z. Bernád and M. Kaiser, The biographical formula: Types and dimensions of biographical networks, in: Proceedings of the Second Conference on Biographical Data in a Digital World 2017, Linz, Austria, November 6–7, 2017, CEUR Workshop Proceedings, Vol. 2119: , (2018) . |
[2] | Ú. Bhreathnach, C. Burke, J.M. Fhinn, G.Ó. Cleircín and B.Ó. Raghallaigh, A quantitative analysis of biographical data from Ainm, the Irish-language Biographical Database, 2019, presented at the 3rd Conference on Biographical Data in a Digital World (BD 2019), http://doras.dcu.ie/23774/1/Ainm%20BD%20FINAL.docx.pdf. |
[3] | M. Bianchini, M. Gori and F. Scarselli, Inside PageRank, ACM Transactions on Internet Technology (TOIT) 5: (1) ((2005) ), 92–128. doi:10.1145/1052934.1052938. |
[4] | S. Brin and L. Page, The anatomy of a large-scale hypertextual web search engine, Computer Networks 30: ((1998) ), 107–117. doi:10.1016/s0169-7552(98)00110-x. |
[5] | C. Chiarcos and C. Fäth, CoNLL-RDF: Linked corpora done in an NLP-friendly way, in: Language, Data, and Knowledge First International Conference, LDK 2017, Proceedings, Galway, Ireland, June 19–20, 2017, LNAI, Vol. 10318: , Springer, Cham, (2017) , pp. 74–88. doi:10.1007/978-3-319-59888-8_6. |
[6] | A. Fokkens, S. ter Braake, N. Ockeloen, P. Vossen, S. Legêne, G. Schreiber and V. de Boer, BiographyNet: Extracting relations between people and events, in: Europa Baut Auf Biographien, New Academic Press, Berlin, Germany, (2017) , pp. 193–224. |
[7] | A. Fokkens, S. ter Braake, R. Sluijter, P. Arthur and E. Wandl-Vogt (eds), BD-2017 Biographical Data in a Digital World 2017, CEUR Workshop Proceedings, Vol. 2119: , (2017) . |
[8] | A. Gangemi, V. Presutti, D.R. Recupero, A.G. Nuzzolese, F. Draicchio and M. Mongiovì, Semantic web machine reading with FRED, Semantic Web – Interoperability, Usability, Applicability 8: (6) ((2017) ), 873–893. doi:10.3233/sw-160240. |
[9] | V. Gunter, S. Matthias and G. Vogeler, Data exchange in practice: Towards a prosopographical API (preprint), in: Proceedings of the Third Conference on Biographical Data in a Digital World (BD 2019), Varna, Bulgaria, September, 2019, (2019) . |
[10] | H. Hakosalo, S. Jalagin, M. Junila and H. Kurvinen, in: Historiallinen elämä – Biografia ja historiantutkimus, Suomalaisen Kirjallisuuden Seura (SKS), Helsinki, (2014) , pp. 1–342. |
[11] | A. Hashmi, F. Zaidi, A. Sallaberry and T. Mehmood, Are all social networks structurally similar? in: Advances in Social Networks Analysis and Mining (ASONAM), 2012 IEEE/ACM International Conference on, IEEE, (2012) , pp. 310–314. doi:10.1109/asonam.2012.59. |
[12] | T. Heath and C. Bizer, Linked Data: Evolving the Web into a Global Data Space, Synthesis Lectures on the Semantic Web: Theory and Technology, Morgan & Claypool, Palo Alto, CA, (2011) . doi:10.2200/S00334ED1V01Y201102WBE001. |
[13] | S. Hellmann, J. Lehmann and S. Auer, NIF: An ontology-based and linked-data-aware NLP Interchange Format, 2012, http://scholar.google.com.au/scholar?q=nlp2rdf+hellman&btnG=&hl=en&as_sdt=0%2C5&as_ylo=2010#5. |
[14] | S. Hellmann, J. Lehmann and S. Auer, Towards an ontology for representing strings, 2012, http://svn.aksw.org/papers/2012/WWW_NIF/public/string_ontology.pdf. |
[15] | S. Hellmann, J. Lehmann, S. Auer and M. Brümmer, Integrating NLP using linked data, in: Proceedings, Part II, The Semantic Web – ISWC 2013: 12th International Semantic Web Conference, Sydney, NSW, Australia, October 21–25, 2013, Springer, Berlin Heidelberg, (2013) , pp. 98–113. doi:10.1007/978-3-642-41338-4_7. |
[16] | E. Hyvönen, Publishing and Using Cultural Heritage Linked Data on the Semantic Web, Morgan & Claypool, Palo Alto, CA, (2012) . doi:10.2200/S00452ED1V01Y201210WBE003. |
[17] | E. Hyvönen, “sampo” model and semantic portals for digital humanities on the semantic web, in: Proceedings of the Digital Humanities in the Nordic Countries 5th Conference, Riga, Latvia, October 21–23, 2020, CEUR Workshop Proceedings, Vol. 2612: , (2020) , pp. 373–378, http://ceur-ws.org/Vol-2612/poster1.pdf. |
[18] | E. Hyvönen, Using the semantic web in digital humanities: Shift from data publishing to data-analysis and serendipitous knowledge discovery, Semantic Web – Interoperability, Usability, Applicability 11: (1) ((2020) ), 187–193. doi:10.3233/SW-190386. |
[19] | E. Hyvönen, M. Alonen, E. Ikkala and E. Mäkelä, Life stories as event-based linked data: Case semantic national biography, in: Proceedings of the ISWC 2014 Posters & Demonstrations Track, a Track Within the 13th International Semantic Web Conference (ISWC 2014), Riva del Garda, Italy, October 21, 2014, CEUR Workshop Proceedings, Vol. 1272: , (2014) , pp. 1–4. |
[20] | E. Hyvönen, P. Leskinen, E. Heino, J. Tuominen and L. Sirola, Reassembling and enriching the life stories in printed biographical registers: Norssi high school alumni on the semantic web, in: Proceedings, Language, Technology and Knowledge (LDK 2017), LNAI, Vol. 10318: , Springer, Cham, (2017) , pp. 113–119. doi:10.1007/978-3-319-59888-8_9. |
[21] | E. Hyvönen, P. Leskinen, M. Tamper, H. Rantala, E. Ikkala, J. Tuominen and K. Keravuori, BiographySampo – publishing and enriching biographies on the semantic web for digital humanities research, in: The Semantic Web – 16th International Conference, ESWC 2019, Proceedings, Portorož, Slovenia, June 2–6, 2019, LNCS, Vol. 11503: , Springer, (2019) , pp. 574–589, ISSN 16113349. ISBN 9783030213473. doi:10.1007/978-3-030-21348-0_37. |
[22] | E. Hyvönen, P. Leskinen, M. Tamper, H. Rantala, E. Ikkala, J. Tuominen and K. Keravuori, Linked data – a paradigm change for publishing and using biography collections on the semantic web, in: Proceedings of the Third Conference on Biographical Data in a Digital World (BD 2019), Varna, Bulgaria, September, 2019, (2019) . |
[23] | E. Hyvönen, P. Leskinen, M. Tamper, J. Tuominen and K. Keravuori, Semantic National Biography of Finland, in: Proceedings of the Digital Humanities in the Nordic Countries 3rd Conference (DHN 2018), Helsinki, Finland, March 7–9, 2018, Vol. 2084: , CEUR Workshop Proceedings, (2018) , pp. 372–385. |
[24] | E. Hyvönen and H. Rantala, Knowledge-based relation discovery in cultural heritage knowledge graphs, in: Proceedings of the Digital Humanities in the Nordic Countries 4th Conference, Copenhagen, Denmark, March 5–8, 2019, CEUR Workshop Proceedings, (2019) , pp. 230–239. http://www.ceur-ws.org/Vol-2364/. |
[25] | E. Hyvönen, J. Tuominen, M. Alonen and E. Mäkelä, Linked data Finland: A 7-star model and platform for publishing and re-using linked datasets, in: The Semantic Web: ESWC 2014 Satellite Events – ESWC 2014 Satellite Events, Anissaras, Crete, Greece, May 25–29, 2014, Revised Selected Papers, Springer-Verlag, (2014) , pp. 226–230. doi:10.1007/978-3-319-11955-7_24. |
[26] | E. Ikkala, E. Hyvönen, H. Rantala and M. Koho, Sampo-UI: A Full Stack JavaScript Framework for Developing Semantic Portal User Interfaces, Semantic Web, Interoperability, Usability, Applicability (2021). |
[27] | E. Ikkala, J. Tuominen and E. Hyvönen, Contextualizing historical places in a gazetteer by using historical maps and linked data, in: Digital Humanities 2016, Krakow, Abstracts, (2016) , pp. 573–577, https://dh2016.adho.org/abstracts/. |
[28] | S. Jänicke, G. Franzini, M.F. Cheema and G. Scheuermann, Visual text analysis in digital humanities, in: Computer Graphics Forum, Vol. 36: , Wiley Online Library, (2017) , pp. 226–250. doi:10.1111/cgf.12873. |
[29] | A. Jatowt, D. Kawai and K. Tanaka, Time-focused analysis of connectivity and popularity of historical persons in Wikipedia, International Journal on Digital Libraries 20: (4) ((2019) ), 287–305. doi:10.1007/s00799-018-0231-4. |
[30] | T. Keith, Changing Conceptions of National Biography, Cambridge University Press, (2005) . doi:10.1017/cbo9780511497582. |
[31] | M. Klinge (ed.), in: Suomen Kansallisbiografia 1–10, Suomalaisen Kirjallisuuden Seura, Helsinki, Finland (2003) –(2007) , p. 9519. |
[32] | M. Koho, E. Heino and E. Hyvönen, SPARQL faceter-client-side faceted search based on SPARQL, in: Joint Proceedings of the 4th International Workshop on Linked Media and the 3rd Developers Hackshop Co-Located with the 13th Extended Semantic Web Conference ESWC 2016, Heraklion, Crete, Greece, May 30, 2016, CEUR Workshop Proceedings, Vol. 30: , (2016) . |
[33] | A. Langmead, J. Otis, C. Warren, S. Weingart and L. Zilinski, Towards interoperable network ontologies for the digital humanities, International Journal of Humanities and Arts Computing 10: ((2016) ). doi:10.3366/ijhac.2016.0157. |
[34] | R. Larson, Bringing Lives to Light: Biography in Context, Final Project Report, 2010, University of Berkeley. |
[35] | P. Leskinen and E. Hyvönen, Extracting genealogical networks of linked data from biographical texts, in: The Semantic Web: ESWC 2019 Satellite Events, Springer-Verlag, (2019) , pp. 121–125. doi:10.1007/978-3-030-32327-1_24. |
[36] | P. Leskinen, E. Hyvönen and J. Tuominen, Analyzing and visualizing prosopographical linked data based on biographies, in: Proceedings of the Second Conference on Biographical Data in a Digital World 2017, Linz, Austria, November 6–7, 2017, Vol. 2119: , (2018) , pp. 39–44. |
[37] | E. Mäkelä, K. Lagus, L. Lahti, T. Säily, M. Tolonen, M. Hämäläinen, S. Kaislaniemi and T. Nevalainen, Wrangling with non-standard data, in: Proceedings of the Digital Humanities in the Nordic Countries 5th Conference, Riga, Latvia, October 21–23, 2020, CEUR Workshop Proceedings, (2020) , pp. 81–96. |
[38] | J.L. Martinez-Rodriguez, A. Hogan and I. Lopez-Arevalo, Information extraction meets the semantic web: A survey, Semantic Web – Interoperability, Usability, Applicability 11: (2) ((2020) ), 255–335. doi:10.3366/ijhac.2015.0140. |
[39] | D. Metilli, V. Bartalesi and C. Meghini, A Wikidata-based tool for building and visualising narratives, International Journal on Digital Libraries 20: (4) ((2019) ), 417–432. doi:10.1007/s00799-019-00266-3. |
[40] | G. Miyakita, P. Leskinen and E. Hyvönen, Using linked data for prosopographical research of historical persons: Case U.S. congress legislators, in: Proceedings. Part II, Digital Heritage. Progress in Cultural Heritage: Documentation, Preservation, and Protection: 7th International Conference, EuroMed 2018, Proceedings. Part II, Nicosia, Cyprus, October 29–November 3, 2018, LNCS, Vol. 11197: , Springer, (2018) , pp. 150–162. doi:10.1007/978-3-030-01765-1_18. |
[41] | F. Moretti, Distant Reading, Verso Books, (2013) . |
[42] | F. Moretti and A. Piazza, Graphs, maps, trees: Abstract models for a literary history, Modern Language Quarterly 68: (1) ((2007) ), 132–135. doi:10.1215/00267929-2006-032. |
[43] | M.C. Pattuelli, M. Miller, L. Lange and H.K. Thorsen, Linked jazz 52nd street: A LOD crowdsourcing tool to reveal connections among jazz artists, in: 8th Annual International Conference of the Alliance of Digital Humanities Organizations, DH 2013, Lincoln, NE, USA, July 16–19, 2013, Conference Abstracts, Alliance of Digital Humanities Organizations (ADHO), (2013) , pp. 337–339. |
[44] | L. Rietveld and R. Hoekstra, The YASGUI family of SPARQL clients, Semantic Web – Interoperability, Usability, Applicability 8: (3) ((2017) ), 373–383. doi:10.3233/SW-150197. |
[45] | B. Roberts, Biographical Research, Understanding Social Research, Open University Press, (2002) . |
[46] | M. Rospocher, M. van Erp, P. Vossen, A. Fokkens, I. Aldabe, G. Rigau, A. Soroa, T. Ploeger and T. Bogaard, Building event-centric knowledge graphs from news, Web Semantics: Science, Services and Agents on the WWW 37: ((2016) ), 132–151. doi:10.2139/ssrn.3199233. |
[47] | M. Schlögl and K. Lejtovicz, A prosopographical information system (APIS), in: Proceedings of the Second Conference on Biographical Data in a Digital World 2017, Linz, Austria, November 6–7, 2017, CEUR Workshop Proceedings, Vol. 2119: , (2018) . |
[48] | M. Tamper, E. Hyvönen and P. Leskinen, Visualizing and analyzing networks of named entities in biographical dictionaries for digital humanities research, in: Proceedings of the 20th International Conference on Computational Linguistics and Intelligent Text Processing (CICling 2019), Springer-Verlag, (2019) , Accepted. https://seco.cs.aalto.fi/publications/2019/tamper-et-al-cicling-2019.pdf. |
[49] | M. Tamper, P. Leskinen, K. Apajalahti and E. Hyvönen, Using biographical texts as linked data for prosopographical research and applications, in: Digital Heritage. Progress in Cultural Heritage: Documentation, Preservation, and Protection. 7th International Conference, EuroMed 2018, Springer-Verlag, Nicosia, Cyprus, (2018) , pp. 125–137. doi:10.1007/978-3-030-01762-0_11. |
[50] | M. Tamper, P. Leskinen, J. Tuominen and E. Hyvönen, Modeling and publishing Finnish person names as a linked open data ontology, in: Proceedings of the Third Workshop on Humanities in the Semantic Web (WHiSe 2020) Co-Located with 15th Extended Semantic Web Conference (ESWC 2020), Heraklion, Greece, June 2, 2020, CEUR Workshop Proceedings, (2020) , pp. 3–14. |
[51] | S. ter Braake, A. Fokkens, R. Sluijter, T. Declerck and E. Wandl-Vogt (eds), BD2015 Biographical Data in a Digital World 2015, CEUR Workshop Proceedings, Vol. 1399: , (2015) . |
[52] | J. Tuominen, E. Hyvönen and P. Leskinen, Bio CRM: A data model for representing biographical data for prosopographical research, in: Proceedings of the Second Conference on Biographical Data in a Digital World 2017, Linz, Austria, November 6–7, 2017, CEUR Workshop Proceedings, Vol. 2119: , (2018) . |
[53] | K. Verboven, M. Carlier and J. Dumolyn, A short manual to the art of prosopography, in: Prosopography Approaches and Applications. A Handbook, Unit for Prosopographical Research (Linacre College), (2007) , pp. 35–70. doi:1854/8212. |
[54] | C. Warren, D. Shore, J. Otis, L. Wang, M. Finegold and C. Shalizi, Six degrees of Francis bacon: A statistical method for reconstructing large historical social networks, Digital Humanities Quarterly 10: ((2016) ), 1–16. |
[55] | C.N. Warren, Historiography’s two voices: Data infrastructure and history at scale in the Oxford Dictionary of National Biography (ODNB), Journal of Cultural Analytics 1: (2) ((2018) ), 1–31. doi:10.22148/16.028. |
[56] | Y. Wu, H. Sun and C. Yan, An event timeline extraction method based on news corpus, in: 2017 IEEE 2nd International Conference on Big Data Analysis, IEEE, (2017) , pp. 697–702. doi:10.1109/icbda.2017.8078725. |