Linked Dataset description papers at the Semantic Web journal: A critical assessment
Abstract
Since 2012, the Semantic Web journal has been accepting papers in a novel Linked Dataset description track. Here we motivate the track and provide some analysis of the papers accepted thus far. We look at the ratio of accepted papers in this time-frame that fall under this track, the relative impact of these papers in terms of citations, and we perform a technical analysis of the datasets they describe to see what sorts of resources they provide and to see if the datasets have remained available since publication. Based on a variety of such analyses, we present some lessons learnt and discuss some potential changes we could apply to the track in order to improve the overall quality of papers accepted.
1.Introduction
Linked Data provides a basic set of principles outlining how open data can be published on the Web in a highly-interoperable interconnected manner using Semantic Web technologies [17]. Hundreds of Linked Datasets have been published through the years, introducing data on a plethora of topics to the Semantic Web. These datasets have played an important part not only in various applications, but also for furthering research, where they are used, for example, as reference knowledge-bases, as evaluation test-beds, and so forth. As evidence of the potential value of a dataset for research, one need look no further than DBpedia [23], whose associated research papers have been cited several thousand times in the past nine years according to Google Scholar.1 1
However, publishing papers describing datasets has not always been straightforward in our community. To meet the criteria for a traditional research track, papers must provide some novel technical contribution, evaluation, etc. Likewise, for in-use tracks, the focus is on applications, with emphasis on industrial use-cases. And so, despite the evident impact that datasets can have on research, there were few (if any) suitable venues for publishing descriptions of such datasets.
This historical undervaluation of datasets is far from unique to the Semantic Web community. Data are the lifeblood of many scientific research communities where the availability of high-quality datasets is crucial to their advancement. In recognition of the central role of data, and in order to incentivise and adequately reward researchers working on dataset compilation and curation, there are a growing number of journals that accept descriptions of datasets as part of a dedicated track in areas such as bioinformatics, geosciences, astronomy, experimental physics, and so forth. In fact, there are now also data journals whose primary goal is to publish such descriptions; one of the most prominent examples is Nature’s Scientific Data journal: an open access, peer-reviewed journal dedicated to publishing descriptions of datasets judged to have scientific merit. Thus, in a variety of scientific communities, there are now a variety of options for publishing descriptions of datasets without the need to meet traditional research-track criteria.2 2
Motivated by similar reasoning, the Semantic Web Journal likewise decided to begin soliciting dataset description papers about 4 years ago.
Dataset papers at the Semantic Web journal
On February 29th, 2012, the Semantic Web Journal (SWJ) announced the first “Special Call” for Linked Dataset descriptions.3 3 This was followed up by further calls and, eventually, the addition of a permanent call for dataset descriptions.
When compared with data journals and tracks in other disciplines, the SWJ calls have some subtle but key differences. While in other areas the emphasis is on the potential scientific value of that dataset to that research community, for SWJ datasets the core requirement is that the dataset is modelled appropriately using Semantic Web standards and published correctly using Linked Data principles. In this sense, the topic and domain of the dataset for SWJ is not as important. For example, a Linked Dataset about historical events may not have a direct influence on Semantic Web research in the same way a dataset about genes may have impact in the Bioinformatics community, but can still be accepted by SWJ if deemed of potential impact in another area (or in practice). Thus, the goal for SWJ is in publishing descriptions of high-profile exemplars of what a Linked Dataset can and should be, no matter their topic.
Criteria for review
The criteria for accepting dataset papers differ from those for other tracks. Specifying these criteria in a precise way in the call for papers is crucial to ensure that authors know what requirements they need to fulfil before their paper can be accepted and to ensure that reviewers for different papers can apply a consistent standard. However, given the nature of the track, specifying precise criteria proved challenging and the call has undergone various revisions. The current call for dataset descriptions is as follows:
Linked Dataset Descriptions – short papers (typically up to 10 pages) containing a concise description of a Linked Dataset. The paper shall describe in concise and clear terms key characteristics of the dataset as a guide to its usage for various (possibly unforeseen) purposes. In particular, such a paper shall typically give information, amongst others, on the following aspects of the dataset: name, URL, version date and number, licensing, availability, etc.; topic coverage, source for the data, purpose and method of creation and maintenance, reported usage etc.; metrics and statistics on external and internal connectivity, use of established vocabularies (e.g., RDF, OWL, SKOS, FOAF), language expressivity, growth; examples and critical discussion of typical knowledge modeling patterns used; known shortcomings of the dataset. Papers will be evaluated along the following dimensions: (1) Quality and stability of the dataset – evidence must be provided. (2) Usefulness of the dataset, which should be shown by corresponding third-party uses – evidence must be provided. (3) Clarity and completeness of the descriptions. Papers should usually be written by people involved in the generation or maintenance of the dataset, or with the consent of these people. We strongly encourage authors of dataset description paper to provide details about the used vocabularies, ideally using the 5 star rating [20].
This captures much of the criteria that the SWJ editors currently feel important for a good dataset description paper, but indeed is always subject to further refinement.
This leads to the second significant challenge. Based on these criteria, it is important in the peer-review process that not only the paper but also the dataset itself is scrutinised. While it is possible to define upfront some high-level criteria that the dataset must meet, deciding that a particular dataset is, e.g., modelled appropriately, free of technical errors, provides high-quality links, uses existing vocabulary in a suitable manner, etc., requires finding reviewers with strong experience in Linked Data publishing, including a variety of technical issues such as HTTP content negotiation, RDF modelling, datatypes, awareness of popular vocabularies that could be reused, datasets that could be linked to, the semantics of RDFS and OWL, the SPARQL protocol and query language, representing taxonomies in SKOS, vocabularies such as VoID used to describe a dataset, services such as Datahub used to publicise a dataset, and so on. Even aside from the current state of the art, many best practices in terms of how Linked Datasets should be published are still in formation.
Furthermore, aside from technical issues, reviewers must also judge the usefulness of a dataset; however, such datasets can be in areas unfamiliar to the reviewer in question – they may be historical datasets, geographic datasets, etc. Thus, within such specialised communities, it may be difficult for reviewers to assess how useful the topic of the dataset is, how complete the data are, whether all of the interesting dimensions of the domain are captured or not, and so forth. For this reason, the call was amended (to the version listed above) to put the burden of proof of quality, stability and usefulness on authors, where, e.g., the paper must now demonstrate evidence of third-party use, such as referencing a third-party paper where the dataset is used: previously the ability to demonstrate the potential usefulness of a dataset was sufficient to be considered for acceptance.
Volume of dataset papers
The first 15 dataset papers were published in the original Special Issue, Volume 4(2), 2013. By the end of 2015, 38 papers had been accepted under the dataset description track at SWJ, of which 33 have been published in print up to, and including Volume 7(1), 2016. To put these figures in perspective, 96 non-dataset papers were accepted for publication in the same time frame (starting with Volume 4(3)), of which 61 have been published in print (up to and including Volume 7(1)).4 4 Hence we see that 28.4% of papers accepted in this time frame and 35.1% of papers published in print have been dataset papers, constituting a significant ratio of the articles published by the journal.
Table 1 provides the number of such papers published per year, where by In Press we include those papers that are accepted and published online but have not yet appeared in print. In 2013, a single issue – the first special issue – accounted for all 15 dataset papers; taking this as an equal starting point, only one non-dataset issue with 3 papers was counted thereafter in the same year. One may note the comparatively small number of dataset papers that are in press. We cannot be sure exactly why there has been a drop in these papers, but it may be possible that the stricter version of the call (e.g., requiring concrete evidence of third-party dataset use) has reduced the number of recent, eligible submissions.
Table 1
Number of dataset and non-dataset papers per year, starting from Volume 4(2) in 2013
Year | Dataset Papers | Non-dataset Papers |
2013 | 15 | 3 |
2014 | 4 | 11 |
2015 | 13 | 43 |
2016 | 1 | 4 |
In Press | 5 | 35 |
Open questions
Given that we have had 2–3 years of collecting and publishing dataset papers, and given the relative novelty of such a call, here we wish to offer a retrospective on this track. In particular, we wish to perform some analyses to try to answer two key questions about these papers and, more importantly, the datasets they describe:
– Have these datasets had impact in a research setting? (Section 2)
∗ We look at the citations to the dataset description papers (assuming that research works that make use of the dataset will likely cite this paper).
– Are the dataset-related resources the paper links to still available? (Section 3)
∗ We look to see if the Linked Data URIs, SPARQL endpoints, and so forth, associated with the published datasets are still available or if they have gone offline.
Based on these results, in terms of the future of the track, we discuss some lessons learnt in Section 4, before concluding in Section 5.
2.Research impact
We first wish to measure the impact that the published datasets have had within research – not only within the Semantic Web or broader Computer Science community, but across all fields. To estimate this, we look at the citations that the descriptions have received according to Google Scholar, which indexes technical documents from various fields as found on the Web. The citations were manually recorded on 2016-01-26 for each paper of interest using keyword search on the title.
To provide some context for the figures, we compare metrics considering dataset and non-dataset papers. More precisely, we may refer to the following four categories of papers (corresponding with Table 1):
Published dataset (33) | All dataset papers published, in print, up to Volume 7(1), 2016, inclusive. |
Published non-dataset (61) | All non-dataset papers published, in print, from Volume 4(3), 2013, to issue Semantic Web 7(1), 2016, inclusive. |
Accepted dataset (38) | Published dataset papers as above and all dataset papers in-press on 2016-01-27. |
Accepted non-dataset (96) | Published non-dataset papers as above and all non-dataset papers in-press on 2016-01-27. |
We consider both accepted (but not yet published) and published versions given the disproportionate number of in press non-dataset papers, which are less likely to have gathered citations. Finally, it is important to note that some of these papers appeared very recently and have not accumulated any citations thus far.
Fig. 1.
Distribution of citations for published dataset and non-dataset papers (given the logarithmic x-axis, we do not plot papers with zero citations, of which there were 2 for dataset papers and 8 for non-dataset papers).
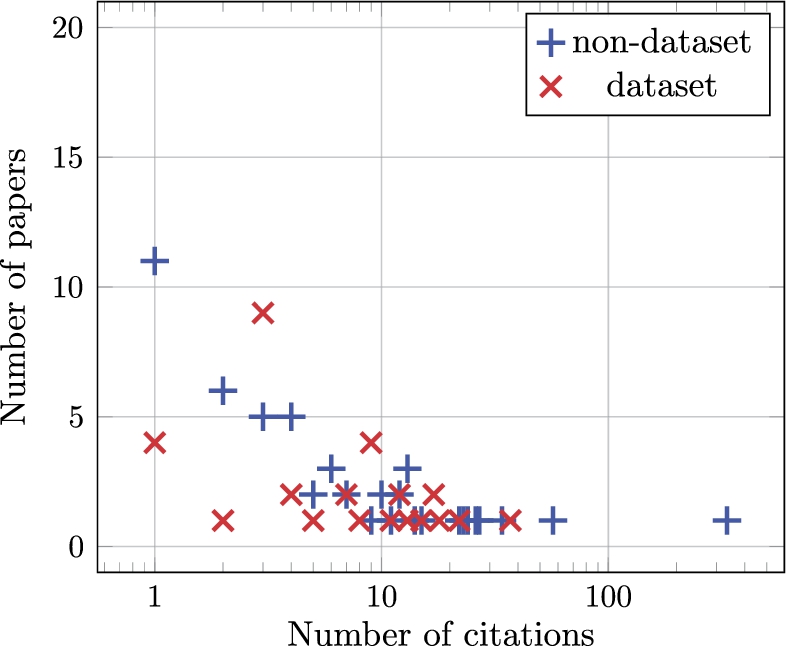
Fig. 2.
Distribution of citations for accepted dataset and non-dataset papers (given the logarithmic x-axis, we do not plot papers with zero citations, of which there were 4 for dataset papers and 24 for non-dataset papers).
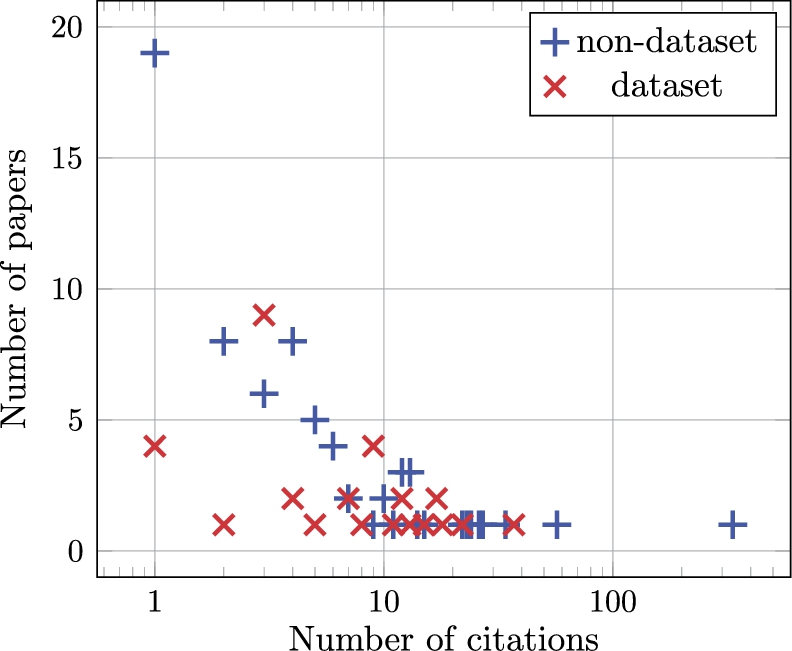
In Fig. 1, we present the distribution of citations for both types of published paper and in Fig. 2, we present the results for accepted papers. From the raw data collected, we draw the following observations:
– The sum of citations for published dataset papers was 257, implying a mean of 7.79 (std. dev. 7.57) citations per paper. The median number of citations per paper was 5.
∗ The sum of citations for published non-dataset papers was 779, implying a mean of 12.77 (std. dev. 42.67) citations per paper. The median number of citations per paper was 4.
– The sum of citations for accepted dataset papers was 278, implying a mean of 7.32 (std. dev. 7.54) citations per paper. The median number of citations per paper was 4.
∗ The sum of citations for accepted non-dataset papers was 839, implying a mean of 8.74 (std. dev. 34.46) citations per paper. The median number of citations per paper was 2.
– Considering accepted dataset papers, the h-index of the track is 10, meaning that 10 papers have 10 or more citations.
∗ For the accepted non-dataset papers, the h-index was 13.
∗ Considering both dataset and non-dataset papers together, the h-index was 16, including 5 dataset papers above the threshold.
– The most highly-cited dataset paper was by Caracciolo et al. [7] describing the AGROVOC dataset. It was published in 2013 and has received 37 citations.
∗ The most highly-cited non-dataset paper was by Lehmann et al. [23] describing the DBpedia knowledge-base. It was published in 2015 and has received 334 citations.
∗ Considering accepted dataset and non-dataset papers together, the most cited dataset paper would rank number 3 in the list of most cited papers.
– The dataset paper with the most citations per year was by Krouf & Troncy [22] describing the EventMedia dataset. It is not yet published but has received 17 citations.5 5
∗ The non-dataset paper with the most citations per year was the same DBpedia paper by Lehmann et al. [23].
From these results, we can conclude that although the most cited dataset track paper falls an order of magnitude behind the most cited non-dataset equivalent in terms of raw citations, it would still count as the third most cited paper overall in the time period. However, it is important to note, that the non-dataset paper with the most citations, which was officially evaluated under the criteria for a tools-and-systems paper, centres around a dataset: DBpedia.
In terms of mean and median citation results, the dataset papers are at least competitive with, or perhaps even outperforming, non-dataset papers over the same time-frame. We highlight that the dataset papers increase the overall h-index of papers in this time-frame by 3, with 5 dataset papers contributing to the increased h-index.
While we cannot draw general conclusions about the impact of these papers and datasets merely based on citations – particularly given the youth of many of the papers – we do at least have evidence to suggest that this impact, when measured through citations, has been more or less on par with the other papers of the journal.
3.Dataset availability
We now look in more detail at some of the resources provided by the 38 accepted dataset papers and check if they are still available for the public to access or not. In order to do this, we looked though the papers to find links to:
Datahub Entries | provide some meta-data about the dataset in a centralised catalogue, including tags, links to resources, examples, etc. |
Linked Data IRIs | denote Linked Data entities from the dataset that should, upon dereferencing, return content in a suitable RDF format about that entity. |
SPARQL Endpoints | provide a public interface that accepts queries in the SPARQL query language and returns answers over the dataset in question. |
Entries in Datahub – a central catalogue of datasets – are crucial to help third parties find the dataset and its related resources. Linked Data IRIs are the defining feature needed for publishing a Linked Dataset. SPARQL endpoints, though not strictly necessary for publishing a Linked Dataset, offer clients a convenient manner to query the dataset in question. Though we focus just on these three core aspects, datasets may provide other types of resources, such as VoID descriptions, custom vocabularies, etc., that we do not consider.
While in some papers we could not find direct links to one or more of these resources, we could find links with some further search; for example, a paper may have linked to a Datahub entry which itself contained pointers to a SPARQL endpoint or a Linked Data IRI, which we would include. Given the number of papers and the diversity in how resources were linked to, we were limited in how thorough we could be; that is to say, it is possible that we overlooked resources, especially if not linked directly from the paper in an obvious manner (e.g., a link to SPARQL endpoint not containing the keyword “sparql” or “endpoint” in the URL or surrounding text).
In Table 2, we summarise the availability of Datahub Entries and Linked Data IRIs for all of the accepted dataset papers. Note that in the following, all percentages are presented relative to the 38 accepted dataset papers.
Table 2
Availability of Linked Data resources for dataset papers
– In the Ref column, we provide a pointer to the associated dataset paper.
– In the DH column, we indicate whether or not we could find a Datahub entry relating to the dataset described by the paper: ‘✓’ indicates that a link was found to an online entry, ‘✗’ indicates that a link was found but the entry was offline, while ‘?’ indicates that no link could be found.6 6 Again, in some cases although there were no explicit links in the papers, we tried to find the entry through searching Datahub.
∗ We could find a link to a Datahub entry in 32 cases (84.2%), where 31 of these entries were still online (81.6%).
– In the Linked Data IRI column, we provide a single example Linked Data IRI pertaining to the dataset that we could find either in the paper, or in the Datahub entry, or from querying the SPARQL endpoint, or by some other means: ‘?’ indicates that no such IRI could be found. In the A? column, we indicate whether or not we could retrieve some information about the resource (in RDF) by dereferencing the Linked Data IRI that identifies it: ‘✓’ indicates (partial) success, ‘✗’ indicates failure, ‘?’ indicates we could find no IRI to test; these experiments were run on 2016-01-28. In the Note column, we identify problems that we encountered: where we could retrieve information, we remark on various basic (but “non-fatal”) configuration problems;7 7 where we could not retrieve information or could not find an IRI, we give a brief indication of the type of error we encountered.
∗ We could find an example Linked Data IRI in 33 cases (86.8%). From these, we could retrieve useful information in 24 cases (63.2%), where for 15 such cases (39.5%) we could not detected any basic configuration problems.
From these results, we can conclude that the majority of papers provide Datahub entries; however, we found that the information provided in these Datahub entries tended to vary greatly: some datasets provided links to a wide variety of resources, some datasets provided the minimum necessary to have an entry.
With respect to hosting Linked Data, of the 24 papers (63.2%) for which we found an operational Linked Dataset, 9 papers (23.7%) exhibited some basic issues, including not distinguishing information resources from general resources (i.e., not using a 303 redirect or a hash IRI), or not managing content negotiation correctly (i.e., not considering the accept header or not returning the correct content-type). For the 13 datasets (34.2%) that were offline, informally speaking, in almost all cases, the issue seemed long-term (e.g., the web-site associated with the dataset was no longer available). In one other case, we could find no links in the paper leading to any relevant website or any example data resource, meaning that, at the time of writing in January 2016, for 14 datasets (36.8%), we could no longer find an operational Linked Dataset; 4 of these were for papers published in 2013, 3 in 2014, 4 in 2015, 1 in 2016, and 1 paper in press.
Next we looked at the SPARQL endpoints associated with the datasets. Although not implicitly required for a Linked Dataset nor explicitly required by the call, many papers offer a link to such an endpoint as a central point-of-access for the dataset. In Table 3, we provide an overview of the endpoints provided by various papers. In certain cases, such as the Linked SDMX dataset [6] or the eagle-i dataset [35], papers may link to multiple endpoints; in these cases, we found that the endpoints were always hosted on the same server and tended to exhibit very similar behaviour with respect to availability, hence we select and present details for one sample endpoint.
Table 3
Availability of SPARQL endpoints for dataset papers
– The Ref column again offers a pointer to the relevant dataset paper.
– The SPARQL Endpoint column offers a pointer to one of the endpoints associated with that dataset; ‘?’ indicates that no such endpoint could be found (from the paper, the Datahub entry, etc.).
∗ We could find a SPARQL endpoint URL in 31 cases (81.6%).
– In order to assess the availability of the endpoints, we search for them in the online SPARQLES tool [3],8 8 which monitors public SPARQL endpoints announced on Datahub, regularly sending them queries to determine their health, performance, etc. In the Month column, we list the uptime recorded for the endpoint for the month ending on 2016-01-27: SPARQLES sends each endpoint a simple SPARQL query9 9 every hour to see if it can respond, where the monthly uptime indicates the ratio of these hourly queries that succeeded. In the Last Seen column, we record the last time the system saw the endpoint as being available (which SPARQLES displays for the past year). For both columns, ‘?’ indicates that there was no endpoint to check while ‘!’ indicates that the endpoint was not monitored by SPARQLES (most likely because the endpoint is not linked from Datahub). In the latter column, ‘Active’ indicates that the endpoint was alive at the time of the tests, while ‘Pre-2015’ indicates that the endpoint went offline in 2014 or earlier, but we cannot pinpoint precisely when (since it falls outside the time-window of availability that SPARQLES displays).
∗ Of the 31 SPARQL endpoints (81.6%) considered, 21 endpoints were tracked by SPARQLES (55.3% of all datasets). Of these, 15 (39.5% of all datasets) were found to be active at the time of the tests; 1 went offline in 2016, 3 went offline in 2015, and 2 went offline prior to 2015.
∗ Of the 21 endpoints tracked by the system, we see that for the previous month, 5 endpoints had 0% uptime, 2 endpoints fell into the 40–60% bracket, 1 endpoint fell into the 90–95% bracket, and 13 endpoints fell into the 95–100% bracket.
– Given that we could not find all endpoints in SPARQLES, we ran a local check to see if all the endpoints listed were available at the time of writing. More specifically, we used the same procedure as SPARQLES [3] to determine availability, using a script to send each endpoint a simple query (using Jena) and seeing if it could return a valid response per the SPARQL standard. The results are presented in column A?, where ‘?’ indicates that there was no endpoint to check, ‘✓’ indicates success, ‘✗’ indicates failure, and ‘✻’ indicates that although the script failed due to some configuration problems on the server, a working interface was found where a user could manually enter a query.
∗ Of the 31 SPARQL endpoints (81.6%) considered, 18 endpoints (47.4% of datasets) were found to be accessible via the local script, with an additional endpoint found to be accessible manually.
∗ Of the 10 endpoints not tracked by SPARQLES, 2 endpoints were accessible by the local script and another was accessible manually; 7 were inaccessible.
∗ Of the endpoints tracked by SPARQLES, our local script corresponded with those deemed ‘Active’ but for one exception: we deemed the AGROVOC endpoint to be available at the time of writing while SPARQLES lists it as offline (we are not sure why this is the case).
In summary, at the time of writing, we found an operational endpoint (including one that required manual access) for 19 datasets (50%), we could only find non-operational endpoint links for 12 datasets (31.6%), and we could not find any endpoint link for 7 datasets (18.4%).
4.Lessons learnt
With respect to lessons learnt, there are various points to improve upon. We present some ideas we have in mind to – in some respect – tighten the requirements for the track and to help prevent accepting certain types of problematic papers.
Papers with inadequate links
In some cases, it was difficult to find links to a Datahub entry, a Linked Data IRI, or a SPARQL endpoint (where available) in the papers. In other cases, the links provided were out-of-date although resources were available elsewhere. In other cases still, the links provided were dead. In terms of links, the current call simply requires a “URL”.
One possibility to counteract the lack of links is to make a link to a Datahub entry mandatory, and links to at least 2 or 3 Linked Data IRIs mandatory. Likewise any other resources described in the paper, such as a SPARQL endpoint, a VoID description, a vocabulary, a dump, and so forth., must be explicitly linked from the paper. All such links should be clearly listed in a single table.
We could also ask that all corresponding links be provided on the Datahub entry; this would allow locations to be updated after the paper is published (if they change) and allow the public to discover the dataset more easily. If accepted, we could also encourage authors to add a link from Datahub to the description paper.
Datasets with technical issues
We found a number of datasets with core technical issues in terms of how the Linked Dataset is published: how entities are named, how IRIs are dereferenced, how the SPARQL endpoint is hosted, and so on. The current call lists the quality of the dataset as an important criterion for acceptance, but as mentioned in the introduction, finding reviewers able and willing to review a dataset for basic technical errors – let alone more subjective notions of quality, such as succinctness of the model, re-use of vocabulary, etc. – is difficult. Currently the call requires authors to provide evidence of quality; however, it is not clear what sort of evidence reviewers could or should expect.
One possibility is to put in place a checklist of tests that a dataset should pass. For example, in Footnote 7, we mentioned two validators for Linked Data IRIs that we used in these papers to help find various technical errors in how the datasets surveyed were published; unfortunately however, both of these tools were themselves sometimes problematic, throwing uninformative bugs, not supporting non-RDF/XML formats, and so on. An interesting (and perhaps more general) exercise, then, would be to consider what are the basic checks that a Linked Dataset should meet to be considered a “Linked Dataset” and to design systems that help authors and reviewers to quickly evaluate these datasets.
Datasets with poor potential impact
Some datasets are perhaps too narrow, too small, of too low quality, etc., to ever have notable impact, but it may be difficult for reviewers to assess the potential impact of a dataset when its topic falls outside their area of expertise.
Recently, the call was amended such that authors need to provide evidence of third-party use of the dataset before it can be accepted. As such, this sets a non-trivial threshold for the impact of the dataset: it must have at least one documented use by a third-party. Judging by the relatively fewer dataset papers in press at the moment, it is possible that this criterion has reduced the number of submissions we have received; in fact, many dataset papers have recently been rejected in a pre-screening phase. Thus, for the moment, we feel that this modification for the call suffices to address this issue (for now at least).
Short-lived or unstable datasets
We encountered a notable number of papers describing datasets where nothing is available online any longer, not even the website. Other papers offer links to services, such as SPARQL endpoints, that are only available intermittently. Of course, there are a number of factors making the stable hosting of a dataset difficult: many such datasets are hosted by universities on a non-profit basis, researchers may move away, projects may end, a dataset may go offline and not be noticed for some time, etc. Sometimes, nobody, including the dataset maintainers, can anticipate downtimes or periods of instability. At the same time, we wish to avoid the Linked Dataset description track being a cheap way to publish a journal paper: we wish to avoid the (possibly hypothetical) situation of authors putting some data online in RDF, writing a quick journal paper, getting it accepted, then forgetting about the dataset.
Now that papers in this track need to demonstrate third-party usage, we would expect this to naturally increase the threshold for acceptance and to encourage publications describing datasets where the authors are serious about the dataset and about seeing it adopted in practice. Likewise the call requires authors to provide evidence about the stability of their dataset; though what sort of evidence is not specified, it could, e.g., include statistics from SPARQLES about the historical availability of a relevant SPARQL endpoint, etc.
Summary
On the one hand, we need to keep the burden on reviewers manageable and allow them to apply their own intuition and judgement to individual cases; providing them long lists of mandatory criteria to check would likely frustrate reviewers and make it unlikely for them to volunteer. Likewise, we wish to keep some flexibility to ensure that we do not create criteria that rule out otherwise interesting datasets for potentially pedantic reasons.
On the other hand, to avoid accepting papers describing datasets with the aforementioned issues, we may need to (further) tighten the call and provide more concrete details on the sorts of contributions we wish to see. The results from this paper have certainly helped us gain some experiences that we will use to refine the call along the lines previously discussed.
5.Conclusion
Datasets play an important role in many research areas and the Semantic Web is no different. Recognising this importance, the Semantic Web journal has been publishing Linked Dataset description papers for the past 2.5 years, comprising 28.4% of accepted papers and 35.1% of papers published in print. In this paper, we offer an interim retrospective on this track, and try to collect some observations on the papers and the datasets accepted and/or published thus far by the journal.
With respect to impact, we found that these dataset papers have received citations that are on-par with the other types of papers accepted by the journal in the same time-frame. For example, the top cited dataset paper is the third most cited paper overall. Likewise, with the inclusion of dataset papers, the h-index of papers accepted in this time-frame increases from 13 to 16. We thus see that these papers – and, by extension, perhaps, their datasets – are having moderate research impact and receiving moderate numbers of citations. This, in some sense, justifies the original motivation for the track: to give researchers working on important datasets a research venue where their work can be properly recognised and counted.
With respect to the availability and sustainability of the datasets, results are mixed. While many of the datasets and the related resources originally described in the paper are still online, many have also gone offline. For example, by dereferencing Linked Data IRIs, we could find some data in RDF for 63.2% of the datasets, and could find an operational SPARQL endpoint for 50% of the datasets. On the other hand, some datasets went offline soon after acceptance, in some cases even before the paper was published in print.
Even for those datasets that were still accessible, a variety of technical issues were encountered. For example, although 63.2% of datasets had Linked Data IRIs that were still dereferenceable at the time of writing, only 39.5% were deemed to be following best practices and be free of technical HTTP-level errors (at least to the limited extent that our rather brief tests could detect). Likewise, some of the endpoints we found to be accessible had configuration problems, or uptimes below the basic “two nines” 99%, limiting their external usability, particularly, for example, in critical and/or real-time applications.
With respect to the future of the track, we identified four types of papers/datasets that we wish to take measures to avoid: papers with inadequate (or no) links to the dataset, or papers that describe datasets with technical issues, or that have poor potential usefulness, or that are short-lived or otherwise unstable. Though there are no clear answers in all cases, our general approach thus far has been to place the burden of proof on authors to demonstrate that their dataset is of potential use, high quality, stable, etc. Likewise, we will now consider and discuss the possibility of tightening the call to make further criteria mandatory.
In summary, while we found papers describing datasets that have disappeared, we also found papers describing stable, high-quality datasets that have had impact on research measurable in terms of citations – papers describing impactful datasets (and labour) worthy of recognition through a journal publication. Our future goal, then, is to increase the volume and ratio of the latter type of dataset description paper accepted by the journal.
Notes
1 See https://scholar.google.cl/scholar?hl=en&q=dbpedia; retrieved 2016-01-26.
2 For a list of such journals, please see Akers’ blog-post at https://mlibrarydata.wordpress.com/2014/05/09/data-journals/; retrieved 2016-01-28.
3 See http://www.semantic-web-journal.net/blog/semantic-web-journal-special-call-linked-dataset-descriptions, last retrieved 2016-01-06.
4 Here we only consider articles with more than one page, which may include editorials.
5 We count unpublished papers or papers published in 2016 as having 1 year, papers published in 2015 as having 2 years, etc. We note that many papers in the Semantic Web Journal may attract citations once they are published online, which may be months before publication in print.
6 In the PDF version of this paper, the former two symbols provide a hyperlink to a respective entry; for space reasons, we do not print the URLs in text.
7 To help detect configuration problems, we used, e.g., Linked Data validators such as http://www.hyperthing.org/ and http://vafu.redlink.io/; retrieved 2016-01-28.
8 http://sparqles.ai.wu.ac.at/; retrieved 2016-01-28.
9 “SELECT * WHERE { ?s ?p ?o } LIMIT 1”, or if that fails, “ASK WHERE { ?s ?p ?o }”; a valid SPARQL response to either is deemed a successful request.
Acknowledgements
This work was supported in part by FONDECYT Grant no. 11140900, by the Millennium Nucleus Center for Semantic Web Research under Grant NC120004, by the National Science Foundation (NSF) under award 1440202 EarthCube Building Blocks: Collaborative Proposal: GeoLink – Leveraging Semantics and Linked Data for Data Sharing and Discovery in the Geosciences, and NSF award 1540849 EarthCube IA: Collaborative Proposal: Cross-Domain Observational Metadata Environmental Sensing Network (X-DOMES).
References
1 | [[1]] R. Albertoni, M.D. Martino, S.D. Franco, V.D. Santis and P. Plini, EARTh: An environmental application reference thesaurus in the Linked Open Data cloud, Semantic Web 5: (2) ((2014) ), 165–171. doi:10.3233/SW-130122. |
2 | [[2]] S. Anibaldi, Y. Jaques, F. Celli, A. Stellato and J. Keizer, Migrating bibliographic datasets to the Semantic Web: The AGRIS case, Semantic Web 6: (2) ((2015) ), 113–120. doi:10.3233/SW-130128. |
3 | [[3]] C.B. Aranda, A. Hogan, J. Umbrich and P. Vandenbussche, SPARQL web-querying infrastructure: Ready for action? in: The Semantic Web – ISWC 2013: Proceedings of the 12th International Semantic Web Conference, Part II, Sydney, NSW, Australia, October 21–25, 2013, H. Alani, L. Kagal, A. Fokoue, P.T. Groth, C. Biemann, J.X. Parreira, L. Aroyo, N.F. Noy, C. Welty and K. Janowicz, eds, Lecture Notes in Computer Science, Vol. 8219: , Springer, (2013) , pp. 277–293. doi:10.1007/978-3-642-41338-4_18. |
4 | [[4]] G.A. Atemezing, Ó. Corcho, D. Garijo, J. Mora, M. Poveda-Villalón, P. Rozas, D. Vila-Suero and B. Villazón-Terrazas, Transforming meteorological data into Linked Data, Semantic Web 4: (3) ((2013) ), 285–290. doi:10.3233/SW-120089. |
5 | [[5]] E.D. Buccio, G.M.D. Nunzio and G. Silvello, A curated and evolving linguistic Linked Dataset, Semantic Web 4: (3) ((2013) ), 265–270. doi:10.3233/SW-2012-0083. |
6 | [[6]] S. Capadisli, S. Auer and A.N. Ngomo, Linked SDMX data: Path to high fidelity Statistical Linked Data, Semantic Web 6: (2) ((2015) ), 105–112. doi:10.3233/SW-130123. |
7 | [[7]] C. Caracciolo, A. Stellato, A. Morshed, G. Johannsen, S. Rajbhandari, Y. Jaques and J. Keizer, The AGROVOC Linked Dataset, Semantic Web 4: (3) ((2013) ), 341–348. doi:10.3233/SW-130106. |
8 | [[8]] I. Celino, Geospatial dataset curation through a location-based game: Description of the Urbanopoly Linked Datasets, Semantic Web 6: (2) ((2015) ), 121–130. doi:10.3233/SW-130129. |
9 | [[9]] C. Chichester, O. Karch, P. Gaudet, L. Lane, B. Mons and A. Bairoch, Converting neXtProt into Linked Data and nanopublications, Semantic Web 6: (2) ((2015) ), 147–153. doi:10.3233/SW-140149. |
10 | [[10]] G. Correndo and N. Shadbolt, Linked nomenclature of territorial units for statistics, Semantic Web 4: (3) ((2013) ), 251–256. doi:10.3233/SW-2012-0079. |
11 | [[11]] E. Daga, M. d’Aquin, A. Adamou and S. Brown, The Open University Linked Data – data.open.ac.uk, in: Semantic Web (2016) (to appear). doi:10.3233/SW-150182. |
12 | [[12]] V. de Boer, J. Wielemaker, J. van Gent, M. Oosterbroek, M. Hildebrand, A. Isaac, J. van Ossenbruggen and G. Schreiber, Amsterdam Museum Linked Open Data, Semantic Web 4: (3) ((2013) ), 237–243. doi:10.3233/SW-2012-0074. |
13 | [[13]] G. de Melo, Lexvo.org: Language-related information for the linguistic Linked Data cloud, Semantic Web 6: (4) ((2015) ), 393–400. doi:10.3233/SW-150171. |
14 | [[14]] S. Dietze, D. Taibi and M. d’Aquin, Facilitating scientometrics in learning analytics and educational data mining – the LAK dataset, Semantic Web (2016) (to appear). doi:10.3233/SW-150201. |
15 | [[15]] J. Eckle-Kohler, J.P. McCrae and C. Chiarcos, LemonUby – A large, interlinked, syntactically-rich lexical resource for ontologies, Semantic Web 6: (4) ((2015) ), 371–378. doi:10.3233/SW-140159. |
16 | [[16]] R.D. Gratta, F. Frontini, F. Khan and M. Monachini, Converting the PAROLE SIMPLE CLIPS lexicon into RDF with lemon, Semantic Web 6: (4) ((2015) ), 387–392. doi:10.3233/SW-140168. |
17 | [[17]] T. Heath and C. Bizer, Linked Data: Evolving the Web into a global data space, in: Synthesis Lectures on the Semantic Web, Morgan & Claypool, (2011) . doi:10.2200/S00334ED1V01Y201102WBE001. |
18 | [[18]] K. Höffner, M. Martin and J. Lehmann, LinkedSpending: OpenSpending becomes Linked Open Data, Semantic Web 7: (1) ((2016) ), 95–104. doi:10.3233/SW-150172. |
19 | [[19]] A. Isaac and B. Haslhofer, Europeana Linked Open Data – data.europeana.eu, Semantic Web 4: (3) ((2013) ), 291–297. doi:10.3233/SW-120092. |
20 | [[20]] K. Janowicz, P. Hitzler, B. Adams, D. Kolas and C. Vardeman, Five stars of Linked Data vocabulary use, Semantic Web 5: (3) ((2014) ), 173–176. doi:10.3233/SW-140135. |
21 | [[21]] T. Kauppinen, G.M. de Espindola, J. Jones, A. Sánchez, B. Gräler and T. Bartoschek, Linked Brazilian Amazon rainforest data, Semantic Web 5: (2) ((2014) ), 151–155. doi:10.3233/SW-130113. |
22 | [[22]] H. Khrouf and R. Troncy, LinkedSpending: OpenSpending becomes Linked Open Data. Semantic Web (2016) (to appear). doi:10.3233/SW-150172. |
23 | [[23]] J. Lehmann, R. Isele, M. Jakob, A. Jentzsch, D. Kontokostas, P.N. Mendes, S. Hellmann, M. Morsey, P. van Kleef, S. Auer and C. Bizer, DBpedia – A large-scale, multilingual knowledge base extracted from Wikipedia, Semantic Web 6: (2) ((2015) ), 167–195. doi:10.3233/SW-140134. |
24 | [[24]] E. Mäkelä, K. Hypén and E. Hyvönen, Fiction literature as Linked Open Data – the BookSampo dataset, Semantic Web 4: (3) ((2013) ), 299–306. doi:10.3233/SW-120093. |
25 | [[25]] M. Martin, C. Stadler, P. Frischmuth and J. Lehmann, Increasing the financial transparency of European Commission project funding, Semantic Web 5: (2) ((2014) ), 157–164. doi:10.3233/SW-130116. |
26 | [[26]] A. Meroño, A. Ashkpour, C. Guéret and S. Schlobach, CEDAR: The Dutch historical censuses as Linked Open Data, Semantic Web (2016) (to appear). |
27 | [[27]] J.A. Miñarro-Giménez, M.E. Aranguren, B. Villazón-Terrazas and J.T. Fernández-Breis, Translational research combining orthologous genes and human diseases with the OGOLOD dataset, Semantic Web 5: (2) ((2014) ), 145–149. doi:10.3233/SW-130109. |
28 | [[28]] N. Queralt-Rosinach, T. Kuhn, C. Chichester, M. Dumontier, F. Sanz and L.I. Furlong, Publishing DisGeNET as nanopublications, Semantic Web (2016) (to appear). doi:10.3233/SW-150189. |
29 | [[29]] M. Sabou, I. Arsal and A.M.P. Brasoveanu, TourMISLOD: A tourism Linked Data set, Semantic Web 4: (3) ((2013) ), 271–276. doi:10.3233/SW-2012-0087. |
30 | [[30]] M. Salvadores, P.R. Alexander, M.A. Musen and N.F. Noy, BioPortal as a dataset of linked biomedical ontologies and terminologies in RDF, Semantic Web 4: (3) ((2013) ), 277–284. doi:10.3233/SW-2012-0086. |
31 | [[31]] G. Sérasset, DBnary: Wiktionary as a Lemon-based multilingual lexical resource in RDF, Semantic Web 6: (4) ((2015) ), 355–361. doi:10.3233/SW-140147. |
32 | [[32]] M.A. Sherif and A.N. Ngomo, Semantic Quran, Semantic Web 6: (4) ((2015) ), 339–345. doi:10.3233/SW-140137. |
33 | [[33]] N. Simou, J. Evain, N. Drosopoulos and V. Tzouvaras, Linked European television heritage, Semantic Web 4: (3) ((2013) ), 323–329. doi:10.3233/SW-130104. |
34 | [[34]] M. Solanki, J. Skarka and C. Chapman, Linked data for potential algal biomass production, Semantic Web 4: (3) ((2013) ), 331–340. doi:10.3233/SW-130105. |
35 | [[35]] C. Torniai, D. Bourges-Waldegg and S. Hoffmann, eagle-i: Biomedical research resource datasets, Semantic Web 6: (2) ((2015) ), 139–146. doi:10.3233/SW-130133. |
36 | [[36]] M. Vafopoulos, M. Meimaris, I. Anagnostopoulos, A. Papantoniou, I. Xidias, G. Alexiou, G. Vafeiadis, M. Klonaras and V. Loumos, Public spending as LOD: The case of Greece, Semantic Web 6: (2) ((2015) ), 155–164. doi:10.3233/SW-140155. |
37 | [[37]] D. Vila-Suero, B. Villazón-Terrazas and A. Gómez-Pérez, datos.bne.es: A library linked dataset, Semantic Web 4: (3) ((2013) ), 307–313. doi:10.3233/SW-120094. |
38 | [[38]] M. Villegas and N. Bel, PAROLE/SIMPLE ‘lemon’ ontology and lexicons, Semantic Web 6: (4) ((2015) ), 363–369. doi:10.3233/SW-140148. |
39 | [[39]] J. Weaver and P. Tarjan, Facebook Linked Data via the Graph API, Semantic Web 4: (3) ((2013) ), 245–250. doi:10.3233/SW-2012-0078. |
40 | [[40]] P. Westphal, C. Stadler and J. Pool, Countering language attrition with PanLex and the Web of Data, Semantic Web 6: (4) ((2015) ), 347–353. doi:10.3233/SW-140138. |
41 | [[41]] B. Zapilko, J. Schaible, P. Mayr and B. Mathiak, TheSoz: A SKOS representation of the thesaurus for the social sciences, Semantic Web 4: (3) ((2013) ), 257–263. doi:10.3233/SW-2012-0081. |
42 | [[42]] A. Zaveri, J. Lehmann, S. Auer, M.M. Hassan, M.A. Sherif and M. Martin, Publishing and interlinking the Global Health Observatory dataset – towards increasing transparency in Global Health, Semantic Web 4: (3) ((2013) ), 315–322. doi:10.3233/SW-130102. |