MMoOn Core – the Multilingual Morpheme Ontology
Abstract
In the last years a rapid emergence of lexical resources has evolved in the Semantic Web. Whereas most of the linguistic information is already machine-readable, we found that morphological information is mostly absent or only contained in semi-structured strings. An integration of morphemic data has not yet been undertaken due to the lack of existing domain-specific ontologies and explicit morphemic data. In this paper, we present the Multilingual Morpheme Ontology called MMoOn Core which can be regarded as the first comprehensive ontology for the linguistic domain of morphological language data. It will be described how crucial concepts like morphs, morphemes, word forms and meanings are represented and interrelated and how language-specific morpheme inventories can be created as a new possibility of morphological datasets. The aim of the MMoOn Core ontology is to serve as a shared semantic model for linguists and NLP researchers alike to enable the creation, conversion, exchange, reuse and enrichment of morphological language data across different data-dependent language sciences. Therefore, various use cases are illustrated to draw attention to the cross-disciplinary potential which can be realized with the MMoOn Core ontology in the context of the existing Linguistic Linked Data research landscape.
1.Introduction
Morphological language data (MLD) plays a crucial role across various interdisciplinary research fields. Traditionally, linguists have fundamentally studied morphology on both language-independent and language-specific levels for centuries in order to investigate the underlying mechanisms that a) allow new words to emerge that are not yet recorded in dictionaries (i.e. word formation), b) are required to alter words so that they take the appropriate form within a certain syntactic environment (i.e. inflection) and c) explain to what extent languages structurally differ in encoding lexical or grammatical meanings within words (i.e. comparative linguistics). This work is the basis for the far younger research field of natural language processing (NLP) which strives to apply linguistic knowledge on morphology (in conjunction with other linguistic areas) on large amounts of text in order to automatically analyze, process or create natural language content. While the methods and aims of linguistics and NLP differ, both sciences can highly benefit each other. Within an ideal cycle of interdisciplinary exchange NLP would take the insights on morphology provided by linguists, apply them to large amounts of text and feed back their results to the linguists who could refine their studies on morphology, which in turn would lead to a better research basis that can be taken up by NLP research again.
Both research fields heavily rely on MLD. The realization of the described scientific exchange and advancement is, however, prevented because of the existing data silos on both sides which use many different and non-interoperable data formats, thus, impeding an easy data transfer. Due to the emergence of Semantic Web technologies this state can change. Being based on the principles of Linked Data, they have proven to evoke true data-driven interdisciplinarity for research domains shared by different sciences. This research manifests itself in the area of Linguistic Linked Open Data (LLOD) which was initiated in 2010 with the foundation of the Working Group on Open Data in Linguistics (OWLG) [9,41]. Since then a significant rise of language data on the Semantic Web emerged. Academic, industrial and technological interest into Linguistic Linked Data appeared and materialized in three areas: (1) W3C community groups such as Linked Data for Language Technology (LD4LT)11 or BPMLOD,22 and (2) European research projects such as LIDER,33 Falcon44 or FREME55 as well as (3) scientific workshops and special issues such as the workshop series on Linked Data in Linguistics,66 the Multilingual Semantic Web workshop series77 or the special issue of the Semantic Web Journal on Multilingual Linked Open Data [27].88
A cross-disciplinary usage of LLOD has already been proven to be achievable in the case of the OntoLex-lemon model99 [40] which successfully unified linguistic and NLP research data for lexical language data (LLD). However, a similar approach for MLD is not yet established. While a plethora of linguistic resources1010 for the LLD domain exists and is highly reused, there is still a great gap for equivalent morphological datasets and ontologies [6,27]. Therefore, the aim of this paper is to present the Multilingual Morpheme Ontology, in short MMoOn Core. The goal of the MMoOn Core ontology is to represent the domain of morphology in a granular way and to assign semantics at the appropriate subword layers in order to derive compositional semantics on the morph, morpheme and word levels. In particular it enables the representation of the morphemes including their written representations and meanings as well as their relations to the words in which they can occur. It is designed to meet the documentary needs of linguists and the applicatory needs of NLP researchers alike. MMoOn Core serves as an extensible schema conceptualizing the domain of morphology and is not bound to any specific natural language but also enables the creation of language-specific MMoOn morpheme inventories. Because of the language-independent conceptualization as well as the evolutionary process of the model, MMoOn Core is suitable for describing any inflectional language. Multilingualism is accounted for automatically since the created MMoOn morpheme inventories are inherently interconnected through the MMoOn Core ontology. With a rising number of morpheme inventories multilingual interlinking will constantly increase over time, hence, the name Multilingual Morpheme Ontology. Ultimately, MMoOn Core has been created to serve as a shared semantic model for representing MLD and to enable the exchange, reuse and enrichment of MLD across different data-dependent language sciences.
Extracting and explicating the morphological semantics of words, however, requires not only a domain expert with detailed linguistic knowledge about morphology but also close to native-speaker level knowledge about the language. Even though ontologies such as the OntoLex-lemon model [40], LexInfo [11], OLiA [8], or GOLD [18] partially define a minimal RDF vocabulary to describe morphemes and morphological data as such, a dedicated morpheme ontology capturing and formalizing semantics is still missing.
This becomes obvious through the fact that morphological information is predominantly still attached to the lexeme (the unit that carries lexical meaning) or the whole word form (cf. Example 11111) and not to the morphological segment (cf. Example 2). The current research gap has two dimensions: First, none of the above-mentioned ontologies provides sufficiently granular terminology to properly describe and tag word segments and second, interoperable morphological data is consequently not available.
In contrast to digital and Linked Data dictionaries or lexicons, morphemic language resources are mostly available in layout-centric formats, such as HTML website contents, PDF documents, tables or even only in printed media. What is more, the domain of morphology is to a large extent treated by linguists who do not only differ in their understanding of this linguistic area but also compile morphological data with a focus on consumption by humans and not on machine processability. The creation of the MMoOn Core model consequently strives to tackle these challenges and will add the following contributions:
– Provide a fine-grained and extensive semantic model for representing MLD suitable for linguistic and NLP tasks.
– Publication of MMoOn Core as a language-independent conceptualization of the MLD domain as a freely available, reusable and extensible linguistic resource.
– Linking of MMoOn Core to already existing linguistic data models.
– First compilation of derivational meanings.
– Representation of morphemic glosses as Linked Data.
– Usage of MMoOn Core as a unifying building block to compile language-specific morpheme inventories which:
∗ integrate heterogeneous data sources with semantic consistency,
∗ provide resource descriptions for word forms and morphemic language data,
∗ interrelate language elements across the morph, word form and lexeme level,
∗ include direct extensions of the vocabulary with language-specific meanings,
∗ are automatically multilingually interconnected through an underlying shared semantic,
∗ result in a compilation of natural language data in a machine-readable manner by adhering to Linked Data principles and interlinking.
The remainder of the paper is structured as follows: Section 2 states the motivation and background and is followed by an outline of related work in Section 3, also pointing to gaps in existing resources. After introducing a brief domain analysis in Section 4, the main part of the paper – the Multilingual Morpheme Ontology – will be presented in detail in Section 5. This part includes its architectural setup, design principles as well as its basic elements. A more detailed comparison of MMoOn Core to OntoLex-lemon is provided in Section 6 by taking a closer look at the currently developing morphology module. Furthermore, use cases for the application of MMoOn Core for linguistic and NLP research will be outlined in Section 7. Finally, the paper closes with concluding remarks and a prospect of the future work in Sections 8 and 9.
2.Motivation and background
The need for the development of a data model that is able to describe the morphemic inventories of natural languages was expressed by two major research communities. The first one centers around the community groups OWLG,1414 LD4LT1515 and BPMLOD1616 and consists of researchers coming from the areas of computational linguistics, NLP, machine translation and language technologies. They express a high demand on interoperable and fine-grained (multilingual) linguistic data that models subword information and which can be integrated in and applied to the existing content and language analyzing systems. The above-mentioned groups also expressed a strong preference for free and open data to increase reusability and reproducibility.
The second group of researchers involves linguists whose main subject area is the investigation of natural language per se. Especially linguists who document endangered and under-resourced languages as well as general comparative linguists both produce and rely on adequate linguistic data. A rising awareness of methodological standards in the compilation of language data has emerged in linguistic research “for the sake of [the] speech communities [of languages threatened by extinction] and their interest in their cultural tradition and for the sake of the very database of the discipline itself” [36]. In linguistics the usage of interlinear or morpheme-by-morpheme glosses as a means for the representation of the segments and meanings of text are an established common practice. Due to their widespread application, efforts of standardization have been introduced [13,37]. As a result, a great amount of interlinear-glossed text resources exist in linguistic databases or as text examples in linguistic publications. Unfortunately, this wealth of data is not easily accessible or reusable due to the (1) technical heterogeneity, (2) license restrictions or unavailability of licenses, and (3) nonformal description of linguistic documentation. Here, the field of linguistic documentation is in need of a model that allows for the (automatic) creation, retrieval, processing and publishing of its morphological data in compliance with the granularity of the linguistic representation levels.
In order to fulfill the demands of both research communities just outlined, the MMoOn Core ontology has been created. It presents a new vocabulary which is easily integrable into already existing lexical resources and expressive enough to capture the various correspondences between subword elements and their associated meanings. Hence, all specific MMoOn language inventories will contribute to the development of natural language analyzing methods and tools. At the same time, MMoOn allows linguists to adequately represent their high-quality language data using a vocabulary with well-defined semantics and in a data format that ensures interoperability with a large range of formats and systems. Thus, we believe that, both the NLP research area and linguistics as an empiric discipline will benefit from the reuse of the MMoOn Core vocabulary.
The developmental approach underlying the creation process of the MMoOn Core ontology is grounded in a thorough domain analysis (cf. Section 4) and guided by a defined set of requirements as well as design choices (which are explained in detail in Section 5.3). To this extent, it has been developed from scratch as a standalone ontology without originating from any existing vocabulary or model. On the contrary, the aim of the MMoOn Core ontology is to unite morphological data represented in differing formats or underlying varying linguistic theories and descriptions. Since MMoOn Core further pursues the aim to function as a language-independent domain ontology for MLD, the generalizable elements, relations and characteristics which have been identified for the linguistic research field of morphology [4,25] have been derived and transformed within the semantic modeling of the ontology. These include linguistic concepts such as affix, inflection, derivation, segmentation, meaning or interlinear glossing as described in the foundational linguistic works about morphology and are not only assumed to be applicable to a wide range of languages but also to be familiar concepts to linguists. Under consideration that linguists create the most fine-grained MLD, MMoOn Core is motivated by the provision of as many descriptive domain elements as possible to keep the entry barrier into working with RDF for linguists as low as possible. To conclude, the MMoOn Core ontology can be regarded as the first extensive representation model for MLD to create inventories of the smallest meaningful elements of language similar to dictionaries or lexical databases within the lexical data domain.
3.Gaps in existing resources and related work
An inventory of morphemes requires an appropriate data model on the one side and morphemic data on the other side. In what follows an overview will be given that investigates the applicability of existing linguistic ontologies as well as existing Linked Data morphological resources but also datasets and sources that are based on other formats.
3.1.Vocabularies modeling MLD
Within the last few years, ontologies emerged that contain vocabularies partially describing morphological aspects of language. These include the lemon model [39] and the decomp and ontolex submodules of the OntoLex-lemon model [40], LexInfo [11], OLiA [8] and the GOLD [18] ontology. Even though, none of these vocabularies were explicitly designed to capture the domain of MLD, they include conceptual information on the meaning side of morphemes and/or information of morphemic elements. For that reason the MMoOn Core ontology has been interlinked to some of these vocabularies (cf. Section 5 and Section 6) in order to comply to the Semantic Web best practices for reusing existing data models. In this context LexInfo, OLiA and GOLD are mainly reusable as terminological datasets providing the theoretical description of the linguistic concepts involved in lexicography and morphology.
With regard to the representation of subword units lemon and OntoLex-lemon provide elements that belong to the domain of MLD. Lemon was the first model to offer a morphology module1717 that allows the representation of different forms of lexical entries including lemon:Part which describes affixes. This module evolved to be a standalone ontology called LIAM (Lemon Inflectional Agglutinative Morphology).1818 However, this vocabulary focuses on a regular expression based description of morphological processes and pattern transformation [40]. The crucial information – namely the morphemic segment – is contained as string in the data type property liam:rule and, therefore, not machine processable and not further interrelatable to other segments. In addition to that, the applicability of lemon and the LIAM ontology with regard to language-specific modeling of morphological data has been questioned in previous work [7].
The latest advancement in modeling MLD is presented in the W3C report of the OntoLex-lemon model specification.1919 Especially the ontolex and decomp modules are highly reused for representing lexical data but also compositional morphology. Still, the morphological elements such as decomp:Component and ontolex:Affix are too coarse grained and mainly intended to represent compounding morphology. Further, specific elements like roots and stems or more specific affixes like the transfix or empty morph are missing together with the necessary relations that represent the segmentation steps and relations between the morphemic elements. Additionally, word forms are only encoded as strings via the ontolex:otherForm datatype property which prohibits a further specification of the derivational and inflectional segments a word form may consist of. Nonetheless, the OntoLex-lemon model serves as the ontological standard for modeling linguistic language data to a large extent of the LLOD community and is highly reused. For that reason – and because of the significant overlap of the two domains of lexical and morphological language data – it was out of question to interconnect MMoOn Core with OntoLex-lemon in order to enable an interconnection but also the supplementation of both domain models [32] (cf. Section 6).
The recently published Ligt vocabulary has to be mentioned as a possibility for representing morphological data as well [10]. It is specialized to enable the transformation of interlinear glossed text into RDF data. In particular, it can be used to transform resources based on Toolbox, FLEx and Xigt (eXtensible Interlinear Glossed Text) to Ligt-RDF. The main contribution of Ligt is the unification of several heterogeneous interlinear glossed text resources based on different formats within a homogeneous RDF data graph. With respect to its usability for representing MLD, however, the Ligt vocabulary differs fundamentally from MMoOn Core and OntoLex-lemon in that the morphemic elements it describes identify single occurrences of morphs within an interlinear text similar to tokens within a corpus. As a result, the only element relevant for the domain of MLD in Ligt is the class ligt:Morph which is specified with a string and for its position within the morph tier, paragraph and document it occurs. No semantics is established interrelating ligt:Morph resources or specifying them, e.g. as suffixes, derivational or inflectional morphs or for their meanings. In fact, a gloss tier that would interrelate the morphs with the abstract identities of their morphemic meanings, i.e. the glosses, is not provided in Ligt. Since the objective of Ligt is to represent unique occurrences of morphs instead of unique morphemic concepts that can be applied to an unlimited number of occurrences in primary language data, reasoning over the ligt:Morph resources to obtain more insights is not possible. Whereas MMoOn Core is intended to provide a vocabulary for obtaining domain knowledge about the morphological inventory of a language, in the realm of the MLD domain Ligt-based datasets rather function as the attestations for the morphs of a language. In that respect, the Ligt creators deliberately decided to consider the provision of comprehensive MLD semantics out of scope for this vocabulary in favor of gaining unified representations of various interlinear glossed text formats. This choice is especially advantageous because it not only facilitates the application of the vocabulary in practice but also allows for an easier interlinking – if required – with already existing semantically richer domain vocabularies for MLD, including MMoOn Core. Even though no published dataset based on Ligt exists to date, the significance and need of such datasets is already obvious given that interlinear text resources are quite often the only existing documented language resources for less- or under-resourced languages (cf. Section 3.3). In this respect Ligt datasets could be potential sources to derive an attested MMoOn morpheme inventory for a language from interlinear text resources, similarly to a dictionary that is derived from corpus data.
3.2.Overview of Linked Data resources
So far, two datasets have been created and published based on the MMoOn Core model and architecture (cf. Section 5.2), i.e. the Hebrew Morpheme Inventory [34] and the Xhosa RDF dataset [5] together with a dictionary alignment to Kalanga and Ndebele lexical datasets [17].
To the best of our knowledge, all other existing Linked Data resources including MLD are based on the lemon/LIAM model or the OntoLex-lemon model. As a consequence, these datasets contain morphological data only to a limited extent, e.g. the decomposition of compounds or unrelated affix resources (e.g. [16]).
As a specific example for a dataset containing inflectional language data, the Dbnary “morpho” Wiktionary extractions for German, French, English and Serbo-Croation need to be mentioned.2020 These datasets contain the Wiktionary headwords and inflected word forms in lemon-RDF and are annotated for their inflectional meanings with OLiA [46]. However, in a strict view of the domain of MLD (cf. Section 4) this representation of morphological data covers only the morphological meanings as word form annotations instead of segmented morphs that correspond to a specific meaning. Notwithstanding the fact that the Wiktionary data does not contain segmentations of word forms, an adequate representation of these segments and their interrelation to each other and within the word forms is not possible with the existing vocabularies, with the exception of the MMoOn Core ontology.
3.3.Overview of non-Linked Data resources
Due to the fact that the Linked Data paradigm is in comparison to linguistic research and documentation very young, it is not surprising that the majority of MLD exists in non-Linked Data formats. In fact, the largest part of linguistic data is preserved in documents. However, this overview of MLD will not touch upon such data in unstructured formats but focuses on structured data only. Among the datasets which can be found a high variance with regard to aspects like accessibility, data quality, reusability, complexity of morphological data, covered languages and data format can be observed:
a) MLD in linguistic field work data: This kind of data entails fine-grained, complex and segmented MLD documented in interlinear glossed texts that are edited with specific tools like FieldWorks2121 or FLEx. Usually the data is compiled by one linguist for an undocumented, small or endangered language. Hence, the resulting datasets are of high quality but often not very large and commonly meant for linguistic research. The formats of the field linguists’ tools are very specific and the output dataset is not seldom published at all. Instead, only a part of it is used for giving language examples in resulting text publications, i.e. in PDF documents. However, efforts like TypeCraft2222 [2] and Dictionaria2323 emerged that aim at providing an open and data driven publication platform for publishing full FieldWorks datasets. What is more, they also provide the data in common formats like XML, CSV, JSON and XLS.2424
b) MLD as a part of large language databases: For large and well documented languages usually more linguistic data is available to date. Whole research groups and institutes are devoted to collecting and editing resources such as word lists, dictionaries and corpora and also strive to organize and manage all the linguistic data available in large databases. These datasets also cover MLD like word forms, inflection tables and affix lists. These language resources are the outcome of a collaborative work between linguists and computer linguists that merge and structure manually compiled data as well as automatically transformed or created language data. Examples include the Oxford Online Database of Romance Verb Morphology,2525 the work of the Surrey Morphology Group 2626 and the French project ALEXINA2727 [45] which develops morphological NLP lexicons. For German language data in particular, the German Institute of Language (IDS2828) poses a considerable source for basic words and word forms2929 and also provides the dictionary of affixes.3030
In this context, the Lexical Markup Framework (LMF) [20,21] has to be mentioned as well. It enables the representation of machine-readable dictionaries (MRD) and NLP lexicons and has been applied to create numerous datasets, e.g. ALEXINA, including morphological data based on the morphological extension of the LMF core model. It provides two strategies for representing word forms. The first one applies to an extensional listing of all forms of a lexical entry which are specified for linguistic categories and values. This approach, however, does not explicitly contain morphemes. The second strategy allows for an intensional modeling of so called morphological patterns and inflectional paradigms. These are formalized in detail and specific to lexical entries, however, with no explicit listing of the forms in the lexicon. While the usage of the morphological extension of LMF is very powerful in terms of machine-processing, it is less suitable as a human-understandable basis for a linguistic analysis of the morphology of a language. The lexicon-centric view on morphology additionally reduces morphology to the lexical entry level and impedes the identification of the smallest meaning bearing units of a language on the word form level. Moreover, LMF-based databases are often realized in structured formats such as XML and very customized. As a result, a considerable effort to understand the data is required and a direct data reuse and interoperability is, therefore, reduced.
c) MLD as morphological segmentation tool output: One of the most challenging tasks in computational linguistics is the creation of segmentation tools. Irrespective of the accuracy and quality of the segmentations, such data outputs also create MLD which can be used in several NLP tasks and linguistic research alike. The IDS developed the Morphisto segmentation tool3131 which is freely available. It analyzes a word form with regard to its grammatical features, the lexical word it belongs to as well as it identifies prefixes and suffixes. Nonetheless, the corresponding morphemic parts of the word, even though involved in the analysis process, are not given in the segmented output. Furthermore, morphological data and tools are provided by the Morpho Challenge workshops3232 which aim at discovering morphemes from text input by statistical machine learning algorithms. One considerable development in this area is the Morfessor tool.3333 In contrast to Morphisto, Morfessor is a generic language-independent segmentation tool that outputs a morphological lexicon on the basis of probabilistic measurements. While the initial effort did not go beyond the identification of morphemes as string sequences [14], it has been extended to consider meaning parameters as well [15]. Albeit, these comprise rather formal aspects again, such as frequency and length, with the authors admitting that “so far the modeling of meaning has only been touched upon” [15]. It has to be stressed that, even though, such tools present a promising method for obtaining MLD for any language, the actual application of these tools requires a lot of time, i.e. time to understand the customized (and often proprietary) output data as well as time for the postprocessing needed for the quality assessment or even data clean up.
The presented overview of Linked and non-Linked Data resources for MLD illustrates two research fields which develop independently from another, even though, both would increase their scientific outcomes by joining their methods and resources as it has been shown for the domain of lexical language data already. In line with the need for lexical data there is also a demand for morphological data that applies both to the language specific morphological domain requirements and to cross-lingual interoperable data modeling. Given the current state of the art, Linked Data vocabularies are not suitable enough to represent the various existing morphological data that will stay isolated and hard to reuse without the unifying RDF data format.
Fig. 1.
Overview of the linguistic domain of morphology with the English example lexeme play (verb).
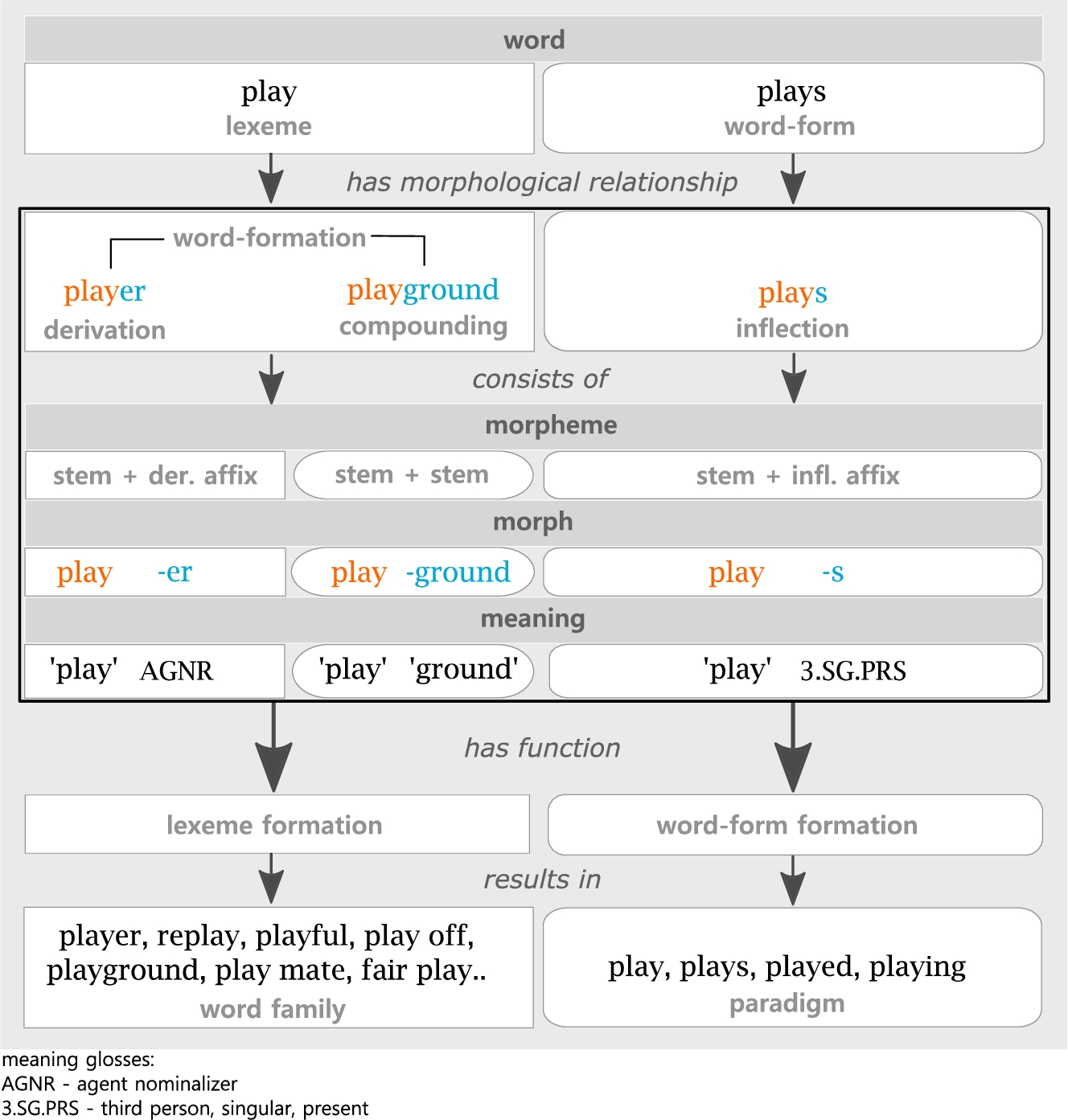
4.Domain analysis
The development of MMoOn Core is based on the following domain analysis for MLD. It has been conducted in order to clarify and decide which linguistic elements and relations need to be represented. The linguistic domain of morphology deals with the internal structure of words including the elements and meanings of which they consist, i.e. the morphs and morphemes of a language. In the context of MMoOn Core we define the term morpheme as the smallest component of a word that contributes some sort of meaning, or a grammatical function to the word to which it belongs, whereas the term morph is defined as the perceivable side, i.e. the written or spoken realization, of a single morpheme. Just as other linguistic domains, e.g. syntax or phonology, the study of morphology can either refer to that part of language in general or to the morphological system of a specific language. For the purpose of outlining the domain this section is concerned with the first sense of morphology, although, the second meaning plays a crucial role when it comes to the description and investigation of the MLD of a specific language.
Figure 1 gives a basic overview of the conceptualization of the domain. It depicts a condensed summary based on linguistic works that outline the area and study of morphology in a general way [4,25] and which can be assumed to portray the common agreement among linguists as to what elements and relations are part of morphology. The word level is divided into lexemes and word forms. The former are abstract words which contain a core meaning and are usually listed as entries in dictionaries. The latter are concrete realizations of a lexeme which combine the lexical core meaning with additional grammatical meanings that are relevant for their embedding in a syntactic environment. Lexemes and word forms can enter two morphological relationships, i.e. word formation and inflection, respectively. Word formation can be further divided into derivation and compounding. These terms address the morphological components of which they can consist. The major part of morphology is then devoted to “the study of the systematic covariation in the form and meaning of words that can be identified by segmentation” [25]. For the English example of the verb play it is shown in Fig. 1 that these segments can be divided into free and bound realizations, i.e. stems and affixes. Stems are morphs that can usually stand alone whereas affixes are always attached to a stem. The two lexemes player and playground and the word form plays all contain the lexical stem play. The difference between these three types of words lies in their morphological building patterns. Derived lexemes consist of a stem and a derivational affix, which is in this example the suffix -er that encodes the meaning of ‘agent noun’ and also entails a word-class change from verb to noun. The morph -er is very productive in English and can be used to form a variety of agent nouns from verbs, e.g. winner (noun) from win (verb) or writer (noun) from write (verb). Compound lexemes, in contrast, consist of two stems, i.e. play and ground in the given example. Both processes of word formation have the function to form new lexemes, by extending the meaning of a lexeme with additional meaningful elements. As a result, word families of lexemes emerge which contain all lexemes that share the same lexical core meaning. Accordingly, all lexemes of the word family play in Fig. 1 are derivatives or compounds encoding some extended but related lexical meaning of the verb play.
In contrast to word formation, inflection does not result in new lexemes. Rather, it involves the morphological modification of a lexeme in order to use the word form of it in a certain syntactic environment. Consequently, word forms consist of a lexical stem and an inflectional affix. In the example plays is a word form of the lexeme play and consists of the stem play and the suffix -s which encodes ‘third person’, ‘singular’ and ‘present tense’. Thus, the process of inflection has the function to build word forms of a lexeme. This results in paradigms that contain all word forms that can be build from one lexeme. Usually, an inflectional paradigm is a cross-classification according to the grammatical features involved. These are often linguistic categories such as person, number and tense in inflectional languages. Since English marks only the word forms encoding the third person, singular and present tense with the suffix -s, the paradigm is not very extensive and encompasses only four word forms. Similarly to the derivational affixes, the inflectional affixes occur in other word forms with the same (grammatical) meaning.
Overall, the domain of morphology is mainly concerned with the identification of the smallest meaning bearing units of language and the investigation of their concrete realization, meaning, function, relation to each other and the systematization of the underlying building (ir)regularities.
5.MMoOn Core – the Multilingual Morpheme Ontology
Everything developed by us around MMoOn Core can be accessed under the following websites: http://mmoon.org/ and https://github.com/MMoOn-Project. The ontology is published under http://mmoon.org/core.rdf and open for any kind of reuse under a CC BY 4.0 license. Altogether, the MMoOn Core model comes with 430 classes, 37 object properties, five datatype properties and 301 instances which have been all created manually. An overview of the model is given in Fig. 2 that illustrates the eight main classes and their division into further subclasses. As will be shown in the following subsections, the seemingly large setup of MMoOn Core is well structured and can be used from a reduced extent up to its full possibilities, which will enable a sufficient description of MLD according to the conducted domain analysis.
Fig. 2.
Overview of the MMoOn Core main classes and properties.
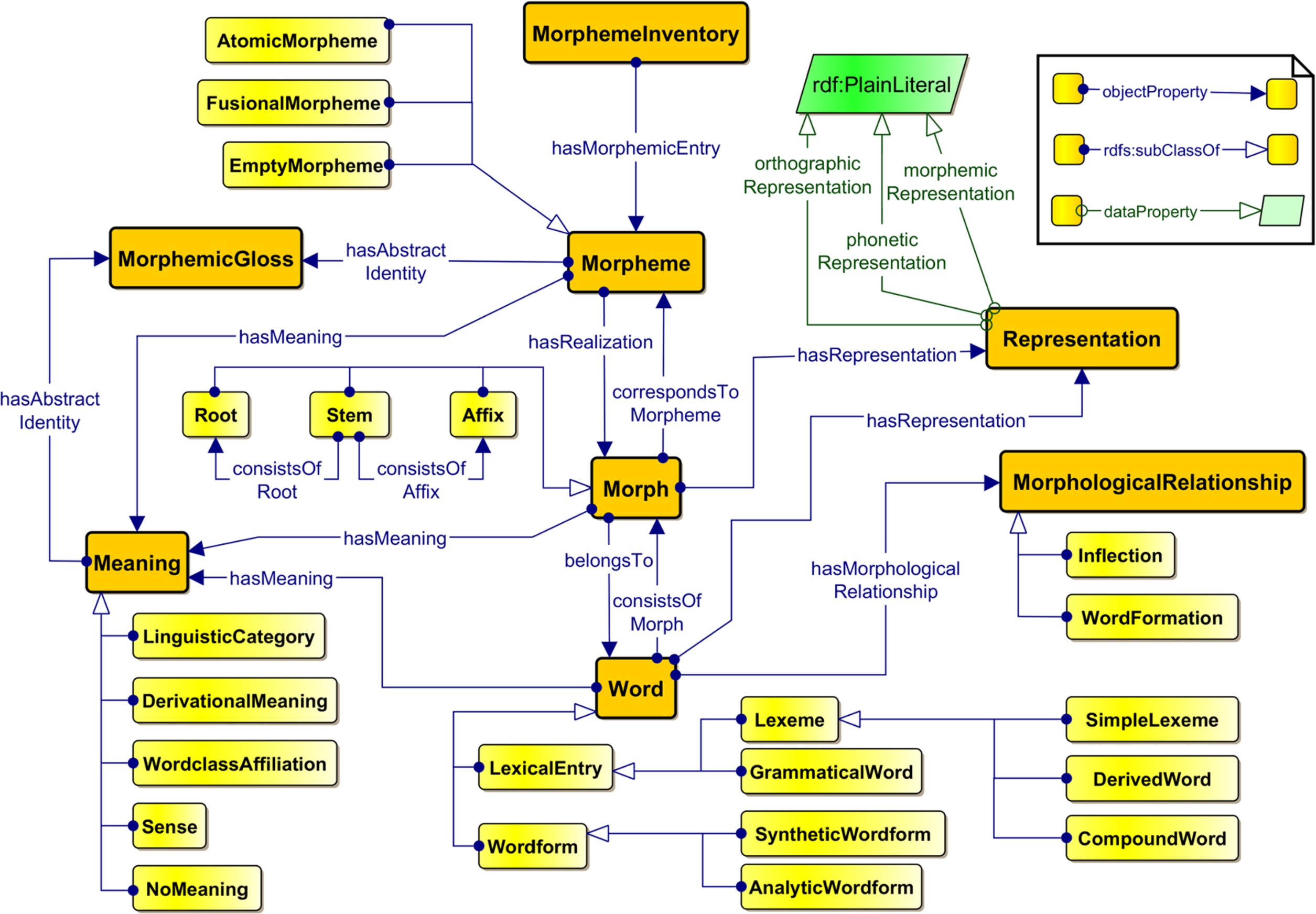
5.1.MMoOn Core basic elements
In the following an overview of the eight main classes and central properties provided in MMoOn Core will be given. Due to the size of the ontology vocabulary it is recommended to additionally consult the ontology file to receive more detailed insights into the definitions and interrelations established between the ontology elements.
5.1.1.Main classes
MorphemeInventory: Each compilation of morphemic data with MMoOn Core will result in a morpheme inventory that is specified for the language of the data by using the object property mmoon:forLanguage. Every MMoOn language inventory should be named according to its given lexvo ISO language code and is an instance of mmoon:MorphemeInventory. Since MMoOn shall describe morphemes, each morpheme inventory consists of mmoon:Morpheme and/or mmoon:Morph resources.
Word: The word is the basic constituent at the phrase level and unit of morphological analysis. MMoOn Core further subdivides this class into mmoon:LexicalEntry and mmoon:Wordform, which both consist of further subclasses (cf. Fig. 2). The mmoon:Word class serves as a very broadly defined superclass subsuming everything that consists of a contiguous sequence of letters or phonemes. In this sense both mmoon:LexicalEntry and mmoon:Wordform are subclasses of mmoon:Word and differ in that the former class instances represent abstract words and the latter class instances represent concrete words. Instances of mmoon:LexicalEntry are, therefore, words as they appear as entries in a dictionary. The two subclasses mmoon:Lexeme and mmoon:GrammaticalWord distinguish between lexical entries that have a lexical or a grammatical meaning. The instances of the class mmoon:Wordform are inflectional variants of mmoon:LexicalEntry instances and represent words as they are used in text or speech [25]. The classification of words in MMoOn Core is more fine-grained than in vocabularies modeling lexical language data. It mainly serves to distinguish words according to their morphological formation. In particular this entails that morphs occurring in mmoon:LexicalEntry instances are morphs that are involved in word formation processes and morphs occurring in mmoon:Wordform instances are part of word form formation processes.
In order to allow for an easy extension of an existing lexical dataset with morphological data, mmoon:LexicalEntry is interconnected with the ontolex:LexicalEntry class via the rdfs:subClassOf property and with gold:LexicalItem via skos:broadMatch.
MorphologicalRelationship: This class serves as a means to specify the relationship between word forms of a lexical entry (inflection) or the relationship between lexical entries of a word family (derivation and compounding). Accordingly, the two subclasses mmoon:Inflection and mmoon:WordFormation are established. Several subclasses for both of them are also provided, e.g. the class mmoon:Declension that can be used to document nominal inflectional paradigms as they are provided in inflection tables. All word forms that are included in such a table can be then associated with its respective declension class, for instance a Latin noun belonging to the first declension paradigm. Similarly, the two classes mmoon:Derivation and mmoon:Compounding, being subclasses of mmoon:WordFormation, provide more specific subclasses that are ready to use. The derived word smallish, for instance, is a lexeme that can be specified for the derivational relation mmoon:DeadjectivalAdjective. This allows for a morphological classification of the words of a language which is usually described in the grammatical sections of language descriptions discussing inflectional paradigms and word families. In this regard, however, the MMoOn Core mmoon:MorphologicalRelationship subclasses are primarily designed to cover an extensional representation of inflection and derivation classes by listing mmoon:Lexeme and mmoon:Wordform instances which are interconnected with the mmoon:hasWordform or mmoon:isDerivedFrom object properties and point to the same mmoon:MorphologicalRelationship instance. An intensional usage of the mmoon:MorphologicalRelationship class is also possible, however, not in an explicit machine-processable manner (as provided in LMF, for instance). Morphological patterns that subsume inflected or derived forms sharing the same transformation processes for inflection or word formation can be only described with rdfs:comment or a similar annotation property. The reason for this is the inability to explicitly specify a mmoon:MorphologicalRelationship class or instance for grammatical or derivational categories contained in the mmoon: Meaning class. Additionally, a specific object property that would allow to interconnect mmoon:Lexeme or mmoon:Wordform instances with each other as prototypical references to the shared morphological patterns would have to be created. In this respect, the generation of word forms and lexemes based on explicitly defined morphological patterns from within an ontology is regarded out of scope of MMoOn Core which – being an ontology – is regarded as a means to the describe and not generate MLD.3434
Moreover, with the two classes mmoon:NoInflection and mmoon:NoWordFormation words that exhibit an inability to undergo certain morphological processes can be explicitly represented.
Morph: The morph resources are concrete realizations of a single morpheme which usually result from segmentation. In the MMoOn Core vocabulary they are the manifestations of the form side of a linguistic sign and as such constitute perceivable elements in the form of graphemes or phonemes. Therefore, a mmoon:Morph has a corresponding mmoon:Morpheme (see below) and together both form one linguistic sign based on a one-to-one correspondence between form and meaning. Several subclasses enable the specification of the morph type, e.g. mmoon:Affix, mmoon:Stem and mmoon:Root. Again, the MMoOn Core vocabulary provides here a more fine-grained classification. Especially the affix subclasses mmoon:Simulfix, mmoon:Transfix, mmoon:EmptyMorph and mmoon:ZeroMorph constitute a valuable addition next to the commonly provided prefix, suffix, infix and circumfix classes that exist already in other vocabularies, e.g. GOLD, OLiA or OntoLex-lemon, but also in MMoOn Core as well. What is unique to MMoOn in addition to these classes, is the possibility to interrelate morph instances with the mmoon:isAllopmorphTo and mmoon:isHomonymTo object properties.
Morpheme: The morpheme class contains the smallest meaning-bearing elements of a language. These comprise all semantically distinct concepts which are encoded by the morph the morpheme realizes, i.e. the morpheme resources are manifestations of the inseparable meaningful side of corresponding morphs in a language. These meanings can be lexical meanings, grammatical meanings or senses. Determined by the occurring kind of morph-to-morpheme correspondence, morpheme resources can be further specified for being 1) a mmoon:AtomicMorpheme, i.e. the realization by the morph resource entails exactly one meaning, or 2) a mmoon:FusionalMorpheme, i.e. more than one meaning is encoded within the morph realizing such a morpheme but these are not separately identifiable by further segmentation, or 3) a mmoon:EmptyMorpheme, which is by definition a morpheme that has no meaning but is realized by an empty morph. This class has been established to explicitly capture the non-existing meaning correspondence of mmoon:EmptyMorph instances and the statement mmoon:EmptyMorpheme mmoon:hasRealization mmoon:EmptyMorph is already provided with the vocabulary for convenience.
Meaning: The mmoon:Meaning class is the largest class in MMoOn Core. It comprises meanings a word, morph or morpheme can be associated with, e.g. mmoon:LinguisticCategory, mmoon:DerivationalMeaning or mmoon:WordclassAffiliation. Since the domain of MLD is concerned with meanings, MMoOn Core aims at providing already a wide range of meanings that are attested among many of the world’s languages. With the advanced usage of the vocabulary it is planned to extend it with meanings that are currently not available in MMoOn Core at the moment but will be necessary for dataset creators of specific languages. The linguistic categories are collected from three different sources, i.e. the OLiA ontology, the GOLD ontology and the LiDo Glossary of Linguistic Terms database.3535 They contain usually obligatory expressed linguistic features such as person, number, tense and case, but also clusivity, relative person or social deixis. In contrast, MMoOn Core is the first vocabulary that also provides and collects derivational meanings which are useful to represent word formation processes. These include, for instance, diminution, inhabitant, aktionsart or applicative. The modeling of word classes as a type of meaning might seem unusual but follows the narrow purpose to provide the possibility to express conversion which is also called zero-derivation. Conversion is regarded as the formation of a lexeme from a lexeme with another part of speech which contains no further derivational meaning except that which is entailed in the word class change, e.g. the noun call derived from the verb (to) call. Further, for describing the meanings of lexemes, stems and roots the mmoon:Sense class can be used. Providing sense resources here, however, exceeds the domain scope of MMoOn Core. Thus, senses must be defined based on existing data or can point to an appropriate external sense resources, e.g. synsets from WordNet RDF, by using the mmoon:senseLink object property. Finally, the class mmoon:NoMeaning is established to explicitly state that an empty morph has no meaning.
MorphemicGloss: The morphemic gloss is the abstract identity of a morpheme and serves as a metalinguistic representation of meanings. MMoOn Core already contains 300 instances of morphemic glosses, most of which are taken from the Leipzig Glossing Rules [13] or from Lehmann’s glossing list [37]. Furthermore, for each mmoon:Meaning class and every instance that will have a type assertion to one of these classes, glosses are established that are interrelated to the meanings, e.g. mmoon:Singular mmoon:hasAbstractIdentity mmoon:MorphemicGloss_SG. The glosses can be also used to represent mmoon:Morpheme resources, e.g. the English morpheme for ‘third person’, ‘singular’ and ‘present tense’ is represented as eng_inv:FusionalMorpheme_3P_SG_PRS mmoon:hasAbstractIdentity mmoon:MorphemicGloss_3P, mmoon:MorphemicGloss_SG, mmoon:MorphemicGloss_PRS. The provision of morphemic glosses and their association to meanings in MMoOn Core fulfills the following three objectives. First, the existence of gloss instances facilitates the data compilation and saves the time for creating glosses. Second, consistency of glosses among different MMoOn morpheme inventory datasets is guaranteed because of a shared set of preassigned glosses. Nonetheless, if necessary or desired, new glosses can be created as well but should be linked via owl:sameAs to the existing MMoOn Core gloss. Finally, the glosses enable a cross-linguistic comparison of how specific meanings are morphologically encoded across different languages.
Representation: In this class the linguistic representations of mmoon:Morph and mmoon:Word resources are collected as abstract representation instances, e.g. eng_inv:Suffix_er mmoon:hasRepresentation eng_inv:Rep_er. These instances can be further specified for their string realization with the four different datatype properties mmoon:orthographic-, phonetic- and morphemicRepresentation as well as mmoon:transliteration. Morphemic representation literals include the marking of the morph boundary according to the defined typographic conventions of mmoon:morphemicRepresentation that demarcate them from plain orthographic representations, e.g. the instance eng_inv:Rep_er points to the morphemic representation literal “-er”@en. For the reason of consistency the morphemic representation for the mmoon:ZeroMorph instances, which have by definition no phonological and orthographic representation, has been already established, i.e. mmoon:Representation_ZM mmoon:morphemicRepresentation "-Z"^^xsd:string. Together with the mmoon:Meaning resources the mmoon:Representation data enables the identification and explication (cf. Section 5.1.2) of allomorphs (two morphs that link to the same meaning but to different representations) and homonymous morphs (two morphs that link to the same representation but to different meanings) within a dataset.
As this overview of the eight main classes shows, the class hierarchies in MMoOn Core are very elaborate. Irrespective of the level of granularity of the source data both the very specific subclasses and the more general superclasses enable the representation, identification and classification of the linguistic elements that are involved in the domain of MLD.
5.1.2.Properties
A key feature of modeling the domain of MLD constitutes a sufficient set of relations that is able to capture the segmentation of words. Altogether, the MMoOn Core vocabulary provides 37 object properties which can be used to state more or less specific relations for modeling the morphemic elements of the data that should be represented. Figure 3 illustrates a part of the example data that has been introduced in Fig. 1 by using the most specific properties, i.e. the subproperties which are lowest within the hierarchy of an object property.
Fig. 3.
Modeling of relations between morphological data with the example segmentation of the word form plays.
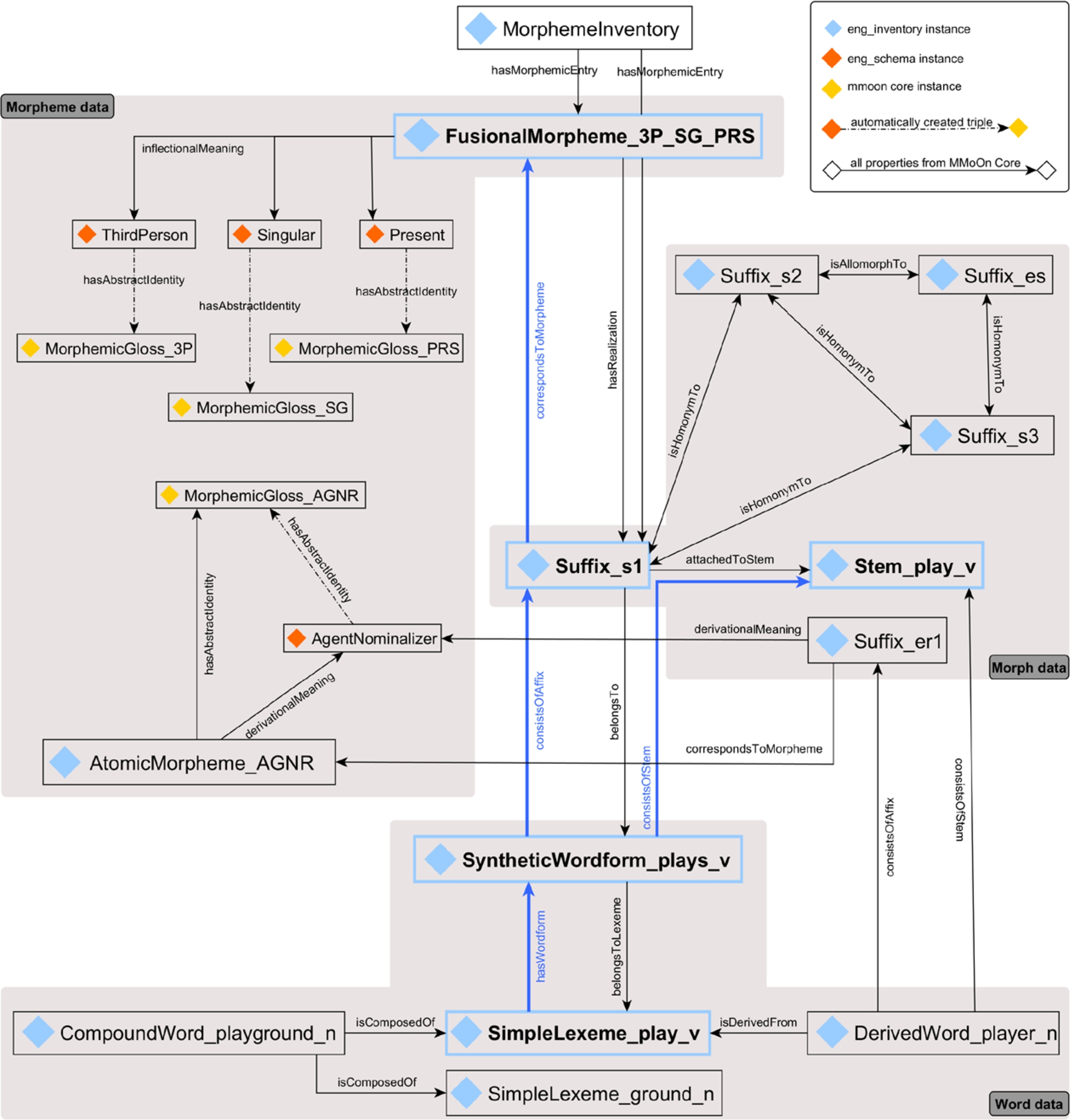
In practice, datasets containing morphological data highly differ in terms of coverage and granularity. As a result, the variety of the created object properties emerged because of the intention to increase the applicability of the MMoOn Core vocabulary to as many differing kinds of morphological datasets as possible. This aspect is not trivial, since morphological data does not exist to the same extent as lexical language data and ranges from simple tables containing lexemes, stems and affixes over texts with interlinear morphemic glosses to morphological segmentation tool outputs. In what follows, it will be first outlined how morphological data is ideally expressed with the MMoOn Core vocabulary and second, further possibilities for deviating data representations will be motivated.
An ideal MMoOn-based dataset contains instances of the three main classes mmoon:Word, mmoon:Morph and mmoon:Morpheme. They are interrelated according to the part of the graph that is highlighted in blue. This case is exemplified for the word form plays in Fig. 3. It is classified as a mmoon:SyntheticWordform which can be segmented into the two mmoon:Morph instances Stem_play_v and Suffix_s1. These in turn are interconnected with their corresponding mmoon:Morpheme instances, in this example Suffix_s1 with FusionalMorpheme_3P_SG_PRS. This modeling is chosen because it enables an explicit distinction between the form and meaning side of subword elements. It resolves a prevalent ambiguity that exists in the discourse about the morphology domain when, for example, speaking of “the third person, singular, present -s morpheme”. Therefore, within the MMoOn vocabulary the -s is referred to as a mmoon:Morph instance and the “third person, singular, present” as a mmoon:Morpheme instance. Since the form and meaning sides of linguistic signs are inseparable, both resources are interrelated with the mmoon:correspondsToMorpheme object property and its inverse property mmoon:hasRealization.
The grey areas in Fig. 3 illustrate how the instances of the three main classes in this ideal modeling can be further described to represent word, morph and morpheme data.
On the word level the interrelation between different types of words can be stated. Word form resources are always interconnected to lexemes by using the property mmoon:belongsToLexeme which is inverse of the property mmoon:hasWordform as exemplified for the instance SyntheticWordform_plays_v. Further, an assignment to an inflectional paradigm can be stated. The property mmoon:inflectionalRelation is used to express which verbal inflection class applies, similar to inflection tables in dictionaries. In the given example the following statement can be realized:
SyntheticWordform_plays_v
mmoon:inflectionalRelation
ex:regularConjugation.
DerivedWord_player_n
mmoon:derivationalRelation
ex:agentNoun.
CompoundWord_playground_n
mmoon:compoundingRelation
ex:nominalCompound.
On the morph level the mmoon:Morph instances as the perceivable side of the morphemes are represented as strings via the three datatype properties mmoon:phoneticRepresentation, mmoon:othographicRepresentation and mmoon:morphemicRepresentation for rendering phoneme, grapheme and morphemic representations. The latter consists of a morphemic boundary marking and the conventional orthographic representation of it, e.g.: Rep_Suffix_s1 mmoon:morphemicRepresentation “-s”@de. It is further possible to interrelate affixes with stems or roots by using the superproperties mmoon:attachedTo and mmoon:consistsOfMorph or their more specific subproperties, e.g.:
Suffix_s1
mmoon:attachedToStem
Stem_play_v.
Stem_player_n
mmoon:consistsOfAffix
Suffix_er2;
mmoon:consistsOfRoot
Root_play.
Suffix_er1
mmoon:isAllomorphTo
Suffix_er2.
On the morpheme level the meanings that are encoded by the morphs are assigned to the mmoon:Morpheme instances. In accordance to this, Suffix_s1 corresponds to the fusional morpheme FusionalMorpheme_3P_SG_PRS which is further specified with the object property mmoon:inflectionalMeaning for consisting of the non-segmentable inflectional meanings ThirdPerson, Singular and Present. This property is a subproperty of mmoon:hasMeaning next to other properties that can be used to declare derivational, grammatical, contextual or inherent inflectional meanings and senses. The URI of mmoon:Morpheme resources reuses the morphemic glosses that are already interconnected with the meanings within the MMoOn Core ontology. This is done since morphemes are concepts and as such need some kind of representation in order to be referenceable. The abstract identities provided by the morphemic glosses are widely known and, therefore, suitable to serve this purpose. Moreover, since the mmoon:Morpheme resources represent concepts only, statements about their perceivable forms, for example their ordering, segmentation or position within a word, are made by means of the corresponding morphs by which they are realized. To this extent, the modeling of the linguistic concepts of ‘morph’ and ‘morpheme’ in MMoOn Core formalizes the distinction between signifier and signified which constitute the – usually inseparable – sides of the linguistic sign. By explicitly separating them, information about both – as just illustrated – can be described in detail by avoiding ambiguities at the same time.
However, comprehensive datasets containing resources that are involved in the blue graph just explained are rather an exception. Especially the mmoon:Morpheme instances as defined in MMoOn Core only exist in interlinear glossed text sources. Therefore, the object properties are modeled in a way that allows to represent any fraction of MLD with MMoOn Core. As single requirement, a MMoOn based dataset needs to have at least one morphemic entry, i.e. a mmoon:Morpheme or a mmoon:Morph resource. Apart from that, one can start representing data from any level. The three inverse object properties mmoon:hasRealization, mmoon:belongsTo and mmoon:belongsToLexeme enable the representation of data in the opposite direction of the blue graph in Fig. 3 from the morpheme or morph to the word form and word data. In addition to that, it is necessary that MLD can be modeled independently from the complexity of the data. Especially the possibility to assign meanings not only to the morpheme resources but also to morph and word resources had to be considered carefully. For datasets containing only morphs together with the information of the meanings they encode, mmoon:Meaning instances can be also directly explicated. This is illustrated in Fig. 3 with the instance Suffix_er1 which can also be directly associated with the derivational meaning instance AgentNominalizer in case the AtomicMorpheme_AGNR instance does not exist to declare the morph-to-morpheme correspondence. What can be also seen is that the morphemic gloss instance mmoon:MorphemicGloss_AGNR already exists in the MMoOn Core vocabulary and is automatically assigned to the meaning instance AgentNominalizer (cf. Section 5.1.1). Since the URIs of the mmoon:Morpheme instances are based on the labels of the mmoon:MorphemicGloss instances, mmoon:Morpheme instance data might be later derived from the established meaning-to-gloss associations that are given for mmoon:Morph instances lacking corresponding mmoon:Morpheme data. Likewise, meanings can be directly assigned to mmoon:Word resources (however, not shown in Fig. 3). This might be useful for datasets similar to DBnary that contain only word forms of a lexeme that are annotated with the corresponding grammatical meanings on the word level. Albeit, for this case a fully valid MMoOn dataset can not evolve, because no morph or morpheme resources are contained. It is, however, possible to use the MMoOn vocabulary then as an extension of another vocabulary for lexical data such as OntoLex-lemon.
The decision to define not only mmoon:Morpheme but also mmoon:Morph and mmoon:Word as domains of the mmoon:hasMeaning object property compensates for the lack of morpheme data as defined in the MMoOn Core vocabulary. Under the assumption that dataset creators start with the most suitable usage of the ontology according to their source data and make use of a later generation of mmoon:Morpheme resources from the initial MMoOn-RDF data, it can be expected that the dataset is likely to become semantically over-expressive. It might be the case, for example, that a later addition of the instance AtomicMorpheme_AGNR creates two more triples; one that interlinks it with the morph Suffix_er1 via mmoon:hasRealization and another that interconnects this mmoon:Morpheme instance to AgentNominalizer via the mmoon:hasMeaning property. This leads to a semantic overload but does neither reduce the interoperability nor the quality of a dataset. Overall, the heterogeneity of existing non-RDF morphological data representations had to be taken into account. Therefore, this modeling option is regarded as a reasonable compromise to enable a less constrained data modeling which can in turn serve as a basis to arrive at the intended usage of MMoOn Core due to the possibility to create mmoon:Morpheme resources from mmoon:Meaning data. The alternative would have been to restrict the usage of mmoon:hasMeaning to mmoon:Morpheme instances and to accept a largely reduced applicability of the vocabulary and, consequently, less morphemic datasets in RDF.
The presented overview of the MMoOn Core object properties illustrated the possibilities of their usage for representing MLD of different complexity and coverage. On this basis MLD (if newly created) can be modeled according to the ideal graph just exemplified or (if covering only a part of the domain data) extended later on to include more fine-grained MLD. It shall be noted that datasets containing morpheme, morph, word form and lexeme resources that are interconnected in the most granular way will allow to derive the greatest insights into the morphological elements and structures of a specific language that is represented with the MMoOn Core vocabulary.
5.2.Architectural setup of MMoOn morpheme inventories
Given the complexity of the MMoOn Core ontology the question arises how language-specific MMoOn morpheme inventories are meant to be built. Therefore, an integrational architectural setup (cf. Fig. 4) has been developed which interconnects the language data of each morpheme inventory with MMoOn Core and, thus, ensures the multilinguality of all MMoOn datasets. The architectural setup comprises three data layers that serve to cover the following two aspects of linguistic data, i.e. 1) the difference between primary and secondary language data and 2) their description by assuming either language-independent or language-specific linguistic categories. The first aspect is based on the general assumption that most linguistic datasets comprise primary as well as secondary language data [36]. The former data type is defined here as language data which originates from a certain text compilation or could be applied to any text or token in order to identify the word forms, morphs and morphemes of the morpheme inventory. The latter is then defined as the kind of data which enables the description of the primary language data. E.g. the German plural suffix deu_inventory:Suffix_er1 is a primary language data instance which is specified with the secondary language data instance deu_schema:Plural for its grammatical meaning. The second aspect is then concerned with the assignment of both instances to language-independent or language-specific categories. In this respect, linguistic categories like suffix or plural tend to be modeled as language-independent concepts, even though, in practice they are used in the context of describing the data of a specific language and consequently then carry a more specific meaning.
Fig. 4.
Architectural setup of MMoOn morpheme inventories exemplified with morphological German and Hebrew data.
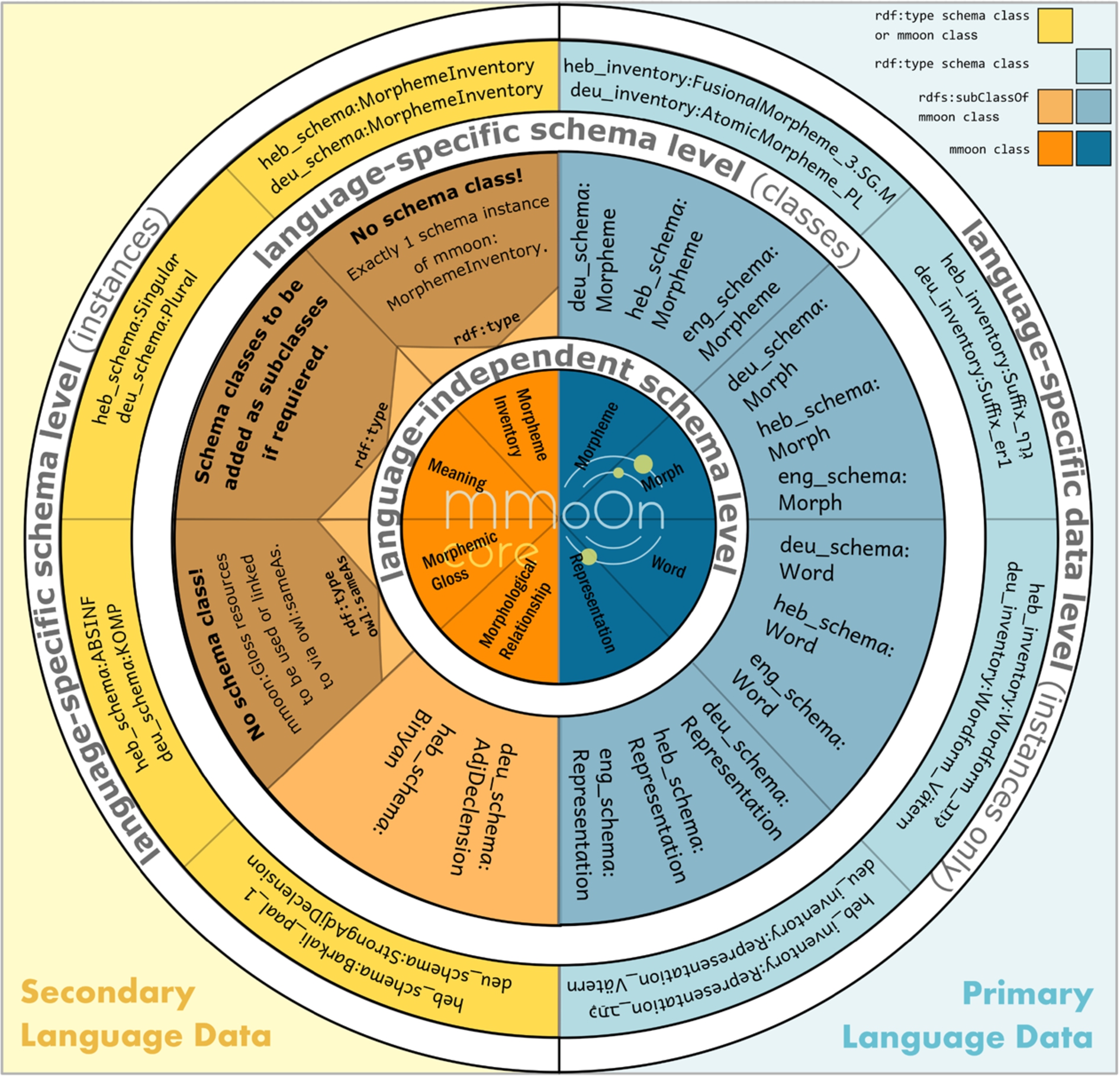
In what follows, the three data layers of MMoOn morpheme inventories will be described and how they allow to model primary and secondary language data simultaneously in the context of a language-independent data model that subsumes and interrelates language-specific data.
The first layer builds the MMoOn Core ontology as the underlying formal and conceptual model shared by all morpheme inventories. Since it models the domain of morphology as a subfield of the study of language it functions at the language-independent schema level describing the domain of morphology in a general way. It aims at providing the starting point for creating language-specific models of the morphology of a certain language based on unifying and comparable generic concepts. In that respect, it can be seen in Fig. 4 that the eight main classes are divided into four classes for the representation of secondary language data which can be directly applied to describe the primary language data that is represented by means of the other four main classes. This modeling satisfies the practical implication that primary language data is rarely collected on its own but most often accompanied with respective secondary language data that needs to be specified as well. Especially the provision of the numerous fine-grained grammatical and derivational meanings facilitates, thus, the creation of a morphological dataset because it reduces the time which is usually required to search for the necessary linguistic meanings in other external vocabularies.
The second layer in the architectural setup builds the language-specific schema level (i.e. the entire middle and outer left circle) being exemplified for a German and Hebrew morpheme inventory in Fig. 3. This level is meant to provide the formalized schematic vocabulary which enables a description of the general linguistic concepts provided in MMoOn Core in compliance to their actual language-specific realization. Consequently, the domain of morphology on this level is modeled as the descriptive linguistic part of a certain language. In practice this layer is realized by a language-specific ontology that imports the MMoOn Core ontology and contains language-specific extensions via added subclasses and instances. These include subclasses of all four MMoOn Core main classes (and their subclasses) representing primary language data as well as subclass extensions of the mmmon:MorphologicalRelationship class. Morphemic glosses, however, are not meant to be created but preferably reused from the MMoOn Core vocabulary to ensure consistency across multiple MMoOn-based datasets. On this level MMoOn Core class mmoon:MorphemeInventory is populated with only one instance specifying the language of the morpheme inventory according to the dataset it contains. Moreover, the language-specific variants of the MMoOn Core mmoon:Meaning class are realized as instances. Assuming that MMoOn Core does by far not cover all grammatical and derivational meanings that exist across the languages of the world, missing meanings can be added by creating a new (sub)class. As a result, the deu_schema ontology and the heb_schema ontology are derived as extensions of MMoOn Core by creating appropriate subclasses and instances, e.g. deu_schema:Word rdfs:subClassOf mmoon:Word or deu_schema:Plural rdf:type mmoon:Plural. Similarly, necessary but missing relations can be added by creating new object or datatype properties. However, it is assumed that the properties already provided by the MMoOn Core ontology will be sufficient for representing most of the existing morphological data and can be, therefore, directly used. This language-specific ontology as an extension of the MMoOn Core model serves the purpose to enable the definition of the linguistic elements according to their language-internal peculiarities by being interconnected with a higher cross-linguistic meta layer at the same time. In order to facilitate the creation of MMoOn morpheme inventories a schema template file that contains the MMoOn Core import as well es the described class extensions is available for immediate reuse.3636 Further, an advantage of the language-specific ontologies is that they can be directly reused by other researchers who have morphemic language data on the same language and would like to contribute their dataset as a MMoOn morpheme inventory as well. An example for this is the Bantu Language Model, a schema ontology for the whole language family of the Bantu languages,3737 which served to create the Xhosa, Kalanga and Ndebele morpheme inventories.
The largest part of each dataset constitutes the primary language data. Within the architectural setup it is realized by instances on the language-specific data level (i.e. the outer blue circle in Fig. 4). Given that the primary language data is formally described by the secondary (but language-specific) language data, the former usually takes the subject position while the latter takes the object position within a RDF statement. Further, language-specific data instances can also take the object position, whenever primary language data is interrelated, e.g. when two suffixes are explicated to be allomorphs to each other.
In sum, the aim of this architectural setup is to create a unified multilingual data graph of all MMoOn morpheme inventories to come. The presented layers correspond in practice to three RDF files, i.e. mmoon.ttl, schema.ttl and inventory.ttl.3838 Even though the creation process of a MLD dataset as outlined with MMoOn seems more complex or even tedious, we like to encourage data set creators to adhere to the creation of MLD according to the design of the architectural setup of MMoOn Core-based morpheme inventories because it directly impacts the following four indirect outcomes:
1) Facilitated multilingual Linked Data usage: Due to the unifying function of the MMoOn Core model language-specific instance data of different languages can be cross-linguistically traversed through a single data graph.
2) Exploitation of linguistic data in NLP tasks for linguistics and vice versa: The rather flat structured language data NLP systems rely on could be supported and extended by also taking fine-grained linguistic data into account to arrive at more stable data-driven approaches. Conversely, empirical linguistic research benefits from vast amounts of language data that can be collected in a structured way with NLP methods, which in turn, can serve as a starting point to create more accurate and interrelated linguistic datasets.3939
3) Enable onomasiological and semasiological data retrieval: Most linguistic datasets only allow for unidirectional data retrieval. A MMoOn morpheme inventory, however, is more flexible in this respect. Because it provides the means to represent the association of a linguistic meaning with its language-specific expression within the same model, the meanings a certain morph or word form encodes as well as the kind of morphemic expressions that are used to encode a certain meaning can be retrieved simultaneously.
4) Development of a meta-collection of linguistic concepts: Every MMoOn Core based language-specific schema ontology automatically adds to the extension of the MMoOn Core mmoon:Meaning class and its subclasses. E.g. the generic meaning of the language-independent mmoon:Singular class is extended by all language-specific Singular instances. At the same time, additional and newly created linguistic concepts that appear in the schema ontologies indicate missing language-independent MMoOn Core concepts which will be regularly complemented. In this respect the MMoOn Core ontology is under constant development. As a result, the MMoOn Core ontology will evolve to a kind of meta-collection for linguistic concepts that also comprises and interconnects their language-specific realizations. To the best of the authors’ knowledge another ontology offering such an explicit distinction for representing language-independent and language-specific linguistic concepts does not exist.
5.3.Domain requirements and design principles
The creation of a domain ontology is guided by several influencing aspects ranging from the granularity of the domain representation, the intended usage of the resulting datasets and possible user groups to the choice of the vocabulary as well as the technical possibilities and limitations of the data format. Thus, modeling the MMoOn Core ontology entailed several design decisions. In order to comprehend the motivations that accompanied the development of MMoOn Core, the design principles and determining domain requirements will be outlined in what follows.
5.3.1.Design principles for the domain of MLD
Domain delimitation: The elements and relations of the domain of MLD in MMoOn Core are based on the domain analysis as outlined in Section 4. However, some of the included linguistic elements such as lexemes, word forms and morphs overlap with other linguistic domains, e.g. lexicography, phonology and syntax. Study areas like morphophonology and morphosyntax indicate that basic linguistic concepts are considered to be part of several linguistic domains depending on their defined characteristics and functions. As a consequence, the domain of morphology can be either described in a very strict way, ignoring possible domain interrelations or in a broader way which would result in an overlap with other domains. The MMoOn Core model takes up the strict approach and, thus, provides anything that is necessary to describe words and the meaningful segmentable subword elements of which they consist. Accordingly, the mentioned overlapping elements are not further specified for postulated functions and usages in other linguistic domains. In that respect, the model strives to be as detailed as possible (on a language-comparative level) and as broad as necessary at the same time. Therefore, MMoOn Core constitutes a quite narrow and fine-grained vocabulary for the domain of MLD but also provides prominent classes, such as mmoon:LexicalEntry, that appear across various linguistic domains and can be used as interlinking or alignment points. Furthermore, explicit cross-domain information can be also added by directly linking resources of a MMoOn morpheme inventory to an already existing dataset providing the necessary phonological or syntactic domain information for the same language. The reuse and interlinking to vocabularies describing other linguistic domains is recommended whenever possible.
Framework neutrality: Even though no model comes without any predisposition, MMoOn Core aims at completeness and a comprehensive application rather than fitting the descriptive needs of a certain linguistic framework, model or theory of morphology. It is a first proposal of modeling MLD comprising the relevant categories and relations in order to extend and integrate morphemic data into already existing linguistic datasets which are mainly framework neutral models as well. However, if required, the MMoOn Core vocabulary is easily adjustable so that the data that shall be represented is integrable according to strict theoretical descriptive needs.
Modeling of linguistic concepts and categories: One of the main challenges when it comes to the description of language data is the choice and modeling of the concepts for linguistic categories. A highly controversial debate exists among the linguistic research community about the treatment of concepts such as ‘case’, ‘gender’ or ‘noun’ as being interlingual comparative or language-specific descriptive categories (cf. for example [23] and [38]). Given that MMoOn Core serves as an upper ontology to create language-specific morpheme inventories both kinds of concepts needed to be considered. Due to the RDF format this particular issue could be solved by adhering to the Semantic Web’s standard which already entails the representation of commonality and variability through the hierarchy of classes [1]. In line with this, MMoOn Core classes are regarded as prototypical interlingual concepts and consequently function as the least common denominator for a linguistic category. Every instance of the classes is then a language-specific concept of the upper interlingual MMoOn class concept as described in the setup of the language-specific schema file. According to this, MLD of different MMoOn morpheme inventories can be described with all language-specific features while staying comparable because of the shared MMoOn class membership. As a result, all MMoOn Core based datasets will contribute to a multilingual data graph of interconnected MLD of specific languages.
Coverage: The MMoOn Core model covers concepts and relations that are necessary for synchronic language description, i.e. the representations and meanings of the words, morphs and morphemes are given according to a certain point in time (present or past). Thus, etymological and historical information is not considered in the class or property modeling. As Section 5.1 outlined, MMoOn Core encompasses a fine-grained vocabulary that enables the identification and description of linguistic elements that are necessary for representing MLD. Also, a considerable set of object properties allows for a detailed specification of the relations that hold among the words and the morphemes and morphs of which they consist. As mentioned before, the morphological rules underlying the data are not considered explicitly and need to be inferred indirectly from the data or have to be described by using another vocabulary along with MMoOn Core. The main approach pursued provides granular descriptive means for the morph and morpheme elements and their interrelations to word elements by outsourcing granular phonological, lexicographic or syntactic concepts at the same time. This is not seen as a disadvantage because including them would entail the preference of some theoretical framework which is meant to be avoided.
Target user groups: The use of the MMoOn Core model is directed towards linguists, computational linguists, NLP researchers, lexicographers and anyone who has an interest in compiling and managing MLD. It is anticipated that MMoOn language inventories will be set up by data compilers of the various user groups mentioned. That way synergies can evolve between the smaller but high-quality and mainly manually compiled datasets that are expected from the linguists and the large but not as fine-grained data produced by users with an interest in the machine-processable aspect of linguistic data. The emergence of these cross-disciplinary synergies are assumed to advance the whole LLOD community in general.
5.3.2.Data modeling requirements
Linked Data principles: The choice to model MMoOn Core in the RDF format is motivated by the underlying Linked Data principles [3] which promote the creation of structurally and semantically interoperable datasets. This aspect adheres to the aim of providing a data-unifying domain modeling that is based on technical integrability. Furthermore, due to the creation of unique resources as URIs, the ontology is easily accessible on the Web. Consequently, all emerging MMoOn-based datasets will, therefore, contribute to a growing interconnected data graph and, thus, not join the ranks of the already existing morpheme data silos on the Web.
Reuse: In general it is understood as a good practice to reuse existing vocabularies when creating a new ontology. Since the largest part of the MMoOn Core vocabulary aims at representing meanings, we decided to create a new taxonomy within the mmoon:Meaning class and to describe every subclass as a MMoOn Core-specific resource, even though other vocabularies contain similar or the same linguistic meanings and categories as well. By doing so, the assignments of meanings to morphemic elements or words when creating a MMoOn dataset should be facilitated and, moreover, a consistent assignment of morphemic glosses to vocabulary-internal elements could be achieved. Nonetheless, the considerate overlap with other vocabularies for representing language data is accounted for by interrelating mostly mmoon:Meaning but also mmoon:Morph classes to the highly used GOLD [18] and OLiA [8] ontologies. Classes that are regarded as either equivalent, similar or usable as a defining description for a MMoOn Core class are interrelated via the owl:equivalentClass, rdfs:seeAlso or rdfs:isDefinedBy properties. Furthermore, an alignment with MMoOn Core and the OntoLex-lemon model has been established by stating that mmoon:LexicalEntry is a subclass of ontolex:LexicalEntry. This enables a more specific description of mmoon:LexicalEntry resources by using the OntoLex-lemon vocabulary for lexicographic information and prevents an overload of the MMoOn Core model by including already existing lexical data.
Extensibility: Finally, a data compilation is rarely ever complete and a single domain model can never capture all practical and theoretical aspects of MLD in general and even less the aspects of MLD of single languages. Given these circumstances, the MMoOn Core model serves as a starting point for morphological data description that might be sufficient for a considerable number of datasets, but must be also prepared to allow for necessary extensions and/or adjustments. This requirement is also assured by the Linked Data format meeting these needs by taking up the assumption of an open world [1]. Consequently, the RDF format allows for a liberate reuse of all classes and properties as well as for an unrestricted extension of the model with new classes and properties. It is, however, assumed that the central comprehensive elements are provided by MMoOn Core and shared by the majority of the emerging MMoOn-based datasets.
URI design: As outlined in Section 5.2 every MMoOn morpheme inventory consists of three files with the MMoOn Core ontology being shared by all datasets. In order to facilitate the identification of and navigation through a dataset, the following URI scheme is implemented for all MMoOn datasets created by the authors: http://mmoon.org/lang/schema/pi/ for the language-specific schema ontologies and http://mmoon.org/lang/inventory/pi/ for the language data, where lang is replaced by the ISO 639-3 language code and pi by an identifier for the project name, e.g. http://mmoon.org/deu/schema/og/. For all other dataset creators it is recommended to adhere to the following URI pattern for establishing greater consistency among all MMoOn-based datasets to come: http://hostname/lang/schema/pi/ and http://hostname/lang/inventory/pi/, respectively.
In sum, it appears that the data modeling requirements posed by the morphology domain are very well accomplished by the underlying Linked Data format. The MMoOn Core model as a proposal to start with a homogeneous morphemic data compilation fulfils the needs of a specified linguistic data description model and integrates the resulting data into the Semantic Web environment, thus, benefiting from all of its advantages.
6.MMoOn and OntoLex-lemon
In contrast to existing ontologies for describing language data, linguistic datasets rarely contain linguistic information that neatly corresponds to one single linguistic domain. The OntoLex-lemon model [40] being a W3C community group specification tackled this issue by covering the domain of lexicology by enabling the representation of related linguistic domains via dedicated submodules. With this modular extensible approach the representation of a wide range of the existing linguistic data can be already realized. Consequently, an all-encompassing vocabulary covering any potential or existing kind of linguistic data point is neither feasible nor desirable. Rather, the development and usage of more fine-grained and specific vocabularies that are interconnected with a commonly shared ontological basis, i.e. OntoLex-lemon, will provide the necessary means to enable an appropriate modeling of existing or future linguistic data as Linked Data.
This holds true especially for the domain of MLD, which tends to include lexical as well as morphological data. Depending on the use case and dataset, OntoLex-lemon, i.e. the ontolex and decomp submodules in particular, may be used for describing MLD. This has been, for instance, done for representing the components of compound words [16]. Nonetheless, as already mentioned in Section 3.1 for linguistic data corresponding to the domain analysis of MLD (cf. Section 4), the ontolex and decomp modules are mostly limited to compositional morphology and, hence, leave the larger part of the MLD domain to be non-expressible with the provided vocabulary.
A comparative overview based on detailed examples that shows how data on the lexeme, word form, morph and morpheme levels can be described by using either OntoLex-lemon or MMoOn Core can be consulted in Klimek 2017 [32]. Here, a list shall suffice that summarizes the main results, i.e. aspects that reach representability through the MMoOn Core vocabulary and which are not covered in OntoLex-lemon respectively:
1) Inflectional affixes: Since inflectional information is usually no central part of lexical data, means to represent inflectional affixes are not part of OntoLex-lemon. In fact, even consistently collected number information for nouns by providing the respective morph together with the lexical entry, is not describable with it. Instances that are allowed within the ontolex:Affix class are restricted to affixes that form new lexical entries, i.e. derivational affixes. However, a huge part of MLD is comprised by inflectional affixes that are necessary to represent the formation of word forms. The MMoOn Core vocabulary, in contrast, does not distinguish between derivational and inflectional affixes in its assertion being of the type mmoon:Affix. Instead, the inflectional or derivational meaning underlying a specific affix is contained in the corresponding morpheme instance as well as its occurrence within a lexical entry or word form, respectively.
2) Stems and roots: Those two elements are crucial for describing MLD, not only for decomposing word forms but also lexical entries. While OntoLex-lemon provides the possibility to identify the underlying stems in compound words only (which are not termed as stems but widely included within the class decomp:Component), it is not possible to represent the stems or roots of word forms. MMoOn Core provides classes for both elements. Even though the granularity of a segmentation differs from dataset to dataset and depends on the applied linguistic analysis, in many languages root resources are the building blocks of lexical data, e.g. in Arabic languages, and, hence, should be covered as well. As a result, MMoOn allows for the representation of whole inflectional paradigms, including the decomposition into underlying roots, stems and inflectional affixes of the word forms belonging to a specific paradigm.
3) Morphemic interrelations: Part of the description of morphemic elements is also the representation of their relation to other morphs. Therefore, stating the allomorphs and homonyms of a morph is important for their identification, function and the combinatoric rules that apply to them. While the MMoOn Core vocabulary contains two object properties to specify allomorphy and homonymy between morphs, these relations are not part of the lexical domain and, hence, not expressible with OntoLex-lemon.
4) Morphemes and meanings: Also not part of the lexical domain is the representation of morphemes. Meanings, i.e. lexical senses in OntoLex-lemon, differ largely from the grammatical and derivational meanings that are necessary for describing MLD. The 300 meaning classes provided in MMoOn Core are far from being extensive with regard to the large variety across languages. However, they are a first step towards collecting and documenting meanings that are encoded by morphs and constitute a useful starting point for representing morpheme resources.
As a result of the introduced suggestion to create an interconnection between OntoLex-lemon and MMoOn Core in Klimek 2017 [32] both domain ontologies have been aligned, as already mentioned in Section 5.3.2, with the established subclass relation between mmoon:LexicalEntry and ontolex:LexicalEntry. The two ontologies are intended to be separately usable to describe morphological as well as lexical data in an independent and specific manner by simultaneously maintaining the semantic interconnectivity between all data elements. Consequently, the MMoOn Core model shall not be understood as an OntoLex-lemon extension but serves as a stand-alone vocabulary that can be used in conjunction with OntoLex-lemon. Still, the MMoOn Core ontology and its proposed alignment raised awareness within the W3C Ontology-Lexica Community group.4040
As a result, the creators of MMoOn Core have been invited to lead the development of a new OntoLex-lemon morphology module which is currently under development.4141 As the interim results for this emerging OntoLex-lemon module report [35], the morphology module aims to represent MLD in the context of lexical language data and is not intended to be a vocabulary for the domain of MLD per se. MMoOn Core has built the main orientation basis in the module creation process, however, with the goal to reduce complexity. Especially the morph and its specification of affix types is taken up from MMoOn Core and also the possibility to express inflectional and derivational morphs is now considered. A novelty in the morphology module will be the creation of a means to automatically generate word forms for a lexical entry which is not an integral part of MMoOn Core. In general this module differs from MMoOn Core in that it is more suitable for advanced users of Semantic Web technologies and the Linked Data framework. This is due to the embedding of new vocabulary elements into the existing OntoLex-lemon modules and the outsourcing of meanings and glosses by referring to recommended external vocabularies as well as the considerable data preprocessing that is required for the automatic generation of word form data. After all, the data creators, their level of training with Linked Data and their intended usage of the MLD in RDF will influence the choice for MMoOn Core or OntoLex-lemon (including the future morphology module) or both models in conjunction. On the whole, it is advisable to start the initial transformation to RDF with the vocabulary that is more expressive with regard to the underlying linguistic domain of the source data, i.e. OntoLex-lemon for lexical or MMoOn Core for morphological data.
7.Use cases
In what follows, possible usages of the MMoOn Core ontology will be outlined. This serves to exemplarily indicate the research potential it entails for the two application areas of linguistics and NLP it has been designed for. It shall be noted that all mentioned usages are equally realizable with the commonly applied methods of language representation and analysis in these fields. However, special awareness should be given to this Linked Data-based approach of MLD representation by using MMoOn Core (alone or in conjunction with other ontologies) because it yields the benefit of interdisciplinary reuse, extension and application as an opportunity to overcome the current limitations of scientific progress caused by data silos and heterogeneous formats.
7.1.Use cases for linguistic research
7.1.1.Enhancement of morphological data in dictionaries
Dictionaries and lexical datasets contain a considerable amount of MLD. This includes derivational morphs and the lexical entries they can be productively combined with but also elements and building patterns of inflectional paradigms that vary in the degree of their descriptive granularity across dictionaries of different languages. In dictionaries of Semitic languages, for instance, headwords are collected around roots which are followed by the full list of word forms but also lexemes which can be derived from them. For the description of such fine-grained morphological data, the creation of MMoOn morpheme inventories enables the representation of this data in an appropriate manner which can serve as an addition to vocabularies that are usually used for representing lexical data. The Hebrew Morpheme Inventory can be seen as a proof for this application of the MMoOn Core ontology [34].
7.1.2.Language acquisition
With the availability of more and more language data the applied linguistic research area of (second) language acquisition is provided with new possibilities for creating language learning materials and tools. Within this setting morphological data plays a significant role for the acquisition of inflection and formation patterns of words. The future morphological datasets, therefore, have the potential to broaden and complement already existing data-driven learning tools and techniques for corpus linguistics [22] with valuable morphological data. Provided by MMoOn morpheme inventories, inflection tables, word families and the grammatical as well as lexical morphs with their usage restrictions can be obtained. In this respect single MMoOn-based datasets can be already regarded as source data for language learning and teaching materials. The created Xhosa RDF dataset [5] is an example for a MMoOn-based dataset with an intended usage for language revitalization efforts for Bantu languages by using the MMoOn Core ontology as the uniting model for collecting interoperable data of multiple Bantu languages [17] to develop various learning materials.
7.1.3.Language documentation
The area of language documentation has the intention to “to provide a comprehensive record of the linguistic practices characteristic of a given speech community” [28]. Since the publication of this paper in 1998, this area has sparked a community which aims to create linguistic resources for endangered and minority languages. As mentioned in Section 3.3, due to the work of the language documentation community, a great amount of interlinear-glossed text resources exist in linguistic databases or as text examples in linguistic publications. However, these linguistic resources do not use the same representation format. Hence, sharing it within and especially outside of this community is difficult. If a language was documented using the MMoOn Core ontology, it would be possible to create other output formats such as tables, dictionaries, etc. That way the resulting language resource could not only be shared with the language documentation community but, moreover, this data would become usable by the NLP and Semantic Web communities to create tools supporting minority languages.
7.1.4.Representation of morphemic glosses in linguistic literature
Morphemic glosses are part of many linguistic publications and usually used in given examples. A standardized set for interlinear morphemic glosses does not exist and each publication is accompanied with a customized list of glosses. Nonetheless, an adoption of the proposed standardized application within the Leipzig Glossing Rules [13] as well as the reuse of the therein provided set of glosses can be observed. However, the majority of glosses being used is still heterogeneous in that different glosses are used for the same morphemic concepts across the literature. The morphemic glosses provided in MMoOn Core can be regarded as a reference set of glosses since MMoOn Core already reused the existing glosses provided within the Leipzig Glossing Rules which are already widely accepted and applied by linguists. Given that the links between all mmoon:MorphemicGloss instances and the linguistic concepts they represent, i.e. the instances of all mmoon:Meaning subclasses, are already created, an unambiguous reference can be established. Consequently, including the morphemic gloss URIs within the digital versions of publications of linguistic works can not only contribute to a more consistent usage of glosses but also to a better findability of language examples that are, hitherto, hidden in unstructured text documents.
7.1.5.Comparative linguistics
The internally provided links between the mmoon:Meaning classes and mmoon:MorphemicGloss instances that come with MMoOn Core entail another possibility, i.e. they are especially suitable for comparative linguistic analyses. This is because a multilingual semantic interconnection is automatically established since all schema ontology files of the MMoOn morpheme inventories are interconnected within a single graph via the imported MMoOn Core ontology. As a result, this allows for a flexible conversion or newly created representation of multiple language datasets taking language-specific characteristics into account while maintaining semantic interoperability simultaneously. Due to this architectural setup of MMoOn Core, reasoning is enhanced and specific queries enable exact investigations of comparative synchronic cross-lingual phenomena and, moreover, tracing historical linguistic changes across multiple datasets at once. In particular the use of the morphemic glosses is facilitating semasiological as well as onomasiological querying because every created language-specific meaning in a morpheme inventory is automatically interlinked to the respective language-independent gloss.
7.2.Use cases for NLP research
7.2.1.Conversion of Wiktionary datasets
The already mentioned MLD provided by Wiktionary (cf. Section 3.2) is one of the largest openly available datasets. In the context of Linked Data-based NLP research it is desirable to create an RDF version of this data. The existing Dbnary morpho dataset is, however, not appropriate for NLP tasks because it covers only four languages, uses an outdated lemon vocabulary and contains only a morphological annotation of the grammatical meanings of the word forms given in the Wiktionary inflection tables. Instead, it seems promising to convert existing data provided by UniMorph [30,31] and paradigm extractions4242 [19] which have already normalized and segmented Wiktionary data into structured formats. The UniMorph 2.0 [30] dataset contains data of 47 languages from Wiktionary that has been normalized with regard to the differing inflection tables and that is semantically annotated with a set of grammatical features which correspond essentially to the mmoon:MorphemicGloss instances in MMoOn Core. The data provided by paradigm extract [19] covers only nine languages but is of special interest because the inflectional paradigms extracted from Wiktionary also contain the segmented morphs of a word form. Combined, these two datasets constitute a substantial foundation to convert the word forms and morphs contained within Wiktionary inflection tables into RDF. The architectural setup for creating MMoOn morpheme inventories is suitable to represent the UniMorph and paradigm extract data. Hence, the existing data could not only be made available as Linked Data but also merged within a single data graph in which they would be automatically semantically enriched (by the interlinking of the glosses to meanings and the meanings to morphs) and multilingually interconnected due to the uniting function of the underlying MMoOn Core model.
7.2.2.Morphological text annotation
Morphological annotation tools could be created with a data-driven approach based on MMoOn datasets similar to the task of part-of-speech tagging. The initially required RDF representation of corpora can be provided by using the Natural Language Processing Interchange Format (NIF) [26,44]. The resulting NIF corpus can be then extended with several layers of annotations depending on the granularity of the interconnected MMoOn dataset. This could range from the identification of lexemes, stems, morphosyntactic meanings and also part-of-speech data on the word form level of the tokens up to the segmentation into their morphs together with the underlying inflectional and derivational meanings on the morph level of the tokens. In any case, the mmoon:MorphemicGloss resources can be regarded as a ready-to-use tagset for meanings which facilitates the creation of annotations. Such a MMoOn-based morphological text annotation approach could also provide suggestions for unknown tokens due to the possible lookup of their contained morphs (which are likelier to exist in the dataset). The more fine-grained the underlying MMoOn dataset for such an annotation tool is the more detailed linguistic information can be automatically extracted from large amounts of texts. This can in turn impact the results of other NLP tasks and might even lead to the automatic creation of interlinear glossed text.
7.2.3.Named entity recognition
Recent work in the field of named entity recognition (NER) in German has revealed that the complexity of morphology is rarely considered in existing NER tools, even though considering it could lead to improved results [33]. This holds true especially for the identification of NEs (or linguistically termed: proper nouns) which have undergone several morphological transformations and appear within complex lexemes. E.g. in order to retrieve the NE Alpen (engl. ‘the Alps’) within the inflected German noun Skilalpinistinnen (engl. ‘female ski alpinists’) all compositional, derivational and inflectional transformations that have been applied to Alpen have to be deconstructed. But also nontransformed proper nouns that are only obligatory affected by inflectional marking can already pose a challenge for NER tools. Within a German MMoOn morpheme inventory the involved morphs -en, -in(1), -ist, Ski, alp and -in(2) would be available and could help to identify the NE within the common noun. A very elaborate MMoOn dataset could also contain the complete token with its full segmentation, which allows for a direct retrieval of the underlying NE from within the data graph. Since the MMoOn Core ontology enables a comprehensive explication of morphological data, the lack of appropriate morphological data can be overcome. Consequently, future morpheme inventories could be a promising consideration in the development of NER tools and systems.
7.2.4.Machine translation
Machine translation belongs to one of the most complex and challenging tasks in NLP. Dictionaries and lexical data play a crucial role as one of the sources that are utilized for identifying the sense of a word in a text in one language and the respective expressions used for this sense in another language. However, depending on the morphological type of the languages that are to be translated this task is getting increasingly difficult the more the word-to-morpheme ratio deviates from one-to-one correspondences. Machine translation systems that would be complemented by MMoOn-based datasets could rely on the more fine-grained morphological data. This might be especially improving when translating from analytical languages, e.g. Vietnamese, to polysynthetic languages (marking the extremes of the typological continuum) or vice versa. A lexical approach only will not be able to capture for instance sentences like angya-ghlla-ng-yug-tuq, ‘I have a fierce headache’ (Siberian Yupik) [12] because it consists of a single word. Within the MMoOn representation, however, the individual morphs are explicated and could be translated into an isolating or agglutinative language through the senses and grammatical meanings they consist of. Since all MMoOn datasets share the MMoOn Core ontology within the unified graph of a multilingual dataset the atomic morphemes of isolating languages and the fusional morphemes of polysynthetic languages can be identified and translated in an onomasiological way (in contrast to the semasiological approach of lexical data).
7.2.5.Sentiment analysis
Comprehensive MLD also has the potential to contribute to the NLP research field of sentiment analysis. Subjective information about topics within texts is not only encoded lexically but also by morphological means. E.g. the detection of negation, being one of the main issues for sentiment analysis [47], could benefit from a morphological data source such as a MMoOn morpheme inventory because negation can be very productively expressed by using prefixes like un- for English together with adjectives. Furthermore, bound morphemes for comparative, superlative or intensification can be easily retrieved from such a dataset and also identified even if the lexemes they are attached to are unknown. In general, MLD represented with MMoOn can explicitly describe obligatory grammatical and highly productive lexical morphemes that express various concepts relevant for sentiment analysis. Consequently, an integration of MLD in the form of MMoOn morpheme inventories poses a promising application case for extending existing resources, algorithms, models and frameworks in the field of sentiment analysis.
8.Concluding remarks
The development of the MMoOn Core ontology started in 2015. Since then, the ontology has been evaluated for its applicability resulting in the Hebrew Morpheme Inventory [34] as proof of concept. Simultaneously, the architectural setup has been developed, morphemic glosses and meanings have been extended and refined. The interim status of the ontology has been presented at various scientific events to gain feedback from the target user groups which has been considered and integrated into the final publication state of MMoOn Core as well. Despite this longstanding process from conceptualizing to actually publishing this accompanying article for the MMoOn Core ontology, no comparable advancement in creating a domain ontology for representing MLD is recorded [6].
As far as the vocabulary use of MMoOn Core is concerned, it achieves a four out of the five star ranking of Linked Data vocabulary use [29]. According to this, MMoOn Core contains dereferencable human-readable information about the used vocabulary (1 star), available information as machine-readable explicit axiomatization of the vocabulary (2 stars), a linking to other vocabularies, i.e. OntoLex-lemon (3 stars) and provides metadata about the vocabulary (4 stars). At the current state the fifth star, i.e. vocabularies that link to MMoOn Core, is not achieved. With the awareness that exists already for this domain ontology, however, it is very likely that other vocabularies, e.g. OntoLex-lemon or Ligt will create links to MMoOn Core in the future.
In summary, the presentation of the MMoOn Core ontology in this paper has explained how this model will enable the conversion of existing as well as the creation of new morphological datasets and, thus, reaches its aim of contributing to a rising number of homogenized, interoperable linguistic datasets. This result is mainly based on two characteristics of the ontology. First, the rather unusual granularity of the provided meaning classes and their interlinkings with their respective glosses reduce the time for mapping source data of different formats with the ontology and enhances the consistency across datasets. Being embedded within the whole MMoOn Core ontology, these concepts explicate the large part of the linguistic domain of morphology and, therefore, enable the creation, transformation and semantic enrichment of the of MLD that was hitherto inaccessible for machine-processing, e.g. inflection tables, interlinear glossed text, morphological data accompanying lexical databases and dictionaries. The second crucial characteristic of MMoOn Core is its capacity to strengthen the interdisciplinary reuse of MLD originating from the linguistic, NLP and Semantic Web communities. Due to the architectural setup that is based on MMoOn Core, both, language-independent as well as language-specific representations of MLD can be realized. Therefore, depending on the use case and the intended application of the MLD that shall be described as Linked Data either the MMoOn Core ontology can be used to create a very generic and language-independent morpheme inventory or a language-specific schema file that enables specific extensions. Due to the fact that all emerging MMoOn-based datasets are inherently interconnected through the MMoOn Core ontology, datasets that had been of potential interest for a specific user group but have been eventually rejected for an actual reuse (because they were considered too general or too specific in their description) can be now directly adjusted to the required granularity of the representation needs. In this respect it is through the architectural setup of MMoOn Core that the creation of MLD is enabled not only for different user groups and usages but also that all resulting morpheme inventories are semantically unified, thus, leading to an enhanced interoperability and reusability. To conclude, it could be shown that the MMoOn Core ontology contributes to a facilitated and flexible cross-disciplinarity MLD data generation and exchange.
9.Future work
Even though the MMoOn Core ontology as it is published now can be regarded as a ready to use domain ontology, it is intended to evolve in the future. Collecting and representing all concepts that can be morphologically expressed across the word’s languages can not be achieved by a few scientists. Therefore, the meanings provided in MMoOn Core can be regarded as a starting point of the ontology which shall be constantly adapted and extended according to emerging MMoOn morpheme inventories and their schema files. Especially the list of derivational meanings is envisioned to be enlarged and integrated into MMoOn Core from the language-specific datasets.
Another prospective step entails to outreach to other LLOD communities in order to strengthen collaborative research. This is desirable in order to reach the most consistent usage of existing linguistic domain models and data since the considerable overlap of linguistic data compilations of different research areas can not be avoided. Given that MMoOn Core presents a further addition to existing ontologies for the representation of linguistic domains it is advisable to reach a shared agreement on aligning phonological, morphological and lexical data by interconnecting PHOIBLE [42], MMoOn Core and OntoLex-lemon respectively.
Similarly, the connection of MMoOn Core and the Ligt ontology will be promoted. In doing so, a higher number of semantically richer morphological datasets from interlinear glossed text sources, especially for less-resourced languages, can be expected in the future.
Finally, work on Linked Data-based solutions for an integration and the transformation of non-RDF resources such as the Typecraft or UniMorph datasets into LLOD based on MMoOn is planned.
Notes
10 Cf. the emergence and development of the Linguistic Linked Open Data Cloud: http://linguistic-lod.org/llod-cloud.
11 This paper follows the generic style rules for linguistic [24]. This means that italics are used for all object-language forms (words and morphs) that are cited within the text or examples and single quotation marks are used for indicating linguistic meanings (morphemes).
12 Taken from the Lancaster tagset: http://www.scs.leeds.ac.uk/amalgam/tagsets/lob.html.
13 This kind of morphological representation is well established practice in linguistics and widely known as interlinear morphemic glossing [13,37].
24 Even though the datasets published by Dictionaria are also provided in RDF, this information is omitted here because no standard vocabulary for linguistic Linked Data has been used and only a part of the original data is transformed into RDF, i.e. only the headwords encoded in literals. Instead, very basic vocabularies such as SKOS and DCTERMS have been used. As a consequence, the morphological data that is entailed in the original source dataset is either missing completely or not differentiable from the lexical data within the delivered RDF datasets.
27 Atelier pour les LEXiques INformatiques et leur Acquisition, http://gforge.inria.fr/projects/alexina.
34 Efforts to achieve this goal are currently under development within the OntoLex-lemon morphology module [35].
36 The template file can be downloaded here: https://github.com/MMoOn-Project/MMoOn/blob/master/schema_template.ttl and only needs to be specified for the language of the morpheme inventory.
38 Usually the schema and inventory files are specified for the language of the morpheme inventory, e.g. deu_schema, heb_schema and deu_inventory, heb_inventory in Fig. 3.
39 For more details on how the MMoOn dataset creation setup is involved here, cf. Section 7.2.
Acknowledgement
We acknowledge support from Leipzig University for Open Access Publishing.
References
[1] | D. Allemang and J. Hendler, Semantic Web for the Working Ontologist: Effective Modeling in RDFS and OWL, Elsevier, (2011) . doi:10.1016/c2010-0-68657-3. |
[2] | D. Beermann and P. Mihaylov, TypeCraft collaborative databasing and resource sharing for linguists, Language Resources and Evaluation 48: (2) ((2014) ), 203–225, Elsevier. doi:10.1007/s10579-013-9257-9. |
[3] | T. Berners-Lee, Linked data-design issues, 2006, http://www.w3.org/DesignIssues/LinkedData.html (2011). |
[4] | G. Booij, The Grammar of Words: An Introduction to Linguistic Morphology, Oxford University Press, (2012) . |
[5] | S. Bosch, T. Eckart, B. Klimek, D. Goldhahn and U. Quasthoff, Preparation and usage of Xhosa lexicographical data for a multilingual, federated environment, in: Proceedings of the Eleventh International Conference on Language Resources and Evaluation (LREC 2018), N. Calzolari, K. Choukri, C. Cieri, T. Declerck et al., eds, (2018) , pp. 4372–4378. |
[6] | J. Bosque-Gil, J. Gracia, E. Montiel-Ponsoda and A. Gómez-Pérez, Models to represent linguistic linked data, Natural Language Engineering 24: (6) ((2018) ), 811–859, Mitkov, R., Tait, J. and Boguraev, B.K. (eds). doi:10.1017/s1351324918000347. |
[7] | C. Chavula and C.M. Keet, Is lemon sufficient for building multilingual ontologies for Bantu languages?, in: 11th OWL: Experiences and Directions Workshop (OWLED), C.M. Keet and V. Tamma, eds, (2014) , pp. 61–72. |
[8] | C. Chiarcos, An ontology of linguistic annotations, GLDV – Journal for Computational Linguistics and Language Technology 23: (1) ((2008) ), 1–16, Mönnich, U. and Kühnberger, K. (eds). |
[9] | C. Chiarcos and S. Hellmann, Working group for open data in linguistics: Status quo and perspectives, in: Proceedings of the 6th Open Knowledge Conference (OKCon 2011), S. Hellmann, P. Frischmuth, S. Auer and D. Dietrich, eds, ceur-ws.org, (2011) . |
[10] | C. Chiarcos and M. Ionov, Ligt: An LLOD-native vocabulary for representing interlinear glossed text as RDF, in: 2nd Conference on Language, Data and Knowledge (LDK 2019), M. Eskevich et al., eds, Schloss Dagstuhl-Leibniz-Zentrum Für Informatik, (2019) . |
[11] | P. Cimiano, P. Buitelaar, J. McCrae and M. Stintek, LexInfo: A declarative model for the lexicon-ontology interface, Journal of Web Semantics 9: (1) ((2011) ), 29–51, Elsevier. doi:10.1016/j.websem.2010.11.001. |
[12] | B. Comrie, Language Universals and Linguistic Typology: Syntax and Morphology, University of Chicago Press, (1989) . |
[13] | B. Comrie, M. Haspelmath and B. Bickel, The Leipzig Glossing Rules: Conventions for interlinear morpheme-by-morpheme glosses, 2008, available online at https://www.eva.mpg.de/lingua/pdf/Glossing-Rules.pdf (July 2016). |
[14] | M. Creutz and K. Lagus, Unsupervised morpheme segmentation and morphology induction from text corpora using Morfessor 1.0, Helsinki University of Technology, Helsinki, 2005. |
[15] | M. Creutz and K. Lagus, Inducing the morphological lexicon of a natural language from unannotated text, in: Proceedings of the International and Interdisciplinary Conference on Adaptive Knowledge Representation and Reasoning (AKRR’05) 1, T. Honkela, V. Könönen, M. Pöllä and O. Simula, eds, (2005) , pp. 106–113. |
[16] | T. Declerck, Representation of polarity information of elements of German compound words, in: Proceedings of the 5th Workshop on Linked Data in Linguistics: Managing, Building and Using Linked Language Resources (LDL 2016), J.P. McCrae, C. Chiarcos, E. Montiel Ponsoda, T. Declerck, P. Osenova and S. Hellmann, eds, (2016) , pp. 46–49. |
[17] | T. Eckart, S. Bosch, D. Goldhahn, U. Quasthoff and B. Klimek, Translation-based dictionary alignment for under-resourced Bantu languages, in: 2nd Conference on Language, Data and Knowledge (LDK 2019), M. Eskevich et al., eds, Schloss Dagstuhl-Leibniz-Zentrum Fuer Informatik, (2019) , pp. 17:1–17:11. doi:10.4230/OASIcs.LDK.2019.17. |
[18] | S. Farrar and D.T. Langendoen, An OWL-DL implementation of gold, in: Linguistic Modeling of Information and Markup Languages, A. Witt and D. Metzing, eds, Text, Speech and Language Technology, Vol. 41: , Springer, Dordrecht, (2010) , pp. 45–66. doi:10.1007/978-90-481-3331-4_3. |
[19] | M. Forsberg and M. Hulden, Learning transducer models for morphological analysis from example inflections, in: Proceedings of the SIGFSM Workshop on Statistical NLP and Weighted Automata, B. Jurish, A. Maletti, K. Würzner and U. Springmann, eds, (2016) , pp. 42–50. doi:10.18653/v1/W16-2405. |
[20] | G. Francopoulo, M. George, N. Calzolari, M. Monachini, N. Bel, M. Pet and C. Soria, Lexical markup framework (LMF), in: Proceedings of Theition of the International Conference on Language Resources and Evaluation (LREC 2006), N. Calzolari, K. Choukri, A. Gangemi et al., eds, 5th edn, (2006) , pp. 233–236. |
[21] | G. Francopoulo and P. Paroubek (eds), LMF Lexical Markup Framework, John Wiley & Sons, (2013) . doi:10.1002/9781118712696. |
[22] | G. Gaëtanelle and S. Granger, How can data-driven learning be used in language teaching?, in: The Routledge Handbook of Corpus Linguistics, A. O’Keeffe and M. McCarthy, eds, Routledge, London, (2010) , pp. 387–398. doi:10.4324/9780203856949.ch26. |
[23] | M. Haspelmath, Comparative concepts and descriptive categories in crosslinguistic studies, Language 86: (3) ((2010) ), 663–687, Carlson, G.N. (ed), Linguistic Society of America. doi:10.1353/lan.2010.0021. |
[24] | M. Haspelmath, The Leipzig style rules for linguistics, Max Planck Institute for Evolutionary Anthropology, Leipzig, 2014, http://www.uni-regensburg.de/sprache-literatur-kultur/sprache-literatur-kultur/allgemeine-vergleichende-sprachwissenschaft/medien/pdfs/haspelmath_2014_style_rules_linguistics.pdf. |
[25] | M. Haspelmath and A. Sims, Understanding Morphology, Routledge, (2013) . doi:10.4324/9780203776506. |
[26] | S. Hellmann, Integrating natural language processing (NLP) and language resources using linked data, PhD dissertation, Leipzig University, Leipzig, 2014. |
[27] | S. Hellmann, S. Moran, M. Brümmer and J. McCrae (eds), Special Issue on Multilingual Linked Open Data (MLOD), Semantic Web 6: (4) ((2015) ), IOS Press. |
[28] | N.P. Himmelmann, Documentary and descriptive linguistics, Linguistics 36: (1) ((1998) ), 161–196, Walter de Gruyter, Berlin. doi:10.1515/ling.1998.36.1.161. |
[29] | K. Janowicz, P. Hitzler, B. Adams, D. Kolas and C. Vardeman II, Five stars of linked data vocabulary use, Semantic Web 5: (3) ((2014) ), 173–176, IOS Press. doi:10.3233/sw-140135. |
[30] | C. Kirov, R. Cotterell, J. Sylak-Glassman, G. Walther, E. Vylomova, P. Xia, M. Faruqui et al., UniMorph 2.0: Universal morphology, in: Proceedings of the Eleventh International Conference on Language Resources and Evaluation (LREC 2018), N. Calzolari, K. Choukri, C. Cieri, T. Declerck et al., eds, (2018) , pp. 1868–1873. |
[31] | C. Kirov, J. Sylak-Glassman, R. Que and D. Yarowsky, Very-large scale parsing and normalization of Wiktionary morphological paradigms, in: Proceedings of the Tenth International Conference on Language Resources and Evaluation (LREC 2016), N. Calzolari, K. Choukri, T. Declerck, S. Goggi et al., eds, (2016) , pp. 3121–3126. |
[32] | B. Klimek, Proposing an OntoLex – MMoOn alignment: Towards an interconnection of two linguistic domain models, in: Proceedings of the LDK Workshops: OntoLex, TIAD and Challenges for Wordnets, J.P. McCrae, F. Bond, P. Buitelaar et al., eds, (2017) , pp. 68–83. |
[33] | B. Klimek, M. Ackermann, A. Kirschenbaum and S. Hellmann, Investigating the morphological complexity of German named entities: The case of the GermEval NER challenge, in: Proceedings of the 27th Biennial Conference of the German Society for Computational Linguistics and Language Technology, GSCL 2017: Language Technologies for the Challenges of the Digital Age, G. Rehm and T. Declerck, eds, Springer, Cham, (2017) , pp. 130–145. doi:10.1007/978-3-319-73706-5_11. |
[34] | B. Klimek, N. Arndt, S. Krause and T. Arndt, Creating linked data morphological language resources with MMoOn – the Hebrew morpheme inventory, in: Proceedings of the Tenth International Conference on Language Resources and Evaluation (LREC 2016), N. Calzolari, K. Choukri, T. Declerck, S. Goggi et al., eds, (2016) , pp. 892–899. |
[35] | B. Klimek, J. McCrae, J. Bosque-Gil, M. Ionov, J.K. Tauber and C. Chiarcos, Challenges for the representation of morphology in ontology lexicons, in: Electronic Lexicography in the 21st Century. Proceedings of the eLex 2019 Conference, I. Kosem, T. Zingano Kuhn, M. Correia et al., eds, (2019) , pp. 570–591. |
[36] | C. Lehmann, Data in linguistics, The Linguistic Review 21: (3–4) ((2004) ), 175–210, De Gruyter Mouton. doi:10.1515/tlir.2004.21.3-4.175. |
[37] | C. Lehmann, Interlinear morphemic glossing, in: Morphologie. Ein Internationales Handbuch zur Flexion und Wortbildung 2, G. Booij, C. Lehmann, J. Mugdan and S. Skopeteas, eds, Walter de Gruyter, (2004) , pp. 1834–1857. doi:10.1515/9783110172782.2. |
[38] | C. Lehmann, Linguistic concepts and categories in language description and comparison, in: Typology, Acquisition, Grammaticalization Studies, M. Chini and P. Cuzzolin, eds, Franco Angeli, Milano, (2018) , pp. 27–50. |
[39] | J. McCrae, G. Aguado-de-Cea, P. Buitelaar, P. Cimiano, T. Declerck, A. Gómez-Pérez, J. Gracia et al., Interchanging lexical resources on the semantic web, Language Resources and Evaluation 46: (4) ((2012) ), 701–719, Springer Science and Business Media (LLC). doi:10.1007/s10579-012-9182-3. |
[40] | J.P. McCrae, J. Bosque-Gil, J. Gracia, P. Buitelaar and P. Cimiano, The OntoLex-Lemon model: Development and applications, in: Electronic Lexicography in the 21st Century: Proceedings of ELex 2017 Conference: Lexicography from Scratch, I. Kosem, C. Tiberius, M. Jakubíček, J. Kallas, S. Krek and V. Baisa, eds, (2017) , pp. 587–597. |
[41] | J.P. McCrae, C. Chiarcos, F. Bond, P. Cimiano, T. Declerck, G. De Melo, J. Gracia, S. Hellmann, B. Klimek, S. Moran and P. Osenova, The open linguistics working group: Developing the Linguistic Linked Open Data cloud, in: Proceedings of the Tenth International Conference on Language Resources and Evaluation (LREC 2016), N. Calzolari, K. Choukri, T. Declerck, S. Goggi et al., eds, (2016) , pp. 2435–2441. |
[42] | S. Moran, D. McCloy and R. Wright, PHOIBLE online, Max Planck Institute for Evolutionary Anthropology, Leipzig, 2014, http://phoible.org/. |
[43] | D. Perlmutter, M. Hammond and M. Noonan, The split morphology hypothesis: Evidence from Yiddish, in: Theoretical Morphology: Approaches in Modern Linguistics, M. Hammond and M. Noonan, eds, Academic Press, San Diego, (1988) , pp. 79–100. |
[44] | M. Röder, R. Usbeck, S. Hellmann and D. Gerber, N3 – a collection of datasets for named entity recognition and disambiguation in the NLP interchange format, in: Proceedings of the Ninth International Conference on Language Resources and Evaluation (LREC 2014), N. Calzolari, K. Choukri, T. Declerck, H. Loftsson et al., eds, (2014) , pp. 3529–3533. |
[45] | B. Sagot, The Lefff, a freely available and large-coverage morphological and syntactic lexicon for French, in: Proceedings of the 7th International Conference on Language Resources and Evaluation (LREC 2010), N. Calzolari, K. Choukri, B. Maegaard et al., eds, (2010) , pp. 2744–2751. |
[46] | G. Sérasset, Dbnary: Wiktionary as a lemon-based multilingual lexical resource in RDF, Special Issue on Multilingual Linked Open Data (MLOD), Semantic Web 6: (4) ((2015) ), 355–361, Hellmann, S., Moran, S., Brümmer, M. and McCrae, J. (eds), IOS Press. doi:10.3233/sw-140147. |
[47] | M. Wiegand, A. Balahur, B. Roth, D. Klakow and A. Montoyo, A survey on the role of negation in sentiment analysis, in: Proceedings of the Workshop on Negation and Speculation in Natural Language Processing, R. Morante and C. Sporleder, eds, (2010) , pp. 60–68. |