Compositional changes in human gut microbiota reveal a putative role of intestinal mycobiota in metabolic and biological decline during aging
Abstract
BACKGROUND:
Age-related alterations in the composition and function of gut microbiota may influence human health and disease mechanisms. However, connections between compositional changes in gut bacterial and fungal communities, and their role in the aging process, remain poorly understood.
OBJECTIVE:
Compare the gut microbiota and mycobiota composition in different age groups and evaluate the functionality.
METHODS:
In this study, we performed 16S rRNA and ITS2 gene-based microbial profiling analysis and shotgun metagenomics using the NextSeq platform.
RESULTS:
We observed a shift in compositional changes of human gut microbiota with age. Older individuals revealed a significantly different gut microbiota profile compared to younger individuals. For example, gut microbiota composition of the older individuals showed increase in genera Bacteroides, Blautia, Ruminococcaceae, and Escherichia coli. Additionally, older individuals had significant reduction in fungi belonging to saccharomyces cerevisiae and candida albicans in comparison to their younger counterparts. Moreover, metagenomics functional profiling analysis using shotgun metagenomics sequencing data showed substantial differences in the enrichment of 48 pathways between the young and older age groups. Metabolic pathways such as amino acid biosynthesis, carbohydrate metabolism, cell structure biosynthesis and vitamin biosynthesis were declined in the older age group, in comparison with the younger individuals.
CONCLUSIONS:
The identified differences provide a new insight to enrich our understanding of age-related changes in gut microbiota, their metabolic capabilities, and potential impact on health and disease conditions.
1Introduction
The gut microbiota is a complex ecological system consisting of trillions of microorganisms, including bacteria, archaea, fungi and viruses that play important roles in human health and disease predisposition [1]. Symbiotic gut microbiota contribute to metabolic homeostasis, immune regulation, and the protection against pathogenic microbes [2]. Recent studies have revealed radical age-related differences in the composition, i.e., including both abundance and richness, and the function of gut microbiota [3–5]. Accumulating evidence from the biomedical literature, pointed to a significant decline in the diversity and richness of gut microbiota with age, hinting at possible roles in the age-associated physiologic decline and the pathogenesis of age-related diseases [6, 7]. For example, the diversity of gut microbiota and the number of commensals such as Bacteroides, bifidobacteria, and lactobacilli shrank, while the levels of opportunists such as enterobacteria, Clostridium perfringens, and Clostridium difficile expanded in the older individuals [8, 9]. Another study reported a shift in the microbiota composition toward a Bacteroidetes-dominant population with frailer older individuals [10], while other reports have indicated that the age-associated decline in the abundance of gut lactobacilli and bifidobacteria was linked to the severity of inflammatory disorders such as inflammatory bowel diseases or colonic inflammation [11, 12]. However, these changes in the gut microbiota are also governed by other factors such as diet, sex, and geography [6, 10, 13].
Another important factor that might contribute to age-associated differences in gut microbiota composition and function, is the fungal component of the microbiota (i.e., mycobiota). This factor has not been studied thoroughly before. However, fungi are well-established members of the gut commensals, comprising a small percentage of the total composition of gut microbiota [14]. Recent reports have indicated that despite the small percentage of mycobiota from the total gut microbiota, fungi exert surprisingly strong effects on dampening the inflammatory responses in the gut [15, 16]. Other studies have also revealed a significant role for fungi in shaping host immune responses in inflammatory bowel diseases [15, 17]. Contrarily, other studies suggested that fungi do not routinely colonize the gastrointestinal tract, rather they may have a transient presence derived from the oral cavity and diet [18, 19]. Altogether, previous findings and observations suggest that mycobiota can have a strong impact on the gut ecological communal composition [20, 21]. However, the role of fungi in the biological aging processes and their interactions with the host plus other members of the gut community, remain largely unknown.
In the light of the recent demographical data of the global community, there is a great need to understand the biological aging processes and all factors impacting them to properly plan the future healthcare systems. A recent report from the United Nations indicated that the World population is increasingly aging, with expectations that one in six people in the World (i.e., 16%of the total World population) will be over the age of 65 by 2050 [22], in comparison to the current estimate of one in eleven making up 9%of the World population [23]. This predicted shift in World demographics will eventually result in an increasingly aged population, underscores the importance to identify and understand all factors affecting the biology of aging which will eventually impact healthcare systems and plans.
Human biological aging is characterized by a gradual functional decline in most body organs and biological systems affecting the morbidity and mortality of aging individuals. In fact, the term senescence was coined as an indicator for age-related morbidity and mortality [24]. While earlier research efforts have largely focused on understanding the genetic and environmental factors associated with the onset of age-related diseases, there is a great need to understand all the underlying biological mechanisms that influence one’s health, including the changes in the gut microbiota [6, 25, 26]. Changes in the composition and functionality of gut microbiota have been shown to influence the predisposition and severity of various inflammatory conditions (Claesson et al. 2012). Moreover, homeostasis in gut microbiota has been suggested as a critical factor for healthy aging and human longevity [27, 28].
In this study, we investigated the compositional and functional changes of the gut microbiota with aging using stool samples from 51 human subjects: 18 young individuals (18–29 years old), 13 middle-aged individuals (30–49 years old), and 20 older individuals (50 years and above). Our studies showed significant differences in abundant bacterial and fungal taxa between the young and older study groups. Furthermore, in order to understand the functional effects of the gut microbiome on the host; we conducted a shotgun metagenomics sequencing analysis for ten human subjects representatives of the young and older age groups.
2Materials and methods
This study was performed after receiving the necessary ethical approval from the University Hospital Sharjah, United Arab Emirates. All methods were performed in accordance with relevant guidelines and regulations. An informed consent was obtained from all individuals included in the study. A total of 51 freshly passed stool specimens, each 2 to 4 grams, were collected in sterile container. The stool specimens were stored immediately into liquid nitrogen and then transferred to –80°C for further storage. Liquid (diarrheal) stool, use of probiotics and/or antibiotics in the last 3 months among the volunteers were the only exclusion criteria used in this study. All volunteers reported no major chronic illness and use of other medications with the exception of occasional use of painkillers and aspirin. The demographic information such as age, gender, diet (using dietary fiber intake short food frequency questionnaire (DFI-FFQ) [29], ethnicity, marital status, education level, occupation, exercise, height, and weight were documented. Further, a total of 51 participants were divided into age groups that, broadly defined, young adulthood (18 to 29 years), middle age (30 to 49 years), and older adulthood (50 years and older).
2.1DNA extraction
Fecal samples were subjected to DNA extraction and library preparation. DNA was extracted using the QIAamp®PowerFecal®DNA kit (Qiagen Ltd. GmbH, Germany) following the manufacturer’s instruction (Qiagen Ltd.). DNA quality was evaluated visually via gel electrophoresis and quantified using a Qubit 3.0 fluorometer (Thermo-Fischer, Waltham, MA, USA). Negative controls (no added sample) were used to check for contamination of kit reagents. The extracted DNA was stored at –80°C for further analysis.
2.2PCR, sequencing and sequence processing
Deep amplicon sequencing combined with the principles of statistical ecology was used to survey microbiota communities. Utilizing deep sequencing of the small ribosomal rRNA subunit (16S rRNA) gene and Internal Transcribed Spacer (ITS) region to survey the bacterial and fungal community and gain insights on their population structural composition. Broadly, this was achieved by taking copy variants of the 16S rRNA gene and ITS region, clustering the sequence data into count data in the form of Operational Taxonomic Units (OTUs) following the recommended mothur SOP. The count data, or OTUs, were then classified into taxonomic assignments. Bacterial 16S rRNA genes were amplified using polymerase chain reaction (PCR) with dual-barcoded primers targeting the V4 region (515F 5′-GTGCCAGCMGCCGCGGTAA-3′, and 806R 5′-GGACTACHVGGGTWTCTAAT-3′), as per procedure previously described [30]. Next, amplicons were sequenced with an Illumina MiSeq using the 250-bp paired-end kit (v.2). Sequences were denoised, taxonomically classified using Greengenes (v. 13_8) as the reference database, and clustered into 97%-similarity operational taxonomic units (OTUs) with the mothur software package (v. 1.39.5) previously described [31], following the recommended procedure (https://www.mothur.org/wiki/MiSeq_SOP; accessed August 2018). ITS2 regions were sequenced on an Illumina MiSeq (v. 2 chemistry) using the dual barcoded primers (ITSF 5′-CCTCCGCTTATTGATATGC-3′, ITSR 5′-CCGTGARTCATCGAATCTTTG-3′) as per protocol previously described [30]. Primers and PCR conditions used for 16S and ITS2 sequencing were identical to those previously described [30, 32]. Paired-end reads were merged and curated to reduce sequencing error as described previously [33]. Fungal processing pipeline was identical as the one used for bacteria, except for the high-quality reads were classified using UNITE (v. 7.1) as described before as the reference database [34].
2.3Quality control
The possibility for contamination was examined by co-sequencing DNA amplified from samples and from each template-free controls and extraction kit reagents treated the same way as the samples. Two positive controls, consisting of cloned SUP05 DNA, were also added (number of copies = 2*106). Operational taxonomic units were considered putative contaminants (and were removed) if their mean abundance in controls reached or surpassed 25%of their mean abundance in samples as described before [35].
2.4Statistical analysis
We assessed the alpha diversity with Shannon, Simpson and Chao1 indices on raw OTU abundance tables after filtering out spurious OTUs, and then the significance of diversity changes was tested with Wilcoxon rank sum test (Mann Whitney test) between young and older groups. Next, we evaluated the beta diversity across samples. We excluded OTUs occurring in fewer than 10%of the samples with a count of less than three and calculated Bray-Curtis distance. Then, we evaluated beta diversity, underscoring differences across samples, plotted network and constructed the principal coordinate analysis (PCoA). The dissimilarity in community structure was assessed with permutational multivariate analyses of variance (PERMANOVA) having age groups as the main fixed factor and using 1000 permutations for conducting the test. In order to find significant taxa between young and older age groups, we conducted Wilcoxon rank sum test on relative abundance for each taxon. False Discovery Rate (FDR) p-value adjustments using Benjamini and Hochberg method is used. An adjusted p-value of less than 0.05 is considered significant. It is noteworthy mentioning that throughout the paper we have avoided using tests based on normal theory. All analyses were conducted on the relative OUT abundance with the help of “vegan” package on R 3.5.3 environment.
2.5Shotgun metagenomic analysis
2.5.1DNA extraction and library preparation
DNA was extracted as mentioned before and libraries were prepared using an Illumina Nextera library preparation kit following the standard protocol (Illumina, San Diego, CA, USA).
2.5.2Sequence technology and processing
Sequencing were generated using an Illumina NextSeq. Around 31.6 Gbases generated using 2×150 paired end reads. Each sample yielded a median of 1.8 Gbases. After sequencing, reads were separated according to the barcode used in the library preparation. Initial quality evaluation was done using FastQC v0.11.5. Processing took part in three steps: Paired ends read joining, removing of contaminants, and trimming. Paired-end reads were joined using a fast computational tool to extend the length of short reads by overlapping paired-end reads from fragment libraries that are sufficiently short (FLASH v1.2.11) [36]. Reads were then compared to the Human Genome (hg19, GRCh37 Genome Reference Consortium Human Reference 37) and sequences that mapped to it were removed. Finally, sequences were trimmed according to their quality values using Trimmomatic v0.36 [37] with custom parameters (LEADING:5 TRAILING:5 SLIDINGWINDOW:4 : 15 MINLEN:70).
Joining the paired reads reduced the library size in average by 41.78%. 0.06 %of the stitched reads mapped to the human genome and were removed. Read trimming using quality filters removed 13.56%of the screened reads. At the end of quality control, the median number of quality-filtered reads per sample was 9044385.
2.5.3Functional diversity
Functional profiling of the metagenomic reads was performed using the HUMAnN2 pipeline (http://huttenhower.sph.harvard.edu/humann2). HUMAnN2 performs species-specific and species-agnostic quantification of gene families, EC enzyme modules, and pathways, using the UniRef and MetaCyc databases. Functional profiles produced by HUMAnN2 enumerate the abundance of each gene family in the analyzed microbial communities using the Uniref database (https://www.uniprot.org/help/uniref). Recovered genes were then used to identify a parsimonious set of pathways that explain the observed reactions in the microbial community and uncover important interactions between host and overrepresented microbial communities. This step was also performed by HUMAnN2 and utilizing the Metacyc database [38]. The default gene abundances generated from HUMAnN2 are in reads per Kilobase units. These abundances were then normalized to account for gene length and for sample sequencing depth. Normalized abundances are relative abundances in copies per million units.
3Results
3.1Demographic data of the study subjects
Stool samples from the 51 individuals enrolled in this study were obtained in order to identify and assess the compositional changes in the gut microbiota with age. The 51 human subjects were divided into 18 young individuals, 13 middle-aged individuals, and 20 older individuals. No statistically significant difference in the number of participants in the young group versus the older age group was observed at level of error α= 0.05. All 51 participant were of Emirati ethnicity. The demographic data of the study participant based on age, gender, and ethnicity and high-fiber content diet are reported in Table S1.
3.2Bacterial and fungal sequence curation analysis
The resulting 16S rRNA dataset had 26292 OTUs, with an average of 19887 quality-filtered reads generated per sample. Sequencing quality was determined using FastQC 0.11.5, followed by visualization (Figure S1). Additionally, we sequenced ITS2 amplicons generated from DNA samples on a MiSeq, resulting in a dataset consisting of 4158 OTUs, with an average of 10331 quality-filtered reads generated per sample. The sequencing quality for R1 and R2 was determined using FastQC 0.11.5 followed by visualization (Figure S2).
3.3Alpha (α) diversity metrics
To study the variations in the gut microbiota, alpha diversity metrics were calculated on OTU data for the comparison groups generated based on the age of the 51 study participants. These metrics included Chao1 index for assessing community richness, Simpson index for assessing community diversity, and Shannon index for assessing community evenness or entropy, within each comparison group, i.e., older individuals versus younger individuals. Statistically significant differences at adjusted p-value < 0.05 were determined using Wilcoxon sum rank test with alpha diversity as the response variable on the y-axis, and age as a crossed predictor variable on the x-axis (Fig. 1). The results show the bacterial community diversity was significantly lower in the young age group in comparison with the older age group. Statistical analysis, showed that both young and older age groups differ significantly based on Wilcoxon rank sum test (p-value < 0.05) (Fig. 1A). Alternatively, for fungi, the community richness and diversity in the older age group was significantly lower than that in the young age group. Statistical analysis showed that both age groups differ significantly based on Wilcoxon rank sum test (p-value < 0.05) (Fig. 1B).
Fig. 1
Boxplots of the three studied alpha diversity metrics Chao1, Shannon, and Simpson indices. A) Alpha diversity metrics for the gut bacterial community. B) Alpha diversity metrics for the gut fungal community. Statistically significant differences were determined using Wilcoxon sum rank test, with alpha diversity as the response variable, and age as a crossed predictor variable. Wilcoxon sum rank results show significant differences of Shannon and Simpson diversity indices between young and older age groups for bacteria (max P-value < 0.02). Wilcoxon sum rank results show significant differences of Chao1, Shannon and Simpson diversity indices between young and older age groups for fungi (max p-values < 0.042). Friedman test results show insignificant differences of Chao1, Shannon and Simpson diversity indices among young, middle and older age groups (each P-values > 0.10). The bold point in each box plot represents the average value.
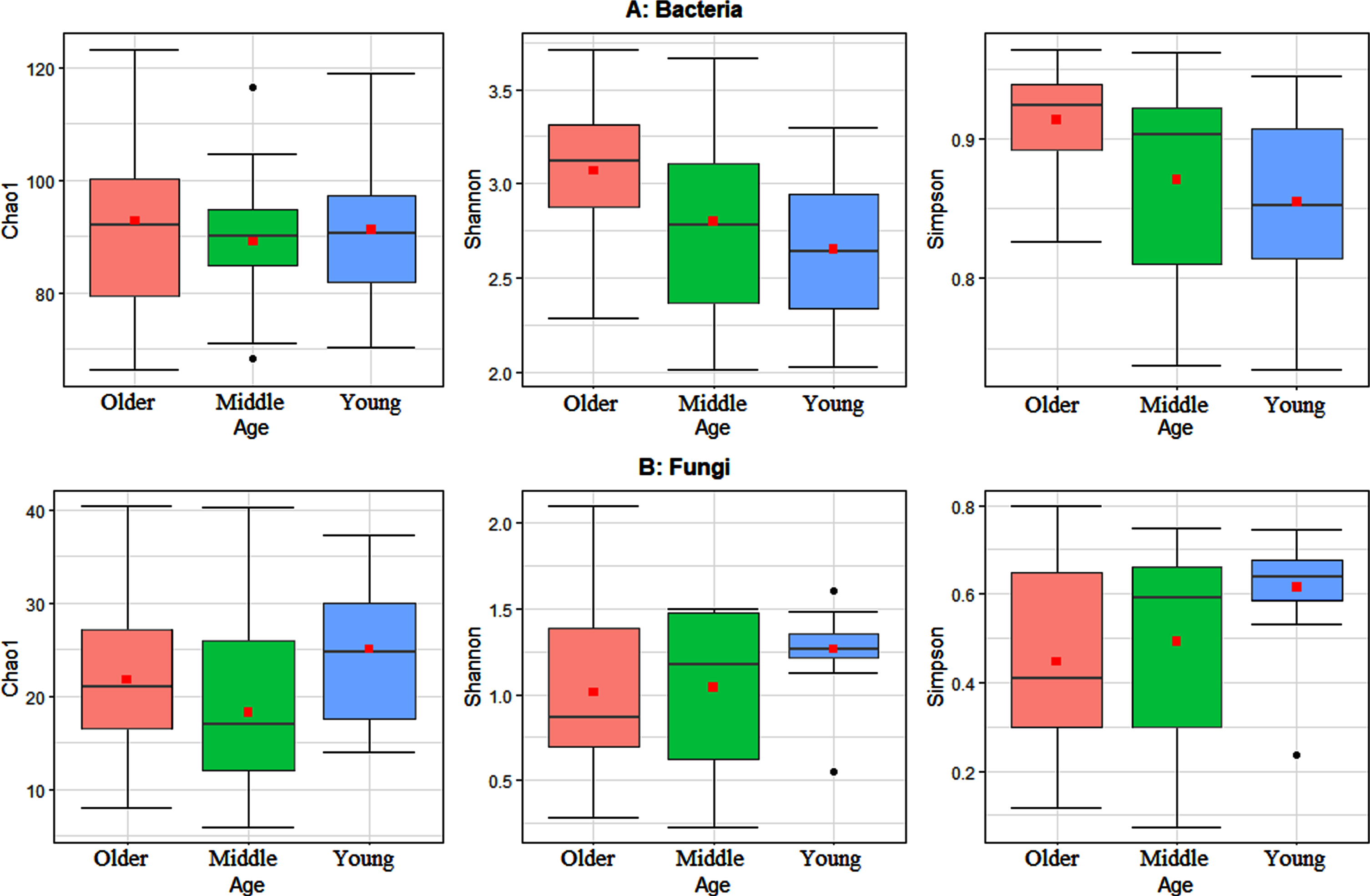
3.4Beta (β) diversity metrics
Principal Coordinates Analysis (PCoA) is a tool commonly used to visualize the profiling of sample clustering based on the similarity to each other, and this helps determine whether changes identified during beta diversity analysis are directed changes or a mere random noise. Therefore, plots for distance matrices were generated using Bray-Curtis dissimilarities as a beta diversity, to assess the separation of samples per each group based on the composition of bacterial and fungal gut communities. Our results showed a significant segregation (p-value < 0.001; permutation test with pseudo-F ratios) between the young and older age groups for both bacteria (Fig. 2A) and fungi (Fig. 2B). Additionally, we outlined relationships among samples of each subgroup in using networks plotted based on Bray-Curtis dissimilarities between both the young and older age groups showing correlation in microbiota composition among samples within each age subgroup for both bacterial (Fig. 2C) and fungal (Fig. 2D) taxa. The beta diversity comparisons between the young, middle and older age groups for both bacterial and fungal microbiota is shown in Figure S3.
Fig. 2
Exploration of beta diversity of bacterial and fungal taxa between young and older individuals. A) A plot of the Constrained Principal Coordinate Analysis (PCoA) based on Bray-Curtis distance matrix between young and older age groups for bacteria. B) A plot of the Constrained Principal Coordinate Analysis (PCoA) based on Bray-Curtis distance matrix between young and older age groups for fungi. A significant segregation between the two groups for both bacteria and fungi was found (p-value < 0.001; permutation test with pseudo-F ratios). C) A network plot based on Bray-Curtis distances for bacteria. D) A network plot based on Bray-Curtis distances for fungi. The nodes are the subjects and two vertices are connected with an edge if the maximum distance is less than or equal a default value of 0.7. The length of the edge is the maximum distance between the two vertices. The package igraph from R software was used to create the network. Older and young corresponding clusters are represented by ♦ and ▲, respectively. The solid shapes in the network plots indicate the 10 samples that were analyzed by shotgun metagenomics. CAP1 and CAP2 represent the canonical principal coordinates 1 and 2.
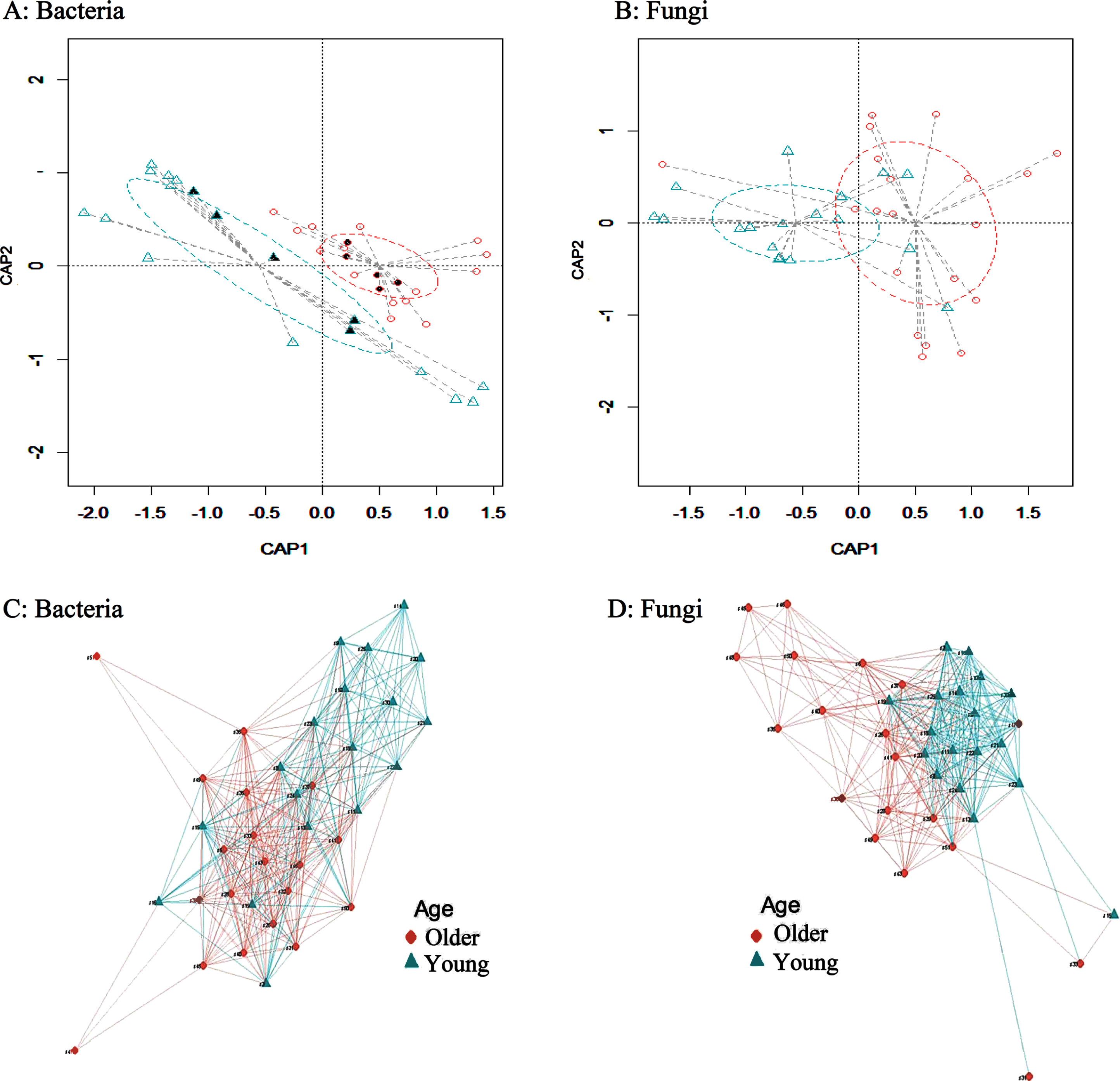
All performed group comparisons of β-diversity were assessed with a non-parametric pairwise permutational multivariate ANOVA (PERMANOVA) as described in the methods section. Our PERMANOVA results showed an overall significant difference of the microbiome communities between young and older groups (p-value < 0.001). PERMANOVA results for the age and gender effects on the bacterial and fungal microbiome communities showed that the interaction effect age*gender (p-value >0.889), and the gender effect were insignificant (p-value > 0.970), whereas age effect was found to be significant (p-value < 0.001), for the identified bacterial taxa. Similarly, the interaction effect age*gender (p-value > 0.271), and the gender effect were statistically insignificant (p-value > 0.163), while the age effect was significant (p-value < 0.001), for the identified fungal taxa. As an additional validation, we also performed pairwise comparisons (using PREMANOVA) between age and gender. Our results indicated that compositional changes in the microbiome were due to age and not due to gender (Table S2). Additional PERMANOVA results showed that confounders such as BMI (p-value > 0.260), diet (p-value > 0.953), and use of probiotics (p-value > 0.319) were all insignificant in comparison to age effect (p-value < 0.001), which was the only significant factor using at 5%level of error (Table S2).
3.5Visualization of the taxonomic profiling results
Stacked bar charts (precisely, 100%stacked) were used for the visualization of the taxonomic profiling results generated from abundance data in OTU tables. These charts enabled the display of the composition and the distribution of most abundant bacterial and fungal taxa at different phylogenetic levels (Figure S4) across studied fecal samples from 51 study participants. The figure clearly show that the taxonomic identity and distribution of the gut microbiota were conserved among tested samples from the same age group.
3.6Univariate analysis
To test if there are any statistically significant differences in the abundance of abundant taxa between young and older age groups, we performed a non-parametric univariate analysis using the Wilcoxon rank sum test. Our results revealed that profiles obtained from older individuals showed significant increase in Bacteroides, Blautia, Ruminococcaceae, and Escherichia coli and depletion in Prevotella, Megamonas, Firmicutes and Sutterella (Fig. 3A). Additionally, we found that profiles obtained from older individuals showed significant differences in the composition of fungal taxa between young and older age groups. The top three most significant differences were observed in Saccharomyces cerevisiae, unclassified Ascomycota, and Candida albicans. (Fig. 3B). The false discovery rate (FDR) p-values reported in Table 1 were used to assess statistical significance of the univariate analysis results. FDR p-value adjustments were calculated using the Benjamini and Hochberg (details are in the Methods section). Adjusted p-values (i.e., FDRs) < 0.05 were considered significant.
Fig. 3
Investigation of significant bacterial and fungal relative abundance difference between young and older individuals. Wilcoxon sum rank test was used to test the relative abundance difference for each taxon. P-values were adjusted using Benjamini and Hochberg False Discovery Rate (FDR) method. The bar plot reports the relative abundance differences between older and young human subjects for significant taxa for both the bacterial (A) and fungal (B) genera.
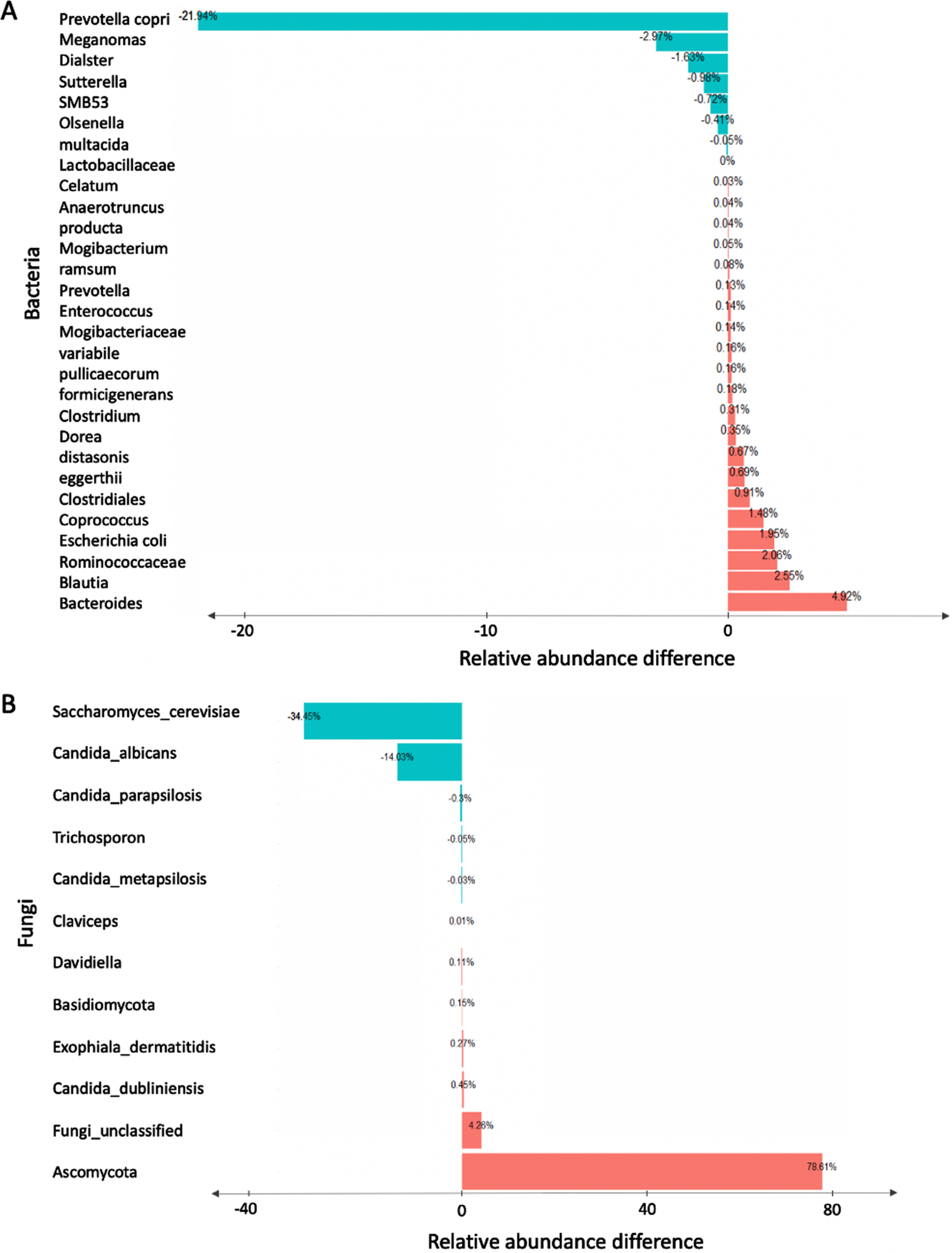
Table 1
The top taxonomic features identified by univariate analysis for the young versus older age groups of study participants
Taxon | Relative Abundance Difference | FDR |
Bacteria | ||
Prevotella | –21.94 % | 0.02 |
Megamonas | –2.97 % | 0.03 |
Firmicutes | –1.63 % | 0.02 |
Sutterella | –0.98 % | 0.03 |
Fungi | ||
Unclassified Ascomycota | 78.61 % | 0.01 |
Unclassified fungi | 4.26 %, | 0.05 |
Candida dubliniensis | 0.45 % | 0.05 |
3.7Shotgun metagenomics
Shotgun metagenomics data for 10 human subjects (S1–S10), from the young and older age groups, was run through the standard workflow for whole metagenome shotgun sequencing described in the Methods section, with the exception of strain profiling (StrainPhlAn). This analysis gave us an idea about the taxonomic profiling of the analyzed shotgun metagenomic DNA reads, in addition to the functional profiling of the gut microbiota.
3.7.1Taxonomic profiling of metagenomics reads
Supplementary Table S3 summarizes the bacterial taxonomy results, on the species level, for all DNA samples obtained from 10 human subjects (S1–S10). These samples were run through MetaPhlAn2 described in the Methods section. Species abundances were passed through a basic filter requiring each species to have at least 0.01%abundance in at least 10%of all samples. A total of 124 species remained after applying the basic filtering of the 126 total unfiltered species. The top 15 most abundant bacterial species in all analyzed samples (S1–S10) are shown in supplementary Figure S5.
3.7.2Functional profiling of the metagenomics reads
In order to identify the functional relationships between the composition of gut microbiota and age-related biological processes, we conducted a metagenomics functional profiling analysis using shotgun metagenomic sequencing data for 10 human study subjects from the young and older age groups. Representative samples for the metagenomics analysis were selected based on their compositional microbial profiles identified from the β-diversity analysis; selecting samples from human subjects that had microbial composition closer to the average for each group (i.e., for both young and older groups) obtained from the PCoA analysis (Fig. 2). The metagenomics analysis was performed by mapping around 63.54 %reads to established functional genes. Statistical differences in pathway richness (i.e., the number of unique pathways) were calculated using a generalized linear model. Our pathway results showed substantial differences in the enrichment results of 48 pathways between the young and older age groups (Fig. 4). Pathways that had lower abundance in the older age group, in comparison with the young group, involved the following pathway categories: 1) amino acid biosynthesis pathways, such as PWY-724 superpathway of L-lysine, L-threonine and L-methionine biosynthesis II, PWY-2942 L-lysine biosynthesis III, and PWY-5097 L-lysine biosynthesis VI; 2) Carbohydrate metabolism pathways, such as PWY-7111 pyruvate fermentation, PWY-1042 glycolysis IV, and PWY-6737 starch degradation V; 3) cell structure biosynthesis pathways, such as PWY-6386, UDP-N-acetylmuramoyl-pentapeptide biosynthesis II and PWY-6387, UDP-N-acetylmuramoyl-pentapeptide biosynthesis I; and 4) Vitamin biosynthesis pathways, such as PWY-3841 folate transformations II and PWY-7357 thiamine formation from pyrithiamine and oxythiamine.
Fig. 4
Functional profiling of young and older adulthood microbiota based on shotgun metagenomics. Derived from whole metagenome shotgun data, the bar plot shows log2 ratios of the average pathway abundance for young and older subjects of each pathway. Older and young pathways that are more abundant are represented in white and black, respectively.
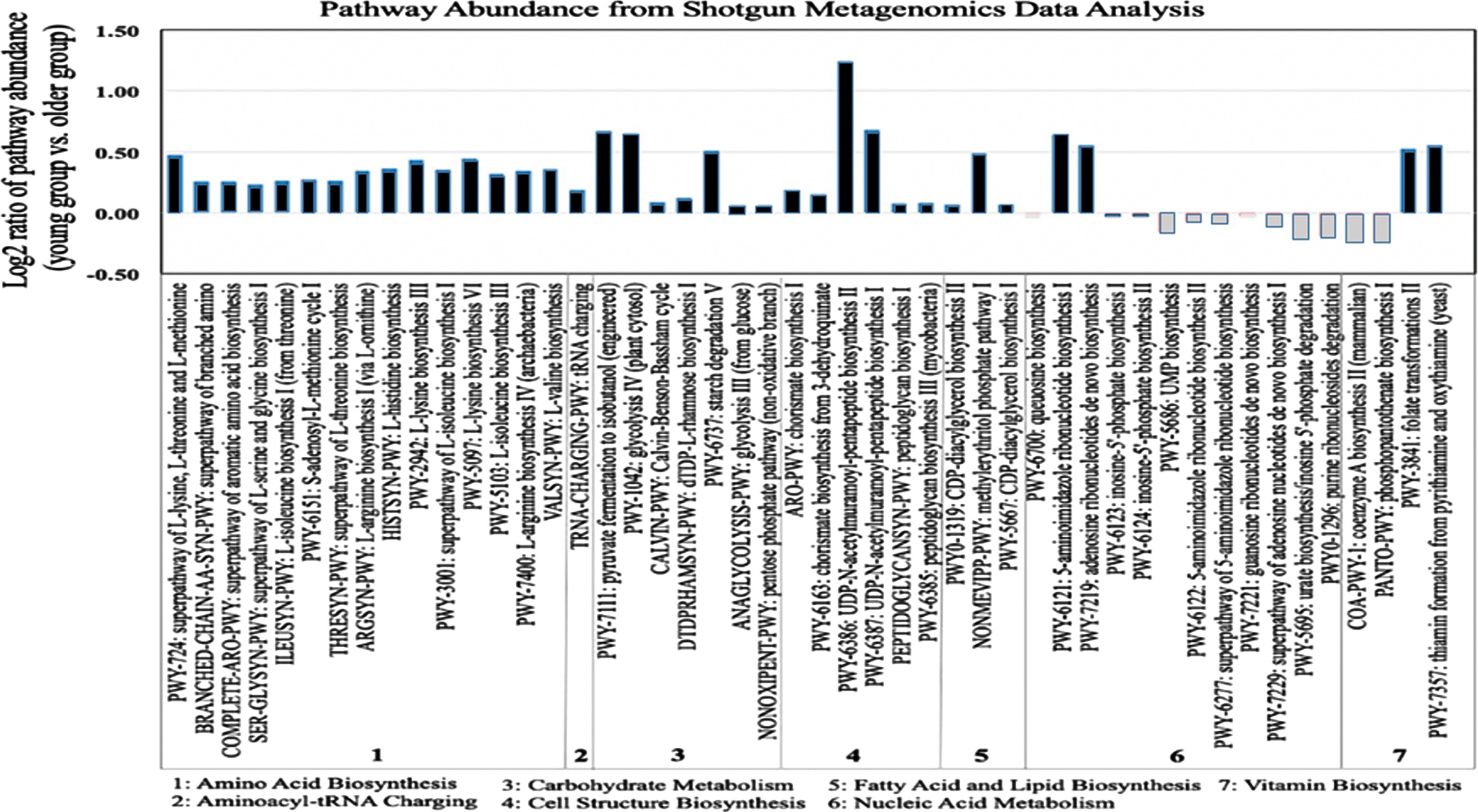
Furthermore, we evaluated the correlation between bacterial genera and metabolic pathways with statistically significant differential abundance between young and older age groups (Table S4). PWY-6386 (UDP-N-acetylmuramoyl-pentapeptide biosynthesis II) was the most differentially abundant pathway between the young and older group, showing a statistically significant bias towards the young group. In order to understand the significance on this pathway for biological aging, we mined the MetaCyc pathway database for further information and identified list of genes enriching PWY-6386 pathway. The expected genomic range for this pathway included the genera Actinobacter and Firmicutes (Table S5).
4Discussion
The study of age-related changes in human gut microbiota is a rapidly developing area of research [7, 39, 40], but a detailed description of the gut mycobiota impact is still missing. To the best of our knowledge, this is the first report on the emerging gut mycobiota role in aging among Middle Eastern populations. In this study, we determined shift in compositional changes of human gut microbiota with age. First, we described the bacterial taxonomic composition among different age groups. Biological aging exerts strong effect on bacterial richness and complexity [6, 41, 42]. The observed higher bacterial richness among the older adulthood age group may correlate with certain biological functions related to aging. Indeed, changes in bacterial composition were found to play a major role in age-related illnesses such as chronic inflammation, constipation, and impaired cognition [25, 43, 44]. For example, we reported change in ratio of Prevotella taxa (relative abundance difference between older and young groups of –21.9351%, p-value < 0.0232) among the older age group which can contribute to an instability in the microbiota composition and increase inflammation according to Claesson et al. [6]. On the other side, we noted greater proportion of Bacteroides spp. (relative abundance difference of 4.9234%, p-value < 0.029) in our older age group when compared to younger individuals and distinct density patterns of Clostridium (relative abundance difference of 0.91%, p-value < 0.0430) and E.coli (relative abundance difference of 1.95%, p-value < 0.0112) in the older [6, 8, 45]. These bacterial alterations belonging to pathobionts, a microbiota opportunist that are able to thrive and sustain inflammatory environment (Biagi et al. 2013). We therefore correlate that pathobiont overgrowth might contribute to inflammation which affect health condition of older individuals.
As a second objective of our study, we explored gut fungal communities (mycobiota). Gut mycobiota was first characterized as members of the normal gut flora in 1967, comprises a small percentage of the total gut microbiota (1%) [46]. Very little is known about the impact of gut mycobiota on aging [47]. In this study, we report an intriguing compositional change in the bacterial and fungal communities of the gut microbiota with age. Our results showed significant changes in richness and composition of the gut fungal taxa between young and older human subjects. Perhaps age-associated changes in the gut microenvironment, including a declining host metabolic rate, could possibly drive these compositional changes in the commensal fungal community to maintain homeostasis. Several reports suggested that mycobiota can significantly influence the gut bacterial composition and may induce chronic inflammatory responses [15, 16, 20, 21]. For example, several reports have suggested that mycobiota-induced dysbiosis in mice led to worsened outcome of colitis and allergic airway disease [15, 48]. Interestingly, our results revealed an increased density of unclassified Ascomycota among the older individuals (relative abundance difference of 78.61%p-value < 0.05) in comparison to the young population. Alternatively, a significant reduction in Saccharomyces (relative abundance difference of –0.30%, p-value < 0.0020) was observed. A recent study found that Candida albicans mono-colonization efficiently reversed dextran sodium sulfate (DSS)-induced colitis after antibiotic induced eradication of commensal bacteria in mice [49]. Extrapolating the findings of Jiang et al to our study results suggests that the age-associated increase in unclassified Ascomycota composition may reduce age-related inflammation in by attempting to maintain the overall microbial gut homeostasis. Additionally, our results also showed a decrease in Candida albicans, and an increase in Candida dubliniensis number among the older age group (relative abundance difference of 0.45%, p-value < 0.05). The overall compositional changes in gut mycobiota with age, identified in our study, suggest a putative role in biological processes of aging, underscoring the need for comprehensive functional investigations on the gut microbiota.</para>
In order to detect further taxonomic differences at a higher resolution, and also get a glimpse of the putative functional relationships between the observed compositional changes in gut microbiota and age-related biological processes, we performed a shotgun metagenomics analysis. Our results revealed that the top statistically significant genes (Tables S4 and S5) in differentiating between the older and young individuals led to the enrichment of important biological pathways involved in aging, including amino acid biosynthesis, carbohydrate metabolism, fatty acid and lipid biosynthesis among others (Fig. 4). These biological pathways are implicated in production of short-chain fatty acids (SCFAs) and the regulation of the saccharolytic potential [50–52]. For example, a putrefactive dysbiosis could be caused by an increase in putrefactive bacteria such as the unclassified Bacteroides (relative abundance difference of 4.923%, p-value < 0.0294) known to produce toxic metabolites such as ammonia, amines, and phenols which may constitute important predisposition factors for many gut diseases [53, 54]. Furthermore, SCFAs are also known to play an important role in immune regulation and gut barrier function [55–57]. Interactions between SCFAs and GPR43 receptor affect inflammatory responses in mice models of colitis, arthritis and asthma [57]. Therefore, the lack of SCFAs may sustain a pro-inflammatory environment, worsening the health condition of aged population [27, 58]. Altogether, these results suggest that the gut microbiota in older individuals was structurally and functionally compromised, and biologically biased towards putrefactive metabolism. Most importantly, our analysis identified PWY-6386 (UDP-N-acetylmuramoyl-pentapeptide biosynthesis II) as the top most differentially abundant biological pathway in the young individuals versus their older counterparts. This pathway is particularly important for peptidoglycan biosynthesis in most Gram-positive bacteria. Mounting evidence indicates that gut microbiota exerts a wide range of effects on host physiology and development through the effects of their diverse peptidoglycans [59]. For example, peptidoglycans can cross the blood brain barrier, and they can also signal through Nod2 leading to the suppression the pathological inflammatory response that may predispose to aging and disease [60] Glucosamine is highly concentrated in joint tissues, and these storage sites are depleted with age resulting in adverse age-related changes in joints.. However, more studies are needed to elucidate the exact mechanisms of action of glucosamine, UDP-N-acetyl-α-D-glucosamine, and peptidoglycan as signaling molecules with putative effects on aging pathways.
It is important to mention that the participants in this study belonged to a limited geographic area (Sharjah, UAE), and shared similar lifestyle and dietary habits that facilitated studying the effect of age on gut bacterial and fungal compositional changes. We also controlled for dietary fiber intake using DFI-FFQ and determined no significant difference between all age groups (p-value > 0.74) (Table S1).
5Conclusions
In conclusion, our data reports on important compositional and functional changes in the gut microbiota with age. We observed an overgrowth of potential pathobionts based on the relative ratio changes of significant taxa between older human subjects and younger individuals. The investigated bacterial and fungal taxonomic profiles, suggested a biological shift towards inflammation in older people. Furthermore, older individuals exhibited lower density of bacterial genes enriched in pathways that are directly involved in the biosynthesis of SCFAs and the regulation of the saccharolytic potential. We also showed that the compositional changes in the gut microbiota were not affected by gender, weight, diet or the use of probiotics assessed in our study cohort. Altogether, our findings suggest a putative role for the gut microbiota in the development of biological aging. However, these finding showed be followed by additional validation studies on larger cohorts involving populations with different environmental conditions and genetic backgrounds. Most likely, these results can provide screening biomarkers to predict age-related disease and promote healthy aging in an era of expanded elderly population with increased demands of proper healthcare.
Acknowledgments
Microbiota sequence data provided by microbiota insights, Canada.
Funding
This study was funded by Research Institute for Medical and Health Sciences at University of Sharjah. (Grant no P1701090226). The funding source stated above had no role in study design, data collection and analysis, decision to publish, or preparation of the manuscript.
Conflict of interest
The authors declare that the research was conducted in the absence of any commercial or financial relationships that could be construed as a potential conflict of interest.
Supplementary data
[1] The supplementary data is available is the repository GitHub. Can be accessed using the link https://github.com/nihardash69/Gut-Microbiota-and-age.git.
References
[1] | Blaser MJ , Falkow S . What are the consequences of the disappearing human microbiota? Nature reviews Microbiology. (2009) ;7: (12):887–94. Epub 2009/11/10. doi: 10.1038/nrmi-cro2245. PubMed PMID: 19898491. |
[2] | Ballal SA , Gallini CA , Segata N , Huttenhower C , Garrett WS . Host and gut microbiota symbiotic factors: lessons from inflammatory bowel disease and successful symbionts. Cellular microbiology. (2011) ;13: (4):508–17. Epub 2011/02/15.doi: 10.1111/j.1462-5822.2011.01572.x. PubMed PMID: 21314883. |
[3] | Merchant HA , Liu F , Orlu Gul M , Basit AW . Age-mediated changes in the gastrointestinal tract. International journal of pharmaceutics. (2016) ;512: (2):382–95. Epub 2016/04/18. doi: 10.1016/j.ijpharm.2016.04.024. PubMed PMID: 27085646. |
[4] | Odamaki T , Kato K , Sugahara H , Hashikura N , Takahashi S , Xiao JZ , et al. Age-related changes in gut microbiota composition from newborn to centenarian: a cross-sectional study. BMC microbiology. (2016) ;16: :90. Epub 2016/05/26. doi: 10.1186/s12866-016-0708-5. PubMed PMID: 27220822; PubMed Central PMCID: PMC4879732. |
[5] | Partridge L , Deelen J , Slagboom PE . Facing up to the global challenges of ageing. Nature. (2018) ;561: (7721):45–56. Epub 2018/09/07. doi: 10.1038/s41586-018-0457-8. PubMed PMID: 30185958. |
[6] | Claesson MJ , Jeffery IB , Conde S , Power SE , O’Connor EM , Cusack S , et al. Gut microbiota composition correlates with diet and health in the elderly. Nature. (2012) ;488: (7410):178–84. Epub 2012/07/17. doi: 10.1038/nature11319. PubMed PMID: 22797518. |
[7] | Yatsunenko T , Rey FE , Manary MJ , Trehan I , Dominguez-Bello MG , Contreras M , et al. Humangut microbiome viewed across age and geography. Nature. (2012) ;486: (7402):222–7. Epub 2012/06/16. doi: 10.1038/nature11053. PubMed PMID: 22699611; PubMed Central PMCID: PMC3376388. |
[8] | Claesson MJ , Cusack S , O’Sullivan O , Greene-Diniz R , de Weerd H , Flannery E , et al. Composition, variability, and temporal stability of the intestinal microbiota of the elderly. Proceedings of the National Academy of Sciences of the United States of America. (2010) ;108 Suppl 1: :4586–91. Epub 2010/06/24. doi: 10.1073/pnas.1000097107. PubMed PMID: 20571116; PubMed Central PMCID: PMC3063589. |
[9] | O’Toole PW , Jeffery IB . Gut microbiota and aging. Science. (2015) ;350: (6265):1214–5. Epub 2016/01/20. doi: 10.1126/sci-ence.aac8469. PubMed PMID: 26785481. |
[10] | Jeffery IB , Lynch DB , O’Toole PW . Composition and temporal stability of the gut microbiota in older persons. The ISME journal. (2015) ;10: (1):170–82. Epub 2015/06/20. doi: 10.1038/ismej.2015.88. PubMed PMID: 26090993; PubMed Central PMCID: PMC4681863. |
[11] | Tojo R , Suarez A , Clemente MG , de los Reyes-Gavilan CG , Margolles A , Gueimonde M , et al. Intestinal microbiota in health and disease: role of bifidobacteria in gut homeostasis. World journal of gastroenterology. (2014) ;20: (41):15163–76. Epub 2014/11/12. doi: 10.3748/wjg.v20.i41.15163. PubMed PMID: 25386066; PubMed Central PMCID: PMC4223251. |
[12] | Arnbjerg CJ , Vestad B , Hov JR , Pedersen KK , Jespersen S , Johannesen HH , et al. Effect of Lactobacillus rhamnosus GG Supplementation on Intestinal Inflammation Assessed by PET/MRI Scans and Gut Microbiota Composition in HIV-Infected Individuals. J Acquir Immune Defic Syndr. (2018) ;78: (4):450–7. Epub 2018/06/07. doi: 10.1097/QAI.0000000000001693. PubMed PMID: 29874201. |
[13] | Fransen F , van Beek AA , Borghuis T , Meijer B , Hugenholtz F , van der Gaast-de Jongh C , et al. The Impact of Gut Microbiota on Gender-Specific Differences in Immunity. Frontiers in immunology. (2017) ;8: :754. Epub 2017/07/18. doi: 10.3389/fimmu.2017.00754. PubMed PMID: 28713378; PubMed Central PMCID: PMC5491612. |
[14] | Gorbach SL , Nahas L , Lerner PI , Weinstein L . Studies of intestinal microflora. I. Effects of diet, age, and periodic sampling on numbers of fecal microorganisms in man. Gastroenterology. (1967) ;53: (6):845–55. Epub 1967/12/01. PubMed PMID: 4863721. |
[15] | Wheeler ML , Limon JJ , Bar AS , Leal CA , Gargus M , Tang J , et al. Immunological Consequences of Intestinal Fungal Dysbiosis. Cell host & microbe. (2016) ;19: (6):865–73. Epub 2016/05/31. doi: 10.1016/j.chom.2016.05.003. PubMed PMID: 27237365; PubMed Central PMCID: PMC4900921. |
[16] | Sokol H , Leducq V , Aschard H , Pham HP , Jegou S , Landman C , et al. Fungal microbiota dysbiosis in IBD. Gut. (2016) ;66: (6):1039–48. Epub 2016/02/05. doi: 10.1136/gutjnl-2015-310746. PubMed PMID: 26843508; PubMed Central PMCID: PMC5532459. |
[17] | Levy M , Kolodziejczyk AA , Thaiss CA , Elinav E . Dysbiosis and the immune system. Nature reviews Immunology. (2017) ;17: (4):219–32. Epub 2017/03/07. doi: 10.1038/nri.2017.7. PubMed PMID: 28260787. |
[18] | Hoffmann C , Dollive S , Grunberg S , Chen J , Li H , Wu GD , et al. Archaea and fungi of the human gut microbiome: correlations with diet and bacterial residents. PloS one. (2013) ;8: (6):e66019. Epub 2013/06/27. doi: 10.1371/journal.pone.0066019. PubMed PMID: 23799070; PubMed Central PMCID: PMC3684604. |
[19] | Auchtung TA , Fofanova TY , Stewart CJ , Nash AK , Wong MC , Gesell JR , et al. Investigating Colonization of the Healthy Adult Gastrointestinal Tract by Fungi. mSphere. (2018) ;3: (2). Epub 2018/03/31. doi: 10.1128/mSphere.00092-18. PubMed PMID: 29600282; PubMed Central PMCID: PMC5874442. |
[20] | Zuo T , Wong SH , Cheung CP , Lam K , Lui R , Cheung K , et al. Gut fungal dysbiosis correlates with reduced efficacy of fecal microbiota transplantation in Clostridium difficile infection. Nature communications. (2018) ;9: (1):3663. Epub 2018/09/12. doi: 10.1038/s41467-018-06103-6. PubMed PMID: 30202057; PubMed Central PMCID: PMC6131390. |
[21] | Limon JJ , Skalski JH , Underhill DM . Commensal Fungi in Health and Disease. Cell host & microbe. (2017) ;22: (2):156–65. Epub 2017/08/12. doi: 10.1016/j.chom.2017.07.002.. PubMed PMID: 28799901; PubMed Central PMCID: PMC5573128. |
[22] | United Nations NY, Ny. Department of Economic, Affairs S. World population ageing, 1950-2050: United Nations Publications; (2002) . |
[23] | Newgard CB , Sharpless NE . Coming of age: molecular drivers of aging and therapeutic opportunities. The Journal of clinical investigation. (2013) ;123: (3):946–50. Epub 2013/03/05. doi: 10.1172/JCI68833. PubMed PMID: 23454756; PubMed Central PMCID: PMC3582156. |
[24] | Levine ME . Modeling the rate of senescence: can estimated biological age predict mortality more accurately than chronological age? The journals of gerontology Series A, Biological sciences and medical sciences. (2012) ;68: (6)::667–74. Epub 2012/12/06. doi: 10.1093/gerona/gls233. PubMed PMID: 23213031; PubMed Central PMCID: PMC3660119. |
[25] | Maffei VJ , Kim S , Blanchard Et , Luo M , Jazwinski SM , Taylor CM , et al. Biological Aging and the Human Gut Microbiota. The journals of gerontology Series A, Biological sciences and medical sciences. (2017) ;72: (11):1474–82. Epub 2017/04/27. doi: 10.1093/gerona/glx042. PubMed PMID: 28444190; PubMed Central PMCID: PMC5861892. |
[26] | Biagi E , Franceschi C , Rampelli S , Severgnini M , Ostan R , Turroni S , et al. Gut Microbiota and Extreme Longevity. Current biology : CB. (2016) ;26: (11):1480–5. Epub 2016/05/18. doi: 10.1016/j.cub.2016.04.016. PubMed PMID: 27185560. |
[27] | Biagi E , Candela M , Turroni S , Garagnani P , Franceschi C , Brigidi P . Ageing and gut microbes: perspectives for health maintenance and longevity. Pharmacological research. (2012) ;69: (1):11–20. Epub 2012/10/20. doi: 10.1016/j.phrs.2012.10.005. PubMed PMID: 23079287. |
[28] | Han B , Sivaramakrishnan P , Lin CJ , Neve IAA , He J , Tay LWR , et al. Microbial Genetic Composition Tunes Host Longevity. Cell. (2017) ;169: (7):1249–62 e13. Epub 2017/06/18. doi: 10.1016/j.cell.2017.05.036. PubMed PMID: 28622510; PubMed Central PMCID: PMC5635830. |
[29] | Healey G , Brough L , Murphy R , Hedderley D , Butts C , Coad J . Validity and Reproducibility of a Habitual Dietary Fibre Intake Short Food Frequency Questionnaire. Nutrients. (2016) ;8: (9). Epub 2016/09/15. doi: 10.3390/nu8090558. PubMed PMID: 27626442; PubMed Central PMCID: PMC5037543. |
[30] | Kozich JJ , Westcott SL , Baxter NT , Highlander SK , Schloss PD . Development of a dual-index sequencing strategy and curation pipeline for analyzing amplicon sequence data on the MiSeq Illumina sequencing platform. Applied and environmental microbiology. (2013) ;79: (17):5112–20. Epub 2013/06/25. doi: 10.1128/AEM.01043-13. PubMed PMID: 23793624; PubMed Central PMCID: PMC3753973. |
[31] | Schloss PD , Westcott SL , Ryabin T , Hall JR , Hartmann M , Hollister EB , et al. Introducing mothur: open-source, platform-independent, community-supported software for describing and comparing microbial communities. Applied and environmental microbiology. (2009) ;75: (23):7537–41. Epub 2009/10/06. doi: 10.1128/AEM.01541-09. PubMed PMID: 19801464; PubMed Central PMCID: PMC2786419. |
[32] | Gweon HS , Oliver A , Taylor J , Booth T , Gibbs M , Read DS , et al. PIPITS: an automated pipeline for analyses of fungal internal transcribed spacer sequences from the Illumina sequencing platform. Methods in ecology and evolution. (2016) ;6: (8):973–80. Epub 2016/08/30. doi: 10.1111/2041-210X.12399. PubMed PMID: 905 27570615; PubMed Central PMCID: PMC4981123. |
[33] | Huse SM , Welch DM , Morrison HG , Sogin ML . Ironing out the wrinkles in the rare biosphere through improved OTU clustering. Environmental microbiology. (2010) ;12: (7):1889–98. Epub 2010/03/20. doi: 10.1111/j.1462-2920.2010.02193.x. PubMed PMID: 20236171; PubMed Central PMCID: PMC2909393. |
[34] | Koljalg U , Larsson KH , Abarenkov K , Nilsson RH , Alexander IJ , Eberhardt U , et al. UNITE: a database providing web-based methods for the molecular identification of ectomycorrhizal fungi. The New phytologist. (2005) ;166: (3):1063–8. Epub 2005/05/05. doi: 10.1111/j.1469-8137.2005.01376.x. PubMed PMID: 15869663. |
[35] | Dash NR , Khoder G , Nada AM , Al Bataineh MT . Exploring the impact of Helicobacter pylori on gut microbiome composition. PloS one. (2019) ;14: (6):e0218274. Epub 2019/06/19. doi: 10.1371/journal.pone.0218274. PubMed PMID: 31211818; PubMed Central PMCID: PMC6581275. |
[36] | Magoc T , Salzberg SL . FLASH: fast length adjustment of short reads to improve genome assemblies. Bioinformatics. (2011) ;27: (21):2957–63. Epub 2011/09/10. doi: 10.1093/bioinformatics/btr507. PubMed PMID: 21903629; PubMed Central PMCID: PMC3198573. |
[37] | Bolger AM , Lohse M , Usadel B . Trimmomatic: a flexible trimmer for Illumina sequence data. Bioinformatics. (2014) ;30: (15):2114–20. Epub 2014/04/04. doi: 10.1093/bioinformatics/btu170. PubMed PMID: 24695404; PubMed Central PMCID: PMC4103590. |
[38] | Caspi R , Billington R , Ferrer L , Foerster H , Fulcher CA , Keseler IM , et al. The MetaCyc database of metabolic pathways and enzymes and the BioCyc collection of pathway/genome databases. Nucleic acids research. (2015) ;44: (D1):D471–80. Epub 2015/11/04. doi: 10.1093/nar/gkv1164. PubMed PMID: 26527732; PubMed Central PMCID: PMC4702838. |
[39] | Aleman FDD , Valenzano DR . Microbiome evolution during host aging. PLoS pathogens. (2019) ;15: (7):e1007727. Epub 2019/07/26. doi: 10.1371/journal.ppat.1007727. PubMed PMID: 31344129; PubMed Central PMCID: PMC6657895. |
[40] | Vandeputte D , Kathagen G , D’Hoe K , Vieira-Silva S , Valles-Colomer M , Sabino J , et al. Quantitative microbiome profiling links gut community variation to microbial load. Nature. (2017) ;551: (7681):507–11. Epub 2017/11/17. doi: 10.1038/nature24460. PubMed PMID: 29143816. |
[41] | Callaway E . ‘Young poo’ makes aged fish live longer. Nature. (2017) ;544: (7649):147. Epub 2017/04/14. doi: 10.1038/nature.2017.21770. PubMed PMID: 28406214. |
[42] | Smith P , Willemsen D , Popkes M , Metge F , Gandiwa E , Reichard M , et al. Regulation of life span by the gut microbiota in the short-lived African turquoise killifish. eLife. 2017;6. Epub 2017/08/23. doi: 10.7554/eLife.27014. PubMed PMID: 28826469; PubMed Central PMCID: PMC5566455. |
[43] | Fransen F , van Beek AA , Borghuis T , Aidy SE , Hugenholtz F , van derGaast-de Jongh C , et al. Aged Gut Microbiota Contributes to Systemical Inflammaging after Transfer to Germ-Free Mice. Frontiers in immunology. (2017) ;8: :1385. Epub 2017/11/23. doi: 10.3389/fimmu.2017.0138. PubMed PMID: 29163474; PubMed Central PMCID: PMC5674680. |
[44] | Marizzoni M , Provasi S , Cattaneo A , Frisoni GB . Microbiota and neurodegenerative diseases. Current opinion in neurology. (2017) ;30: (6):630–8. Epub 2017/09/15. doi: 10.1097/WCO.0000000000000496. PubMed PMID: 28906270. |
[45] | Buford TW . (Dis)Trust your gut: the gut microbiome in age-related inflammation, health, and disease. Microbiome. (2017) ;5: (1):80. Epub 2017/07/16. doi: 10.1186/s40168-017-0296-0. PubMed PMID: 28709450; PubMed Central PMCID: PMC5512975. |
[46] | Gorbach SL , Plaut AG , Nahas L , Weinstein L , Spanknebel G , Levitan R . Studies of intestinal microflora. II. Microorganisms of the small intestine and their relations to oral and fecal flora. Gastroenterology. (1967) ;53: (6):856–67. Epub 1967/12/01. PubMed PMID: 4863722. |
[47] | Richard ML , Sokol H . The gut mycobiota: insights into analysis, environmental interactions and role in gastrointestinal diseases. Nature reviews Gastroenterology & hepatology. (2019) ;16: (6):331–45. Epub 2019/03/03. doi: 10.1038/s41575-019-0121-2. PubMed PMID: 30824884. |
[48] | Underhill DM , Iliev ID . The mycobiota: interactions between commensal fungi and the host immune system. Nature reviews Immunology. (2014) ;14: (6):405–16. Epub 2014/05/24. doi: 10.1038/nri3684. PubMed PMID: 24854590; PubMed Central PMCID: PMC4332855. |
[49] | Jiang TT , Shao TY , Ang WXG , Kinder JM , Turner LH , Pham G , et al. Commensal Fungi Recapitulate the Protective Benefits of Intestinal Bacteria. Cell host & microbe. (2017) ;22: (6):809–16 e4 . Epub 2017/11/28. doi: 10.1016/j.chom.2017.10.013. PubMed PMID: 29174402; PubMed Central PMCID: PMC5730478. |
[50] | Wang G , Yu Y , Wang YZ , Wang JJ , Guan R , Sun Y , et al. Role of SCFAs in gut microbiome and glycolysis for colorectal cancer therapy. Journal of cellular physiology. 2019. Epub 2019/03/20. doi: 10.1002/jcp.28436. PubMed PMID: 30888065. |
[51] | Rampelli S , Candela M , Turroni S , Biagi E , Collino S , Franceschi C , et al. Functional metagenomic profiling of intestinal microbiome in extreme ageing. Aging. (2013) ;5: (12):902–12. Epub 2013/12/18. doi: 10.18632/aging.100623. PubMed PMID: 24334635; PubMed Central PMCID: PMC3883706. |
[52] | Vieira-Silva S , Falony G , Darzi Y , Lima-Mendez G , Garcia Yunta R , Okuda S , et al. Species-function relationships shape ecological properties of the human gut microbiome. Nature microbiology. (2016) ;1: (8):16088. Epub 2016/08/31. doi: 10.1038/nmicrobiol.2016.88. PubMed PMID: 27573110. |
[53] | Murphy EA , Velazquez KT , Herbert KM . Influence of high-fat diet on gut microbiota: a driving force for chronic disease risk. Current opinion in clinical nutrition and metabolic care. (2015) ;18: (5):515–20. Epub 2015/07/15. doi: 10.1097/MCO.0000000000000209. PubMed PMID: 26154278; PubMed Central PMCID: PMC4578152. |
[54] | Gagliardi A , Totino V , Cacciotti F , Iebba V , Neroni B , Bonfiglio G , et al. Rebuilding the Gut Microbiota Ecosystem. International journal of environmental research and public health. (2018) ;15: (8). Epub 2018/08/09. doi: 10.3390/ijerph15081679. PubMed PMID: 30087270; PubMed Central PMCID: PMC6121872. |
[55] | Chen L , Sun M , Wu W , Yang W , Huang X , Xiao Y , et al. Microbiota Metabolite Butyrate Differentially Regulates Th1 and Th17 Cells’ Differentiation and Function in Induction of Colitis. Inflammatory bowel 1028 diseases. 2019. Epub 2019/03/29. doi: 10.1093/ibd/izz046. PubMed PMID: 30918945. |
[56] | Parada Venegas D , De la Fuente MK , Landskron G , Gonzalez MJ , Quera R , Dijkstra G , et al. Short Chain Fatty Acids (SCFAs)-Mediated Gut Epithelial and Immune Regulation and Its Relevance for Inflammatory Bowel Diseases. Frontiers in immunology. (2019) ;10: :277. Epub 2019/03/28. doi: 10.3389/fimmu.2019.00277. PubMed PMID: 30915065; PubMed Central PMCID: PMC6421268. |
[57] | Maslowski KM , Vieira AT , Ng A , Kranich J , Sierro F , Yu D , et al. Regulation of inflammatory responses by gut microbiota and chemoattractant receptor GPR43. Nature. (2009) ;461: (7268):1282–6. Epub 2009/10/30. doi: 10.1038/nature08530. PubMed PMID: 19865172; PubMed Central PMCID: PMC3256734. |
[58] | Structure, function and diversity of the healthy human microbiome. Nature. (2012) ;486: (7402):207–14. Epub 2012/06/16. doi: 10.1038/nature11234. PubMed PMID: 22699609; PubMed Central PMCID: PMC3564958. |
[59] | Tosoni G , Conti M , Heijtz RD . Bacterial peptidoglycans as novel signaling molecules from microbiota to brain. Current opinion in pharmacology. (2019) ;48: :107–13. |
[60] | Wheeler R , Chevalier G , Eberl G , Gomperts Boneca I . The biology of bacterial peptidoglycans and their impact on host immunity and physiology. Cellular Microbiology. (2014) ;16: (7):1014–23. |