Metabolism of sleep and aging: Bridging the gap using metabolomics
Abstract
Sleep is a conserved behavior across the evolutionary timescale. Almost all known animal species demonstrate sleep or sleep like states. Despite extensive study, the mechanistic aspects of sleep need are not very well characterized. Sleep appears to be needed to generate resources that are utilized during the active stage/wakefulness as well as clearance of waste products that accumulate during wakefulness. From a metabolic perspective, this means sleep is crucial for anabolic activities. Decrease in anabolism and build-up of harmful catabolic waste products is also a hallmark of aging processes. Through this lens, sleep and aging processes are remarkably parallel— for example behavioral studies demonstrate an interaction between sleep and aging. Changes in sleep behavior affect neurocognitive phenotypes important in aging such as learning and memory, although the underlying connections are largely unknown. Here we draw inspiration from the similar metabolic effects of sleep and aging and posit that large scale metabolic phenotyping, commonly known as metabolomics, can shed light to interleaving effects of sleep, aging and progression of diseases related to aging. In this review, data from recent sleep and aging literature using metabolomics as principal molecular phenotyping methods is collated and compared. The present data suggests that metabolic effects of aging and sleep also demonstrate similarities, particularly in lipid metabolism and amino acid metabolism. Some of these changes also overlap with metabolomic data available from clinical studies of Alzheimer’s disease. Together, metabolomic technologies show promise in elucidating interleaving effects of sleep, aging and progression of aging disorders at a molecular level.
1Introduction
For the first time in human history, most people of the world are expected to live beyond 60 years [1]. Such an achievement is possible due to low infant mortality in low income countries and declining mortality in old age population from high income countries [2]. The aging population will bring significant challenges in maintaining a healthy population. Aging is a leading risk factor in common diseases including cancer, cardiovascular disorders, metabolic and neurodegenerative diseases [2]. The process of aging is in part the result of progressive decline of physiological function at the molecular level. Key physiological processes impacted by aging include genomic instability, telomere attrition, epigenetic alteration, loss of proteastasis, nutrient sensing dysregulation, mitochondrial dysfunction, cellular senescence, stem cell exhaustion and altered intercellular communication [3].
Along with molecular level changes, behavioral changes are also apparent with healthy aging. Memory impairment [4] and altered sleep wake activities are perhaps the most common signatures of aged individuals [4]. Both human population and rodent model studies have shown that aging individuals are unable to sustain sleep/wake state and generally display lower sleep efficiency [5, 6]. Lack of sleep could be attributed a potential reason behind memory impairment with aging. The role of healthy sleep in memory consolidation and stabilization of formed memories is well documented [7]. Specifically, slow wave sleep (SWS) is thought to contribute to consolidation of acquired memories while rapid eye movement (REM) is important for stabilization of the transformed memories [7]. For example, Mander et al. has demonstrated that lack of SWS in cognitively normal older adults originates from grey matter atrophy of medial prefrontal cortex that results in impaired long-term memory [8]. Moreover, sleep has been shown to be crucial in clearing brain wastes that could otherwise be accumulated and result in typical aging pathologies such as Alzheimer’s disease [9]. Sleep, or lack thereof, has also been shown to be crucial in incidental development of Alzheimer’s disease for at least a subset of patients [10]. It has been postulated that sleep and AD maintains a bidirectional relationship. For example, prolonged wakefulness is thought to increase orexin production and sympathetic output, leading to excessive neuronal activity and decreased glymphatic clearance, respectively. Elevated neuronal activity leads to Aβ production while its clearance decreases, leading to AD pathologies, which, in turn leads to lack of nighttime sleep and excessive daytime sleepiness [11].
Recent studies reveal that metabolic processes are central regulators of aging processes [12] as well as correlated with sleep [13]. Processes that impact metabolic activity such as caloric restriction have been shown to improve aging related parameters such as disease onset and mortality [14]. On the other hand, metabolic nutrient sensing by mTOR (amino acids), Sirtuins (NAD+/NADH) and AMPK (AMP/ADP) have been shown to regulate cellular and organismal processes such as mitochondrial respiration [15], autophagy [16], growth [17] and lifespan [18] that are known to control aging and longevity.
The importance of sleep in factors that control organismal well-being is unquestionable, although the mechanism by which sleep regulates such factors is not clearly understood. Sleep is perhaps the most well preserved activity throughout the evolutionary timescale [19]. From a metabolic perspective, sleep is thought to balance the catabolic activities experienced by organism during wake periods by switching to anabolic functions [20]. In turn, this metabolic role is responsible, at least partly, for the well-recognized reparatory functions of sleep [21]. The corollary hypothesis is that lack of sleep and/or sleep disorder can lead to maladaptive changes due to depleted repair activity leading to metabolic disorders such as hypertension [22], cardiovascular diseases [23], diabetes [24] and obesity [25]. Recent research suggests that changes imparted by loss of sleep may manifest effects that are typical in aging individuals. In humans, insufficient sleep was shown to trigger gene expression related to oxidative stress and immune response [26]. Other molecular processes related to biological aging such as DNA damage response and cellular senescence was also associated to partial sleep deprivation in humans [27]. On the other hand, neurobehavioral studies on younger and older populations have convincingly shown that young individuals are more affected by loss of sleep from perspectives of attention, cognition and learning and memory [28]. Such observation suggests that older individuals are more resistant to effects of sleep loss while younger ones are vulnerable, even demonstrating signatures of “aging-like” characteristics post sleep loss. Combining the molecular and neurobehavioral results, it is tempting to speculate that sleep loss creates a molecular milieu in young individuals that resemble aging phenotypes such as acute cognitive decline and increased response time. To this end, the global scale study of metabolites biomarkers, more commonly known as metabolomics, offers unique opportunities to capture these phenotypic transformations. For example, one can identify the combined effect of sleep and aging at a systems level with pathway level information. Moreover, quantitative metabolomics may lead to unique biomarkers representing aging-like effects of sleep loss. So far, NMR spectroscopy and mass spectrometry, often hyphenated with prior separation technologies, have served as two principal analytical platforms in the metabolomics field and both techniques offer unique advantages and are highly complementary [29]. Table 1 describes the advantages and limitations of different analytical platform mentioned in this review.
The potential of metabolomics analysis has been fairly extensively explored separately in aging studies and sleep studies across translational space. However, metabolomics studies addressing the interaction of these two factors are rare/ nonexistent. In this review, we aim to address the present status of knowledge on aging and sleep from metabolomics analysis and to explore the future potential of present knowledge in understanding the metabolic role of sleep in aging processes.
2Metabolomics of healthy aging
Historically, aging has been studied using model organisms such as C. elegans, Drosophila, and rodents in addition to largely epidemiological human studies. Each species offers its own advantages. For example, investigation of lifespan across generations is easy in invertebrates and in some cases, rodents. However, the association of aging and clinical phenotypes is relevant in humans and rodents/primates with working model of relevant phenotype. Irrespective of the species, metabolomics studies have looked at either healthy aging or age-related disorders such as Alzheimer’s and/or Parkinson’s disease over the past decade. This section will focus on healthy aging with specific disease cases discussed in a separate section that follows.
2.1Lifespan metabolomics studies using invertebrate models
The interplay of nutrient sensing and mitochondrial function have long been known to affect lifespan [12], suggesting a crucial role of metabolism in controlling healthy aging and longevity [12]. Studies on humans and rodents have shown significant alterations of amino acid and fatty acid levels with age [30, 31]. Gao et al. recently demonstrated metabolic changes of life history traits in C. elegans [32] using mass spectrometry. They demonstrated that most FAs peaked during reproductive phase and remained low during larval and aging stages by independently examining amino acid (AA), fatty acid (FA) and phospholipids (PL) from differentially aged worms. Most AAs, on the other hand, were shown to increase during larval stage and early adulthood and decrease throughout the adult phase. Similar observation in a glp-4(bn2) mutant worms led to the conclusion that FA/AA profile changes during life history are independent of germline, and the authors suggest changes in FA profiles across life stages is probably driven by theaak-2 (AMPK) gene. Copes et al. reported somewhat contradictory changes in FA metabolism in older nematodes [33]. Specifically, they observed increased FA content in older animals along with other changes such as altered amino acid pool, decreased purine metabolites, changes in methylation pathway and phospholipid synthesis, increased sorbitol level with age and altered ascorbate metabolism and redox states. These changes were complemented by proteomic investigations. Specifically, changes in key transmethylation metabolite S-Adenosylmethionine (SAM) was corroborated with alteration ofS-Adenosylmethionine synthetase (SAMS-1) protein [33]. The importance of lipid metabolism on nematode lifespan was further posited by Mahanti et al. using comparative NMR based metabolomics [34]. A novel endogenous steroid, DAF-12 was identified by NMR that regulates larval development, fat metabolism and lifespan. Clearly, such changes point towards biological phenomena that are known to accompany human aging including oxidative stress and nutrient imbalance. Changes in fatty acid and lipid metabolism can also correspond to altered mitochondrial activity, another factor that is associated with human and rodent aging [35].
2.2Aging metabolomics studies in rodents
Rodent models of aging have served to characterize the phenotypic changes such as learning and memory along with changes in metabolic physiology. In rodents, significant changes in RBC fatty acid concentration has been reported in 93 week old C57Bl/6J mice compared to 13 weeks old animals [30]. Changes in acylcarnitines along with other intermediates and substrates of fatty acid biosynthesis pathway suggest that lipid metabolism is perhaps highly sensitive towards aging related changes. Effects of lipid metabolism on aging is further affirmed as dietary intervention, such as global caloric restriction, can recover specific lipid metabolites such as lysophosphatidylcholines, sphingomyelins, tetracosahexanoic acid and cholesterol derivatives in 26 months old C57Bl/6J mice compared to ad libitum fed 26 months old animals and 3 months old animals [36]. Moreover, time restricted feeding is also shown to positively affect healthy aging by synchronizing the environmental cues of metabolism with the diurnal cycle, which in turn ameliorates typical aging disorders such as cancer and cardiometabolic disorders and affects longevity [37, 38]. Like caloric restriction, altered exercise programming has also been suggested as an intervention to positively affect healthy aging [39]. To this end, 10 metabolites have been identified in the urine of exercising Wister rats (17 months duration) that were affected by both exercising and aging [40]. Among those, 8 metabolites – arabinose, methylamine, myoinositol, pantothenic acid, threonine, GABA, leucine and creatinine - were shown to be impacted differentially by aging and exercising suggesting profiling urinary metabolome may be a simple and non-invasive way of monitoring effect of intervention protocol forhealthy aging.
While sampling blood and urine offer major advantages such as quantitative biomarkers and insight to general physiology, drawing mechanistic conclusion is often complicated due to the systemic nature of total metabolite patterns observed. One of the advantages of using vertebrate models in metabolomics studies is physiological proximity to humans and availability of tissue samples, specifically those which are highly impacted by aging such as muscle and bone. Cellular mechanisms that are known to be associated with aging such as autophagy and telomere shortening can also be studied using such models. Mitochondrial swelling has been shown to be an important factor associated with loss of bone mass with aging [41]. Clearly, such effect is associated to metabolic impairments that are driven by mitochondrial activity such as glycolytic shift, nucleotide imbalance, and decreased NAD+/NADH ratio. Skeletal and cardiac muscles are also of interest in context of aging that are manifested in decreased muscle strength, increased fatigue, cardiac hypertrophy and ventricle wall thickening as individuals age. Decay in skeletal and cardiac muscle health has been associated with mitochondrial damage due to excessive oxidative stress [42]. Mitochondrial quality control is thus a crucial factor to manage the detrimental effects of aging at the cellular level which is achieved in part by mitochondrial autophagy (Mitophagy) that refers to turnover of damaged mitochondria by autophagy. Autophagy is also crucial for maintaining cardiac and skeletal muscle function [43, 44]. It would therefore be interesting to uncover the metabolic events that are linked to autophagy in those tissues. In stem cells, mitophagy was shown to be crucial in maintaining the mitochondrial rejuvenation thereby maintaining pluripotency. In fact, mitophagy deficiency was shown to alter key bioenergetics pathways such as TCA cycle and glycolysis that are associated with nuclear reprogramming [45]. Zhou et al. demonstrated that autophagy decreases with aging in both muscle and heart although mitophagy decreases only in muscle. The metabolic events associated to aging in heart and muscle also seems different. Specifically, skeletal muscle shows signature of insulin resistance (BCAA and ceramide accumulation) and fatty acid fuel inflexibility while heart showed decreased fatty acid β-oxidation [46] demonstrating the fact that cardiac and skeletal muscle may need differential therapeutic intervention to prevent aging related effects.
2.3Aging metabolomics studies in humans and non-human primates
Studying aging in human population is difficult because humans are the longest living primates. Cross sectional studies from large population cohorts can be used to identify age related metabolic features after correcting for other covariates such as BMI. Such approach was adopted by Yu et al. using two population cohorts (KORA F4, Germany and TwinsUK, UK). They found several lipid species to be significantly associated with age in both cohorts among women participants [47]. The lipid species included four acylcarnitines, five phosphatidylcholines (1 C-linked and 4 O-linked) and two sphingomyelins (Supplementary information S1). These observations suggest aging specific processes such as incomplete mitochondrial fatty acid oxidation lead to measurable imprints in circulation. In addition, histidine and tryptophan were also found to be significantly changing with age [47]. The same cohort samples were used to demonstrate metabolic association of leucocyte telomere length (LTL), a key cellular phenotype of aging [48]. Specifically, 2 lysolipids, 1-stearoylglycerophosphpinositol and1-palmitoylglycerophosphoinositol were found to be negatively associated to LTL along with two γ-glutamylamino acids (Supplementary information S1) [48]. Altered lipid metabolism and its association with LTL is also evident from American Indian population, with 19 metabolites enriched in lipids (glycerophosphoethanolamines, glycerophosphocholines, glycerolipids, bile acids, isoprenoids, fatty amides, or L-carnitine ester, (Supplementary information S1), were significantly associated with LTL [49].
Global lipid metabolic alterations were also evident from studies conducted to discriminate centenarians and elderly individuals [50, 51]. Such studies are important in deciphering keys to healthy aging. Two phospholipids (LPC 22:6 and PC 38:6), along with ten small molecules (cotidine, histidine, lysine, threonine, aconitate, 3-hydroxybutyrate, isocitrate, malate, taurocholate, uridine) were proposed to be metabolites associated with longevity defined by attaining 80 years of age (Supplementary information S1) [52]. Stricter definition of longevity, for example, ability to attain 100 years of age, demonstrated regulatory role of lipid metabolic pathways. Specifically - five triacylglycerols, ten sphingomyelins, two phosphatdylinositols, five phosphatidylethanolamines, nine plasmalogens, five phosphatidylcholines, one lysophosphatidylcholine, two diacylglycerols and one ceramide species were found to be altered in centenarians compared to an elderly population (Supplementary information S1) [51]. Furthermore, NMR metabolomics found 6 other metabolites including phenylalanine and glutamine to be significantly different across centenarians and elderly individuals (Supplementary information S1) [51]. Another study in a similar setting demonstrated three plasmalogens (oPC 38:6, o-PC 34:1, o-PC 32:1) are replicated (Supplementary information S1), suggesting regulation of key oxidative pathways may be key to healthy aging [50]. Along with that, mechanism of longevity of centenarians may be regulated by a cellular detoxification mechanism regulated by an arachidonic acid metabolic cascade [51]. In another cross-sectional analysis Chaleckis et al. showed 14 age related metabolites in whole blood using LC-MS, 6 of which are RBC enriched [53]. This suggests RBCs play a crucial role in the aging process and deserves further investigation in this context. The 14 metabolites included 1,5-anhydroglucitol, dimethyl-guanosine, acetyl-carnosine, carnosine, ophthalmic acid, UDP-N-acetyl-glucosamine, N-acetyl-arginine, N6-acetyl-lysine, pantothenate, citrulline, leucine, isoleucine, NAD+ and NADP+. Of note, this study employed a HILIC based LC-MS technique and thus could not analyze many lipid metabolites. Nevertheless, their study points to typical aging phenotypes such as activity decline, changes in energy metabolism, cellular maintenance and oxidative status. (Supplementary information S1) compiles relevant biomarkers from recent plasma/serum based aging metabolomics studies.
While cross-sectional studies can provide useful information, true aging biomarker studies need extensive longitudinal analysis. However, longitudinal studies are limited and difficult to span across lifetime, specifically for primates. This issue is somewhat addressed using small primate models such as common marmosets [54]. Hoffman et al. performed a longitudinal analysis of plasma metabolome from common marmosets and demonstrated specific metabolic pathways to be associated to aging that were not found in cross sectional analysis [55]. Interestingly, tryptophan and tyrosine metabolism were found among the most significant pathways. Of note, the kynurenine pathway, the major breakdown pathway of tryptophan, has been implicated in several aging related processes and disorders [56]. Overall, aging is a conglomeration of degenerative cellular processes that includes changes in mitochondrial quality and activity along with altered nutrient sensing, thereby creating a metabolic environment that leads to changes in oxidative status. To this end, specific lipid classes such as circulatory acylcarnitines and plasmalogens have been found to be enriched in response to aging (supplementary information S1). Similar enrichment in phosphatidylcholines and sphingolipids possibly suggest altered cellular membrane composition and changes in cell differentiation [57]. Other classes of enriched lipids, such as diacylglycerol, are known to be important for signaling processes [58] which are significantly affected in aging individuals [59]. Specifically, three plasmalogens, two phosphatidylcholines, tryptophan and histidine were replicated across at least two aging studies (supplementary information S1). The plasmalogens probably manifests altered oxidative status while phosphatidylcholines are indicative of membrane dysregulation. Tryptophan metabolic pathways impact different physiological processes. For example, tryptophan controls central nervous system neurotransmission via the serotonin (serotonin/melatonin) pathway. Tryptophan is also known to show immunomodulatory behavior via kynurenine pathway by producing kynurenine, an endogenous ligand of aryl hydrocarbon receptors that suppresses antitumour immune response [60]. Overall, metabolomics studies of aging indicate modulation in oxidative status, membrane dysregulation, neurotransmission and immune response. It is possible that a fraction of such changes also impact aging memory, most probably in a bidirectional manner. Some of these features overlap with metabolic changes observed in Alzheimer’s disease and dementia in general (supplementary information S1, Fig. 1). However, any direct relationship, to the best of our knowledge, is yet to be established. On the other hand, metabolomics technologies have established that similar pathways are impacted by sleep as discussed in the next section, thereby raising the question of a common route of metabolic mediation between sleep and aging.
3Metabolomic investigation of sleep and crossover with aging studies
The connection between metabolism and sleep has become increasingly established [61]. For example, sleep promoting small molecules, such as adenosine and melatonin have been extensively studied [62–66]. Recent advances in high throughput metabolomics technologies have resulted in deeper understanding of the link between sleep and metabolism [61]. Although sleep is conserved across evolution and several animal models for sleep study have been developed, metabolomics technologies investigating global metabolic alterations in response to sleep perturbation has been primarily explored in human clinical populations. Metabolomics research in human sleep field can be divided into two major categories – experimental sleep intervention (sleep deprivation/restriction) of healthy volunteers [67, 68] as well as chronic sleep disorders such as insomnia and obstructive sleep apnea [69, 70]. The bulk of metabolomics work has been performed on the former group and will be discussed first.
3.1Metabolomics studies of experimental sleep deprivation
Chronic sleep disruption of healthy individuals has been associated with typical aging-like signatures across a range of neuropsychiatric measures such as cognitive decline, impaired performance and attention and memory [71]. Interestingly, younger populations are more vulnerable towards sleep loss in the above contexts compared to older populations. A group of young women (20–30 years) were shown to be more vulnerable to 4 hours of sleep loss for 3 nights compared to older women (55–65 years) when tested by psychomotor vigilance test [72]. In a remarkable parallel, rodent studies have shown that sleep deprivation induced memory loss affects young animals more than the older animals [28]. Greater susceptibility of younger individuals that reflect in worsened neurobehavioral parameters post sleep loss might stem from the fact that young age group needs more sleep compared to older individuals. However, such possibility appears somewhat improbable since older individuals usually sleep much less and more fragmented [73]. Therefore, the apparent invulnerability of the elderly people might be due to the fact that they are already adapted to less sleep and perhaps achieve a physiological homeostasis in response to the sleep loss due to aging.
Although no study, to best of our knowledge, has looked into the overlapping metabolic effects of sleep and aging, it is tempting to speculate that metabolic biochemistry is in play behind the mutual relation of sleep and aging. Recent studies have established a significant metabolic effect of sleep curtailment with a majority of those effects involves changes in lipid metabolism. Four hours of sleep restriction (SR) for five days in adult healthy volunteers showed significant alteration in plasma lipidome compared to the baseline level of same individuals [67]. Lysophosphatidylcholine (LPC), triglyceride (TG), phosphatidylcholine (PC) and acylcarnitines are among the perturbed lipid species. Specifically, the phospholipids were increased post SR while the TGs are decreased. Long chain acylcarnitines were also decreased post SR and not recovered after a single night of recovery sleep. Among the small molecules, tryptophan and phenylalanine were elevated post SR and recovered after recovery sleep. In a more acute SR paradigm, Bell et al. explored plasma metabolic changes in healthy volunteers with familial diabetic history [74]. The volunteers were allowed 5.5 h sleep opportunity on the SR night and 8 h sleep opportunity on the baseline night. Plasma metabolomics analysis on fasting samples collected post SR/baseline sleep night demonstrated significant changes in lipid metabolism. Specifically, medium chain fatty acid (caproate), carnitine (2-decenoylcarnitine), lysolipid (2-myristoylglycerophosphocholine), sterol (cholesterol, β-sitosterol, 7-α-hydroxy-3-oxo-4-cholestenoate) and bile acid (deoxycholate, glycocholenate sulfate) metabolism were found to be affected. Plasma levels of most of the lipid metabolites were elevated except β-sitosterol. This work also reported alterations in amino acid and carbohydrate metabolism. Interestingly, they identified significant changes in tryptophan metabolism in terms of elevated serotonin and C-glycosyltryptophan [74]. We hypothesize that such profound changes in the lipid metabolism is arising in part from energy metabolism pathways [67]. Alteration in mitochondrial activity may also play a key role in such changes. Specifically, mitochondrial fatty acid oxidation was indicated to be impacted by acute sleep restriction in both healthy and diabetic individuals as evident from elevation in three specific acylcarnitines – C14:1, C18:1, C18:2 carnitines [75] In humans, connection of lipid metabolism and chronic sleep restriction was illustrated by Aho et al. using two population cohorts [76]. The ABCG1 pathway, a sterol metabolism regulating pathway [77] was found to be impacted in two separate population cohorts obtained from Finland [76]. Sleep insufficiency was assessed using individual questionnaire from the DILGOM cohort (N = 472) and the YFS cohort (N = 2221) that revealed similar fractions of individuals with subjective sleep insufficiency (18.2% and 16.2%, respectively). Serum metabolomics using NMR spectroscopy showed depletion of HDL particles across two populations manifesting detrimental effect of chronic sleep restriction on cardiometabolic health [76].
The studies discussed to this point were performed using a sleep restriction design, where some amount of sleep opportunity is allowed. While, this design reflects the real life situation, a landmark comprehensive metabolomics study in the sleep field was done using a total sleep deprivation (SD) design [68] where the individuals were deprived of a single night’s sleep. Mass spectrometric investigation on these subjects demonstrated changes in 41 lipids and other metabolites. Among the lipids, nine acylcarnitines were significantly elevated post SD, which suggests altered mitochondrial activity post-acute SD. Among others, four lysophosphatidylcholines, four sphingomyelins and 20 phosphatidylcholines were significantly elevated post total SD possibly due to changes in mitochondrial activity and membrane degeneration.. Additionally, the small molecules, tryptophan, serotonin and taurine were found to be impacted suggesting perturbation of tryptophan metabolic pathways [68]. Urine metabolome of the same subjects from Davies et al. study corroborated with the results from plasma metabolome [78]. Specifically, elevation in 3-indoxylsulfate, a tryptophan metabolite and carnitine post SD suggest prominent effect of total SD on tryptophan metabolism and lipid metabolism. Along with this, 13 other metabolites were found to be altered including elevated taurine, formate, citrate, 3-hydroxyisobutyrate, TMAO, acetate and decreased dimethylamine, 4-deoxythreonic acid, creatinine, ascorbate, 2-hydroxyisobutyrate, allantoin, 4-deoxyerythronic acid and 4-hydroxyphenylacetate [78].
It is tempting to speculate that some of the metabolic changes imparted by sleep loss are correlated to adverse phenotypic effects of sleep loss via metabolic function; however this is difficult to test in human subjects. There is support from model systems however. Lipid metabolism was shown to be closely linked to the cognitive decline of mice after chronic sleep interruption along with purine, amino acid and retinoid metabolism [79]. In order to understand the mechanistic association of altered fatty acid metabolism and sleep, Zhan et al. undertook a rat model study using 1H NMR spectroscopy and GC-MS. Male Sprague-Dawley rats were sleep restricted by platform in water model. Analysis of blood serum demonstrated that reduction in serum fatty acid is associated to sleep loss induced islet dysfunction [80]. Such alteration in fatty acid levels could be restored by dietary intervention [80]. However, such model could also impart stress and the results observed may in part be due to the stress. Comprehensive metabolomics analysis of rats sleep restricted for one (acute) and five (chronic) days showed somewhat different signature compared to baseline sleep [67]. Altered lipid metabolism was demonstrated as the major component of metabolic change. Specifically, sphingomyelins and phospholipids were elevated after acute SR along with several small molecules. Chronic SR seems to affect the lipidome even more by sphingomyelins, phospholipids and ceramides. Recovery sleep opportunity normalizes levels of sphingomyelins but could not recover plasmalogens. Interestingly, this study discovered to specific markers of sleep debt – namely, oxalic acid and diacylglycerol 36:3 – that were decreased in both humans and rats, suggesting the translational relevance of the results [67]. Such significant changes in lipid levels appears to be arising from changes in hepatic lipogenesis as suggested by metabolomics and gene expression analysis in same experimental regime [81]. Nicotinamide metabolism and transmethylation pathways were also perturbed in post sleep restricted liver [81].
Sleep curtailment clearly affects the global metabolic physiology of organisms. We posit that sleep restriction/deprivation leads to a metabolic setting that could resemble aging and may serve as lead to understand the connection of sleep loss and incident neurodegenerative disorders. Comparison of some recent aging metabolomics studies [47–52] and sleep metabolomics studies [61] reveals a striking signature of metabolic similarity. We have analyzed recent aging metabolomics literatures (Fig. 1) and compared biomarkers reported with replicated sleep biomarkers that we have previously analyzed [61]. Five metabolites were replicated across multiple sleep studies and at least one aging study (Supplementary information S1). These metabolites are – carnitine C18:1, tryptophan, PC 36:6, PC 38:4 and PC 32:1. Tryptophan was also replicated across multiple aging studies (supplementary information S1). Together, these metabolites indicate changes in mitochondrial fatty acid oxidation, tryptophan metabolism and cellular membrane integrity. Some of these processes, as we will discuss in later section, are crucial in development of neurodegenerativedisorders.
Fig. 1
Overlap of metabolic features across plasma/serum human metabolomics studies from the field of aging, sleep and AD. Detailed data is presented in supplementary information S1. A: Overlap of sleep, aging and AD biomarkers. Metabolites that are replicated across multiple sleep, aging and AD studies were compared and the overlaps among them were analyzed. Tryptophan was the only metabolite found to be unique across the sets. B. Overlap of aging and AD effects of sleep loss: Metabolites that are found to be replicated across multiple sleep and at least one aging/AD studies were compared. Tryptophan and PC 36:6 were found to be overlapping between these sets.
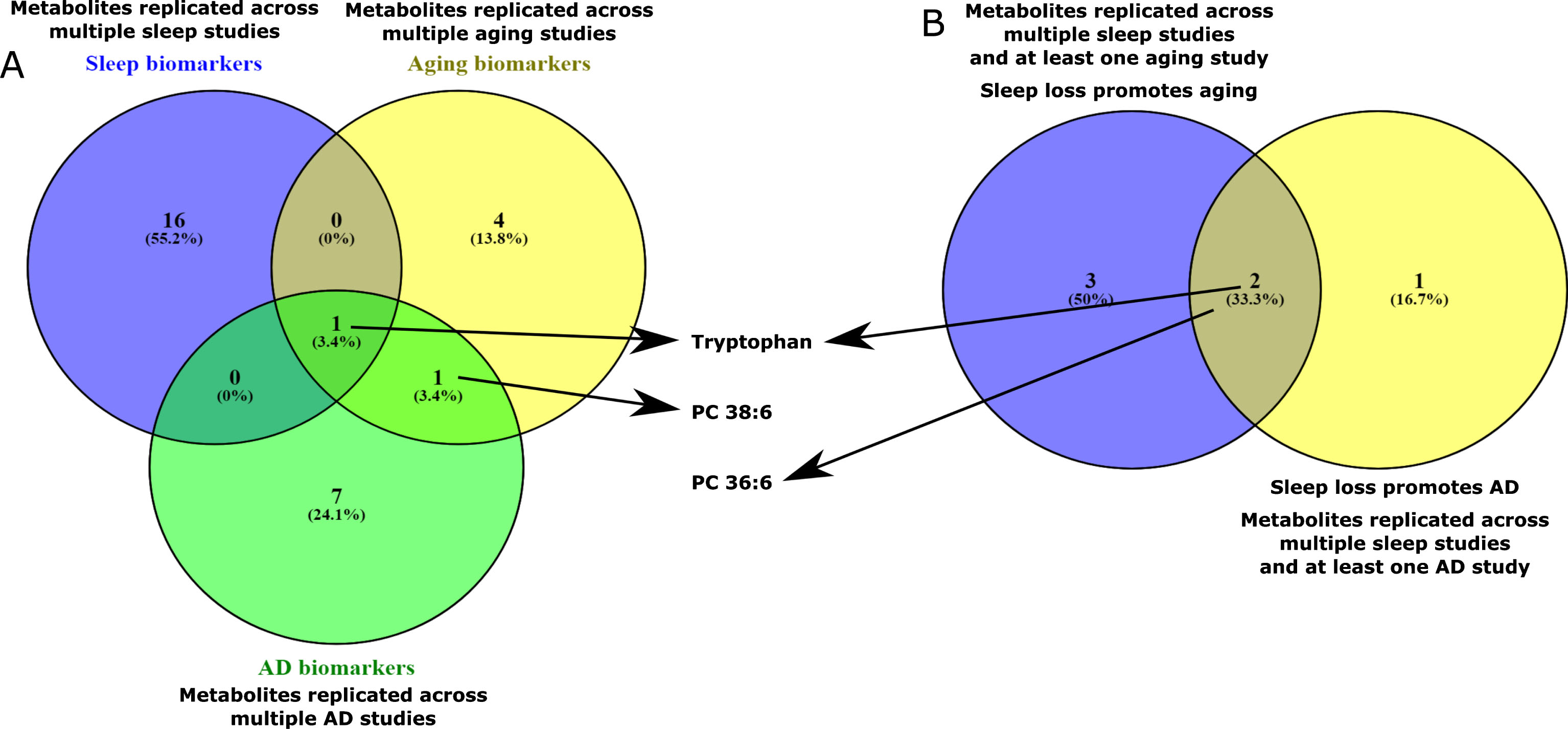
3.2Metabolomic studies of sleep disorders
With regards to work on chronic sleep disorders, the picture remains less clear. Obstructive sleep apnea has been studied to some extent using metabolomics technologies. Clear evidences of perturbation of lipid metabolism are apparent [82]. OSA is known to be associated to cardiovascular morbidity and mortality [83]. Animal model studies point towards both lipid metabolic dysregulation and dyslipidemia to be potentially important for accelerated atherogenesis in OSA [84, 85]. In humans, OSA seem to elevate both fasting [86] and post-prandial lipid levels [87]. Interestingly, post-prandial hypertriglyceridemia has also been linked to elevated cardiovascular morbidity and mortality, suggesting altered lipidomic equilibrium is crucial for OSA related co-morbidities [88, 89]. Xu et al. reviewed the studies that employed mass spectrometry based metabolomics in clinical population to identify biomarkers of OSA [90]. At least 10 different studies employed mass spectrometry based techniques to identify plasma biomarkers of OSA. One of the issues that mask the discovery of a consistent biomarker panel is the variability in analytical platform. However, lipids or related metabolites still occupy a significant fraction of reported biomarkers. For example, Ferrarini et al. identified various classes of differentially expressed phospholipids (PC, PS, LPC, LPC, LPA, PE) across severe and non-severe OSA patients [91] while Engeli et al. discovered that circulating endocannabinoids strongly correlate with the blood pressure in OSA patients [92]. More recently, alteration in lipid metabolism is further evident by comprehensive metabolomics analysis of OSA patients. For example, eight metabolites were found to be differentially expressed in the urine of moderate and severe OSA patients (N = 18 and 16, respectively) compared to controls (N = 14). The metabolites included three acylcarnitines, two glycerophospholipids and two sphingomyelins [93]. Additional analysis suggested acylcarnitine C14:1, sphingomyelin 18:1 and symmetric dimethylarginine may serve as putative biomarkers for OSA. In a different study, UPLC-MS based metabolomics of urine was employed to distinguish between OSA (N = 60), simple snorers (N = 30) and healthy controls (N = 30) [69]. 24 metabolites were found to be consistently higher or lower in simple snorers and OSA patients compared to controls. Among them, combination of six metabolites (4-hydroxypentenoic acid, arabinose, glycochenodeoxycholate-3-sulfate, isoleucine, serine, and xanthine) were found to produce moderate diagnostic score between OSA and non-OSA individuals. 4-hydroxypentenoic acid, 5-dihydrotestosterone sulfate, serine, spermine, and xanthine were combined to distinguish OSA from simple snorers with a sensitivity of 85% and specificity of 80%.
Overall, recent development of metabolomics studies in the sleep field clearly suggests alterations in specific metabolic pathways that imply concerted changes. It is worth emphasizing that cross comparison of these studies is challenging due to differences in study populations, and technologies used for metabolite profiling. Nevertheless, a common pattern seems to arise in terms of changes in lipid and tryptophan metabolic pathways. These two metabolic pathways are also implicated in several aging metabolomics studies. Therefore, we expect to observe further investigations focusing on these pathways in pathologies relating to sleep and aging.
4Closing the loop: Metabolomics of Alzheimer’s disease (AD)
Neurodegenerative disorders such as Alzheimer’s disease (AD), Huntington’s disease (HD) and Parkinson’s disease (PD) are related to aggressive death of brain neurons [94, 95]. Interestingly, sleep disturbance is a common problem and one of the earliest symptoms in all the above disorders [96]. Aging is also a risk factor for such diseases [97] while HD is a genetically inherited disorder [94]. Recent studies have indicated that sleep curtailment is a prominent risk factor for amyloid β overload and incident neurodegenerative pathologies [9] thereby strengthening the notion that changes in rest-activity and sleep-wake cycle resulting from underlying environmental and biological factors increases the risk of AD [98–100]. Sleep disorders may also precede PD pathologies [101]. Metabolic changes are reported in all three neurodegenerative disorders by analyzing comprehensive metabolomics datasets [102–104]. However, this review will be focused on AD since a bidirectional sleep-AD pathology relationship is emerging from literature as discussed previously.
Definitive identification and treatment of AD remains elusive primarily because lack of definitive biomarkers [104]. Therefore, identification of biomarkers of different stages of AD is of paramount importance. For example, preclinical AD, mild cognitive impairment (MCI) due to AD and dementia related to AD are defined as the stages of the disease which need to be categorized for molecular disease prognosis/diagnosis. Development of metabolomics technologies led to extensive research of AD biomarkers at different stages of the disease from different parts of the world [104–112]. Of note, cerebrospinal fluid metabolomics analysis comprises a significant fraction of AD metabolomics literature [113–115]. However, in order to compare with sleep and aging metabolomics markers, the current discussion is limited to serum/plasma based metabolomics studies from humans, in spite of metabolomics studies on invertebrate [116] and rodents [117] models of AD.
Recently, Gonzalez-Dominiguez et al. reported three successive studies on identifying AD biomarkers in Spanish population [109–112] using three different analytical techniques for each study (GC-MS, FIA-APPI-MS, DI-MS). Therefore, overlapping biomarkers can be considered analytically validated. In the DI-MS study comprising of 22 AD patients and 18 age matched controls, the AD biomarkers from fasting blood serum were enriched by phosphatidylcholines and diacylglycerols– signatures of cellular membrane breakdown [110]. Increase of membrane rigidity is long been recognized as a characteristic feature of aging [118] which may result from loss of fluid unsaturated side chains of membrane lipids. Interestingly, 50% of the PC s that are differentially expressed in AD patients were found to have two unsaturated side chains while the other 50% had one unsaturated side chain [111] (Supplementary information S1) suggesting loss of membrane fluidity with aging may also be associated with AD. Of note, REM sleep deprivation was found to decrease membrane fluidity in rat brain [119]. Lipid membrane alterations were also evident from changes in sphingomyelin metabolism [109]. In total, 8 metabolites were found to be replicated across two of these three studies. These metabolites were DG 36:3, DG 34:3, DG 36:4, oleamide, oleic acid, taurine, histidine and urea [109–112].
Interestingly, DG 36:3 was found to be a cross-species biomarker in previous sleep restriction study in humans and rats in our previous work [67]. Diacylglycerols (DG) are known to have crucial role in neuronal signaling. DGs are precursor molecules for synthesis of endocannabinoids such as 2-archidonylglycerol (2-AG) via the action of DG lipases [120]. 2-AG is a key molecule for endocannabinoid mediated retrograde synaptic submission [120]. Imbalance in endocannabinoid signaling is known to hamper the postsynaptic transmission in AD patients [121]. DGs are also known to regulate phosphatidylcholine synthesis during membrane formation by saturating cholinephosphotransferase activity. Therefore, replicated observation of specific DGs in AD biomarker studies may suggest a concerted change in membrane structure and neuronal signaling [122]. Such replication also suggests that a specific type of lipid metabolism plays a crucial role in the development and progression of aging and related pathologies. This notion is supported in studies from other population as well. For example, at least 4 different studies in Chinese population reported alteration in serum lipids [106, 107, 123, 124]. Three metabolites were found to be replicated in at least two studies – lysoPC 18:0, lysoPC 18:2 and dihydrosphingosine (Supplementary information S1). Plasma lysoPC 18:0 was found to decrease in two studies [106, 123] which has been recently shown to increase cellular membrane rigidity in vivo [125] suggesting alteration in membrane integrity by means of changes in membrane lipid composition plays a critical role in development of AD. The role of membrane lipids in AD is also manifested by upregulation of sphingomyelin in a study based in the United States using MS based shotgun lipidomics [126]. Phospholipids are enriched within the set of lipids that are reported as AD biomarkers thus far and thus validation of these targets is worthwhile. Mapstone et al. performed a lipidomic analysis using a panel of 10 lipids that successfully predicted the phenoconversion to mild cognitive impairment or AD with over 90% accuracy [104]. 8 of those 10 lipids were phospholipids – lysoPC 18:2, PC 36:6, PC 38:0, PC 38:6, PC 40:1, PC 40:2, PC 40:6 and o-PC 40:6 (Supplementary information S1) [104]. The other two being propionylcarnitine and hexadecenoylcarnitine (Supplementary information S1). Interestingly, serum levels of other phospholipid species such as ethanolamine plasmalogens were also shown to reliably predict translation into AD [127]. Among the literature reviewed above, 8 metabolites were found to be replicated across at least two AD metabolomics studies – LPC 18:2, PC 38:6, DG 34:3, DG 36:4, palmitic acid, oleic acid, oleamide and glutamine (supplementary information S1). At a broader level, 6 circulatory lipid classes were found to be replicated and enriched among AD patients (Supplementary information S1). Among them, PCs, sphingolipids and DGs were also enriched in aging studies. This suggests that some lipidomic signatures of aging may be associated to incidences of AD (supplementary information S1). More interestingly, we identified tryptophan, PC 36:6 and taurine to be replicated across sleep and AD studies (supplementary information S1, Fig. 1). PC 36:6 may be of particular interest as a functional marker of sleep impact on AD since it was reported to be significantly elevated in at least two sleep deprivation/restriction studies [67, 68] and also elevated in individuals after phenoconversion to MCI/AD [104].
5Sleep loss creates a metabolic environment resembling aging and related disorders
Review of the aging, sleep and AD metabolomics literature brings out several commonalities in terms of perturbed metabolites as detailed in supplementary information S1. Several metabolites were replicated across aging metabolomics studies (Supplementary information S1, Fig. 1). Out of the biomarker panels of those studies, six metabolites were found to be replicated across at least two studies. These metabolites are – histidine, tryptophan, PC 38:6, o-PC 32:1, o-PC 34:1, o-PC 38:6 (Fig. 1, supplementary information S1). Such replication across independent studies clearly suggests that specific metabolic cascades are impacted by aging which probably include tryptophan metabolism and phospholipid metabolism. Enrichment of this metabolite set by phospholipids and ether lipids perhaps suggest changes in cellular membrane structure and oxidative status with age. The three plasmalogen species mentioned above were also found consistently in two studies investigating markers of healthy aging by metabolic profiling of centenarians and elederly individuals [50, 51]. This replication reaffirms the notion that maintenance of a healthy oxidative status could be the key to healthy aging. On the other hand, distinct signature of changes in tryptophan may be related to dysregulation in immune system via the kynurenine pathway [128] and neurotransmission impacting the serotonin-melatonin pathway and competition with the tyrosine dependent neurotransmitter production [129]. A similar, but not identical, set of metabolites were found to be replicated across multiple sleep studies and at least one aging study (Supplementary information S1, Fig. 1). This set includes a long chain acylcarnitine, three phosphatidylcholines and tryptophan and may reveal the aging phenotype imparted by sleep loss.
On a broad spectrum, overlapping metabolic changes impacted by sleep curtailment seems to affect cellular membrane dysregulation, tryptophan metabolism and altered mitochondrial regulation (Fig. 2). Aging leads to altered nutrient sensing and utilization leading to oxidative stress, altered mitochondrial activity, membrane disintegration and dysregulated immune system [3, 12]. Some of these effects are manifested by sleep curtailment as well. For example, sleep is thought to impact immune system by modulating redistribution of T cells and enhancing cytokine activity [130]. Role of sleep in reducing oxidative stress is also well studied [131]. From a metabolic perspective, the overlapping effects are exemplified by specific metabolites including phosphatidylcholine species and long chain carnitines (supplementary information S1). Altered mitochondrial activities could also affect tryptophan metabolism, specifically the kynurenine pathway [132]. Tryptophan metabolism is also known to be crucial for controlling sleep wake activity via the melatonin pathway [133]. On the other hand, role of tryptophan metabolism via kynurenine pathway is known to impact immune activity [128]. Taken together, metabolic changes impacted by sleep and aging has remarkable overlaps that probably represents some of the key biological processes affected by both, including oxidative stress, immune response and membrane integrity (Fig. 2).
Fig. 2
Interplay of metabolic changes manifested by aging, sleep loss and AD in connection to altered physiological processes. Aging and sleep loss both induces oxidative stress and disrupts immune function. In addition, aging also impacts membrane integrity. Such events are also related to AD pathologies. These changes in physiological processes are also exemplified by specific overlapping peripheral metabolic changes, in particular, changes in tryptophan metabolism and phosphatidylcholine level.
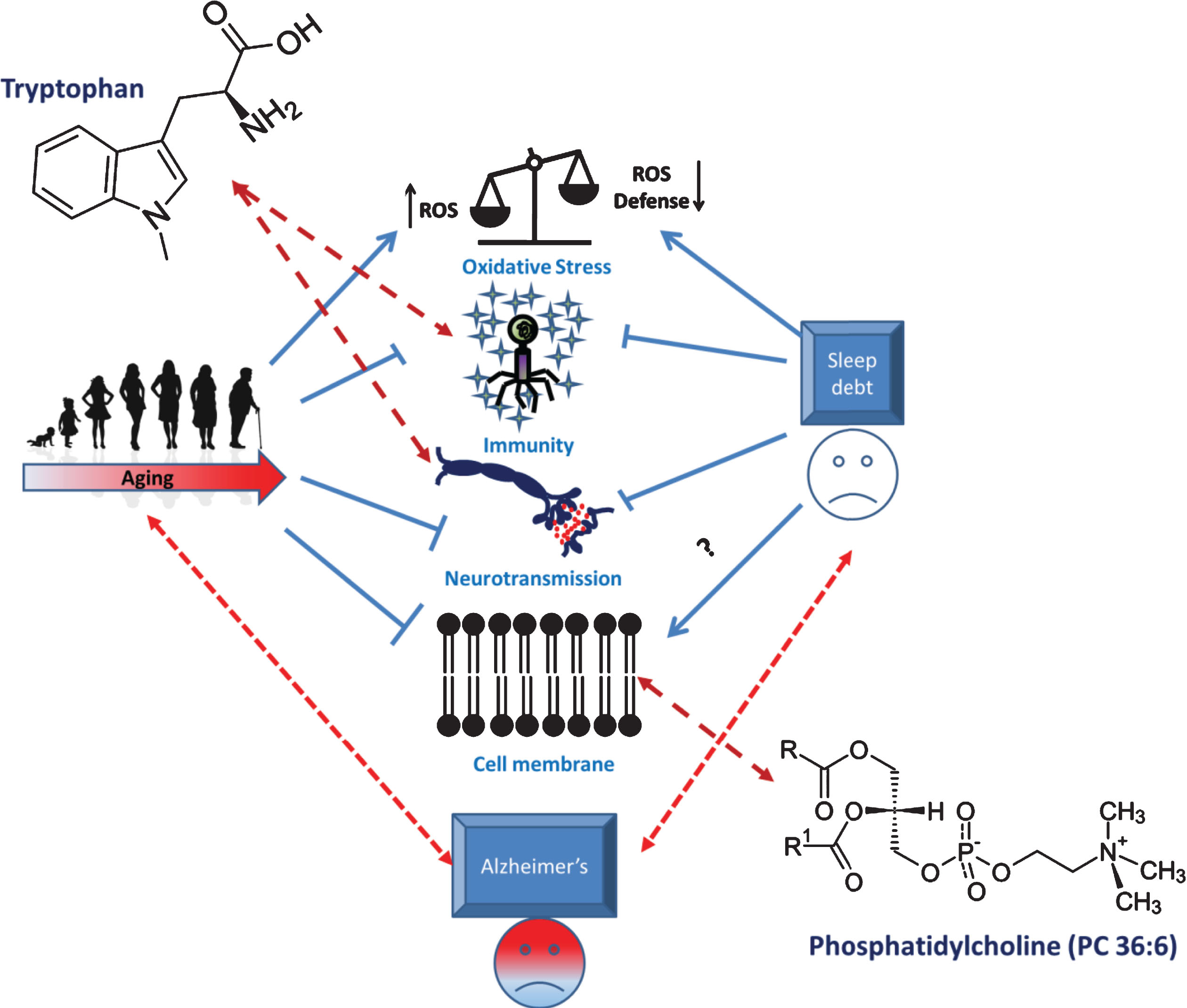
Similar analysis across AD metabolomics literature demonstrated existence of 8 metabolites that are replicated across multiple blood metabolomics studies from AD patients (Supplementary information S1). These metabolites are – LPC 18:2, palmitic acid, oleic acid, glutamine, PC 38:6, DG 34:3, DG 36:4 and oleamide (Supplementary information S1). This metabolite set presents clear indication of alterations in fatty acid processing, cellular membrane changes and alteration in cellular signaling. Tryptophan, PC 36:6 and taurine were replicated across multiple sleep studies and at least one AD study. Such overlap suggests some of the metabolic changes imparted by sleep loss may have implications in AD. Among them, tryptophan metabolism and cellular membrane dysregulation remain of interest. Activation of innate immune response is also viewed as a crucial disease promoting factor [134] which may be metabolically represented by changes in tryptophan levels [135]. To this end, quinolinic acid, a neurotoxin generated by kynurenine pathway was shown to be a critical factor for neuronal damage in AD [136]. On the other hand, AD, as discussed earlier, is also thought to be mediated in part by altered membrane composition manifested by changes in phosphatidylcholine species overlap between sleep loss and AD metabolic features (Supplementary information S1).
Remarkably, we note that the metabolic changes imparted by sleep loss that resembles AD and/or aging metabolic feature seems to have commonalities. At molecular level, this is exemplified by tryptophan and PC 36:6, two metabolites that are common between sleep, AD and aging studies (supplementary information S1, Fig. 1). We hypothesize that sleep loss affect metabolism in a multifarious fashion. Some fraction of such effects may contribute to aging like phenotypes, which in turn could lead to AD pathologies (Fig. 2). Overall, we posit that aging and sleep loss lead to similarly altered biological pathways including oxidative stress (acylcarnitine metabolism), immune systems, neurotransmission (tryptophan metabolism) and membrane integrity (phosphatidylcholine metabolism). Such changes lead to specific metabolic perturbation in the periphery. Moreover, some of these pathways are also related to development and progression of neurodegenerative disorders such as AD (Fig. 2). Therefore, overlapping metabolomic signature of sleep, AD and aging offer a unique opportunity to unravel the connection between them. Moreover, analysis of such overlap would also help addressing the question from a therapeutic point of view. For example, lifestyle intervention such as regular sleep schedule may be used to healthy aging and omission (or delaying) of symptoms related to neurodegenerative disorders [137]. However, we acknowledge that the crossovers of metabolomics signature of sleep, aging and AD are still limited. Such limitations may be attributed to diversity in analytical approaches to metabolomics studies in general as discussed in the followingsection.
6Limitations of metabolomics approaches in existing literature
While some overlapping features between sleep, AD and aging metabolomics features could be identified, we note that there are several technical challenges to such meta-analysis. Aging is associated to phenotypical changes including cognition and memory. To the best of our knowledge, little or no study has been conducted in the field to associate metabolomics profile to such changes. We hypothesize that a fraction of metabolic changes due to aging is associated to the decline in memory. This hypothesis could be tested in a population of elderly individuals using a longitudinal study design. Short term mechanistic studies could also be conducted in animal models. Such knowledge holds immense potential in understanding and treating age related memory decline.
Perhaps the most important reason is the different analytical techniques used across studies. In the field of sleep metabolomics, such issues are previously discussed [138]. In fact, variation in analytical platforms remain one of the major bottlenecks in the metabolomics field for validation of results across studies, partly due to different strengths and weaknesses of each platform (Table 1). Across the six aging metabolomics studies reviewed in supplementary information S1, five different analytical platforms were used. Similarly, six different analytical platforms were used across eight AD metabolomics studies (supplementary information S1). Accordingly, the metabolites identified by these studies are of varying nature including small polar molecules to large polar and non-polar lipids. Moreover, in the field of lipidomics, extent of lipid chain length information across studies remains variable. For example, in the AD metabolomics literature reported here, two studies reported individual chain length and unsaturation of PC and DG while other two reported gross lipid chain length and unsaturation. Since sleep, aging and AD are potentially closely knit at the metabolic level as discussed in this review, investigation of these fields will become more meaningful as minimum standards are maintained across studies in terms of analytical platforms and coverage of metabolites. Hopefully, such uniformity will be achieved as the field of metabolomics matures.
7Conclusion
Sleep and aging are two basic processes associated to organismal life. Physiological impacts of sleep curtailment and aging share a striking resemblance in several processes including oxidative stress and deregulation of nutrient sensing. At the metabolic level, these changes are reflected by alteration in metabolites and lipids. We reviewed the present literature and concluded that lipid and aromatic amino acid metabolism, particularly impacting phospholipids and tryptophan metabolism are two pathways commonly perturbed in both aging and sleep curtailment. Although, global metabolomics studies of sleep are still at infancy and there exists no study that follow aging and sleep jointly, we believe that lack of sleep could potentially create a metabolic milieu that may resemble aging specifically in young population. Such studies are warranted since some of the metabolic alteration associated to both aging and sleep may hold key to the progression of neurodegenerative disorders such as Alzheimer’s disease. For example, phospholipid metabolism seems to be crucial for the progression, pathophysiology and prediction of AD. Therefore, both global and targeted studies are needed to characterize the overlapping features of sleep and aging along with their potential role in development of disorders typical to aged population. Recent initiatives within the metabolomics community towards standardization of experiments will also be useful in cross-comparison across the two fields.
Conflict of interest
The authors have no conflict of interest to report.
Acknowledgments and Funding
This work is supported by NIH/NIA grant no. R21-AG-052905.
Supplementary material
[1] The supplementary material is available in the electronic version of this article: https://dx.doi.org/10.3233/NHA-180043.
References
[1] | World Health Organization. World Report on Ageing and Health [Internet]. (2015) [cited 2017 Jul 14]. Available from: http://apps.who.int/iris/bitstream/10665/186463/1/9789240694811_eng.pdf?ua=1 |
[2] | Inouye SK , Studenski S , Tinetti ME , Kuchel GA . Geriatric syndromes: Clinical, research, and policy implications of a core geriatric concept. J Am Geriatr Soc [Internet]. NIH Public Access; 2007 May [cited 2017 Jul 14];55: (5):780–91. Available from: http://www.ncbi.nlm.nih.gov/pubmed/17493201 |
[3] | López-Otín C , Galluzzi L , Freije JMP , Madeo F , Kroemer G . Metabolic Control of Longevity. Cell. (2016) ;166: :802–21. |
[4] | Dorffner G , Vitr M , Anderer P . The Effects of Aging on Sleep Architecture in Healthy Subjects, In Springer, Cham; 2015 [cited 2017 Jul 14]. 93–100. Available from: http://link.springer.com/10.1007/978-3-319-08939-3_13 |
[5] | Wimmer ME , Rising J , Galante RJ , Wyner A , Pack AI , Abel T . Aging in Mice Reduces the Ability to Sustain Sleep/Wake States, Norris CM , editor. One [Internet]. Public Library of Science; (2013) Dec 16 [cited 2017 Jul 14];8: (12):e81880. Available from: http://dx.plos.org/10.1371/journal.pone.0081880 |
[6] | Zdanys KF , Steffens DC . Sleep Disturbances in the Elderly. Psychiatr Clin North Am [Internet]. (2015) Dec [cited 2017 Jul 14];38: (4):723–41. Available from: http://linkinghub.elsevier.com/retrieve/pii/S0193953X15000854 |
[7] | Rasch B , Born J . About sleep’s role in memory. Physiol Rev [Internet]. American Physiological Society; (2013) Apr [cited 2017 Jul 14];93: (2):681–766. Available from: http://www.ncbi.nlm.nih.gov/pubmed/23589831 |
[8] | Mander BA , Rao V , Lu B , Saletin JM , Lindquist JR , Ancoli-Israel S , et al, Prefrontal atrophy, disrupted NREM slow waves and impaired hippocampal-dependent memory in aging. Nat Publ Gr [Internet]. 2013 [cited 2017 Jul 14];16: (3). Available from: https://www.nature.com/neuro/journal/v16/n3/pdf/nn.3324.pdf |
[9] | Xie L , Kang H , Xu Q , Chen MJ , Liao Y , Thiyagarajan M , et al, Sleep Drives Metabolite Clearance from the Adult Brain. Science (80-) [Internet]. 2013 [cited 2017 Jul 20]; 342: (6156), Available from: http://science.sciencemag.org/content/342/6156/373 |
[10] | Lim MM , Elkind J , Xiong G , Galante R , Zhu J , Zhang L , et al, Dietary therapy mitigates persistent wake deficits caused by mild traumatic brain injury. Sci Transl Med [Internet]. (2013) Dec 11 [cited 2016 Dec 8];5: (215):215ra173.Available from: http://stm.sciencemag.org/cgi/doi/10.1126/scitranslmed.3007092 |
[11] | Musiek ES , Holtzman DM . Mechanisms linking circadian clocks, sleep, and neurodegeneration. Science (80-) [Internet]. (2016) ;354: (6315):1004–8. Available from: http://www.sciencemag.org/lookup/doi/10.1126/science.aah4968 |
[12] | López-Otín C , Blasco MA , Partridge L , Serrano M , Kroemer G . The Hallmarks of Aging. Cell [Internet]. (2013) Jun [cited 2017 Jul 14];153: (6):1194–217. Available from: http://linkinghub.elsevier.com/retrieve/pii/S0092867413006454 |
[13] | Huang W , Ramsey KM , Marcheva B , Bass J . Circadian rhythms, sleep, and metabolism. Journal of Clinical Investigation. (2011) ;121: :2133–41. |
[14] | Finkel T . The Metabolic Regulation of Aging. Nat Med [Internet]. (2015) [cited 2017 Jul 20];21: (12):1416–23. Available from: https://www.nature.com/nm/journal/v21/n12/pdf/nm.3998.pdf |
[15] | Dominy JE , Puigserver P . Mitochondrial biogenesis through activation of nuclear signaling proteins. Cold Spring Harb Perspect Biol. (2013) ;5: (7). |
[16] | Salminen A , Kaarniranta K . AMP-activated protein kinase (AMPK) controls the aging process via an integrated signaling network. Ageing Research Reviews. (2012) ;11: :230–41. |
[17] | Mihaylova MM , Shaw RJ . The AMPK signalling pathway coordinates cell growth, autophagy and metabolism. Nat Cell Biol [Internet]. (2011) 13: (9): 1016–23. Available from: http://www.nature.com/doifinder/10.1038/ncb2329 |
[18] | Kanfi Y , Naiman S , Amir G , Peshti V , Zinman G , Nahum L , et al, The sirtuin SIRT6 regulates lifespan in male mice, 2012 [cited 2017 Jul 20]; Available from: https://www.nature.com/nature/journal/v483/n7388/pdf/nature10815.pdf |
[19] | Karmanova IG , Lazarev SG . Stages of sleep evolution (facts and hypotheses). Waking Sleep. (1979) ;3: (2):137–47. |
[20] | Datta S . Cellular and chemical neuroscience of mammalian sleeSleep Med [Internet]. NIH Public Access; 2010 May [cited 2017 Jul 20];11: (5):431–40. Available from: http://www.ncbi.nlm.nih.gov/pubmed/20359944 |
[21] | Bellesi M , Bushey D , Chini M , Tononi G , Cirelli C . Contribution of sleep to the repair of neuronal DNA doublestrand breaks: evidence from flies and mice. (2016) [cited 2017 Jul 20]; Available from: https://www.nature.com/articles/srep36804.pdf |
[22] | Calhoun DA , Harding SM . Sleep and hypertension. Chest [Internet]. American College of Chest Physicians; 2010 Aug [cited 2017 Jul 20];138: (2): 434–43. Available from: http://www.ncbi.nlm.nih.gov/pubmed/20682533 |
[23] | Nagai M , Hoshide S , Kario K . Sleep duration as a risk factor for cardiovascular disease- a review of the recent literature. Curr Cardiol Rev [Internet]. Bentham Science Publishers; 2010 Feb [cited 2017 Jul 20];6: (1):54–61. Available from: http://www.ncbi.nlm.nih.gov/pubmed/21286279 |
[24] | Cappuccio FP , D’Elia L , Strazzullo P , Miller MA . Quantity and Quality of Sleep and Incidence of Type 2 Diabetes: A systematic review and meta-analysis. Diabetes Care [Internet]. 2010 Feb 1 [cited 2017 Jul 20];33: (2): 414–20. Available from: http://www.ncbi.nlm.nih.gov/pubmed/19910503 |
[25] | Romero-Corral A , Caples SM , Lopez-Jimenez F , Somers VK . Interactions between obesity and obstructive sleep apnea: Implications for treatment. Chest [Internet]. Amer ican College of Chest Physicians; (2010) Mar [cited 2017 Jul 20];137: (3):711–9. Available from: http://www.ncbi.nlm.nih.gov/pubmed/20202954 |
[26] | Möller-Levet CS , Archer SN , Bucca G , Laing EE , Slak A , Kabiljo R , et al, Effects of insufficient sleep on circadian rhythmicity and expression amplitude of the human blood transcriptome. Proc Natl Acad Sci U S A [Internet]. National Academy of Sciences; (2013) Mar 19 [cited 2017 Jul 24];110: (12):E1132–41. Available from: http://www.ncbi.nlm.nih.gov/pubmed/23440187 |
[27] | Carroll JE , Cole SW , Seeman TE , Breen EC , Witarama T , Arevalo JMG , et al, Partial sleep deprivation activates the DNA damage response (DDR) and the senescence-associated secretory phenotype (SASP) in aged adult humans. Brain Behav Immun [Internet]. 2016 Jan [cited 2017 Jul 24];51: :223–9. Available from: http://linkinghub.elsevier.com/retrieve/pii/S0889159115300088 |
[28] | Vecsey CG , Park AJ , Khatib N , Abel T . Effects of sleep deprivation and aging on long-term and remote memory in mice. Learn & Mem (Cold Spring Harb NY) [Internet]. Cold Spring Harbor Laboratory Press; (2015) ;22: (4):197–202. Available from: http://www.ncbi.nlm.nih.gov/pubmed/25776037 |
[29] | Emwas AHM . The strengths and weaknesses of NMR spectroscopy and mass spectrometry with particular focus on metabolomics research. Methods Mol Biol. (2015) ;1277: :161–93. |
[30] | Houtkooper RH , Argmann C , Houten SM , Canto C , Jeninga EH , Andreux PA , et al. The metabolic footprint of aging in mice. Sci Rep [Internet]. Nature Publishing Group; (2011) Oct 31 [cited 2016 Nov 2];1: :9–14. Available from: http://www.nature.com/articles/srep00134 |
[31] | Lawton KA , Berger A , Mitchell M , Milgram KE , Evans AM , Guo L , et al. Analysis of the adult human plasma metabolome. Pharmacogenomics [Internet]. Future Medicine Ltd London, UK; (2008) Apr 2 [cited 2017 Jul 25];9: (4):383–97. Available from: http://www.futuremedicine.com/doi/10.2217/14622416.9.4.383 |
[32] | Gao AW , Chatzispyrou IA , Kamble R , Liu YJ , Herzog K , Smith RL , et al. A sensitive mass spectrometry platform identifies metabolic changes of life history traits in C. elegans. Sci Rep [Internet]. (2017) ;7: (1):2408. Available from: http://www.nature.com/articles/s41598-017-02539-w |
[33] | Copes N , Edwards C , Chaput D , Saifee M , Barjuca I , Nelson D , et al. Metabolome and proteome changes with aging in Caenorhabditis elegans. Exp Gerontol. (2015) ;72: :67–84. |
[34] | Mahanti P , Bose N , Bethke A , Judkins JC , Wollam J , Dumas KJ , et al. Comparative metabolomics reveals endogenous ligands of DAF-12, a nuclear hormone receptor, regulating C. elegans development and lifespan. Cell Metab. (2014) ;19: (1):73–83. |
[35] | Bratic A , Larsson NG . The role of mitochondria in aging. Journal of Clinical Investigation. (2013) ;123: :951–7. |
[36] | De Guzman JM , Ku G , Fahey R , Youm YH , Kass I , Ingram DK , et al. Chronic caloric restriction partially protects against age-related alteration in serum metabolome. Age (Omaha). (2013) ;35: (4):1091–104. |
[37] | Melkani GC , Panda S . Time-restricted feeding for prevention and treatment of cardiometabolic disorders. Journal of Physiology. (2017) ;595: :3691–700. |
[38] | Manoogian EN , Panda S . Circadian rhythms, time-restricted feeding, and healthy aging. Ageing Res Rev. (2017) ;39: :59–67. |
[39] | Garatachea N , Pareja-Galeano H , Sanchis-Gomar F , Santos-Lozano A , Fiuza-Luces C , Moran M , et al. Exercise attenuates the major hallmarks of aging. Rejuvenation Res [Internet]. Mary Ann Liebert, Inc.; (2015) ;18: (1):57–89. Available from: http://www.ncbi.nlm.nih.gov/pubmed/25431878 |
[40] | Deda O , Gika HG , Taitzoglou I , Raikos N , Theodoridis G . Impact of exercise and aging on rat urine and blood metabolome. An LC-MS based metabolomics longitudinal study. Metabolites. (2017) ;7: (1). |
[41] | Shum LC , White NS , Nadtochiy SM , De Mesy Bentley KL , Brookes PS , HJonason J , et al. Cyclophilin D Knockout mice show enhanced resistance to osteoporosis and to metabolic changes observed in aging bone. PLoS One. (2016) ;11: (5):e0155709. |
[42] | Payne BAI , Chinnery PF . Mitochondrial dysfunction in aging:Much progress but many unresolved questions. Biochim Biophys Acta - Bioenerg. (2015) ;1847: (11):1347–53. |
[43] | Taneike M , Yamaguchi O , Nakai A , Hikoso S , Takeda T , Mizote I , et al. Inhibition of autophagy in the heart induces age-related cardiomyopathy. Autophagy [Internet]. (2010) Jul [cited 2017 Jul 28];6: (5):600–6. Available from: http://www.ncbi.nlm.nih.gov/pubmed/20431347 |
[44] | Masiero E , Agatea L , Mammucari C , Blaauw B , Loro E , Komatsu M , et al. Autophagy Is Required to Maintain Muscle Mass. Cell Metab [Internet]. (2009) Dec [cited 2017 Jul 28];10: (6):507–15. Available from: http://www.ncbi.nlm.nih.gov/pubmed/19945408 |
[45] | Vazquez-Martin A , Van den Haute C , Cufi S , Corominas Faja B , Cuyas E , Lopez-Bonet E , et al. Mitophagy-driven mitochondrial rejuvenation regulates stem cell fate. Aging (Albany NY). (2016) ;8: (7):1330–52. |
[46] | Zhou J , Chong SY , Lim A , Singh BK , Sinha RA , Salmon AB , et al. Changes in macroautophagy, chaperone-mediated autophagy, and mitochondrial metabolism in murine skeletal and cardiac muscle during aging. Aging (Albany NY). (2017) ;9: (2):583–99. |
[47] | Yu Z , Zhai G , Singmann P , He Y , Xu T , Prehn C , et al. Human serum metabolic profiles are age dependent. Aging Cell. (2012) ;11: (6):960–7. |
[48] | Zierer J , Kastenmiiller G , Suhre K , Gieger C , Codd V , Tsai PC , et al. Metabolomics profiling reveals novel markers for leukocyte telomere length. Aging (Albany NY). (2016) ;8: (1):77–94. |
[49] | Zhao J , Zhu Y , Uppal K , Tran VT , Yu T , Lin J , et al. Metabolic profiles of biological aging in American Indians:The strong heart family study. Aging (Albany NY) [Internet]. (2014) Mar 20 [cited 2017 Aug 13];6: (3):176–86. Available from: http://www.ncbi.nlm.nih.gov/pubmed/24799415 |
[50] | Collino S , Montoliu I , Martin F-PJ , Scherer M , Mari D , Salvioli S , et al. Metabolic Signatures of Extreme Longevity in Northern Italian Centenarians Reveal a Complex Remodeling of Lipids, Amino Acids, and Gut Microbiota Metabolism. Wertheimer A , editor. PLoS One [Internet]. Public Library of Science; (2013) Mar 6 [cited 2017 Aug 13];8: (3):e56564. Available from: http://dx.plos.org/10.1371/journal.pone.0056564 |
[51] | Montoliu I , Scherer M , Beguelin F , DaSilva L , Mari D , Salvioli S , et al. Serum profiling of healthy aging identifies phospho- and sphingolipid species as markers of human longevity. Aging (Albany NY) [Internet]. Impact Journals, LLC; (2014) ;6: (1):9–25. Available from: http://www.ncbi.nlm.nih.gov/pubmed/24457528 |
[52] | Cheng S , Larson MG , McCabe EL , Murabito JM , Rhee EP , Ho JE , et al. Distinct metabolomic signatures are associated with longevity in humans. Nat Commun [Internet]. NIH Public Access; (2015) Apr 13 [cited 2017 Aug 13];6: :6791. Available from: http://www.ncbi.nlm.nih.gov/pubmed/25864806 |
[53] | Chaleckis R , Murakami I , Takada J , Kondoh H , Yanagida M . Individual variability in human blood metabolites identifies age-related differences. Proc Natl Acad Sci U S A [Internet]. (2016) ;113: (16):4252–9. Available from: http://www.pnas.org/cgi/content/long/113/16/4252 |
[54] | Fischer KE , Austad SN . The development of small primate models for aging research. ILAR J [Internet]. (2011) [cited 2017 Aug 4];52: (1):78–88. Available from: http://www.ncbi.nlm.nih.gov/pubmed/21411860 |
[55] | Hoffman JM , Tran V , Wachtman LM , Green CL , Jones DP , Promislow DEL . A longitudinal analysis of the effects of age on the blood plasma metabolome in the common marmoset, Callithrix jacchus. Exp Gerontol. (2016) ;76: :17–24. |
[56] | Ramirez Ortega D , Gonzalez Esquivel D , Pineda B , Rios C , Perez de la Cruz V . Role of Kynurenine Pathway in Aging. In:Targeting the Broadly Pathogenic Kynurenine Pathway [Internet]. Cham:Springer International Publishing; (2015) [cited 2017 Aug 4]. p. 63–74. Available from: http://link.springer.com/10.1007/978-3-319-11870-3_5 |
[57] | Pralhada Rao R , Vaidyanathan N , Rengasamy M , Mammen Oommen A , Somaiya N , Jagannath MR . Sphingolipid Metabolic Pathway:An Overview of Major Roles Played in Human Diseases. J Lipids [Internet]. (2013) ;2013: :1–12. Available from: http://www.hindawi.com/journals/jl/2013/178910/ |
[58] | Almena M , Meirida I . Shaping up the membrane:Diacylglycerol coordinates spatial orientation ofsignaling. Trends in Biochemical Sciences. (2011) ;36: :593–603. |
[59] | Riera CE , Merkwirth C , De Magalhaes Filho CD , Dillin A . Signaling Networks Determining Life Span. Annu Rev Biochem [Internet]. (2016) ;85: (1):35–64. Available from: http://www.annualreviews.org/doi/10.1146/annurev-biochem-060815-014451 |
[60] | Opitz CA , Litzenburger UM , Sahm F , Ott M , Tritschler I , Trump S , et al. An endogenous tumour-promoting ligand of the human aryl hydrocarbon receptor. Nature. (2011) ;478: (7368):197–203. |
[61] | Rhoades SD , Sengupta A , Weljie AM . Time is ripe:Maturation of metabolomics in chronobiology. Curr Opin Biotechnol. (2017) ;43: :70–6. |
[62] | Jones BE . Glia, Adenosine, and Sleep. Neuron. (2009) ;61: :156–7. |
[63] | Dawson D , Encel N . Melatonin and sleep in humans. Journal of Pineal Research. (1993) ;15: :1–12. |
[64] | Stenberg D , Porkka-Heiskanen T . Adenosine and sleep-wake regulation. In: Neurochemistry of Sleep and Wakefulness. (2008) . pp. 337–62. |
[65] | Porkka-Heiskanen T . Adenosine in sleep and wakefulness. Annalsof Medicine. (1999) ;31: :125–9. |
[66] | Gandhi AV , Mosser EA , Oikonomou G , Prober DA . Melatonin Is required for the circadian regulation of sleep. Neuron. (2015) ;85: (6):1193–9. |
[67] | Weljie AM , Meerlo P , Goel N , Sengupta A , Kayser MS , Abel T , et al. Oxalic acid and diacylglycerol 36:3 are cross-species markers of sleep debt. Proc Natl Acad Sci U S A [Internet]. (2015) Feb 24 [cited 2016 Jun 6];112: (8):2569–74. Available from: http://www.ncbi.nlm.nih.gov/pubmed/25675494 |
[68] | Davies SK , Ang JE , Revell VL , Holmes B , Mann A , Robertson FP , et al. Effect of sleep deprivation on the human metabolome. Proc Natl Acad Sci U S A [Internet]. (2014) Jul 22 [cited 2016 Jun 6];111: (29):10761–6. Available from: http://www.ncbi.nlm.nih.gov/pubmed/25002497 |
[69] | Xu H , Zheng X , Qian Y , Guan J , Yi H , Zou J , et al. Metabolomics Profiling for Obstructive Sleep Apnea and Simple Snorers. Sci Rep [Internet]. Nature Publishing Group; (2016) Aug 2 [cited 2017 Aug 9];6: :30958. Available from: http://www.ncbi.nlm.nih.gov/pubmed/27480913 |
[70] | Spiegelhalder K , Regen W , Nissen C , Feige B , Baglioni C , Riemann D , et al. Magnetic resonance spectroscopy in patients with insomnia:A repeated measurement study. PLoS One. (2016) ;11: (6). |
[71] | Scullin MK , Bliwise DL . Sleep, cognition, and normal aging:Integrating a half century of multidisciplinary research. Perspect Psychol Sci [Internet]. (2015) ;10: (1):97–137. Available from: http://www.ncbi.nlm.nih.gov/pubmed/25620997 |
[72] | Stenuit P , Kerkhofs M . Age modulates the effects of sleep restriction in women. Sleep [Internet]. (2005) ;28: (10):1283–8. Available from: http://eutils.ncbi.nlm.nih.gov/entrez/eutils/elink.fcgi?dbfrom=pubmed%7B&%7Did=16295213%7B&%7Dretmode=ref%7B&%7Dcmd=prlinks%7B%25%7D5Cnpapers3://publication/uuid/B4E8B14E-EE30-4C21-93A0-7F776A693757 |
[73] | Crowley K . Sleep and sleep disorders in older adults. Neuropsychology Review. (2011) ;21: :41–53. |
[74] | Bell LN , Kilkus JM , Booth JN , Bromley LE , Imperial JG , Penev PD . Effects of sleep restriction on the human plasma metabolome. Physiol Behav [Internet]. (2013) Oct 2 [cited 2016 Jun 6];122: :25–31. Available from: http://www.ncbi.nlm.nih.gov/pubmed/23954406 |
[75] | Van Den Berg R , Mook-Kanamori DO , Donga E , Van Dijk M , Van Dijk JG , Lammers GJ , et al. A single night of sleep curtailment increases plasma acylcarnitines:Novel insights in the relationship between sleep and insulin resistance. Arch Biochem Biophys. Elsevier Inc. (2016) ;589: :145–51. |
[76] | Aho V , Ollila HM , Kronholm E , Bondia-Pons I , Soininen P , Kangas AJ , et al. Prolonged sleep restriction induces changes in pathways involved in cholesterol metabolism and inflammatory responses. Sci Rep [Internet]. (2016) ;6: (1):24828. Available from: http://www.nature.com/articles/srep24828 |
[77] | Tarling EJ , Edwards PA . ATP binding cassette transporter G1 (ABCG1) is an intracellular sterol transporter. Proc Natl Acad Sci U S A [Internet]. National Academy of Sciences; (2011) Dec 6 [cited 2017 Aug 7];108: (49):19719–24. Available from: http://www.ncbi.nlm.nih.gov/pubmed/22095132 |
[78] | Giskepdegard GF , Davies SK , Revell VL , Keun H , Skene DJ . Diurnal rhythms in the human urine metabolome during sleep and total sleep deprivation. Sci Rep. Nature Publishing Group. (2015) ;5: :14843. |
[79] | Feng L , Wu H , Song G , Lu C , Li Y , Qu L , et al. Chronical sleep interruption-induced cognitive decline assessed by a metabolomics method. Behav Brain Res [Internet]. (2016) Apr [cited 2017 Aug 4];302: :60–8. Available from: http://linkinghub.elsevier.com/retrieve/pii/S016643281530348X |
[80] | Zhan S , Wu Y , Sun P , Lin H , Zhu Y , Han X . Decrease in Circulating Fatty Acids Is Associated with Islet Dysfunction in Chronically Sleep-Restricted Rats. Int J Mol Sci [Internet]. (2016) Dec 14 [cited 2017 Aug 4];17: (12):2102. Available from: http://www.ncbi.nlm.nih.gov/pubmed/27983645 |
[81] | Sengupta A , Rhoades SD , Kim EJ , Nayak S , Grant GR , Meerlo P , et al. Sleep restriction induced energy, methylation and lipogenesis metabolic switches in rat liver. Int J Biochem Cell Biol. (2017) ;93: :129–35. |
[82] | Drager LF , Polotsky VY , Lorenzi-Filho G . Obstructive sleep apnea:An emerging risk factor for atherosclerosis. Chest. (2011) ;140: :534–42. |
[83] | Marin JM , Carrizo SJ , Vicente E , Agusti AGN . Longterm cardiovascular outcomes in men with obstructive sleep apnoea-hypopnoea with or without treatment with continuous positive airway pressure: An observational study. Lancet. (2005) ;365: (9464):1046–53. |
[84] | Savransky V , Nanayakkara A , Li J , Bevans S , Smith PL , Rodriguez A , et al. Chronic intermittent hypoxia induces atherosclerosis. Am J Respir Crit Care Med. (2007) ;175: (12):1290–7. |
[85] | Savransky V , Jun J , Li J , Nanayakkara A , Fonti S , Moser AB , et al. Dyslipidemia and atherosclerosis induced by chronic intermittent hypoxia are attenuated by deficiency of stearoyl coenzyme a desaturase. Circ Res. (2008) ;103: (10):1173–80. |
[86] | Keenan BT , Maislin G , Sunwoo BY , Arnardottir ES , Jackson N , Olafsson I , et al. Obstructive sleep apnoeatreatment and fasting lipids:A comparative effectiveness study. Eur Respir J. (2014) ;44: (2):405–14. |
[87] | Phillips CL , Yee BJ , Marshall NS , Liu PY , Sullivan DR , Grunstein RR . Continuous positive airway pressure reduces postprandial lipidemia in obstructive sleep apnea:A randomized, placebo-controlled crossover trial. Am J Respir Crit Care Med. (2011) ;184: (3):355–61. |
[88] | Bansal S , Buring JE , Rifai N , Mora S , Sacks FM , Ridker PM . Fasting Compared With Nonfasting Triglycerides and Risk of Cardiovascular Events in Women. JAMA [Internet]. (2015) ;298: (3):309–16. Available from: http://jama.jamanetwork.com/article.aspx?articleid=208018 |
[89] | Eberly LE , Stamler J , Neaton JD . Relation of triglyceride levels, fasting and nonfasting, to fatal and nonfatal coronary heart disease. Arch Intern Med. (2003) ;163: (9):1077–83. |
[90] | Xu H , Zheng X , Jia W , Yin S . Chromatography/Mass Spectrometry-Based Biomarkers in the Field of Obstructive Sleep Apnea. Med. (2015) ;94: (40):e1541. |
[91] | Ferrarini A , Ruperez FJ , Erazo M , Martinez MP , Villar Q , Alvarez F , Peces-Barba G , et al. Fingerprinting-based metabolomic approach with LC-MS to sleep apnea and hypopnea syndrome:A pilot study. Electrophoresis. (2013) ;34: (19):2873–81. |
[92] | Engeli S , Bluher M , Jumpertz R , Wiesner T , Wirtz H , Bosse-Henck A , et al. Circulating anandamide and blood pressure in patients with obstructive sleep apnea. J Hypertens. (2012) ;30: (12):2345–51. |
[93] | Cho K , Yoon DW , Lee M , So D , Hong I-H , Rhee CS , et al. Urinary Metabolomic Signatures in Obstructive Sleep Apnea through Targeted Metabolomic Analysis:A Pilot Study. Metabolomics [Internet]. Springer US; (2017) Aug 19 [cited 2017 Aug 9];13(8: ):88. Available from: http://link.springer.com/10.1007/s11306-017-1216-9 |
[94] | Schapira AHV , Olanow CW , Greenamyre JT , Bezard E . Slowing of neurodegeneration in Parkinson’s disease and Huntington’s disease:Future therapeutic perspectives. The Lancet. (2014) ;384: :545–55. |
[95] | Enche Ady CNA , Lim SM , Teh LK , Salleh MZ , Chin A-V , Tan MP , et al. Metabolomic-guided discovery of Alzheimer’s disease biomarkers from body fluid. J Neurosci Res [Internet]. (2017) Mar; Available from: http://doi.wiley.com/10.1002/jnr.24048 |
[96] | Gagnon J-F , Petit D , Latreille V , Montplaisir J . Neurobiology of sleep disturbances in neurodegenerative disorders. Curr Pharm Des. (2008) ;14: (32):3430–45. |
[97] | Niccoli T , Partridge L . Ageing as a risk factor for disease. Current Biology. (2012) ;22. |
[98] | Lobo A , López-Antón R , De-la-Cámara C , Quintanilla MA , Campayo A , Saz P . Non-cognitive psychopathological symptoms associated with incident mild cognitive impairment and dementia, Alzheimer’s type. Neurotox Res. (2008) ;14(2-3):263–72. |
[99] | Benito-Leon J , Bermejo-Pareja F , Vega S , Louis ED . Total daily sleep duration and the risk of dementia:A prospective population-based study. Eur J Neurol. (2009) ;16: (9):990–7. |
[100] | Foley D , Monjan A , Masaki K , Ross W , Havlik R , White L , et al. Daytime sleepiness is associated with 3-year incident dementia and cognitive decline in older Japanese-American men. J Am Geriatr Soc. (2001) ;49: (12):1628–32. |
[101] | Claassen DO , Josephs KA , Ahlskog JE , Silber MH , Tippmann-Peikert M , Boeve BF . REM sleep behavior disorder preceding other aspects of synucleinopathies by up to half a century. Neurology. (2010) ;75: (6):494–9. |
[102] | Cheng M-L , Chang K-H , Wu Y-R , Chen C-M . Metabolic disturbances in plasma as biomarkers for Huntington’s disease. J Nutr Biochem [Internet]. (2016) ;31: :38–44. Available from: http://www.sciencedirect.com/science/article/pii/S0955286315003629 |
[103] | Amara AW , Standaert DG . Metabolomics and the Search for Parkinson Biomarkers. Mov Disord. (2013) ;28: (12):1620–1. |
[104] | Mapstone M , Cheema AK , Fiandaca MS , Zhong X , Mhyre TR , MacArthur LH , et al. Plasma phospholipids identify antecedent memory impairment in older adults. Nat Med [Internet]. (2014) Mar 9 [cited 2017 Aug 14];20: (4):415–8. Available from: http://www.ncbi.nlm.nih.gov/pubmed/24608097 |
[105] | Han X , Rozen S , Boyle SH , Hellegers C , Cheng H , Burke JR , et al. Metabolomics in Early Alzheimer’s Disease:Identification of Altered Plasma Sphingolipidome Using Shotgun Lipidomics. Wang Y , editor. PLoS One [Internet]. (2011) Jul 11 [cited 2017 Aug 14];6(7):e21643. Available from: http://www.ncbi.nlm.nih.gov/pubmed/21779331 |
[106] | Li N , Liu W , Li W , Li S , Chen X , Bi K , et al. Plasma metabolic profiling of Alzheimer’s disease by liquid chromatography/mass spectrometry. Clin Biochem [Internet]. (2010) Aug [cited 2017 Aug 14];43: (12):992–7. Available from: http://linkinghub.elsevier.com/retrieve/pii/S0009912010002055 |
[107] | Wang G , Zhou Y , Huang F-J , Tang H-D , Xu X-H , Liu JJ , et al. Plasma Metabolite Profiles of Alzheimer’s Disease and Mild Cognitive Impairment. J Proteome Res [Internet]. (2014) May 2 [cited 2017 Aug 18];13: (5):2649–58. Available from: http://www.ncbi.nlm.nih.gov/pubmed/24694177 |
[108] | Armirotti A , Basit A , Realini N , Caltagirone C , Bossu P , Spalletta G , et al. Sample preparation and orthogonal chromatography for broad polarity range plasma metabolomics:Application to human subjects with neurodegenerative dementia. Anal Biochem [Internet]. (2014) Jun 15 [cited 2017 Aug 14];455: :48–54. Available from: http://www.ncbi.nlm.nih.gov/pubmed/24708938 |
[109] | Gonzalez-Domínguez R , García-Barrera T , Gomez-Ariza JL . Metabolite profiling for the identification of altered metabolic pathways in Alzheimer’s disease. J Pharm Biomed Anal. (2015) ;107: :75–81. |
[110] | Gonzalez-Domínguez R , García-Barrera T , Gomez-Ariza JL . Metabolomic study of lipids in serum for biomarker discovery in Alzheimer’s disease using direct infusion mass spectrometry. J Pharm Biomed Anal. (2014) ;98: :321–6. |
[111] | González-Domínguez R , García-Barrera T , Gómez-Ariza JL . Using direct infusion mass spectrometry for serum metabolomics in Alzheimer’s disease. Anal Bioanal Chem. (2014) ;406: (28):7137–48. |
[112] | Gonzalez-Domínguez R , García-Barrera T , Gomez-Ariza JL . Application of a novel metabolomic approach based on atmospheric pressure photoionization mass spectrometry using flow injection analysis for the study of Alzheimer’s disease. Talanta [Internet]. (2015) Jan [cited 2017 Aug 14];131: :480–9. Available from: http://linkinghub.elsevier.com/retrieve/pii/S0039914014006432 |
[113] | Ibanez C , Simo C , Barupal DK , Fiehn O , Kivipelto M , Cedazo-Minguez A , et al. A new metabolomic workflow for early detection of Alzheimer’s disease. J Chromatogr A [Internet]. (2013) Aug 9 [cited 2017 Aug 14];1302: :65–71. Available from: http://www.ncbi.nlm.nih.gov/pubmed/23827464 |
[114] | Ibañez C , Simo C , Martin-Alvarez PJ , Kivipelto M , Winblad B , Cedazo-Minguez A , et al. Toward a Predictive Model of Alzheimer’s Disease Progression Using Capillary Electrophoresis-Mass Spectrometry Metabolomics. Anal Chem [Internet]. (2012) Oct 16 [cited 2017 Aug 14];84: (20):8532–40. Available from: http://www.ncbi.nlm.nih.gov/pubmed/22967182 |
[115] | Kaddurah-Daouk R , Zhu H , Sharma S , Bogdanov M , Rozen SG , Matson W , et al. Alterations in metabolic pathways and networks in Alzheimer’s disease. Transl Psychiatry [Internet]. (2013) Apr [cited 2017 Aug 14];3(4: ):e244. Available from: http://www.nature.com/doifinder/10.1038/tp.2013.18 |
[116] | Van Assche R , Temmerman L , Dias DA , Boughton B , Boonen K , Braeckman BP , et al. Metabolic profiling of a transgenic Caenorhabditis elegans Alzheimer model. Metabolomics. (2015) ;11: (2):477–86. |
[117] | Johnson LA , Torres ERS , Impey S , Stevens JF , Raber J . Apolipoprotein E4 and Insulin Resistance Interact to Impair Cognition and Alter the Epigenome and Metabolome. Sci Rep [Internet]. (2017) ;7:43701. Available from: http://www.nature.com/articles/srep43701 |
[118] | Zs-Nagy I . A membrane hypothesis of aging. J Theor Biol [Internet]. (1978) Nov [cited 2017 Aug 18];75: (2):189–95. Available from: http://linkinghub.elsevier.com/retrieve/pii/0022519378902308 |
[119] | Nath Mallick B , Thakkar M , Gangabhagirathi R . Rapid eye movement sleep deprivation decreases membrane fluidity in the rat brain. Neurosci Res [Internet]. (1995) Mar [cited 2017 Aug 18];22: (1):117–22. Available from: http://linkinghub.elsevier.com/retrieve/pii/016801029593696Y |
[120] | Tanimura A , Yamazaki M , Hashimotodani Y , Uchigashima M , Kawata S , Abe M , et al. The Endocannabinoid 2- Arachidonoylglycerol Produced by Diacylglycerol Lipase α Mediates Retrograde Suppression of Synaptic Transmission. Neuron. (2010) ;65: (3):320–7. |
[121] | Mulder J , Zilberter M , Pasquare SJ , Alpar A , Schulte G , Ferreira SG , et al. Molecular reorganization of endocannabinoid signalling in Alzheimer’s disease. Brain. (2011) ;134: (4):1041–60. |
[122] | Araki W , Wurtman RJ . Control of membrane phosphatidylcholine biosynthesis by diacylglycerol levels in neuronal cells undergoing neurite outgrowth. Proc Natl Acad Sci USA [Internet]. (1997) ;94: (22):11946–50. Available from: http://www.pubmedcentral.nih.gov/articlerender.fcgi7artid=23665%7B&%7Dtool=pmcentrez%7B&%7Drendertype=abstract |
[123] | Cui Y , Liu X , Wang M , Liu L , Sun X , Ma L , et al. Lysophosphatidylcholine and Amide as Metabolites for Detecting Alzheimer Disease Using Ultrahigh Performance Liquid Chromatography-Quadrupole Time-of-Flight Mass Spectrometry-Based Metabonomics. J Neuropathol Exp Neurol [Internet]. Oxford University Press; (2014) Oct 1 [cited 2017 Aug 18];73: (10):954–63. Available from: mycharhttps://academic.oup.com/jnen/article-lookup/doi/10.1097/NEN.0000000000000116 |
[124] | Wang D-C , Sun C-H , Liu L-Y , Sun X-H , Jin X-W , Song W-L , et al. Serum fatty acid profiles using GC-MS and multivariate statistical analysis:Potential biomarkers of Alzheimer’s disease. Neurobiol Aging [Internet]. (2012) Jun [cited 2017 Aug 18];33: (6):1057–66. Available from: http://www.ncbi.nlm.nih.gov/pubmed/20980076 |
[125] | Ross T , Jakubzig B , Grundmann M , Massing U , Kostenis E , Schlesinger M , et al. The molecular mechanism by which saturated lysophosphatidylcholine attenuates the metastatic capacity of melanoma cells. FEBS Open Bio [Internet]. Wiley-Blackwell; (2016) Dec [cited 2017 Aug 18];6: (12):1297–309. Available from: http://www.ncbi.nlm.nih.gov/pubmed/28255537 |
[126] | Han X , Rozen S , Boyle SH , Hellegers C , Cheng H , Burke JR , et al. Metabolomics in Early Alzheimer’s Disease:Identification of Altered Plasma Sphingolipidome Using Shotgun Lipidomics. Wang Y , editor. PLoS One [Internet]. (2011) Jul 11 [cited 2017 Aug 18];6(7: ):e21643. Available from: http://www.ncbi.nlm.nih.gov/pubmed/21779331 |
[127] | Wood PL , Mankidy R , Ritchie S , Heath D , Wood JA , Flax J , et al. Circulating plasmalogen levels and Alzheimer Disease Assessment Scale-Cognitive scores in Alzheimer patients. J Psychiatry Neurosci. (2010) ;35: (1):59–62. |
[128] | Moffett JR , Namboodiri MA . Tryptophan and the immune response. Immunology and Cell Biology. (2003) ;81: :247–65. |
[129] | Fernstrom JD . Effects of the diet on brain neurotransmitters. Metabolism. (1977) ;26: (2):207–23. |
[130] | Besedovsky L , Lange T , Born J . Sleep and immune function. Pflugers Archiv European Journal of Physiology. (2012) ;463: :121–37. |
[131] | Villafuerte G , Miguel-Puga A , Murillo Rodriguez E , Machado S , Manjarrez E , Arias-Carrion O . Sleep deprivation and oxidative stress in animal models:A systematic review. Oxidative Medicine and Cellular Longevity. (2015) ;2015. |
[132] | Szalardy L , Klivenyi P , Zadori D , Fulop F , Toldi J , Vecsei L . Mitochondrial disturbances, tryptophan metabolites and neurodegeneration:Medicinal chemistry aspects. Curr Med Chem [Internet]. (2012) ;19: (13):1899–920. Available from: http://www.ncbi.nlm.nih.gov/pubmed/22429096 |
[133] | Kokturk O , Kanbay A . Tryptophan Metabolism and Sleep. In Humana Press, Cham; (2015) [cited 2017 Sep 15]. pp. 23952. Available from: http://link.springer.com/10.1007/978-3-319-15630-9-10 |
[134] | Heneka MT , Golenbock DT , Latz E . Innate immunity in Alzheimer’s disease. Nat Immunol [Internet]. (2015) ;16: (3):229–36. Available from: http://dx.doi.org/10.1038/ni.3102%7B%25%7D0Ahttp://10.0.4.14/ni.3102 |
[135] | Mcgaha TL , Metz R , Prendergast GC , Mellor AL . Amino acid catabolism:A pivotal regulator of innate and adaptive immunity. Immunol Rev. (2012) ;249: :135–57. |
[136] | Guillemin GJ , Brew BJ . Implications of the kynurenine pathway and quinolinic acid in Alzheimer’s disease. Redox Rep [Internet]. (2002) ;7: (4):199–206. Available from: http://www.maneyonline.com/doi/abs/10.1179/135100002125000550?url%7B_%7Dver=Z39.88-2003%7B&%7Drfr%7B_%7Did=ori:rid:crossref.org%7B&%7Drfr%7B_%7Ddat=cr%7B_%7Dpub=pubmed |
[137] | Irwin MR . Sleep and inflammation in resilient aging. Interface Focus [Internet]. (2014) ;4: (5):20140009. Available from: http://www.pubmedcentral.nih.gov/articlerender.fcgi?artid=4142016%7B&%7Dtool=pmcentrez%7B&%7Drender©type=abstract |
[138] | Rhoades SD , Sengupta A , Weljie AM . Time is ripe:Maturation of metabolomics in chronobiology. Curr Opin Biotechnol [Internet]. (2017) Feb [cited 2017 Mar 15];43: :70–6. Available from: http://linkinghub.elsevier.com/retrieve/pii/S0958166916301938 |