Examining the Association of Academic Rank and Productivity with Metrics of Twitter Utilization Amongst Kidney Cancer Specialists
Abstract
BACKGROUND:
Twitter has emerged as an important platform for conversation surrounding cancer-related topics. As use has proliferated, a better classification of physicians engaging in cancer discussions on Twitter is warranted.
OBJECTIVES:
To better characterize the medical specialists involved in disseminating kidney cancer information on social media through academic and Twitter metrics.
METHODS:
Clinical practitioners with an expertise in kidney cancer were identified. Demographics, metrics of academic rank and productivity, and Twitter usage data were collected. Correlations were calculated for the generation of a model predictive of the number of Twitter followers. Analysis of the experts’ Twitter content was performed.
RESULTS:
Among 59 kidney cancer experts identified, 14 (23.7%) were assistant professors, 24 (40.7%) were associate professors, and 21 (35.6%) were full professors. A total of 5424 tweets were analyzed, 86% of which were medically-related. We identified several differences between academic rank and Twitter variables. Associate professors registered a greater median number of followers subscribed to their Twitter accounts (2360) versus assistant professors (1253) and full professors (934) (p = 0.03) and a greater median number of accounts they themselves followed (752 vs. 290 vs. 235, respectively; p = 0.0009). Use of a more generalized approach (ANCOVA) showed that the most predictive variables for the number of followers are number of tweets, H-index, and percentage of medical tweets (R2 = 0.70).
CONCLUSIONS:
This study supported correlations between metrics of academic and Twitter activity. The generation of a model to predict the number of followers on Twitter is novel – future work will validate this in other disease types.
INTRODUCTION
Social media has become an ever-present framework of communication in the modern digital age. Currently 72% of all Americans use at least one social media platform, a figure that has risen from just 5% in 2005 [1]. Usage of social media amongst oncologists and trainees has mirrored that of the public. In a survey of oncology specialists and fellows, 72% of respondents noted using social media [2]. This pervasiveness of social media in American society and, more specifically, in the domain of medical oncology has allowed for an increase in social and professional connectivity through platforms including, but not limited to, Facebook, LinkedIn, Instagram, and Twitter.
Twitter specifically has served as an amalgamation of the social and professional hemispheres. Nearly one quarter of all adult social media users are active on Twitter [3]. Within the field of oncology, Twitter has emerged as a predominant platform through which cancer information is publicized and propagated by healthcare professionals, survivors, community activists, and industry liaisons [4–8]. The novel opportunity for education and outreach through “social medicine” has led to various perspectives on how to best navigate and maximize Twitter as a communication tool for the practicing oncologist [9–12].
Although Twitter activity within the field of oncology has steadily risen over the past decade, there is little formal study of this phenomenon. Amongst the limited reports available, most focus on content analysis and characterization of tweets related to specific cancer types, such as lung cancer and breast cancer [13–15]. These studies are useful for assessing the content of Twitter dialogue and providing an understanding of what topics are being discussed with respect to cancer care. However, we recognize the additional importance of developing a better classification of Twitter users, particularly physicians, engaging in these discussions.
We sought to perform such an analysis in the context of kidney cancer. There are expected to be 73,820 new kidney cancer diagnoses and 14,770 deaths due to kidney cancer in 2019 [16]. There have been monumental changes in renal cell carcinoma (RCC) therapy over the past two decades, shifting from cytokine-based therapies to small-molecule inhibitors (targeted therapy) and/or immune checkpoint inhibitors (immunotherapy) – these have been the subject of a great deal of discussion on social media [17]. A recent study examining communication around kidney cancer on Twitter reported that dialogues related to support and treatment were most common, but noted that patients or loved ones typically initiated and directed such conversations, rather than physicians or medical providers [18]. As the nature of medical providers engaged on Twitter is poorly characterized, the current study seeks to determine associations between provider characteristics and Twitter activity amongst physicians specializing in kidney cancer.
METHODS
Study participants
Kidney cancer specialists were defined as physicians who (1) maintained a faculty appointment at an academic center, (2) listed an expertise in kidney cancer online (in their institutional profile, Google Scholar profile, and/or Twitter profile), and/or (3) had at least 2 MEDLINE citations emerging with the joint search terms “kidney cancer” or “renal cell carcinoma”. All experts included in this study also had active Twitter accounts at the time of data collection. Demographic data were collected in addition to data pertaining to academic rank, which included categorical descriptors of faculty status (assistant, associate, or full clinical professor) and years on faculty (0-2, 3-5, 6-10, 11-20, 21+), specialty (medical oncology or urology), and the 2018 US News & World Report ranking of their affiliated cancer center (0-10, 11-40,>40, international). Values of academic productivity – H-Index and number of publications – were also collected. Academic productivity data were retrieved from publicly-available information found on Scopus, a citation and abstract database managed by Elsevier. H-index was chosen as a metric of academic productivity because of its ability to measure both the productivity and citation impact of an individual [19]. Twitter metrics — number of tweets, number following, number of followers, cumulative likes received, and time on platform – were collected to assess an individual’s utilization of the Twitter platform. This study was IRB exempt.
Content analysis
A content analysis was performed on a subset of up to 100 recent tweets from each kidney cancer specialist. If the respective Twitter account had less than 100 tweets, the entire tweet history of the account was analyzed. Tweets were categorized as either “medically-related” or “not-medically-related”. Medical tweets were those related to at least one of the following topics: therapeutics/clinical trials/clinical research, peer or patient support, fundraising/advocacy/outreach, or basic science research. If a tweet did not explicitly include material related to one of these categories but was included in a thread whose original tweet did fall into an above category, the tweet was still categorized as “medically-related”. Each characterization was performed by two authors (N.S. and M.F. or N.S. and M.N.) blinded to the other’s determinations. If there was not a consensus on the classification, the tweet was deemed “not-medically related” by default. Additionally, tweets were also categorized as an “original” or a “retweet”. Percentages of original versus retweet and medically-related versus non-medically-related were calculated for each kidney cancer expert.
Statistical analysis
Subject demographic and academic information as well as Twitter utilization data was summarized and stratified by academic rank, reported as number (percent) and median (inter-quartile range) for categorical and continuous data, respectively. Fisher’s exact test or Kruskal-Wallis test was used to identify any differences between metrics by academic rank. Pearson correlation coefficients were calculated for numeric variables to assess association, and the Kruskal-Wallis test was performed to test for differences in number of followers across values of categorical variables. Natural log (ln) transformations on numeric variables were used to reduce right-skewness, and to improve the overall model prediction. Analysis of covariance (ANCOVA) was chosen for the prediction of the natural log of the number of followers (ln(followers)) to test the main and interaction effects of categorical variables while controlling for effects of continuous data elements. All variables and appropriate interactions were tested, including Twitter-related predictors and academic data. Variables were subsequently included in the model if their p-value (with respect to their correlation with ln(followers)) was below the 0.05 threshold or if the interaction with another variable was significant.
RESULTS
A cohort of physicians with expertise in kidney cancer (n = 59) were identified for analysis, 48 (81.4%) of which were male and 11 (18.6%) female. Fourteen (23.7%) were assistant professors, 24 (40.7%) were associate professors, and 21 (35.6%) were full professors. Thirty-four (57.6%) were identified as medical oncologists compared to 25 (42.4%) urologists. Demographic, academic, and Twitter metrics stratified by academic rank are presented in Table 1. Assistant professors had a median H-index of 16.5 compared to 32.0 for associate professors and 42.0 for full professors (p = 0.0001) while median number of publications for the same groups were 48.5, 115, and 233, respectively (p = 0.0004). Associate professors also registered a greater median number of followers subscribed to their Twitter accounts (2360) versus assistant professors (1253) and full professors (934) (p = 0.03) as well as a greater median number of accounts they themselves followed on Twitter (752 vs. 290 vs. 235, respectively; p = 0.0009).
Table 1
Demographic, academic (A), and Twitter (B) metrics of identified kidney cancer experts based on faculty rank as determined from institutional profiles. * Indicates a significant difference (p < 0.05) across groups using the Kruskal-Wallis Test. Abbreviations: IQR=Interquartile Range
A. | ||||
All experts | Assistant Professor | Associate Professor | Full Professor | |
N=59 | N=14 | N=24 | N=21 | |
Gender (%) | ||||
Female | 11 (19) | 3 | 4 (17) | 4 (19) |
Male | 48 (81) | 11 | 20 (83) | 17 (81) |
Specialty (%) | ||||
Medical Oncology | 34 (58) | 9 (64) | 15 (62) | 10 (48) |
Urology | 25 (42) | 5 (36) | 9 (38) | 11 (52) |
Years on Faculty (%) | ||||
0–2 | 3 (5) | 2 (14) | 0 (0) | 0 (0) |
3–5 | 1 (2) | 0 (0) | 1 (4) | 0 (0) |
6–10 | 9 (15) | 3 (21) | 2 (8) | 4 (19) |
11–20 | 30 (51) | 8 (57) | 15 (63) | 8 (38) |
20+ | 16 (27) | 1 (7) | 6 (25) | 9 (43) |
Ranking of Affiliated Cancer Center by US News & World Report in 2018 (%) | ||||
1–10 | 19 (32) | 5 (36) | 9 (38) | 5 (24) |
11–40 | 16 (27) | 2 (14) | 8 (33) | 6 (29) |
40+ | 15 (25) | 4 (29) | 5 (21) | 6 (29) |
International | 9 (15) | 3 (21) | 2 (8) | 4 (19) |
H-Index, Median (IQR)* | 34 (19–44) | 16.5 (10–22) | 32 (20–41) | 42 (35–63) |
Number of Publications, Median (IQR)* | 132 (66–259) | 48.5 (35–97) | 115 (76–243) | 233 (165–321) |
B. | ||||
Years on Twitter Platform, Median (IQR) | 6.0 (3.4–8.0) | 4.9 (2.8–8.4) | 6.5 (3.9–8.5) | 6.0 (3.7–7.2) |
Number of Tweets, Median (IQR) | 832 (345–2724) | 806 (441–1772) | 1972 (378–4665) | 645 (80–1573) |
Number Following, Median (IQR)* | 380 (172–755) | 290 (225–495) | 753 (213–1103) | 235 (83-514) |
Number of Followers, Median (IQR)* | 1271 (637–2545) | 1253 (631-1823) | 2360 (1073-3166) | 934 (535-1701) |
Number of Likes, Median (IQR) | 1400 (326–5711) | 1051 (475-6222) | 3960 (429-6851) | 470 (79-3114) |
A sampling of 5425 tweets were analyzed across the 59 identified kidney cancer experts. A total of 2295 (42%) tweets were considered original while 3078 (57%) were instances of retweets (52 tweets were considered unevaluable). Median percentage of original tweets was 41% for the cohort, while median percentage of medical tweets was 87% in entirety. 4666 of the 5424 (86%) tweets were characterized as medically-related by concordance of opinions between two blinded authors.
Significant relationships were identified between multiple numeric variables (Fig. 1 and Supplemental Table 1). Associations of Twitter utilization variables were most commonly significant, accounting for 6 of 11 significant (or near-significant) relationships identified and an additional 4 significant associations compared Twitter utilization characteristics to Twitter content metrics.
Fig. 1
Heat map of canonical correlations for all numeric values.
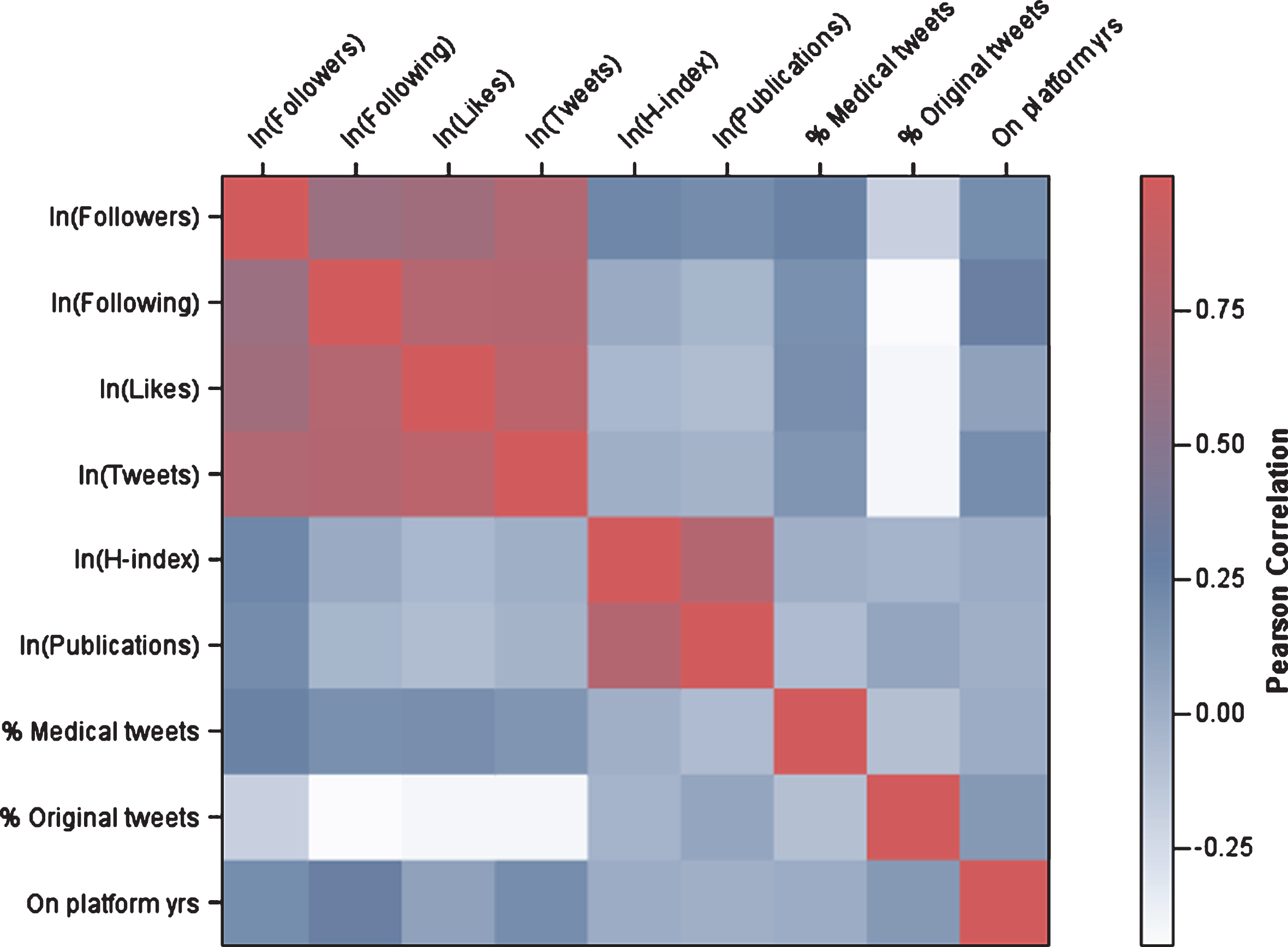
Log transformation was applied to the variables accounting for the number of followers, following, tweets, likes, publications, and clinician H-index to reduce right-skewness of these parameters. Pearson correlation coefficients were used to outline the strength of relationships between these variables, as well as number of years on platform, percentage of original tweets, and percentage of medical tweets. A heat map outlining the correlation coefficients is seen in Figure 1 and demonstrates that the strongest correlations are between ln(followers) and ln(tweets), ln(likes), and ln(following) (ρ: 0.79, 0.66, 0.63, respectively).
A predictive model for the natural log of the number of followers was developed using analysis of covariance (ANCOVA). Covariates tested in the model included years on platform, and natural log transformations of variables # following, # tweets, # likes, # publications, and H-index. Categorical variables tested included gender, U.S. News Ranking (1-10, 11-40,>40, and International), tenure status (Assistant, Associate, or Full), years on faculty (≤10, 11-20, 20+), percentage of medical tweets (<80% vs. ≥80%), and percentage of original tweets (<25% vs. ≥25%). Table 2 displays the estimates of the most complete predictive model for ln(followers), which includes ln(tweets), ln(H-index), and the indicator for ≥80% medical tweets (p ≤ 0.001 for all predictors), and produced an R2 of 0.70. All other potential predictors and interaction terms tested and found statistically insignificant in the presence of these three predictors.
Table 2
Parameter estimates in ANCOVA model for ln(followers)
Parameter | Estimate | Std err | t Value | Pr≥|t| |
ln (Tweets) | 0.45 | 0.045 | 10.12 | ≤0.0001 |
ln (H-index) | 0.29 | 0.085 | 3.40 | 0.0013 |
Medical Tweets≤80% | 2.86 | 0.43 | 6.72 | ≤0.0001 |
(baseline) | ||||
Medical Tweets 80–100% | 3.21 | 0.43 | 7.55 | ≤0.0001 |
The results of the model can be exponentiated to remove the logarithmic transformation and obtain the following formula:
DISCUSSION
This study represents the first effort to investigate the relationship between Twitter metrics and academic rank/productivity amongst cancer experts. Ultimately, number of followers could be built into a model incorporating both academic parameters (e.g., H-index) and parameters related to Twitter utilization (e.g., number of tweets). Twitter following was further enhanced when physicians had a higher proportion of medically relevant tweets. In a growing field of analytics related to Twitter and social media, the data we report here is the first (a) exclusively focused on kidney cancer and (b) is the first to develop an objective model including both academic parameters and metrics of social media utilization. In our opinion, it was reassuring to see the positive association between academic metrics like H-index and popularity on Twitter. However, our results further demonstrate that this alone is not enough in most cases to generate a large pool of followers – the academic Twitter user must also have a sizeable volume of medically relevant tweets.
Additional work examining the network connectivity of physicians engaged in kidney cancer discourse on Twitter would be useful. Recent work mapping physician networks has provided a foundation for understanding the dissemination pattern of medical information on Twitter [20]. Future efforts focused on kidney cancer experts may shine light on the impact of such communication for providers, patients, and advocacy groups.
This study had several limitations – one is the general linear model’s dependence on Twitter metrics as predictors for the number of followers expected for kidney cancer experts. The reliance on Twitter metrics as covariates for the prediction of the number of followers for kidney cancer experts may mask the importance of academic and demographic metrics in predicting the number of Twitter followers. Additionally, the generated model results in 30% unexplained variation in the number of followers. This unaccounted-for variation yields further opportunities to explore the activity of kidney cancer experts on Twitter.
There are certain characteristics that could influence our results that are challenging to account for. For instance, many clinicians use Twitter for both academic and personal purposes – in our review, we found a blend of these tweets in the vast majority of individuals we sampled. Because we limited our analysis to the last 100 tweets from each individual, we may have captured an unrepresentative sample. Further elements that can influence Twitter dialogue, like conflict of interest, for instance, are inconsistently reported by physicians and were therefore not captured in our effort. Although we included a broad spectrum of variables, there were several that others have examined in the context of similar studies which we did not. For instance, Chandrasekar et al have assessed variables such as institutional resident volume and proportion of institutional faculty on Twitter. [21]. Ultimately, this study had some parallel conclusions, linking Twitter activity to academic metrics.
Remaining questions include the accuracy of kidney cancer-related information shared on Twitter. Although a recent study has shown that the majority of cancer information shared on social media platforms was based on factual medical and scientific evidence, it is important to note that Twitter was not one the platforms included in these analyses [22]. An investigation of colorectal cancer information credibility on Twitter also concluded that the majority of tweets were, in fact, accurate [23]. Work outside the domain of oncology concluded that credible news items typically have many reposts and are propagated by authors who have previously written a large number of messages [24]. These criteria are generally characteristic of the Twitter discourse examined in this study, but no study to date has directly investigated the accuracy, credibility, and validity of kidney cancer information accuracy on Twitter or other social medias.
These conclusions coupled with the data from this study provide an initial suggestion that clinical experts are engaging in diverse discussions regarding kidney cancer on Twitter and effectively utilizing both original messaging and retweets, thus supporting the notion that credible information is being propagated by specialists in a responsible manner. A more robust content analysis of tweets examining medically-accurate information from these experts will be required, however, before making a definitive judgement in support of this hypothesis.
FUNDING
No external funding was used in the preparation of this manuscript.
AUTHOR CONTRIBUTIONS
Conception and Design: NJS, MMS, NR, SKP; Acquisition of Data: NJS, MF, DP, MN, RH, YD; Analysis and Interpretation of Data: NJS, NR; Drafting of Manuscript: NJS, SKP; Critical Revision of Manuscript: NJS, SKP, NR; Statistical Analysis: NR Supervision: SKP.
CONFLICT OF INTEREST
Nicholas Salgia, Matthew Feng, Dhruv Prajapati, Richard Harwood, Michael Nissanoff, Yash Dara, Nora Ruel, Meghan Salgia declare that they have no conflicts of interest.
Sumanta K. Pal, MD: Honoraria - Novartis, Medivation, Astellas Pharma; Consulting or Advisory Role - Pfizer, Novartis, Aveo, Myriad, Pharmaceuticals, Genentech, Exelixis, Bristol-Myers Squibb, Astellas Pharma; Research Funding - Medivation.
SUPPLEMENTARY MATERIAL
[1] The supplementary material is available in the electronic version of this article: https://dx.doi.org/10.3233/KCA-200083.
References
[1] | Social Media Fact Sheet 2019. |
[2] | Adilman R , Rajmohan Y , Brooks E , Urgoiti GR , Chung C , Hammad N , Trinkaus M , Naseem M , Simmons C , Adilman R , Rajmohan Y , Brooks E , Roldan Urgoiti G , Chung C , Hammad N , Trinkaus M , Naseem M , Simmons C . Social media use among physicians and trainees: Results of a national medical oncology physician survey. Journal of Oncology Practice. (2016) ;12: (1):79–80, e52-60. |
[3] | Pew Research Center: Social Media Fact Sheet, 2018. |
[4] | Murthy D , Eldredge M . Who tweets about cancer? An analysis of cancer-related tweets in the USA. Digital Health. (2016) ;2: :2055207616657670. |
[5] | Koskan A , Klasko L , Davis SN , Gwede CK , Wells KJ , Kumar A , Lopez N , Meade CD . Use and taxonomy of social media in cancer-related research: a systematic review. American Journal of Public Health. (2014) ;104: (7):e20–37. |
[6] | Pemmaraju N , Thompson MA , Mesa RA , Desai T . Analysis of the use and impact of twitter during American society of clinical oncology annual meetings from 2011 to 2016: Focus on advanced metrics and user trends. Journal of Oncology Practice. (2017) ;13: (7):e623–e31. |
[7] | Tsuya A , Sugawara Y , Tanaka A , Narimatsu H . Do cancer patients tweet? Examining the twitter use of cancer patients in Japan. Journal of Medical Internet Research. (2014) ;16: (5):e137. |
[8] | Sugawara Y , Narimatsu H , Hozawa A , Shao L , Otani K , Fukao A . Cancer patients on Twitter: a novel patient community on social media. BMC Research Notes. (2012) ;5: :699. |
[9] | Sedrak MS , Dizon DS , Anderson PF , Fisch MJ , Graham DL , Katz MS , Kesselheim JC , Miller RS , Thompson MA , Utengen A , Attai DJ . The emerging role of professional social media use in oncology. Future Oncology (London, England). (2017) ;13: (15):1281–5. |
[10] | Abuhadra N , Majhail NS , Nazha A . Impact of social media for the hematologist/oncologist. Seminars in Hematology. (2017) ;54: (4):193–7. |
[11] | Markham MJ , Gentile D , Graham DL . Social Media for Networking, Professional Development, and Patient Engagement. American Society of Clinical Oncology educational book American Society of Clinical Oncology Annual Meeting. (2017) ;37: :782–7. |
[12] | Sedrak MS , Attai DJ , George K , Katz MS , Markham MJ . Integrating social media in modern oncology practice and research. American society of clinical oncology educational book American Society of Clinical Oncology Annual Meeting. (2018) ;38: :894–902. |
[13] | Sedrak MS , Cohen RB , Merchant RM , Schapira MM . Cancer communication in the social media age. JAMA Oncology. (2016) ;2: (6):822–3. |
[14] | Sutton J , Vos SC , Olson MK , Woods C , Cohen E , Gibson CB , Phillips NE , Studts JL , Eberth JM , Butts CT . Lung cancer messages on twitter: Content analysis and evaluation. Journal of the American College of Radiology: JACR. (2018) ;15: (1 Pt B):210–7. |
[15] | Thackeray R , Burton SH , Giraud-Carrier C , Rollins S , Draper CR . Using Twitter for breast cancer prevention: an analysis of breast cancer awareness month. BMC Cancer. (2013) ;13: :508. |
[16] | Siegel RL , Miller KD , Jemal A . Cancer statistics, 2019. CA: A Cancer Journal for Clinicians. (2019) ;69: (1):7–34. |
[17] | Salgia NJ , Dara Y , Bergerot P , Salgia M , Pal SK . The changing landscape of management of metastatic renal cell carcinoma: Current treatment options and future directions. Current Treatment Options in Oncology. (2019) ;20: (5):41. |
[18] | Sedrak MS , Salgia MM , Decat Bergerot C , Ashing-Giwa K , Cotta BN , Adashek JJ , Dizman N , Wong AR , Pal SK , Bergerot PG . Examining public communication about kidney cancer on twitter. JCO Clinical Cancer Informatics. (2019) ;3: :1–6. |
[19] | Hirsch JE . An index to quantify an individual’s scientific research output. Proceedings of the National Academy of Sciences of the United States of America. (2005) ;102: (46):16569–72. |
[20] | Mishori R , Singh LO , Levy B , Newport C . Mapping physician Twitter networks: describing how they work as a first step in understanding connectivity, information flow, and message diffusion. Journal of Medical Internet Research. (2014) ;16: (4):e107-e. |
[21] | Chandrasekar T , Goldberg H , Klaassen Z , Wallis CJD , Leong JY , Liem S , Teplitsky S , Noorani R , Loeb S . Twitter and academic urology in the United States and Canada: A comprehensive assessment of the Twitterverse in 2019. BJU International. (2020) ;125: (1):173–81. |
[22] | Gage-Bouchard EA , LaValley S , Warunek M , Beaupin LK , Mollica M . Is cancer information exchanged on social media scientifically accurate? Journal of Cancer Education: The Official Journal of the American Association for Cancer Education. (2018) ;33: (6):1328–32. |
[23] | Park S , Oh HK , Park G , Suh B , Bae WK , Kim JW , Yoon H , Kim DW , Kang SB . The source and credibility of colorectal cancer information on twitter. Medicine. (2016) ;95: (7):e2775. |
[24] | Castillo C , Mendoza M , Poblete B . Information credibility on twitter. Proceedings of the 20th international conference on World wide web; Hyderabad, India. 1963500: ACM; 2011. p. 675-84. |