The analysis of security and privacy risks in smart education environments
Abstract
The contemporary smart educational environment uses different information technologies like social networks, virtual laboratories, augmented reality, artificial intelligence, big data, and so on. Each of these technologies has its security and privacy threats profile, but their integration in one system can lead to completely new challenges. The article analyses the technological development of smart educational environments from the point of view of their security and privacy issues. Any technological or legislative security control could be broken as the result of one mistake caused by human factors. People with different levels of competence are interacting every day with each other in educational environments. The risks of personal data leaking or hacking of educational services should be minimized during this interaction. Therefore, not only the key technologies that form the architecture of the educational environment but also the main points of interaction between the users and the education environment should be taken into account in the analysis. The article provides a basic analysis of security and privacy risks for smart education environments. As the result, the analysis identifies key information security technologies development of which is necessary for the sustainable development of a smart educational environment as part of a smart city.
1.Introduction
The worldwide transformation to a post-industrial society inevitably has a strong impact on the education system in every country. One of the key trends in the 21th-century education is that it has become mass; the need for higher education arises in an increasing number of people [18]. Therefore, it is necessary to either increase the number of teachers or increase the efficiency of the educational system. In the concept of a traditional research university, where each teacher is also a scientist, it is impossible providing quantity of highly qualified teachers [41] enough to meet the mass need in higher education. Thus, the problem can have only one solution – to increase the efficiency of the educational process.
The diversity of various specialties and the multidisciplinary nature of modern professions has led to the need of education individualization. The computing technologies has ensured a significant increase in labor productivity; those tasks that previously took months of calculations and design are now can be done in several hours due to different computers’ programs. Thus, mass training of specialists with approximately the same competencies is not needed anymore. Nowadays, the challenge for the high education system is the training of specialists with a unique combination of competencies based on individual educational trajectories [7].
The research and development technology cycle is reduced to five years, and the cycles of specialist training in higher education are four to six years at bachelor and master degrees respectively. The traditional education program cannot handle such high rates of technology development. Thus, the principles of life-long/width learning were introduced to meet the requirements of continuous learning [3,11].
Online education tools can partially solve these problems [30]. The module structure of the online course allows upgrading it fast without damaging other parts. Furthermore, a traditional laboratory group contains approximately fifteen people, and around one hundred people can fit in the lecture room, but online classrooms can easily increase these numbers three and more times. However, online education, like ordinary education, is a process of learning, training, and socialization. That is why to substitute traditional education environment, the Smart Educational Environment (SEE) should ensure the effectiveness of each education process component, not just learning.
When developing the smart educational environment, the new education system actively uses different Information Technologies (IT) like cloud services, big data, Artificial Intelligence (AI), machine learning, and others. The development of any technology or system is always associated with the development of their safety and security. For example, modern civil aviation is simply unthinkable without strict protocols that ensure safety. It is also true for smart educational environments. Without a comprehensive analysis of the risks associated with online education, further development of SEE in this direction is impossible.
Taking into account the risks of online education, universities try to implement information technologies in a traditional education environment. Therefore, the different smart campus technologies like flipped classroom [6], blended learning (online labs, self-blended, rotation model, flex model, online drive) [36], and ambient intelligence classroom [2] were developed. In contrast to online education, smart campus technologies do not solve the challenges of mass education. Of course, several problems of traditional education can be mitigated with technologies of smart campus, but not the main problem of «mass higher education». It is still the same campus with the same restrictions in the localization, the capacity of classrooms and buildings, and the availability of social infrastructure for different groups of citizens.
The purpose of the article is to analyze the technologies that form the educational environment from safety, security, and privacy points of view. The analysis focuses on the online education environment and partially raises the questions of smart campuses.
2.Smart education environments
The development of online education systems was focusing on online learning processes at the first stage. As a result, various systems such as MOODLE, OpenEdx, and CANVAS for managing the learning process, i.e., Learning Management System (LMS), and content, i.e., Content Management System (CMS), appeared. A student interacts with the LMS that controls his/her access to educational content and schedule, monitors activity and training effectiveness. Teachers use CMS to structure educational content, set the pace of its passage, evaluation parameters, and other elements of the course.
The architecture of both systems is based on the modular principles that allow further improvement of online education environment functionality. The architecture of the educational environment of the first and second generation is quite simple and ensures the interaction between three main roles (student, teacher, and educational organization) during the education process as shown in Fig. 1.
Fig. 1.
The architecture of the first and second-generation online education platforms.
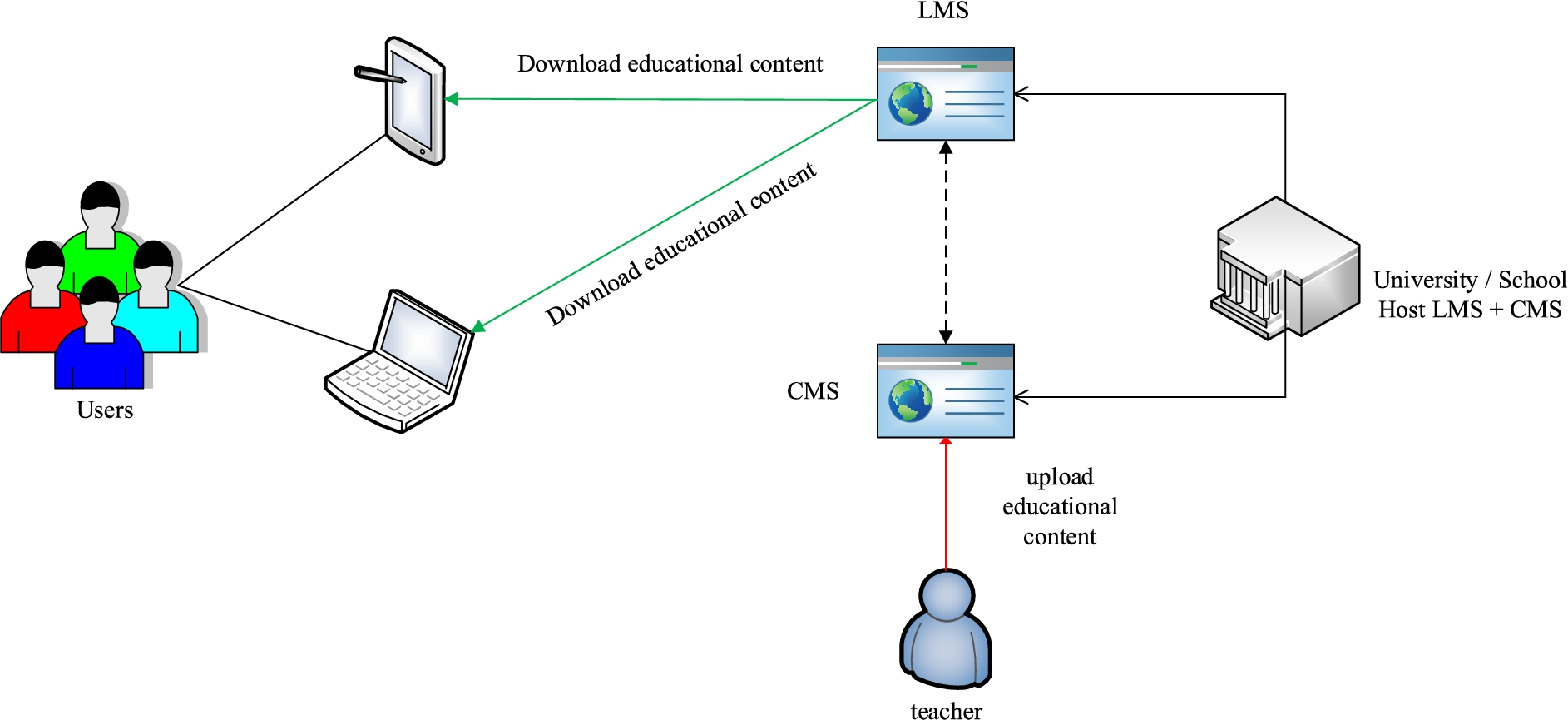
However, these platforms can host only massive open online courses (MOOCs) [1]. Testing the first and second-generation online courses identified the following main problems [12]:
Lack of synchronous interaction with teacher – all education content is in asynchronous format. It means that the engagement times of teacher and student never intersects. Student can interact only with uploaded education content, and teacher can only update educational content after course ends. Changing something here now is impossible without disrupting the individuals’ learning process;
Implementation complexity of remote access to equipment and Computer-Aided Design (CAD) tools for technical and engineering courses – the largest part of the first and second-generation online courses asks to download various software or buy hardware to solve practical tasks. Certain educational programs in engineering, chemistry, biology, and so on cannot implement this model because the software/hardware can be too expensive or unavailable at all;
Lack of knowledge control functions and inability to control soft-skills – tests became a common tool for measuring learning effectiveness. Can be competencies in critical thinking, emotional empathy, and leadership traits measured accurately with only one test? It is usually impossible. Thus, the education course should have much more tools to measure learning outcomes in different skills, including learning outcomes related with practical and laboratory works or soft-skills.
The next important stage in the development of online courses was the introduction of the «connectivism»methodology [42]. This methodology places emphasis on the processes of generating knowledge that include the connection of different knowledge holders like people, books, etc. Students’ competencies suggest an ability to generate proof of statements with peer-to-peer (p2p) assessment.
When studying an online course with p2p elements, each student in the course becomes also a teacher that allows not only to reduce the teacher workload but also to master skills for assessing different solutions [27]. In addition, students create social groups similar to professional groups, and sometimes directly join the professional community at the appropriate venues – forums, conferences, etc. To provide these changes, the educational platform was revised as shown in Fig. 2.
Fig. 2.
The architecture of third-generation online education platforms.
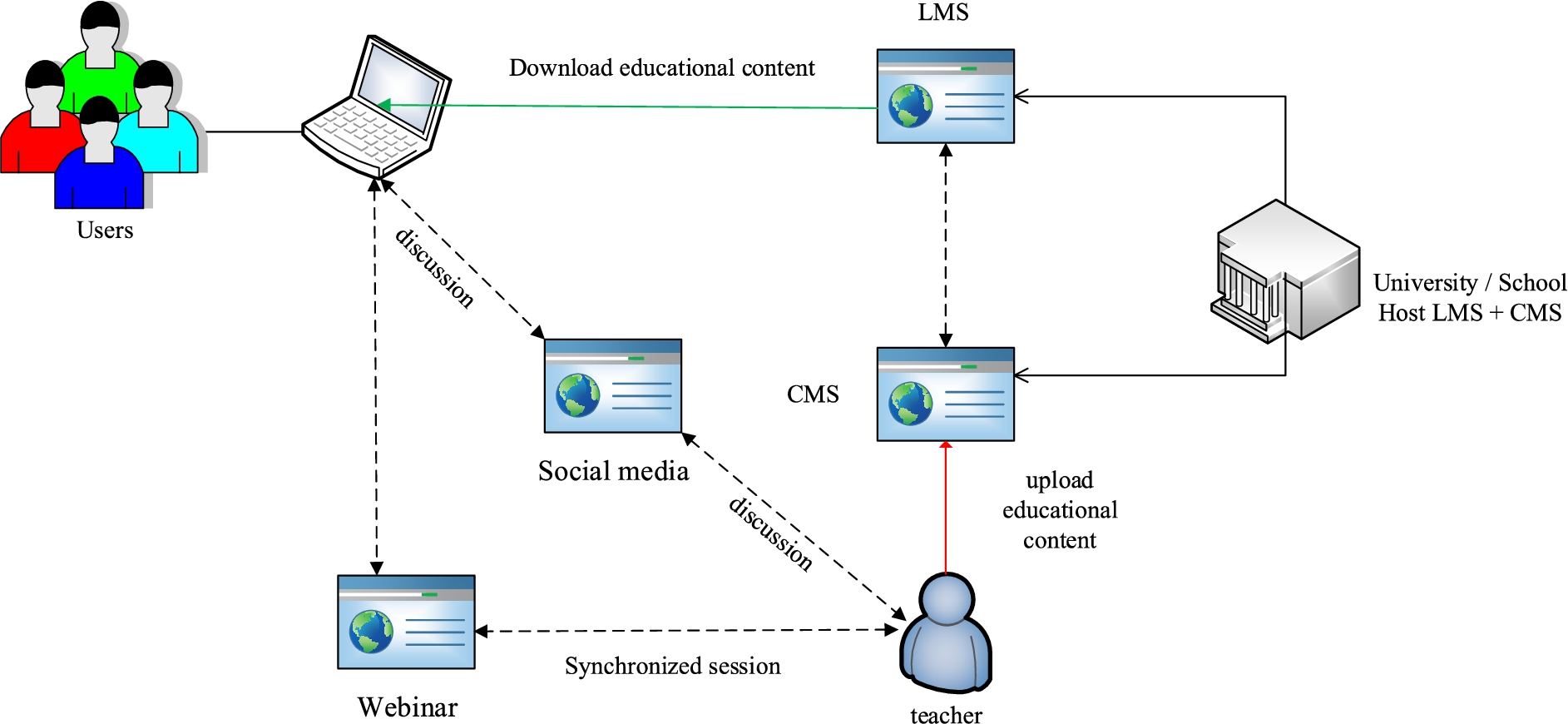
The concept of 3rd generation online courses is the possibility of having a dialogue between both teacher and students, and students themselves. However, the 3rd generation platforms do not provide sufficient opportunities such as educational games, virtual or augmented reality for different types of interaction.
The development of cloud computing, augmented and virtual reality technologies has transformed many professions. A specialist has essentially become a remote operator of a technical system like a robot, aircraft, and machine. Therefore, online education is now possible even in areas such as medicine, wherein full-time education was traditionally required to introduce a person to real working conditions. One more example is the joint projects or collaborative development in IT industry where the product is the result of collaborative work of different specialists – analytics, developers, system administrators, and so on.
The smart educational system should ensure the adaptation of future specialists to such working conditions. Therefore, online education is no longer an opportunity, but a new requirement, especially in the field of IT specialists training. The smart education environment should implement the concept of collective creativity and project work, but these opportunities for joint development are not available within the framework of the 3rd generation platforms. All these factors led to the development of fourth-generation educational platforms with special online courses [40].
The key features of these platforms are the expanded use of design approach in joint projects, synchronous sessions in groups, and usage of cyber-physical/virtual laboratories. The video conferencing system (VCS) solve the problem of synchronous session’s organization only at the first approximation. However, VCS is not able to solve this problem completely, since the effectiveness of training for a student largely depends on the degree of his immersion in the process. It is also true for teachers; it is quite difficult to immerse yourself in the lecture process staying in-home environment because existing different noise sources or other bad conditions. The online lecturing rooms such as Oxford HIVE, Harvard hub, and others [46] are partially mitigating this challenge. However, common universities and schools, where the need for these systems is often high, cannot buy them due to high costs. In this regard, the development of virtual reality technologies and virtual lecture rooms received impetus [35,45].
The support of virtual and online laboratories also changes the educational environment. Such laboratories can be both simple CAD as cloud service and/or a complex laboratory that allows a student to remote control the robotic complex using special programs (Fig. 3(b)) that students are developing during the learning process as shown in Fig. 3(a).
Fig. 3.
(a) The cyber-physical laboratory “autonomous service robots” in PNRPU. (b) The example of program developed by student to control robots in laboratory.
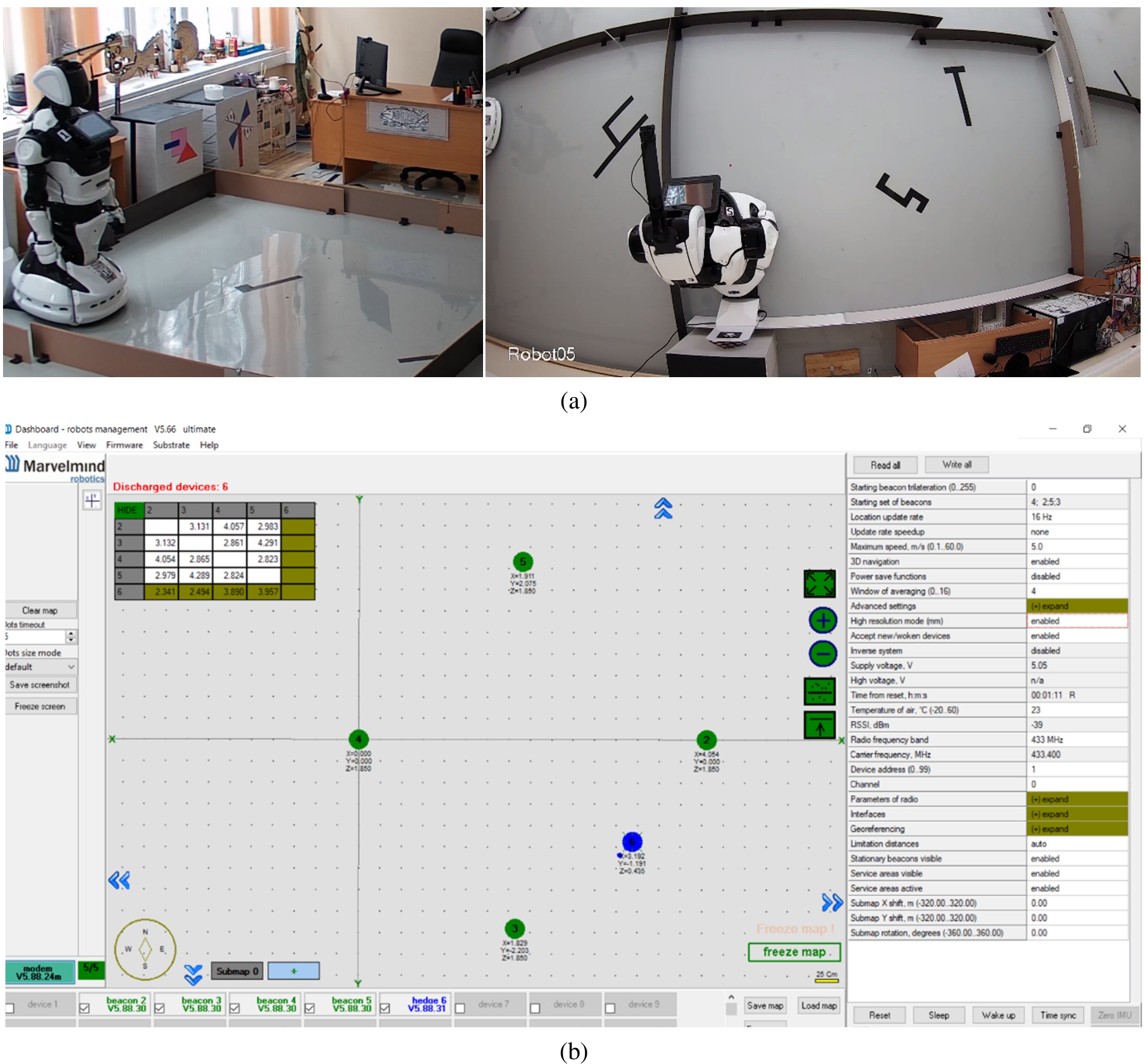
The example of the Autonomous service robotics online laboratory at the master degree program in Perm National Research Polytechnic University (PNRPU) demonstrates that the concept of 4th generation educational platform allows educating specialists in technical sciences and engineering. In this laboratory, students can develop special programs to control, navigate, and solve other tasks on real robots, including joint development of robots’ missions for different business cases. For example, students developed missions of robot waiter and robot animator. Thus, now the educational environment is a complex distributed system in which a plurality of users interacts with a set of services as shown in Fig. 4.
Fig. 4.
The architecture of fourth-generation online education platforms.
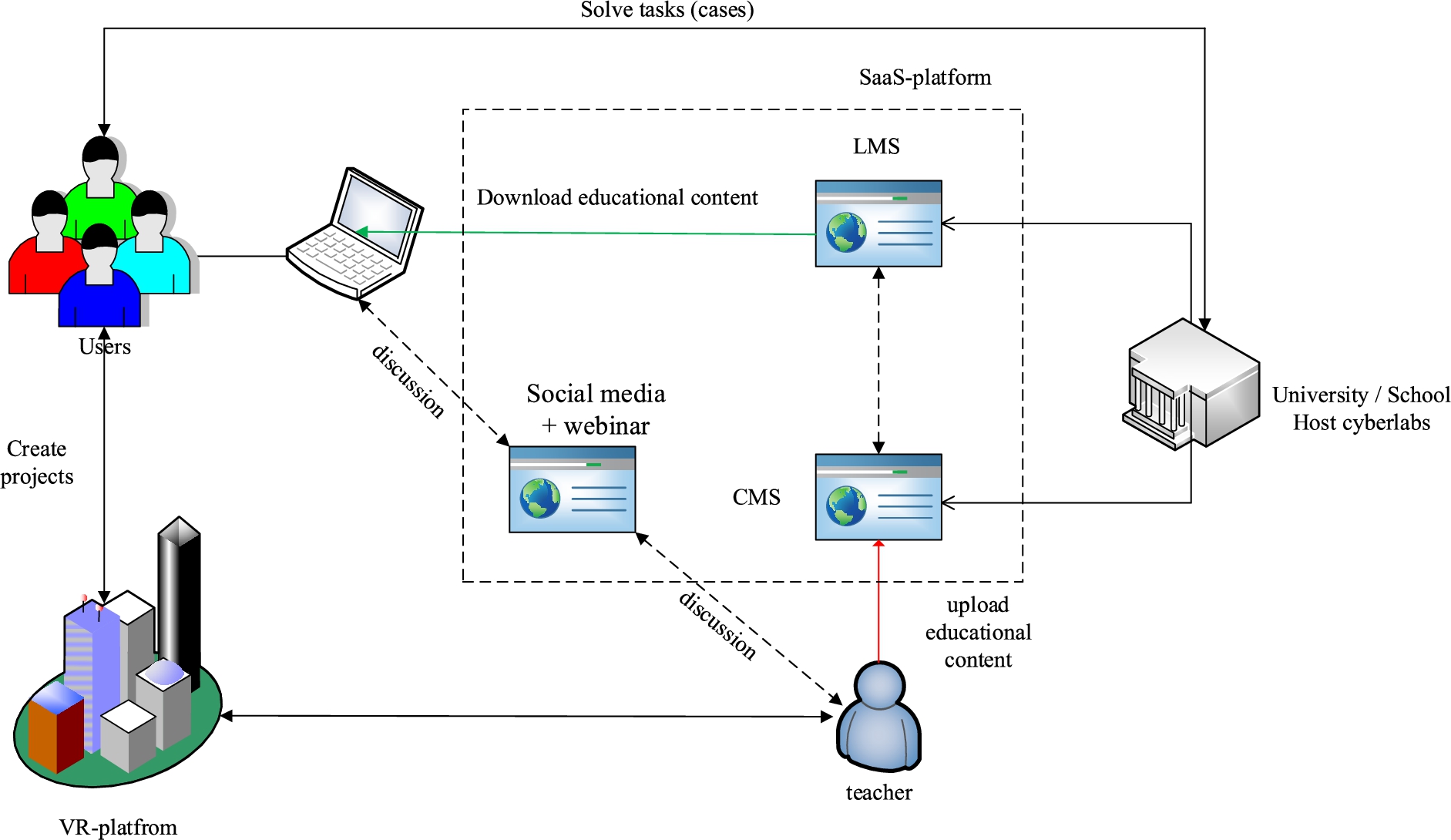
It should be noted that not only the architecture of the system as a whole is complicated but also each component individually. As a result, one teacher is no longer able to provide development and support for all elements of the educational course. Therefore, the development of online courses is usually teamwork nowadays.
The proctoring module in LMS improves the evaluation system. The LMS proctoring module based on AI should have access to the microphone, video camera, and other software on the student’s computer to ensure the honesty of exam or test passing. In addition, SEE proctoring and authentication system stores biometric personal data to recognize voice or face that carries significant information security risks [21,48]. Moreover, AI-based course assistants supplement students learning process in LMS. An example of such modules is chatbots, which helps students answering their questions and reducing the teacher workload [15,38].
The existing problem of cheating and copying answers for practical tasks can be solved through cyber exercises (online training ground or cyber ranges in information security). Training ground is a model of an information system in which user should solve certain tasks with a scenario. The training ground is constructed according to a given template, but each time with a new random answer to the task. Thus, the student should perform an algorithm to solve the task; he/she can copy this algorithm, but not the answer to the task itself.
The technology of online training grounds creates an effective combination with the trend towards the gamification of education [24,31,43,44]. It is quite simple to create competition from the passage of such training grounds and reward the best students. Herewith, the uniqueness of the training grounds provides transparency of the competition results. At the same time, the framework of online training grounds allows all students to repeat tasks until completion. Thus, students who failed exercise can study more effectively since they gain experience faster if nothing prevents them from repeating the attempts to solve exercise until successful result. The students who passed an exercise can further repeat it to improve their skills.
In summary, the key challenges of 4th generation smart education environment:
Implementation of AI-based solutions for education services – personal assistant, smart scoring in tests, AI teachers, and so on;
Usage of biometric personal data – biometric authentication, exam proctoring, behavioral biometric for individual scoring of practical skills;
Implementation of virtual reality, cyber-physical, and augmented reality – development and multiplication of classrooms, laboratories, and educational games;
Usage of collaborative education methods in different fields – co-labs, students’ joint projects.
3.Security and privacy risks in smart education environments
Smart education environments are inheriting both security threats from traditional systems and facing completely new threats in the information space. The first step is the identification of the information security and privacy risks and threats. Risks are associated with key challenges of the IT implementation into the educational environment. These key challenges are:
1. The analysis of smart educational environment security should consider internal threat sources potential not lower than medium and external threat sources potential not lower than high. An internal threat source with high potential, for example, IT system developer, usually should not be considered as relevant and probable due to government control through accreditation and certification;
2. The educational environment should store personal data for a long time, including biometric personal data. The stored data can contain both the general information about the hobby, exam results, education profile, and the biometric data of authentication and proctoring services. According to the lifelong education concept, a person can use SEE approximately from five to seventy years. The SEE stores these personal data to not only grant access or prove education status but also to create and adjust individual educational trajectories. Analysis of these data can disclose different information about personal political opinions, cultural or social identity, biometric data, and so on;
3. The educational environment should provide communication between the internet users and the laboratory equipment in a university or school. This communication is necessary to both socialization and education in engineering programs. Thus, a lot of computing devices will be outside any controlled zone, if SEE will use the traditional policy of zone-based security. As a result, the bring-your-own-device (BYOD) concept increases both the attack surface and the SEE weakness against malware or social engineering attacks;
4. The educational environment should ensure the privacy of students in conditions where artificial intelligence algorithms and big data are used. The AI technology inevitably will be implemented to decrease the number of teacher tasks, and increase the efficiency of the educational system. AI-based solutions also can help in tasks such as learning outcomes assessment, development of individual education trajectory, or assistance in education program or course.
5. The crowd control system in smart campuses should identify potentially dangerous people, including terrorists, with a minimum probability of false-positive events. For the last twenty years, researchers and journalists document more than 240 individual terrorist attacks in schools around the world. Only in the USA were documented 154 incidents in 2013–2015 years [23]. Therefore, this risk should be considered as actual for smart campuses.
The mix of real and virtual space (or deep penetration of digital technologies into reality) causes the impossibility to process risks through avoidance or share. The complexity of IT systems in education does not allow singling out the only entity that will be responsible for risk processing. For an attacker, the entry point into the SEE can be located in a variety of places like students’ or teacher Personal Computers (PC), mobile devices, educational environment servers, course components, web service, and so on.
Following the concept of lifelong learning, the educational system must accompany a person almost throughout his life, especially intensively in the first 25 years [29]. Digital profiles of the student collect all achievements and personal data for this task. The source of the threat to digital profiles can be Cyber Crime (CC) groups, insiders with access to the database, and competitors.
In SEE case, social networking is a platform to support longtime communications between professional groups. Therefore, malefactors can collect users’ data on the platform to apply Open-Source Intelligence (OSINT) methods and Advanced Persistent Threats (APT). Possible threat sources are cybercrime groups, fraudsters, cyber intelligence services, competitors.
The third-party authentication and authorization protocols are common solutions in a distributed online education environment. Perhaps it is the only possible solution. That is why communication channels should have high-level security to avoid Man-in-the-Middle (MitM) attacks or other threats related with information modification. It is also true for remote access protocols, especially in the case of laboratory equipment. Threat sources are insiders, script-kiddy, and CK-groups.
The wide dissemination of AI as assistants on the course leads to the transfer of responsibility for the educational trajectory from a human (teacher) to AI assistant. Malefactors potentially can manipulate human choices through AI. Threat sources are insiders with access to the database, CC-groups, and competitors.
3.1.The evaluation of threat sources potential
To evaluate the potential of the threat source, the Open Web Application Security Project (OWASP) risk-rating methodology will be used [34]. Insiders and competitors with the highest skills can be system administrators or students in computer science. Thus, competitors and insiders skill level (6) are equal to advanced users. CC groups can exploit 0-Day vulnerabilities and create special malware programs, so their skill level is much higher (9). Motivation is set in the range from possible reward (4) to probably high reward (7). CC motivation is set (7) because CC group can convert stolen data and computing resources into money or use it in the next steps of APT. The opportunity parameter for CC groups and insiders is set to (7) as these threat sources need some access or resources to find vulnerabilities. The competitors are usually not part of the SEE, thus, special access or resources to find vulnerabilities in SEE are need. The size of threat sources is set in range
Table 1
The evaluation of threat source potential
Type | Skill level | Motive | Opportunity | Size | Overall | Average | Result |
CC groups | 9 | 7 | 7 | 9 | 32 | 8 | high |
Competitors | 6 | 6 | 4 | 7 | 23 | 5.75 | medium |
Insiders | 6 | 5 | 7 | 6 | 24 | 6 | medium |
Script-kiddy | 5 | 4 | 5 | 7 | 21 | 5.25 | medium |
Cybercrime groups look like the greatest threat source. Moreover, the educational environment provides access to its services for a wide range of people within the concept of life-long (width) education. Thus, the CC groups can start an APT attack as insiders. Accordingly, the assessment of the opportunity of CC groups should be no lower than the internal threat source.
3.2.The estimation of security and privacy risks in smart educational environments
Probable attack surfaces and entry points for a SEE:
1. Authentication and authorization services – public key infrastructures, trusted third-party authentication services like single-sign-on, Open Authorization (OAuth) protocol, and others.
2. Network infrastructure in internet zone – routers and routing protocols, remote admin tools and protocols, public Domain Name System (DNS) servers.
3. Client computers – phishing through e-mail or social media, malware in public software sharing platforms, malware in social media content.
4. Public services of the educational environment – public interfaces of web applications, admin interfaces of web applications.
The SEE assets was identified above in section «Smart Educational Environments». The main threats and threat sources are identified above. The estimation of protective measures and vulnerabilities is possible only within the framework of SEE system implementation. Thus, the analysis will skip this stage. In conclusion, it is necessary to estimate the level of impact from the incident. The different risk assessment frameworks consider only part of the criteria – economics, ecology, or reputation. However, SEE as part of a smart city should consider all main categories and criteria of impacts. The generalized classification of impacts according to NIST 800-82, 800-30, and ISO/IEC 27005 standards are present in Table 2, but it can be specific for different SEE:
Table 2
The estimation criteria the level of impacts from information security incidents
Criteria | Indicator | Impact levels | ||
Low | Medium | High | ||
Economic | Financial loss | <1000$ | <100000$ | >100000$ |
Interruption of production | Minutes | Days | Weeks | |
Social | People affected | <100 | <10000 | >10000 |
Ecological | Environmental Release | Short-term/one-time | Prolonged | Permanent |
Political | Crisis scale | Local | Regional | Global |
Reputation | Temporal persistence | Temporary damage | Long-term damage | Permanent damage |
Injury | Physical | Cuts, bruises requiring first aid | Requires hospitalization | Loss of life or limb |
The risk estimation for the identified threats is based on threat source with maximal potential. In probability estimation, the general classification for IT systems (ISO) will be used, where high probability is equal to range (10:100) times per year, the medium is equal to range (1:10) per year, and low is equal to less than one time per year. Table 3 does not present a complete list of threats, but the set of threats specific to a SEE.
Table 3
The estimation of security and privacy risks for a smart educational environment
Risk | Threat | Probability | Impact |
Inadequate risk assessment for services of smart campus | Insufficient security controls | Medium | Medium |
Excessive security spending | |||
The leakage of students profile and biometric database | Authentication secret disclosure | High | High |
Session token theft | Medium | High | |
Database access control policy violations | Medium | High | |
Leakage of personal data, including biometric data stored in authentication systems | Medium | High | |
Modification of the machine learning algorithm parameters | Manipulating training data set | Medium | High |
Poisoning the machine-learning model through error correction mechanisms | High | High | |
Replicating training sample data through the operation of the I/O interface | Medium | High | |
Violation of service’s security by external users | Leakage of author’s educational content | Medium | Medium |
Violation of service’s security by internal users | Modification of tests information in LMS | Medium | Medium |
Leakage of answers to educational tasks and tests | Medium | Medium | |
Denial of educational environment services | Medium | Medium | |
Blocking/destroying cyber-physical laboratory equipment | Unauthorized access to control services of laboratory equipment | High | High |
Blocking access during the work, which leads to a violation of the control process | High | High | |
Violation of student’s privacy | Unauthorized access to the multimedia equipment of the student’s computer through the proctoring service | Medium | High |
Destruction of social trust in the education system | The manipulating humans’ behavior through AI technologies including discrimination, overconfidence, error propagation, and so on. | Medium | High |
The abuse of service’s monopoly while organizing the access to knowledge and skills | Medium | High |
Thus, serious risks were raised with two technologies – biometric authentication and artificial intelligence.
3.3.The key technologies to mitigate security and privacy risks in smart environments
Biometrics as an authentication factor has serious risks associated with the physical security of the person and the consequences of disclosure. It is easy to fix disclosure consequences for factors such as knowledge (password) or possession (phone). However, what to do if fingerprint or other biometric data are compromised? In this regard, the development of authentication technologies based on the zero-knowledge protocol is important to reduce risks related with an authentication system in SEE [16]. Nowadays, blockchain and cryptocurrency technologies are actively using principles of zero-knowledge proofs [20]. These methods are important not only for SEE but also in smart city services, especially when using biometric data [13,25].
The next question is when and why should robots substitute humans in education? In several cases, AI-based assessments of personal educational programs or skills are useful. In another case, studying a subject just because your case does not fit into the AI model can decrease the effectiveness of the whole education system, not only individuals’ learning process.
The vulnerabilities of machine learning algorithms to various kinds of attacks were described in articles [4,5,10,19,22,37]. People can do mistakes, but a robot will always mistake if its program was corrupted. If the training data set was poisoned or an improper AI algorithm used, an objective machine can deceive the people due to they do not know how this concrete AI operates. The AI technologies’ key threats are focusing around manipulating training data set, poisoning the machine-learning model through error correction mechanisms, and replicating training sample data through using the I/O interface as shown in Fig. 5.
Fig. 5.
The attack vector on AI-model.
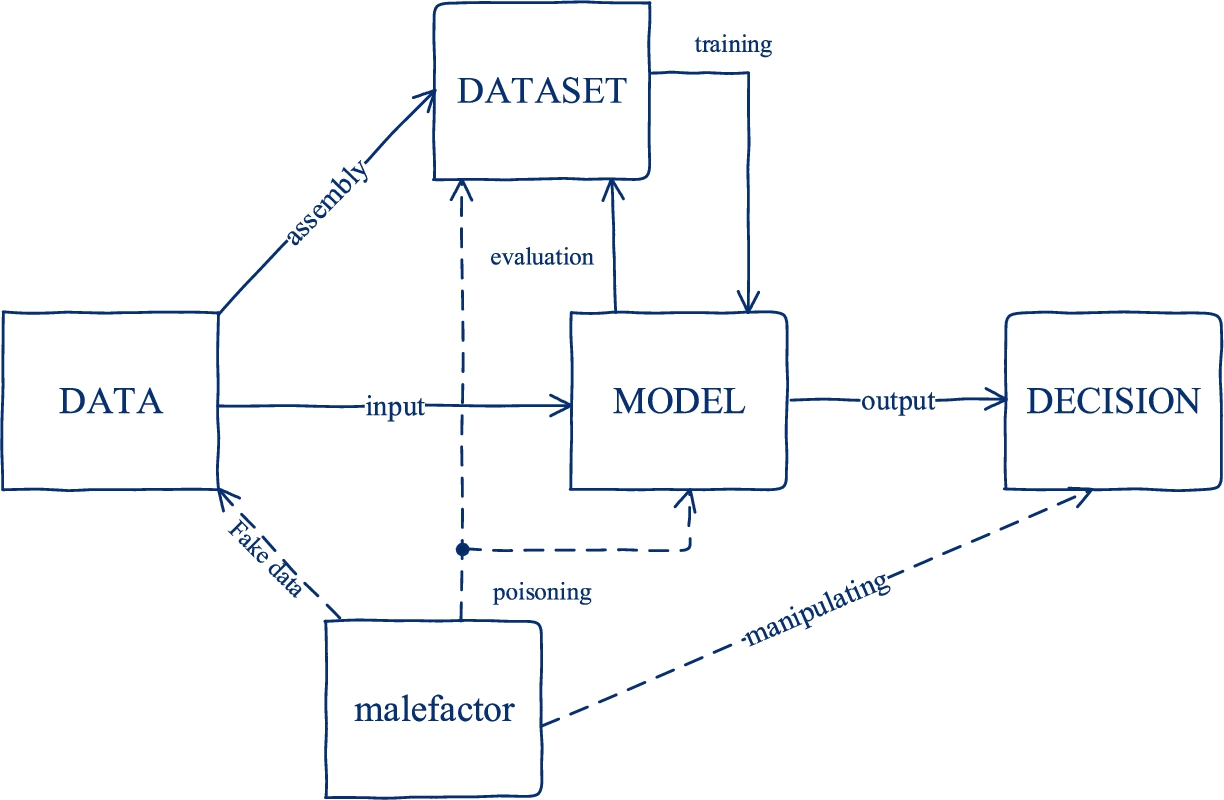
The threats associated with the usage of AI forces countries to implement legislative regulation of the conditions and rules for its use in various services. In the recently published document [9], European Union plans to limit the usage of AI depending on the area of its application. The document assesses various risks that can be applied to SEE. The four main categories of risk according to this EU document:
Unacceptable risk includes AI systems or applications that manipulate human behavior to circumvent users’ free will.
High-risk includes educational or vocational training that may determine the access to education and professional course of someone’s life (e.g. scoring of exams).
Limited risk, when using AI systems such as chatbots, users should be aware that they are interacting with a machine, so they can take an informed decision to continue or step back.
Minimal risk allows the free use of applications such as AI-enabled video games or spam filters.
In conclusion, let us to outline and shortly discuss the key technologies in the field of security and privacy, which development is necessary for the mitigation of the risks identified above and the sustainable operation of any SEE:
1. Technologies for storing and processing biometric personal data – morphological biometrics and behavioral biometrics in proctoring and other services should be secured properly from spoofing and stealing. The integrity of data can be provided by a set of various methods as hash-digest or steganography. The major question is how to generate a unique biometric data sample for each use case? If the stolen data are useless in another service, it will reduce the profit as well as the attraction of an attack for hackers.
2. Technologies for transparent and controlled AI implementation in education – the AI-based tools in the crowd control systems such as the pattern recognition and the social scoring systems can help to detect potentially dangerous people. However, any mistake in a decision can ruin social trust in these systems. People should always know who gives them advice or assessment – human or robot. The securing AI technologies important not only as technical challenge but also as a response to ethical challenges. It will be helpful to have common criteria for AI-based IT systems because it includes not only functional security requirements but also security assurance. If AI can show how it receives a solution, it partially will solve the problem. However, it arises great privacy challenges in SEE because can cause the leakage of private data from the training data set.
3. Risk management technologies not based on expert assessment – in Table 2 the criteria for impact assessment were given, but is it a suitable for concrete Smart City? All current risk management frameworks involve experts’ decisions to receive assessment in impacts, probability, and other parameters. The risk management system is usually improved by n-of-m voting, but it protects only from m minus n mistakes. For example, the 2-of-3 system protects from only one mistake. In reliability theory, this problem is known as observation for the observer. Security specialists are facing the same problem in Public Key Infrastructures (PKI) with the chain of trust and root certification authorities. The development of risks assessment systems not based on an experts’ decisions can help us to look at the risk from the one or close points of view and mitigate this risk.
4. Authentication technologies in distributed and decentralized systems, including the development of authentication methods based on zero-knowledge proof – the best way not to lose a secret is never know it. Storing sensitive data on one server or moreover providing a single entry service for all SEE services are high risk. In an ideal zero-knowledge-proof system, the server does not store any sensitive data. The distributed registry like blockchain help to mitigate risks of trust chain and bottleneck. The problem of a Trusted Third Party (TTP) does not appear if data about pair {public key (authenticator), identity} stores in blockchain [47]. However, blockchain has several problems like well known 51% attack. In addition, it not only complicates spoofing attacks but also increases costs to revoke, delete or replace identities. Nevertheless, the threat source should compromise the majority of nodes in the blockchain system to spoof identity or provide another MitM-attack. In this case, an attack on the system can be too expensive for the threat sources with medium sizes and resources.
5. Secure architecture technologies based on zero-trust methodology – the distributed environments are weak for such attack techniques as social engineering or supply chain compromise. All these techniques are effective against zone-based security systems since entry points are in a trusted zone. The concept of zero-trust security architecture is based on the authentication and authorization independence from zone trust level [26]. The source should receive authorization for each action depending on trust level that can be increased with authentication and regardless from belonging to the zone. The article [32] proposes the access control system based on zero-trust for smart campus networks. The further development of the zero-trust methodology looks like a necessary solution to improve the architecture of all smart city services and the SEE as particular case.
6. The computing and communication based on quantum technologies – the Quantum Computers (QC) are potentially can decrease efficiency of authentication and encryption algorithms used. However, the current QC is far from solving this task. For example, QC needs ten thousands qubits to decrypt the 2048 bits RSA key during one year [17] or 20 million qubits to solve this task during 8 hours [14]. Whereas, the best QC simulations reaches only 256 qubits [8]. The communication system based on QC can provide alternative solution to the TTP problem. Therefore, building network based on QC technology for critical services of Smart City can significantly increase information security level. Although, it can be very expensive and should be considered as alternative solution in comparison to existing technologies with proved level of security.
4.Conclusions
The development of any technology involves the implementation of safety mechanisms, for example, modern civil aviation is not possible without strict flight safety protocols and policies. Smart city information technologies are affecting the interests of citizens, which means the developers should take into account the risks associated with privacy and personal data.
Modern educational platforms as part of smart city services use virtual and augmented reality technologies. Thus, the boundaries of privacy, the rights to anonymity and oblivion, and other privacy factors have to be determined in SEE. The smart educational environment stores confidential data that can be interesting for different threat sources. Moreover, denial of service attacks can destroy laboratory equipment, online classrooms, and other elements of the education environment since the system grants remote access to these objects. That is why the developers should take into account both the privacy and the information security issues. The analysis shows that the usage of biometric data and artificial intelligence in different education technologies causes the most dangerous risks.
In addition, there are issues of artificial intelligence ethics. For example, the applicability of artificial intelligence as personal assistants to teachers. On the one hand, big data analysis allows creating algorithms that are more aware of our preferences and desires; on the other hand, the algorithm does not have moral responsibility for its advice, unlike the teacher, it does not know what is kind or worth. Moreover, there are risks of manipulating the AI training samples to impose a students’ choice. At last, how to use personal data in the training of AI? GDPR gives a person the right to be forgotten, but how can it be implemented in an AI system that is already trained and operates? This question needs a systematic discussion [8,39].
The implementation of the zero-trust, the zero-knowledge proof, and the quantum computing technologies significantly improves security and privacy in SEE. Nevertheless, it is not enough to sustainability of SEE. Further development of technologies for risk management, storing and processing biometric and other sensitive data, and the managing AI security is need.
Acknowledgements
The author would like to thank the reviewers for their useful feedback that helped to make the paper better.
Conflict of interest
None to report.
References
[1] | A. Al-Ajlan and H. Zedan, Why moodle, in: 2008 12th IEEE International Workshop on Future Trends of Distributed Computing Systems, IEEE, (2008) , pp. 58–64. doi:10.1109/FTDCS.2008.22. |
[2] | J.C. Augusto, Ambient intelligence: Opportunities and consequences of its use in smart classrooms, Innovation in Teaching and Learning in Information and Computer Sciences 8: (2) ((2009) ), 53–63. doi:10.11120/ital.2009.08020053. |
[3] | R. Barnett, Lifewide education: A new and transformative concept for higher education, Learning for a complex world: A lifewide concept of learning, education and personal development, 2011, pp. 22–38. |
[4] | M. Barreno et al., Can machine learning be secure?, in: Proceedings of the 2006 ACM Symposium on Information, Computer and Communications Security, (2006) , pp. 16–25. doi:10.1145/1128817.1128824. |
[5] | B. Biggio et al., Evasion attacks against machine learning at test time, in: Joint European Conference on Machine Learning and Knowledge Discovery in Databases, Springer, Berlin, Heidelberg, (2013) , pp. 387–402. |
[6] | .J. Bishop and M.A. Verleger, The flipped classroom: A survey of the research, in: 2013 ASEE Annual Conference & Exposition, pp. 1–18. |
[7] | M. Dollinger, J. Lodge and H. Coates, Co-creation in higher education: Towards a conceptual model, Journal of Marketing for Higher Education 28: (2) ((2018) ), 210–231. doi:10.1080/08841241.2018.1466756. |
[8] | S. Ebadi et al., Quantum phases of matter on a 256-atom programmable quantum simulator, Nature 595: (7866) ((2021) ), 227–232. doi:10.1038/s41586-021-03582-4. |
[9] | Europe fit for the Digital Age: Commission proposes new rules and actions for excellence and trust in Artificial Intelligence [Electronic resources] URL: https://ec.europa.eu/commission/presscorner/detail/en/ip_21_1682 (accessed, 19.05.2021). |
[10] | K. Eykholt et al., Robust physical-world attacks on deep learning visual classification, in: Proceedings of the IEEE Conference on Computer Vision and Pattern Recognition, (2018) , pp. 1625–1634. |
[11] | G. Fischer, Lifelong learning – more than training, Journal of Interactive Learning Research 11: (3) ((2000) ), 265–294. |
[12] | A. Fox et al., Software engineering curriculum technology transfer: lessons learned from MOOCs and SPOCs, UC Berkeley EECS Technical Report, 2014. |
[13] | D. Gabay, K. Akkaya and M. Cebe, Privacy-preserving authentication scheme for connected electric vehicles using blockchain and zero knowledge proofs, in: IEEE Transactions on Vehicular Technology, Vol. 69: , (2020) , pp. 5760–5772. |
[14] | C. Gidney and M. Ekerå, How to factor 2048 bit RSA integers in 8 hours using 20 million noisy qubits, Quantum 5: ((2021) ), 433. doi:10.22331/q-2021-04-15-433. |
[15] | A.K. Goel, L. Polepeddi and J. Watson, A virtual teaching assistant for online education, in: Learning Engineering for Online Education, Routledge, (2018) , pp. 120–143. doi:10.4324/9781351186193-7. |
[16] | S. Goldwasser, S. Micali and C. Rackoff, The knowledge complexity of interactive proof systems, SIAM Journal on Computing 18: (1) ((1989) ), 186–208. doi:10.1137/0218012. |
[17] | É. Gouzien and N. Sangouard, Factoring 2048-bit RSA integers in 177 days with 13436 qubits and a multimode memory, Physical Review Letters, American Physical Society 127: (14) ((2021) ). doi:10.1103/PhysRevLett.127.140503. |
[18] | Gross enrollment ratio in tertiary education, 1971 to 2014 [Electronic resources] URL: https://ourworldindata.org/grapher/gross-enrollment-ratio-in-tertiary-education (accessed 13.03.2021). |
[19] | K. Grosse et al., Adversarial perturbations against deep neural networks for malware classification, in: European Symposium on Research in Computer Security, (2017) . |
[20] | J. Groth et al., Updatable and universal common reference strings with applications to zk-SNARKs, in: Annual International Cryptology Conference, Springer, Cham, (2018) , pp. 698–728. |
[21] | G.J. Hwang et al., Vision, challenges, roles and research issues of artificial intelligence, in: Education, in Computers and Education: Artificial Intelligence, Vol. 1: , (2020) , pp. 1–5. |
[22] | M. Jagielski et al., Manipulating machine learning: Poisoning attacks and countermeasures for regression learning, in: 2018 IEEE Symposium on Security and Privacy (SP), IEEE, (2018) , pp. 19–35. doi:10.1109/SP.2018.00057. |
[23] | B. Kalesan et al., School shootings during 2013–2015 in the USA, Injury prevention 23: (5) ((2017) ), 321–327. doi:10.1136/injuryprev-2016-042162. |
[24] | M. Khalil, M. Ebner and W. Admiraal, How can gamification improve MOOC student engagement, in: Proceedings of the 11th European Conference on Games Based Learning: ECGBL, (2017) , pp. 819–828. |
[25] | H. Kikuchi et al., Privacy-preserving similarity evaluation and application to remote biometrics authentication, in: Soft Computing, Vol. 14: , (2010) , pp. 529–536. |
[26] | J. Kindervag, Build security into your network’s dna: The zero trust network architecture, Forrester Research Inc, pp. 1–26. |
[27] | R. Kop, The challenges to connectivist learning on open online networks: Learning experiences during a massive open online course, in: International Review of Research in Open and Distributed Learning, Vol. 12: , (2001) , pp. 19–38. |
[28] | S.R. Kurupathi and W. Maass, Survey on federated learning towards privacy preserving AI. DFKI LogoDeutsches Forschungszentrum für Künstliche Intelligenz, German Research Center for Artificial Intelligence. |
[29] | K.H. Kyritsi et al., The pursuit of patterns in educational data mining as a threat to student privacy, Journal of Interactive Media in Education 1: ((2019) ), 1–10. doi:10.5334/jime.502. |
[30] | C.S. Li and B. Irby, An overview of online education: Attractiveness, benefits, challenges, concerns and recommendations, College Student Journal 42: (2) ((2008) ). |
[31] | J. Looyestyn et al., Does gamification increase engagement with online programs? A systematic review, PloS one 12: (3) ((2017) ), e0173403. |
[32] | T. Lukaseder et al., Context-Based Access Control and Trust Scores in Zero Trust Campus Networks, SICHERHEIT, (2020) , pp. 53–66. |
[33] | J. Meszaros and C.H. Ho, AI research and data protection: Can the same rules apply for commercial and academic research under the GDPR?, Computer Law & Security Review 41: ((2021) ), 105532. doi:10.1016/j.clsr.2021.105532. |
[34] | M. Meucci and A. Muller, OWASP Testing Guide V. 4.0, Open Web Application Security Project, 30. |
[35] | C.E. Mora-Beltrán, A.E. Rojas and C. Mejía-Moncayo, An immersive experience in the virtual 3D VirBELA environment for leadership development in undergraduate students during the COVID-19 quarantine, Learning 6: ((2020) ), 43–52. |
[36] | R.T. Osguthorpe and C.R. Graham, Blended learning environments: Definitions and directions, Quarterly review of distance education 4: (3) ((2021) ). Retrieved October 11, 2021 from https://www.learntechlib.org/p/97576/. |
[37] | N. Papernot et al., Practical black-box attacks against machine learning, in: Proceedings of the 2017 ACM on Asia Conference on Computer and Communications Security, (2017) , pp. 506–519. doi:10.1145/3052973.3053009. |
[38] | R. Reyes et al., Methodology for the implementation of virtual assistants for education using Google dialogflow, in: Mexican International Conference on Artificial Intelligence, Springer, Cham, (2019) , pp. 440–451. |
[39] | S. Sajeed et al., An approach for security evaluation and certification of a complete quantum communication system, Scientific Reports 11: (1) ((2021) ), 1–16. |
[40] | O. Scharmer, MOOC 4.0: The next revolution in learning & leadership, in Huffington Post [Web Periodical], 2015, Retrieved, 15. |
[41] | U. Schimank and M. Winnes, Beyond Humboldt? The relationship between teaching and research in European university systems, Science and Public Policy 27: (6) ((2000) ), 397–408. doi:10.3152/147154300781781733. |
[42] | G. Siemens, Connectivism: A learning theory for the digital age. International Journal of Instructional Technology & Distance Learning, 2005, [Electronic resources] URL: http://www.itdl.org/Journal/Jan_05/article01.htm. |
[43] | A.M. Toda, P.H. Valle and S. Isotani, The dark side of gamification: An overview of negative effects of gamification in education, in: Researcher Links Workshop: Higher Education for All, Springer, Cham, (2017) , pp. 143–156. |
[44] | R. Van Roy and B. Zaman, Need-supporting gamification in education: An assessment of motivational effects over time, Computers & Education 127: ((2018) ), 283–297. doi:10.1016/j.compedu.2018.08.018. |
[45] | S.W. Volkow and A.C. Howland, The case for mixed reality to improve performance, Performance Improvement 57: (4) ((2018) ), 29–37. doi:10.1002/pfi.21777. |
[46] | D. Waller et al., The HIVE: A huge immersive virtual environment for research in spatial cognition, Behavior Research Methods 39: (4) ((2007) ), 835–843. doi:10.3758/BF03192976. |
[47] | A. Yakubov et al., A blockchain-based pki management framework. In The First IEEE/IFIP International Workshop on Managing and Managed by Blockchain (Man2Block) colocated with IEEE/IFIP NOMS 2018, Tapei, pp. 1–6. |
[48] | Z. Zhang et al., A virtual laboratory system with biometric authentication and remote proctoring based on facial recognition, in: Computers in Education Journal, Vol. 7: , (2016) , pp. 74–84. |