The Associations Among Gut Microbiota, Branched Chain Amino Acids, and Parkinson’s Disease: Mendelian Randomization Study
Abstract
Background:
In experimental and observational studies, the characteristics of gut microbiota have been associated with Parkinson’s disease (PD), among which metabolic pathways played an important role. However, the causality remained unclear.
Objective:
Herein, we aimed to determine the potential impact of gut microbiota and gut microbiota-derived metabolites on PD risk using a Mendelian randomization (MR) approach.
Methods:
We included as exposures gut microbial taxa abundance and gut-derived metabolites (branched chain amino acids [BCAAs]), with PD as the outcome. In addition, we explored whether BCAAs act as a mediating factor in the pathway from gut microbiota to PD.
Results:
We found evidence of a causality of 15 microbial taxa and PD before and after sensitivity analyses, but not after multiple testing correction. There was significant association between BCAAs levels and the risk of PD, especially isoleucine (OR = 0.995, 95% CI 0.992–0.999, p = 0.004, pFDR = 0.012). In addition, the causality of gut microbiota and BCAAs was also explored that the increased g_Coprococcus abundance can result in the decrease in isoleucine level (OR = 1.046; 95% CI, 1.009–1.085; p = 0.016).
Conclusions:
Our findings indicated suggestive association between gut microbiota and its metabolites and PD. Furthermore, higher BCAAs levels were associated with the decreased PD risk. This study may provide new targets for PD treatment, such as dietary BCAAs supplementation.
Plain Language Summary
Dysbiosis of gut microbiota and its metabolites (branched chain amino acids, BCAAs) appears to be a related risk factor for Parkinson’s disease (PD). Thus far, studies mostly focused on cross-sectional observational studies of gut microbiota and its metabolites, but this Mendelian randomization analysis evaluated the potential impact of gut microbiota and gut microbiota derived metabolites (BCAAs) on PD risk. Gut microbiota and BCAAs as exposures and PD as outcome, it was found that there was no significant correlation between gut microbiota and PD, while increasing levels of BCAAs, especially isoleucine, increased the risk of PD. In addition, we also demonstrated that BCAAs could play a role in PD relying on the gut microbiota. For example, an increase in g-Coprococcus abundance can lead to a decrease in isoleucine levels in PD. Therefore, in the future, the PD risk may be reduced by maintaining the homeostasis of gut microbiota and its metabolites.
INTRODUCTION
Parkinson’s disease (PD) is a multifactorial neurodegenerative disease, including genetic factors, environmental factors, and aging, etc.1 For example, previous genome-wide association studies (GWAS) have identified 90 independent genome-wide risk signals that can be used to explain 16–36% of the heritable risk of PD.2 Additionally, a large amount of evidence has indicated that the gut microbiota and gut microbiota-derived metabolites, including short chain fatty acids (SCFAs),3 branched chain amino acids (BCAAs),4 bile acids,5 and various lipid molecules,6 may play a core role in the gut-brain axis communication and the risk of neurodegenerative diseases. However, there are always inconsistent relationship between the gut microbiota and its derived metabolites and PD.
An increasing number of studies have indicated that the composition of gut microbiota in PD patients has changed, compared with healthy controls.7 However, these changes vary in different published studies. For example, Hasegawa and colleagues using quantitative RT-PCR of 16 S or 23 S rRNA found that in PD patients, the number of Lactobacillus was higher, while the abundances of Clostridium coccoides, and Bacteroides fragilis were lower than controls.8 Scheperjans et al. reported that the abundance of Prevotellaceae in feces of PD patients was reduced by 77.6% as compared with controls.9 Another study similarly found the abundance of Bacteroidetes and Prevotellaceae were reduced in fecal samples from PD patients compared to matched controls.10 Keshavarzian, et al. analysed the changes in bacterial genera Blautia, Coprococcus, and Roseburia were significantly lower abundant in PD patients, but the significant decrease in the abundance of Prevotellaceae was not observed.11 Furthermore, the role of the same gut microbiota in PD may be contradictory. For example, animal experiments and randomized controlled trials (RCTs) showed that Bifidobacterium is a protective factor for PD,12,13 while several case-control studies found a decrease in Bifidobacterium abundance in PD patients.14 BCAAs and SCFAs from gut microbiota-derived metabolites, as an important mediator between gut microbiota and PD, can also participate in the pathogenesis of PD through various mechanisms. However, the levels of BCAAs and SCFAs are also inconsistent in the quantitative detection results of PD patients, and even the opposite results.10,15–18 The participant selection bias, confounding bias, and reverse causation may induce these contradictory results. Therefore, the possible relationship between the gut microbiota and its metabolites (BCAAs and SCFAs) and PD is not yet clear.
Mendelian randomization (MR) provides a genetic epidemiological approach that uses genetic variation as instrumental variable to infer the causality between exposures (some biomarkers) and outcomes (various diseases).19,20 Meanwhile, MR can minimize conventional biases arising from reverse causation and confounding factors. Therefore, based on summarized data from the GWAS, the current study evaluated the genetic validity of gut microbiota and its metabolites in the risk of PD by using a two-sample MR method. Then we investigated whether BCAAs as mediators in the pathway from gut microbiota to PD. Study thought that host genetics can influence the human gut microbiota composition,14 thus it is plausible that risk genotypes for PD act in part through the gut microbiota and its metabolites.
MATERIALS AND METHODS
Study exposures
Gut microbiota: The human gut microbiome data (including 207 taxa) from 7738 participants were conducted by the Dutch Microbiome Project and summarized in the published GWAS by Lopera-Maya et al.21 The full GWAS summary statistical data for all 207 taxa can be directly downloaded at NHGRI-EBI GWAS Catalog (https://www.ebi.ac.uk/gwas/) under the study accession numbers GCST90027446-GCST90027857. The GWAS analysis adjusted age, sex, technical covariates, and genetic principal components. The sample of all cohorts came from European descent. As a reference database, SILVA was applied to characterize the gut microbiome profiles of the 16 S ribosomal RNA gene and genotyping data, with truncation of the taxonomic resolution to species level.
Gut microbiota-derived metabolites: Genetic instruments for the individual BCAAs (including leucine, isoleucine and valine) levels were obtained from the IEU Open GWAS project. The GWAS IDs are as follows: met-d-Leu, met-d-Ile and met-d-Val. The detailed information of the SNPs of above exposure and outcome data is shown in Table 1. The conceptual framework of MR analysis is presented in Fig. 1, including 1) the analysis of causal effects of gut microbiota on PD; 2) analysis of causal effects of BCAAs on PD; and 3) mediation analysis of BCAAs in the pathway from gut microbiota to PD.
Table 1
Detailed information of the studies and datasets used for MR analysis
Phenotype | Sample size (cases/controls) | Population | Consortium | Year | Author |
Gut microbiota | 7,738 | European | Dutch Microbiome Project | 2022 | Lopera-Maya EA |
Leucine | 115,074 | European | 2020 | Borges CM | |
Isoleucine | 115,075 | European | 2020 | Borges CM | |
Valine | 115,048 | European | 2020 | Borges CM | |
Parkinson’s disease | 2,005/359,194 | European | MRC-IEU | 2018 | Ben Elsworth |
Fig. 1
The overall design of MR analysis in the present study.
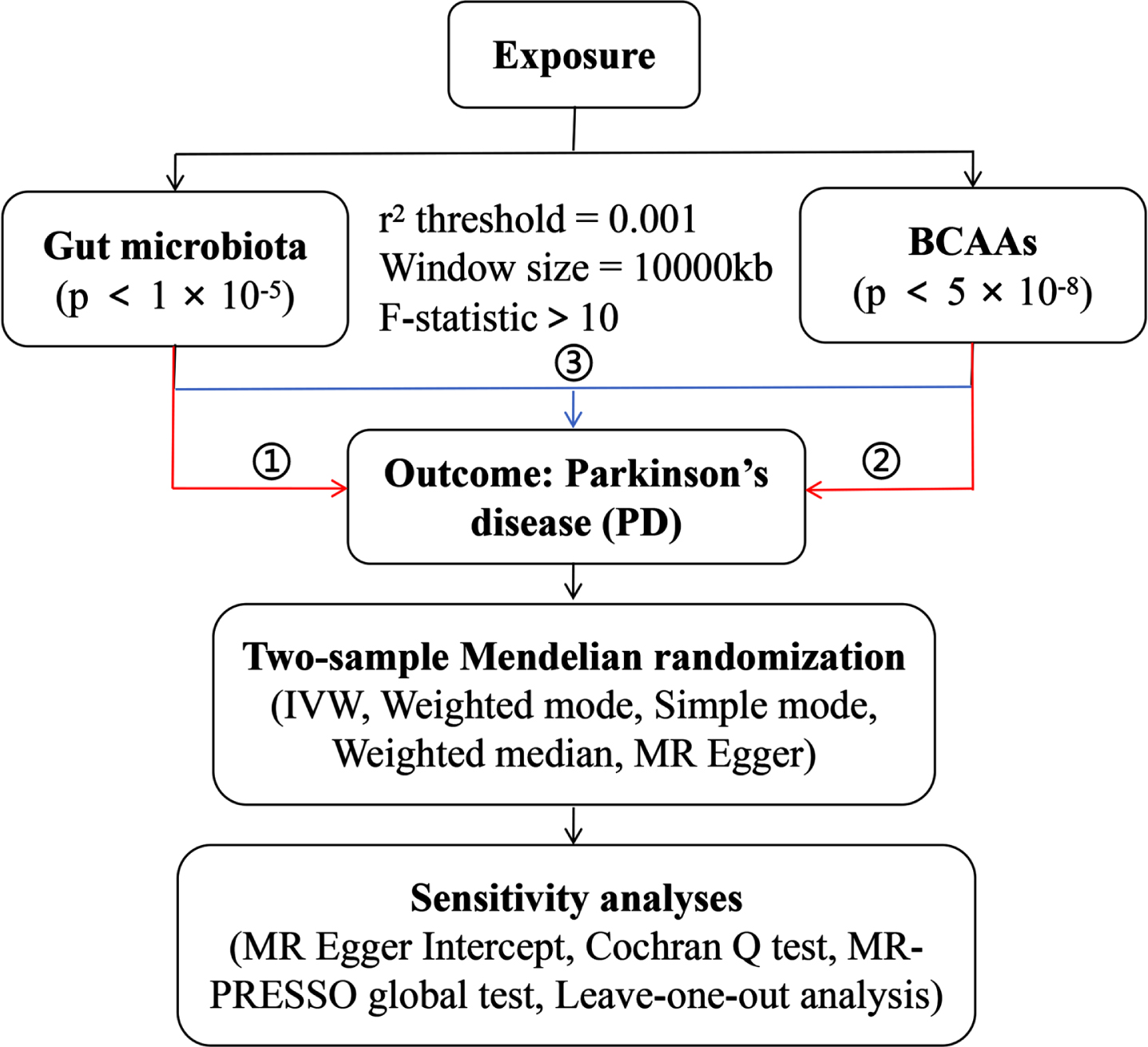
Study outcomes
The publicly available GWAS summary statistics for PD are also from the IEU Open GWAS database. The GWAS ID is ukb-b-16943, and this dataset contains 361,199 participants with European descent (359,194 controls and 2,005 cases) and 9,851,867 SNPs.
Selection of instrumental variables
In order to acquire the significant SNPs of instrumental variables (IVs), the suggestive genome-wide association threshold of the SNPs the intestinal microbiota and gut microbiota-derived metabolites were separately set as p < 1×10–5 and p < 5.0×10–8. To ensure the independence of IVs, we excluded variants for the linkage disequilibrium (LD) based on the default strict parameters (r2 = 0.001, kb = 10,000).22 Next, we extracted the same SNPs from the PD outcome GWAS. The SNPs that were absent in the outcome dataset were replaced by their corresponding proxy SNPs (r2 > 0.8) where available,23 and SNPs with minor allele frequency (MAF) ≤0.01 were removed. Exposure and outcome datasets were harmonized to ensure the effect alleles were aligned, and all palindromic SNPs were deleted. Additionally, the MR Steiger filtering was used to detect the causal direction of the extracted SNPs on exposures and outcomes.24 The IVs with ‘TRUE’ implied causality in the expected direction, whereas “FALSE” implied causality in the reverse direction. The possibility of reverse causality can be eliminated by excluding the IVs with “FALSE". After Steiger filtering, little evidence of reverse causation was detected (see Supplementary Tables 1 and 2). Given that IVs with an F-statistic less than 10 were considered weak instruments,25 we calculated the F-statistic to further determine the SNPs selected in this study met the MR assumptions. The SNPs selected rigorously as the final IVs were used for MR analysis.
Mendelian randomization analysis
We used multiple methods including MR Egger, Weighted median, Inverse variance weighted (IVW), Simple mode, and Weighted model to perform the two sample MR analysis on the association between the intestinal microbiota and its derived metabolites and PD. The IVW with multiplicative random effects is regarded as the primary method for causal inference. The false discovery rate (FDR), as the multiple hypothesis testing, was applied to correct the results of MR analysis, and the significance threshold was set at FDR-corrected p-values <0.05. Then, for associations with IVW-MR p-value <0.05, we performed sensitivity analyses, including the heterogeneity and pleiotropy test, to estimate the robustness of our primary causal estimate. The Cochrane’s Q-test was used for testing the heterogeneity among individual SNPs in MR analysis. The MR-Egger intercept test and MR pleiotropy residual sum and outlier (MR-PRESSO) were utilized to evaluate the presence of horizontal pleiotropy.26,27 Specifically, the MR-Egger intercept term estimated to be equal to zero implies the absence of horizontal pleiotropy. The MR-PRESSO analysis can minimize the impact of horizontal pleiotropy by eliminating notable outliers. Then, the leave-one-out test was conducted by systematically excluding each instrumental SNP one at a time to verify the robustness of results. All analyses were carried out using R software (version 4.3.2).
Mediation analysis
By the MR analysis (ding172 and ding173 in Fig. 1), gut microbiota and BCAAs with significant causality of PD were included in the mediation analysis. We explored whether gut microbiota had a causal relationship with BCAAs (ding174 in Fig. 1), and whether BCAAs were the mediation factors in the pathway from gut microbiota to PD.
RESULTS
Overview
The gut microbiota and its derived metabolites-related exposures were available for MR when the following conditions are satisfied simultaneously: 1) the genetic instruments are supposed to be independent (r2 < 0.001); 2) the genetic instruments should be strongly associated with the exposures (SNPs of gut microbiota: p < 1×10–5; SNPs of BCAAs: p < 5.0×10–8); 3) the weak genetic instruments should be sufficiently removed (F statistics >10). The harmonized dataset of the associations between genetic variant and exposure, and between genetic variant and outcome is presented in Supplementary Table 1.
Effect of gut microbial abundance on PD
We used the publicly available GWAS summary statistics to extract 96 SNPs of gut microbial taxa partly under genetic control (see Supplementary Table 1). We then performed the exposure-outcome tests by IVW-MR method, results reaching a threshold of p < 0.05 are presented in Supplementary Table 2. We found genetically increased abundance of s_Desulfovibrio_piger (OR, 0.998; 95% CI, 0.997–1.000; p = 0.036), s_Parabacteroides_unclassified (OR = 0.998; 95% CI, 0.997–1.000; p = 0.043), o_Bifidobacteriales (OR = 0.998; 95% CI, 0.997–1.000; p = 0.035), f_Bifidobacteriaceae (OR = 0.998; 95% CI, 0.997–1.000; p = 0.035), g_Bifidobacterium (OR = 0.998; 95% CI, 0.997–1.000; p = 0.029), s_Oxalobacter_formigenes (OR = 0.999; 95% CI, 0.997–1.000; p = 0.013), g_Oxalobacter (OR = 0.999; 95% CI, 0.998–1.000; p = 0.010), f_Oxalobacteraceae (OR = 0.999; 95% CI, 0.997–1.000; p = 0.013), f_Prevotellaceae (OR = 0.998; 95% CI, 0.996–1.000; p = 0.029), and s_Ruminococcus_lactaris (OR = 0.998; 95% CI, 0.997–1.000; p = 0.033) were potentially associated with a protective effect of PD, while genetically increased in g_Parasutterella (OR = 1.002; 95% CI, 1.000–1.002; p = 0.007), s_Parasutterella_excrementihominis (OR = 1.027; 95% CI, 1.006–1.048; p = 0.013), g_Coprococcus (OR = 1.001; 95% CI, 1.000–1.002; p = 0.012), g_Sutterella (OR = 1.001; 95% CI, 1.000–1.003; p = 0.044), and s_Sutterella_wadsworthensis (OR = 1.001; 95% CI, 1.000–1.003; p = 0.044) were related to higher risk of PD. However, there was no significant association after FDR correction for multiple testing. Simple mode, weighted mode, weighted median, and MR Egger offered similar results as the IVW approach (Supplementary Table 2). In the sensitivity analysis, MR-Egger intercept, MR-PRESSO and Cochrane’s Q-test proved no substantial evidence for heterogeneity and horizontal pleiotropy (all p values >0.05, Supplementary Table 3). Altogether, this analysis identified some microbes that may be associated with PD.
Effect of plasma BCAAs levels on PD
Under the IVW model, we observed the causal effects of leucine (OR = 0.997; 95% CI, 0.994–1.000; p = 0.032), isoleucine (OR = 0.995; 95% CI, 0.992–0.999; p = 0.004) and valine (OR = 0.997; 95% CI 0.995–1.000; p = 0.029) on the risk of PD (Table 2). After FDR correction of multiple tests, the significant association of isoleucine was remained. The association was almost consistent in sensitivity analysis using weighted median (Table 3). Cochran’s Q test indicated no heterogeneity (Q-value = 5.828, pQ = 0.443). Moreover, MR-PRESSO global test (p = 0.512) and MR-Egger intercept test (intercept = –0.0002, pintercept = 0.604) indicated no horizontal pleiotropy (Table 4). Then, we conducted a leave-one-out analysis to detect the SNPs conformity and generated forest plot (Fig. 2). Suggestive evidence indicates that the increase in plasma isoleucine was related to a lower risk of PD. As shown in Fig. 3a and 3b, the scatter plot and forest plot visually displayed the relationships between plasma isoleucine and PD risk. In addition, we drew the scatter plot, funnel plot and forest plot of leucine, isoleucine and valine all shown in Supplementary Figures 1–3.
Table 2
MR analysis by inverse variance weighted of BCAAs on PD
Exposure | nSNPs | Method | OR (95% CI) | p | pFDR |
Leucine | 9 | IVW | 0.997 (0.994 to 1.000) | 0.032 | 0.058 |
Isoleucine | 7 | IVW | 0.995 (0.992 to 0.999) | 0.004 | 0.013 |
Valine | 11 | IVW | 0.997 (0.995 to 1.000) | 0.029 | 0.058 |
CI, confidence interval; FDR, false discovery rate; IVW, inverse-variance weighted; nSNPs, number of single-nucleotide polymorphisms; OR, odds ratio.
Table 3
Sensitivity analysis of the associations between BCAAs and PD
Exposure | Weighted Median | MR-Egger | Simple mode | Weighted mode |
OR (95% CI) | OR (95% CI) | OR (95% CI) | OR (95% CI) | |
p | p | p | p | |
Leucine | 0.996 | 0.995 | 0.995 | 0.996 |
(0.993–1.000) | (0.989–1.002) | (0.989–1.001) | (0.992–1.000) | |
0.022 | 0.192 | 0.139 | 0.060 | |
Isoleucine | 0.995 | 0.992 | 0.995 | 0.995 |
(0.991–0.999) | (0.980–1.004) | (0.989–1.002) | (0.990–0.999) | |
0.024 | 0.254 | 0.220 | 0.071 | |
Valine | 0.997 | 0.996 | 0.998 | 0.997 |
(0.994–1.000) | (0.992–1.001) | (0.993–1.003) | (0.994–1.000) | |
0.022 | 0.168 | 0.391 | 0.058 |
CI, confidence interval; MR-PRESSO, Pleiotropy Residual Sum and Outlier; OR, odds ratio.
Table 4
Heterogeneity and horizontal pleiotropy analyses for the associations of BCAAs with PD
Exposure | Cochrane’s Q test | MR-Egger intercept test | MRPRESSO global test | Steiger Test | ||
Q-value | pQ | Intercept | pintercept | p | p | |
Leucine | 5.042 | 0.753 | 9.78e-05 | 0.562 | 0.757 | 2.25e-13 |
Isoleucine | 5.828 | 0.443 | 0.0002 | 0.604 | 0.512 | 2.25e-13 |
Valine | 5.470 | 0.858 | 5.66e-05 | 0.646 | 0.834 | 2.25e-13 |
MR-PRESSO, Pleiotropy Residual Sum and Outlier.
Fig. 2
Leave-one-out sensitivity analysis for leucine (a), isoleucine (b) and valine (c) on PD. PD, Parkinson’s disease.
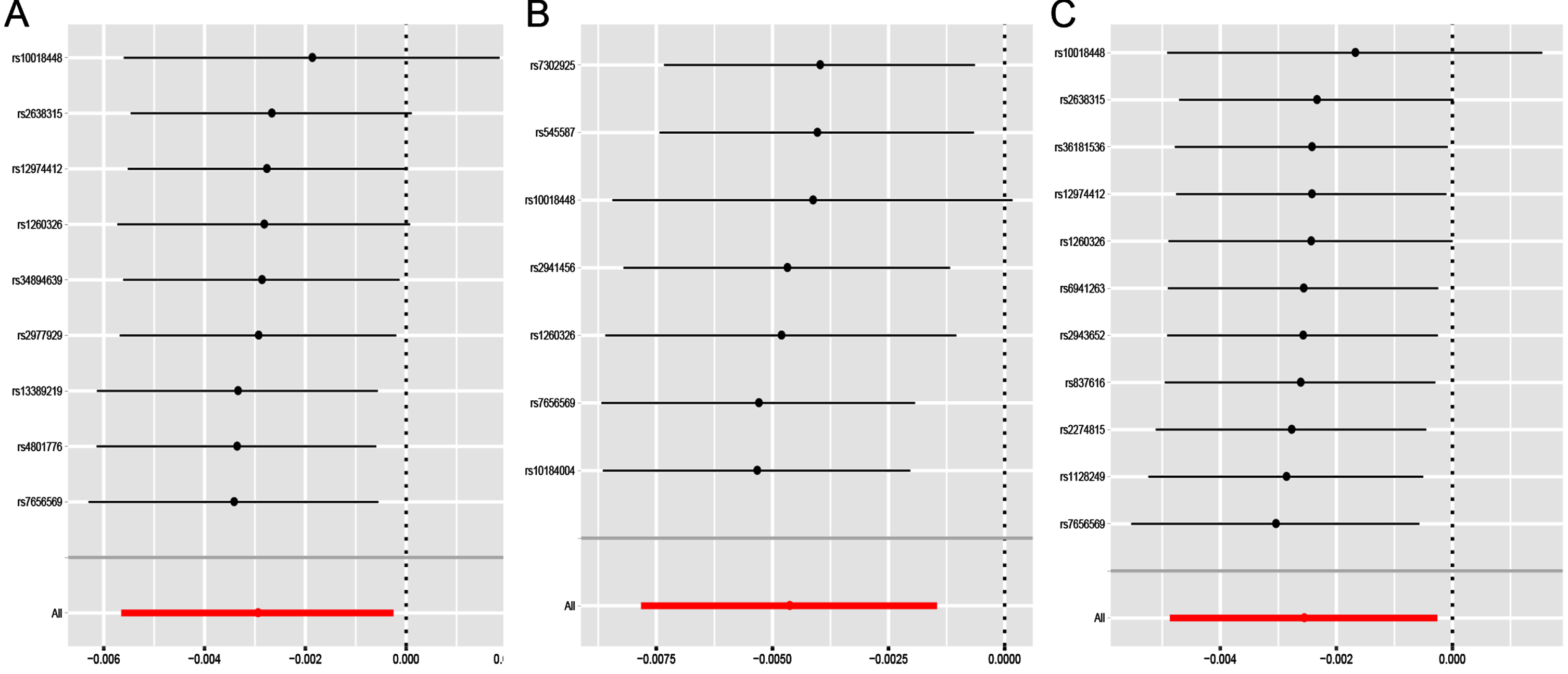
Fig. 3
Scatter plot (a) and forest plot (b) of the causal effect of isoleucine on PD risk. PD, Parkinson’s disease.
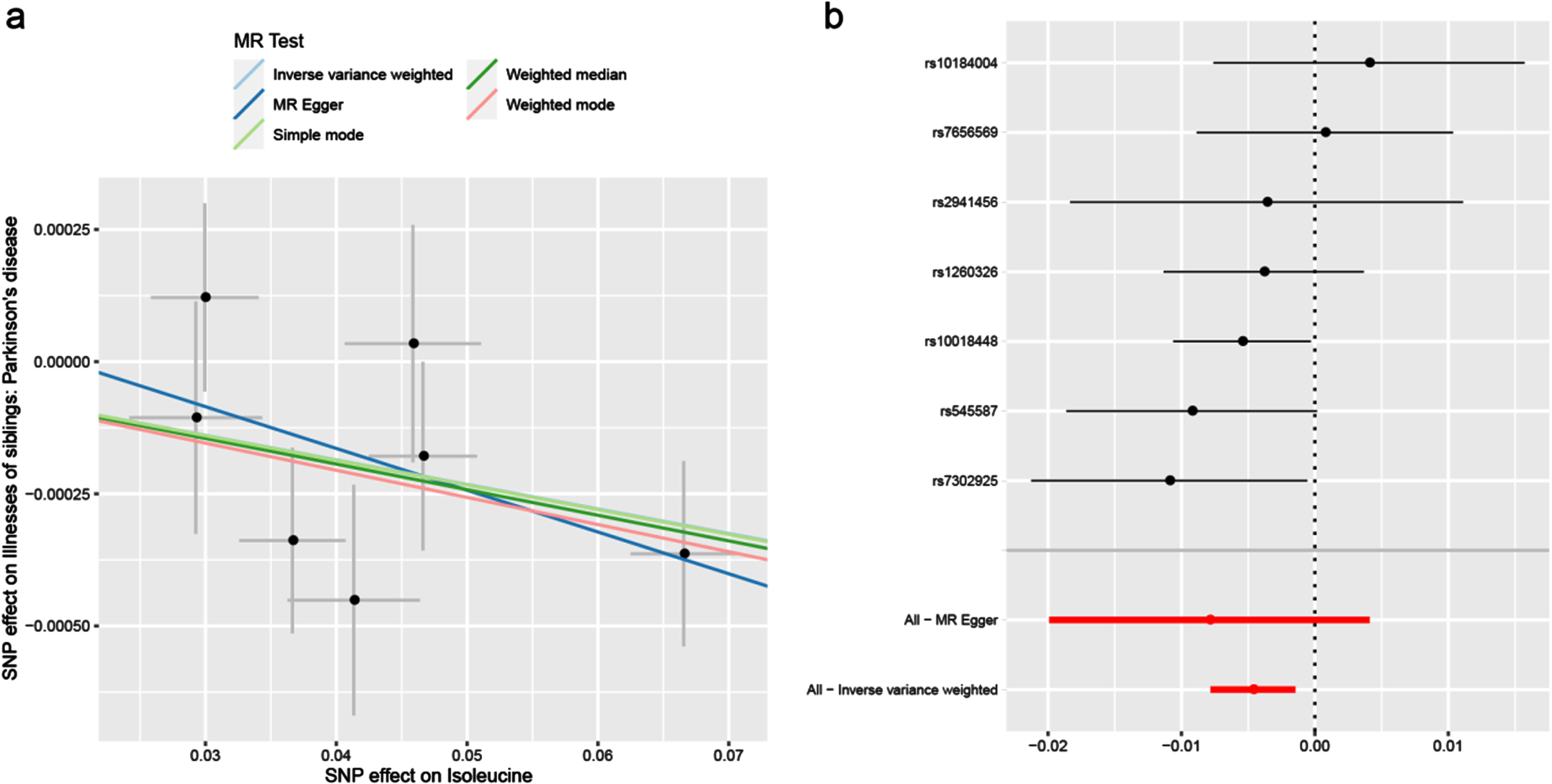
Mediation analysis
In this study, BCAAs rather than gut microbiota had causal effects on PD. We assumed that BCAAs played a mediating effect in the pathway from gut microbiota and PD. Correspondingly, we found that there was a significant relationship between g_Coprococcus associated with PD and isoleucine associated with PD (OR = 1.046; 95% CI, 1.009–1.085; p = 0.016). The heterogeneity and horizontal pleiotropy were validated by Cochran’s Q test (Q-value = 15.848, pQ = 0.104), MR-PRESSO global test (p = 0.135) and MR-Egger intercept test (intercept = –0.003, pintercept = 0.780). Finally, the scatter plot, funnel plot and forest plot were all showed in Fig. 4.
Fig. 4
The causal effect of isoleucine on g_Coprococcus. (a) Scatter plot, (b) Funnel plot and (c) Forest plot.
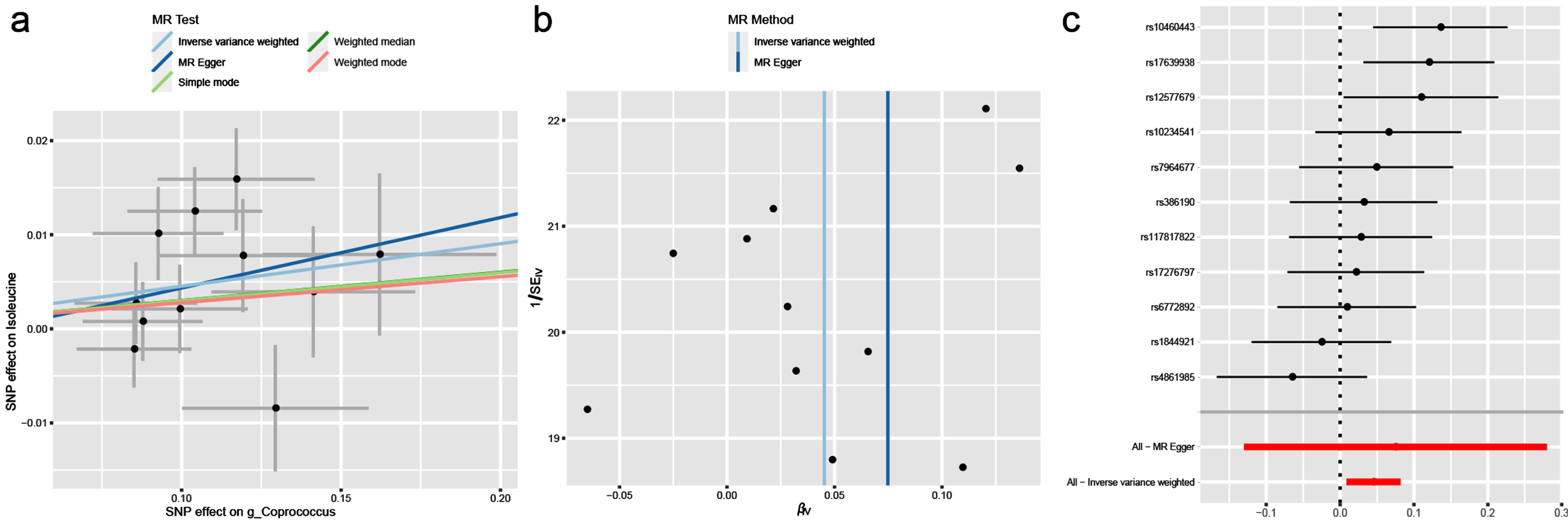
DISCUSSION
The current study is first to employ a two-sample MR method to examine the potential associations between gut microbiota and its derived metabolites (BCAAs) and PD. Firstly, we found that the increased g_Coprococcus abundance can result in the decrease in isoleucine level. Secondly, the elevated levels of BCAAs, especially isoleucine, can reduce the risk of PD. Interestingly, our previous research has also found that BCAAs supplementation contributes to improving dyskinesia, maintaining the gut microbiota homeostasis and reducing the inflammations in the brain in PD mice.28 Overall, we provided more evidence to support the impact of the gut microbiota and its metabolites dysbiosis on the pathogenesis of PD.
In our study, the genetically predicted s_Desulfovibrio_piger, s_Parabacteroides_unclassified, o_Bifidobacteriales, f_Bifidobacteriaceae, g_Bifidobacterium, s_Oxalobacter_formigenes, g_Oxalobacter, f_Oxalobacteraceae, f_Prevotellaceae, and s_Ruminococcus_lactaris are a protective factor for PD, while g_Parasutterella, s_Parasutterella_excrementihominis, g_Coprococcus, g_Sutterella, and s_Sutterella_wadsworthensis are a risk factor for PD. Previous observational studies have demonstrated some differences in microbial composition between PD patients and healthy individuals, but causality remains to be elucidated. Although fecal microbiome transfer (FMT) has been employed to establish the causal relationships between gut microbiota and health, the randomized control studies of FMT on humans can only be successfully replicated in the treatment of recurrent or refractory Clostridioides difficile infections and some ulcerative colitis in the current.29,30 Moreover, multiple confounding factors including antibiotic use, age, gender, diet, geography, etc. can interfere with the correlation between gut microbiota and PD in observational studies.31 In our study, we observed the genetically increased Bifidobacterium was related to the lower risk of PD, which supports previous observations in RCTs and animal studies that prolonged administration of probiotics could inhibit excessive activation of glial cells and neuroinflammation to protect dopaminergic neurons loss in the substantia nigra.32–34 On the contrary, a observational study found the abundance of Bifidobacterium increased in patients with PD, which may be attributed to an increase in levodopa dose or compensatory mechanisms to overcome gut microbial dysbiosis.35 Several researches observed the decreased of Prevotellaceae abundance in PD patients which can disturb the synthesis of neuroactive SCFAs.9,10 Consistent with the above researches, we found that Prevotellaceae is beneficial bacteria in reducing the risk of PD. In addition, we first provided evidence to support the association of the more abundance f_Oxalobacteraceae, g_Oxalobacter with lower PD risk. Recent observational study suggested that a decrease in Oxalobacter abundance in neurodegenerative diseases.36 Nevertheless, as the disadvantageous factors for PD, the inflammation-related bacterial genera Sutterella and Parasutterella was obviously increased in PD mice.37,38 These studies all demonstrated that aberrant composition of gut microbiota are associated with PD, and more importantly, gut microbiota can play a pathogenic or protective role in PD by regulating the microbial metabolites levels, but the specific mechanism by which the gut microbiota and its metabolites causes PD still needs to be elucidated.
Microbial metabolites are correlated with health and disease. In this study, we found that increasing levels of BCAAs can decrease the risk of PD, which is similar with another study cohort.39 Previous animal experiments and cross-sectional studies also supported the potential protective effect of BCAAs on PD.15,28 BCAAs, as nutritionally essential amino acids, are not only derived from abundant in most animal-based foods, but also could be synthesized by gut microbiota.40 Moreover, BCAAs are important signaling molecules that can regulate immune and inflammatory responses, mitochondrial biogenesis, and gut microbial reconstruction.41–43 Besides, research has found that the pforA gene expressed in the gut microbiota can mediate the degradation of BCAAs.44 The pyruvate: ferriredoxin oxidoreductase (PFOR), as a microbial enzyme (mainly encoded by the pforA gene), can convert pyruvate to acetyl CoA.45 Therefore, the acetyl CoA produced by BCAAs decomposition metabolism may be hydrolyzed by acetyl CoA hydrolase to acetate and further converted to butyrate, while succinyl CoA can produce propionate in the succinate pathway.46 Propionate can stimulate the enteroendocrine L cells to release GLP-1 which has clinical benefits in PD treatment.47–49 In addition, SCFAs, as bioactive metabolites produced by beneficial gut bacteria, also activates the peripheral nervous system to transmit its signals to the brain for communication with the gut-brain axis. The schematic diagram of the interaction among the gut microbiota, metabolites (BCAAs and SCFAs) is depicted in Fig. 5.
Fig. 5
The schematic diagram of BCAAs function in PD. The pforA gene of gut microbiota upregulate the expression of PFOR that promotes the conversion of BCAAs into SCFAs. Then, SCFAs stimulate the release of GLP-1 from enteroendocrine L cells, which plays a neuroprotective role to improve motor deficits and dopaminergic neuron loss. BCAAs, branched chain amino acids; GLP-1, glucagon-like peptide 1; PFOR, pyruvate: ferriredoxin oxidoreductase; SCFAs, short chain fatty acids.
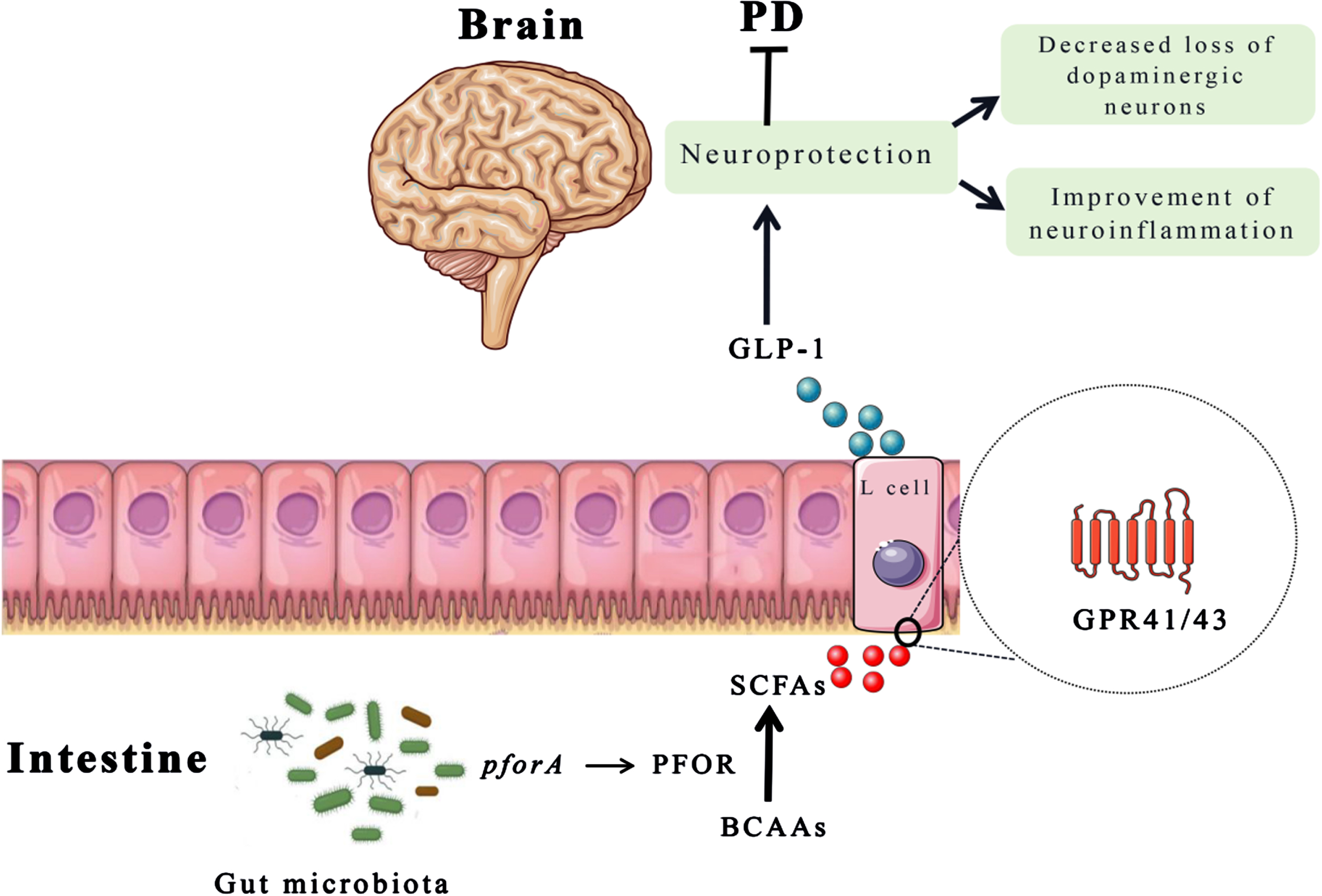
An important strength of this study is that we examined the possible connections between gut microbiota, metabolites (BCAAs) and PD, based on the largest publicly available GWAS datasets. Due to the random allocation and fixation of alleles at conception, biases caused by confounding and reverse causality are alleviated in an MR analysis.50 Inevitably, there are also some limitations in this study. Firstly, when the gut microbiota is used for an exposure factor, we included a loose cutoff of exposure-related SNPs at a threshold of p < 1×10–5, which may result in weak instrument bias affecting our findings. Secondly, metabolite-related SNPs as exposures have widespread pleiotropic effects. Although we systematically conducted multiple sensitivity analyses, it is extremely difficult to completely exclude the bias of pleiotropic variation on the results. Thirdly, our study is lack of knowledge of genetic variability of specific population and confounding factors, which we failed to comprehensively evaluate the correlation between individual genetic variation and potential confounding factors. Fourthly, the participants from GWAS data in the present study were of European descent, which limits the generalizability of our findings to other races. Finally, due to the complexity of PD pathogenesis, MR analysis cannot fully elucidate the exactly causal relationship between gut microbiota and its metabolites (BCAAs) and PD. Therefore, it is essential for greater experimental studies in the future to further complement the gaps in the literature on the linkages with gut microbiota, metabolites and PD.
In conclusion, our findings provide genetic evidence for the possible causality of gut microbiota and its metabolites dysbiosis and PD. In particular, we found a protective association between BCAAs and PD, which point out a new direction for further investigation of the potential mechanism of BCAAs supplementation in PD treatment.
ACKNOWLEDGMENTS
The authors have no acknowledgments to report.
FUNDING
The present study was supported by the Major Health Science and Technology Program of Zhejiang Province [grant number WKJ-ZJ-ZZ08] and the Key Projects of Zhejiang Provincial Natural Science Fund [grant number LZ23H090004].
CONFLICT OF INTEREST
The authors have no conflict of interest to report.
DATA AVAILABILITY
The data used or analyzed during this study are available from the corresponding author on reasonable request.
SUPPLEMENTARY MATERIAL
[1] The supplementary material is available in the electronic version of this article: https://dx.doi.org/10.3233/JPD-240244.
REFERENCES
1. | Tysnes OB and Storstein A. Epidemiology of Parkinson’s disease. J Neural Transm (2017) ; 124: : 901–905. |
2. | Nalls MA , Blauwendraat C , Vallerga CL , et al. Identification of novel risk loci, causal insights, and heritable risk for Parkinson’s disease: a meta-analysis of genome-wide association studies. Lancet Neurol (2019) ; 18: : 1091–1102. |
3. | Chen SJ , Chen CC , Liao HY , et al. Association of fecal and plasma levels of short-chain fatty acids with gut microbiota and clinical severity in patients with Parkinson disease. Neurology (2022) ; 98: : e848–e858. |
4. | Yan Z , Yang F , Cao J , et al. Alterations of gut microbiota and metabolome with Parkinson’s disease. Microb Pathog (2021) ; 160: : 105187. |
5. | Li P , Killinger BA , Ensink E , et al. Gut microbiota dysbiosis is associated with elevated bile acids in Parkinson’s disease. Metabolites (2021) ; 11: : 29. |
6. | Pereira PAB , Trivedi DK , Silverman J , et al. Multiomics implicate gut microbiota in altered lipid and energy metabolism in Parkinson’s disease. NPJ Parkinsons Dis (2022) ; 8: : 39. |
7. | Elfil M , Kamel S , Kandil M , et al. Implications of the gut microbiome in Parkinson’s disease. Mov Disord (2020) ; 35: : 921–933. |
8. | Hasegawa S , Goto S , Tsuji H , et al. Intestinal dysbiosis and lowered serum lipopolysaccharide-binding protein in Parkinson’s disease. PLoS One (2015) ; 10: : e0142164. |
9. | Scheperjans F , Aho V , Pereira PA , et al. Gut microbiota are related to Parkinson’s disease and clinical phenotype. Mov Disord (2015) ; 30: : 350–358. |
10. | Unger MM , Spiegel J , Dillmann KU , et al. Short chain fatty acids and gut microbiota differ between patients with Parkinson’s disease and age-matched controls. Parkinsonism Relat Disord (2016) ; 32: : 66–72. |
11. | Keshavarzian A , Green SJ , Engen PA , et al. Colonic bacterial composition in Parkinson’s disease. Mov Disord (2015) ; 30: : 1351–1360. |
12. | Walter J , Armet AM , Finlay BB , et al. Establishing or exaggerating causality for the gut microbiome: lessons from human microbiota-associated rodents. Cell (2020) ; 180: : 221–232. |
13. | Zhao JV and Schooling CM. The role of testosterone in chronic kidney disease and kidney function in men and women: a bi-directional Mendelian randomization study in the UK Biobank. BMC Med (2020) ; 18: : 122. |
14. | Kurilshikov A , Medina-Gomez C , Bacigalupe R , et al. Large-scale association analyses identify host factors influencing human gut microbiome composition. Nat Genet (2021) ; 53: : 156–165. |
15. | Zhang Y , He X , Qian Y , et al. Plasma branched-chain and aromatic amino acids correlate with the gut microbiota and severity of Parkinson’s disease. NPJ Parkinsons Dis (2022) ; 8: : 48. |
16. | Yan Z , Yang F , Wen S , et al. Longitudinal metabolomics profiling of serum amino acids in rotenone-induced Parkinson’s mouse model. Amino Acids (2022) ; 54: , 111–121. |
17. | Babu GN , Gupta M , Paliwal VK , et al. Serum metabolomics study in a group of Parkinson’s disease patients from northern India. Clin Chim Acta (2022) ; 480: : 214–219. |
18. | Sampson TR , Debelius JW , Thron T , et al. Gut microbiota regulate motor deficits and neuroinflammation in a model of Parkinson’s disease. Cell (2016) ; 167: : 1469–1480.e12. |
19. | Lawlor DA , Harbord RM , Sterne JA , et al. Mendelian randomization: using genes as instruments for making causal inferences in epidemiology. Stat Med (2008) ; 27: : 1133–1163. |
20. | Verduijn M , Siegerink B , Jager KJ , et al. Mendelian randomization: use of genetics to enable causal inference in observational studies. Nephrol Dial Transplant (2010) ; 25: : 1394–1398. |
21. | Lopera-Maya EA , Kurilshikov A , van der Graaf A , et al. Effect of host genetics on the gut microbiome in 7,738 participants of the Dutch Microbiome Project. Nat Genet (2022) ; 54: : 143–151. |
22. | Hemani G , Zheng J , Elsworth B , et al. The MR-Base platform supports systematic causal inference across the human phenome. Elife (2018) ; 7: : e34408. |
23. | Pierce BL , Ahsan H and Vanderweele TJ. Power and instrument strength requirements for Mendelian randomization studies using multiple genetic variants. Int J Epidemiol (2011) ; 40: : 740–752. |
24. | Hemani G , Tilling K and Davey Smith G. Orienting the causal relationship between imprecisely measured traits using GWAS summary data. PLoS Genet (2017) ; 13: : e1007081. |
25. | Brion MJ , Shakhbazov K and Visscher PM. Calculating statistical power in Mendelian randomization studies. Int J Epidemiol (2013) ; 42: : 1497–1501. |
26. | Bowden J , Davey Smith G and Burgess S. Mendelian randomization with invalid instruments: effect estimation and bias detection through Egger regression. Int J Epidemiol (2015) ; 44: : 512–525. |
27. | Verbanck M , Chen CY , Neale B , et al. Detection of widespread horizontal pleiotropy in causal relationships inferred from Mendelian randomization between complex traits and diseases. Nat Genet (2018) ; 50: : 693–698. |
28. | Yan Z , Yang F , Sun L , et al. Role of gut microbiota-derived branched-chain amino acids in the pathogenesis of Parkinson’s disease: an animal study. Brain Behav Immun (2022) ; 106: : 307–321. |
29. | Wortelboer K , Nieuwdorp M , Herrema H . Fecal microbiota transplantation beyond Clostridioides difficile infections. EBioMedicine (2019) ; 44: : 716–729. |
30. | Costello SP , Waters O , Bryant RV , et al. Short duration, low intensity, pooled fecal microbiota transplantation induces remission in patients with mild-moderately active ulcerative colitis: a randomised controlled trial. Gastroenterology (2017) ; 152: : S198–S199. |
31. | Kim D , Hofstaedter CE , Zhao C , et al. Optimizing methods and dodging pitfalls in microbiome research. Microbiome (2017) ; 5: : 52. |
32. | Perez-Pardo P , Dodiya HB , Engen PA , et al. Gut bacterial composition in a mouse model of Parkinson’s disease. Benef Microbes (2018) ; 9: : 799–814. |
33. | Li T , Chu C , Yu L , et al. Neuroprotective effects of Bifidobacterium breve CCFMin MPTP-induced mouse models of Parkinson’s disease. Nutrients (2022) ; 14: : 4678. |
34. | Tamtaji OR , Taghizadeh M , Daneshvar Kakhaki R , et al. Clinical and metabolic response to probiotic administration in people with Parkinson’s disease: a randomized, double-blind, placebo-controlled trial. Clin Nutr (2019) ; 38: : 1031–1035. |
35. | Wallen ZD , Appah M , Dean MN , et al. Characterizing dysbiosis of gut microbiome in PD: evidence for overabundance of opportunistic pathogens. NPJ Parkinsons Dis (2020) ; 6: : 11. |
36. | Fan KC , Lin CC , Liu YC , et al. Altered gut microbiota in older adults with mild cognitive impairment: a case-control study. Front Aging Neurosci (2023) ; 15: : 1162057. |
37. | Nurrahma BA , Yeh TH , Hsieh RH , et al. Mangosteen pericarp extract supplementation boosts antioxidant status via rebuilding gut microbiota to attenuate motor deficit in 6-OHDA-induced Parkinson’s disease. Antioxidants (Basel) (2022) ; 11: : 2396. |
38. | Zhu Y , Huan F , Wang J , et al. 1-Methyl-4-phenyl-1,2,3,6-tetrahydropyridine induced Parkinson’s disease in mouse: potential association between neurotransmitter disturbance and gut microbiota dysbiosis. ACS Chem Neurosci (2020) ; 11: : 3366–3376. |
39. | Cheng JY , Deng YT , Yu JT . The causal role of circulating amino acids on neurodegenerative disorders: a two-sample Mendelian randomization study. J Neurochem (2023) ; 166: : 972–981. |
40. | Pedersen HK , Gudmundsdottir V , Nielsen HB , et al. Human gut microbes impact host serum metabolome and insulin sensitivity. Nature (2016) ; 535: : 376–381. |
41. | Yao CC , Sun RM , Yang Y , et al. Accumulation of branched-chain amino acids reprograms glucose metabolism in CD8+ T cells with enhanced effector function and anti-tumor response. Cell Rep (2023) ; 42: : 112186. |
42. | Hinkle JS , Rivera CN and Vaughan RA. Branched-Chain amino acids and mitochondrial biogenesis: an overview and mechanistic summary. Mol Nutr Food Res (2022) ; 66: : e2200109. |
43. | Li Z , Zhang R , Mu H , et al. Oral administration of branched-chain amino acids attenuates atherosclerosis by inhibiting the inflammatory response and regulating the gut microbiota in apoE-deficient mice. Nutrients (2022) ; 14: : 5065. |
44. | Qiao S , Liu C , Sun L , et al. Gut Parabacteroides merdae protects against cardiovascular damage by enhancing branched-chain amino acid catabolism. Nat Metab (2022) ; 4: : 1271–1286. |
45. | Guo CJ , Allen BM , Hiam KJ , et al. Depletion of microbiome-derived molecules in the host using Clostridium genetics. Science (2019) ; 366: : eaav1282. |
46. | Nogal A , Valdes AM and Menni C. The role of short-chain fatty acids in the interplay between gut microbiota and diet in cardio-metabolic health. Gut Microbes (2021) ; 13: : 1–24. |
47. | Chambers ES , Viardot A , Psichas A , et al. Effects of targeted delivery of propionate to the human colon on appetite regulation, body weight maintenance and adiposity in overweight adults. Gut (2015) ; 64: : 1744–1754. |
48. | Sun J , Li H , Jin Y , et al. Probiotic Clostridium butyricum ameliorated motor deficits in a mouse model of Parkinson’s disease via gut microbiota-GLP-1 pathway. Brain Behav Immun (2021) ; 91: : 703–715. |
49. | Hou YF , Shan C , Zhuang SY , et al. Gut microbiota-derived propionate mediates the neuroprotective effect of osteocalcin in a mouse model of Parkinson’s disease. Microbiome (2021) ; 9: : 34. |
50. | Davies NM , Holmes MV and Smith GD. Reading Mendelian randomisation studies: a guide, glossary, and checklist for clinicians. BMJ (2018) ; 362: : k601. |