Predicting Parkinson’s Disease and Its Pathology via Simple Clinical Variables
Abstract
Background:
Parkinson’s disease (PD) is a chronic, disabling neurodegenerative disorder.
Objective:
To predict a future diagnosis of PD using questionnaires and simple non-invasive clinical tests.
Methods:
Participants in the prospective Kuakini Honolulu-Asia Aging Study (HAAS) were evaluated biannually between 1995–2017 by PD experts using standard diagnostic criteria. Autopsies were sought on all deaths. We input simple clinical and risk factor variables into an ensemble-tree based machine learning algorithm and derived models to predict the probability of developing PD. We also investigated relationships of predictive models and neuropathologic features such as nigral neuron density.
Results:
The study sample included 292 subjects, 25 of whom developed PD within 3 years and 41 by 5 years. 116 (46%) of 251 subjects not diagnosed with PD underwent autopsy. Light Gradient Boosting Machine modeling of 12 predictors correctly classified a high proportion of individuals who developed PD within 3 years (area under the curve (AUC) 0.82, 95%CI 0.76–0.89) or 5 years (AUC 0.77, 95%CI 0.71–0.84). A large proportion of controls who were misclassified as PD had Lewy pathology at autopsy, including 79%of those who died within 3 years. PD probability estimates correlated inversely with nigral neuron density and were strongest in autopsies conducted within 3 years of index date (r = –0.57, p < 0.01).
Conclusion:
Machine learning can identify persons likely to develop PD during the prodromal period using questionnaires and simple non-invasive tests. Correlation with neuropathology suggests that true model accuracy may be considerably higher than estimates based solely on clinical diagnosis.
INTRODUCTION
Parkinson’s disease (PD) is a chronic, progressive and disabling neurodegenerative disorder beginning in mid to late life [1, 2]. Classical motor features result primarily from degeneration of dopaminergic neurons in the substantia nigra pars compacta (SNpc), and include rest tremor, slowness and paucity of movement, rigidity, impaired balance and autonomic symptoms. PD is now well-recognized to be a systemic disease [1, 2] with widespread intraneuronal accumulations of aggregated phosphorylated alpha-synuclein protein (Lewy bodies and Lewy neurites) and associated symptomatology detected throughout the spinal cord and autonomic nervous system, myenteric plexus and gut, olfactory system, visual system, pancreas and skin [3–9]. Peripheral pathology likely precedes central pathology [10].
There is no diagnostic test for PD, and motor signs and symptoms required to meet diagnostic criteria manifest only after extensive loss of striatal dopamine [1, 2, 11, 12]. By the time of diagnosis, 50–80%of nigral dopaminergic neurons are dead or dying [13]. Therapeutic agents designed to slow disease progression may be less effective at this late stage, and indeed more than three decades of clinical trials targeting early PD have failed to identify a disease modifying intervention. A prodromal period lasting many years precedes the onset of motor parkinsonism [14]. Interventions to delay the onset of parkinsonism could be implemented during this long pathologic evolution if persons at risk for developing PD could be identified with confidence.
Only a few prior studies have collected a broad range of prodromal features and risk factors prospectively, and the correlation between these risk factors and Lewy pathology at autopsy is understudied [15–18]. Importantly, incidental Lewy pathology has been detected in up to 20%of otherwise clinically normal individuals, and is thought by many to reflect early PD [19, 20]. We hypothesized that it is possible to identify those at risk of PD and Lewy body pathology using machine learning modeling of data obtained by questionnaire and simple clinical tests conducted during medical examination of participants in the prospective population-based Kuakini Honolulu-Asia Aging Study (HAAS) [21].
MATERIALS AND METHODS
Study cohort
The Kuakini Honolulu Heart Program (HHP) was established as a prospective cohort study in 1965 with enrollment of 8,006 Japanese-American men born 1900–1919. The original goals were to examine rates and risk factors for heart disease and stroke [22]. In 1991 with establishment of the Kuakini HAAS, the focus shifted to neurodegenerative diseases including PD. Environmental, lifestyle, and physical characteristics were ascertained in 1991 and at follow-up exams every 2–3 years through 2012. Detailed case finding methods have been published [23, 24]. Briefly, during the course of follow-up, all subjects were questioned about a diagnosis of PD and the use of PD medications by structured interview. Study participants received further screening by a technician trained in the recognition of the clinical symptoms of parkinsonism. Those with a history or sign of parkinsonism were referred to a study neurologist for a comprehensive neurologic examination and application of standard diagnostic criteria for PD [25]. For the current study, the exam conducted during 1994–1996 (Exam 5) was set as the index date. Individuals diagnosed with PD at or before the index date were excluded. Cohort members were followed until the latter of death or 2012.
Neuropathological evaluation
Autopsies have been sought on all Kuakini HAAS deaths since 1991 and obtained on about 20%. Full neuropathological methods are reported in Petrovitch et al. [16]. Briefly, examinations of multiple brain regions were performed by neuropathologists unaware of clinical diagnoses. Formalin-fixed hematoxylin and eosin stained sections of the mid-brain were prepared at the level of the exit of the third cranial nerve and mid-pons at the level of the locus coeruleus. Lewy bodies were identified by microscopic evaluation of single sections through the substantia nigra and locus coeruleus. If Lewy bodies were found in any region, then alpha-synuclein immunohistochemistry was also performed on sections of anterior cingulate, insula, frontal, temporal, and parietal lobes, and entorhinal cortex. Cortical Lewy bodies in these regions were then quantified [16]. SNpc neuron counts were determined as previously described for dorsomedial, ventromedial, dorsolateral, and ventrolateral quadrants, and neuron density expressed as neurons/mm2 [26].
Case/control definitions
Final diagnosis of incident PD ascertained after the index date was determined by neurological exam and PD experts using published diagnostic criteria [23, 24]. Participants who did not manifest signs of parkinsonism or dementia were classified as controls. Among controls who went to autopsy, some were found incidentally to have nigral Lewy pathology. Thus, controls comprised three mutually exclusive groups: 1) autopsied with incidental Lewy pathology (iLB-Yes), 2) autopsied with no incidental Lewy pathology (iLB-No), and 3) not autopsied (iLB-Unknown). In order to best investigate the relationship of predictive models with Lewy pathology we included all PD cases (n = 58) and all iLB-Yes controls (n = 84), and age-matched them to iLB-No (n = 32) and iLB-Unknown (n = 135) controls at approximately a 1 : 1 ratio.
Clinical variables
Clinical variables analyzed in the current study were collected during follow-up exams at or prior to the index date (Exam 5, 1994–1996). For variables collected at multiple exams, we selected the measurement temporally closest to the index date. Available variables from Exam 5 included age, simple reaction time [27], and choice reaction time (measured using a computerized reaction time test [28], and modeled as continuous variables (s) [29, 30]), and olfactory discrimination (assessed with the brief smell identification test [31] (BSIT) (total score; range 0–12) [27]). Variables from Exam 4 (1991–1993) included body mass index (BMI; kg/m2) [32], smoking history (never, past, or current [33]), excessive daytime sleepiness (response to the question “are you sleepy most of the day” [23, 27]), bowel movement frequency (defined as < every other day, every other day, once per day, 2–3 times per day, or > 3 times per day [34], modeled as an ordinal variable), and cognitive impairment (evaluated using the Cognitive Abilities Screening Instrument (CASI), with total score modeled as a continuous variable [35]). We also incorporated three variables from the mid-life 1967–8 HHP exam including presence of hypertension (systolic blood pressure ≥140 or diastolic blood pressure ≥90 or taking antihypertensive medication [27] [33]), self-reported history of a head injury with loss of consciousness [36]) and daily average coffee consumption (ounces per day, analyzed as a continuous variable [33]).
Machine learning classifier of a future diagnosis of PD
We implemented Light Gradient Boosting Ma-chine (LGBM) as a classifier, a decision tree-based ensemble method that iteratively builds decision trees with the main goal of reducing classification (or prediction) error from the previous step. LGBM consists of individual shallow decision trees that avoid overfitting problems [37, 38]. In a classification task, LGBM produces a value between 0 and 1 representing the probability of belonging to each class. These values may be further transformed to predicted class labels by processing via a threshold (or cutoff) value. We did not implement a separate missing data imputation since LGBM can automatically handle missing data. We implemented a five-fold cross-validation strategy to avoid overfitting models. A Bayesian optimization algorithm was used for hyperparameter tuning [39] to find optimal values of parameters such as number of trees, tree depth, learning rate, and boosting rate.
We built several LGBM models to predict PD using different sets of controls. In Model 1, we considered all participants without a clinical diagnosis of PD as controls. Model 2 excluded controls who had Lewy bodies at autopsy (iLB-Yes). In Model 3, we excluded both iLB-Yes controls and controls who did not have an autopsy (iLB-Unknown) and included only controls known to be free of Lewy bodies at autopsy (iLB-No). Finally, we generated a Model 4, in which we re-annotated iLB-Yes controls as cases for model development. To avoid any circularity, iLB-Yes controls were excluded from assessments of Model 4 classification accuracy. To ensure that models have practical applicability to future studies of disease modifying interventions, primary analyses used two separate prediction windows, 3- and 5- years from index date, to model both short- and mid-term predictors of PD risk.
Overfitting is a common problem when using machine learning. It occurs when trained machine learning models learn the patterns that are specific to the training sample, rather than learning the patterns representing the input-outcome relationship. Overfitting manifests as high accuracy in training data but poor performance in testing. We implemented a 5-fold cross-validation strategy to ensure generalizability within our study cohort. At each run of the 5-fold cross-validation, we randomly selected 10%of the training data to be used as a validation set for early stopping to minimize overfitting. To avoid information leak during the five steps of the cross validation, we built five different models from scratch independent from the parameters of the model developed in other steps. We assessed classification models using various performance metrics including sensitivity, specificity, and area under the receiver operating characteristics (ROC) curve (AUC) statistics. We additionally analyzed the correlation of predictive models with neuron density. We performed LGBM’s default variable importance analyses to rank variables based on their contribution to the predictions, which calculates an average “gain” value (relative importance) of the corresponding variable to the model. A higher “gain” value of a variable shows its importance over other variables of lesser gain value [40]. We further extended the variable importance analyses to estimate the independent magnitude and direction of effect of the predictors on the risk for PD. This was done by quantifying the average change (with 95%confidence interval) on predicted risk for PD corresponding to one standard deviation increase in continuous predictors or one unit increase in ordinal categorical variables.
All analyses were carried out using Python programming language.
The study was approved by the Institutional Review Boards of Loyola University Chicago (LU 212399), the University of California-San Francisco, and the Kuakini Medical Center.
RESULTS
The analytic sample consisted of 309 Kuakini HAAS participants with complete data and who did not have a PD diagnosis at the index date (Exam 5, 1994–1996). A total of 58 individuals were diagnosed with incident PD. Among these, 25 were diagnosed with PD within 3 years, and an additional 16 were diagnosed within 5 years of the index date. Eleven of 41 participants who developed PD within 5 years had autopsies, all of whom had Lewy body pathology. Among 251 clinically-defined controls, we included 84 with Lewy body pathology at autopsy (iLB-Yes), 32 without Lewy body pathology (iLB-No), and 135 without an autopsy (iLB-Unknown). Cohort characteristics are summarized in Table 1. For most predictor variables, values were most extreme among those who developed PD within 3 years of the index date, and values for iLB-Yes were intermediate between iLB-No and PD. Relative to all controls, cases had significantly lower bowel movement frequency and BSIT olfaction scores, and greater daytime sleepiness. Relative to controls without LB pathology, controls with LB pathology scored lower on the CASI and the BSIT.
Table 1
Study Sample Characteristics
Cases | All Controls (n = 251) | Control Sub-Categories | ||||
PD in 3 years (n = 25) | PD in 5 years Case (n = 41) | LB-Unknown (n = 135) | LB-No (n = 32) | LB-Yes (n = 84) | ||
Age at index date, mean (SD) | 81.4 (4.5) | 80.4 (4.3) | 81.1 (4.4) | 80.7 (4.1) | 81.4 (5.0) | 81.6 (4.7) |
Years from index date until autopsy, mean (SD)* | 3.9 (1.21) | 5.5 (2.2) | 10.2 (3.9) | – | 10.8 (4.3) | 10.1 (3.8) |
Simple reaction time, mean ms (SD) | 532.3 (245.9) | 483.9 (209) | 440.1 (163.3) | 446.3 (164.8) | 401.4 (87.4) | 445.5 (183.7) |
Choice reaction time, mean ms (SD) | 701.7 (316.5) | 628.2 (263) | 573.7 (164.1) | 570.6 (153.7) | 538.9 (126.8) | 593.9 (192.6) |
BMI kg/m2, mean (SD) | 24.2 (2.9) | 24.5 (3.0) | 23.9 (3.3) | 23.9 (3.3) | 23.0 (3.3) | 23. 8 (3.1) |
Coffee oz/day, mean (SD) | 9.4 (6.4) | 9.7 (9.7) | 14 (13.1) | 14.6 (13.7) | 14.3 (12.1) | 12.8 (12.5) |
CASI total score, mean (SD) ** | 81.4 (12.5) | 85.6 (11.3) | 84.4 (12.8) | 86.1 (8.8) | 86.8 (8.4) | 80.8 (18.1) |
Olfaction BSIT score, median [range]*, ** | 4 [0–11] | 6 [0–11] | 7 [0–12] | 8 [0–12] | 7.5 [0–12] | 5 [0–12] |
Bowel Movement Frequency* | ||||||
< 1 every other day | 1 (4%) | 1 (2.4%) | 3 (1.2%) | 0 (0%) | 0 (0%) | 3 (3.6%) |
Every other day | 5 (20%) | 9 (22%) | 13 (5.2%) | 6 (4.4%) | 0 (0%) | 7 (8.3%) |
Once per day | 14 (56%) | 24 (58.5%) | 157 (62.6%) | 87 (64.4%) | 20 (62.5%) | 50 (59.5%) |
2–3 per day | 5 (20%) | 6 (14.7%) | 61 (24.3%) | 32 (23.8%) | 10 (31.3%) | 19 (22.6%) |
> 3 per day | 0 (0%) | 0 (0%) | 8 (3.1%) | 3 (2.2%) | 1 (3.1%) | 4 (4.8%) |
Missing | 0 (0%) | 1 (2.4%) | 9 (3.6%) | 7 (5.2%) | 1 (3.1%) | 1 (1.2%) |
Excess Daytime sleepiness* | ||||||
No | 19 (76%) | 34 (82.9%) | 230 (91.6.%) | 128 (94.8.%) | 31 (88.6%) | 71 (84.5%) |
Yes | 6 (24%) | 7 (17.1%) | 18 (7.2%) | 7 (5.2%) | 1 (2.8%) | 10 (11.9%) |
Missing | 0 (0.0%) | 0 (0%) | 3 (1.2%) | 0 (0%) | 3 (8.6%) | 3 (3.6%) |
Traumatic Brain Injury | ||||||
No | 22 (88%) | 34 (82.9%) | 211 (84.1%) | 118 (87.4%) | 26 (81.3%) | 67 (79.8%) |
Yes | 3 (12%) | 7 (17.1%) | 28 (11.1%) | 11 (8.1%) | 6 (18.7%) | 11 (13.1%) |
Missing | 0 (0%) | 0 (0%) | 12 (4.8%) | 6 (4.5%) | 0 (0.0%) | 6 (7.1%) |
Smoking | ||||||
Never | 9 (36%) | 14 (34.1%) | 99 (39.4%) | 53 (39.3%) | 11 (34.4%) | 35 (41.7%) |
Past | 16 (64%) | 26 (63.4%) | 138 (55%) | 78 (57.8%) | 17 (53.1%) | 43 (51.2%) |
Current | 0 (0.0%) | 1 (2.5%) | 14 (5.6%) | 4 (2.9%) | 4 (12.5%) | 6 (7.1%) |
Hypertension | ||||||
No | 7 (28%) | 11 (26.8%) | 71 (28.2%) | 33 (24.4%) | 8 (25%) | 30 (35.7%) |
Yes | 18 (72%) | 30 (73.2%) | 180 (71.8%) | 102 (75.6%) | 24 (75%) | 54 (64.3%) |
*Significantly different (p < 0.05) between all cases and all controls. **Significantly different (p < 0.05) between LB-Yes and LB-No controls.
Predicting a future diagnosis of PD within 5 years
Five-fold cross-validation accuracies for predicting a diagnosis of PD within 3 or 5 years after index date using several different control subgroups are presented in Table 2. The majority of misclassification in Model 1 occurred among controls with Lewy pathology (iLB-Yes), 38 (45%) of whom were classified by the model as PD. As noted in the Methods, we used this information to generate a Model 4, in which we re-annotated these individuals as cases for model development. Model 4 yielded the best accuracy for predicting future PD with AUC and 95%CIs of 0.82 (0.76–0.89) for a 3-year prediction window and 0.77 (0.71–0.84) for a 5-year prediction window. iLB-Yes controls were not included when calculating the AUCs for this model so as to avoid any circularity. Figure 1 depicts AUCs and examples of the sensitivity and specificity for predicting a clinical diagnosis of PD for several cut-points.
Table 2
Machine Learning Models for Prediction of Incident Clinical PD
AUC (95%CI) | |||
Model | Control group (n = 251) | Case 3-year (PD n = 25) | Case 5-year (PD n = 41) |
1* | LB-Unknown (135) | 0.64 (0.51–0.76) | 0.61 (0.52–0.71) |
LB-No (32) | |||
LB-Yes (84) | |||
2 | LB-Unknown (135) | 0.71 (0.59–0.83) | 0.61 (0.51–0.71) |
LB-No (32) | |||
3 | LB-No (32) | 0.79 (0.67–0.91) | 0.73 (0.62–0.85) |
4** | LB-Unknown (135) | 0.82 (0.76–0.89) | 0.77 (0.71–0.84) |
LB-No (32) |
*We also ran a model by using LB-Yes patients as cases and obtained AUC of 0.63 (0.56–0.70) for 3-year prediction window and AUC of 0.61 (0.55–0.68) for 5-year prediction window. **Controls with LB at autopsy (LB-Yes) were reannotated as PD for model development but excluded from tests of model performance. When we implemented Model 3, among 84 LB-Yes controls, 38 were classified as cases (PD) and 46 as controls). Using this evidence, in Model 4, we rebuilt a model by using these 38 as cases and 46 as controls. In addition, to compare the robustness of Model 4 with Model 3, we further excluded LB-Unknown patients in the AUC calculation and obtained an AUC of 0.91 (0.82–0.99) for 3-year prediction window and 0.80 (0.70–0.90) for 5-year prediction.
Fig. 1
ROC curve of Model 4 for 3-year (left) (AUC 0.82) and 5-year (right) (AUC 0.79) prediction windows.
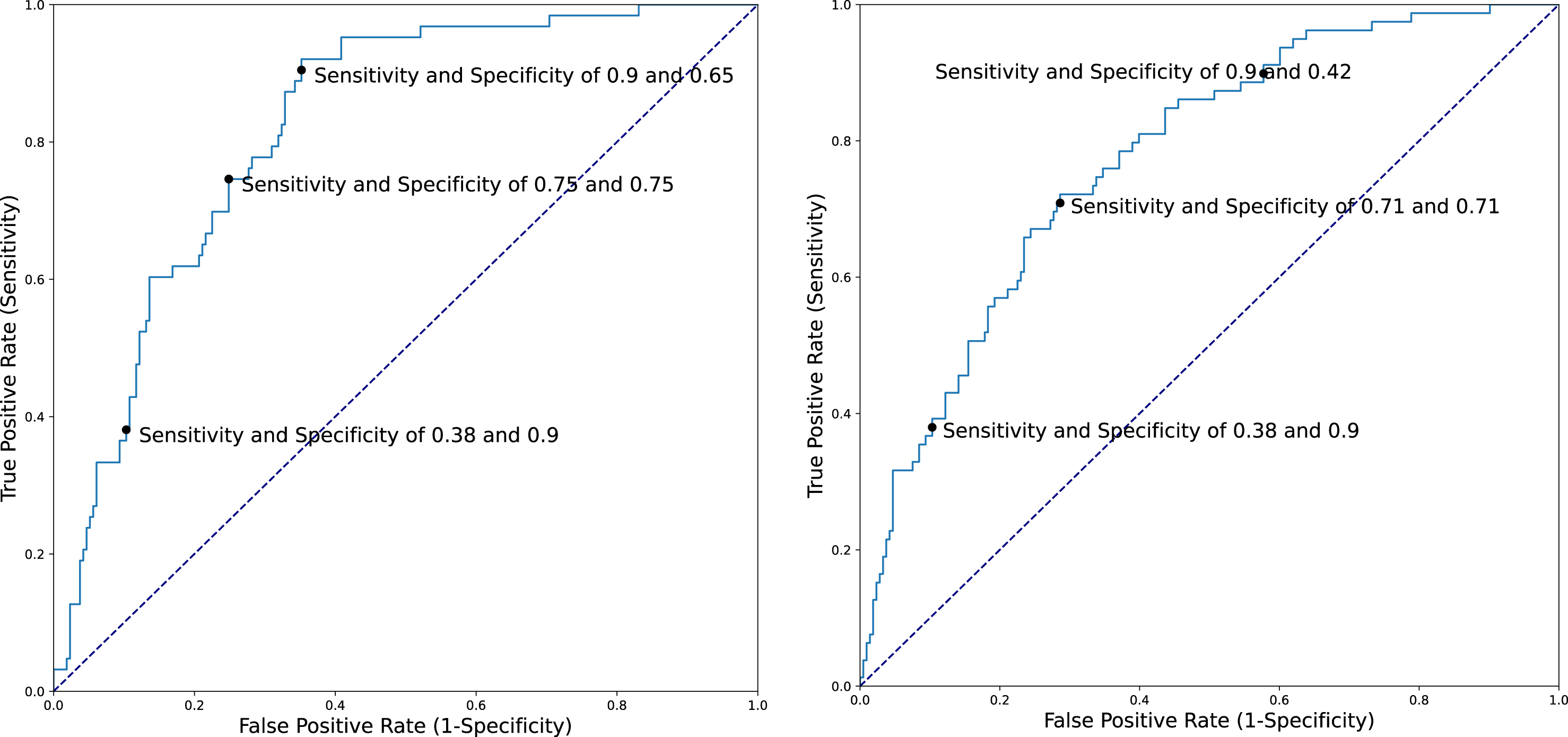
Although our main goal in this study was to develop models to identify patients at risk for being clinically diagnosed with PD within a specified time frame (3 or 5 years), as a sensitivity analysis, we also repeated Model 4 including 17 additional individuals who were diagnosed with PD beyond 5 years of index date (median 8 years, Range 6–17). As expected, the classification accuracy was lower, with an AUC of 0.70 (95%CI 0.64–0.77).
Censor-time based subgroup analysis for controls
Because the time from index date to autopsy was as long as 14 years for some participants, we calculated prediction performance for different time intervals until autopsy. For iLB-Yes controls whose autopsy was within 3 years of the index date, our model classified 79%as PD. This declined to 67%of iLB-Yes controls autopsied within 4 years, 55%within 5 years, and 40%of those autopsied > 7 years after index date. Thus, those with incidental Lewy pathology who were identified closer to the index date were more likely to be classified as PD.
Correlation of predicted PD risk probability with neuron density
Neuron densities and their correlations with predicted 5-year PD risk probability are shown in Table 3. Age at autopsy was not significantly correlated with any of the neuron density variables (all |correlations| < 0.1; data not shown). As expected, neuron density was highest in controls without LBs and lowest in PD cases. The classification scores correlated inversely with neuron density in all SNpc quadrants, with ventromedial neuron density being most strongly correlated to predicted PD risk. This negative correlation suggested that a greater predicted probability of PD is associated with lower nigral neuron density at death. As above, since autopsies were performed after a variable number of years following index date, we further investigated how the correlation of predicted PD risk and ventromedial neuron density varied by the time since the index date. As shown in Fig. 2, correlations were stronger for autopsies performed closer to the index date.
Table 3
SNpc neuron densities and correlations with estimated PD risk
Overall correlation with PD risk probability (n = 134) | Neuron counts by case status mean neurons/mm3 with 95%CI | |||
Diagnosed with PD (n = 31) | Controls | |||
LB-Yes (n = 78) | LB-No (n = 25) | |||
Dorsomedial quadrant | –0.16, p = 0.10 | 9.1 (6.9–11.3) | 15.3 (13.5–17.1) | 19.4 (15.7–23.1) |
Ventromedial quadrant | –0.28, p < 0.01 | 8.6 (6.2–11.0) | 15.1 (13.2–17.0) | 19.5 (15.9–23.1) |
Dorsolateral quadrant | –0.08, p = 0.38 | 7.8 (6.0–9.6) | 10.4 (9.1–11.7) | 12.7 (9.5–15.9) |
Ventrolateral quadrant | –0.23, p < 0.05 | 5.3 (3.6–7.0) | 15.8 (13.8–17.8) | 20.2 (17.5–22.9) |
Total Neuron Density | –0.24, p < 0.01 | 7.7 (6.0–9.4) | 14.3 (12.9–15.7) | 18.2 (15.6–20.8) |
Fig. 2
Correlation between predicted PD risk and ventromedial neuron density is stronger closer to index date.
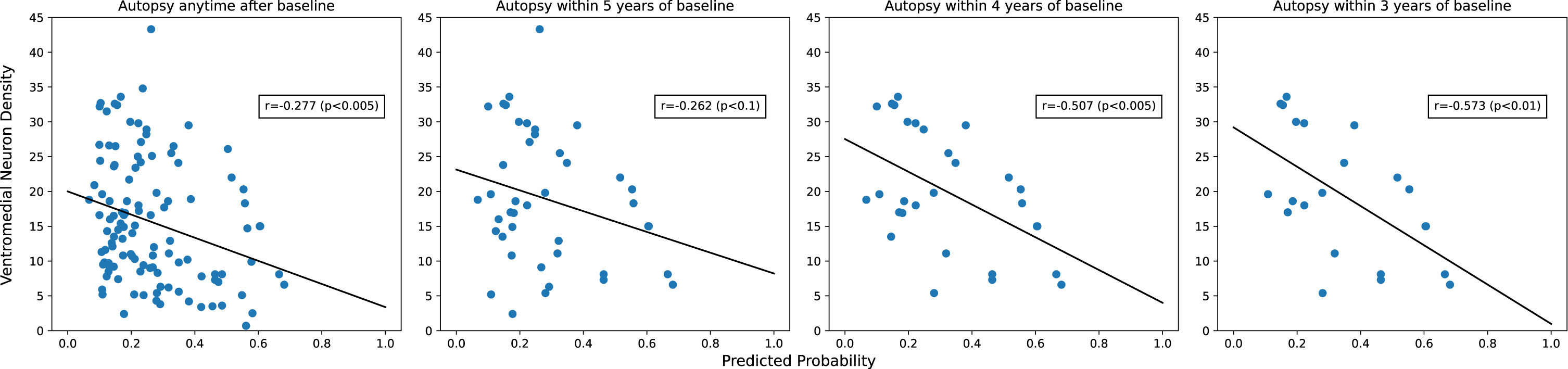
Variable importance analysis
Figure 3 depicts the relative contributions of each variable to LGBM Model 4 at 3 and 5 years after index date. The reaction time variables were most important, followed by olfaction score and BMI. Figure 4 depicts the magnitude, precision (95%CI) and direction (inverse or direct relationship) of independent contributions to the classification model, in which each point with associated bar represents the mean change in predicted PD risk with 95%CI when the variable value was artificially increased by 1 standard deviation for continuous variables and by 1 unit for categorical variables. Most variables contributed in the expected direction with the exception of CASI score and age. The inverse directionality of age may reflect interaction between age and other model variables and/or may be due to the narrow age range of the study cohort. For example, slower reaction time is likely to be a stronger predictor of future PD in younger individuals.
Fig. 3
Variable Importance for predicting PD within 3 (Top) and 5 years (Bottom). The length of the bar depicts the relative importance.
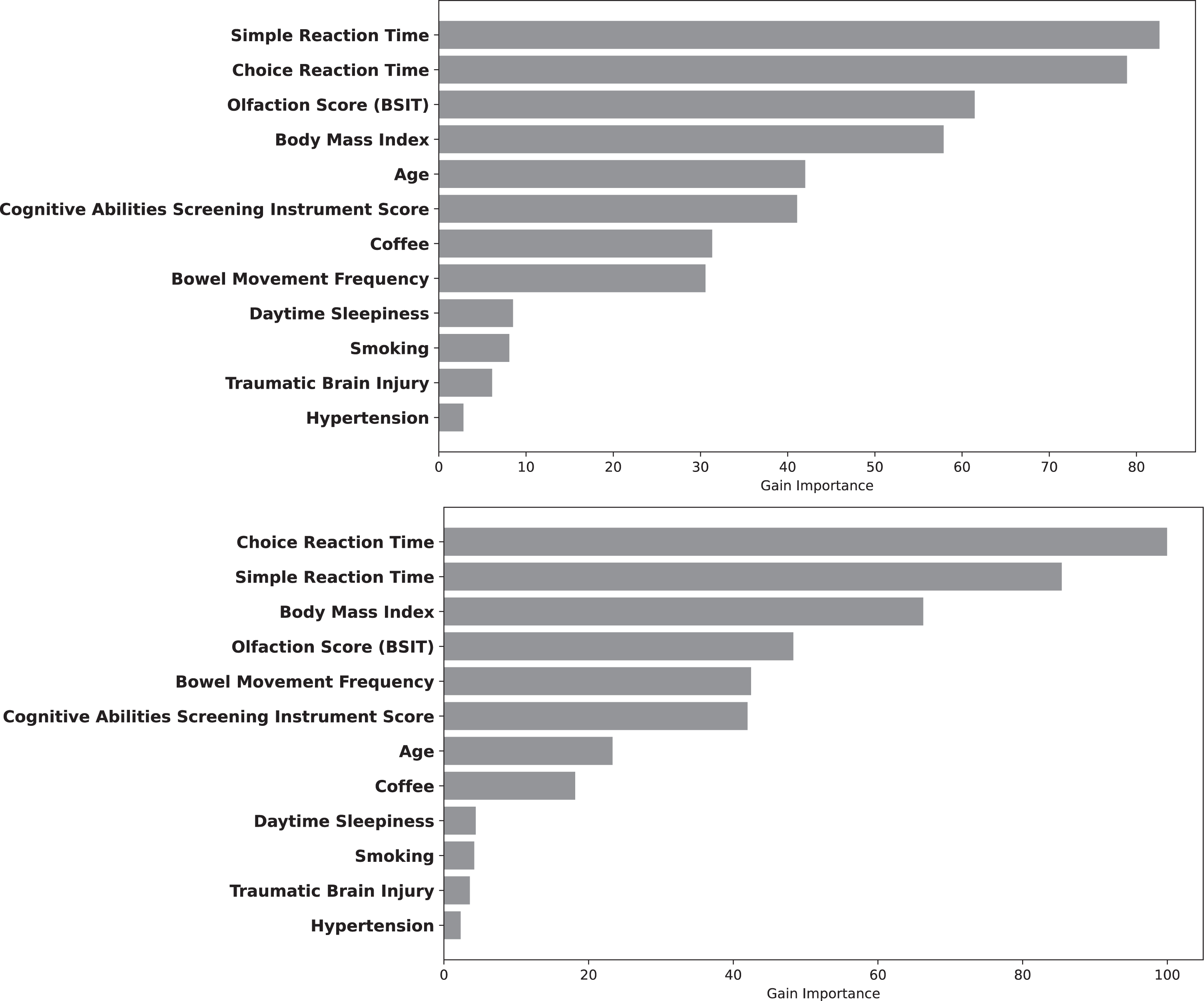
Fig. 4
Independent direction and magnitude of effect for 3-year (Top) and 5-year (Bottom) prediction windows.
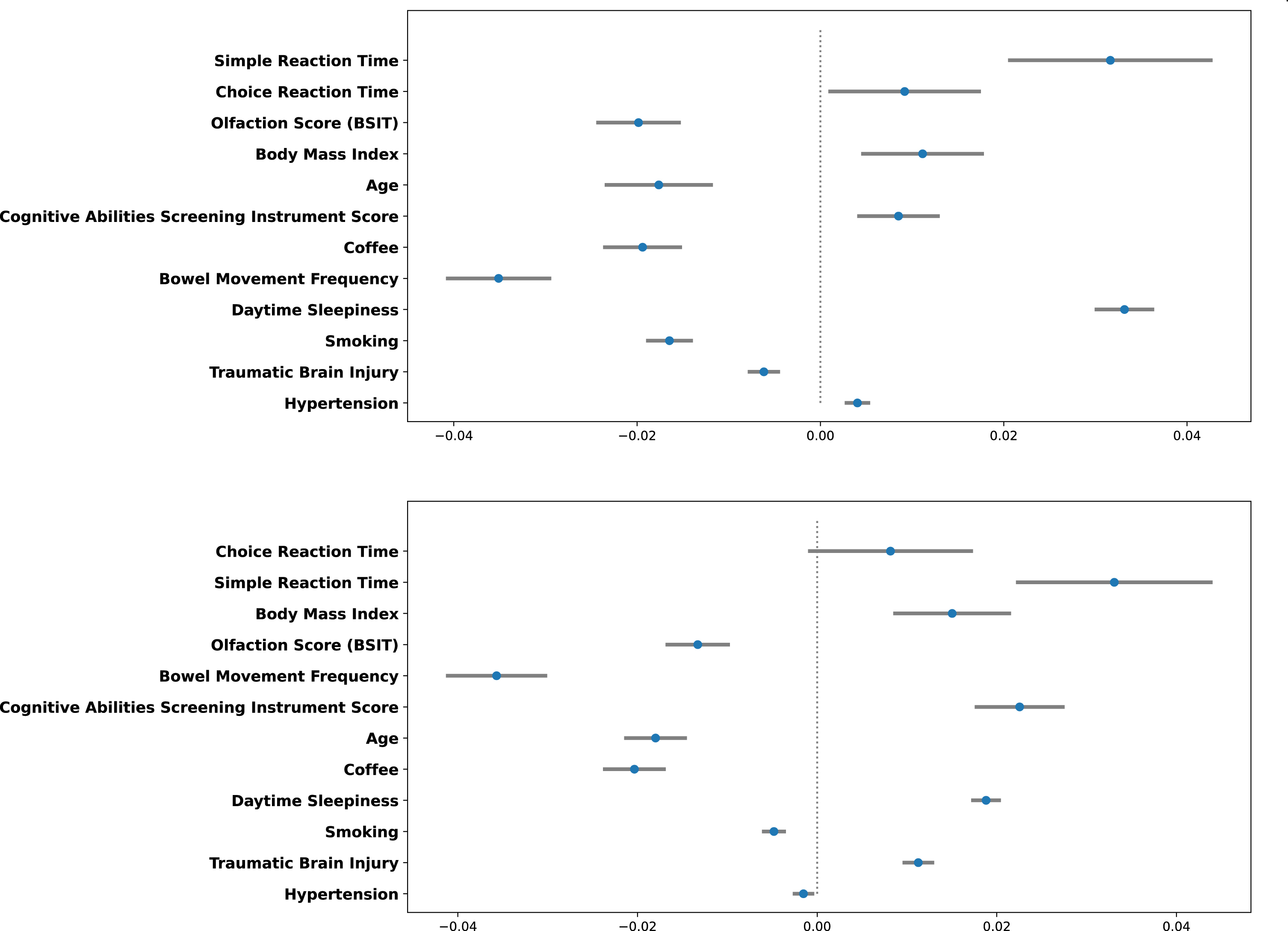
DISCUSSION
Identifying PD in its earliest stages, before significant motor symptoms manifest, may be essential for the development and implementation of disease modifying interventions. However, current criteria for prodromal PD have been validated in only a handful of studies [41–43], and performance has varied among populations [44]. The most specific prodromal indicators, such as dopamine transporter imaging or ultrasonography, can be costly and invasive, while others such as REM sleep behavior disorder are relatively rare in the general population and definitive diagnosis requires polysomnography. In the current study, we applied machine learning techniques to accurately classify persons at risk for developing PD. Because our models relied exclusively on non-invasive and inexpensive tests, many of which could be implemented remotely, such as in an online or mobile phone-based assessment, and historical variables easily determined by self-report, this approach could be efficiently implemented in large, targeted populations.
To our knowledge, this is the first time that post-mortem pathologic findings have been combined with the clinical diagnosis of PD to explore model classification performance. We have also used these pathologic data to tune model performance and maximize classification accuracy. Remarkably, 45%of clinical controls who were misclassified as having PD by our initial model were found to have nigral Lewy bodies at autopsy. Because incidental Lewy bodies likely reflect early-stage PD [25, 45, 46], we propose that the model is in fact correctly classifying people with prodromal PD, though we cannot rule out the possibility of another evolving neurodegenerative synucleinopathy. Further supporting this interpretation, model prediction probabilities correlated significantly with lower nigral neuron density, and correlations were strongest in those whose autopsies were within three years of the index date. Similarly, the proportion of iLB-Yes controls classified as PD was highest for those with the shortest time from index date until autopsy. Although we obtained classification AUCs of 0.82 and 0.77 at 3- and 5-years after the index date, many of the variables included in our model were collected as long as several decades before the index date. The most important predictors—simple reaction time, choice reaction time, and olfactory discrimination—were all collected at the index date. Prediction accuracy would likely have been considerably higher had all variables been collected closer to the index date, as has been previously observed in this cohort for olfactory dysfunction.[47]
The International Parkinson’s Disease and Movement Disorder Society (IPMDS) has identified 23 individual factors in a proposed research definition of prodromal PD [14]. While many of those features were not assessed here, remarkably, the variables with the highest importance for predicting PD in this machine learning derived model based on clinical and pathological outcomes parallel many of those assigned greater importance in the IPMDS model, represented as higher likelihoods [14]. The variables with greatest importance for predicting PD within 3 or 5 years in our model were two quantitative motor tests (simple and choice reaction time). Abnormal quantitative motor tests are also a feature in the IPMDS criteria, but with only moderately strong likelihood ratios. Impaired smell recognition and increasing age are recognized as important in both models. BMI, among the top four most important factors in this model, may be a surrogate for diabetes mellitus and physical inactivity, two IPMDS criteria. Hypertension is the only variable identified in this model that is not represented in the IPMDS criteria. In fact, orthostatic hypotension is strongly weighted in the IPMDS criteria. This may reflect the fact that our model included hypertension in midlife, determined more than 25 years before index date.
Our study has some limitations. Most importantly, the Kuakini HAAS cohort is comprised entirely of Japanese-American men in Hawaii, and with an index date mean age over 80, they are substantially older than study populations likely to be enrolled in disease modifying therapeutic trials. Thus, although our modeling approach may be widely applicable, our model weightings are not likely to be generalizable outside of this population. Additionally, as noted above, predictor variables were collected at differing timepoints before the index date. Although we would expect this to have biased our models toward the null, it nonetheless further hinders generalizability. Further, despite the fact that all individuals defined here as controls (with or without incidental Lewy pathology) did not have clinical evidence of parkinsonism or dementia, we did not consider other types of neuropathology in these analytic models. Finally, although we implemented a comprehensive cross-validation strategy, our sample size was relatively small and variable coefficients imprecise.
Machine learning techniques may provide opportunities to identify individuals during prodromal PD [48], as well as to predict disease progression [49]. In prior work, we successfully implemented a machine learning approach to detect PD autonomic features prior to diagnosis using a single lead of a standard 10-s 12-lead electrocardiogram [50]. Karabayir et al. implemented an LGBM algorithm to accurately classify PD using data generated from a simple speech test [51]. Although some have criticized machine learning methods as non-intuitive, the development of compact models using a small number of clinical variables increases their utility and potentially their portability across healthcare settings and systems [52].
Advances in digital technology are increasingly being applied in the assessment of health outcomes [53]. Many of the variables with highest predictive value in this model, as well as our prior finding associating reduced heart rate variability and future risk of PD [50] can now be determined using personal technologies such as online computerized testing, mobile phone applications or wrist-worn sensors [53]. In the future, machine learning algorithms such as those reported here may be effectively combined with self-reported health measurements and digital assessments to develop an efficient, low cost method for population screening and prospective monitoring of those with prodromal PD.
Investigators wishing to test our model in other study populations can access the source code at https://github.com/akbilgic/AI_PD_ClinicalModel.
ACKNOWLEDGMENTS
We thank Michael J Fox Foundation for supporting this research (MJFF Grant ID 17267, PI Akbilgic). This work was also supported by the National Institute on Aging and National Institute of General Medical Sciences.
We also would like to extend our thanks to the Kuakini Medical Center in Honolulu, HI for providing Kuakini HAAS data, and to the HAAS study participants.
CONFLICT OF INTEREST
The authors have no conflict of interest to report.
REFERENCES
[1] | Poewe W , Seppi K , Tanner CM , Halliday GM , Brundin P , Volkmann J , Schrag AE , Lang AE ((2017) ) Parkinson disease. Nat Rev Dis Primers 3: , 17013. |
[2] | Obeso JA , Stamelou M , Goetz CG , Poewe W , Lang AE , Weintraub D , Burn D , Halliday GM , Bezard E , Przedborski S , Lehericy S , Brooks DJ , Rothwell JC , Hallett M , DeLong MR , Marras C , Tanner CM , Ross GW , Langston JW , Klein C , Bonifati V , Jankovic J , Lozano AM , Deuschl G , Bergman H , Tolosa E , Rodriguez-Violante M , Fahn S , Postuma RB , Berg D , Marek K , Standaert DG , Surmeier DJ , Olanow CW , Kordower JH , Calabresi P , Schapira AHV , Stoessl AJ ((2017) ) Past, present, and future of Parkinson’s disease: A special essay on the 200th Anniversary of the Shaking Palsy. Mov Disord 32: , 1264–1310. |
[3] | Beach TG , Adler CH , Sue LI , Vedders L , Lue L , White Iii CL , Akiyama H , Caviness JN , Shill HA , Sabbagh MN , Walker DG ((2010) ) Multi-organ distribution of phosphorylated alpha-synuclein histopathology in subjects with Lewy body disorders. Acta Neuropathol 119: , 689–702. |
[4] | Braak H , Braak E , Yilmazer D , Schultz C , de Vos RA , Jansen EN ((1995) ) Nigral and extranigral pathology in Parkinson’s disease. J Neural Transm Suppl 46: , 15–31. |
[5] | Orimo S , Takahashi A , Uchihara T , Mori F , Kakita A , Wakabayashi K , Takahashi H ((2007) ) Degeneration of cardiac sympathetic nerve begins in the early disease process of Parkinson’s disease. Brain Pathol 17: , 24–30. |
[6] | Braak H , de Vos RA , Bohl J , Del Tredici K ((2006) ) Gastric alpha-synuclein immunoreactive inclusions in Meissner’s and Auerbach’s plexuses in cases staged for Parkinson’s disease-related brain pathology. Neurosci Lett 396: , 67–72. |
[7] | Doty RL ((2012) ) Olfaction in Parkinson’s disease and related disorders. Neurobiol Dis 46: , 527–552. |
[8] | Gjerloff T , Fedorova T , Knudsen K , Munk OL , Nahimi A , Jacobsen S , Danielsen EH , Terkelsen AJ , Hansen J , Pavese N , Brooks DJ , Borghammer P ((2015) ) Imaging acetylcholinesterase density in peripheral organs in Parkinson’s disease with 11C-donepezil PET. Brain 138: , 653–663. |
[9] | Gibbons CH , Garcia J , Wang N , Shih LC , Freeman R ((2016) ) The diagnostic discrimination of cutaneous alpha-synuclein deposition in Parkinson disease. Neurology 87: , 505–512. |
[10] | Olanow CW , Brundin P ((2013) ) Parkinson’s disease and alpha synuclein: is Parkinson’s disease a prion-like disorder? Mov Disord 28: , 31–40. |
[11] | Berg D , Postuma RB , Adler CH , Bloem BR , Chan P , Dubois B , Gasser T , Goetz CG , Halliday G , Joseph L , Lang AE , Liepelt-Scarfone I , Litvan I , Marek K , Obeso J , Oertel W , Olanow CW , Poewe W , Stern M , Deuschl G ((2015) ) MDS research criteria for prodromal Parkinson’s disease. Mov Disord 30: , 1600–1611. |
[12] | Postuma RB , Berg D , Stern M , Poewe W , Olanow CW , Oertel W , Obeso J , Marek K , Litvan I , Lang AE , Halliday G , Goetz CG , Gasser T , Dubois B , Chan P , Bloem BR , Adler CH , Deuschl G ((2015) ) MDS clinical diagnostic criteria for Parkinson’s disease. Mov Disord 30: , 1591–1601. |
[13] | Fearnley JM , Lees AJ ((1991) ) Ageing and Parkinson’s disease: substantia nigra regional selectivity. Brain 114 (Pt 5): , 2283–2301. |
[14] | Heinzel S , Berg D , Gasser T , Chen H , Yao C , Postuma RB , Disease MDSTFotDoPs ((2019) ) Update of the MDS research criteria for prodromal Parkinson’s disease. Mov Disord 34: , 1464–1470. |
[15] | Abbott RD , Ross GW , Duda JE , Shin C , Uyehara-Lock JH , Masaki KH , Launer LJ , White LR , Tanner CM , Petrovitch H ((2019) ) Excessive daytime sleepiness and topographic expansion of Lewy pathology. Neurology 93: , e1425–e1432. |
[16] | Petrovitch H , Abbott RD , Ross GW , Nelson J , Masaki KH , Tanner CM , Launer LJ , White LR ((2009) ) Bowel movement frequency in late-life and substantia nigra neuron density at death. Mov Disord 24: , 371–376. |
[17] | Ross GW , Abbott RD , Petrovitch H , Tanner CM , Davis DG , Nelson J , Markesbery WR , Hardman J , Masaki K , Launer L , White LR ((2006) ) Association of olfactory dysfunction with incidental Lewy bodies. Mov Disord 21: , 2062–2067. |
[18] | Driver-Dunckley E , Adler CH , Hentz JG , Dugger BN , Shill HA , Caviness JN , Sabbagh MN , Beach TG , Arizona Parkinson Disease Consortium ((2014) ) Olfactory dysfunction in incidental Lewy body disease and Parkinson’s disease. Parkinsonism Relat Disord 20: , 1260–1262. |
[19] | Dugger BN , Hentz JG , Adler CH , Sabbagh MN , Shill HA , Jacobson S , Caviness JN , Belden C , Driver-Dunckley E , Davis KJ , Sue LI , Beach TG ((2014) ) Clinicopathological outcomes of prospectively followed normal elderly brain bank volunteers. J Neuropathol Exp Neurol 73: , 244–252. |
[20] | Iacono D , Geraci-Erck M , Rabin ML , Adler CH , Serrano G , Beach TG , Kurlan R ((2015) ) Parkinson disease and incidental Lewy body disease: Just a question of time? Neurology 85: , 1670–1679. |
[21] | Ross GW , Abbott RD , Petrovitch H , Tanner CM , White LR ((2012) ) Pre-motor features of Parkinson’s disease: the Honolulu-Asia Aging Study experience. Parkinsonism Relat Disord 18 Suppl 1: , S199–202. |
[22] | White L , Petrovitch H , Ross GW , Masaki KH , Abbott RD , Teng EL , Rodriguez BL , Blanchette PL , Havlik RJ , Wergowske G , Chiu D , Foley DJ , Murdaugh C , Curb JD ((1996) ) Prevalence of dementia in older Japanese-American men in Hawaii: The Honolulu-Asia Aging Study. JAMA 276: , 955–960. |
[23] | Abbott RD , Ross GW , White LR , Tanner CM , Masaki KH , Nelson JS , Curb JD , Petrovitch H ((2005) ) Excessive daytime sleepiness and subsequent development of Parkinson disease. Neurology 65: , 1442–1446. |
[24] | Ross GW , Abbott RD , Petrovitch H , Morens DM , Grandinetti A , Tung KH , Tanner CM , Masaki KH , Blanchette PL , Curb JD , Popper JS , White LR ((2000) ) Association of coffee and caffeine intake with the risk of Parkinson disease.[see comment]. JAMA 283: , 2674–2679. |
[25] | Ward CD , Gibb WR ((1990) ) Research diagnostic criteria for Parkinson’s disease. Adv Neurol 53: , 245–249. |
[26] | Ross GW , Petrovitch H , Abbott RD , Nelson J , Markesbery W , Davis D , Hardman J , Launer L , Masaki K , Tanner CM , White LR ((2004) ) Parkinsonian signs and substantia nigra neuron density in decendents elders without PD. Ann Neurol 56: , 532–539. |
[27] | He R , Yan X , Guo J , Xu Q , Tang B , Sun Q ((2018) ) Recent advances in biomarkers for Parkinson’s disease. Front Aging Neurosci 10: , 305. |
[28] | Teng EL ((1990) ) The 3RT Test: Three reaction time tasks for IBM PC computers. Behav Res Methods Instrum Comput 22: , 389–392. |
[29] | Pullman SL , Watts RL , Juncos JL , Chase TN , Sanes JN ((1988) ) Dopaminergic effects on simple and choice reaction time performance in Parkinson’s disease. Neurology 38: , 249–254. |
[30] | Kutukcu Y , Marks WJ Jr., Goodin DS , Aminoff MJ ((1999) ) Simple and choice reaction time in Parkinson’s disease. Brain Res 815: , 367–372. |
[31] | Doty RL , Marcus A , Lee WW ((1996) ) Development of the 12-item Cross-Cultural Smell Identification Test (CC-SIT). Laryngoscope 106: , 353–356. |
[32] | Kim HJ , Oh ES , Lee JH , Moon JS , Oh JE , Shin JW , Lee KJ , Baek IC , Jeong SH , Song HJ , Sohn EH , Lee AY ((2012) ) Relationship between changes of body mass index (BMI) and cognitive decline in Parkinson’s disease (PD). Arch Gerontol Geriatr 55: , 70–72. |
[33] | Hu G , Jousilahti P , Nissinen A , Antikainen R , Kivipelto M , Tuomilehto J ((2006) ) Body mass index and the risk of Parkinson disease. Neurology 67: , 1955–1959. |
[34] | Abbott RD , Petrovitch H , White LR , Masaki KH , Tanner CM , Curb JD , Grandinetti A , Blanchette PL , Popper JS , Ross GW ((2001) ) Frequency of bowel movements and the future risk of Parkinson’s disease. Neurology 57: , 456–462. |
[35] | Teng EL , Hasegawa K , Homma A , Imai Y , Larson E , Graves A , Sugimoto K , Yamaguchi T , Sasaki H , Chiu D , et al. ((1994) ) The Cognitive Abilities Screening Instrument (CASI): a practical test for cross-cultural epidemiological studies of dementia. Int Psychogeriatr 6: , 45–58; discussion 62. |
[36] | Jafari S , Etminan M , Aminzadeh F , Samii A ((2013) ) Head injury and risk of Parkinson disease: a systematic review and meta-analysis. Mov Disord 28: , 1222–1229. |
[37] | Ke G , Meng Q , Finley T , Wang T , Chen W , Ma W , Ye Q , Liu T-Y ((2017) ) LightGBM: a highly efficient gradient boosting decision tree. In Proceedings of the 31st International Conference on Neural Information Processing Systems Curran Associates Inc., Long Beach, CA, USA, pp. 3149–3157. |
[38] | Friedman JH ((2001) ) Greedy function approximation: a gradient boosting machine. Ann Stat 29: , 1189–1232. |
[39] | Nogueira F Bayesian Optimization: Open source constrained global optimization tool for Python, GitHub, https://github.com/fmfn/BayesianOptimization, |
[40] | Minastireanu EA , Mesnita G ((2019) ) Light GBM machine learning algorithm to online click fraud detection. J Inform Assur Cybersecur 2019: , 263928. |
[41] | Marini K , Seppi K , Tschiderer L , Kiechl S , Stockner H , Willeit P , Willeit J , Djamshidian A , Rungger G , Poewe W , Mahlknecht P ((2021) ) Application of the updated movement disorder society criteria for prodromal Parkinson’s disease to a population-based 10-year study. Mov Disord 36: , 1464–1466. |
[42] | Fereshtehnejad SM , Montplaisir JY , Pelletier A , Gagnon JF , Berg D , Postuma RB ((2017) ) Validation of the MDS research criteria for prodromal Parkinson’s disease: Longitudinal assessment in a REM sleep behavior disorder (RBD) cohort. Mov Disord 32: , 865–873. |
[43] | Pilotto A , Heinzel S , Suenkel U , Lerche S , Brockmann K , Roeben B , Schaeffer E , Wurster I , Yilmaz R , Liepelt-Scarfone I , von Thaler AK , Metzger FG , Eschweiler GW , Postuma RB , Maetzler W , Berg D ((2017) ) Application of the movement disorder society prodromal Parkinson’s disease research criteria in 2 independent prospective cohorts. Mov Disord 32: , 1025–1034. |
[44] | Postuma RB , Berg D ((2019) ) Prodromal Parkinson’s disease: the decade past, the decade to come. Mov Disord 34: , 665–675. |
[45] | Beach TG , Adler CH , Sue LI , Peirce JB , Bachalakuri J , Dalsing-Hernandez JE , Lue LF , Caviness JN , Connor DJ , Sabbagh MN , Walker DG ((2008) ) Reduced striatal tyrosine hydroxylase in incidental Lewy body disease. Acta Neuropathol 115: , 445–451. |
[46] | DelleDonne A , Klos KJ , Fujishiro H , Ahmed Z , Parisi JE , Josephs KA , Frigerio R , Burnett M , Wszolek ZK , Uitti RJ , Ahlskog JE , Dickson DW ((2008) ) Incidental Lewy body disease and preclinical Parkinson disease. Arch Neurol 65: , 1074–1080. |
[47] | Ross GW , Petrovitch H , Abbott RD , Tanner CM , Popper J , Masaki K , Launer L , White LR ((2008) ) Association of olfactory dysfunction with risk for future Parkinson’s disease. Ann Neurol 63: , 167–173. |
[48] | Bonanni L ((2019) ) The democratic aspect of machine learning: Limitations and opportunities for Parkinson’s disease. Mov Disord 34: , 164–166. |
[49] | Latourelle JC , Beste MT , Hadzi TC , Miller RE , Oppenheim JN , Valko MP , Wuest DM , Church BW , Khalil IG , Hayete B , Venuto CS ((2017) ) Large-scale identification of clinical and genetic predictors of motor progression in patients with newly diagnosed Parkinson’s disease: a longitudinal cohort study and validation. Lancet Neurol 16: , 908–916. |
[50] | Akbilgic O , Kamaleswaran R , Mohammed A , Ross GW , Masaki K , Petrovitch H , Tanner CM , Davis RL , Goldman SM ((2020) ) Electrocardiographic changes predate Parkinson’s disease onset. Sci Rep 10: , 11319. |
[51] | Karabayir I , Goldman SM , Pappu S , Akbilgic O ((2020) ) Gradient boosting for Parkinson’s disease diagnosis from voice recordings. BMC Med Inform Decis Mak 20: , 228. |
[52] | Wang W , Lee J , Harrou F , Sun Y ((2020) ) Early detection of Parkinson’s disease using deep learning and machine learning. IEEE Access 8: , 147635–147646. |
[53] | Stephenson D , Alexander R , Aggarwal V , Badawy R , Bain L , Bhatnagar R , Bloem BR , Boroojerdi B , Burton J , Cedarbaum JM , Cosman J , Dexter DT , Dockendorf M , Dorsey ER , Dowling AV , Evers LJW , Fisher K , Frasier M , Garcia-Gancedo L , Goldsack JC , Hill D , Hitchcock J , Hu MT , Lawton MP , Lee SJ , Lindemann M , Marek K , Mehrotra N , Meinders MJ , Minchik M , Oliva L , Romero K , Roussos G , Rubens R , Sadar S , Scheeren J , Sengoku E , Simuni T , Stebbins G , Taylor KI , Yang B , Zach N ((2020) ) Precompetitive consensus building to facilitate the use of digital health technologies to support Parkinson disease drug development through regulatory science. Digit Biomark 4: , 28–49. |