Technologies Assessing Limb Bradykinesia in Parkinson’s Disease
Abstract
Background: The MDS-UPDRS (Movement Disorders Society – Unified Parkinson’s Disease Rating Scale) is the most widely used scale for rating impairment in PD. Subscores measuring bradykinesia have low reliability that can be subject to rater variability. Novel technological tools can be used to overcome such issues.
Objective: To systematically explore and describe the available technologies for measuring limb bradykinesia in PD that were published between 2006 and 2016.
Methods: A systematic literature search using PubMed (MEDLINE), IEEE Xplore, Web of Science, Scopus and Engineering Village (Compendex and Inspec) databases was performed to identify relevant technologies published until 18 October 2016.
Results: 47 technologies assessing bradykinesia in PD were identified, 17 of which offered home and clinic-based assessment whilst 30 provided clinic-based assessment only. Of the eligible studies, 7 were validated in a PD patient population only, whilst 40 were tested in both PD and healthy control groups. 19 of the 47 technologies assessed bradykinesia only, whereas 28 assessed other parkinsonian features as well. 33 technologies have been described in additional PD-related studies, whereas 14 are not known to have been tested beyond the pilot phase.
Conclusion: Technology based tools offer advantages including objective motor assessment and home monitoring of symptoms, and can be used to assess response to intervention in clinical trials or routine care. This review provides an up-to-date repository and synthesis of the current literature regarding technology used for assessing limb bradykinesia in PD. The review also discusses the current trends with regards to technology and discusses future directions in development.
INTRODUCTION
Bradykinesia is defined by the Queen Square Brain Bank criteria as, “slowness of initiation of voluntary movement with progressive reduction in speed and amplitude of repetitive actions” [1]. Available tools for assessment of bradykinesia are subjective (clinical rating scales) or objective (technology based tools or TBTs). Rating scales for the assessment of bradykinesia include MDS-UPDRS (Movement Disorders Society – Unified Parkinson’s Disease Rating Scale) [2] and MBRS (Modified Bradykinesia Rating Scale) [3]. Although results are reproducible, rating scales such as the MDS-UPDRS are subject to both inter- and intra-rater variability, which is an important source of error in clinical trials [2]. In particular, measures of bradykinesia in the UPDRS part III (motor section) suffer from low reliability [4–7]. Assessing bradykinesia with the UPDRS relies on a variety of factors such as reductions of speed and amplitude, hesitations, motor arrests and fatigue to produce a single score. Differential priority can be placed on individual components by separate raters, and the MBRS was introduced to clarify each component and rate these separately [3]. Items for rating bradykinesia, such as finger tapping, are amongst the most difficult items to rate on scales [8]. It is easier to rate bradykinesia in advanced Parkinson’s disease (PD) compared with mild/moderate PD [9]. Observations about efficacy of treatment depend on clinical examination, records of medication timing, patient’s subjective reporting of symptoms and ability to perform activities of daily living [9]. Identification of symptoms through history-taking can be mired by recall bias, along with difficulty in differentiating normal, dyskinetic and bradykinetic states [10].
TBTs aim to complement existing clinical measures by providing objective, quantifiable scores of motor dysfunction that can be reviewed during routine clinical visits or in the home environment. Bradykinesia severity may fluctuate in response to medication, meaning that what is observed in the clinic may not be indicative of day-to-day function. Objective measurement of bradykinesia enables clinicians to longitudinally monitor patients and assess treatment outcomes leading to adjustment in medication regimens if necessary.
Recent systematic reviews have assessed the clinimetric validity [11] and technological readiness level (TRL) [12] of technologies monitoring PD-related symptoms. Here we systematically explore the options and features of contemporary TBTs (2006–2016) that measure limb bradykinesia. The review aims to provide a repository of current TBTs for evaluating limb bradykinesia in PD. We also discuss current efforts in the development of TBTs and possible future directions.
METHODS
Methodology for review of literature
PRISMA-P (preferred reporting items for systematic review and meta-analysis protocols) guidelines for systematic reviews were followed throughout the study [13]. To ensure a rigorous search, the following databases were used to retrieve relevant studies: PubMed (MEDLINE), Web of Science, Scopus, IEEE Xplore and Engineering Village (Compendex and Inspec). The date of the final search was 18th of October 2016 and only papers written in English were reviewed. A step-by-step guide to the search method using PubMed is provided in Fig. 1. The final search query used in the other databases is included in the supplementary information file (S1).
One author (H.H) screened the articles for eligibility in the bibliography pool of the search engine results. Duplicates found whilst searching the multiple databases were removed using the Systematic Review Assistant De-duplication Module (SRA-DM) [14]. Inclusion and exclusion criteria were applied to the titles and abstracts, and articles were screened accordingly. Full text articles were retrieved for eligible studies and studies whose abstracts did not provide sufficient information for exclusion. References were hand-searched to find studies potentially missed by the search method. An auto-alert function was set in the search feature to provide a notification of new submissions.
Inclusion criteria
Studies that fulfilled the following inclusion criteria were included in the review:
(a) Technology had been used in patients with PD.
(b) Technology assessed limb bradykinesia.
(c) Published in the English language.
(d) Published between 2006–2016.
Technology was defined according to the Oxford dictionary as electronic “machinery and devices developed from scientific knowledge” [15].
Exclusion criteria
Studies were excluded from the review if they met the following criteria:
(a) Technology was tested in non-PD population only.
(b) Technology was used to measure response of an intervention only (e.g. deep brain stimulation, repetitive transcranial magnetic stimulation).
(c) Technology was used to assess patient suitability for advanced therapy referral in PD.
(d) Technology assessed other symptoms in PD but not limb bradykinesia e.g. gait/body bradykinesia, tremor, rigidity, postural imbalance, nocturnal hypokinesia in sleep.
(e) Sample size of PD patients in study was < 5.
(f) Editorials or review papers that did not report new data, and conference proceedings that could not be retrieved or whose abstracts lacked essential information.
(g) Studies that did not use technology (including timed tasks or non-technological tools) for assessment of limb bradykinesia.
(h) Qualitative studies that utilised questionnaires or scales only to assess feasibility of technology in PD.
Data handling
Summary characteristics of eligible technology from the final stage of the selection process were tabulated. (Tables 1 and 2 in supplementary file S1). The following information from eligible studies was extracted: home and/or clinic-based assessment of bradykinesia, type of technology, validation data, use in additional PD studies, assessment of other PD-related features, and types of movements assessed. Studies were also assessed for selection and information bias.
Selection bias was considered possible when:
(a) The PD population selected was not representative of the spectrum of severity of PD (unless specified in the study aims).
(b) PD patients were recruited from movement disorders clinics or hospital in-patients.
(c) There was no mention of where PD patients or controls were recruited from.
Information bias was considered possible when:
(a) Raters were not blinded to both the technology performance and the clinical status of patients.
(b) No mention was made of whether raters were blinded to the patients’ clinical status and technology performance.
RESULTS
1510 potentially eligible articles were found after removal of duplicates. After applying the inclusion criteria 81 eligible studies were included (see Fig. 2).
A summary of TBTs for limb bradykinesia can be seen in Fig. 3.
Type of assessment
Of the 47 technologies assessing bradykinesia in PD, 17 offered home and clinic-based assessment [16–32] and 30 offered clinic/research centre-based assessment only [33–62]. Of the 17 technologies that offered home and clinic based assessment, 6 offered recurrent assessment of bradykinesia in PD [16, 18, 24, 25, 28, 30] and 11 offered cross-sectional assessment only [17, 19–23, 26, 27, 29, 31, 32]. Of the 6 that offered recurrent assessment, 5 measured bradykinesia continuously [16, 18, 25, 28, 30] and one assessed bradykinesia 4 times per day [24]. Of the 30 technologies that offer clinic/research centre-based assessment only, 29 provided cross-sectional assessment [33–53, 55–62] and 1 provided recurrent (4 times daily) assessment [54].
Type of technology used
16 studies utilised IMUs (inertial measurement units – “a self-contained system that measures linear and angular motion usually with a triad of gyroscopes and accelerometers” [63]) for the evaluation of bradykinesia [16, 18, 25, 28, 34, 35, 38, 39, 43, 44, 47, 48, 51–53, 62], 9 utilised smartphones [19, 23, 24, 27, 33, 45, 49, 54, 58], 10 utilised motion analysis systems [21, 22, 32, 42, 46, 50, 55, 57, 59, 60], 4 used computer-based objective assessments [17, 20, 29, 31], two used a combination of the above methods [30, 56] and 6 used other types of technology [26, 36, 37, 40, 41, 61].
Use in other PD studies
33 technologies had been used in additional studies other than the initial proof-of-concept/pilot study [16–22, 24, 25, 28, 30, 34, 36–44, 46, 48, 53–57, 59–62]. No additional reports were found for 14 technologies [21, 24, 27, 29–31, 33, 43, 45, 47–50, 56].
Assessment of other PD-related features in addition to bradykinesia
28 technologies assess PD-related features in addition to bradykinesia [16–19, 21–25, 27–30, 34, 36, 37, 40–42, 44, 47, 49, 53, 55, 57, 58, 60, 62]. Among these, 7 measured dyskinesia [16, 19, 25, 41, 64–66], 16 measured tremor [17, 23, 25, 27, 28, 30, 47, 49, 58, 67–73], 12 assessed gait [20, 23, 24, 29, 46, 66–72], 6 assessed rigidity [29, 36, 47, 58, 74, 75], 2 assessed speech [17, 24], 3 assessed cognition [27, 30, 76], and 3 assessed sleep [30, 77, 78].
Validation
39 out of the 47 eligible technologies were capable of differentiating PD patients from healthy controls [16, 18–22, 24, 26, 28, 29, 31–44, 46–57, 59–61]. 27 technologies were validated through correlation with MDS-UPDRS part III motor scores or sub-scores [16, 18, 20, 23, 27, 28, 31, 33, 36–38, 41–44, 46, 50–58, 60, 79]. 10 studies used classifiers (defined as “assignment of input observation data to a category e.g. diagnostic class” [80]) to study objective performance of the model in predicting MDS-UPDRS part III scores [19, 21, 24, 25, 32, 35, 48, 61, 62, 81]. 4 technologies were validated by comparison with other objective methods (e.g. dot slide method [16], gold-standard motion capture systems [22, 50] and mechanical tappers [33]). 16 studies described clinimetric properties of their respective technology – repeatability [19, 27, 54, 56, 82, 83], responsiveness [54, 83], sensitivity and specificity [16, 20, 24, 31, 36, 37, 46, 48, 52, 54, 58].
Movements assessed
33 of the 47 technologies assessed finger tapping movement [3, 17, 20–22, 24, 25, 27, 28, 31–39, 41–43, 45, 47–55, 57, 61]. 7 technologies measured hand grasping [3, 22, 32, 34, 44, 45, 51] and 14 technologies measured pronation/supination movements [3, 22, 27, 28, 32, 34, 35, 37, 51, 52, 56, 58–60]. With respect to lower limb bradykinesia, only 4 technologies measured toe tapping [18, 22, 35, 84] and 3 measured leg agility [18, 22, 62]. 5 technologies enabled monitoring of bradykinesia continuously during activities of daily living [16, 25, 28, 30, 85]. Other miscellaneous movements measured include spiral drawing [19, 49], pursuit tracking [26, 29], step tracking [26] and finger-to-chin movements [55].
Further information on included studies
40 studies included both PD patients and controls as part of the study [16, 19–22, 24–26, 28, 29, 31–44, 46–61], whereas 7 studies were validated in a PD population only [17, 18, 23, 25, 30, 45, 62]. 2 studies also included patients with atypical parkinsonian syndromes – 1 with PSP (progressive supranuclear palsy) [42] and another both PSP-R (Richardson’s syndrome) and MSA (multiple system atrophy) [53]. 1 study included patients with ET (essential tremor) as well as PD [52].
Of 29 task-based studies, 13 described sequence/learning effects [17, 19, 20, 24, 27, 35, 38, 39, 42, 44, 45, 54, 56]. 8 explored feasibility regarding user compliance and/or acceptability [17, 24, 25, 27, 30, 51, 54, 85]. Only 6 studies described mechanisms to preserve privacy of patient data [17, 24, 25, 41, 45, 53].
Bias
Selection bias was felt to be possible in 24 studies [16, 18, 19, 21–23, 27, 30, 32, 34, 35, 37, 39, 43, 46, 50–52, 55, 56, 58, 59, 61, 62]. Information bias was felt to be possible in 17 studies [16–21, 24, 27, 28, 30, 33, 42, 50, 54, 55, 57, 58, 60].
DISCUSSION
Here we describe a comprehensive list of TBTs which have been used to measure limb bradykinesia in PD. We used a thorough search strategy to identify all relevant technologies and critically appraised each of these in the context of their aim (to measure limb bradykinesia). It is clear that substantial heterogeneity exists between available technologies, not least in the methods they employ, but also the extent to which they have been validated, and also their potential availability and accuracy.
TBTs can augment existing instruments for assessment of bradykinesia in the following ways:
1) Variables generated from subjective scales are ordinal in nature and might not adequately capture motor deterioration. For example, there are multiple potential changes that can occur in seeing progression from a score of 1 on finger tapping to a score of 2 using the UPDRS, or further still from stage 2 to 2.5 on the modified Hoehn and Yahr scale (a separate global rating scale). Many of the TBTs described here generate data on the specific motor impairment and in a continuous quantitative manner rather than on an ordinal scale.
2) For some TBTs, the assessment can be performed remotely by the patient and data can be accessed from a central source by the treating physician. This has the potential to reduce frequency and duration of patient visits [86].
3) TBT’s are not typically subject to the same inter- and intra-rater variability of rating scales and can provide objective, quantitative data in clinical trials to reduce variability between different raters and sites.
4) Clinical trials in the early stages of PD include patients with ratings of 0-1 in motor tasks of the UPDRS [83]. These scores are susceptible to a floor effect, wherein subtle changes as a result of the drug (or placebo) might not be captured. Such a floor effect may have affected results of past clinical trials with disease modifying aims [10].
5) Again, in the research setting, TBT’s may have the potential to identify motor dysfunction prior to current diagnosis, which may in turn be relevant in future clinical trials. For example, in a study of 78 patients with REM sleep behaviour disorder, which is a strong risk factor for a number of neurodegenerative diseases, objective motor dysfunction was observed between 4–8 years prior to diagnosis of parkinsonism [87].
Cross-sectional assessments provide ‘snapshots’ of motor function between clinic visits. Whilst most of the studies included in this review offer cross-sectional assessments (85%), there has been successful implementation of longitudinal assessment in recent years [16, 18, 25, 28, 30].
Longitudinal assessment can help in the early detection of subtle motor change, which may go unnoticed by both physician and patient, especially where it may be transitory in nature [88]. Later in the course of the disease, unpredictable fluctuations in response can occur which are more difficult to manage and determine accurately based on history in clinical consultations, particularly if there is a language barrier between patient and clinician. In addition, if patients happen to be ‘on’ during clinic visits this can influence treatment decisions more strongly than the accompanying history or information obtained from diaries or questionnaires [10]. It has to be stressed that many TBTs in this review (30%) have been validated in small sub-sets of patients with PD and have not been followed up with results from large-scale studies.
TBTs such as SENSE-PARK system, Kinesia system, PKG, Physilog and PERFORM system offer continuous remote monitoring of PD patients, which enables monitoring of fluctuations throughout the day in a “real world” environment i.e. at home or at work, as opposed to cross-sectional assessment. Software Applications (Apps) are now available on smartphones and offer the advantage of testing without purchase of hardware by patients, clinicians or researchers [23, 24, 27, 30, 33, 45, 49, 58]. mPower is an observational study in a large cohort conducted using smartphones, assessing feasibility of remote assessment for tracking daily changes in symptom severity [24, 76]. Data from the study have also been released enabling access by research communities around the world [76].
36% of the TBTs offered home-based monitoring of patients. In addition to the above advantages, home-based testing may also provide opportunities to patients who are otherwise unable to participate in clinical trials due to work or geographical limitations [17, 86]. Home-based monitoring was found to be cost-effective in terms of improvement in functional status, motor severity, and motor complications in a recent prospective study [89]. Whilst home-based testing offers certain advantages, it may be difficult for patients with apathy and/or depression, both of which are common in PD, to find the motivation to complete tasks for remote monitoring. Moreover, testing may be inaccurate if performed without supervision, and therefore patients may need to be observed performing tests, at least at the outset.
The clinical value of technology in addition to routine care in PD has been evaluated in a retrospective analysis of 591 patients using the PKG device [90]. Significant improvements in patient symptoms were observed and maintained over a 6 month period [90, 91]. This suggests that devices such as this can be used successfully in addition to routine care to improve outcome of PD management by guiding interventions. A retrospective study examined the use of wearable Kinesia technology for advanced therapy referral (such as DBS (deep brain stimulation) and LCIG (levodopa carbidopa intestinal gel)) for a year and a half in 40 individuals [92]. It found that use of kinematic data from remote monitoring, in addition to receiving standard care, resulted in higher referral rate in the group of patients whose clinicians had access to monitoring reports in contrast to the group who received standard care alone (63.6% versus 11.8%, p < 0.01) [92].
The majority (57%) of technologies assessing bradykinesia were validated using correlation with the MDS-UPDRS. Considering inter-rater variability in the scale, and that differential priority is placed on rating speed, amplitude and rhythm to produce a single bradykinesia score [3], this represents a clinimetric validation pitfall for objective measures [93]. Moreover, a single cross-sectional rating score might reflect the patient’s motor dysfunction at the time of testing only. The symptoms of PD fluctuate and scores may not correlate well with data obtained from continuous objective monitoring of a patient’s status [16]. The scores on the rating scale may contribute more than objective measures do to the margin of error in correlation coefficients. A device that has higher correlation with the MDS-UPDRS may not necessarily be better than one that shows a weak correlation. In this light, accuracy of a technology can be tested by evaluating the technology’s own clinimetric properties (test-retest repeatability, sensitivity, specificity, responsiveness, feasibility, respondent and administrative burden etc). Other studies utilised classifiers in conjunction to sensors to objectively predict the performance of patients on the bradykinesia subsection of the MDS-UPDRS using machine-learning algorithms [19, 21, 24, 25, 32, 35, 48, 61, 62, 81], validation of motion capture devices against gold-standard motion analysis systems [22, 50], and other objective methods such as dot slide method [16] and mechanical tappers [33] with other technologies.
Limitations
One of the limitations of this review is that a single reviewer (H.H) undertook the search. However, searching for relevant literature was undertaken in five databases and references of eligible studies and rejected reviews were hand-searched to ensure that no studies were missed. Another limitation is that the review explored limb bradykinesia without considering other features of PD. However, limb bradykinesia is a core diagnostic feature of PD and is the most specific. It is important to also note that this field of study is prone to small study bias/publication bias, in which small positive studies are more likely to be published than those with null results. With regards to the level of dissemination of evidence we may not be aware of studies that have failed, or whose negative or non-significant results led to non-publication of research findings.
Future directions
The Internet of Things (IoT) platform describes the shift to the delivery of wearable sensors in terms of diagnostics and treatment, which can be applied to PD (see Fig. 4) [94]. It describes a scenario where the use of wearable technologies would reduce the burden on the current healthcare system, whilst at the same increasing patient engagement and providing personalised care. PD population-wide data could be collected and validated using algorithms and data management systems. Data generated from wearable sensors could be sent via the internet to the physician, enabling adjustment of medications based on objective measures obtained. Patients could access their data and understand what it means, which may in turn increase patient engagement, and reduce burden on health care systems. MercuryLive, PERFORM, HopkinsPD and game-based Health Monitoring System (HMS) are examples of systems that are based on the IoT framework [25, 95–97].
Only 6 out of 47 of studies in this review described privacy of patient’s data. Access to patient’s personal data by third party represents a risk to the successful implementation of the IoT framework and mHealth apps (mHealth defined as “medical and public health practice supported by mobile devices, such as mobile phones, patient monitoring devices, personal digital assistants (PDAs), and other wireless devices” [98]). This may take the form of medical identity theft through hacking or even financial losses [99], the transmission of sensitive information over unencrypted internet, logging of sensitive information, component exposure threats and unencrypted SD (security digital) card storage, all of which raise concerns about secure treatment of health data [100]. In a study of 2,062 respondents regarding consumer perceptions of privacy in the IoT, 52% of respondents described discomfort with companies using data related to their bodies (e.g.: wearables) and 58% described discomfort with companies selling their data [101]. Amongst the various age groups sampled, older segments of the population described more extreme concerns around data privacy [101].
A promising future direction is a shift from open loop data capture to sensor-based closed loop technologies that can deliver treatments (infusion pumps, sensors connected to DBS electrodes) [93]. REMPARK (Personal Health Device for the Remote and Autonomous Management of Parkinson’s Disease) [102] and HELP (Home-based Empowered Living for Parkinson’s Disease patients) [103] are two examples.
CONCLUSION
As technology evolves it is important that clinicians and patients have access to up-to-date information about product availability and use. Regulatory processes must be streamlined to account for rapid evolution providing that concerns around data security are met, and cost effectiveness is incorporated into technology evaluation. Here we have discussed the current state of the art in terms of TBTs that measure bradykinesia, but regular updates will be required to keep abreast of this rapidly changing field. Regular updates to the tables in supplementary file S1 can be accessed at Google drive – http://bit.ly/2bomTIM.
Author roles: (A) Writing the first draft of the manuscript: HH
(B) Review and critique: AN, DA, TF
Financial disclosures/Conflicts of interest
Full financial disclosures of all the authors for the past year: DA and HH no financial disclosures. TF has received research grants from Michael J Fox Foundation, European Union FP7, John Black Charitable Foundation and Brain Research Trust. He has received honoraria for speaking at meetings sponsored by Brittania, Medtronic and UCB, and has served on Advisory boards for Oxford Biomedica & Bial. TF and DA are investigators on the Exenatide-PD study which utilizes the BRAIN test alongside a battery of other assessments. AJN – Salary: Parkinson’s UK, Barts Health NHS Trust. Grants: Parkinson’s UK, Élan/Prothena Pharmaceuticals, GE Healthcare. Shares: LifeLab Ltd. Advisory board: myHealthPal. Honoraria: Global Kinetics Corporation, Henry Stewart Talks, Britannia Pharmaceuticals Ltd. Non-financial: AJN designed the existing version of the BRAIN test which features in this manuscript (www.braintaptest.com) and is the Principal Investigator on the PREDICT-PD study (www.predictpd.com).
Appendices
The supplementary material is available in the electronic version of this article: http://dx.doi.org/10.3233/JPD-160878.
REFERENCES
[1] | Hughes AJ , Daniel SE , Kilford L , & Lees AJ ((1992) ) Accuracy of clinical diagnosis of idiopathic Parkinson’s disease: A clinico-pathological study of 100 cases. J Neurol Neurosurg Psychiatry, 55: , 181–184. |
[2] | Goetz CG , Tilley BC , Shaftman SR , Stebbins GT , Fahn S , Martinez-Martin P , Poewe W , Sampaio C , Stern MB , Dodel R , Dubois B , Holloway R , Jankovic J , Kulisevsky J , Lang AE , Lees A , Leurgans S , LeWitt PA , Nyenhuis D , Olanow CW , Rascol O , Schrag A , Teresi JA , van Hilten JJ , & LaPelle N ((2008) ) Movement Disorder Society-Sponsored Revision of the Unified Parkinson’s Disease Rating Scale (MDS-UPDRS): Scale presentation and clinimetric testing results. Mov Disord, 23: , 2129–2170. |
[3] | Heldman DA , Giuffrida JP , Chen R , Payne M , Mazzella F , Duker AP , Sahay A , Kim SJ , Revilla FJ , & Espay AJ ((2011) ) The modified bradykinesia rating scale for Parkinson’s disease: Reliability and comparison with kinematic measures. Mov Disord, 26: , 1859–1863. |
[4] | Camicioli R , Grossmann SJ , Spencer PS , Hudnell K , & Anger WK ((2001) ) Discriminating mild parkinsonism: Methods for epidemiological research. Mov Disord, 16: , 33–40. |
[5] | Bennett DA , Shannon KM , Beckett LA , Goetz CG , & Wilson RS ((1997) ) Metric properties of nurses’ ratings of parkinsonian signs with a modified Unified Parkinson’s Disease Rating Scale. Neurology, 49: , 1580–1587. |
[6] | Henderson L , Kennard C , Crawford TJ , Day S , Everitt BS , Goodrich S , Jones F , & Park DM ((1991) ) Scales for rating motor impairment in Parkinson’s disease: Studies of reliability and convergent validity. J Neurol Neurosurg Psychiatry, 54: , 18–24. |
[7] | Siderowf A , Mcdermott M , Kieburtz K , Blindauer K , Plumb S , & Shoulson I ((2002) ) Test – Retest Reliability of the Unified Parkinson’s Disease Rating Scale in Patients with Early Parkinson’s Disease: Results from a Multicenter Clinical Trial. Mov Disord, 17: , 758–763. |
[8] | Goetz CG , & Stebbins GT ((2004) ) Assuring interrater reliability for the UPDRS motor section: Utility of the UPDRS teaching tape. Mov Disord, 19: , 1453–1456. |
[9] | Giuffrida JP , & Rapp EJ ((2011) ) Ambulatory and remote monitoring of Parkinson’s disease motor symptoms. In Healthcare Sensor Networks: Challenges toward practical implementation, Lai D, Palaniswami M, Begg R, eds. CRC Press, pp. 247–282. |
[10] | Bergquist F , & Horne M ((2014) ) Can objective measurements improve treatment outcomes in Parkinson’s disease? Eur Neurol Rev, 9: , 27–30. |
[11] | Godinho C , Domingos J , Cunha G , Santos AT , Fernandes RM , Abreu D , Gonçalves N , Matthews H , Isaacs T , Duffen J , Al-Jawad A , Larsen F , Serrano A , Weber P , Thoms A , Sollinger S , Graessner H , Maetzler W , & Ferreira JJ ((2016) ) A systematic review of the characteristics and validity of monitoring technologies to assess Parkinson’s disease. J Neuroeng Rehabil, 13: , 24. |
[12] | Sanchez-Ferro A , Elshehabi M , Godinho C , Salkovic D , Hobert MA , Domingos J , Van Uem JM , Ferreira JJ , & Maetzler W ((2016) ) New methods for the assessment of Parkinson’s disease (2005 to 2015): A systematic review. Mov Disord, 31: , 1283–1292. |
[13] | Shamseer L , Moher D , Clarke M , Ghersi D , Liberati A , Petticrew M , Shekelle P , Stewart L , & Group PP ((2015) ) Preferred reporting items for systematic review and meta-analysis protocols (PRISMA-P) 2015: Elaboration and explanation. BMJ, 7647: , 1–25. |
[14] | Rathbone J , Carter M , Hoffmann T , & Glasziou P ((2015) ) Better duplicate detection for systematic reviewers: Evaluation of Systematic Review Assistant-Deduplication Module. Syst Rev, 4: , 1–6. |
[15] | Technology, Oxford dictionary, Last updated 2016, Accessed on 2016. |
[16] | Griffiths RI , Kotschet K , Arfon S , Xu ZM , Johnson W , Drago J , Evans A , Kempster P , Raghav S , & Horne MK ((2012) ) Automated assessment of bradykinesia and dyskinesia in Parkinson’s disease. J Parkinsons Dis, 2: , 47–55. |
[17] | Goetz CG , Stebbins GT , Wolff D , DeLeeuw W , Bronte-Stewart H , Elble R , Hallett M , Nutt J , Ramig L , Sanger T , Wu AD , Kraus PH , Blasucci LM , Shamim EA , Sethi KD , Spielman J , Kubota K , Grove AS , Dishman E , & Taylor CB ((2009) ) Testing objective measures of motor impairment in early Parkinson’s disease: Feasibility study of an at-home testing device. Mov Disord, 24: , 551–556. |
[18] | Heldman DA , Filipkowski DE , Riley DE , Whitney CM , Walter BL , Gunzler SA , Giuffrida JP , & Mera TO ((2012) ) Automated Motion Sensor Quantification of Gait and Lower Extremity Bradykinesia. In Annual International Conference of the IEEE Engineering in Medicine and Biology Society, pp. 1956–1959. |
[19] | Memedi M , Sadikov A , Groznik V , Žabkar J , Možina M , Bergquist F , Johansson A , Haubenberger D , & Nyholm D ((2015) ) Automatic Spiral Analysis for Objective Assessment of Motor Symptoms in Parkinson’s Disease. Sensors, 15: , 23727–23744. |
[20] | Noyce AJ , Nagy A , Acharya S , Hadavi S , Bestwick JP , Fearnley J , Lees AJ , & Giovannoni G ((2014) ) Bradykinesia-Akinesia Incoordination test: Validating an online keyboard test of upper limb function. PLoS One, 9: , e96260. |
[21] | Khan T , Nyholm D , Westin J , & Dougherty M ((2014) ) A computer vision framework for finger-tapping evaluation in Parkinson’s disease. Artif Intell Med, 60: , 27–40. |
[22] | Galna B , Barry G , Jackson D , Mhiripiri D , Olivier P , & Rochester L ((2014) ) Accuracy of the Microsoft Kinect sensor for measuring movement in people with Parkinson’s disease. Gait Posture, 39: , 1062–1068. |
[23] | Kassavetis P , Saifee TA , Roussos G , Drougkas L , Kojovic M , Rothwell JC , Edwards MJ , & Bhatia KP ((2016) ) Developing a tool for remote digital assessment of Parkinson’s disease. Mov Disord Clin Pract, 3: , 59–64. |
[24] | Arora S , Venkataraman V , Zhan A , Donohue S , Biglan KM , Dorsey ER , & Little MA ((2015) ) Detecting and monitoring the symptoms of Parkinson’s disease using smartphones: A pilot study. Park Relat Disord, 21: , 650–653. |
[25] | Tzallas AT , Tsipouras MG , Rigas G , Tsalikakis DG , Karvounis EC , Chondrogiorgi M , Psomadellis F , Cancela J , Pastorino M , Waldmeyer MT , Waldmeyer A , Konitsiotis S , & Fotiadis DI ((2014) ) PERFORM: A system for monitoring, assessment and management of patients with Parkinson’s disease. Sensors, 14: , 21329–21357. |
[26] | Allen DP , Playfer JR , Aly NM , Duffey P , Heald A , Smith SL , & Halliday DM ((2007) ) On the Use of Low-Cost Computer Peripherals for the Assessment of Motor Dysfunction in Parkinson’s Disease — Quantification of Bradykinesia Using Target Tracking Tasks. IEEE Trans Neural Syst Rehabil Eng, 15: , 286–294. |
[27] | Lee W , Evans A , & Williams DR ((2016) ) Validation of a Smartphone application measuring motor function in Parkinson’s disease. J Parkinsons Dis, 6: , 371–382. |
[28] | Salarian A , Russmann H , Wider C , Burkhard PR , Vingerhoets FJ , & Aminian K ((2007) ) Quantification of Tremor and Bradykinesia in Parkinson’s Disease Using a Novel Ambulatory Monitoring System. IEEE Trans Biomed Eng, 54: , 313–322. |
[29] | Cunningham LM , Nugent CD , Moore G , Finlay DD , & Craig D ((2012) ) Computer-based assessment of movement difficulties in Parkinson’s disease. Comput Methods Biomech Biomed Engin, 15: , 1081–1092. |
[30] | Ferreira JJ , Godinho C , Santos AT , Domingos J , Abreu D , Lobo R , Gonçalves N , Barra M , Larsen F , Fagerbakke Ø , Akeren I , Wangen H , Serrano JA , Weber P , Thoms A , Meckler S , Sollinger S , van Uem J , Hobert MA , Maier KS , Matthew H , Isaacs T , Duffen J , Graessner H , & Maetzler W ((2015) ) Quantitative home-based assessment of Parkinson’s symptoms: The SENSE-PARK feasibility and usability study. BMC Neurol, 15: , 89. |
[31] | Giancardo L , Sanchez-Ferro A , Arroyo-Gallego T , Butterworth I , Mendoza CS , Montero P , Matarazzo M , Obeso JA , Gray ML , & San José Estépar R ((2016) ) Computer keyboard interaction as an indicator of early Parkinson’s disease. Sci Rep, 6: , 34468. |
[32] | Dror B , Yanai E , Frid A , Peleg N , Goldenthal N , Schlesinger I , Hel-Or H , & Raz S ((2014) ) Automatic Assessment of Parkinson’s Disease From Natural Hands Movements Using 3D Depth Sensor. In 28th IEEE Convention of Electrical and Electronics Engineers in Israel, pp. 1–5. |
[33] | Lee CY , Kang SJ , Hong SK , Ma H , Lee U , & Kim YJ ((2016) ) A validation study of a smartphone-based finger tapping application for quantitative assessment of bradykinesia in Parkinson’s disease. PLoS One, 11: , e158852. |
[34] | Jia X , Duroseau N , Chan V , Ciraco C , Wang R , Nia SM , Ho K , Govindavari JP , Delgosha F , Chan T , Pergament KM , Krishnamachari B , & Farajidavar A ((2014) ) Objective Quantification of Upper Extremity Motor Functions in Unified Parkinson’s Disease Rating Scale Test. In Annual International Conference of the IEEE Engineering in Medicine and Biology Society, pp. 5345–5348. |
[35] | Martinez-Manzanera O , Roosma E , Beudel M , Borgemeester R , van Laar T , Maurits N ((2016) ) A Method for Automatic and Objective Scoring of Bradykinesia Using Orientation Sensors and Classification Algorithms. IEEE Trans Biomed Eng, 63: , 1016–1024. |
[36] | Bronte-Stewart HM , Ding L , Alexander C , Zhou Y , & Moore GP ((2000) ) Quantitative Digitography (QDG): A Sensitive Measure of Digital Motor Control in Idiopathic Parkinson’s Disease. Mov Disord, 15: , 36–47. |
[37] | Papapetropoulos S , Katzen HL , Scanlon BK , Guevara A , Singer C , & Levin BE ((2010) ) Objective quantification of neuromotor symptoms in Parkinson’s disease: Implementation of a portable, computerized measurement tool. Parkinsons Dis, 2010: , 1–6. |
[38] | Yokoe M , Okuno R , Hamasaki T , Kurachi Y , Akazawa K , & Sakoda S ((2009) ) Opening velocity, a novel parameter, for finger tapping test in patients with Parkinson’s disease. Park Relat Disord, 15: , 440–444. |
[39] | Kandori A, Yokoe M, Sakoda S, Abe K, Miyashita T, Oe H, Naritomi H, Ogata K, & Tsukada K. Quantitative magnetic detection of finger movements in patients with Parkinson’s disease. Neurosci Res, 49: , 253–260. |
[40] | Meigal AI , Rissanen S , Tarvainen MP , Karjalainen PA , Iudina-Vassel IA , Airaksinen O , & Kankaanpää M ((2009) ) Novel parameters of surface EMG in patients with Parkinson’s disease and healthy young and old controls. J Electromyogr Kinesiol, 19: , 206–213. |
[41] | Maetzler W , Ellerbrock M , Heger T , Sass C , Berg D , & Reilmann R ((2015) ) Digitomotography in Parkinson’s Disease: A cross-sectional and longitudinal study. PLoS One, 10: , e0123914. |
[42] | Ling H , Massey LA , Lees AJ , Brown P , & Day BL ((2012) ) Hypokinesia without decrement distinguishes progressive supranuclear palsy from Parkinson’s disease. Brain, 135: , 1141–1153. |
[43] | Kim JW , Lee JH , Kwon Y , Kim CS , Eom GM , Koh SB , Kwon DY , & Park KW ((2011) ) Quantification of bradykinesia during clinical finger taps using a gyrosensor in patients with Parkinson’s disease. Med Biol Eng Comput, 49: , 365–371. |
[44] | Dai H , Lin H , & Lueth TC ((2015) ) Quantitative assessment of parkinsonian bradykinesia based on an inertial measurement unit. Biomed Eng Online, 14: , 68. |
[45] | Printy BP , Renken LM , Herrmann JP , Lee I , Johnson B , Knight E , Varga G , & Whitmer D ((2014) ) Smartphone Application for Classification of Motor Impairment Severity in Parkinson’s Disease. In Annual International Conference of the IEEE Engineering in Medicine and Biology Society, pp. 2686–2689. |
[46] | Bettray LM , Eggers C , Quatuor E , Florin E , Reck C , Pauls AK , Barbe MT , Fink GR , & Timmermann L ((2013) ) Discontinuities in slow finger movements in patients with Parkinson’s disease. Neurosci Lett, 548: , 10–14. |
[47] | Niazmand K , Tonn K , Kalaras A , Fietzek UM , Mehrkens JH , & Lueth TC ((2011) ) Quantitative Evaluation of Parkinson’s Disease using sensor based smart Glove. In 24th International Symposium on Computer-Based Medical Systems. |
[48] | Stamatakis J , Ambroise J , Crémers J , Sharei H , Delvaux V , Macq B , & Garraux G ((2013) ) Finger Tapping Clinimetric Score Prediction in Parkinson’s Disease Using Low-Cost Accelerometers. Comput Intell Neurosci, 2013: , 1–13. |
[49] | Graca R , Sarmento e Castro R , & Cevada J ((2014) ) ParkDetect: Early diagnosing Parkinson’s Disease. In 2014 IEEE International Symposium on Medical Measurements and Applications (MeMeA), pp. 1–6. |
[50] | Krupicka R , Szabo Z , Viteckova S , & Ruzicka E ((2014) ) Motion capture system for finger movement measurement in Parkinson disease. Radioengineering, 23: , 659–664. |
[51] | Rovini E , Esposito D , Maremmani C , Bongioanni P , & Cavallo F ((2014) ) Using wearable sensor systems for objective assessment of Parkinson’s disease. In 20th IMEKO TC4 International Symposium and 18th International Workshop on ADC Modelling and Testing, pp. 862–867. |
[52] | Costa J , Gonzalez HA , Valldeoriola F , Gaig C , Tolosa E , & Valles-Sole J ((2010) ) Nonlinear dynamic analysis of oscillatory repetitive movements in Parkinson’s disease and essential tremor. Mov Disord, 25: , 2577–2586. |
[53] | Djurić-Jovičić M , Petrovic I , Ječmenica-Lukić M , Radovanovic S , Dragašević-Mišković N , Belić M , Miler-Jerkovic V , Popovic MB , Kostic VS ((2016) ) Finger tapping analysis in patients with Parkinson’s disease and atypical parkinsonism. J Clin Neurosci, 30: , 49–55. |
[54] | Memedi M , Khan T , Grenholm P , Nyholm D , & Westin J ((2013) ) Automatic and objective assessment of alternating tapping performance in Parkinson’s disease. Sensors, 13: , 16965–16984. |
[55] | Espay AJ , Beaton DE , Morgante F , Gunraj CA , Lang AE , & Chen R ((2009) ) Impairments of speed and amplitude of movement in Parkinson’s disease: A pilot study. Mov Disord, 24: , 1001–1008. |
[56] | Delrobaei M , Tran S , Gilmore G , McIsaac K , & Jog M ((2016) ) Characterization of multi-joint upper limb movements in a single task to assess bradykinesia. J Neurol Sci, 368: , 337–342. |
[57] | Bologna M , Leodori G , Stirpe P , Paparella G , Colella D , Belvisi D , Fasano A , Fabbrini G , & Berardelli A ((2016) ) Bradykinesia in early and advanced Parkinson’s disease. J Neurol Sci, 369: , 286–291. |
[58] | Choi JH , Ma H , Kim YJ , & Lee U ((2016) ) Development of an assessment method of forearm pronation / supination motor function based on mobile phone accelerometer data for an early diagnosis of Parkinson’s disease. Int J Biosci Biotechnol, 8: , 1–10. |
[59] | Ghassemi M , Lemieux S , Jog M , Edwards R , & Duval C ((2006) ) Bradykinesia in patients with Parkinson’s disease having levodopa-induced dyskinesias. Brain Res Bull, 69: , 512–518. |
[60] | Koop MM , Shivitz N , & Bronte-Stewart H ((2008) ) Quantitative measures of fine motor, limb, and postural bradykinesia in very early stage, untreated Parkinson’s disease. Mov Disord, 23: , 1262–1268. |
[61] | Lones MA , Alty JE , Lacy SE , Jamieson DRS , Possin KL , Schuff N , & Smith SL ((2013) ) Evolving Classifiers to Inform Clinical Assessment of Parkinson’s Disease. In 2013 IEEE Symposium on Computational Intelligence in Healthcare and e-health (CICARE), pp. 76–82. |
[62] | Giuberti M , Ferrari G , Contin L , Cimolin V , Azzaro C , Albani G , & Mauro A ((2014) ) Linking UPDRS Scores and Kinematic Variables in the Leg Agility Task of Parkinsonians. In 11th International Conference on Wearable and Implantable Body Sensor Networks, 115–120. |
[63] | Inertial Measurement Unit definition, https://www.xsens.com/tags/imu/ (retrieved 13/10/2016). |
[64] | Pulliam CL , Burack MA , Heldman DA , Giuffrida JP , & Mera TO ((2014) ) Motion sensor dyskinesia assessment during activities of daily living. J Parkinsons Dis, 4: , 609–615. |
[65] | Roy SH , Cole BT , Gilmore LD , De Luca CJ , Thomas CA , Saint-Hilaire MM , & Nawab SH ((2013) ) High-resolution tracking of motor disorders in Parkinson’s disease during unconstrained activity. Mov Disord, 28: , 1080–1087. |
[66] | Burkhard PR , Shale H , Langston JW , & Tetrud JW ((1999) ) Quantification of dyskinesia in Parkinson’s disease: Validation of a novel instrumental method. Mov Disord, 14: , 754–763. |
[67] | Giuffrida JP , Riley DE , Maddux BN , & Heldman DA ((2009) ) Clinically deployable kinesia technology for automated tremor assessment. Mov Disord, 24: , 723–730. |
[68] | Papapetropoulos S , Jaqid JR , Sengun C , Singer C , & Gallo BV ((2008) ) Objective monitoring of tremor and bradykinesia during DBS surgery for Parkinson disease. Neurology, 70: , 1244–1249. |
[69] | Sturman MM , Vaillancourt DE , Metman LV , Bakay RA , & Corcos DM ((2004) ) Effects of subthalamic nucleus stimulation and medication on resting and postural tremor in Parkinson’s disease. Brain, 127: , 2131–2143. |
[70] | Dai H , Zhang P , & Lueth TC ((2015) ) Quantitative assessment of parkinsonian tremor based on an inertial measurement unit. Sensors, 15: , 25055–25071. |
[71] | Spyers-Ashby JM , & Stokes MJ ((2000) ) Reliability of tremor measurements using a multidimensional electromagnetic sensor system. Clin Rehabil, 14: , 425–432. |
[72] | Bologna M , Di Biasio F , Conte A , Iezzi E , Modugno N , & Berardelli A ((2015) ) Effects of cerebellar continuous theta burst stimulation on resting tremor in Parkinson’s disease. Park Relat Disord, 21: , 1061–1066. |
[73] | Moore GP , Ding L , & Bronte-stewart HM ((2000) ) Concurrent Parkinson tremors. J Physiol, 529: , 273–281. |
[74] | Powell D , Threlkeld AJ , Fang X , Muthumani A , & Xia R ((2012) ) Amplitude- and velocity-dependency of rigidity measured at the wrist in Parkinson’s disease. Clin Neurophysiol, 123: , 764–773. |
[75] | Dai H , Otten B , Mehrkens JH , & D’Angelo LT ((2013) ) A portable system for quantitative assessment of parkinsonian rigidity. In 35th Annual International Conference of the IEEE Engineering in Medicine and Biology Society, pp. 6591–6594. |
[76] | Bot BM , Suver C , Neto EC , Kellen M , Klein A , Bare C , Doerr M , Pratap A , Wilbanks J , Dorsey ER , Friend SH , & Trister AD ((2016) ) The mPower study, Parkinson disease mobile data collected using ResearchKit. Sci Data, 3: , 1–9. |
[77] | Klingelhoefer L , Rizos A , Sauerbier A , Mcgregor S , Martinez-Martin P , Reichmann H , Horne M , & Chaudhuri KR ((2016) ) Night-time sleep in Parkinson’s disease – the potential use of Parkinson’s KinetiGraph: A prospective comparative study. Eur J Neurol, 23: , 1275–1288. |
[78] | Chahine LM , Kauta SR , Daley JT , Cantor CR , & Dahodwala N ((2014) ) Surface EMG activity during REM sleep in Parkinson’s disease correlates with disease severity. Park Relat Disord, 20: , 766–771. |
[79] | Parisi F , Ferrari G , Giuberti M , Contin L , Cimolin V , Azzaro C , Albani G , & Mauro A ((2015) ) Body-Sensor-Network-Based Kinematic Characterization and Comparative Outlook of UPDRS Scoring in Leg Agility, Sit-to-Stand, and Gait Tasks in Parkinson’s Disease. IEEE J Biomed Heal Informatics, 19: , 1777–1793. |
[80] | Kubota KJ , Chen JA , & Little MA ((2016) ) Machine learning for large-scale wearable sensor data in Parkinson’s disease: Concepts, promises, pitfalls, and futures. Mov Disord, 31: , 1314–1326. |
[81] | Shima K , Tsuji T , Kandori A , Yokoe M , & Sakoda S ((2009) ) Measurement and evaluation of finger tapping movements using log-linearized gaussian mixture networks. Sensors, 9: , 2187–2201. |
[82] | Sano Y , Kandori A , Shima K , Tamura Y , Takagi H , Tsuji T , Noda M , Higashikawa F , Yokoe M , & Sakoda S ((2011) ) Repeatability evaluation of finger tapping device with magnetic sensors. Trans Soc Instrum Control Eng, 47: , 272–281. |
[83] | Heldman DA , Espay AJ , LeWitt PA , & Giuffrida JP ((2014) ) Clinician versus machine: Reliability and responsiveness of motor endpoints in Parkinson’s disease. Park Relat Disord, 20: , 590–595. |
[84] | Kim JW , Kwon Y , Kim YM , Chung HY , Eom GM , Jun JH , Lee JW , Koh SB , Park BK , & Kwon DK ((2012) ) Analysis of lower limb bradykinesia in Parkinson’s disease patients. Geriatr Gerontol Int, 12: , 257–264. |
[85] | Mera TO , Heldman DA , Espay AJ , Payne M , & Giuffrida JP ((2012) ) Feasibility of home-based automated Parkinson’s disease motor assessment. J Neurosci Methods, 203: , 152–156. |
[86] | Dorsey ER , Vlaanderen FP , Engelen LJ , Kieburtz K , Zhu W , Biglan KM , Faber MJ , & Bloem BR ((2016) ) Moving Parkinson Care to the Home. Mov Disord, 31: , 1258–1262. |
[87] | Postuma RB , Lang AE , Gagnon JF , Pelletier A , & Montplaisir JY ((2012) ) How does parkinsonism start? Prodromal parkinsonism motor changes in idiopathic REM sleep behaviour disorder. Brain, 135: , 1860–1870. |
[88] | Marcello M , & Atonini A ((2012) ) Evaluation of Motor Complications: Motor Fluctuations. In Rating Scales in Parkinson’s Disease, Sampaio C, Goetz CG, Schrag A, eds. Oxford University Press, pp. 99–114. |
[89] | Cubo E , Mariscal N , Solano B , Becerra V , Armesto D , Calvo S , Arribas J , Seco J , Martinez A , Zorrilla L , & Heldman D ((2016) ) Prospective study on cost-effectiveness of home-based motor assessment in Parkinson’s disease. J Telemed Telecare, 1–11. |
[90] | Horne M , McGregor S , Lynch P , & Zoellner Y ((2015) ) Objective data in Parkinson’s disease therapy management – A retrospective analysis of the Parkinson’s Kinetigraph (PKG) database. Value Heal, 18: , A685. |
[91] | Horne M , McGregor S , Lynch P , & Zoellner Y ((2015) ) Objective data in Parkinson’s disease management – A retrospective analysis of the Parkinson’s Kinetigraph database. In 18th Annual European Congress. |
[92] | Heldman DA , Giuffrida JP , & Cubo E ((2016) ) Wearable Sensors for Advanced Therapy Referral in Parkinson’s Disease. J Parkinsons Dis, 6: , 631–638. |
[93] | Espay AJ , Bonato P , Nahab FB , Maetzler W , Dean JM , Klucken J , Eskofier BM , Merola A , Horak F , Lang AE , Reilmann R , Giuffrida J , Nieuwboer A , Horne M , Little MA , Litvan I , Simuni T , Dorsey ER , Burack MA , Kubota K , Kamondi A , Godinho C , Daneault JF , Mitsi G , Krinke L , Hausdorff JM , Bloem BR , & Papapetropoulos S ((2016) ) Technology in Parkinson’s Disease: Challenges and Opportunities. Mov Disord, 31: , 1272–1282. |
[94] | Pasluosta CF , Gassner H , Winkler J , Klucken J , & Eskofier BM ((2015) ) An Emerging Era in the Management of Parkinson’s Disease: Wearable Technologies and the Internet of Things. IEEE J Biomed Heal Informatics, 19: , 1873–1881. |
[95] | Chen BR , Patel S , Buckley T , Rednic R , Mcclure DJ , Shih L , Tarsy D , Welsh M , & Bonato P ((2011) ) A Web-Based System for Home Monitoring of Patients With Parkinson’s Disease Using Wearable Sensors. IEEE Trans Biomed Eng, 58: , 831–836. |
[96] | Zhan A , Little MA , Harris DA , Abiola SO , Dorsey ER , Saria S , & Terzis A ((2016) ) High frequency remote monitoring of Parkinson’s disease via smartphone: Platform overview and medication response detection. arXiv 1–12. |
[97] | Medeiros L , Almeida H , Dias L , Perkusich M , & Fischer R ((2016) ) A Game-Based Approach to Monitor Parkinson’s Disease: The bradykinesia symptom classification. In IEEE 29th International Symposium on Computer-Based Medical Systems. |
[98] | Kay M , Santos J , & Takane M ((2011) ) mHealth: New horizons for health through mobile technologies, WHO Global observatory for eHealth (Retrieved from http://www.who.int/goe/publications/goe_mhealth_web.pdf). |
[99] | Carter A , Liddle J , Hall W , & Chenery H ((2015) ) Mobile phones in research and treatment: Ethical guidelines and future directions. JMIR Mhealth Uhealth, 3: , e95. |
[100] | He D , Naveed M , Gunter CA , & Nahrstedt K ((2014) ) Security Concerns in Android mHealth Apps. In AMIA Annual Symposium Proceedings, pp. 645–654. |
[101] | Brands W , Learn C , & Citizenry C ((2015) ) Consumer Perceptions of Privacy in the Internet of Things: Altimeter Group (Retrieved from http://go.pardot.com/l/69102/2015-07-12/pxzlm). |
[102] | Cabestany J , Pérez-López C , Sama A , Moreno JM , Bayes A , Rodriguez-Molinero A ((2013) ) REMPARK: When AI and Technology Meet Parkinson Disease Assessment. In 20th International Conference “Mixed Design of Integrated Circuits and Systems” (MIXDES), pp. 562–567. |
[103] | Ahlrichs C , Samá A , Rovira J , Herrlich S , & Rodríguez-Molinero A ((2013) ) HELP: Optimizing Treatment of Parkinson’s Disease Patients. In 3rd International Conference on Elderly and New Technologies, pp. 17–24. |
Figures and Tables
Fig.1
Flowchart for search methods used in PubMed (MEDLINE) database. See supplementary material for details of other searches.
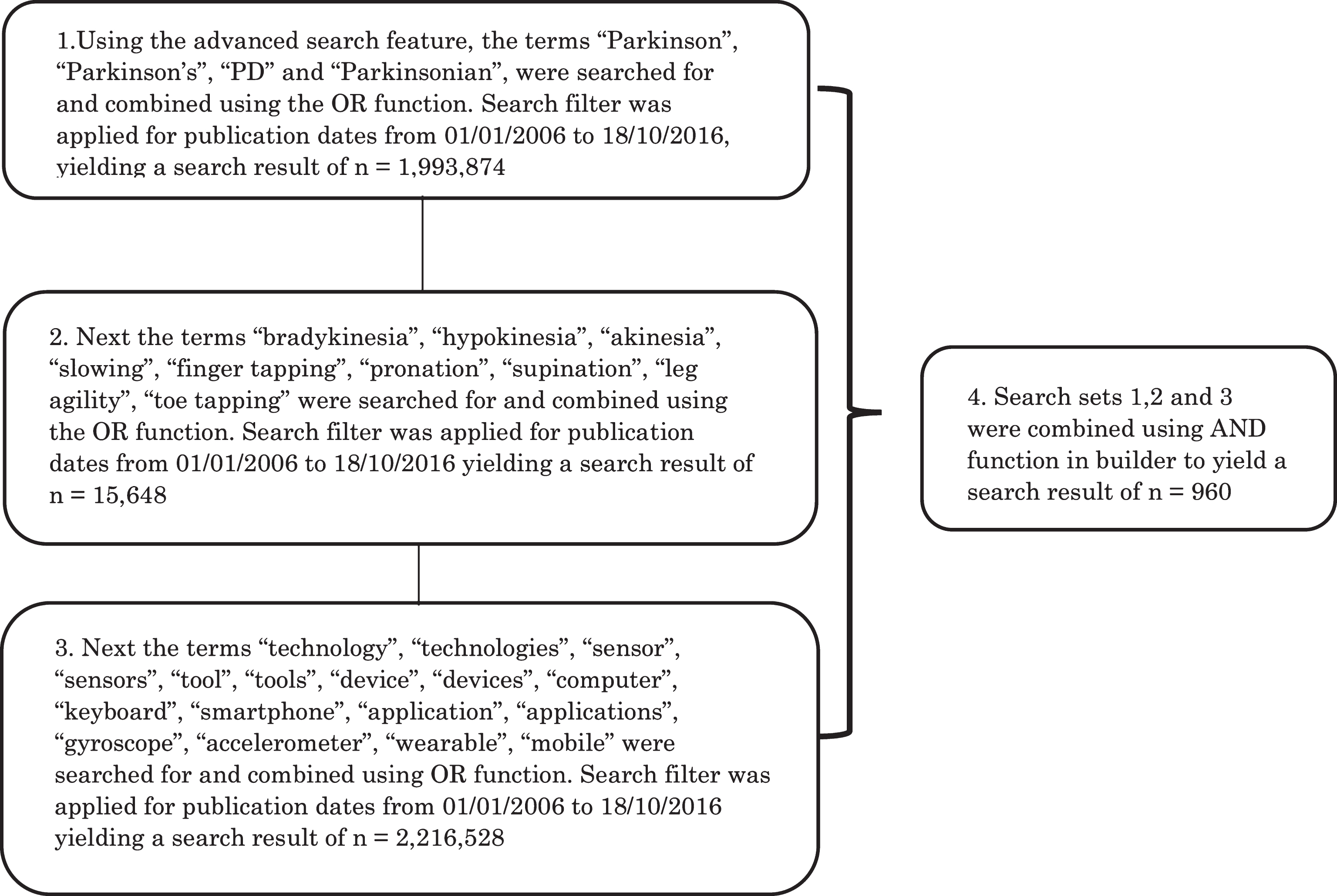
Fig.2
Flowchart for systematic literature search across the database [*PubMed: 960 articles, IEEE Xplore: 132 articles, Web of Science: 598 articles, Scopus: 298 articles, Engineering Village: 78].
![Flowchart for systematic literature search across the database [*PubMed: 960 articles, IEEE Xplore: 132 articles, Web of Science: 598 articles, Scopus: 298 articles, Engineering Village: 78].](https://content.iospress.com:443/media/jpd/2017/7-1/jpd-7-1-jpd160878/jpd-7-jpd160878-g002.jpg)
Fig.3
Summary diagram of measurement tools for limb bradykinesia [MDS-UPDRS – Movement Disorders Society Sponsored Revision of the Unified Parkinson’s Disease Rating Scale, MBRS – Modified Bradykinesia Rating Scale, PKG- Parkinson’s KinetiGraph, AHTD – At Home Testing Device, BRAIN tap test – BRadykinesia Akinesia INcoordination tap test, CV motion analysis – Computer Vision motion analysis, PERFORM – A sophisticated multipaRametric system FOR the continuous effective assessment and Monitoring of motor status in parkinson’s disease and other neurodegenerative diseases, 9DoF sensor – 9 Degrees of Freedom sensor, QDG – Quantitative Digitography, ATP – Alternating Tapping Performance, CATSYS – Co-ordination Ability Testing System, SEMG- Surface electromyography].
![Summary diagram of measurement tools for
limb bradykinesia [MDS-UPDRS – Movement Disorders Society Sponsored Revision of the Unified Parkinson’s
Disease Rating Scale, MBRS – Modified Bradykinesia Rating Scale, PKG- Parkinson’s KinetiGraph, AHTD
– At Home Testing Device, BRAIN tap test – BRadykinesia Akinesia INcoordination tap test, CV motion
analysis – Computer Vision motion analysis, PERFORM – A sophisticated multipaRametric system FOR
the continuous effective assessment and Monitoring of motor status in parkinson’s disease and other
neurodegenerative diseases, 9DoF sensor – 9 Degrees of Freedom sensor, QDG – Quantitative
Digitography, ATP – Alternating Tapping Performance, CATSYS – Co-ordination Ability Testing System, SEMG- Surface electromyography].](https://content.iospress.com:443/media/jpd/2017/7-1/jpd-7-1-jpd160878/jpd-7-jpd160878-g003.jpg)
Fig.4
Diagram describing the Internet of Things (IoT) framework for home-based monitoring of PD using wearable sensors.
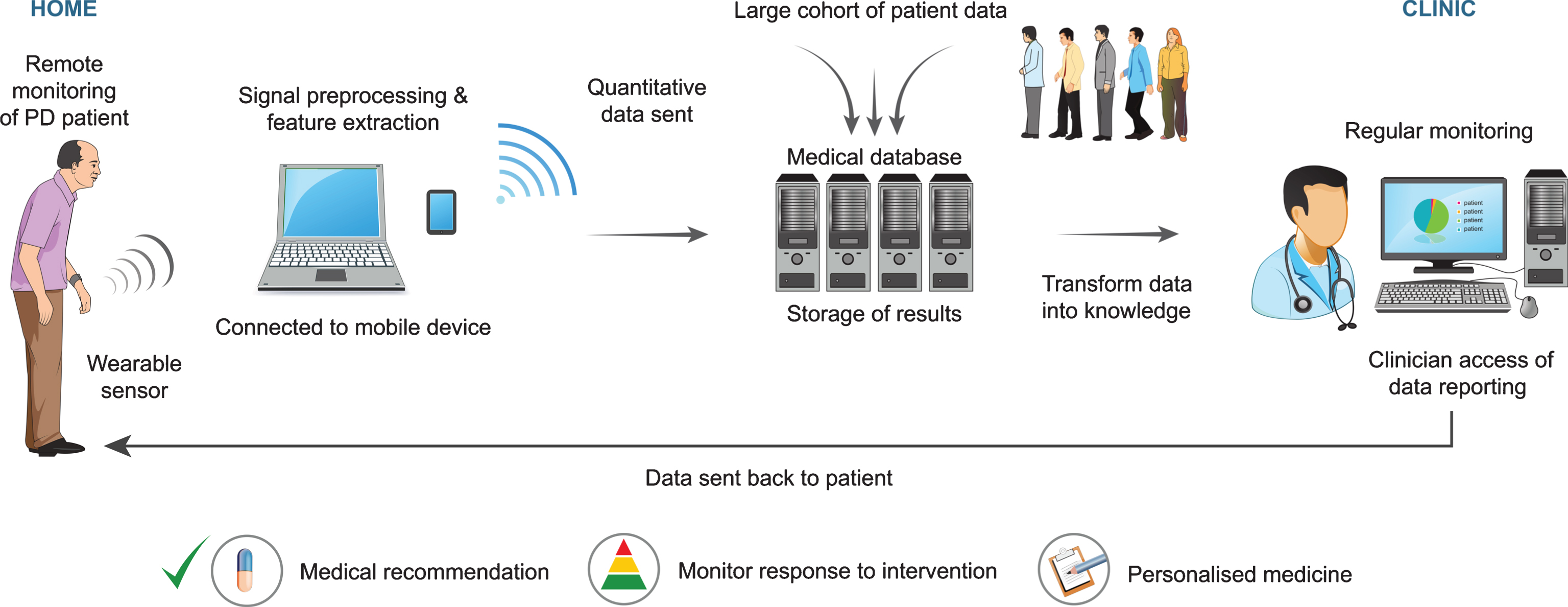