A Viewpoint on Wearable Technology-Enabled Measurement of Wellbeing and Health-Related Quality of Life in Parkinson’s Disease
Abstract
In this viewpoint, we discuss how several aspects of Parkinson’s disease (PD) – known to be correlated with wellbeing and health-related quality of life–could be measured using wearable devices (‘wearables’). Moreover, three people with PD (PwP) having exhaustive experience with using such devices write about their personal understanding of wellbeing and health-related quality of life, building a bridge between the true needs defined by PwP and the available methods of data collection. Rapidly evolving new technologies develop wearables that probe function and behaviour in domestic environments of people with chronic conditions such as PD and have the potential to serve their needs. Gathered data can serve to inform patient-driven management changes, enabling greater control by PwP and enhancing likelihood of improvements in wellbeing and health-related quality of life. Data can also be used to quantify wellbeing and health-related quality of life. Additionally these techniques can uncover novel more sensitive and more ecologically valid disease-related endpoints. Active involvement of PwP in data collection and interpretation stands to provide personally and clinically meaningful endpoints and milestones to inform advances in research and relevance of translational efforts in PD.
INTRODUCTION
There is a growing interest from both people with Parkinson’s disease (PwP) and clinicians to objectively measure disease-related outcomes using wearable devices (‘wearables’) [1]. Wearables have the potential to measure motor function and behaviour continuously beyond the boundaries of a doctor’s office [2]. Monitoring aspects of Parkinson’s disease (PD) over a longer period of time in the domestic environment provides a far more realistic interpretation of both general and disease specific wellbeing while taking into account the variability of symptoms within and between days [3]. Recent studies show that PwP can tolerate the use of wearables over even longer time periods [4, 5].
At present, most of the scientific literature in this field has focused on the assessment of symptoms associated with PD [6]. However, there is no doubt that measuring wellbeing (a state of feeling comfortable, healthy and happy [7]) and Health-Related Quality of Life (HRQoL, which incorporates dimensions of physical, mental, social and role functioning, and includes life satisfaction [8]) are equally, if not more important for PwP. To support this notion with individual experiences and standpoints, this article includes three statements of co-authors (PwP) having exhaustive individual experience with use of wearable devices and with the disease. Moreover, they were involved in the SENSE-PARK project (www.sense-park.eu), which aimed at developing a wearable home-based detection system for PD, with PwP in the driving seat [9]. These statements provide an insight in wellbeing and HRQoL as they are interpreted by individual PwP, and in particular inform about the complexity and uniqueness of every PD.
The evolving field of wearables opens dynamic new options for those with chronic disorders to collect data independently about different aspects of their daily life, wellbeing, and HRQoL as related to the disease, including the interactions thereof.
This viewpoint aims to present examples of the available literature that support the opinion of the authors (including PwP) regarding quantitative measurement of wellbeing and HRQoL in PD in the home environment, as well as possibilities which may develop out of this constellation. It also aims at discussing quantitative assessment approaches of areas that have shown to be strongly associated with wellbeing and HRQoL in PwP, such as physical activity, (societal) participation, sleep quality, autonomic function, coping and stress (Table 1). It then presents examples that support the needs and requirements of PwP to assess wellbeing and HRQoL as objective and continuously as possible at the most important places, including information about how these needs and requirements can potentially be best addressed using currently available techniques, and which aspects may be relevant for future development in this field.
RESULTS
Physical activity
An association has been found between physical activity and HRQoL both in healthy older adults [10], and in PwP [11, 12]. PwP are reported to have a more sedentary lifestyle than older adults without PD [13]. Sedentary lifestyle is associated with worse clinical outcomes [13], and, conversely, physical activity has a positive influence on disease progression [14], presumably through multiple mechanisms including a lower rate of dopaminergic neuron degeneration, improving functioning of motor commands in the basal ganglia, and better control by adaptive mechanisms involving subcortical and cortical areas [14].
Wearables can be worn for weeks [4] and they can measure physical activity accurately [15]. These measurements provide insights into PD-associated physical performance far beyond the boundaries of the doctor’s office. Employed wearables include wrist-mounted accelerometers [16], step activity monitors [15, 17–19], and sensor-units attached to the lower back [13] (Table 1). For example, studies comparing PwP and controls found that PwP have decreased mean activity [16, 20], change of distribution of sedentary behaviour [19], reduced walking time [15, 17], and reduced stepping activity [18]. It is plausible to predict that the use of these wearables by PwP and the data on amount and quality of physical activities they provide could enhance their behavioural awareness and autonomy on wellbeing and HRQoL. Moreover, these data could serve as a ‘pilot domain’ for quantitative assessment in person-centred care [21, 22], where the PwP is, by definition, generating the clinically relevant data and actively involved in the decision-making process [21, 23].
Participation
The World Health Organisation defined ‘participation’ as ‘engagement in a life situation’ [24]. Participation was shown to be of major importance to PwP’s HRQoL [25–27]. Limited participation is the most important shortcoming restricting wellbeing in PwP [28, 29], also confirmed by statement 2. This assumption is also supported by the observation that HRQoL increases with the number of people living in a household [30]. Three factors are acknowledged by PwP to particularly limit participation: (i) negative experiences with social withdrawal due to feeling embarrassed or embarrassing someone else, (ii) the unpredictability of motor (e.g. freezing), as well as non-motor symptoms (e.g. urge incontinence and emotional changes, see also statements 1 and 3), and (iii) loss of energy and strength [28], which is at least partly reflecting fatigue.
Only one study [26] tried to quantify participation in PwP, using the Activity Card Sort [31]. However, this method was partly retrospective and not entirely objective. Inspired by recent developments in technology, such as smart watches that communicate flawlessly with other mobile devices, one can imagine that aspects of participation can soon be measured unobtrusively and objectively. Specifically, in the near future complex networks of multiple sensors and applications can work together in order to create a ‘context aware’ system [32]. An example is shown in Fig. 1.
Such a hypothetical system can provide feedback about aspects of participation, e.g. by interaction of multiple networks. Although such ‘context aware’ systems have their disadvantages, which at present may not be sufficiently addressed (e.g., complex handling, large variety and number of sensors, intrusive or potentially burdensome, data unencrypted), they may offer entirely new options to overcome current limitations in PD-associated participation. With such connection systems, PwP would be able to connect with other PwP with similar disabilities and needs (e.g., through an internet platform), enhancing motivation, autonomy, and intra- and inter-individual engagement.
Sleep quality
Not surprisingly, good sleep patterns are highly associated with wellbeing [33]. Sleep disturbances have been reported to affect as many as 60% [34] to 98% [35] of PwP, and the association between these disturbances and HRQoL has consistently been demonstrated [36, 37]. Moreover, sleep impairment, which may be influenced by dopaminergic treatment [38], predicts lower mood, fatigue, cognitive decline [39] and excessive daytime sleepiness [40].
Sleep patterns in PwP have recently been investigated through a wearable [41]. The study showed that PwP have significantly lower acceleration, amplitude, and duration of axial rotations at night [41]. However, it is not yet known how this relates to wellbeing and HRQoL. The set-up of this study could be expanded to explore the interaction between sleep disturbances (e.g., impaired axial movements, fragmentation of sleep and REM sleep behaviour at night) and daytime wellbeing and HRQoL.
Autonomic function
The autonomic system, which controls various homeostatic processes [42], is often impaired in PD, even before the clinical diagnosis is made [42]. Half of the ten most prevalent non-motor features in PwP are autonomic [43], encompassing cardiovascular (e.g. orthostatic hypotension), gastrointestinal (e.g. drooling, incontinence, constipation) and sexual dysfunction, and problems with thermoregulation (e.g. hypothermia, diaphoresis) [42]. These dysfunctions have been shown to directly affect HRQoL [27, 43–45].
Close monitoring could increase the understanding by PwP of these impairments [20], leading to changes in behaviour and/or treatment. A variety of wearables have recently been developed that allow for autonomic assessment [46, 47]. For example, continuous tracking of cardiovascular function, including heart rate, O2 saturation, and blood pressure [46] as well as skin conductance and skin temperature [47] through a non-invasive wrist based monitor is already possible (Table 1). It must be noted that the relationship between autonomic abnormalities in PD and wellbeing/ HRQoL remain poorly understood. Wearables offer an opportunity to fill this gap.
Statement 1 (Male, 47 years old, diagnosed with PD 19 years ago)
Waking up in the morning, I usually know within seconds whether my day is going to be a really good one or a really bad one. Over the past 15 years, I have, more often than not, started the day on a rowing machine. I always travel the same distance, but the speed with which I can complete the task has varied from my best: 6m6s, to my worst: 12m12s. The precise time it takes me each day depends not on my strength but on the coordination of mind and body. There is no better predictor of how the rest of my day will be than this. Other measures I use are the Wii Fit and golf, both of which measure balance and rhythmicity. The ease and speed with which my first medication starts to work each day is also an important indicator of the extent to which I will be in the ‘off’ state for the rest of the day. Absorption of medication and the ease with which it is allowed to pass through the gut and blood brain barrier has a huge effect on my quality of life.
One other interesting feature of trying to forecast my health is that it becomes increasingly difficult as each day progresses. In other words, I am much more in tune with my body and sensitive to any change in the status quo early in the morning. I feel that I become more detached and more desensitised to myself and my surroundings as the day progresses and I take more and more medication.
My overall wellbeing is defined day to day more by the things that I am incapable of doing than those that I can do. On a good day I am capable of doing most of the things I need to (even if these things have been slightly adapted in some way). Conversely, on a bad day I can become almost completely incapacitated and this inability to complete, or even start tasks, correlates directly to my perception of HRQoL.
My hope is that new technology might allow me to better map the patterns of my wellbeing which in turn might allow me to understand what causes bad days. This kind of information could help me define a ‘regime for living’ which allows my quality of life to be optimised through self-interpretation of objective continuous measures and by adapting my lifestyle accordingly.
Coping and stress
Coping and stress play particularly important roles in chronic disorders such as PD, especially since increased vulnerability to stress is a symptom of PD itself [28]. Good coping strategies increase wellbeing and HRQoL [48, 49]. Conversely, stress reduces wellbeing [50–52] and increases the frequency of psychological and physical comorbidities [51, 53]. These observations hold true for both PwP [54, 55] and their caregivers [56].
Several wearables are currently under development to measure stress in real time [47, 57–60]. See Table 1. For example, one device uses context information – GPS and motion activity–to estimate the level of stress, and extracts information from heart rate, heart rate variability and breath rate [60]. A second uses a combination of electrocardiogram and thoracic electrical bio-impedance [57]. Even though measuring stress in a domestic or remote environment is highly complex and challenging, preliminary data from these devices appear useful and reliable to the user [47, 58]. By integrating the software on commercially available smartphones the data may be further strengthened by regular use (Fig. 1) [58, 59].
The relevance of this development is obvious: more detailed insight into stress levels can lead to behavioural changes, and, consequently, increased wellbeing and HRQoL. Tantalizingly, a recent study has shown that exercises performed on a mobile application to self-manage stress reduces users’ discomfort and stress [51].
Issues
We acknowledge that some pitfalls exist, and some challenges have to be overcome before such technology-based assessment tools are ready for use by a broad PwP community. Two of the main challenges for the field, from a PwP‘s point of view, are discussed here. First, valid and relevant questions such as ‘who has access to collected data?’ and ‘how do I protect my collected data?’ have to be solved. A promising approach could be to include such data into patient-controlled medical records. Examples of solved technical and legal issues regarding digital collection and share of health information across different stakeholders do already exist (for an example see www.patientsknowbest.com). Second, the burden of using relatively bulky wearables continuously over a longer period of time has to be overcome. In case PwP benefit from the provided results by the wearable, the burden seems already acceptable in comparison to the benefits and can increase HRQoL for existing system [5]. However, it is probable that the development of technology-based tools will move the field rapidly forward. As an example, wireless, tiny, implantable and bioresorbable sensors, which can continuously measure motion, temperature, pressure, flow rate, resistance and conductance already exist [61]. Therefore, it is very probable that the design of technology-based tools for the assessment of (practically any kind of) human behaviour will improve in due course, reducing the burden of users.
Statement 2 (Male, 88 years old, diagnosed with PD 15 years ago)
Unlike the expression ‘being well’ which normally refers just to one’s physical condition, its derivative ‘wellbeing’ embraces both physical and mental states – in other words, it is holistic. I recall experiencing wellbeing as a profound feeling of contentment enjoying a fulfilled lifestyle of home, family and occupation undisturbed by severe illness or demanding emotional factors. Being in control gave me self-esteem and confidence – both important elements of wellbeing. But this was before I became aware of symptoms subsequently diagnosed as idiopathic Parkinson’s.
Having endured a progressive combination of motor, non-motor and cognitive symptoms for over 15 years I recognise that I no longer experience my earlier sense of wellbeing and lack the same level of control over my life. My earlier holistic wellbeing and joie de vivre has been degraded to a HRQoL that is frequently interrupted by unpredictable ‘off’ periods which can be both painful and depressing.
I don’t want Parkinson’s to progressively take over or become a preoccupation, but I do need to regain control if I am to retain my present HRQoL. Fortunately, there is a light at the end of this tunnel! How fortunate to be living in a technocratic age with innovative technology that will enable Parkies like me, in collaboration with our usual clinician or medical adviser, achieve better disease control and management 24/7 even within our own home environments. I have been privileged to test drive an early prototype system (SENSE-PARK) of wearable battery powered sensors which record a wide range of symptoms and ancillary information that is then converted by scientifically designed algorithms into comprehensive data from which a PwP can learn more about managing individual medical idiosyncrasies.
How will this help my HRQoL? I expect the design of the chosen system to be capable of measuring critical symptomatic and autonomic elements of my Parkinson’s condition and general health over extended periods and provide reliable data for me and my clinician to plan appropriate treatments that I can respond to and that can benefit me holistically. I believe that a reliable measured perspective of what to expect in the future will free me from constant preoccupation and allow me to concentrate on those activities that support my desired lifestyle.
CONCLUSION
The revolutionary development of using wearables in the domestic environment to measure personal functioning opens entirely new assessment strategies in chronic disorders such as PD. Whereas the initial developments in the field focused on the assessment of symptoms and signs of the disease – which are particularly relevant for researchers and clinicians–the gap on monitoring wellbeing and HRQoL will be filled by devices under development that allow remote, individual and unencumbered collection of data, summarized in real time to PwP, both the generator and recipient of such data. Wellbeing and HRQoL endpoints, of greater relevance to patient-centric outcomes, is expected to assume critical importance in shaping future therapeutic efforts and in monitoring the effects of routine medical care [22, 23]. Through the adoption of wearables, PwP themselves stand to become actively engaged in their care and management, thereby potentially increasing wellbeing and HRQoL.
Statement 3 (Male, 47 years old, diagnosed with PD 13 years ago)
The day to day experiences I have is how I assess my wellbeing and HRQoL. Can I still do all the things I could do yesterday, last week or even last year? I see wellbeing as measureable based on combination of both the physical as well as mental markers. The same could be said of HRQoL. At the end of each day I subconsciously take stock of how the day has passed. I look back at how my body has performed and process that information. The final assessment determines whether my day has been a good one or a bad one, or even somewhere in between. I have noticed that as my Parkinson’s progresses, it does not progress in tiny increments, but surprisingly in large steps. It would be very handy if there was some way of measuring what has happened internally and thus caused this step. As a general rule, I usually find I am at my best in the mornings. The first task of the day is the taking of my medications so as to avoid the onset of the dreaded ‘Dystonia’. You would think that taking the same medication every day would give you the same result, but it is quite the contrary. Everything I eat can affect the uptake of my meds, moreover if I get too hungry it does make my Parkinson’s worse, so I eat smaller meals but more often. If I am tired through a lack of sleep or just by pushing myself too hard then it really has a big impact on my Parkinson’s too. If I have a more active day then it usually keeps my dystonia away but if I spend too much time behind my computer, then I find dystonia visits more often. Combine hunger, tiredness and lack of activity and it usually does not end well. I try to avoid this terrible trilogy of triggers.
Ideally, it would be very handy to have some way of performing an on-going diagnostic of my body throughout the day on a 24/7 basis. This could be achieved via a wearable device or even an implant that measures my on-going condition in a passive way. It would be even better if this device could link to my phone sending me updates, recommendations and medication reminders.
CONFLICT OF INTEREST
The authors report no conflicts of interest concerning the content of this article.
DISCLOSURES
JvU and DS have nothing to disclose.
TI serves on the Editorial Board of the Journal of Parkinson’s Disease.
TI, AL, EB and HM are members of the Cure Parkinson’s Trust. The Cure Parkinson’s Trust has received funding from the European Union. The charity works with all pharmaceutical companies in the sector (most notably UCB, Lundbeck, Teva, AbbVie, Britannia, Medtronic) on a variety of projects as well as a variety of technology groups.
AJE is supported by the NIH (1K23MH092735); has received grant support from CleveMed/Great Lakes Neurotechnologies, and the Michael J Fox Foundation; personal compensation as a consultant/scientific advisory board member for Abbvie, Chelsea Therapeutics, TEVA, Impax, Merz, Pfizer, Acadia, Cynapsus, Solstice Neurosciences, Lundbeck, and USWorldMeds; royalties from Lippincott Williams & Wilkins and Cambridge University Press; and honoraria from Abbvie, UCB, USWorldMeds, Lundbeck, Acadia, the American Academy of Neurology, and the Movement Disorders Society.
WM serves on the editorial board of PLOS ONE, received funding from the European Union, the Michael J. Fox Foundation, Robert Bosch Foundation, Neuroalliance and Janssen, and holds part of a patent for the assessment of dyskinesias (German patent office, 102015220741.2). He received speaker honoraria from GlaxoSmithKline, Abbvie, UCB, Licher MT and Rölke Pharma.
FUNDING
The present research is part of the Seventh Framework EU project Moving beyond (no. 316639).
REFERENCES
[1] | Stamford J , Schmidt P , & Friedl K ((2015) ) What engineering technology could do for quality of life in Parkinson’s disease: A review of current needs and opportunities. IEEE J Biomed Health Inform, 19: , 1862–1872. |
[2] | Ossig C , Antonini A , Buhmann C , Classen J , Csoti I , Falkenburger B , Schwarz M , Winkler J , & Storch A ((2016) ) Wearable sensor-based objective assessment of motor symptoms in Parkinson’s disease. J Neural Trans (Vienna), 123: , 57–64. |
[3] | Maetzler W , Domingos J , Srulijes K , Ferreira JJ , & Bloem BR ((2013) ) Quantitative wearable sensors for objective assessment of Parkinson’s disease. Mov Disord, 28: , 1628–1637. |
[4] | Ferreira JJ , Santos A , Godinho C , Domingos J , Abreu D , Larsen F , Serrano A , Weber P , Thoms A , Meckler S , Sollinger S , vanUem J , Hobert M , Maier K , Matthews HJD , Isaacs T , Duffen J , Graessner H , & Maetzler W ((2015) ) Quantitative 12 weeks home-based assessment of Parkinson‘s symptoms: The SENSE-PARK feasibility and usability study. BMC Neurol, 15: , 89. |
[5] | van Uem JM , Maier K , Hobert M , Santos A , Serrano A , Larsen F , Akeren I , Wangen H , Scheck O , Ferreira J , & Maetzler W ((2015) ) The effect of wearing sensors continuously over 12 weeks on health-related quality of life in patients with Parkinson’s disease. Seville, Spain. International Society of Posture and Gait Research World Congress 2015. |
[6] | Soh SE , Morris ME , & McGinley JL ((2011) ) Determinants of health-related quality of life in Parkinson’s disease: A systematic review. Parkinsonism Relat Disord, 17: , 1–9. |
[7] | Well-being N. ((2015) ) Oxford English Dictionary online. Oxford University Press, http://www.oxforddictionaries.com/us/definition/english/well-being, accessed October 27, 2015. |
[8] | Wood-Dauphinee ((1999) ) Assessing quality of life in clinical research: From where we come and where are we going? J Clin Epimemiol, 52: , 355–363. |
[9] | Serrano JA , Larsen F , Isaacs T , Matthews H , Duffen J , Riggare S , Capitanio F , Ferreira JJ , Domingos J , Maetzler W , & Graessner H ((2015) ) Participatory design in Parkinson’s research with focus on the symptomatic domains to be measured. J Parkinsons Dis, 5: , 187–196. |
[10] | Acree LS , Longfors J , Fjeldstad AS , Fjeldstad C , Schank B , Nickel KJ , Montgomery PS , & Gardner AW ((2006) ) Physical activity is related to quality of life in older adults. Health Qual Life Outcomes, 4: , 37. |
[11] | Soh SE , McGinley JL , Watts JJ , Iansek R , Murphy AT , Menz HB , Huxham F , & Morris ME ((2013) ) Determinants of health-related quality of life in people with Parkinson’s disease: A path analysis. Qual Life Res, 22: , 1543–1553. |
[12] | Ashburn A , Fazakarley L , Ballinger C , Pickering R , McLellan LD , & Fitton C ((2007) ) A randomised controlled trial of a home based exercise programme to reduce the risk of falling among people with Parkinson’s disease. J Neurol Neurosurg Psychiatry, 78: , 678–684. |
[13] | Speelman AD , van Nimwegen M , Borm GF , Bloem BR , & Munneke M ((2011) ) Monitoring of walking in Parkinson’s disease: Validation of an ambulatory activity monitor. Parkinsonism Relat Disord, 17: , 402–404. |
[14] | Speelman AD , van de Warrenburg BP , van Nimwegen M , Petzinger GM , Munneke M , & Bloem BR ((2011) ) How might physical activity benefit patients with Parkinson disease? Nat Rev Neurol, 7: , 528–534. |
[15] | Busse ME , Pearson OR , Van Deursen R , & Wiles CM ((2004) ) Quantified measurement of activity provides insight into motor function and recovery in neurological disease. J Neurol Neurosurg Psychiatry, 75: , 884–888. |
[16] | Whitehead DL , Davies ADM , Playfer JR , & Turnbull CJ ((2008) ) Circadian rest-activity rhythm is altered in Parkinson’s disease patients with hallucinations. Mov Disord, 23: , 1137–1145. |
[17] | Cavanaugh JT , Ellis TD , Earhart GM , Ford MP , Foreman KB , & Dibble LE ((2012) ) Capturing ambulatory activity decline in Parkinson’s disease. J Neurol Phys Ther, 36: , 51–57. |
[18] | Skidmore FM , Mackman CA , Pav B , Shulman LM , Garvan C , Macko RF , & Heilman KM ((2008) ) Daily ambulatory activity levels in idiopathic Parkinson disease. J Rehabil Res Dev, 45: , 1343–1348. |
[19] | Chastin SFM , Baker K , Jones D , Burn D , Granat MH , & Rochester L ((2010) ) The pattern of habitual sedentary behavior is different in advanced Parkinson’s disease. Mov Disord, 25: , 2114–2120. |
[20] | Niwa F , Kuriyama N , Nakagawa M , & Imanishi J ((2011) ) Circadian rhythm of rest activity and autonomic nervous system activity at different stages in Parkinson’s disease. Auton Neurosci, 165: , 195–200. |
[21] | Ekman I , Swedberg K , Taft C , Lindseth A , Norberg A , Brink E , Carlsson J , Dahlin-Ivanoff S , Johansson I-L , Kjellgren K , Lidén E , Öhlén J , Olsson L-E , Rosén H , Rydmark M , & Sunnerhagen K ((2011) ) Person-centered care–ready for prime time. Eur J Cardiovasc Nurs, 10: , 248–251. |
[22] | Aarts JWM , Vennik F , Nelen WLDM , van der Eijk M , Bloem BR , Faber MJ , & Kremer JAM ((2014) ) Personal health communities: A phenomenological study of a new health-care concept. Health Expect, 18: , 2091–2106. |
[23] | van der Eijk M , Nijhuis FAP , Faber MJ , & Bloem BR ((2013) ) Moving from physician-centered care towards patient-centered care for Parkinson’s disease patients. Parkinsonism Relat Disord, 19: , 923–927. |
[24] | WHO, The International Classification of Functioning, Disability and Health, (2006) . |
[25] | Carod-Artal FJ , Vargas AP , & Martinez-Martin P ((2007) ) Determinants of quality of life in Brazilian patients with Parkinson’s disease. Mov Disord, 22: , 1408–1415. |
[26] | Duncan RP , & Earhart GM ((2011) ) Measuring participation in individuals with Parkinson disease: Relationships with disease severity, quality of life, and mobility. Disabil Rehabil, 33: , 1440–1446. |
[27] | Visser M , van Rooden SM , Verbaan D , Marinus J , Stiggelbout AM , & van Hilten JJ ((2008) ) A comprehensive model of health-related quality of life in Parkinson’s disease. J Neurol, 255: , 1580–1587. |
[28] | Thordardottir B , Nilsson MH , Iwarsson S , & Haak M ((2014) ) “You plan, but you never know”–participation among people with different levels of severity of Parkinson’s disease. Disabil Rehabil, 36: , 2216–2224. |
[29] | van Uem JMT , Marinus J , Canning C , van Lummel RC , Dodel R , Liepelt-Scarfone I , Berg D , Morris ME , Maetzler W ((2016) ) Health-related quality of life in patients with Parkinson’s disease - a systematic review based on the ICF model. Neurosci Biobehav Rev, 61: , 26–34. |
[30] | Winter Y , von Campenhausen S , Gasser J , Seppi K , Reese JP , Pfeiffer KP , Botzel K , Oertel WH , Dodel R , & Poewe W ((2010) ) Social and clinical determinants of quality of life in Parkinson’s disease in Austria: A cohort study. J Neurol, 257: , 638–645. |
[31] | Baum CM , & Edwards D ((2008) ) Activity Card Sort, 2nd Ed. AOTA Press, http://myaota.aota.org/shop_aota/prodview.aspx?Type=D&SKU=1247 (accessed October 14, 2015). |
[32] | Jameson A ((2001) ) Modelling both the context and the user. Pers Ubiquitous Comput, 5: , 29–33. |
[33] | Totterdell P , Reynolds S , Parkinson B , & Briner RB ((1994) ) Associations of sleep with everyday mood, minor symptoms and social interaction experience. Sleep, 17: , 466–475. |
[34] | Tandberg E , Larsen JP , & Karlsen K ((1998) ) A community-based study of sleep disorders in patients with Parkinson’s disease. Mov Disord, 13: , 895–899. |
[35] | Lees AJ , Blackburn NA , & Campbell VL ((1988) ) The nighttime problems of Parkinson’s disease. Clin Neuropharmacol, 11: , 512–529. |
[36] | Andreadou E , Anagnostouli M , Vasdekis V , Kararizou E , Rentzos M , Kontaxis T , & Evdokimidis I ((2011) ) The impact of comorbidity and other clinical and sociodemographic factors on health-related quality of life in Greek patients with Parkinson’s disease. Aging Ment Health, 15: , 913–921. |
[37] | Ylikoski A , Martikainen K , Sieminski M , & Partinen M ((2015) ) Parkinson’s disease and insomnia. Neurol Sci, 36: , 2003–2010. |
[38] | Zibetti M , Rizzone M , Merola A , Angrisano S , Rizzi L , Montanaro E , Cicolin A , & Lopiano L (Scand) ((2013) ) Sleep improvement with levodopa/carbidopa intestinal gel infusion in Parkinson disease. Acta Neurol, 127: , e28–e32. |
[39] | Neikrug AB , Maglione JE , Liu L , Natarajan L , Avanzino JA , Corey-Bloom J , Palmer BW , Loredo JS , & Ancoli-Israel S ((2013) ) Effects of sleep disorders on the non-motor symptoms of Parkinson disease. J Clin Sleep Med, 9: , 1119–1129. |
[40] | Larsen JP , & Tandberg E ((2001) ) Sleep disorders in patients with Parkinson’s disease: Epidemiology and management. CNS Drugs, 15: , 267–275. |
[41] | Louter M , Maetzler W , Prinzen J , van Lummel RC , Hobert M , Arends JBAM , Bloem BR , Streffer J , Berg D , Overeem S , & Liepelt-Scarfone I ((2015) ) Accelerometer-based quantitative analysis of axial nocturnal movements differentiates patients with Parkinson’s disease, but not high-risk individuals, from controls. J Neurol Neurosurg Psychiatry, 86: , 32–37. |
[42] | Li K , Reichmann H , & Ziemssen T ((2015) ) Recognition and treatment of autonomic disturbances in Parkinson’s disease. Expert Rev Neurother, 15: , 1189–1203. |
[43] | Gallagher DA , Lees AJ , & Schrag A ((2010) ) What are the most important nonmotor symptoms in patients with Parkinson’s disease and are we missing them? Mov Disord, 25: , 2493–2500. |
[44] | Bach JP , Riedel O , Klotsche J , Spottke A , Dodel R , & Wittchen HU ((2012) ) Impact of complications and comorbidities on treatment costs and health-related quality of life of patients with Parkinson’s disease. J Neurol Sci, 314: , 41–47. |
[45] | Duncan GW , Khoo TK , Yarnall AJ , O’Brien JT , Coleman SY , Brooks DJ , Barker RA , & Burn DJ ((2014) ) Health-related quality of life in early Parkinson’s disease: The impact of nonmotor symptoms. Mov Disord, 29: , 195–202. |
[46] | Thomas SS , Nathan V , Zong C , Soundarapandian K , Shi X , & Jafari R ((2015) ) BioWatch: A non-invasive wrist-based blood pressure monitor that incorporates training techniques for posture and subject variability. IEEE J Biomed Health Inform. doi: 10.1109/JBHI.2015.2458779. |
[47] | Kusserow M , Amft O , & Troster G ((2013) ) Monitoring stress arousal in the wild. IEEE Pervasive Comput, 12: , 28–37. |
[48] | Bucks RS , Cruise KE , Skinner TC , Loftus AM , Barker RA , & Thomas MG ((2011) ) Coping processes and health-related quality of life in Parkinson’s disease. Int J Geriatr Psychiatry, 26: , 247–255. |
[49] | Whitworth SR , Loftus AM , Skinner TC , Gasson N , Barker RA , Bucks RS , & Thomas MG ((2013) ) Personality affects aspects of health-related quality of life in Parkinson’s disease via psychological coping strategies. J Parkinsons Dis, 3: , 45–53. |
[50] | Hammen C ((2005) ) Stress and depression. Annu Rev Clin Psychol, 1: , 293–319. |
[51] | Serino S , Cipresso P , Gaggioli A , Pallavicini F , Cipresso S , Campanaro D , & Riva G ((2014) ) Smartphone for self-management of psychological stress: A preliminary evaluation of positive technology app. Revis Psicopat Psicol Clín, 19: , 253–260. |
[52] | Vrshek-Schallhorn S , Stroud CB , Mineka S , Hammen C , Zinbarg RE , Wolitzky-Taylor K , & Craske MG ((2015) ) Chronic and episodic interpersonal stress as statistically unique predictors of depression in two samples of emerging adults. J Abnorm Psychol, 124: , 918–932. |
[53] | Segerstrom SC , & Miller GE ((2004) ) Psychological stress and the human immune system: A meta-analytic study of 30 years of inquiry. Psychol Bull, 130: , 601–630. |
[54] | Simpson J , Lekwuwa G , & Crawford T ((2014) ) Predictors of quality of life in people with Parkinson’s disease: Evidence for both domain specific and general relationships. Disabil Rehabil, 36: , 1964–1970. |
[55] | Jones CA , Pohar SL , & Patten SB ((2009) ) Major depression and health-related quality of life in Parkinson’s disease. Gen Hosp Psychiatry, 31: , 334–340. |
[56] | Santos-García D , & de la Fuente-Fernández R (Scand) ((2015) ) Factors contributing to caregivers’ stress and burden in Parkinson’s disease. Acta Neurol, 131: , 203–210. |
[57] | Seoane F , Mohino-Herranz I , Ferreira J , Alvarez L , Buendia R , Ayllón D , Llerena C , & Gil-Pita R ((2014) ) Wearable biomedical measurement systems for assessment of mental stress of combatants in real time. Sensors Basel, 14: , 7120–7141. |
[58] | Mohino-Herranz I , Gil-Pita R , Ferreira J , Rosa-Zurera M , & Seoane F (Basel) ((2015) ) Assessment of mental, emotional and physical stress through analysis of physiological signals using smartphones. Sensors (Basel), 15: , 25607–25627. |
[59] | Gaggioli A , Pioggia G , Tartarisco G , Baldus G , Ferro M , Cipresso P , Serino S , Popleteev A , Gabrielli S , Maimone R , & Riva G ((2012) ) A system for automatic detection of momentary stress in naturalistic settings. Stud Health Technol Inform, 181: , 182–186. |
[60] | Tartarisco G , Baldus G , Corda D , Raso R , Arnao A , Ferro M , Gaggioli A , & Pioggia G ((2012) ) Personal Health System architecture for stress monitoring and support to clinical decisions. Comp Comm, 35: , 1296–1305. |
[61] | Kang S-K , Murphy RKJ , Hwang S-W , Lee SM , Harburg DV , Krueger NA , Shin J , Gamble P , Cheng H , Yu S , Liu Z , McCall JG , Stephen M , Ying H , Kim J , Park G , Webb RC , Lee CH , Chung S , Wie DS , Gujar AD , Vemulapalli B , Kim AH , Lee K-M , Cheng J , Huang Y , Lee SH , Braun PV , Ray WZ , & Rogers JA ((2016) ) Bioresorbable silicon electronic sensors for the brain. Nature, 530: , 71–76. |
Figures and Tables
Fig.1
Use of different technical equipment in combination with health-related ‘meta algorithms’ may have the potential to offer novel insights into one’s behaviour including participation, PD-associated features, and the interaction thereof.
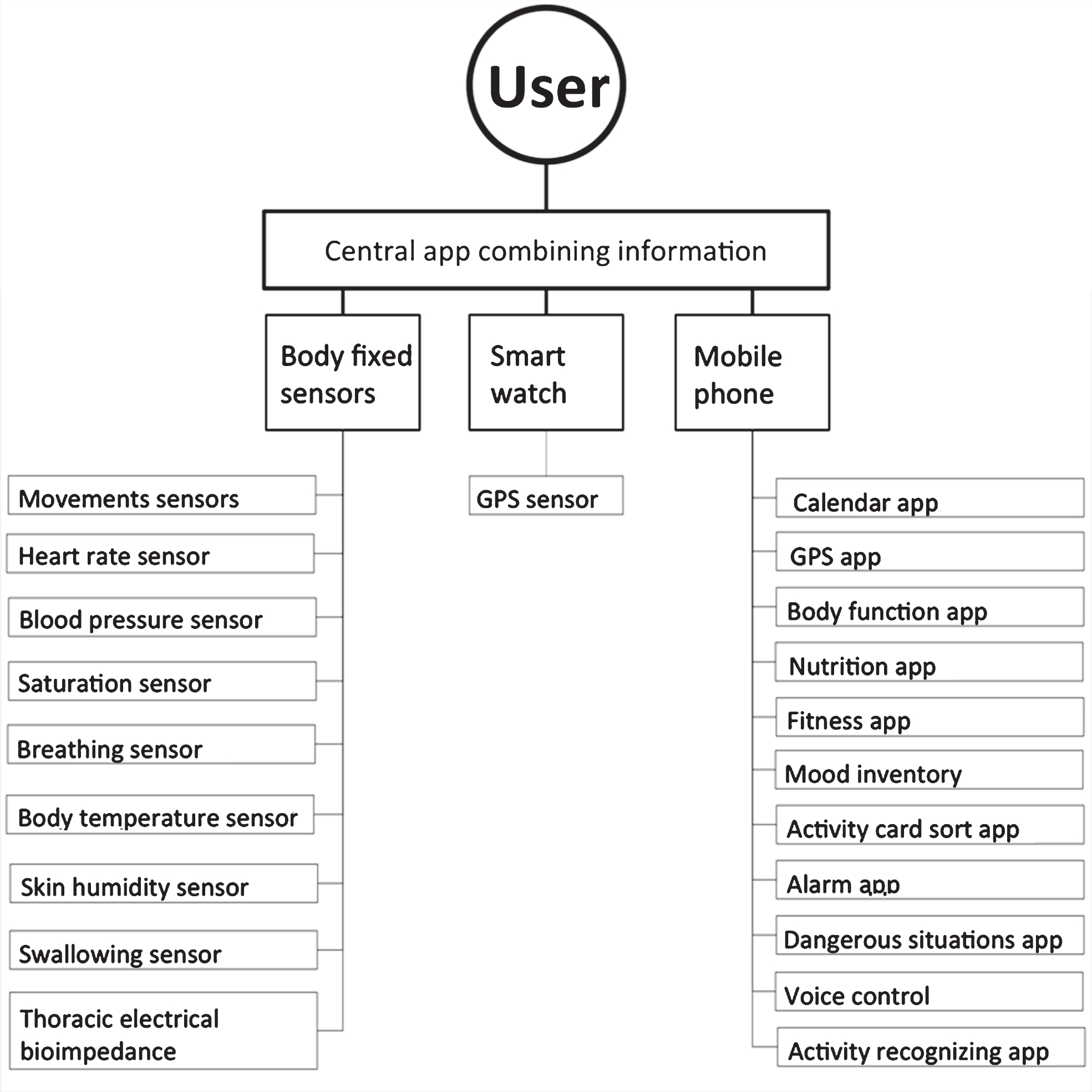
Table 1
An overview of wearables that can measure aspects of Parkinson’s disease that are known to influence wellbeing and HRQoL
PD Aspect | Utilization tool | Measurement | Body placement | Reference |
Physical activity | ||||
Uni-axial accelerometer | Movement | Wrist | [16] | |
Tri-axial accelerometer | Movement | Lower back | [13] | |
Tri-axial accelerometer | Movement | Right lower limb | [15] | |
Tri-axial accelerometer | Movement | Right lower limb | [17] | |
Tri-axial accelerometer | Movement | Right lower limb | [18] | |
Uni-axial accelerometer | Movement | Thigh | [19] | |
(Societal) participation | ||||
Multiple sensors | Multiple modalities | Multiple sites | Not yet available | |
Sleep quality | ||||
Tri-axial accelerometer/ gyroscope | Movement | Lower back | [41] | |
Autonomic function | ||||
Cardio vascular function | Electrocardiogram | Heart rate variability, and ECG-waveform segments | Left wrist | [46] |
Photoplethysmogram | Heart rate, blood oxygen saturation, and blood pressure | Left wrist | [46] | |
Infra red and Red LED’s | Blood oxygenation | Left wrist | [46] | |
Tri-axial accelerometer/ gyroscope/ magnetometer | Movement | Left wrist | [46] | |
Skin conductance/skin temperature | Electrocardiogram | Heart rate monitor | Chest strep | [47] |
Tri-axial accelerometer | Movement | Lower back | [47] | |
Electrodermal activity | Skin conductance values | Wrist | [47] | |
Digital thermometer | Temperature | Wrist | [47] | |
Coping and stress | ||||
Locate the wearer | GPS | Chest strep | [59,60] | |
Tri-axial accelerometer | Movement | Chest strep | [59,60] | |
Electrocardiogram | Heart rate variability, and ECG-waveform segments | Chest strep | [59,60] | |
Electrocardiogram | Heart rate variability, and ECG-waveform segments | Chest strep (textrodes) | [57,58] | |
Thoracic electrical bio-impedance | Cardiogenic biopotentials, thoracic impedance, and breathing movement | Chest strep (textrodes) | [57,58] | |
Wheatstone bridge topology | Skin conductance values/ Galvanic skin response | Hand (glove) | [57,58] | |
Digital thermometer | Skin temperature | Hand (glove) | [57,58] |
A selection of aspects of Parkinson’s disease (PD) that are known to influence wellbeing and health-related-quality of life, and how to measure them using wearable sensors. Summarized are the utilization tools, the typical measurement, and the body placement of the sensors. ECG, electrocardiogram; LED, light-emitting diode.