Assessing Physical Activity Using Accelerometers in Youth with Duchenne Muscular Dystrophy
Abstract
Background:
Physical activity, assessed by accelerometers, has been proposed as a quantitative outcome measure for patients with DMD, but research is limited
Objective:
To assess the total amount and patterns of physical activity in patients with DMD using accelerometers.
Methods:
Physical activity was assessed in patients with DMD (n = 49, 13.6±4.0-year-old) and age- and sex-matched healthy controls (n = 15, 14.0±2.3-year-old) using wrist- and ankle-worn accelerometers. To assess the amount of activity, accelerometer recordings were converted into acceleration estimates (counts/min). Patterns of activity were assessed as the time that participants spent in sedentary, low-intensity, and moderate-to-vigorous physical activity categories. The sedentary category was divided into three (sedentary -1, -2, and -3) and the low-intensity into two (low-intensity-1, and -2) subcategories.
Results:
Physical activity across intensity categories differed between study groups (p < 0.001). Patients with DMD spent on average 98.8% of their daytime in the sedentary and low-intensity categories. Compared to non-ambulatory, ambulatory patients spent more time in sedentary-3 and low-intensity-2 subcategories (p < 0.001). Amount of activity was lower in all patients than controls (p < 0.05) and in non-ambulatory than ambulatory patients and controls (p < 0.001), but similar between ambulatory patients and controls. Activity measures in patients were significantly affected by age and ambulation status (p < 0.05) but not corticosteroid use.
Conclusion:
Patients with DMD spent most of their daytime in sedentary and low-intensity activities. Dividing these intensities into three and two subcategories, respectively, allows better characterization of activity patterns in DMD. Ambulation status and age but not corticosteroid use affected activity measures in patients with DMD.
INTRODUCTION
Duchenne Muscular Dystrophy (DMD) is the most common muscular dystrophy, in which absence or deficiency of dystrophin protein causes progressive muscle breakdown [1]. The rate of DMD progression varies by patient. In general, the progression begins with skeletal muscle weakness, which results in loss of ambulation between ages 8–14 years, followed by death from cardiopulmonary complications [1]. It is therefore clinically relevant to assess muscle function in patients with DMD as a proxy of disease progression [2].
Clinical trials on new therapies for DMD primarily use the 6-Minute Walk Test (6MWT) to assess patients’ muscle function since the test was proved reliable, valid, and sensitive to the natural history of DMD [3–6]. However, non-ambulatory patients with DMD cannot perform the 6MWT, and outcome measures for this non-ambulatory population are also limited [7]. Another validated measure of DMD progression is Quantitative Muscle Testing (QMT) commonly used in research. Still, the results might be influenced by a patient’s physical condition, effort, or familiarity during testing. The Jebsen Hand Function and 9-Hole Peg Tests require sustained concentration, which can challenge patients with DMD who have a relatively high prevalence of neuro-psychological comorbidities [8]. Other commonly used methods like the Brooke Scale, the DMD Functional Ability Self-Assessment Tool (DMDSAT), and the Performance of the Upper Limb (PUL) Scale measure upper extremity function at ordinal levels [9].
Recently accelerometry was proposed as a tool to measure physical activity in patients with DMD of differing ambulation status [7]. Accelerometers are tolerably worn on the wrist, ankle or waist and measure continuous physical activity for several days in the home environment. It has been shown that in ambulatory patients with DMD, accelerometry detected physical activity increases after corticosteroid use, sensed the natural history of DMD, and it was strongly correlated with the 6 MWT [10–12]. In other studies, accelerometry data was strongly correlated with the Brooke Scale, DMDSAT, and PUL Scale in non-ambulatory patients [9, 13]. In patients with DMD of differing ambulation status accelerometry was moderately and strongly correlated with the 6 MWT and QMT, respectively [14, 15].
Research on assessing physical activity via accelerometry in patients with DMD as an objective measure is scarce. Moreover, while physical activity patterns in ambulatory patients have been characterized by step counts [12], no study has characterized physical activity patterns in patients with DMD of differing ambulation status, to our knowledge. The primary goal of this study was to assess total amounts and the patterns of physical activity in patients with DMD using recordings from accelerometers worn on the dominant wrist and ankle for 7 days and 24 hours per day in the natural home environment. The secondary goal was to compare physical activity measures between the patients with DMD and age- and sex-matched healthy controls and between ambulatory and non-ambulatory patients with DMD. The exploratory goal was to examine the effect of ambulation status, age, and corticosteroid use on physical activity measures in patients with DMD.
We hypothesized that patients with DMD would spend most of their daytime in sedentary behaviors and low-intensity physical activity. Our secondary hypothesis was that the amount of physical activity would be higher in ambulatory than non-ambulatory patients with DMD and lower in all patients with DMD than healthy controls.
MATERIALS AND METHODS
Participants
This study included 49 patients with DMD recruited through the Neuromuscular Cardiology Clinic and Muscular Dystrophy Association at Vanderbilt Children’s Hospital. Inclusion criteria were a clinical phenotype of DMD and diagnosis with muscle biopsy or genetic testing of the dystrophin gene. Exclusion criteria were an additional medical diagnosis that could affect physical activity or inability to wear an accelerometer on the dominant wrist and ankle for 7 days.
This study also included 5 age- and sex-matched healthy controls, recruited for this study, and 10 age- and sex-matched healthy controls (with no Crohn’s Disease) from an accelerometry study conducted at the same time on physical activity in youth with Crohn’s Disease. Inclusion criteria were being healthy, male, and aged 8–24 years. Exclusion criteria were a medical diagnosis or genetic condition that could affect physical activity, inability to wear an accelerometer on the dominant wrist for 7 days, or participation in active extracurricular sports activities.
Demographics, current and prior medications, past medical history, and ambulation status (ambulatory or non-ambulatory) were acquired from participants’ medical records at each visit. The Vanderbilt Institutional Review Board approved the study, protocol, and consent forms. Written informed consent was obtained from participants over 18 years of age. For participants under 18 years of age, written consent was obtained from parents and written assent from participants.
Anthropometric measures and demographic information
Participants’ height, weight, body mass index (BMI), and age were determined at each visit. The height of healthy controls and ambulatory patients with DMD was measured to the nearest 1 cm using a calibrated portable stadiometer. The height of non-ambulatory patients with DMD was rounded to the nearest 1 cm from height in their medical records obtained in the last 12 months. Body weight was measured to the nearest 0.1 kg using a calibrated scale. BMI was calculated as a ratio of weight (kg) and height (m2). Chronologic age was calculated by subtracting a participant’s birth date from their start date for wearing an accelerometer.
Participants’ height-for-age, weight-for-age, and BMI-for-age Z-scores were calculated using CDC growth charts of males between 2 and 20 years of age [16]. We were unable to calculate Z-scores for three non-ambulatory patients with DMD older than 20 years.
Amount of physical activity
At the study visit, patients with DMD were instructed to wear an Actigraph GT3X accelerometer (Actigraph, Pensacola FL, USA) on their dominant wrist and ankle for 7 days and 24 hours per day. Since the study’s primary interest was the collection of movement data from the wrist-worn accelerometers as a primary outcome measure, healthy controls were asked to wear an accelerometer only on their wrist. After the monitoring period, accelerometers were mailed to the laboratory in a provided stamped envelope for analysis.
Actigraph GT3X accelerometers recorded acceleration in three orthogonal axes (x, y, z) at 30 Hz (i.e., 30 recordings per second per axis). Accelerometer recordings were uploaded to ActiLife software (Actigraph, Pensacola FL, USA, version 6.13.3), integrated into 15-second epochs, and converted into an omnidirectional acceleration estimate, or vector magnitude (VM), calculated as the square root of the sum of the triaxial signals squared, or √(x2 + y2 + z2). Accelerometer wear and non-wear periods were identified with Choi’s algorithm [17]. A participant’s accelerometer recordings were considered valid if they included ≥3 days with ≥2 weekdays and ≥1 weekend day, each with ≥10 hours of wear from 6 : 00 am to 9 : 00 pm. Participants were assessed as awake for their accelerometer recordings between 6 : 00 am and 9 : 00 pm.
The adherence and physical activity measures calculated for the wrist and ankle accelerometers of patients with DMD and the wrist accelerometer of healthy controls included minutes per day of wearing an accelerometer (min/day wear), minutes per day of wearing and awake (min/day awake), VM’s generated while wearing (VM total), VM’s generated per minute while wearing (VM/min wear), and VM’s generated per minute while wearing and awake (VM/min awake).
For patients with DMD, wrist-to-ankle ratios of VM total, VM/min wear, and VM/min awake were also calculated.
Patterns of physical activity
Wrist accelerometer recordings were integrated into 1-minute epochs and used to determine the time awake that participants spent in activity intensity categories that were previously validated in healthy youth and adolescents including sedentary, low-intensity, and moderate-to-vigorous physical activity or MVPA (Table 1) [18, 19].
Table 1
Activity intensity categories and cut-points in VM/min
Intensity category | VM/min | MET* | Example activities* |
Sedentary | 0–3660 | 1.0–2.0 | Computer or video games while sitting (e.g. handheld devices, mobile phone), lying, playing or doing schoolwork while sitting (e.g. board games, reading, singing, talking, writing), standing, watching TV |
Sedentary-1 | 0–119 | ||
Sedentary-2 | 120–1000 | ||
Sedentary-3 | 1001–3660 | ||
Low-Intensity | 3661–9804 | 2.0–3.5 | Active video games of upper body (e.g. bowling, driving simulation), hand weight exercises, housekeeping (e.g. bed making, dressing, laundry, setting the table), walking |
Low-intensity-1 | 3661–4912 | ||
Low-intensity-2 | 4913–9804 | ||
Moderate-to-vigorous physical activity | >9804 | >3.5 | Active video games of full body (e.g. air hockey, Wii sports), dancing, jogging, playing a sport or ballgame (e.g. basketball, bowling, frisbee, soccer, tennis, volleyball), riding a bike, running, swimming, vacuuming, walking stairs |
*Example activities and metabolic equivalents (MET) for sedentary, low, and moderate-to-vigorous intensity categories were obtained from the Youth Compendium of Physical Activities.
Initial data analysis showed that patients with DMD spent most of their time awake (∼99%) in the sedentary and low-intensity categories. To improve the characterization of physical activity patterns in patients with DMD, we divided the sedentary category into three subcategories named sedentary-1, sedentary-2, sedentary-3, and the low-intensity category into two subcategories named low-intensity-1 and low-intensity-2. The rationale was based on visual inspection of the accelerometer data showing distinct patterns of activity within the intensity categories (Fig. 1). We defined and calculated cut-points for the subcategories as follows. First, for each patient with DMD, we sequentially sorted the VM’s generated from each 15-second epoch while awake from low-to-high. Second, we divided data from 0-915 VM/15 s (sedentary behaviors) into equal thirds and data from 916-2451 VM/15 s (low-intensity activity) into equal halves. Third, patient cut-points were averaged to calculate the subcategory cut-points in VM/15 s. We calculated subcategory cut-points in VM/15 sec to improve the estimation of each value but expressed subcategory cut-points in VM/min since these units are more clinically relevant (Table 1).
Fig.1
Patterns of physical activity in patients with DMD using wrist-worn accelerometers (A) Distribution of physical activity across all intensity categories (B) Distribution of physical activity in sedentary behaviors (C) Distribution of physical activity in low-intensity activity (D) Distribution of physical activity in moderate-to-vigorous physical activity.
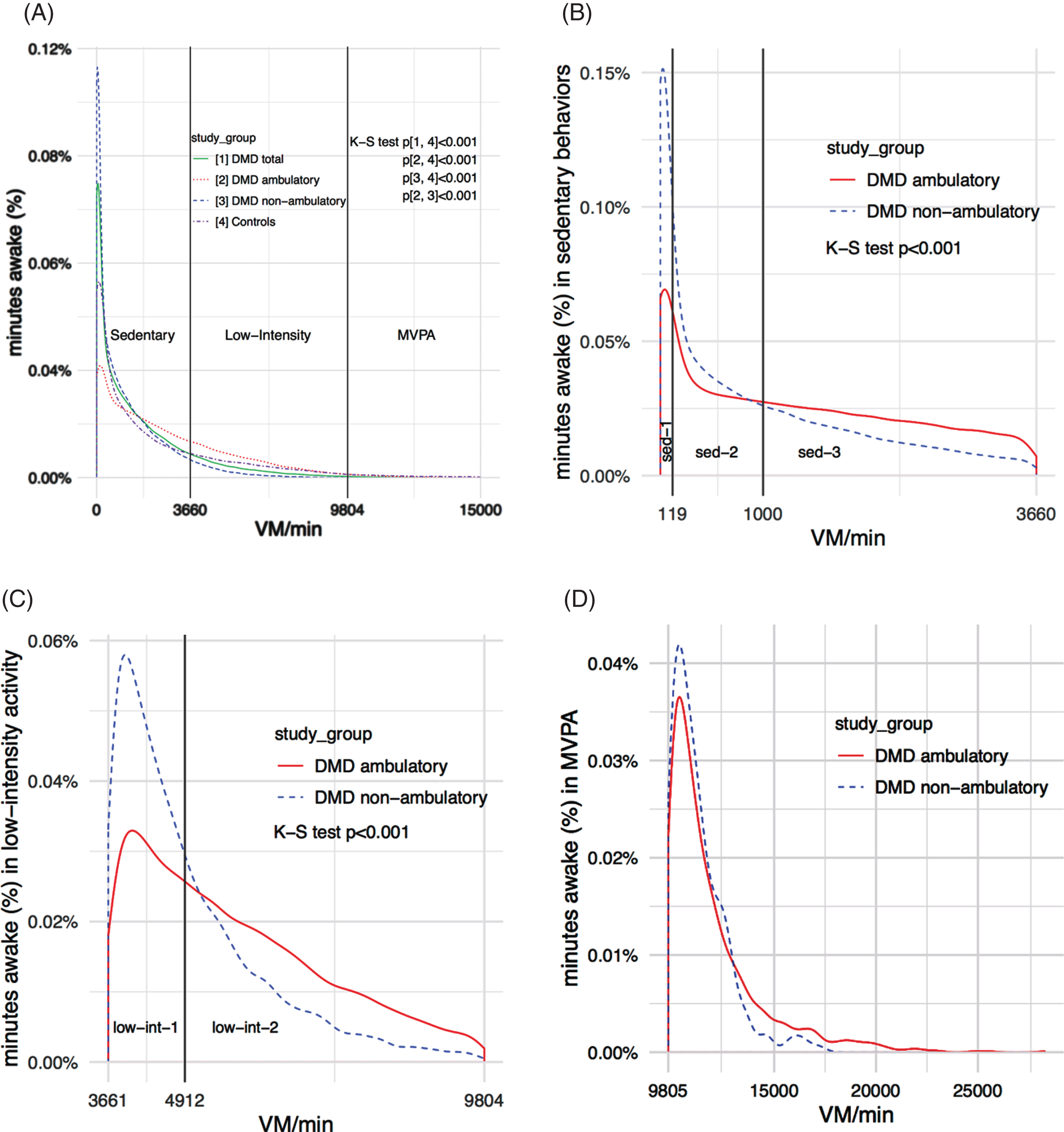
After identifying the intensity subcategories’ cut-points, wrist accelerometer recordings were integrated into 1-minute epochs and used to determine the percent of minutes awake that all participants spent in each intensity category. In patients with DMD, we also calculated the percent of minutes awake in sedentary behaviors and low-intensity activity spent in respective subcategories.
Statistical analysis
To compare anthropometric measures between groups, first, a Shapiro-Wilk test was used to determine the normality of sample data. If samples’ distribution was normal, a two-sample independent t-test was used for comparison. If samples’ distribution was not normal, a Mann-Whitney U test was used. Results are presented as means, standard deviations (SD), and ranges. This methodology was also used to compare adherence and physical activity measures from wrist-worn accelerometers between study groups, as well as physical activity measures from ankle-worn accelerometers and wrist-to-ankle ratios of physical activity measures between ambulation DMD subgroups.
To compare the distribution of physical activity across all intensity categories (i.e. the distribution of VM’s generated in each 1-minute epoch while awake) between study groups, a non-parametric Kolmogorov-Smirnov (K-S) test was used. To compare the percent of time awake spent in each intensity category between the study groups, two-sample independent t-tests with Bonferroni correction were used. The same methodology was used to compare the distribution of physical activity within sedentary and low-intensity activity, as well as the percent of minutes awake in sedentary behaviors and low-intensity activity spent in respective subcategories between ambulation DMD subgroups.
The effect of ambulation status, age, and corticosteroid use on the amount of physical activity (wrist VM/min wear) in patients with DMD was assessed using multiple regression. The first regression model assessed the effect of variable interactions on physical activity. A second regression model assessed the independent effect of variables on physical activity. Results are presented as an estimated coefficient value, 95% confidence interval, and p-value for each variable and variable interaction as well as an R2, adjusted R2, and p-value for each regression model.
For statistical tests, a p-value <0.05 was considered significant. In cases where Bonferroni correction was applied, the adjusted threshold for significance is reported. Analyses were performed using the programming language R version 3.6.1.
RESULTS
Participants’ characteristics
All study participants were male. Among 49 patients with DMD, 44 and 33 had valid recordings from the wrist and ankle accelerometers, respectively, and 11 of 15 healthy controls had valid recordings from the wrist accelerometer and were used in analyses. Of 49 patients with DMD, 16 were ambulatory while 33 were non-ambulatory.
Patients with DMD were monitored during all months of the year and healthy controls from November through February.
Of the five patients with DMD with invalid wrist accelerometer recordings, three did not meet the weekend wear time criterion, and two did not meet the number of hours per day criterion. Among the 16 patients with DMD with invalid ankle accelerometer recordings, 10 did not meet the hours per day criterion, four did not meet the weekend wear time criterion, and two did not wear an ankle accelerometer at all. The 4 healthy controls with invalid wrist accelerometer recordings did not meet the hours per day criterion.
Compared to the control group, the DMD group was similar in age and weight but was significantly shorter and had a higher BMI (Table 2). The DMD group had a similar weight-for-age Z-score but significantly lower height-for-age and higher BMI-for-age Z-scores compared to the control group. Approximately three-quarters of participants in both the DMD and control groups were non-Hispanic white.
Table 2
Anthropometric measures and demographic information
Participants (n) | DMD total | Healthy controls | DMD ambulatory | DMD non-ambulatory | ||
44 | 11 | 13 | 31 | |||
Anthropometric measurements | mean±SD [range] | p-value | mean±SD [range] | p-value | ||
Z-score*±SD | Z-score*±SD | |||||
Age (years) | 13.6±4.0 | 14.0±2.3 | 0.264 | 10.9±1.9 | 14.7±4.1 | <0.001 |
[8.4, 24.3] | [11.5, 18.4] | [8.4, 14.5] | [8.5, 24.3] | |||
Height (cm) | 145.8±16.5 | 165±11.5 | <0.001 | 134±11.0 | 151±15.7 | <0.001 |
[117, 180] | [151, 183] | [117, 150] | [122, 180] | |||
– 1.20±1.60 | 0.38±0.95 | <0.001 | – 1.40±1.70 | – 1.10±1.56 | 0.599 | |
Weight (kg) | 50.9±19.1 | 53.0±14.3 | 0.711 | 39.0±12.9 | 56.1±19.3 | 0.002 |
[18.6, 103] | [36.8, 79.0] | [22, 68.2] | [18.6, 103] | |||
0.15±2.03 | 0.03±1.00 | 0.607 | 0.09±1.41 | 0.18±2.30 | 0.549 | |
BMI (kg/m2) | 23.7±7.8 | 19.2±2.9 | 0.027 | 21.4±4.5 | 24.7±8.8 | 0.176 |
[11.3, 51.1] | [15.6, 25.0] | [15.5, 32.4] | [11.3, 51.1] | |||
0.76±2.23 | – 0.21±0.98 | 0.002 | 1.10±0.82 | 0.59±2.65 | 0.6898 | |
Race/Ethnicity | n (% ) | |||||
Non-Hispanic White | 32 (73) | 8 (73) | 11 (85) | 21 (68) | ||
Non-Hispanic Black | 3 (7) | 2 (18) | 2 (15) | 1 (3) | ||
Hispanic | 7 (16) | 0 | 0 (0) | 7 (23) | ||
Other | 2 (5) | 0 | 0 (0) | 2 (6) | ||
Corticosteroids | n (% ) | |||||
Currently taking | 33 (75) | 0 (0) | 13 (100) | 20 (65) | ||
Currently not-taking | 11 (25) | 11 (100) | 0 (0) | 11 (35) |
*For each study group, the average Z-score for height, weight, and BMI based on age and sex was calculated using CDC growth charts of males between 2 and 20 years of age [16].
Compared to ambulatory, non-ambulatory patients with DMD were significantly older, taller, and heavier but had similar BMI. Non-ambulatory patients had similar height-for-age, weight-for-age, and BMI-for-age Z-scores compared to ambulatory patients. All ambulatory and 65% of non-ambulatory patients were taking corticosteroids at the time of monitoring.
Physical activity measures using wrist-worn accelerometers
Participants in all study groups adhered to the protocol and wore accelerometers for similar amounts of time (Table 3).
Table 3
Adherence measures and physical activity measures using wrist-worn accelerometers
Participants (n) | DMD total [1] | DMD ambulatory [2] | DMD non-ambulatory [3] | Healthy controls [4] | |
44 | 13 | 31 | 11 | ||
Adherence measures | mean±SD [range] | p-value | |||
Wrist min/day wear | 1174±86 | 1180±50 | 1171±98 | 1184±60 | p = 0.942 p[2, 4] = 0.886 p[3, 4] = 0.978 p[2, 3] = 0.960 |
[880, 1339] | [1102, 1260] | [880, 1339] | [1071, 1279] | ||
Wrist min/day awake | 833±49 | 840±38 | 831±53 | 847±39 | p[1, 4] = 0.199 p[2, 4] = 0.361 p[3, 4] = 0.201 p[2, 3] = 0.939 |
[689, 896] | [758, 895] | [689, 896] | [765, 883] | ||
Physical activity measures | |||||
Wrist VM/min wear | 1281±751 | 2104±451 | 936±557 | 2044±668 | p[1, 4] = 0.004 p[2, 4] = 0.805 p[3, 4] < 0.001 p[2, 3] < 0.001 |
[74.7, 2730] | [1250, 2730] | [74.7, 2058] | [832, 2855] | ||
Wrist VM/min awake | 1584±974 | 2696±618 | 1118±669 | 2490±904 | p[1, 4] = 0.010 p[2, 4] = 0.531 p[3, 4] < 0.001 p[2, 3] < 0.001 |
[72.4, 3808] | [1536, 3808] | [72.4, 2288] | [974, 3750] |
Physical activity measures significantly differed between patients with DMD and healthy controls, ambulatory and non-ambulatory patients with DMD, and non-ambulatory patients with DMD and healthy controls. Compared to the healthy controls, patients with DMD had a lower wrist VM/min wear and VM/min awake (Table 3). Compared to ambulatory patients and healthy controls, non-ambulatory patients with DMD had a lower wrist VM/min wear and VM/min awake.
The wrist VM/min wear and VM/min awake were similar between ambulatory patients with DMD and healthy controls (Table 3).
Physical activity measures using ankle-worn accelerometers and wrist-to-ankle ratios
Physical activity measures significantly differed between the ambulation DMD subgroups (Table 4). Compared to ambulatory, non-ambulatory patients with DMD had lower ankle VM/min wear and VM/min awake, but greater wrist-to-ankle ratios of VM total, VM/min wear, and VM/min awake.
Table 4
Physical activity measures using ankle-worn accelerometers and wrist-to-ankle ratios of physical activity measures\\ in patients with DMD
DMD total [1] | DMD ambulatory [2] | DMD non-ambulatory [3] | ||
Participants (n) | 33 | 13 | 20 | |
Physical activity measures | mean±SD [range] | p-value | ||
Ankle VM/min wear | 380±346 | 741±250 | 145±120 | p < 0.001 |
[37.2, 1224] | [275, 1224] | [37.2, 481] | ||
Ankle VM/min awake | 448±427 | 902±301 | 152±128 | p[2, 3] < 0.001 |
[42.5, 1349] | [313, 1349] | [42.5, 494] | ||
Wrist-to-ankle ratios | ||||
VM total | 10.6±10.1 | 3.1±0.8 | 15.5±10.4 | p[2, 3] < 0.001 |
[1.8, 40.1] | [1.8, 4.7] | [4.4, 40.1] | ||
VM/min wear | 8.1±6.9 | 3.0±0.7 | 11.4±7.1 | p[2, 3] < 0.001 |
[1.8, 26.9] | [1.8, 4.5] | [4.3, 26.9] | ||
VM/min awake | 9.3±8.4 | 3.2±0.7 | 13.3±8.7 | p[2, 3] < 0.001 |
[1.9, 30.1] | [1.9, 4.9] | [4.6, 30.1] |
Distribution of physical activity across and within intensity categories
The distribution of physical activity across all intensity categories significantly differed between the DMD and control groups, ambulation DMD subgroups, and the control group and both ambulation DMD subgroups (Fig. 1A).
Compared to the control group, the DMD group spent significantly less time awake in MVPA (Table 5). Compared to ambulatory patients and healthy controls, non-ambulatory patients with DMD spent significantly more time awake in sedentary behaviors and less time in low-intensity activity and MVPA. Time awake in each intensity category did not significantly differ between the ambulatory patients with DMD and healthy controls.
Table 5
Distribution of physical activity across intensity categories using wrist-worn accelerometers
Participants (n) | DMD total [1] | DMD ambulatory [2] | DMD non-ambulatory [3] | Healthy controls [4] | |
44 | 31 | 13 | 11 | ||
Minutes awake (%) | mean±SD | p-value* | |||
Sedentary | 85.0±12.3 | 70.7±8.8 | 91.0±7.7 | 75.8±8.3 | p = 0.007 p[2, 4] = 0.162 p[3, 4] < 0.001 p[2, 3] < 0.001 |
Low-intensity | 13.8±10.9 | 26.0±8.0 | 8.7±7.3 | 19.2±5.8 | p[1, 4] = 0.030 p[2, 4] = 0.026 p[3, 4] < 0.001 p[2, 3] < 0.001 |
Moderate-to-vigorous | 1.2±1.6 | 3.3±1.4 | 0.3±0.6 | 5.0±2.9 | p[1, 4] = 0.001 p[2, 4] = 0.098 p[3, 4] < 0.001 p[2, 3] < 0.001 |
*p-value < 0.0042 considered significant after Bonferroni correction.
Patients with DMD spent 85% of their time awake in sedentary behaviors and 13.8% in low-intensity activity (Table 5). The distribution of physical activity within sedentary behaviors and low-intensity activity significantly differed between ambulation DMD subgroups (Fig. 1B, 1C).
Compared to ambulatory, non-ambulatory patients spent significantly more time of sedentary behaviors in the sedentary-1 subcategory and less time in sedentary-3 (Table 6). Compared to ambulatory, non-ambulatory patients spent significantly more time of low-intensity activity in the low-intensity-1 subcategory and less time in low-intensity-2.
Table 6
Distribution of physical activity in sedentary and low-intensity subcategories using wrist-worn accelerometers\\ in patients with DMD
DMD ambulatory | DMD non-ambulatory | ||
Participants (n) | 31 | 13 | |
Minutes awake in sedentary behaviors (% ) | mean±SD | p-value† | |
Sedentary-1 | 31.5±8.1 | 47.0±18.2 | <0.001 |
Sedentary-2 | 25.5±4.2 | 25.2±6.7 | 0.865 |
Sedentary-3 | 43.0±9.1 | 27.9±14.4 | <0.001 |
Minutes awake in low-intensity activity (%) | p-value‡ | ||
Low-intensity-1 | 36.0±5.2 | 61.4±15.6 | <0.001 |
Low-intensity-2 | 64.0±5.2 | 38.6±15.6 | <0.001 |
†p-value < 0.017 considered significant after Bonferroni correction; ‡p-value < 0.025 considered significant after Bonferroni correction.
Since patients with DMD spent, on average, only 1.2% of their time awake in MVPA, the distribution of MVPA between ambulation DMD subgroups was not compared but is shown graphically (Fig. 1D).
Effect of ambulation status, age, and corticosteroid use on physical activity measures in patients with DMD
The interactions between ambulation status and age and between corticosteroid use and age did not have a significant effect on wrist VM/min wear in patients with DMD (Table 7a). The interaction between ambulation status and corticosteroid use could not be assessed since all ambulatory patients with DMD were taking corticosteroids during the study.
Table 7a
Regression model to assess effect of variable interactions on wrist VM/min wear in patients with DMD
Variable or variable interaction | Coefficient value | 95% confidence interval | p-value |
Ambulation status | –332 | –1736, 1071 | 0.635 |
Age | –106 | –166, –46 | <0.001 |
Steroid status | 111 | –1078, 1300 | 0.851 |
Ambulation status &age interaction | 96.7 | –25, 218 | 0.116 |
Corticosteroid use &age interaction | 2.0 | –72, 77 | 0.956 |
Regression R2 = 0.7758, adjusted R2 = 0.7463, p-value <0.001; Regression equation: y = ao + a1x1 + a2x2 + a3x3 + a4x1x2 + a5x3x2; Variables definitions (units): y = wrist VM/min wear (VM/min), x1 = ambulation status (0 = non-ambulatory, 1 = ambulatory), x2 = age (years), x3 = corticosteroid use (0 = not-taking, 1 = taking).
Ambulation status and age had significant independent effects on the amount of physical activity in patients with DMD (Table 7b). Adjusting for age and corticosteroid use, being ambulatory vs non-ambulatory predicted an approximately 60% increase in wrist VM/min wear (744 VM/min) of the DMD group’s average. Adjusting for ambulation status and corticosteroid use, a one-year increase in age has predicted an approximately 7% decrease in wrist VM/min wear (96.3 VM/min) of the DMD group’s average. Corticosteroid use did not have a significant effect on wrist VM/min wear.
Table 7b
Regression model to assess effect of independent variables on wrist VM/min wear in patients with DMD
Variable | Coefficient value | 95% confidence interval | p-value |
Ambulation status | 744 | 452, 1036 | <0.001 |
Age | –96.3 | –130, –62 | <0.001 |
Corticosteroid use | 165 | –138, 467 | 0.277 |
Regression R2 = 0.7595, adjusted R2 = 0.742, p-value <0.001; Regression equation: y = bo + b1x1 + b2x2 + b3x3; Variables definitions (units): y = wrist VM/min wear (VM/min), x1 = ambulation status (0 = non-ambulatory, 1 = ambulatory), x2 = age (years), x3 = corticosteroid use (0 = not-taking, 1 = taking).
Both regression models explained about three-quarters of the variance in wrist VM/min wear of the DMD group.
DISCUSSION
A major finding of this study was the significant difference in the distribution of physical activity across intensity categories between patients with DMD and healthy controls and between ambulatory and non-ambulatory patients with DMD. Compared to healthy controls, patients with DMD spent less time awake in MVPA. Patients with DMD spent most of their time awake (∼99%) in sedentary behaviors and low-intensity activity. Dividing sedentary behaviors into three and low-intensity activity into two subcategories revealed unique patterns of physical activity in patients with DMD for the first time. Within these intensity categories, the distribution of physical activity between ambulation DMD subgroups significantly differed. The ambulatory patients spent more time at the categories’ higher thresholds (sedentary-3 and low-intensity-2), and non-ambulatory patients spent more time at the lower thresholds (sedentary-1 and low-intensity-1). These findings affirmed that the characterization of physical activity patterns in patients with DMD could be improved by dividing sedentary and low-intensity activity into three and two subcategories, respectively. Of note, our novel approach to calculating subcategory cut-points ensured that each patient had an equal contribution to the final thresholds.
The study also found a significant difference in the total amount of physical activity between ambulatory and non-ambulatory patients with DMD. Wrist VM/min wear and VM/min awake were lower in non-ambulatory than ambulatory patients. It is difficult to compare our results to those from prior studies since our methodology differs from existing reports. In one study, Kimura et al. (2014) similarly to this study found that the frequency and quantity of physical activity monitored by wrist accelerometers were significantly lower in non-ambulatory than ambulatory patients with DMD [14]. In this study, ankle and wrist-to-ankle ratios of physical activity measures significantly differed between the ambulation DMD subgroups as well. Since wrist, ankle, and wrist-to-ankle accelerometry data yielded similar results in both subgroups; we recommend that researchers and clinicians consider using a wrist accelerometer to monitor physical activity in both ambulatory and non-ambulatory patients with DMD, rather than two or more accelerometers worn at different locations. This recommendation is supported by more valid recordings obtained from wrist-worn (90%) than ankle-worn (67%) accelerometers in participants with DMD. In a recent study, Geest et al. (2019) found a strong correlation between movement data from accelerometers worn on the upper and lower arm of patients with DMD. They also suggest the use of a single wrist accelerometer to monitor physical activity in patients with DMD rather than two accelerometers [13].
As expected, wrist accelerometry detected a significant difference in the total amount of physical activity between patients with DMD and age- and sex-matched healthy controls. Wrist VM/min wear and VM/min awake were lower in the DMD group than the control group. Our results are in agreement with those reported by Heutinck et al. (2017), who found that ambulatory and non-ambulatory patients with DMD self-reported less and lower-demanding physical activity in the home environment compared to healthy controls [21]. Subsequent DMD ambulation subgroup analysis showed that wrist VM/min wear and VM/min awake were significantly lower in non-ambulatory patients than healthy controls but similar between ambulatory patients and controls. The latter finding was somewhat unexpected, but it emphasizes the variability in the amount and patterns of physical activity among patients with DMD. One feasible explanation for this finding is the natural progression of DMD, whereby lower extremity weakness occurs before upper extremity weakness [1]. Another possible explanation is that the healthy controls were not participating in active extracurricular sports activities and were asked to carry out their normal activities of daily living while wearing wrist accelerometers. Also, healthy controls were monitored during colder months of the year (November-February), while the patients with DMD were monitored across all months of the year. It is also possible that wearing two monitors mobilized patients with DMD to be more active than usual. In a study by Davidson et al. (2015), ankle-worn accelerometers detected significantly fewer steps per day in ambulatory patients with DMD than healthy controls [12]. It is difficult to compare our results to prior studies because of differing methodologies. Of note, while the amount of physical activity did not differ between ambulatory patients with DMD and healthy controls, there was a significant difference in the distribution of physical activity across all intensity categories between the two groups. On average, patients with DMD spent more percent of time awake in low-intensity activity and less percent of time in sedentary behaviors and MVPA compared to healthy controls. However, the percent of time awake in each intensity category did not significantly differ between the two groups.
Regarding the study’s exploratory goal, multiple regression showed that ambulation status and age had significant independent effects on wrist VM/min wear of the DMD group. Our results regarding the effect of age are comparable to those reported by Kimura et al (2014). In their study, wrist accelerometry detected a drop in the quantity of physical activity between a baseline and ensuing one-year visit in 13 out of 14 patients 7-years-old or older with DMD and different ambulation status [14]. In this study, corticosteroid use did not have a significant independent effect on wrist VM/min wear of the DMD group. Jeannet et al. (2011) reported different results; wrist accelerometry detected activity increases in a group of ambulatory patients with DMD after corticosteroid administration [10]. It is possible that corticosteroid use increases physical activity measures in ambulatory patients with DMD, but that the medication’s effect is less notable in ambulatory and non-ambulatory patients. We were unable to assess if corticosteroid use affected physical activity through its interaction with ambulation status since all ambulatory patients with DMD were taking corticosteroids during the study. Similar to our study, prior studies found that patients with DMD were shorter and had a greater BMI than healthy controls, likely due to long-term corticosteroid use [14, 21, 22].
The study’s strengths were objective assessment of physical activity in the natural home environment using triaxial accelerometers and using a novel method to assess physical activity patterns. In the absence of a practical standard method to assess physical activity patterns in the daily life of patients with DMD, we consider dividing sedentary and low-intensity activity into subcategories as a potentially clinically useful approach. Proposed subcategories could ameliorate potential floor or ceiling effects when using intensity categories as an outcome measure since intensity subcategories are more sensitive than intensity categories to detect small changes in physical activity. Dividing intensity categories into subcategories is analogous to the CHOP INTEND motor scale used for spinal muscular atrophy clinical trials, where there is a need for an outcome measure that is sensitive enough to detect small treatment effects in infants who are weak at baseline [23]. Future studies should test the proposed subcategory approach to assess physical activity patterns from accelerometers recordings in other populations of patients with DMD and in different neuromuscular diseases.
The study has some limitations. First, the sample size was relatively small and unequally distributed among ambulatory and non-ambulatory patients with DMD and healthy controls. Second, the study was conducted at one center, which makes results less generalizable, though Vanderbilt’s Neuromuscular Cardiology and Muscular Dystrophy Clinics draw patients from a large catchment area including Middle Tennessee, Northern Alabama, and Southern Kentucky. Third, the decision to consider participants as awake between 6 : 00 am and 9 : 00 pm might have misclassified participants as asleep vs awake during early mornings and late evenings. Fourth, we did not investigate other variables that might affect the amount of physical activity in patients with DMD including participant’s mental health, weight, BMI, or the season during which monitoring took place, due to concerns of over-fitting the regression model in the setting of the study’s small sample size. Fifth, the study used a binary classification of ambulation status (ambulatory vs non-ambulatory) commonly used in clinical practice. Given the progressive deterioration of lower limb strength in patients with DMD, ambulation status could be considered a continuous rather than binary variable. For example, magneto-inertial sensors can provide more sophisticated measures of physical activity than accelerometry in patients with DMD such as angular velocity, elevation rate, and Stride Velocity 95th Centile (SV95C), which the European Medicines Agency recently qualified as a valid outcome in patients with DMD [24–26]. However, using vector magnitude (VM) as a measure of the amount of physical activity is at this point relatively accessible to clinicians and researchers using Actigraph or similar accelerometers. Sixth, the study used intensity category cut-points validated in healthy children to assess physical activity patterns. However, estimates of time and amount of physical activity in intensity categories are affected by values of cut-points and epoch length, so the results might not be comparable with other studies using differing values [27]. Seventh, the study did not investigate why some participants with DMD did not meet the weekend wear time criterion or number of hours per day criterion for wrist- and ankle-worn accelerometers. Optimizing adherence to accelerometer wearing protocol in patients with DMD is important for effective data collection in future studies. Eighth, the study did not investigate the reliability and variability of accelerometry as an outcome measure. However, we previously reported that accelerometry recordings were strongly correlated with QMT-measured muscle strength in patients with DMD [15].
In conclusion, patients with DMD spent most of their awake time in sedentary behaviors and low-intensity physical activity. Dividing these intensities into three and two subcategories, respectively, allows better characterization of physical activity patterns in patients with DMD. Physical activity was lower in patients with DMD than healthy controls and non-ambulatory than ambulatory patients with DMD. Ambulation status and age but not corticosteroid use affected physical activity measures in patients with DMD.
CONFLICT OF INTEREST STATEMENT
The authors have no conflict of interest to report.
ACKNOWLEDGMENTS
We express thanks to the patients and their families for their participation in this study. We also thank Ms. Tao Liang for advice on statistical analyses.
This study was funded by the National Heart, Lung, and Blood Institute (grant # K23HL123938 and # R56HL141248) and the Office of Medical Student Research at Vanderbilt University. Additional funds were provided by grants (DK058404, DK20593) from the National Institute of Diabetes and Digestive and Kidney Diseases and by the National Center for Advancing Translational Sciences (CTSA) award (UL1-TR002243).
SUPPLEMENTARY MATERIAL
[1] The supplementary material is available in the electronic version of this article: https://dx.doi.org/10.3233/JND-200478.
REFERENCES
[1] | Ryder S , Leadley RM , Armstrong N , Westwood M , de Kock S , Butt T , et al. The burden, epidemiology, costs and treatment for Duchenne muscular dystrophy: An evidence review. Orphanet Journal of Rare Diseases. (2017) ;12: (1):79. 10.1186/s13023-017-0631-3. |
[2] | Scott E , Mawson SJ . Measurement in Duchenne muscular dystrophy: Considerations in the development of a neuromuscular assessment tool. Developmental Medicine and Child Neurology. (2006) ;48: (6):540–4. doi: 10.1017/S0012162206001137. |
[3] | Bushby K , Connor E . Clinical outcome measures for trials in Duchenne muscular dystrophy: Report from International Working Group meetings. Clin Investig (Lond). (2011) ;1: (9):1217–35. doi: 10.4155/cli.11.113. |
[4] | Mazzone E , Martinelli D , Berardinelli A , Messina S , D’Amico A , Vasco G , et al. North Star Ambulatory Assessment, 6-minute walk test and timed items in ambulant boys with Duchenne muscular dystrophy. Neuromuscul Disord. (2010) ;20: (11):712–6. doi: 10.1016/j.nmd.2010.06.014. |
[5] | Pane M , Mazzone ES , Sivo S , Sormani MP , Messina S , D’Amico A , et al. Long Term Natural History Data in Ambulant Boys with Duchenne Muscular Dystrophy: 36-Month Changes. PLOS ONE. (2014) ;9: (10):e108205. doi: 10.1371/journal.pone.0108205. |
[6] | Mendell JR , Goemans N , Lowes LP , Alfano LN , Berry K , Shao J , et al. Longitudinal effect of eteplirsen versus historical control on ambulation in Duchenne muscular dystrophy. Ann Neurol. (2016) ;79: (2):257–71. doi: 10.1002/ana.24555. |
[7] | Herrington WG , Goldsack JC , Landray MJ . Increasing the use of mobile technology–derived endpoints in clinical trials. Clin Trials. (2018) ;15: (3):313–5. doi: 10.1177/1740774518755393. |
[8] | Hendriksen RGF , Vles JSH , Aalbers MW , Chin RFM , Hendriksen JGM . Brain-related comorbidities in boys and men with Duchenne Muscular Dystrophy: A descriptive study. Eur J Paediatr Neurol. (2018) ;22: (3):488–97. doi: 10.1016/j.ejpn.2017.12.004. |
[9] | Fujii T , Takeshita E , Iwata Y , Yajima H , Nozaki F , Mori M , et al. Cumulative jerk as an outcome measure in nonambulatory Duchenne muscular dystrophy. Brain and Development. (2019) ;41: (9):796–802. doi: 10.1016/j.braindev.2019.06.002. |
[10] | Jeannet P-Y , Aminian K , Bloetzer C , Najafi B , Paraschiv-Ionescu A . Continuous monitoring and quantification of multiple parameters of daily physical activity in ambulatory Duchenne muscular dystrophy patients. Eur J Paediatr Neurol. (2011) ;15: (1):40–7. doi: 10.1016/j.ejpn.2010.07.002. |
[11] | Fowler EG , Staudt LA , Heberer KR , Sienko SE , Buckon CE , Bagley AM , et al. Longitudinal community walking activity in Duchenne muscular dystrophy. Muscle Nerve. (2018) ;57: (3):401–6. doi: 10.1002/mus.25743. |
[12] | Davidson ZE , Ryan MM , Kornberg AJ , Walker KZ , Truby H . Strong correlation between the 6-minute walk test and accelerometry functional outcomes in boys with Duchenne muscular dystrophy. J Child Neurol. (2015) ;30: (3):357–63. doi: 10.1177/0883073814530502. |
[13] | van der Geest A , Essers JMN , Bergsma A , Jansen M , de Groot IJM . Monitoring daily physical activity of upper extremity in young and adolescent boys with Duchenne muscular dystrophy: A pilot study. Muscle Nerve. (2019) . doi: 10.1002/mus.26763. |
[14] | Kimura S , Ozasa S , Nomura K , Yoshioka K , Endo F . Estimation of muscle strength from actigraph data in Duchenne muscular dystrophy. Pediatr Int. (2014) ;56: (5):748–52. doi: 10.1111/ped.12348. |
[15] | Killian M , Buchowski MS , Donnelly T , Burnette WB , Markham LW , Slaughter JC , et al. Beyond Ambulation: Measuring Physical Activity in Youth with Duchenne Muscular Dystrophy. Neuromuscular Disorders [Internet]. (2020) . Available from: https://www.sciencedirect.com/science/article/pii/S096089662030033X. |
[16] | Growth Charts - Percentile Data Files with LMS Values [Internet]. (2019) . Available from: https://www.cdc.gov/growthcharts/percentile_data_files.htm. |
[17] | Choi L , Liu Z , Matthews CE , Buchowski MS . Validation of accelerometer wear and nonwear time classification algorithm. Med Sci Sports Exerc. (2011) ;43: (2):357–64. doi: 10.1249/MSS.0b013e3181ed61a3. |
[18] | Chandler JL , Brazendale K , Beets MW , Mealing BA . Classification of physical activity intensities using a wrist-worn accelerometer in 8–12-year-old children. Pediatric Obesity. (2016) ;11: (2):120–7. doi: 10.1111/ijpo.12033. |
[19] | van Loo CMT , Okely AD , Batterham MJ , Hinkley T , Ekelund U , Brage S , et al. Wrist Accelerometer Cut-points for Classifying Sedentary Behavior in Children. Med Sci Sports Exerc. (2017) ;49: (4):813–22. doi: 10.1249/MSS.0000000000001158. |
[20] | Butte NF , Watson KB , Ridley K , Zakeri IF , McMurray RG , Pfeiffer KA , et al. A Youth Compendium of Physical Activities: Activity Codes and Metabolic Intensities. Med Sci Sports Exerc. (2018) ;50: (2):246–56. doi: 10.1249/MSS.0000000000001430. |
[21] | Heutinck L , Kampen N van , Jansen M , Groot I de . Physical activity in boys with DMD is lower and less demanding compared to healthy boys. Neuromuscular Disorders. (2015) ;25: :S303–4. doi: 10.1016/j.nmd.2015.06.418. |
[22] | Werneck LC , Lorenzoni PJ , Ducci RD-P , Fustes OH , Kay CSK , Scola RH . Duchenne muscular dystrophy: An historical treatment review. Arq Neuropsiquiatr. (2019) ;77: (8):579–89. doi: 10.1590/0004-282X20190088. |
[23] | Glanzman AM , Mazzone E , Main M , Pelliccioni M , Wood J , Swoboda KJ , et al. The Children’s Hospital of Philadelphia Infant Test of Neuromuscular Disorders (CHOP INTEND): Test development and reliability. Neuromuscul Disord. (2010) ;20: (3):155–61. doi: 10.1016/j.nmd.2009.11.014. |
[24] | Le Moing A-G , Seferian AM , Moraux A , Annoussamy M , Dorveaux E , Gasnier E , et al. A Movement Monitor Based on Magneto-Inertial Sensors for Non-Ambulant Patients with Duchenne Muscular Dystrophy: A Pilot Study in Controlled Environment. PLoS ONE. (2016) ;11: (6):e0156696. doi: 10.1371/journal.pone.0156696. |
[25] | Lilien C , Grelet M , Gasnier E , Gidaro T , Seferian A , Rigaud A , et al. DUCHENNE MUSCULAR DYSTROPHY – PHYSIOTHERAPY: P.312Factors influencing spontaneous maximal stride speed in individual Duchenne muscular dystrophy boys. Neuromuscular Disorders. (2018) ;28: :S125. doi: 10.1016/j.nmd.2018.06.360. |
[26] | Haberkamp M , Moseley J , Athanasiou D , de Andres-Trelles F , Elferink A , Rosa MM , et al. European regulators’ views on a wearable-derived performance measurement of ambulation for Duchenne muscular dystrophy regulatory trials. Neuromuscular Disorders. (2019) ;29: (7):514–6. doi: 10.1016/j.nmd.2019.06.003. |
[27] | Migueles JH , Cadenas-Sanchez C , Ekelund U , Delisle Nystroöm C , Mora-Gonzalez J , Loöf M , et al. Accelerometer Data Collection and Processing Criteria to Assess Physical Activity and Other Outcomes: A Systematic Review and Practical Considerations. Sports Med. (2017) ;47: (9):1821–45. doi: 10.1007/s40279-017-0716-0. |