Measuring Habitual Physical Activity in Neuromuscular Disorders: A Systematic Review
Abstract
Background: Free-living or habitual physical activity (HPA) refers to someone’s performance in his or her free-living environment. Neuromuscular disorders (NMD) manifest through HPA, and the observation of HPA can be used to identify clinical risks and to quantify outcomes in research. This review summarizes and analyses previous studies reporting the assessment of HPA in NMD, and may serve as the basis for evidence-based decision-making when considering assessing HPA in this population.
Methods: A systematic review was performed to identify all studies related to HPA in NMD, followed by a critical appraisal of the assessment methodology and a final review of the identified HPA tools.
Results: A total of 22 studies were selected, reporting on eight different direct tools (or activity monitors) and ten structured patient-reported outcomes. Overall, HPA patterns in NMD differ from healthy control populations. There was a noticeable lack of validation studies for these tools and outcome measures in NMD. Very little information regarding feasibility and barriers for the application of these tools in this population have been published.
Conclusions: The variety and heterogeneity of tools and methods in the published literature makes the comparison across different studies difficult, and methodological guidelines are warranted. We propose a checklist of considerations for the assessment and reporting of HPA in NMD.
INTRODUCTION
New technology has been developed over the last decade that is able to monitor and measure daily life activity behaviour, generating a large amount of data for analysis and mass-market applications. Increasingly, this technology has been implemented into research and many of these tools have been correlated to long-term health outcomes and motor capacity in diseases with impaired mobility such as Parkinson’s disease [1], stroke [2] and cerebral palsy [3].
Patients with neuromuscular disorders (NMD) frequently experience limitations caused by muscle weakness, pain, fatigue, reduced mobility and overall functionality, all of which result in a limited participation in activities of daily living and a sedentary lifestyle with its associated risks [4–8]. Consequently, the need for further research has been emphasized with the goal of analysing and improving physical activity (PA) safely and developing evidence-based exercise recommendations [5, 9, 10]. However, the rareness and heterogeneity of NMDs [11], have resulted in relatively few clinical trials utilizing this technology and there is still a lack of awareness of the potential of this as an outcome measure and it is still unclear which tool may be most appropriate in which setting [12, 13].
Assessing free-living or habitual physical activity
Free-living or habitual physical activity (HPA) encompasses any activity that people do in their daily life and natural environment. HPA qualifies as an outcome of performance rather than capacity, as it refers to someone’s involvement and real participation in daily-life activities (DLA) rather than their ability to execute an action (usually tested in clinic, i.e. under request and ideal circumstances) [14, 15]. Important outcomes associated with HPA patterns are survival and disease progression; however, a more immediate benefit of a healthy HPA behaviour would be quality of life [16].
Assessing someone’s HPA is essential to: [1] determine whether altered activity behaviours are present; [2] establish an appropriate dose of PA to impact on specific health parameters; [3] set measurable goals for PA-related interventions; and [4] establish a surrogate outcome measure for clinical trials [17–22].
In research, HPA can be measured either directly or indirectly. Indirect tools refer to patient-reported outcomes (PRO) such as questionnaires and activity diaries, which are often simple to apply, cost-efficient and a good option for large cohort studies. However, PRO are susceptible to subjectivity and bias related to self-reporting and/or interpretation usually requiring larger sample sizes when assessing changes over time or differences between groups [23, 24]. Nonetheless, regulatory agencies have acknowledged the importance of PRO in drug development and marketing approval as long as these come with enough evidence to validate their reliability [25]. To validate an indirect tool objectively, it should be compared to a direct tool.
Direct methods, on the other hand, assess actual physiological changes; these can be: physiological markers (e.g. heart rates), motion sensors (e.g. pedometers or accelerometers) or calorimeters (e.g. doubly labelled water method) that correlate with physical activity. Direct tools are considered more objective and accurate [26, 27]; however, these also present limitations [28, 29]. They are typically more expensive, time-consuming and tend to place a higher burden on the researcher and the participant [17, 28, 30, 31]. Additionally, the most common outputs of these devices rely on the manufacturers’ algorithmic estimations and cannot always be generalizable to the target population and the raw data’s full interpretation still depends upon the researcher’s experience [32]. Doubly labelled water is the gold standard for the other tools to validate Energy Expenditure (EE) estimations, currently. Observation is the standard method to validate type-of-activity detection (i.e. steps) [27].
There is yet no gold standard tool for assessing HPA in NMD and the adaptation of any of these tools into clinical practice and research is not straightforward. Future application in clinical practice requires further research to confirm the validity and reliability of tools in the particular population being studied [33–38]. A good start for this is to learn from previous experiences [1–3, 33–35].
Review aims
The aim of this review was to identify and analyse the tools and methodology previously used to assess HPA in NMD. To accomplish this, studies in NMD that reported any type of HPA assessment were selected and reviewed. Any HPA tools and methodologies used were identified and critically analysed. Finally, we conclude with an evidence-based checklist of interest to researchers and clinicians measuring HPA in NMD.
This review’s protocol was initially registered with the PROSPERO (International prospective register of systematic reviews) database [39].
METHODS
Search methodology
A systematic literature search was carried out through the following databases: EMBASE; MEDLINE; and PsychINFO. The search strategy was to retrieve literature that investigated the use of free-living (habitual) activity assessment tools used in NMD. Hence the search used identified keywords from each database as appropriate. Terms included “free living activity”, “performance”, “physical activity”, and “daily life activity” in combination with “neuromuscular disease(s)”, “neuromuscular disorder(s)”, “muscle disease(s)” and/or “muscular dystrophy”.
Study selection
The search included publications from 1996 until the end date of search (March 2016).
Papers fulfilling the following criteria were included for analysis: [1] participants diagnosed with genetic neuromuscular disorders (NMD); [2] utilised measurement tools for habitual physical activity (HPA) assessing the subject’s performance for at least 24 successive hours; [3] patient-reported outcomes should include at least 60% items related to HPA. Publications were excluded when they: [1] were not published in English; [2] were single cases, on-going studies, non-research reports, conference abstracts or pre-clinical studies; [3] measured “capacity” or “life participation” instead of HPA “performance” or were of use only in controlled laboratory environments [14, 40].
The titles and abstracts of all retrieved references were screened by one reviewer (AJ) to exclude papers that did not instantly fulfil the inclusion criteria. Publications selected were properly revised by two independent reviewers (AJ, JN) to verify the selection criteria. Conflicting viewpoints and exclusion decisions were discussed with a third reviewer (SC) until a consensus was reached. Finally, the three main reviewers (AJ, JN, SC) proceeded with a deep analysing and summarising of the final selection of papers. After that, all HPA-tools identified were subsequently labelled as a patient’s (or next of kin) reported outcome (PRO) or as a direct tool (D).
1. Summary of direct HPA tools
The variables analysed are presented in Table 1. This review methodology follows criteria which adhere to the methods proposed by Matthews et al. of ‘Best Practices for Using Physical Activity Monitors in Population-Based Research’ [41]. When more than two variables were missing on the report, an attempt was made to contact the study authors by email.
2. Summary of indirect HPA tools
Patient-reported outcomes (e.g. questionnaires or PA diaries) were analysed by the identification of: [1] tool utilised; [2] data collection: respondent (i.e. patient, carer, etc.); location of completion (i.e. clinical settings, home, etc.); recollection method (i.e. face to face, phone, post, online, etc.); [3] data analysis criteria and methodology; [4] endpoints reported.
RESULTS
Study selection
The results of the literature and selection processes are displayed in Fig. 1. The initial search yielded 1,070 titles and abstracts from which 88 were selected using the predefined criteria, a good number of these refer to a physical activity or an exercise parameter different from HPA as defined previously. Of these, the second double-peered filter excluded 67 papers. Four conference abstracts and three on-going studies with published protocols were excluded at this stage [42–48]. One paper included only a minor number of patients with a muscle disease among a larger non-muscle disease population (2/75 muscular dystrophy) [49], this was excluded too. The remaining twenty-two papers were selected for analysis and are summarised in Table 2 [9, 36–38, 50–67].
Study sample characteristics
Study-sample numbers ranged from five [56] to 321 participants [65] and included both paediatric and adult populations. Twelve studies (54%) reported on a specific NMD entity: Duchenne muscular dystrophy (DMD) [37, 54–56, 66]; spinal and bulbar muscular atrophy (SBMA) [52]; myotonic dystrophy type 1 (DM1) [38, 53]; mitochondrial myopathies [58]; Charcot-Marie-Tooth [60]; Pompe disease [61]; McArdle’s disease [51]; and facioscapulohumeral muscular dystrophy (FSHD) [63]. The remaining publication-samples were grouped as NMD, including three studies reporting a mixed-diseased sample involving non-neuromuscular patients [49, 64, 67]. Nine studies (40%) involved a healthy control group for comparison, referred as “healthy controls” or “able-bodied” [9, 36–38, 51, 54, 58, 59, 62].
Study design and methodology
Four studies were randomized controlled trials (RCT) assessing an intervention [52, 53, 63, 67].
Only three studies investigated the validation of an HPA tool [37, 56, 64]. Two studies reported assessing the feasibility of these tools as outcome measures for the primary study aim [49, 54]. The remaining studies did not specifically test the HPA tool but utilised it for a secondary aim e.g. Wiles et al. [38]. The primary aim of this study was to quantify falls and stumbles in DM1, with a secondary aim of investigating the correlation between these falls and the patients’ step count [38].
Eleven studies reported the use of more than one HPA tool, five of these as a control method (e.g. PA diaries for PA monitors) or to supplement the endpoints’ report (e.g. Energy Expenditure calculations) [9, 36, 37, 59, 63].
Direct HPA assessment tools and methodology
Of the selected studies, sixteen reported the use of a direct HPA tool [9, 36–38, 50, 52, 54, 56–59, 61–64, 67]. Seven of these studies compared against healthy controls and showed differences between the groups [9, 36–38, 59, 64, 69]. Characteristics of their methodology are presented in Table 3 and the eight different tools identified as direct are presented in Fig. 2. Full data collection protocol (data criteria for analysis, definition of non-wear episode and the processing of missing data) was only identifiable in three of the papers [54, 56, 61]. Four papers reported the use of a patient-reported outcome (i.e. activity diary) as a quality control method [9, 36, 37, 64].
Patient reported HPA outcomes used in NMD
Fifteen papers reported the use a patient-reported HPA tool (PRO) (Table 4). The PRO most commonly used in this cohort was a type of written diary of the patient’s activities during the day. The questionnaires used to measure HPA were: Physical Activity Scale for Elderly (PASE) [67]; Bouchard Three-Day Physical Activity Record [51, 55]; International Physical Activity Questionnaire (IPAQ) [58]; EPIC-Norfolk Physical Activity Questionnaire-2 [59]; Physical Activity Disability Survey-Revised (PADS-R) [60]; Sickness Impact Profile (SIP) – mobility and ambulation subscales [62]; Checklist Individual Strength (CIS) – decreased Physical Activity subscale [63]; Godin Leisure Time Exercise Questionnaire (GLTEQ) [65]; and a modified version of the Baecke Physical Activity Questionnaire [66].
Two papers reported a third person as the respondent, either the patient’s parents or patient’s next of kin [53, 55], whereas two papers did not provide any details about their methodology [58, 66].
Evidence-based background of HPA assessment tools previously used in NMD
A selection of ten systematic reviews appropriate to this topic, and published between 2011 and 2016, were reviewed by the authors in search of additional evidence to back up each tool identified [13, 22, 28, 29, 34, 35, 69–72]. Validation studies were most reported in Cerebral Palsy (CP) for paediatrics and Multiple Sclerosis (MS) for adults and only McDonald et al.’s StepWatch validation study in DMD [37] was identified as an NMD-population paper; reported by Oftedal et al. [35].
From the seven identified direct HPA tools, six have been mentioned in previous systematic reviews: ActiGraph; StepWatch; Actical; and Yamax; Actical and SenseWear™ Pro3 [13, 22, 28, 29, 34, 35, 69–73]. The most reported endpoints have been: energy expenditure (EE); physical activity levels (PAL); and step count [29]. Table 5 condenses the validity evidence behind the direct tools identified in this review and ten different PROs have been described in Table 6. The reviewers identified at least one study comparing each PRO to an objective tool; three used double-labelled water (DLW) [74–76], twelve against an accelerometer [77–89] and one against a HR-monitor [83].
DISCUSSION
This systematic review examines previous studies measuring habitual physical activity (HPA) in people affected with neuromuscular disorders (NMD). This report highlights that HPA assessment in this population has been carried out in an increasing number of studies over recent years, but is still in its infancy as compared to populations affected by more common conditions such as diabetes, both in the number of studies and in the number of patients included.
The first point of note was the use of wide and overlapping terminology to describe activity. Differences between ‘physical activity’, ‘exercise’, ‘physical fitness’ [40], ‘performance’ and ‘capacity’ [14] were not always clear. This led to the exclusion of a large number of papers in the screening process. More accurate distinction of these terms would have allowed for a more precise identification of outcome measures and facilitated comparisons between publications. Caspersen et al. [40] explain the difference between physical activity and exercise, where exercise includes an element of structure and planning. Physical fitness refers to the attributes someone has to achieve for either one or both of them (i.e. strength, endurance and flexibility). The difference between capacity and performance has been introduced before and is based on the ICF terminology [14].
The rationale for measuring HPA (directly or indirectly) in the studies reviewed was rarely explained in detail. Some studies report assessing a behavioural pattern whilst others assessed a dose-response effect to an intervention. The materials and methodology used were also heterogeneous. This variability made comparisons between results and the drawing of specific conclusions difficult. Nonetheless, it is possible to conclude that PA levels and patterns of NMD subjects differ significantly from the matched healthy controls [9, 36–38, 51, 54, 58, 59, 64].
Despite reviewing a large number of publications only three papers allowed for comparisons between direct and indirect methods [58, 63, 69]. Kalkman et al. [69] reported a relationship between the Sickness Impact Profile (SIP) scores for mobility and ambulation and the actometer activity reports in a mixed NMD cohort. Both tools strongly correlated to the PA-dependent variables of fatigue and functional impairment. However when the Myotonic Dystrophy patients were analysed independently, the correlation with the fatigue score was lost. Apaphabi et al. [58] reported a moderate association between subjective PA reports obtained from the IPAQ with those obtained from the SenseWear Armband; however, the r-values provided are not strong (0.25 to 0.35). Finally, Voet et al. [63] provided a tangible example of a possible risk faced when only utilising one assessment method. In this study, the aerobic exercise group (AET) showed increased physical activity levels when measured directly (actometer), but no significant changes were reported on the PRO (CIS-reduced PA questionnaire), which, if used alone, would have missed these changes. On the other hand, the actometer, if considered in isolation, might have led to overestimated conclusions as the results might not reflect the patient’s perception of activity levels.
Of the 22 papers reviewed, five reported estimations of Energy Expenditure (EE) and Physical Activity Levels (PAL) following previously published methodologies [9, 36, 51, 55, 139]. However, the presumed validity and reliability of these methods do not always translate into a disease population in particular due to the physiological differences in the cardiovascular and respiratory systems. Only three studies presented clearer attempts to validate the device used in the particular population investigated beforehand or attached to their HPA investigation [37, 56, 64]. Three other studies combined tools to provide information from one tool that could support the other, like an activity diary combined with a heart rate monitor [9, 37, 59].
Only two studies commented on both the strengths and barriers of the tools presented, allowing the reader to get a better idea of what to expect from these and the possible limitations of the results [37, 56]. Tool limitations that can resume in a loss of data or a higher inter-rater variability should be considered in advance as these might impact on study logistics and sample estimations.
It is important to emphasize that the reported outcomes and devices presented and discussed in this paper represent only those identified through the literature search; however, this is by no means an exhaustive list and does not cover some of the other options currently available and that have been used in other diseases or epidemiology studies [13, 29, 69, 73]. Still, it certainly presents a wider variety that the one discussed in 2007 at the TREAT-NMD meeting on PA monitoring in NMD [12], which emphasizes the growing interest in experimenting with these types of tools. Currently, the authors are aware of ongoing clinical trials in NMDs using more modern tools such as: ActiGrapha and GeneActivb accelerometers in DM1; GeneActivc in mitochondrial diseases and in idiopathic inflammatory myopathies, the StepWatch Activity Monitord and ActiMyoe in DMD [140] and MoveMonitorf in myasthenia gravis. These well-controlled trials usually employ direct along with indirect measures and will contribute to a growing body of evidence for the use of these tools in clinical NMD research. Despite the use of HPA devices being at an early phase in NMDs, lessons learnt in larger clinical groups with movement impairments are likely to be transferrable to the measurement of HPA in NMDs [1].
IMPLICATIONS AND RECOMMENDATIONS
Assessing HPA effectively has many advantages and may allow the identification of subject changes and day-to-day limitations possibly missed with standard clinical measures [12]. This was reported for a study in Parkinson’s disease, where no significant changes in clinical measures of gait and disease burden over time were seen, but a meaningful reduction in daily ambulatory activity [141]. Identifying the appropriate tool and methodology appears equally important in NMD, not only to generate high-quality data for research and regulatory purposes, but also to avoid loss of time and resources through the use of tools or methods not suitable or informative for this population.
A few concepts should be considered when measuring HPA: reliability, validity and responsiveness of the tool to use; feasibility of the research site; and acceptability of the study participants [18, 142, 143]. Ideally, the selected tool should have as much evidence as possible supporting these for the targeted population or similar cohorts as several studies have encountered barriers when assessing participants with altered movement patterns or physiology. The following examples have relevant learning points:
1. McDonald et al.’s findings regarding heart rate (HR) adaptations to activity in DMD patients compared to controls demonstrate how established formulas to estimate EE or PAL based on HR cannot directly translate to a disease group [37].
2. Indirect tools tend to overestimate physical activity levels when the respondents are subjects with flawed physiological responses to activity [23, 144, 145]. Even the Physical Activity Scale for Individuals with Physical Disabilities (PASIPD) has shown overestimation when compared to activity monitors [146].
3. Altered mobility has a big impact on the reliability of motion sensors. Bachasson et al. [147] and Barak et al.[148] have described altered gait patterns commonly present in NMD patients, such as slower gait speed, lower stride frequency, shorter stride length, impaired balance and low walking endurance. All these variables will impact on the outputs obtained by activity monitors where validations were previously performed with subjects without gait abnormalities [29, 148].
4. Wheel-chair users should be considered independently as the circumstances of these subjects require specific estimations, both for direct [140, 149] or indirect tools [146]. Ideally, activity monitors will be located on either trunk or upper limb and PRO should include activities that can be performed in a wheelchair.
5. Finally, cognitive impairment should also be considered when assuming compliance rates and reliability for both direct and indirect tools. Certain characteristics, such as a shorter attention span, memory deficits, and errors in comprehension and reporting [24, 150] may interfere with reporting reliability and compliance rates [151].
The authors are not recommending a complete homogenization of tools or methods utilized for HPA assessment. This decision will always be subject to site experience and resources, and most importantly to the study aims. However, having a checklist of considerations prior to HPA assessment may facilitate the efficiency of the protocol design and the quality of the study report (Appendix A). We want to encourage publications to include enough information to allow for repeatability and a clear understanding of the methodology used.
CONCLUSION
This review captures the emerging evidence supporting the use of measures that assess habitual physical activity in NMD and some of the possible settings where this seems feasible.
Because of the wide variety of options and methodology, clinicians and researchers in the field need to become familiar with the concept and the different constructs within HPA measurement. The evidence-based knowledge presented here will assist the clinician and/or researcher to select the most appropriate tool and methodology for their defined aim.
Careful consideration of the study aim should precede choosing a particular tool and method for assessing HPA. Understanding their strengths and weaknesses is essential when considering available resources at the study site. The authors provide a considerations-checklist for designing a protocol to assess HPA in NMD in Appendix A.
CONFLICT OF INTEREST
The authors have no conflict of interest relevant to this study.
AUTHORS’ CONTRIBUTIONS
AJM carried out the study design, study selection process, data extraction, data interpretation, and manuscript drafting. JN and SC participated in the process of study selection, data extraction, and paper revision equally. JYH and HL supervised the whole manuscript process. All authors read, commented on and approved the final manuscript.
Notes
a ClinicalTrials.gov Identifier: NCT02858908
b ClinicalTrials.gov Identifier: NCT02118779
c ClinicalTrials.gov Identifier: NCT02398201
d ClinicalTrials.gov Identifier: NCT00847379
e ClinicalTrials.gov Identifier: NCT02500381, NCT01826474
f ClinicalTrials.gov Identifier: NCT02066519
Appendices
APPENDIX A
In this section the authors propose a checklist of considerations to have in mind prior to assessing and reporting habitual physical activity (HPA) in neuromuscular disorders (NMD). The following segment summarizes previously proposed methodological guidelines or strategies for assessing physical activity in population-based research [1, 41, 142, 152] and has been adapted based on this review’s findings and authors’ previous experience utilising these tools with this population. A standardized methodology such as the Delphi method should follow to develop and validate guidelines for HPA assessment in NMD [153].
HPA in NMD considerations checklist
1. Establish the aim for assessing HPA as part of your research question (i.e. What question(s) are you trying to answer?).
Do you want to:
-Identify any altered activity behaviour present in the specific disease group;
-Identify a specific dose of activity that differentiates patients based on health parameters;
-Measure an intervention effect;
-Measure activity or intervention compliance;
-Establish a surrogate outcome measure for disease progression;
-Identify ‘real-life’ barriers for patients’ best performance;
-Patients’ own perception of their own daily performance or participation?
2. Establish the variable(s) needed to fulfil your question.
Examples:
-Time spent on certain activity like: walking, sedentary time;
-Overall activity levels (PAL) or energetic estimations (EE or METs);
-Characterization of a specific type of activity (e.g. gait speed during walking in daily life, transitions, sleep patterns);
-Factors associated with HPA (like fatigue, falls, season effect).
3. Establish the level of priority the HPA outcome will have within your study. (i.e. primary or secondary outcome). This will define the time and resources to be invested.
4. Define your sample criteria.
-Establish your sample characteristics and identify those that may impact on the HPA (i.e. ambulation status, employment characteristics, level of independence).
5. Establish your sample number.
-It is expected that direct tools will allow a smaller sample but it will still vary depending on the variable to compare.
-Are there any known factors such as non-compliance or possible loss of data associated with your selected tool that could impact on your sample number?
6. Identify all possible HPA tools that could fulfil your study needs and identify each one’s strengths and weaknesses for your study aims and resources.
-Have these been used before in a similar population?
-Would they require a population-specific validation?
-Is there any established standard practice associated with the tool? Is this feasible in your study?
-Are your resources enough to fulfil the tool requirements (i.e. equipment costs, analysis, data rights, human resources, ethical approvals)?
-Does the research team involved have any previous experience with that tool or similar ones? If not, consider an interdisciplinary collaboration.
-Would only one tool be enough to fulfil the study aims?
7. Consider the participant’s potential acceptability. This can impact on the overall experience and final results.
-How many days of assessment (or questions) are really required (i.e. not too short, neither too long)? For this point, consider previous publications and special characteristics of your cohort (i.e. differences between paediatrics and adults, differences between employed and unemployed, weekends vs weekdays).
-Consider staff and patient’s acceptability when defining: [1] body location of the monitor (i.e. comfortable, easy to collocate, visually acceptable) or [2] the interview process for PRO.
8. Establish all the logistics required to increase the efficiency in your study.
-Establish standard operation procedures applicable for the site or sites involved.
-Consider appointing someone specific or a team for the coordination of the HPA operations.
-Consider staff training prior trial starts.
9. As soon as the HPA outcome to investigate and the HPA tool to use have been established, define the exact methodology to follow and to report as follows:
Direct (objective) HPA-tools | Indirect (reported outcomes) HPA-tools |
1. Rationale behind the outcome. | 1. Rationale behind the outcome. |
2. Validity and reliability available and background (references) supporting the tool and methodology selected. | 2. Validity and reliability available and background (references) supporting the tool selected. |
3. Established methodology: i.e. body-placement location, number and type of days, number of hours per day. | 3. Established methodology: i.e. location (clinic or home), respondent (patient, carer, researcher), format (electronic, paper). |
4. Definition of wear and non-wear periods. | 4. Specific management procedures for missing data. |
5. Definition of variables presented and background (references) for their use. | 5. Definition of summary variables to report (and references if applicable). |
6. Compliance criteria to include for analysis. | |
7. Specific management of missing or invalid data. |
ACKNOWLEDGMENTS
The authors acknowledge current funding grants from: [1] the National Institute of Health Research IHR and Wyck (PHENO-DM1 trial); and [2] the European Community’s Seventh Framework Programme (FP7/2007–2013) under grant agreement n° 305697 (OPTIMISTIC Trial) as partners Newcastle University, UK and Catt-Sci Ltd, UK.
A.C. Jimenez-Moreno PhD studentship is a combined funding from the Medical Research Council (MRC) Centre for Neuromuscular Diseases (reference G1002274, grant ID 98482), Consejo Nacional de Ciencia y Tecnologia (CONACYT), Mexico (ID 611819) and the Barbour Foundation, UK.
REFERENCES
[1] | Del Din S , Godfrey A , Mazza C , Lord S , Rochester L . Free-living monitoring of Parkinson’s disease: Lessons from the field. Mov Disord. (2016) ;31: (9):1293–313. |
[2] | Fini NA , Holland AE , Keating J , Simek J , Bernhardt J . How is physical activity monitored in people following stroke? Disabil Rehabil. (2015) ;37: (17):1717–31. |
[3] | Keawutan P , Bell K , Davies PS , Boyd RN . Systematic review of the relationship between habitual physical capacity and motor capacity in children with cerebral palsy. Res Dev Disabil. (2014) ;35: (6):1301–9. |
[4] | Abresch RT , Carter GT , Jensen MP , Kilmer DD . Assessment of pain and health-related quality of life in slowly progressive neuromuscular disease. Am J Hosp Palliat Care. (2002) ;19: (1):39–48. |
[5] | McDonald CM . Physical activity, health impairments, and disability in neuromuscular disease. Am J Phys Med Rehabil. (2002) ;81: (11 Suppl):S108–20. |
[6] | Cup EH , Pieterse AJ , Ten Broek-Pastoor JM , Munneke M , van Engelen BG , Hendricks HT , et al. Exercise therapy and other types of physical therapy for patients with neuromuscular diseases: A systematic review. Arch Phys Med Rehabil. (2007) ;88: (11):1452–64. |
[7] | Kalkman JS , Schillings ML , van der Werf SP , Padberg GW , Zwarts MJ , van Engelen BG , et al. Experienced fatigue in facioscapulohumeral dystrophy, myotonic dystrophy, and HMSN-I. J Neurol Neurosurg Psychiatry. (2005) ;76: (10):1406–9. |
[8] | Skalsky AJ , McDonald CM . Prevention and management of limb contractures in neuromuscular diseases. Phys Med Rehabil Clin N Am. (2012) ;23: (3):675–87. |
[9] | Aitkens S , Kilmer DD , Wright NC , McCrory MA . Metabolic syndrome in neuromuscular disease. Arch Phys Med Rehabil. (2005) ;86: (5):1030–6. |
[10] | Anziska Y , Sternberg A . Exercise in neuromuscular disease. Muscle Nerve. (2013) ;48: (1):3–20. |
[11] | Emery AE . Population frequencies of inherited neuromuscular diseases – a world survey. Neuromuscul Disord. (1991) ;1: (1):19–29. |
[12] | Mercuri E , Mayhew A , Muntoni F , et al. Towards harmonisation of outcome measures for DMD and SMA within TREAT-NMD; report of three expert workshops: TREAT-NMD/ENMC workshop on outcome measures, 12th-13th May 2007, Naarden, The Netherlands; TREAT-NMD workshop on outcome measures in experimental trials for DMD, 30th June-1st July 2007, Naarden, The Netherlands; conjoint Institute of Myology TREAT-NMD meeting on physical activity monitoring in neuromuscular disorders, 11th July 2007, Paris, France. Neuromuscul Disord. (2008) ;18: (11):894–903. |
[13] | Steins D , Dawes H , Esser P , Collett J . Wearable accelerometry-based technology capable of assessing functional activities in neurological populations in community settings: A systematic review. J Neuroeng Rehabil. (2014) ;11: :36. |
[14] | World Health Organization. International Classification of Functioning, Disability and Health: ICF. Towards a Common Language for Functioning, Disability and Health. WHO; Geneva 2001. |
[15] | Bjornson KF , Zhou C , Stevenson RD , Christakis D . Relation of stride activity and participation in mobility-based life habits among children with cerebral palsy. Arch Phys Med Rehabil. (2014) ;95: (2):360–8. |
[16] | Gill DL , Hammond CA , Reifsteck EJ , Jehu CM , Williams RA , Adams MM , et al. Physical activity and quality of life. J Prev Med Public Health. (2013) ;46: (Suppl 1):s28–s34. |
[17] | Berlin JE , Storti KL , Brach JS . Using activity monitors to measure physical activity in free-living conditions. Phys Ther. (2006) ;86: (8):1137–45. |
[18] | Mokkink LB , Prinsen CA , Bouter LM , de Vet HC , Terwee CB . The COnsensus-based Standards for the selection of health Measurement INstruments (COSMIN) and how to select an outcome measurement instrument. Braz J Phys Ther. (2016) ;20: (2):105–13. |
[19] | Trost SG . State of the art reviews: measurement of physical activity in children and adolescents. American Journal of Lifestyle Medicine. (2007) ;1: (4):299–314. |
[20] | Clanchy KM , Tweedy SM , Boyd RN . Validation of accelerometry in ambulatory children and adolescents with cerebral palsy. Eur J Appl Physiol. (2010) ;111: (12):2951–9. |
[21] | Trost SG , O’Neil M . Clinical use of objective measures of physical activity. Br J Sports Med. (2014) ;48: (3):178–81. |
[22] | Byrom B , Rowe DA . Measuring free-living physical activity in COPD patients: Deriving methodology standards for clinical trials through a review of research studies. Contemp Clin Trials. (2016) ;47: :172–84. |
[23] | Shephard RJ . Limits to the measurement of habitual physical activity by questionnaires. Br J Sports Med. (2003) ;37: :197–206. |
[24] | Sallis JF , Saelens BE . Assessment of physical activity by self-report: Status, limitations, and future directions. Res Q Exerc Sport. (2000) ;71: (2 Suppl):S1–14. |
[25] | Speight J , Shalleen M . FDA guidance on patient reported outcomes. BMJ. (2010) (340):c2921. |
[26] | Klein PD , James WP , Wong WW , Irving CS , Murgatroyd PR , Cabrera M , et al. Calorimetric validation of the doubly-labelled water method for determination of energy expenditure in man. Hum Nutr Clin Nutr. (1984) ;38: (2):95–106. |
[27] | Hills AP , Mokhtar N , Byrne NM . Assessment of physical activity and energy expenditure: An overview of objective measures. Front Nutr. (2014) ;1: :5. |
[28] | Falck RS , McDonald SM , Beets MW , Brazendale K , Liu-Ambrose T . Measurement of physical activity in older adult interventions: A systematic review. Br J Sports Med. (2015) . |
[29] | Van Remoortel H . et al. Validity activity monitors in health and chronic disease: A systematic review. Int J Behav Nutr Phys Act. (2012) ;9: :84. |
[30] | Bassett DRJ . Validity and reliability issues in objective monitoring of physical activity. Res Q Exerc Sport. (2000) ;71: (2). |
[31] | Andre D , Wolf DL . Recent advances in free-living physical activity monitoring: A review. J Diabetes Sci Technol. (2007) ;1: (5):760–767. |
[32] | Van Hees VT , Pias M , Taheran S , Ekelund U , Brage S . A method to compare traditional accelerometery data in physical activity monitoring. IEEE Int Symp “A World Wireless, Mob Multimed Networks”. (2010) :1–6. |
[33] | Hale LA , Pal J , Becker I . Measuring free-living physical activity in adults with and without neurologic dysfunction with a triaxial accelerometer. Arch Phys Med Rehabil. (2008) ;89: (9):1765–71. |
[34] | Clanchy KM , Tweedy SM , Boyd R . Measurement of habitual physical activity performance in adolescents with cerebral palsy: A systematic review. Dev Med Child Neurol. (2011) ;53: (6):499–505. |
[35] | Oftedal S , Bell KL , Mitchell LE , Davies PS , Ware RS , Boyd RN . A systematic review of the clinimetric properties of habitual physical activity measures in young children with a motor disability. Int J Pediatr. (2012) ;2012: :976425. |
[36] | McCrory MA , Kim HR , Wright NC , Lovelady CA , Aitkens S , Kilmer DD . Energy expenditure, physical activity, and body composition of ambulatory adults with hereditary neuromuscular disease. Am J Clin Nutr. (1998) ;67: (6):1162–9. |
[37] | McDonald CM , Widman LM , Walsh DD , Walsh SA , Abresch RT . Use of step activity monitoring for continuous physical activity assessment in boys with Duchenne muscular dystrophy. Arch Phys Med Rehabil. (2005) ;86: (4):802–8. |
[38] | Wiles CM , Busse ME , Sampson CM , Rogers MT , Fenton-May J , van Deursen R . Falls and stumbles in myotonic dystrophy. J Neurol Neurosurg Psychiatry. (2006) ;77: (3):393–6. |
[39] | Jimenez-Moreno AC , Newman J , Charman S , Catt M , Trenell M , Gorman G , Hogrel J-Y , Lochmüller H . Assessing habitual physical activity in neuromuscular diseases: Deriving methodology guidance through a systematic review. University of York Centre for Reviews and Dissemination. PROSPERO UK. CRD42016035428. |
[40] | Caspersen CJ , Powell KE , Christenson GM . Physical activity, exercise, and physical fitness: Definitions and distinctions for health-related research. Public Health Reports. (1985) ;100: (2):126–31. |
[41] | Matthews CE , Hagstromer M , Pober DM , Bowles HR . Best practices for using physical activity monitors in population-based research. Med Sci Sports Exerc. (2012) ;44: (1 Suppl 1):S68–76. |
[42] | Totoescu A , Le Moing AG , Moraux A , Hogrel JY , Vissiere D , Sinegre L , et al. S.P.7 Validation trial of a movement Holter monitor based on accelerometry for the non-ambulant neuromuscular patients. Neuromuscular Disorders. (2012) ;22: (9-10):877–8. |
[43] | Le Moing AG , Totoescu A , Moraux A , Hogrel JY , Vissiere D , Sinegre L , et al. S.P.8 Validation of linear accelerations and angular velocities to estimate the efficacy of a subject when performing a quantified task in a controlled environment. Neuromuscular Disorders. (2012) ;22: (9-10):877–8. |
[44] | Pollard A , Ramdharry GM , Moore S , Hallsworth K , Marsden JF , Reilly MM . Comparing activity levels between people with Charcot-Marie-Tooth disease and healthy controls – a pilot study. UK Neuromuscular Translational Research Conference: Neuromuscular Disorders; (2010) . p. S19. |
[45] | McDonald C , Coleman KL , Henricson E , Abresch RT , Eagle M , Florence J , et al. M.P.3.04 Assessment of StepWatch™ activity monitoring in phase 2b study of ataluren (PTC124) in nmDMD/BMD. Neuromuscular Disorders. (2009) ;19: (8-9):602. |
[46] | Veenhuizen Y , Cup EH , Groothuis JT , Hendriks JC , Adang EM , van Engelen BG , et al. Effectiveness and cost-effectiveness of a self-management group program to improve social participation in patients with neuromuscular disease and chronic fatigue: Protocol of the Energetic study. BMC Neurol. (2015) ;15: :58. |
[47] | Lassche S , Ottenheijm C , Voermans N , Westeneng H , Janssen B , Silvere M , et al. Determining the role of sarcomeric proteins in FSHD: A study protocol. BMC Neurol. (2013) ;13: :144–150. |
[48] | van Engelen B , OPTIMISTIC Consortium. Cognitive behaviour therapy plus aerobic exercise training to increase activity in patients with myotonic dystrophy type 1 (DM1) compared to usual care (OPTIMISTIC): Study protocol for randomised controlled trial. Trials. (2015) ;16: (1):224. |
[49] | Schönhofer B , Ardes P , Geibel M , Köhler D , Jones PW . Evaluation of a movement detector to measure daily activity in patients with chronic lung disease. European Respiratory Journal. (1997) ;10: (12):2814–9. |
[50] | Holtebekk ME , Berntsen S , Rasmussen M , Jahnsen RB . Physical Activity and Motor Function in Children and Adolescents with Neuromuscular Disorders. Pediatr Phys Ther. (2013) ;25: :415–20. |
[51] | Ollivier K , Hogrel J-Y , Gomez-Merino D , Romero NB , Laforte P , Eymard B , Portero P . Exercise tolerance and daily life in McArdle’s disease. Muscle & Nerve. (2005) ;31: (5):637–41. |
[52] | Shrader JA , Kats I , Kokkinis A , Zampieri C , Levy E , Joe GO , et al. A randomized controlled trial of exercise in spinal and bulbar muscular atrophy. Ann Clin Transl Neurol. (2015) ;2: (7):739–47. |
[53] | Wintzen AR , Lammers GJ , van Dijk JG . Does modafinil enhance activity of patients with myotonic dystrophy?: A double-blind placebo-controlled crossover study. J Neurol. (2007) ;254: (1):26–8. |
[54] | Davidson ZE , Ryan MM , Kornberg AJ , Walker KZ , Truby H . Strong correlation between the 6-minute walk test and accelerometry functional outcomes in boys with Duchenne muscular dystrophy. J Child Neurol. (2015) ;30: (3):357–63. |
[55] | Elliott SA , Davidson ZE , Davies PS , Truby H . Accuracy of parent-reported energy intake and physical activity levels in boys with duchenne muscular dystrophy. Nutr Clin Pract. (2015) ;30: (2):297–304. |
[56] | Jeannet PY , Aminian K , Bloetzer C , Najafi B , Paraschiv-Ionescu A . Continuous monitoring and quantification of multiple parameters of daily physical activity in ambulatory Duchenne muscular dystrophy patients. Eur J Paediatr Neurol. (2011) ;15: (1):40–7. |
[57] | Kilmer DD , Wright NC , Aitkens S . Impact of a home-based activity and dietary intervention in people with slowly progressive neuromuscular diseases. Arch Phys Med Rehabil. (2005) ;86: (11):2150–6. |
[58] | Apabhai S , Gorman GS , Sutton L , Elson JL , Plotz T , Turnbull DM , et al. Habitual physical activity in mitochondrial disease. PLoS One. (2011) ;6: (7):e22294. |
[59] | Phillips M , Flemming NKT . An exploratory study of physical activity and perceived barriers to exercise in ambulant people with neuromuscular disease compared with unaffected controls. Clinical Rehabilitation. (2009) ;22: :746–55. |
[60] | Anens E , Emtner M , Hellstrom K . Exploratory study of physical activity in persons with Charcot-Marie-Tooth disease. Arch Phys Med Rehabil. (2015) ;96: (2):260–8. |
[61] | Favejee MM , van den Berg LE , Kruijshaar ME , Wens SC , Praet SF , Pim Pijnappel WW , et al. Exercise training in adults with Pompe disease: The effects on pain, fatigue, and functioning. Arch Phys Med Rehabil. (2015) ;96: (5):817–22. |
[62] | Kalkman JS , Schillings ML , Zwarts MJ , van Engelen BG , Bleijenberg G . The development of a model of fatigue in neuromuscular disorders: A longitudinal study. J Psychosom Res. (2007) ;62: (5):571–9. |
[63] | Voet N , Bleijenberg G , Hendriks J , Padberg GW , van Engelen BGM , Geurts ACH . Both aerobic exercise and cognitive-behavioral therapy reduce chronic fatigue in FSHD. Neurology. (2014) ;83: :9. |
[64] | Busse ME , Pearson OR , Van Deursen R , Wiles CM . Quantified measurement of activity provides insight into motor function and recovery in neurological disease. J Neurol Neurosurg Psychiatry. (2004) ;75: (6):884–8. |
[65] | Rosenberg DE , Bombardier CH , Artherholt S , Jensen MP , Motl RW . Self-reported depression and physical activity in adults with mobility impairments. Arch Phys Med Rehabil. (2013) ;94: (4):731–6. |
[66] | Hawker GA , Ridout R , Harris VA , Chase CC , Fielding LJ , Biggar WD . Alendronate in the treatment of low bone mass in steroid-treated boys with Duchennes muscular dystrophy. Arch Phys Med Rehabil. (2005) ;86: (2):284–8. |
[67] | Elsworth C , Winward C , Sackley C , Meek C , Freebody J , Esset P , et al. Supported community exercise in people with long-term neurological conditions: A phase II randomized controlled trial. Clini Rehabil. (2011) ;25: (7):588–98. |
[68] | Washburn RA , Smith KW , Jette AM , Janney CA . The Physical Activity Scale for the Elderly (PASE): Development and evaluation. J Clin Epidemiol. (1993) ;46: (2):153–62. |
[69] | Silsbury Z , Goldsmith R , Rushton A . Systematic review of the measurement properties of self-report physical activity questionnaires in healthy populations. BMJ Open. (2015) ;5: (e008430). |
[70] | Block VA , Pitsch E , Tahir P , Cree BA , Allen DD , Gelfand JM . Remote physical actiivity monitoring in neurological disease: A systematic review. PLoS One. (2016) ;11: (4):e0154335. |
[71] | Mitchell LE , Ziviani J , Oftedal S , Boyd RN . A systematic review of the clinimetric properties of measures of habitual physical activity in primary school aged children with cerebral palsy. Res Dev Disabil. (2013) ;34: (8):2419–32. |
[72] | Sun F , Norman I , While A . Physical acitivity in older people: A systematic review. BMC Public Health. (2013) ;13: :449–464. |
[73] | Phillips RL , Olds T , Boshoff K , Lane AE . Measuring activity and participation in children and adolescents with disabilities: A literature review of available instruments. Aust Occup Ther J. (2013) ;60: :288–300. |
[74] | Bouchard C , Tremblay A , Leblanc C , Lortie G , Savard R , Theriault G . A method to assess energy expenditure in children and adults. Am J Clin Nutr. (1983) ;37: (3):461–7. |
[75] | Ainsworth BE , Bassett DRJ , Strath SJ , Swartz AM , O’Brien WL , Thompson RW , et al. Comparison of three methods for measuring the time spent in physical activity. Med Sci Sports Exer. (2000) ;32: :S457–S64. |
[76] | Tudor-Locke C , Ainsworth BE , Washington TL , Troiano R . Assigning metabolic equivalent values to the 2002 census occupational classification system. J Phys Act Health. (2011) ;8: (4):581–6. |
[77] | Kowalski KC , Crocker PR , Faulkner RA . Physical activity questionnaire for older children. Pediatr Exerc Sci. (1997) ;9: :174–86. |
[78] | Philippaerts RM , Westerterp KR , Lefevre J . Doubly labelled water validation of three physical activity questionnaires. Int J Sports Med. (1999) ;20: (5):289–9. |
[79] | Philippaerts RM , Westertero KR , Lefevre J . Comparison of two questionnaires with a tri-axial accelerometer to assess physical activity patterns. Int J Sports Med. (2001) ;22: (1):34–9. |
[80] | Washburn RA , Ficker JL . Physical activity scale for the elderly (PASE): The relationship with activity measured by a portable accelerometer. J Sports Med Phys Fitness. (1999) ;39: :336–40. |
[81] | Hagstromer M , Oja P , Sjostrom M . The International Physical Activity Questionnaire (IPAQ): A study of concurrent and construct validity. Public Health Nutrition. (2005) ;9: (6):755–62. |
[82] | McKeon M , Slevin E , Taggart L . A pilot survey of physical activity in men with an intellectual disability. J Intellect Disab. (2013) ;17: (2):157–67. |
[83] | Wareham NJ , Jakes RW , Rennie KL , Mitchell JM , Hennings S , Day NE . Validity and repeatability of the EPIC-Norfolk Physical Activity Questionnaire. International Epidemiological Association. (2002) ;31: :168–74. |
[84] | Kayes NM , McPherson KM , Taylor DJ , Schulter PJ , Wilson BJ , Kolt GS . The Physical Activity and Disability Survey (PADS): Reliability, validity and acceptability in people with multiple sclerosis. Clin Rehabil. (2007) ;21: (7):628–39. |
[85] | Motl RW , McAuley E , Snook EM , Scott JA . Validity of physical activity measures in ambulatory individuals with multiple sclerosis. Disability and Rehabilitation. (2005) ;28: (18):1151–6. |
[86] | Page AS , Cooper AR , Griew P , Davis L , Hillsdon M . Independent mobility in relation to weekday and weekend physical activity in children aged 10-11 years: The PEACH Project. Int J Behav Phys Act. (2009) ;7: (6):2. |
[87] | Martinez-Gomez D , Puertollano MA , Warnberg J , Calabro MA , Welk GJ , Sjostrom M , et al. Comparison of the ActiGraph accelerometer and Bouchard diary to estimate energy expenditure in Spanish adolescents. Nutr Hos. (2009) ;24: (6):701–10. |
[88] | Bonnefoy M , Normand S , Pachiaudi C , Lacour JR , Laville M , Kostka T . Simultaneous validation of ten physical activity questionnaires in older men: A doubly labeled water study. J Am Geriatr Soc. (2001) ;49: (1):28–35. |
[89] | Southward EF , Page AS , Wheeler BW , Cooper AR . Contribution of the school journey to daily physical activity in children aged 11-12 years. Am J Prev Med. (2012) ;43: (2):201–104. |
[90] | Spurr GB , Prentice AM , Murgatroyd PR , Goldberg GR , Reina JC , Christman NT . Energy expenditure from minute-byminute heart-rate recording: Comparison with indirect calorimetry. Am J Clin Nutr. (1988) ;48: (3):552–559. |
[91] | Emons HJG , Groenenboom DC , Westerterp KR , Saris WHM . Comparison of heart rate monitoring combined with indirect calorimetry and the doubly labeled water (2H2 18O) method for the measurement of energy expenditure in children. Eur J Appl Physiol. (1992) ;65: :99–103. |
[92] | Leonard WR . Measuring human energy expenditure: What have we learned from the flex-heart rate method? Am J Hum Biol. (2003) ;15: :479–89. |
[93] | Ceesay SM , Prentice AM , Day KC , et al. The use of heart rate monitoring in the estimation of energy expenditure: A validation study using indirect whole-body calorimetry. Br J Nutr. (1989) ;61: :175–86. |
[94] | Maltais DSEB , Pierrynowski MR , Galea VA , Bar-Or O . Physical activity level is associated with the O2 cost of walking in cerebral palsy. Med Sci Sports Exerc. (2005) ;37: (3):347–53. |
[95] | van den Berg-Emons RJG , Saris WHM , Westerterp KR , Van Baak MA . Heart rate monitoring to assess energy expenditure in children with reduced physical activity. Med Sci Sports Exerc. (1996) ;28: (4):496–501. |
[96] | McDonald CM , Widman L , Abresch RT , Walsh SA , Walsh DD . Utility of a step activity monitor for the measurement of daily ambulatory activity in children. Arch Phys Med Rehabil. (2005) ;86: (4):793–801. |
[97] | Brage S , Westgate K , Franks PW , Stegle O , Wright A , Ekelund U , et al. Estimation of free-living energy expenditure by heart rate and movement sensing: A doubly-labelled water study. PLoS One. (2015) ;10: (9):e0137206. |
[98] | Bjornson KF , Belza B , Kartin D , Logsdon R , McLaughlin JF . Ambulatory physical activity performance in youth with cerebral palsy and youth who are developing typically. Phys Ther. (2007) ;87: (3):248–60. |
[99] | Bjornson KF , Belza B , Kartin D , Logsdon R , McLaughlin J , Thompson EA . The relationship of physical activity to health status and quality of life in cerebral palsy. Pediatr Phys Ther. (2008) ;20: (3):247–53. |
[100] | Stevens SL , Holbrook EA , Fuller DK , Morgan DW . Influence of age on step activity patterns in children with cerebral palsy and typically developing children. Arch Phys Med Rehabil. (2010) ;91: (12):1891–6. |
[101] | van Wely L , Becher JG , Balemans AC , Dallmeijer AJ . Ambulatory activity of children with cerebral palsy: Which characteristics are important? Dev Med Child Neurol (2012) ;54: (5):436–42. |
[102] | Nguyen HQ , Steele BG , Dougherty CM , Burr RL . Physical activity patterns of patients with cardiopulmonary illnesses. Arch Phys Med Rehabil. (2012) ;93: (12):2360–6. |
[103] | Patel SA , Benzo RP , Slivka WA , Sciurba FC . Activity monitoring and energy expenditure in COPD patients: A validation study. COPD. (2007) ;4: (2):107–12. |
[104] | Dwyer TJ , Alison JA , McKeough ZJ , Elkins MR , Bye PT . Evaluation of the SenseWear activity monitor during exercise in cystic fibrosis and in health. Respir Med. (2009) ;103: (10):1511–7. |
[105] | Hill K , Dolmage TE , Woon L , Goldstein R , Brooks D . Measurement properties of the SenseWear armband in adults with chronic obstructive pulmonary disease. Thorax. (2010) ;65: (6):486–91. |
[106] | Van Remoortel H , Hornikx M , Demeyer H , et al. Daily physical activity in subjects with newly diagnosed COPD. Thorax. (2013) ;68: (10):962–3. |
[107] | Munguia-Izquierdo D , Santalla A , Legaz-Arrese A . Evaluation of a wearable body monitoring device during treadmill walking and jogging in patients with fibromyalgia syndrome. Arch Phys Med Rehabil. (2012) ;93: (1):115–22. |
[108] | St-Onge M , Mignault D , Allison DB , Rabasa-Lhoret R . Evaluation of a portable device to measure daily energy expenditure in free-living adults. Am J Clin Nutr. (2007) ;85: :742–9. |
[109] | Conger SA , Scott SN , Fitzhugh EC , Thompson DL , Bassett DR Jr . Validity of physical activity monitors for estimating energy expenditure during wheelchair propulsion. J Phys Act Health. (2015) ;12: (11):1520–6. |
[110] | Drenowatz C , Eisenmann JC . Validation of the SenseWear Armband at high intensity exercise. Eur J Appl Physiol. (2011) ;111: (5):883–7. |
[111] | Arvidsson D , Slinde F , Larsson S , Hulthen L . Energy cost of physical activities in children: Validation of SenseWear Armband. Med Sci Sports Exerc. (2007) ;39: (11):2076–84. |
[112] | Coote S , O’Dwyer C . Comparative validity of accelerometer-based measures of physical activity for people with multiple sclerosis. Arch Phys Med Rehabil. (2012) ;93: (11):2022–8. |
[113] | Hermann A , Ried-Larsen M , Jensen AK , Holst R , Andersen LB , Overgaard S , et al. Low validity of the Sensewear Pro3 activity monitor compared to indirect calorimetry during simulated free living in patients with osteoarthritis of the hip. BMC Musculoskelet Disord. (2014) ;15: :43–50. |
[114] | Maltais DB , Pierrynowski MR , Galea VA , Matsuzaka A , Bar-Or O . Habitual physical activity levels are associated with biomechanical walking economy in children with cerebral palsy. Am J Phys Med Rehab. (2005) ;84: (1):36–45. |
[115] | Gorter JW , Noorduyn SG , Obeid J , Timmons BW . Accelerometry: A feasible method to quantify physical activity in ambulatory and nonambulatory adolescents with cerebral palsy. Int J Pediatr. (2012) :1–6. |
[116] | Puyau MR , Vohra FA , Zakeri IF , Butte NF . Prediction of activity energy expenditure using accelerometers in children. Med Sci Sports Exerc. (2004) ;36: (9):1625–31. |
[117] | Heil DP . Predicting activity energy expenditure using the Actical activity monitor. Res Q Exerc Sport. (2006) ;77: (1):64–80. |
[118] | Wong SL , Colley R , Connor Gorber S , Tremblay M . Actical accelerometer sedentary activity thresholds for adults. J Phys Act Health. (2011) ;8: (4):587–91. |
[119] | Esliger DW , Probert A , Connor Gorber S , Bryan S , Laviolette M , Tremblay MS . Validity of the Actical accelerometer step-count function. Med Sci Sports Exerc. (2007) ;39: (7):1200–4. |
[120] | Rosenkranz R , Weber C , Rosenkranz S . Validity of the Actical accelerometer step count function in children. J Sci Med Sport. (2010) ;13: :e97. |
[121] | Kayes NM , Schluter PJ , McPherson KM , Leete M , Mawston G , Taylor D . Exploring actical accelerometers as an objective measure of physical activity in people with multiple sclerosis. Arch Phys Med Rehabil. (2009) ;90: (4):594–601. |
[122] | Moore R , Berlowitz D , Denehy L , Bruce J , McDonald B , Christine F . Comparison of pedometer and activity diary for measurement of physical activity in chronic obstructive pulmonary disease. J Cardiopulm Rehab Prev. (2009) ;29: (1):57–61. |
[123] | Tudor-Locke C , Craig CL , Aoyagi Y , Bell RC , Croteau KA , De Bourdeaudhuij I , et al. How many steps/day are enough? For older adults and special populations. Int J Behav Nutr Phys Act. (2011) ;8: :80. |
[124] | Bassett DR , John D . Use of pedometers and accelerometers in clinical populations: Validity and reliability issues. Phys Ther Reviews. (2010) ;15: (3):135–42. |
[125] | Elsworth C , Dawes H , Winward C , Howells K , Collett J , Dennis A , Sackley C , Wade D . Pedometer step counts in individuals with neurological conditions. Clin Rehabil. (2009) ;23: (2):171–5. |
[126] | Salarian A , Russmann H , Vingerhoets FJ , Aminian K . Ambulatory monitoring of physical activities in patients with Parkinson’s disease. IEEE Trans Biomed Eng. (2007) ;54: :22296–9. |
[127] | Najafi B , Aminian K , Paraschiv-Ionescu A , Loew F , Bula C , Robert P . Ambulatory system for human motion analysis using a kinematic sensor: Monitoring a daily physical activity in the elderly. IEEE Trans Biomed Eng. (2003) ;50: :711–23. |
[128] | Page AS , Cooper AR , Griew P , Jago R . Independent mobility, perceptions of the built environment and children’s participation in play, active travel and structured exercise and sport: The PEACH Project. Int J Behav Nutr Phys Act. (2010) ;7: :17–26. |
[129] | Washburn RA , Zhu W , McAuley E , Frogley M , Figoni SF . The physical activity scale for individuals with physical disabilities: Development and evaluation. Arch Phys Med Rehabil. (2002) ;83: :193–200. |
[130] | Hallal PC , Victora CG . Reliability and validity of the International Physical Activity Questionnaire (IPAQ). Med Sci Sports Exerc. (2004) ;36: (3):556. |
[131] | Rosenberg DE , Bombardier CH , Artherholt S , Jensen MP , Motl RW . Self-reported depression and physical activity in adults with mobility impairments. Arch Phys Med Rehabil. (2013) ;94: (4):731–6. |
[132] | São-João T , Rodrigues R , Gallani M , Miura C , Domingues Gde B , Godin G . Cultural adaptation of the Brazilian version of the Godin-Shephard Leisure-Time Physical Activity Questionnaire. Rev Saude Publica. (2013) ;47: (3):479–87. |
[133] | João T , Rodrigues R , Gallani M , Miura C , Domingues Gde B , Amireault S , et al. Validity of the Brazilian version of the Godin-Shephard Leisure-Time Physical Activity Questionnaire. Cad Saude Publica. (2015) ;31: (9):1825–38. |
[134] | Pols MA , Peeters PHM , Bueno-de-Mesquita HB , Ocke MC , Wentink CA , Kemper HCG , et al. Validity and repeatability of a modified baecke questionnaire on physical activity. International Journal of Epidemiology. (1995) ;24: (2):381–8. |
[135] | Vercoulen JHMM , Swanink CMA , Fennins JFM , et al. Dimensional assessment of chronic fatigue syndrome. J Psychosom Res. (1994) ;38: :383–93. |
[136] | Vercoulen JH , Hommes OR , Swanink CM , Jongen PJ , Fennis JF , Galama JM , et al. The measurement of fatigue in patients with multiple sclerosis: A multidimensional comparison with patients with chronic fatigue syndrome and healthy subjects. Arch Neurol. (1996) ;53: (7):642–9. |
[137] | Rongen-van Dartel SA , Repping-Wuts H , van Hoogmoed D , Knoop H , Bleijenberg G , van Riel PL , et al. Relationship between objectively assessed physical activity and fatigue in patients with rheumatoid arthritis: Inverse correlation of activity and fatigue. Arthitis Care Res (Hoboken). (2014) ;66: (6):852–60. |
[138] | van der Werf SP , Prins JB , Vercoulen JH , van der Meer JW , Bleijenberg G . Identifying physical activity patterns in chronic fatigue syndrome using actigraphic assessment. J Psychosom Res. (2000) ;49: (5):373–9. |
[139] | Kilmer DD , Wright NC , Aitkens S . Impact of a home-based activity and dietary intervention in people with slowly progressive neuromuscular diseases. Arcg Phys Med Rehabil. (2005) ;86: (11):2150–6. |
[140] | Le Moing AG , Seferian AM , Moraux A , Annoussamy , Dorveaux E , Gasnier E , et al. Movement monitor based on magneto-inertial sensors for non-ambulant patients with Duchenne muscular dystrophy. A pilot study in controlled environment. PLoS One. (2016) ;11: (6):e0156696. |
[141] | Cavanaugh JT , Ellis TD , Earhart GM , Ford MP , Foreman KB , Dibble LE . Capturing ambulatory activity decline in Parkinson’s disease. J Neurol Phys Ther. (2012) ;36: (2):51–7. |
[142] | Warren JM , Ekelund U , Besson H , Mezzani A , Geladas N , Vanhees L . Assessment of physical activity – a review of methodologies with reference to epidemiological research: A report of the exercise physiology section of the European Association of Cardiovascular Prevention and Rehabilitation. Eur J Cardiovasc Prev Rehabil. (2010) ;17: :127–39. |
[143] | Fitzpatrick R , Davey C , Buxton MJ , Jones DR . Evaluating patients based outcome measures for use in clinical trials. Health Technol Assess. (1998) ;2: :1–71. |
[144] | Prince SA , Adamo KB , Hamel ME , Hardt J , Gorber SC , Tremblay M . A comparison of direct versus self-report measures for assessing physical activity in adults: A systematic review. Int J Behav Nutr Phys Activ. (2008) ;5: (56). |
[145] | Neilson HK , Robson PJ , Friedenreich CM , Csizmadi I . Estimating activity energy expenditure: How valid are physical activity questionnaires? Clin Nutr (2008) ;87: :279–91. |
[146] | van der Berg-Emons R , L’Ortye AA , Buffart LM , Nieuwenhuijsen C , Nooijen CF , Bergen MP , et al. Validation of the physical activity scale for individuals with physical disabilities. Arch Phys Med Rehabil. (2011) ;92: :923–8. |
[147] | Bachasson D , Moraux A , Ollivier G , Decostre V , Ledoux I , Gidaro T , et al. Relationship between muscle impairments, postural stability, and gait parameters assessed with lower-trunk accelerometry in myotonic dystrophy type 1. Neuromuscul Disord. (2016) ;26: (7):428–35. |
[148] | Barak S , Wu SS , Dai Y , Duncan PW , Behrman AL . Adherence to accelerometry measurement of community ambulation poststroke. Phys Ther. (2014) ;94: (1):101–10. |
[149] | Gorter JW , Noorduyn SG , Obeid J , Timmons BW . Accelerometry: A feasible method to quantify physical activity in ambulatory and nonambulatory adolescents with cerebral palsy. Int J Paed. (2012) ;50: :711–23. |
[150] | Soundy A , Taylor A , Faulkner G , et al. Psychometric properties of the 7-Day Physical Activity Recall questionnaire in individuals with severe mental illness. Arch Psychiatr Nurs. (2007) ;21: (6):309–16. |
[151] | Erickson KI , Barr LL , Wesintein AM , Banducci SE , Akl SL , Santo NM , et al. Measuring physical activity using accelerometery in a community sample with dementia. J Am Geriat Soc. (2013) ;61: (1):158–9. |
[152] | Strath SJ , Kaminsky LA , Ainsworth BE , Ekelund U , Freedson PS , Gary RA , et al. Guide to the Assessment of Physical Activity: Clinical and Research Applications: A scientific statement from the American Heart Association. Circulation. (2013) ;128: :2259–79. |
[153] | Sinha IP , Smyth RL , Williamson PR . Using the delphi technique to determine which outcomes to measure in clinical trials: Recommendations for the future based on a systematic review of existing studies. PLos Med. (2011) ;8: (1):e1000393. |
Figures and Tables
Fig.1
Literature selection Flow Diagram.
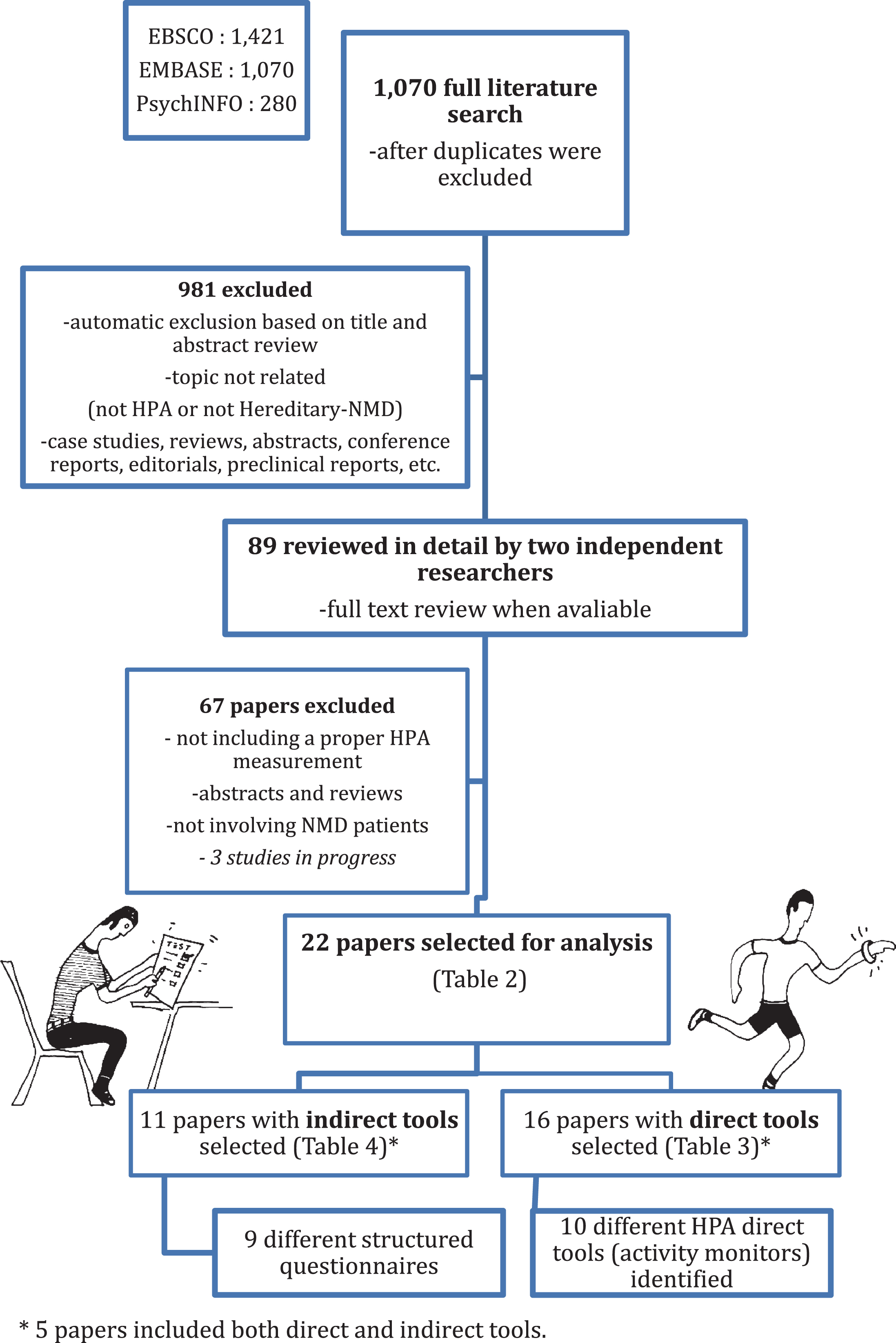
Fig.2
Types of direct HPA tools reported. Two studies combined a heart rate (HR) monitor with an additional activity monitor: [1] Yamax Digi-Walker and [2] StepWatch Activity Monitor (SAM) [37].
![Types of direct HPA tools reported. Two studies combined a heart rate (HR) monitor with an additional activity monitor: [1] Yamax Digi-Walker and [2] StepWatch Activity Monitor (SAM) [37].](https://content.iospress.com:443/media/jnd/2017/4-1/jnd-4-1-jnd160195/jnd-4-jnd160195-g002.jpg)
Table 1
Direct HPA-tools report – methodological variables for analysis
Area of Consideration | Variable reviewed | Definition |
MONITOR CHARACTERISTICS | Activity monitor | Device manufacturer and model. |
Body location | Placement location of device. | |
DATA COLLECTION | Wear period | Requested days or time to wear the device. |
Number of hours per day | Number of hours per day requested to be used. Identification whether worn full-time or just worn whilst awake. | |
DATA MANAGEMENT | Number of valid days | Number of days of data considered for the analysis. Criteria for days excluded. |
Definition of non-wear period | Specific criteria to identify a non-worn device. | |
Missing data methodology | Methodology and criteria to handle missing data. | |
DATA ANALYSIS | Endpoints analysed and reported | PA measurement(s) used, definition(s) and units presented. |
OTHERS | Quality assurance tool | Information about any additional tool used for quality control – i.e. to verify activity or non-activity periods detected by the device. |
Methodology Reference | Inclusion of references to any prediction values. Classification method used or directly to device developer. |
Table 2
Summary of all identified papers assessing HPA in NMD
Paper | Study sample (mean age in years±SD) | Study design (follow up duration) | Primary aim of study | HPA measurement tool | Reported habitual physical activity (HPA)-related results |
∧no SD provided NMD: mixed sample of neuromuscular disorders. DMD: Duchenne muscular dystrophy DM1: Myotonic dystrophy type 1 SBMA: spinal and bulbar muscular atrophy CMT: Charcot-Marie Tooth | RCT: Randomised Controlled Trial C: Cross-sectional L: Longitudinal | D: direct or objective tool I: indirect or patient reported (PRO) tool | EE: energy expenditure TEE: total EE REE: resting EE PA: physical activity PAL: PA levels | ||
McCrory et al. 1998 [36] | 26 NMD patients (45±15) 19 healthy controls | L (1 week) | (1) Test the hypothesis that REE, TEE and PAL are altered in ambulant NMD subjects and (2) Determine the feasibility of plethysmography | D: Polar heart-rate monitor worn for 3 successive days | Estimated time of PA was significantly lower in the NMD group (74±45 mins in NMDs vs. 206±110 mins in controls, p < 0.03). |
Busse et al. 2004 [64] | 10/30 NMD patients/total sample (mean 52.1±12.5) 30 healthy controls | L (7 days). | Investigate the validity and application of long-term ambulatory monitoring in a neurological population | D: StepWatch activity monitor (SAM) worn for 7 consecutive days | The NMD group had a significantly lower mean step count than the healthy control group (–2,365 95% CI –861 to –3,868). A moderate correlation was noted between gait speed and mean step count (r = 0.45, p = 0.01) in the patient groups. |
Ollivier et al. 2004 [51] | 30 McArdle’s disease patients (38±17) 87 healthy controls | C (single visit) | Describe a population suffering from McArdle’s disease regarding daily-life experiences and clinical symptoms. | I: Leisure activity questionnaire and Bouchard questionnaire | After normalizing to body weight, the daily energy expenditure (EE) estimated was not different between patients and control subjects (44.1±6.9 and 44.5±5.6 kcal/kg, respectively; p = 0.8) |
Hawker et al. 2005 [66] | 16 DMD boys (10.8, ∧range 6.9–15.6) | L (2 years) | Examine the side-effects, profile and effect on bone density in alendronate-treated DMD boys | I: Modification of Baecke Physical Activity Questionnaire (PRO) | The number of participants in high-energy expenditure activities decreased from 4 at baseline, to none at follow-up, and patients walking >6 km/week decreased from 10 to 4. |
McDonald et al. 2005 [37] | 16 DMD boys (9.1±2.1) 21 healthy controls | C | (1) Evaluate the SAM monitor as a measurement for HPA in DMD boys (accuracy, reliability and validity) | D: StepWatch activity monitor (SAM) D: Polar Vantage heart rate monitors Both worn for 3 days I: Activity and sleep-wake diary for quality control (PRO) | D: DMD subjects demonstrated prolonged inactivity periods (fewer steps per day) than controls. Control group = 6,311±493 vs. DMD group 4,456±513, (p = 0.014). Increased HR at rest in DMD boys whereas the Max-HR reached at same step rate was lower. Poor correlation between HR and step rate in the DMD group (r = 0.3) and not in controls. Strong correlation between step count and BMI and body fat (%) in the DMD group. |
Aitkens et al. 2005 [9] | 11 NMD patients (50.6±3.7) 8 able-bodied controls. | L (2.5 years) (This study is a follow-up from McCrory et al. 1998) | Determine risk factors for Metabolic Syndrome in NMD | D: Portable heart rate monitors I: Written Activity Record (PRO) | D &I: Decreased activity within patient group at baseline (144 min/d vs. 214, p = 0.037) and on PA Levels (PAL) (1.6 vs. 2.1 p = 0.027) but no difference on the Resting EE (REE). At follow up, the patient group showed a further reduction (–14±17 min/d) whereas controls increased (77±49 min/d) Still, at the 2.5 years follow-up there were no associated significant changes regarding the prevalence of metabolic syndrome. |
Kilmer et al. 2005 [57] | 20 NMD patients (49.9±13.2) | L (12 months) | Effectiveness of a home-based activity and dietary intervention | D: Heart rate monitor D: Pedometer Both worn for 3 successive days | Increased activity post-intervention was noted with a significant increment in step count of 27% (p = 0.001) after 6 months from baseline. |
Wiles et al. 2006 [38] | 13 DM1 patients (46.5±1.68) 12 healthy controls | L (13 weeks) | Investigate the incidence of falls and stumbles in DM1 and its association with relevant clinical features | D: StepWatch activity monitor (SAM) worn for 7 consecutive days | Falls and stumbles were expressed in relation to step count; patients had significantly more events per 5,000 steps taken compared to healthy volunteers (p = 0.007). Within the patient group, ankle strength had the highest correlation with gait speed (r = 0.92, p < 0.001). |
Kalkman et al. 2007 [62] | 198 NMD patients (41.3±9.6) | L (18 months) | Identify factors that predict fatigue in NMD and contribute to its persistence | D: actometer (Actilog V3.0) worn for 12 consecutive days I: Subscales of mobility and ambulation of the SIP (Sickness Impact Profile) (PRO) | D: physical activity levels (PAL) differed considerably between the three NMD groups (DM, FSHD and CMT). For the overall sample, actometer reports correlated significantly with reported fatigue (r = –0.316, p < 0.01) and functional impairment (r = –0.423, p < 0.01). However, when analysed by sub-groups, MD’s correlation to fatigue disappeared. D &I: Each HPA variable showed a stronger correlation to impairment rather than fatigue. |
Wintzen et al. 2007 [53] | 13 DM1 patients (43.5±13.9) | RCT (5 weeks) | Assess changes in spontaneous activity as a treatment (modafinil) effect | I: novel’ PA-increment scoring (1–5) system for patients and carers (PRO) | No significant findings. |
Phillips et al. 2009 [59] | 13 NMD patients (44±11.8) 18 controls | C (single visit) | Explore types and levels of PA and barriers to exercise in people with NMD compared to healthy controls | D: Polar heart rate monitor worn for 3 days (D) I: PA diary for quality control (PRO). I: The EPIC-Norfolk Physical Activity Questionnaire-2 completed at home (PRO) | D: The average of active minutes per day was significantly higher in the control group (283 vs. 129 vs. NMD). No PA difference shown on the NMD group between weekdays and weekends compared to controls. I: The reported total hours spent in PA was significantly higher in the control group, with a total of 38 (IQ 32) hours/week on the NMD group vs. 63 [16] in the control group. |
Apabhabi et al. 2011 [58] | 100 Mitochondrial disease patients (50±12) – 100 healthy controls | C (single visit) | Characterize HPA in patients with mitochondrial disease and evaluate the relationship with genotype and phenotype | D: SenseWear Armband activity monitor (SWA) worn over 3 days I: International Physical Activity Questionnaire (IPAQ) long version (PRO) | D: On average, patients walked 3,041 steps/day less than control (95%, CI 1,966 – 4,117). Disease severity explained 4 to 15% of the PA variance. D &I: Accelerometer measures showed a low to moderate association to the IPAQ score; r value ranging from 0.25 to 0.35 (95% CI 0.05–0.5) |
Jeannet et al. 2011 [56] | 5 DMD boys (∧age range 4 – 6 years) – Plus 20 DMD on the validation study. | L (1 month) | (1) Assess the feasibility and accuracy of a home environment PA monitoring in DMD and (2) assess if Prednisolone could improve HPA | D: Autonomous Sensing Unit Recorder (ASUR) worn for two consecutive days | Overall the group went from a total of 18,942 (±3,137) to 22, 087 (±2,328) steps. Increase seen in all patients except one. Walking cadence increased in all patients at follow up. Patients spent 87% of the monitoring time in some other activity than walking. 91%–95% of walking episodes lasted <1 min |
Elsworth et al. 2011 [67] | 19/99 NMD patients/total sample (56±12.9) | RCT &I (12 weeks) | Examine the feasibility and safety of community exercise for long-term neurological conditions | I: Physical Activity Scale for Elderly (PASE) (PRO) [68] and records of gym attendance D: StepWatch Activity Monitor (SAM) for 8 consecutive days (D) | Overall PA levels as measured by both step counts (D) and total PASE score (I) did not change significantly during the intervention (p > 0.05). |
Rosenberg et al. 2013 [65] | 321/1676 NMD patients/total sample [56] | C (single visit) | Test hypothesis that depression and physical activity are related in adults with mobility impairments | I: International Physical Activity Questionnaire (IPAQ) (PRO) I: Godin Leisure Time Exercise Questionnaire (GLTEQ) (PRO) | The IPAQ and GLTEQ explained a small but statistically significant variance in the depression scores of participants which were not confounded by condition, age, or mobility status (IPAQ R2 = .004; GLTEQ R2 = .02; both p < .02). |
Holtebekk et al. 2013 [50] | 17 NMD patients (∧age range 10–18) | C (single visit) | Determine level of physical function and PA in daily life activity in children and adolescents with NMD | D: SenseWear Armband activity monitor (SWA) worn for 4 successive days I: Computer-based questionnaire from the Personal and Environment Associations with Children’s Health (PEACH) study (PRO) | D: None of the participants registered vigorous PA. 6MWT correlated to the total steps in daily life (r = 0.067, p < 0.01). Significantly lower levels of PA were reported on weekends compared to weekdays. Results were compared to previous publications showing >50% less of overall activity. I: Only 3 participants were physically active during school breaks. 9 participants reported regular physical activity in leisure time. |
Voet et al. 2014 [63] | 77 FSHD patients (28 exercise, 25 CBT and 24 usual care) | RCT &I (28 weeks) | Study the efficacy of Exercise Therapy (AET) and Cognitive Based Therapy (CBT) for decreasing chronic fatigue in patients with FSHD. | D: actometer (Activlog V3.0) worn for 12 consecutive days I: Daily Observed Spontaneous PA for 2 weeks (PRO) I: Reduced PA section of the CIS questionnaire (PRO) | D &I: patients receiving CBT had an increase in registered (D) and experienced (PRO), physical activity and sleep quality plus on reported social participation. Patients receiving AET had an increase in registered (D) physical activity only. The increase in registered (D) physical activity in both groups and the improvement in social participation following CBT were still present at follow-up. |
Elliott et al. 2014 [55] | 14 DMD boys (8.44±1.9) | C (single visit) | Assess if parents of DMD patients accurately report their child’s Energy Intake (EI) and PA compared to a reference measure of total energy expenditure (TEE) | I: Simplified version of Bouchard et al. activity diary (PRO) | No difference between mean measured PAL and mean estimated PAL. A mean margin of error of –0.08±0.25 between the measures was detected (95% confidence of agreement of –0.57 to 0.40) |
Shrader et al. 2015 [52] | 54 SBMA adult men (>18 years) | RCT (12 weeks). | Examine the effects of a home-based functional exercise programme on physical function and endurance | D: Actical accelerometer worn for 10 days | High functioning individuals in the exercise groups increased PA levels (+7,848 counts) compared to the group performing only stretching (control) (+1,171 counts) but not significantly (p = 0.19). |
Davidson et al. 2015 [54] | 16 DMD boys (9.0±2.1) 13 healthy controls | C (single visit) | Describe the relationship between community ambulation and functional assessment (6MWT) in DMD. Explore accelerometry as a complimentary outcome measure | D: StepWatch activity monitor (SAM) worn for 5 days | DMD boys were inactive for longer periods of time compared to healthy controls (1103±134 minutes vs. 1016±62, p = .036) and spent less time performing high level activities (25±17 vs. 53±34 minutes, p = 0.018). The 6MWT distance correlated to the sedentary time measured by StepWatch (r = 0.8, p = .0003) |
Anens et al. 2015 [60] | 44 CMT patients (59.5 ∧inter quartile range 45.3–64.8) | C (single visit – survey) | Explore the perceived facilitators and barriers to PA, and the use of a quantitative approach to examine PA | I: Physical Activity Disability Survey-Revised (PADS-R) | Higher PA (vs. low PA) was reported by younger participants, those still working and that walked outdoors. They were also less limited in their activities, experienced less fatigue and e more enjoyment from PA. When correlated: PA self-efficacy was a stronger predictor than fatigue. |
Favejee et al. 2015 [61] | 25 mildly affected Pompe disease patients (46 ∧range: 20–71) | L &I (12 weeks) | Assess the effectiveness of a 12-week exercise intervention in adults with Pompe disease | D: Actigraph GT3X accelerometer worn for at least 3 days | No changes in PA or motor function were reported. |
Table 3
Direct HPA-tools report and methodology. This table presents all papers identified using direct HPA-tools and display the methodological variables reported. Y: information has been reported
Study | Monitor Characteristics | Data Collection | Data Management | Data Analysis | Reference | |||||
Activity Monitor | Monitor | Period of Wear (days) | Number of | Valid Days | Additional | Definition of | Missing Data | Summary | For | |
(model specific) | Placement | wd: weekday | Hours/Day | Included For | Tool For | Non-Wear | Methodlogy | Endpoints | Methods | |
we: weekend | Analysis | Quality | Episode | Analysed | ||||||
McCrory [36] | Polar HR monitor (Vantage XL) | – | 3 (2 wd + 1 we) | Awake time | – | Y | Y | – | Y | Y |
Busse [64] | StepWatch step activity monitor (SAM) (Cymatech) | Right ankle | 7 | 24 hours period | 7 | Y | Y | – | Y | Y |
Kilmer [57] | HR monitor (not specified)& Yamax pedometer (Digi-Walker) | – | 3 | Awake time | – | – | – | – | Y | Y |
Aitkens [9] | Polar HR monitor (Vantage XL) | – | 3 (2 wd + 1 we) | Awake time | 3 | Y | Y | – | Y | Y |
McDonald [37] | StepWatch activity monitor (SAM) (Cymatech) & Polar HR monitor (Vantage NV) | Right ankle | 3 (2 wd + 1 we) | Awake time | 3 | Y | – | Y | Y | Y |
Wiles [38] | StepWatch step activity monitor (SAM) (Cymatech) | Right ankle | 7 | 24 hours period | 7 | – | – | – | Y | Y |
Kalkman [62] | actometer (Actilog V3.0) | Ankle (side?) | 12 | – | 12 | – | – | – | Y | Y |
Philips [59] | Polar HR monitor | – | 3 | Awake time | 3 | – | – | Y | Y | Y |
Apabhai [58] | SenseWear Armband activity monitor (SWA) (Pro3) | Upper arm (side?) | 3 | – | – | – | – | – | Y | Y |
Elsworth [67] | StepWatch activity monitor (SAM) | – | 8 | – | – | – | – | Y | Y | Y |
Jeannet [56] | ASUR-Autonomous Sensing Unit Recorder | Chest (shirt) | 2 | Awake time | 2 | – | Y | Y | Y | Y |
Holtebekk [50] | SenseWear Armband activity monitor (SWA) (Pro3) | Upper | 4 (Sunday to | 24 hours | At least 2 c | – | – | Y | Y | Y |
right arm | Thursday) | 24 hours period | (with at least 11 hrs.) | |||||||
Voet [63] | actometer | Ankle (side?) | 12 | 24 hours period | 2 | – | – | – | Y | Y |
Shrader [52] | Actical accelerometer | – | 10 | – | At least 6 | – | – | Y | Y | Y |
Davidson [54] | StepWatch activity monitor (SAM) | Ankle (side?) | 5 | Awake time | At least 4 | Y | Y | Y | Y | Y |
Favejee [61] | ActiGraph accelerometer (GT3X) | Waist (side?) | – | Awake time | At least 3 | – | Y | Y | Y | Y |
(with at least 8 hrs.) |
Table 4
Indirect HPA-tools report and methodology. This table presents all papers identified using an indirect HPA-tool or patient-reported outcome (PRO) and display the methodological variables reported. Y: information has been reported
Paper | Tool name | Tool | Administration Protocol | Summary of | Published |
description | (e.g. respondent, | Endpoints | References | ||
in detail | location, method, etc.) | Analysed | |||
McCrory [36] | Physical Activity (PA) diary | Y | Y | Y | Y |
Ollivier [51] | Bouchard questionnaire | Y | Y | Y | Y |
Aitkens [9] | Activity records | Y | Y | Y | – |
Hawker [66] | Modified Baecke questionnaire | – | Y | Y | Y |
McDonald [37] | Activity-sleep diary | Y | Y | Y | – |
Kalkman [62] | Subscales of the Sickness Impact Profile (SIP) | – | Y | Y | Y |
Wintzen [53] | PA increment scoring | Y | Y | Y | Y |
Phillips [59] | PA diary | Y | Y | Y | Y |
Apabhai [58] | International PA Questionnaire (IPAQ) | – | – | Y | Y |
Elsworth [67] | PA Scale for Elderly (PASE) | Y | Y | Y | – |
Rosenberg [65] | Godin Leisure Time Exercise Questionnaire (GLTEQ) | Y | Y | – | Y |
Holtebekk [50] | PEACH project questionnaire | Y | – | Y | Y |
Voet [63] | Decreased PA subscale from the Checklist Individual Strength (CIS) | Y | Y | Y | Y |
Elliott [55] | Simplified version of Bouchard questionnaire | Y | Y | Y | Y |
Anens [60] | PA Disability Survey-Revised (PADS-R) | Y | Y | Y | Y |
Table 5
Direct tools description. Each of the direct tools identified in this review are presented in detail: tool description; available endpoints; validation background; strengths; and weaknessess
Tool Description (key references) | Available HPA endpoints to report | Identified validation studies (specific targeted population) | Strengths | Weakness |
EE: energy expenditure TEE: total EE REE: resting EE HR: heart rate PA: physical activity PAL: physical activity levels MET: metabolic equivalent unit | DMD: Duchenne Muscular Dystrophy COPD: chronic obstructive pulmonary disease | |||
Heart-Rate (HR) monitor – Flex-HR methodology Estimates each-individual’s daily energy expenditure (EE) [90]. It assumes that above a given threshold (the FLEX point) there is a linear relationship between HR and oxygen consumption; below this threshold the relationship is variable [91–93]. | “Flex” heart rate/ Sedentary time (mins/day <flex HR) and active time (mins/day >flex HR)/ estimation of TEE / estimation of PAL (TEE/REE) | [94, 95] (Cerebral Palsy) | Suitable for ambulant and non-ambulant participants [95]/Continuous monitoring of the heart rate (HR)/Evidence of psychometric properties on children and adults [91, 93] | Susceptible to data loss (i.e. Chest strap worn incorrectly or non-worn at all) [96]/Assumes a linear relationship between HR and EE/When not combined with a movement monitor or PA diary it only assess cardiovascular patterns that may not relate to participation in PA [27, 97] |
StepWatch Activity Monitor (SAM) Ankle-mounted two-dimensional accelerometer that translates captured data into strides, counts over time intervals and patterns. Comes with PC software to process the data. http://www.orthocareinnovations.com/ | Total steps/day/mean step count/peak activity index (highest mean step per 1 min intervals)/sustained activity (>60 mins)/Active time: 1) low (1–15 steps/min); 2) moderate (16–30 steps/min); and 3) high (>30 steps/min)/Inactive time (%) (zero step rate) | [98, 99] (Cerebral Palsy), [37] (DMD), [100, 101] (Cerebral Palsy), [102] (COPD) | Allows individual calibration for different gait patterns (including assistive devices) which has shown to be feasible and reliable in DMD [96]/can record data up to 2 months/Used on a Phase 2b drug trial in DMD [45]/Previously used in numerous motor impaired populations (i.e. Cerebral Palsy, Parkinson, Stroke and Polio) and strongly correlated to patients’ participation in Daily Life Activities (Cerebral Palsy) [15] | Not suitable for non-ambulant/The established intensity counts cut points (low (<15 steps/min), moderate (15–42 steps/min), and high (>42 steps/min)) may not be translated directly to all NMD patients |
SenseWear Pro3 Armband (SWA) Combination of sensors (triaxial accelerometer, galvanic skin temperature and heat flux) providing a multi-parameter report. Worn around the mid-upper arm. Works with online software to process the data. http://sensewear.bodymedia.com | Average METs/day/Total steps/day/Sedentary bouts/Transitions from sedentary to active/PA intensity when active: moderate (3–6 METs) and vigorous (6–9 METs)/Detection of non-wear timings | [103] (COPD), [104] (Cystic Fibrosis), [105, 106] (COPD), [107] (fibromyalgia) | Identifies physiological changes not associated with movement (i.e. HR and METs)/Gives an estimation of energy expenditure [108]/Has a wheelchair-specific equation [109] | Estimation validity is reduced at high-intensity activities [110]/Estimation validity is reduced for the paediatric population [111]/Reduced reliability in motor-impaired populations [112, 113] |
ActiGraph GT3X accelerometer Tri-directional accelerometer, typically wrist-worn. Data are summed over a user-defined sampling period (epoch) that can be converted into activity intensity using cut-points over a given time-interval. Works with specific software. http://actigraphcorp.com/ | Vector magnitude units (mean number of counts/day)/Estimation of PAL (EE) overall and per specific time period | [20, 114] (Cerebral Palsy) | Real time measure of physical activity duration, intensity and frequency/Good validation for sleep-patterns analysis/Feasible for non-ambulant [115] | Does not differentiate ambulation from other activities of similar intensities/The readouts and interpretations are not straightforward for clinical practice/For any meaningful PA outcome, the raw data need to be converted |
Actical accelerometer Tri-directional accelerometer, typically waist-worn. Measures acceleration giving results in both: [1] acceleration counts per time-slots (epochs) – as short as one second—and [2] step counts, simultaneously. Works with specific software. http://www.actigraphy.com/devices/actical/ | Total activity (activity counts/day)/counts/hour/steps count/identification of intervention periods (example: exercising time) | n/a | Dual function extensively validated for healthy adults and children [116–120]: Provides direct information (steps) straightaway and raw data (epochs) for analysis simultaneously/With the standard 1 hour training, devices and software package shown to be straightforward and easy to use [121]/Allows highlighting of time of intervention, subjects can press a button at a predefined event time (i.e. starting treatment or exercise regime)/Validated for wheelchair bound patients [109]/90% of MS patients score it as “very comfortable” to wear [121] | Psychometric barriers when analysed for motor-impaired diseases like Multiple Sclerosis (MS) [121]: when counts were plotted against HR and exertion scores, a weak relationship was shown, especially for activities of daily life such as reading, vacuuming and washing. |
Yamax pedometer Waist-mounted step counter. [163. 164, 165, 196] http://www.yamax.co.uk/ | Total steps/day | [122] (COPD) | Similar to other pedometers: simple, practical and cheap/10,000 steps activity guidelines are based on data collected with this device [123]/Real time display allows it to be used as a PA motivator device [124] | Similar to other pedometers: poor data collection and variables captured/Slow walking and obesity lead to undercounting of steps/Not valid for Energy Expenditure estimations/Significant underestimation noted in neurological patients [125]. |
Autonomous Sensing Unit Recorder (ASUR) Non-commercialized tri-dimensional accelerometer and gyroscope (55×40×18 mm, 50 g). It is fixed to subject’s t-shirt. [56] | Sitting and lying periods/walking and standing periods/walking episodes (at least 3 successive steps)/number of steps and step rate (similar to the StepWatch’s classification system) | [56] (DMD) [126] (Parkinson’s disease) | Long-term recording capability/allows assessment of different body postures (sitting, standing, lying and walking activity) with high specific and sensitiveness [56, 126, 127]/“epoch” length of ASUR is 25 ms long (vs 1 min-length of SAM) giving more opportunity to detect high step rate periods in DMD [56] | Needs overnight re-charging/proposed body location (t-shirt) might not always be feasible and the lack of ‘body attachment’ might be a risk for losing equipment. |
Table 6
Indirect tools description. Each of the indirect tools identified in this review are presented in detail: tool description; available endpoints; validation background; and previous reports comparing it to a direct tool
Tool Description | Available HPA | Identified validation | Compared to Direct-Methods |
endpoints to report | studies | ||
PEACH Project Questionnaire previous week’s activity (24 questions adapted from the PAQ-C) [50, 128]. | Duration and level of exhaustion during activities. PA self-reported patterns and time for: 1) transportation to school; 2) school breaks activity; 3) organized PA in leisure time; and 4) weekend activities. | Children from 10 to 12 years old [77, 89, 128] | Kowalski et al. study – PAQ-C correlated to Caltrac single-plane accelerometer (r = 0.39) [77] Page et al. studies – Against accelerometer (Actigraph GT1M) and GPS [86, 89] |
Bouchard activity diary [55, 74]. Activity diary completed following provided instructions. 96 fifteen-minute interval blocks per day over three days. | Duration and level of exhaustion during activities. PA self-reported patterns and time for: 1) transportation to school; 2) school breaks activity; 3) organized PA in leisure time; and 4) weekend activities. Activities categorised into 9 levels based on average energy costs and respective metabolic energy equivalents (METs) | Children from 10 to 12 years old [77, 89, 128] Healthy adolescents [87] | Kowalski et al. study – PAQ-C correlated to Caltrac single-plane accelerometer (r = 0.39) [77] Page et al. studies – Against accelerometer (Actigraph GT1M) and GPS [86, 89] METs and Energy Expenditure calculated with Double Labelled Water testing [74–76] In Martinez-Gomez study – vs. ActiGraph accelerometer [87]. |
Activity logs/diaries Usually self-reported. Recalled time varies, typically 3 to 7 days. | Activity frequency (times/day, times/week, days/week)/Ratings of perceived exertion (i.e. Borg scale)/Activity mode and assigned intensity (METs) | Variety | METs and Energy Expenditure calculated with Double Labelled Water testing [74–76] Bonnefoy et al. found a significant strong correlation between DLW and moderate to high PA seven-day recall [88]. |
Physical Activity Scale for Elderly (PASE) [67, 68] Self-reported questionnaire of activities undertaken over the previous seven days. Scored 0–400, where the higher score indicates a greater level of PA. | PASE score total, and sub-scores: social, home and work (Mean and SD)/PA classified according time (<hour, 1-2 hours, 2–4 hours >4 hours), frequency (never, seldom, sometimes, often) and type (walking, exercise, household and occupational activities). | The Physical Activity Scale for Individuals with Physical Disabilities population [129] | PASE scores correlated with average 3-day accelerometer readings (r = 0.49, p < 0.05) in the total sample and in those over age 70 years (r = 0.64, p < 0.5) [80] |
International Physical Activity Questionnaire (IPAQ) [58, 130] Open access questionnaire that comprises of a set of 4 sections. Long and short versions available for use by either telephone or self-administered. Validated in 12 countries. http://www.ipaq.ki.se/ | Four domains of physical activity (work-related, transportation, housework/gardening and leisure-time) activity/frequency (days/week), type (moderate, vigorous, walking or sitting) and time (min and hours/day) | Healthy volunteers [81] Men with intellectual disability (included non-ambulant) [82] | From Hagstromer et al. The MTI activity monitor and IPAQ related for total PA and vigorous PA (rho = 0.55 and 0.71, P < 0.001) but a weaker relationship for moderate PA (rho = 0.21, P = 0.051) [81]. McKeon et al. obtained relationship when compared to SWA [83]. Apaphabi et al. showed low correlation values vs the SenseWear Armband in mitochondrial diseases [58] |
The EPIC-Norfolk Physical Activity Questionnaire-2 [59, 83] self-reported questionnaire that records activity in three domains – in and around the house, traveling and at work, and recreational – for the first two years. | Self-reported time (hours/week) spent in different activities (e.g. cooking, shopping for food, housework, walking, DIY, swimming, etc.). | Healthy volunteers [83] | Wareham et al. study – a 4 day estimation using a heart-rate monitor [83] |
Physical Activity Disability Survey-Revised (PADS-R) [60, 121] Scores PAL during the previous week. Includes 6 subscales: exercise; leisure-time; general activity; therapy; employment; and wheelchair use. The higher the PADS-R score, the higher the PAL. | Differences between low and high physical activities. Low PAL (score <mean) and High PAL (score >mean) and self-reported time spent at each subscale | Test retest reliability study in people with chronic neurological conditions [121] | Kayes et al. in 2007 – Reported that (Actical) accelerometer activity counts were not accurately predicted by standardized PADS scores (wide 95% prediction intervals) in people with multiple sclerosis [84]. |
Godin Leisure Time Exercise Questionnaire (GLTEQ) [131, 132] Assesses the number of times per week an individual performs an activity for more than 15 minutes during free time. *Rosenberg et al. adapted it for wheelchair or assistive device users. | Weekly time, frequency and a total score for leisure activity (strenuous, moderate and light activities by their respective METs of 9, 5 and 3). | Validity of PA measures in MS patients [85] Recognized use in oncology [133] | Motl et al. study – Correlations between GLTEQ and pedometer and ActiGraph-accelerometer were mod-strong [85] |
Modification of Baecke Physical Activity Questionnaire. [66, 134] – Self-reported HPA of the last year, summing up household, sports and leisure activity scores. | Activity distance walked per week/activity frequency (never, sometimes, mostly, always, months/year, stairs/day)/activity type (walking, leisure, household, sports) time and intensity/% of non-ambulatory | Validity and Reliability testing [134] | Bonnefoy et al. compared it against DLW and maximal oxygen and found non-significant mild correlation in older men [88]. The full version against DLW and (Tracmor) accelerometer showed a high correlation level with PAL (r = 0.69, p < 0.001) [78, 79] |
The checklist individual strength questionnaire (CIS) – Reduced activity score. 20-item PRO created for chronic fatigue assessment. Reduction in activity is one of the four assessed dimensions composed by three items scored from 1 to 7 each [135]. | Patient reported PAL. The higher the score the more sedentary the patient reports to be. | Chronic-fatigued and multiple sclerosis [136] Rheumatoid arthritis [137] | According to van der Werf et al., patients with a low activity level were defined objectively as those with a PAL lower than the group average [138]. |