Analysis of learning behaviour in immersive virtual reality
Abstract
Immersive virtual reality technology has been widely used in teaching and learning scenarios because of its unique visual and interactive experiences that bring learners a sense of immersive reality. However, how to better apply immersive virtual reality technology to learning environments to promote learning effectiveness is a direction that has been studied and explored by many scholars. Although a growing number of studies have concluded that immersive virtual reality technology can enhance learners’ attention in teaching and learning, few studies have directly linked both learning behaviors and attention to investigate the differences in behavioral performance across attention. In this study, attention data monitored by EEG physiological brainwaves and a large number of videos recorded during learning were used to explore the differences in the sequence of high attention behaviors across performance levels in an immersive virtual reality environment using behavioral data mining techniques. The results found that there was a strong correlation between attention and performance in immersive virtual reality, that thinking and looking may be more conducive to learners’ concentration, and that high concentration behaviors in the high-performing group accompanied the test and appeared after the monitoring, while the action continued to be repeated after the high concentration behaviors in the low-performing group. Based on this, this study provides a reference method for the analysis of the learning process in this environment, and provides a theoretical basis and practical guidance for the improvement of participants’ attention and learning effectiveness.
1Introduction
Immersive virtual technology (IVR) uses head-mounted displays (HMDs) to provide a sense of reality reproduction for the participants’ senses of sight, hearing, touch and smell [1], and is widely used in teaching and learning scenarios because it brings the user an immersive virtual world [2]. A growing body of research is proving the benefits of this technology in the educational field by increasing the attention span of learners and actively engaging them in instructional situations [3, 4]. Sustained attention of participants in the learning process is one of the important factors affecting academic performance [5, 6]. It has also been shown that students who maintain good attention while completing assigned tasks during the learning process will help them to better recall what they have learned in class [7, 8]. This may be because maintaining good attention helps the learner’s brain to process and encode information to a certain extent, leading to better performance in learning [9].
Although learners are more interested and motivated in learning content when immersed in a virtual environment [10, 11], few studies have explored the differences in behavioral performance across attention in an immersive virtual reality environment. If learning behaviors and attention can be combined to explore the differences in high attentional behaviors across performance levels, it can provide an effective reference method for the analysis of the learning process in an immersive virtual reality environment and also provide a reliable guide for the improvement of learning effectiveness in this environment.
Currently, the most common method for exploring learners’ behavioral performance in immersive virtual reality environments is behavioral sequence analysis, which is an in-depth analysis of learners’ behavior during the learning process through data mining techniques to explain the “why” and “how” of the virtual environment. “promote better learning for learners [12, 13]. IVR due to its specific application environment, has led to new breakthroughs in research related to social sciences, allowing for the extraction of more accurate and important information [14]. An analysis of the literature reveals a growing number of recent studies mining relevant learning data to explore the learning effects of participants in immersive learning environments [15, 16].
However, few studies have explored the learning process based on a direct link between learners’ attention and behavioural performance. To bridge this gap, this study proposes a two-tier research method based on EEG biosignals combined with video-recorded behavioural data to explore whether there are different patterns of transitions between learners’ high-focus behaviours in immersive virtual reality. Firstly, brainwave equipment was used to measure and record learners’ high and low concentration time points during the activity during the experiment; secondly, learners’ behavioural characteristics and verbal content during the experiment were analysed in the form of video recordings and qualitative methods, thus forming a behavioural coding framework and coding the video-recorded behavioural data with a fixed time span; finally, the concentration time points recorded during the experiment were combined with action data were combined to sequence highly focused behaviours between different performance levels. In order to clearly describe the purpose of this study, the following questions are listed for exploration:
1. The relevance of participants’ attention to performance in an immersive virtual reality environment?
2. Explore what behaviours are more conducive to attention in immersive virtual reality based on EEG feedback?
3. Explore whether high and low performing groups have different sequential patterns of high-focus behaviour?
The remaining sections are organised as follows. Section 2 presents related work. Section 3 describes the experimental design and methodology, including participants, experimental setting, research tool and a two-tier study method based on EEG biosignals combined with video-recorded behavioural data. Section 4 presents the experimental results and data analysis. Section 5 discusses the experimental results and also analyses the limitations of this study, opening up ideas for future research. Section 6 presents the conclusions and possible future implications or applications of this paper.
2Related work
2.1EEG feedback-based attention monitoring in learning
Since attention helps the information processing and encoding process of learners’ brains to some extent, thus facilitating their better performance in the learning process, more and more scholars are concerned with attention monitoring during learning. There are three methods for monitoring attention: the first is learner self-evaluation, which is reported using relevant scales; the second is non-physiological signals, such as tracking the learner’s head posture; and the third is physiological signals, such as measurements of physiological signals by EEG sensor devices, heart rate and blood oxygen sensors, and other devices that monitor attention. Among the above three measurements, the most effective is EEG-based attention monitoring [17].
Most of the current studies have applied EEG data from EEG sensor devices to monitor learners’ attention levels during learning, for example, Ko et al. [18] measured students’ EEG activity in real classrooms by EEG technology and analyzed the neural activity associated with sustained attention. The results showed that students showed different levels of sustained attention in different subjects and tasks, and also that noise and distractions in the classroom environment could affect students’ sustained attention. Abeer et al. [19] explored the use of EEG data to predict students’ attention in online learning. It describes the use of EEG data to collect students’ brain activity and the use of machine learning algorithms to analyze this data to predict students’ attention levels. This study shows that the use of EEG data can effectively predict students’ attention, thus helping educators to better understand students’ learning status and provide better teaching strategies. Victor et al. [20] used a machine learning-based approach in a virtual reality learning environment to help students maintain their attention and focus by responding to different attention levels through EEG signals and providing appropriate feedback and suggestions when students’ attention decreases.
An analysis of the relevant literature reveals that most studies have focused on how to use EEG techniques to respond to learners’ attention data and to improve and provide feedback in conjunction with factors influencing learning effectiveness and individual differences to promote optimal learning outcomes. However, few studies have directly linked both learning behaviors and attention to explore differences in behavioral performance across attention.
2.2Analysis of learning behavior in immersive virtual reality
With the advent of immersive virtual technologies, a number of studies have been considered to offer possibilities for better exploration of human behavior and psychosocial phenomena [21, 22]. In traditional social psychology research, laboratory or questionnaire surveys are mainly used, and these methods have certain limitations. The application of immersive virtual environment technology in social psychology research has many advantages. First, the technology can provide a more realistic and controllable research environment, which can better simulate the real social situation and make the research results more representative. Second, the technology can provide a more detailed way of data collection and analysis, which can record more behavioral and emotional data and help to study human behavior and social psychological phenomena in depth. Third, the technology can provide a more flexible way of research, which can be customized according to different research purposes and needs.
As a result, more and more researchers are exploring learners’ behaviors in immersive virtual reality teaching environments. For example, Li et al. [23] applied learning behavior analysis to an English education classroom with virtual reality technology using an experimental research method, during which data on students’ behavioral interactions were collected through video recordings to explore the effects of virtual reality technology in an English education classroom. Hasenbein et al. [24] used an eye-tracking device to collect virtual reality classroom learners’ gaze behaviors and also collected data on students’ learning experiences to explore the effects of different socially relevant configurations, such as simulated virtual classmates and virtual teachers, on students’ visual attention in a virtual reality classroom. Cheng et al. [25] used video recording and observation logging to collect data on teacher-student interaction behaviors to explore the effects of using immersive virtual reality for field trips in elementary classrooms on students’ learning experiences and teacher-student interaction behaviors. Elliot et al. [26] used experimental research methods to record students’ learning behavior data in realistic and virtualized labs using video recording, eye-tracking, EEG, and heart rate monitors, respectively, and analyzed the collected behavioral data to compare the differences in students’ learning and behavior in realistic and virtualized labs. Shin [27] used a variety of biometric instruments to record students’ behavioral data and analyzed the data to explore the impact of usability on the learning experience in virtual reality learning. Yang et al. [28] used video recordings, eye-tracking devices, and questionnaires to record students’ behavioral data and analyzed the data through descriptive statistics, ANOVA and correlation analysis were conducted on the data to explore the application of virtual reality technology in promoting students’ writing performance and engagement in learning behaviors.
According to the relevant literature, three main methods were found to collect learner behavior data in virtual reality environments, including video recording, biometric instruments, and questionnaires. In this study, video recording was chosen to visualize the behavioral data of learners in virtual learning environments, and then further explored using behavioral analysis methods.
2.3Machine learning based behavioral sequence analysis method
In order to explore how to better facilitate learners’ learning in the learning process, many researchers have applied learning behavior analysis methods to the teaching process. There are five commonly used learning behavior analysis methods: descriptive statistical analysis, analysis of variance, correlation analysis, sequence analysis, and machine learning. Valiente et al. [29] pointed out that with the continuous development of learning analytics research, researchers have started to study learning prediction using machine learning methods and tools.
For example, Rivas et al. [30] used machine learning techniques to explore the relationship between students’ learning behaviors and academic performance and proposed a machine learning-based framework for analyzing student performance. Tong et al. [31] proposed a performance prediction assessment model for learning process behavior based on machine learning, and detailed the construction process and feature extraction method of the model. Yan et al. [32] also suggested in their study that applying machine learning to learning behavior analysis has the following advantages: efficiency: machine learning can quickly analyze and mine large amounts of online learning data; automation: machine learning can automatically analyze and process online learning data without human intervention; personalization: machine learning can provide students with personalized learning resources and learning paths for students; predictive: machine learning predicts and evaluates students’ learning behaviors and academic performance. This study uses machine learning-based behavioral sequence analysis to explore differences in behavioral patterns across performance levels in an immersive virtual reality environment.
Behavioural sequence analysis is the most com-monly used method in education to analyse various patterns of behavioural sequences, which examines the importance of sequential behaviours by coding participants’ learning behaviours [33]. Hou et al. [34] also noted that behavioural sequence analysis, which shows the transitions between each behaviour through statistical data and sequence relationship diagrams, is an effective way to use teachers in im-proving teaching strategies and tools. Sequence pattern algorithms were first proposed by Agrawal and Srikant to algorithmically identify subsequences in a set of sequences that occur at least as often as the minimum support value [35]. The commonly used sequence pattern mining methods in recent years are GSP, FreeSpan, SPADE and PrefixSpan, while PrefixSpan has the advantages of highest performance, fastest speed and smallest memory consumption compared with other methods, in addition, the method can also formulate rules to mine sequence patterns with user-specified conditions [36]. Therefore, this study uses a constraint-based sequence pattern mining approach to mine all possible sequence patterns based on highly focused behaviours by specifying constraints on highly focused behaviours to provide a restricted dataset for the mining process before performing the behavioural sequence mining task.
3Research design and methodology
3.1Participants and experimental environment
In this study, participants learned in the Fire Safety Lab, a fire safety education game developed by Oculus Rift and IMP Studios, with the aim of learning and acquiring fire safety skills through an immersive virtual reality environment. The game is divided into three scenarios: basic instruction, exploration scenarios and exam scenarios. Participants are required to complete a series of fire-fighting operations in the designated exploration scenarios, including wearing a fire mask and gloves, turning off the electricity, pressing the fire alarm, calling 119 and using a fire extinguisher, etc. The exploration time is set at 10 minutes per person.
Prior to the formal experiment, in order to reduce the gap between learners’ knowledge about fire fighting, participants were organised to take a fire fighting knowledge quiz, which consisted of 8 single choice questions and 4 judgement questions, designed to reduce the interference with the experiment. A final selection of 59 junior high school students took part in the experiment in the same environment and equipment. The participants were 30 males and 29 females and the participants were aged between 11 and 13 years old.
Each participant was required to wear an OculusRift virtual device and MindWave brainwave device connected to the same computer before the experiment, and the HD HD video device and the computer’s recording software were turned on at the start of the experiment to record the participants’ entire learning process.
3.2Research tool
The integrated EEG monitoring and immersive virtual reality system in this study was implemented by connecting the OculusRift virtual device and the MindWave brainwave device to a single computer (see Fig. 1). The reliability of the OculusRift virtual device has been confirmed in previous studies and is one of the leading devices for providing immersive virtual environments [37, 38]. The device consists of a head-mounted display, spatial sensors, and an interactive control handle that allows participants to interact in the virtual reality three-dimensional space provided by the device by moving through the real world.The MindWave brainwave device is a biosensor that captures participants’ states of concentration and relaxation through brainwave biosignals from the brain [39], and the device consists of The device consists of a sensor support arm attached to the forehead, an ear clip and a power controller. The sensor monitors signals from the human brain, but it also captures noise and electrical interference from the environment, while the ear clip acts as a basis and reference, filtering out such interference through the device chip [40]. The device transmits the monitored brain signals to the computer, which converts them algorithmically into focus and relaxation values, and then records the participants’ high and low focus points through a computer program set up in advance.
Fig. 1
Immersive virtual reality integration system for EEG monitoring.
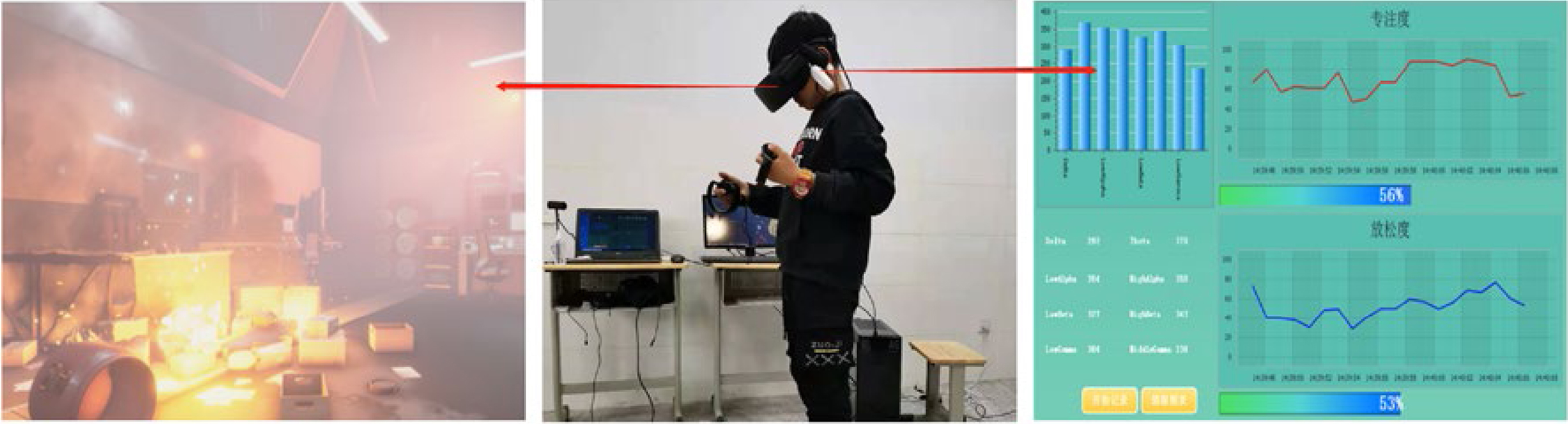
In previous studies, it was found that when the value of attention was between 40–60 it was usually considered normal; 60–80 was a higher concentration state; 80–100 was a high concentration state; similarly, 20–40 was a lower concentration state and 0–20 was a low concentration state [41, 42]. Therefore, before the formal experiment, we conducted a pre-experiment to determine the defined values of high and low concentration, and found that most participants’ concentration values fluctuated between 40-60, so this study finally set the defined values of high and low concentration to 60 and 40, and recorded the time point as a high concentration state when the participants’ concentration value was greater than 60 and the duration was greater than 2.5 seconds, and when the concentration value was less than 40 and When the concentration value was less than 40 and the duration was greater than 2.5 seconds, the time point was recorded as a low concentration state.
3.3Research methodology
This study proposes a two-tier research method based on EEG biosignals combined with video-recorded behavioural data (see Fig. 2).
Fig. 2
Immersive virtual reality integration system for EEG monitoring.
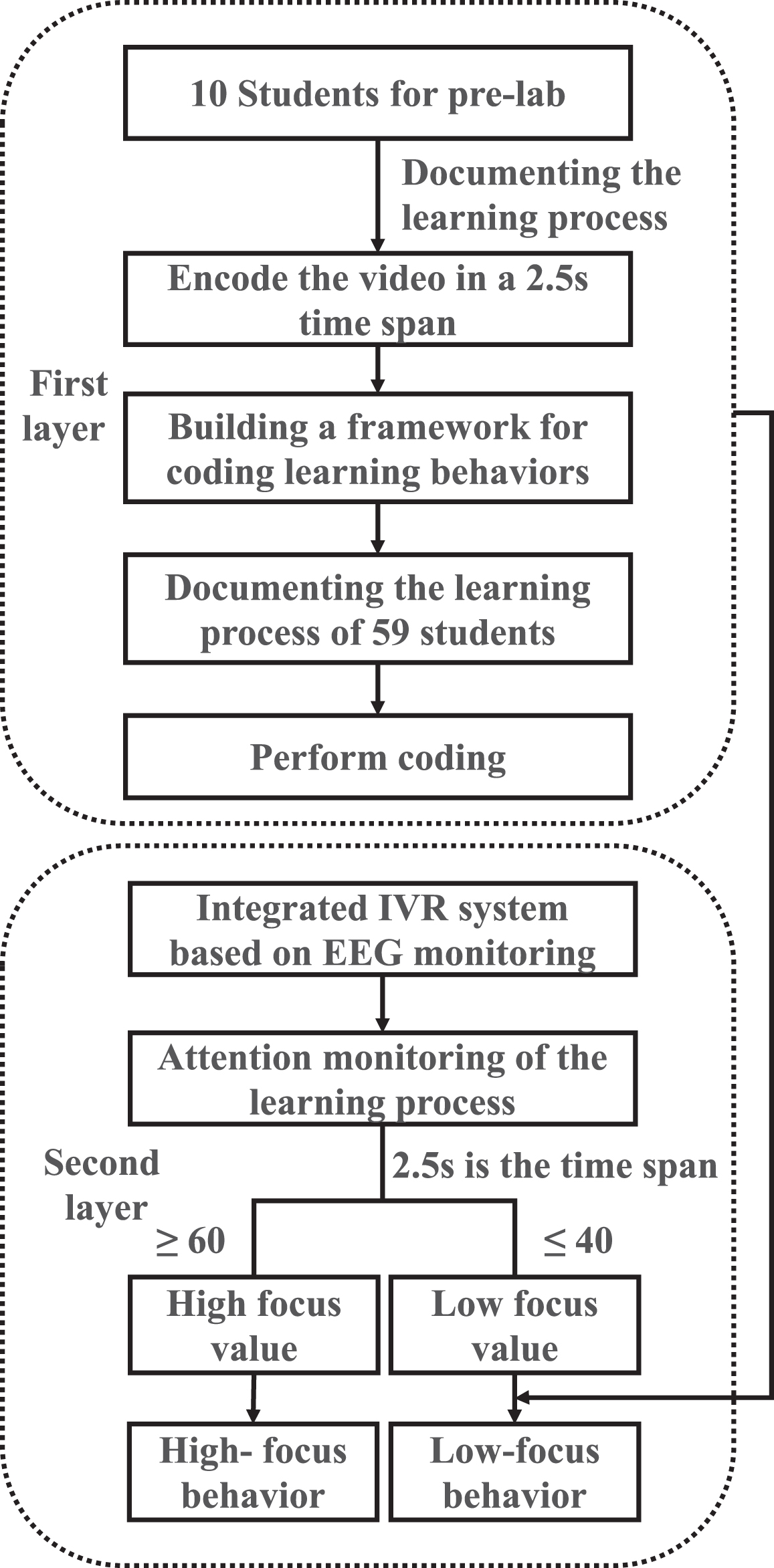
In the first tier, 10 students were selected for preliminary experiments, and by recording videos of their learning process, it was found that if the time span of each type of behavior was set to 5 S, some effective actions of the participants would be ignored, while if the behavior span was set to 1 S, it was difficult to observe and locate the action attributes of the participants in a short period of time, therefore, the time span of encoded behaviors was finally determined to be set to 2.5 S.
Subsequently, a learning behavior coding framework was constructed for the recorded videos based on the participants’ pre and post action intentions (consent was obtained from the participants before the experiment), and the coding scheme was designed to further explore the learning behaviors based on 2.5 S time intervals and then qualitative observations of these videos based on the behavioral attributes of the learners, including eight types: help seeking, monitoring, thinking, adjusting, testing, finding, and irrelevant operations (see Table 1).
Table 1
Behavioural codes
Coding | Category | Example |
SH | Seeking help | Ask how to grab items in the environment |
MO | Monitoring | Ask about the remaining learning tasks |
TH | Thinking | Stop and look around |
AD | Adjusting | Adjusting the position of the fire extinguisher |
TE | Testing | Press the fire extinguisher lever to extinguish the fire |
FI | Finding | Find fire alarms, phones, etc. |
IO | Irrelevant operation | Grabbing cups, chairs, etc. in the environment |
EX | Exploration | Explore the use of fire extinguishers, fire masks etc. |
In the second layer, firstly, the immersive virtual reality integration system monitored by EEG classifies the participants’ states into high and low concentration points, and then combines the behavior classification in the first layer to define the learners’ behaviors as high or low concentration behaviors. Finally, the sequence patterns of high-focus behaviors are explored based on the behavioral sequence mining of machine learning.
4Experimental results
4.1Participants’ attention and performance correlations in an immersive virtual reality environment?
To further investigate the correlation between participants’ concentration and performance in the immersive virtual reality environment, the final scores of the game and the mean values of concentration obtained from EEG monitoring of 59 participants were subjected to Pearson correlation analysis through SPSS software, and the results are shown in Table 2. As can be seen from Table 2, the correlation coefficient between students’ final scores and attention was 0.525 and the p-value was 0 < 0.01, thus it can be concluded that students’ concentration was positively correlated with performance and was statistically significant.
Table 2
Attention and performance correlation
Score | Attention | ||
Score | Pearson Correlation | 1 | 0.725** |
Sig.(bobtail) | 0.000 | ||
Number of cases | 59 | 59 | |
Attention | Pearson Correlation | 0.725** | 1 |
Sig.(bobtail) | 0.000 | ||
Number of cases | 59 | 59 |
4.2Exploring what behaviours are more conducive to concentration in immersive virtual reality?
To further analyse the correlation between each behaviour and concentration, the correlation coefficient between each behaviour and attention was calculated by Spearman’s correlation analysis and the statistical results are shown in Table 3. It was found that TH, FI and AD were correlated with attention and the correlation was significant (p < 0.05).
Table 3
Correlation of each behaviour with attention
SH | MO | TH | AD | ||
Attention | Pearson Correlation | 0.094 | – 0.039 | .533** | .406** |
Sig.(bobtail) | 0.480 | 0.772 | 0.000 | 0.041 | |
TE | FI | IO | EX | ||
Attention | Pearson Correlation | 0.057 | .446** | – 0.124 | – 0.015 |
Sig.(bobtail) | 0.668 | 0.000 | 0.349 | 0.912 |
According to the experiment, each experimenter’s learning time was 10 minutes, and the monitoring and coding of concentration and learning behaviours were carried out in a time span of 2.5 s. 59 participants performed a total of 14,160 monitoring events, and then the concentration points with attention values equal to and greater than 60 or more in every 2.5 s were recorded through the integrated system, resulting in 5,476 high concentration behaviours, with a statistical distribution of specific behavioural frequencies See Fig. 3. As can be seen from the table, the frequency of high concentration behaviours were AD (546), EX (244), FI (1088), IO (154), MO (206), SH (202), TE (758) and TH (2278), while the total frequency of each behaviour during the experiment was AD (2218), EX (962), FI (2396), IO (1166), MO (535), SH (894), TE (1840), TH (4149), and the frequency of each type of high-focus behaviour as a percentage of the total frequency of occurrence of the behaviour was AD (24.62%), EX (25.36%), FI (45.41%), IO (13.21%), MO (38.50%), SH (22.60%), TE (41.20%), TH (54.90%).
Fig. 3
Frequency of highly focused behavioural.
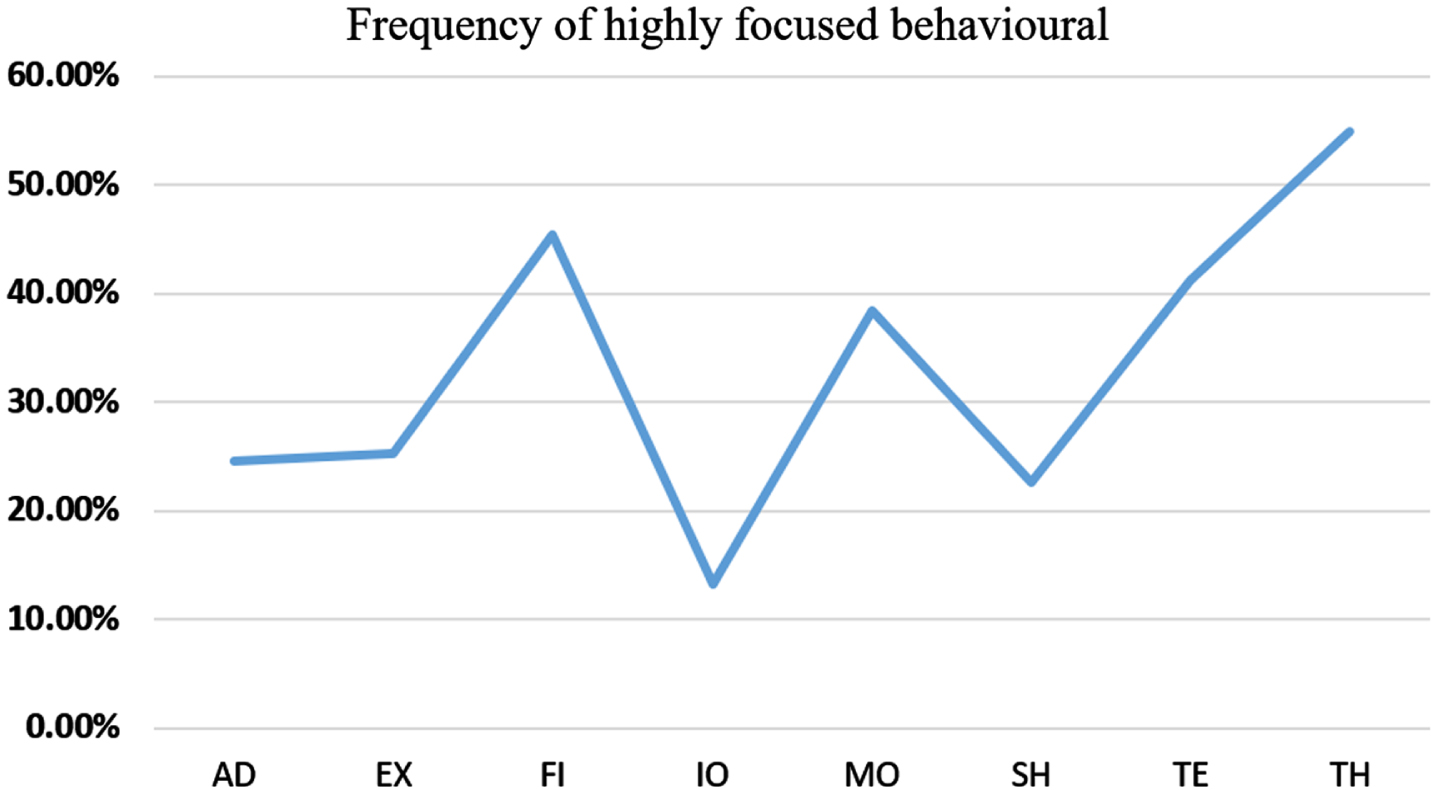
4.3Explore whether high and low performing groups have different sequential patterns of high-focus be-haviour
The test scenario of the immersive virtual fire safety education system automatically scores participants according to how well they complete the tasks. 50 points are awarded for each of the five tasks of wearing a fire mask and gloves, turning off the electricity, pressing the fire alarm and calling 119, and 500 points are awarded for choosing the correct fire extinguisher and successfully extinguishing the fire at the same time, for a total of 750 points. The scores were converted into percentages based on the participants’ test scores, and participants with a score of 65.11 and above were defined as the high performance group, while those with a score of 65.11 and below were defined as the low performance group, which resulted in 23 participants in the high performance group and 36 participants in the low performance group.
Table 4
Highly focused behavioural sequences
Sequence | Support | |
High Performance Group | TH-TE | 0.96 |
MO-FI | 0.91 | |
FI-TE | 0.86 | |
TH-AD | 0.86 | |
MO-TH-EX | 0.81 | |
Low Performance Group | TH-TH | 0.99 |
TH-TH-FI | 0.97 | |
FI-FI | 0.94 | |
AaD-FI | 0.94 | |
FI-FI-TE | 0.82 |
In order to explore whether there are different highly focused behavioural sequence model in the high and low performing groups, before data mining, the mining conditions were formulated, the high-focused behaviours TH and FI were set as constraint rules, and all relevant sequence patterns involving both TH and FI were mined, and the final results are shown in the table (sequence patterns with support below 0.8 have been removed).
The table presents the pattern of sequences of high-focused behaviours for the high and low performance groups. 5 sub-series are presented for the high performance group, including the sequence (TH) – (TE), which has a likelihood of occurrence and support of 0.96; the sequence (MO) – (FI), which has support of 0.91; the sequence (FI) – (TE), which has support of 0.86; the sequence (TH) – (AD), which has support of 0.86; sequence (MO) – (TH) – (EX), with support of 0.81. There were five sub-series in the low performance group, including sequence (TH) – (TH), which had a probability of occurrence and support of 0.99; sequence (TH) – (TH) – (FI), with support of 0.97; sequence (FI) – (FI), with support of 0.94; sequence (AD) – (FI) with a support of 0.94; sequence (FI) – (FI) – (TE) with a support of 0.82.
5Discussion
5.1Empirical contributions
5.1.1The task setting of the virtual educational environment needs to be improved
Table 2 shows the correlation between learners’ attention and learning performance in an immersive virtual reality environment. The results in the table indicate that there is a high correlation between attention and learning performance, and learners who maintain their attention will learn better in an immersive virtual reality. This result is consistent with recent research on the correlation between attention and learning, where learners can improve their learning performance if they focus their attention, regardless of whether they are highly motivated to learn or not [43, 44].
At the same time, a growing number of studies have demonstrated that learners’ attention plays an important role in contextual memory and the perception of scenes [45, 46]. For example, Wolfe’s (2007) study noted the effect of attentional load on scene perception and memory, demonstrating that participants performing dual tasks in the same scenes produced greater interference than single tasks [46], and that attention was well directed to process, encode and store information when learners were performing a single task [47]. In this study, however, due to the special environmental configuration and the inconvenience of wearing the equipment, learners were required to enter the learning environment with five tasks prior to formal learning, so the nature and number of tasks and scenario objects throughout the learning process may have an impact on the retention of learners’ attention, and future research is necessary to further enhance students’ attention in terms of scenario setting.
5.1.2Attention to highly focused behavior needs to be strengthened
James (1890) defined attention as the process of selecting the neural representations most relevant to the goal of the current behaviour [48]. When researchers have measured attention scientifically, they have considered how attention manifests itself through an observable organism, that is, how participants behave during that process and the degree of attention [49]. A growing number of studies have focused on the cognitive processes in the brain that target learners’ attention during learning, while fewer studies have addressed the direct relationship between learners’ attention and behavioural performance.
In this study, we calculated the correlation between each behaviour and attention through correlation analysis, and found that three types of behavioural activities, TH, FI and AD, were significantly correlated with attention. Subsequently, we counted each behaviour at high concentration points and calculated the percentage of total frequency of occurrence of high concentration points for that behaviour, and found that TH and FI had the highest frequency of occurrence at high concentration points of 54.90% and 45.41%, respectively, and the results consistent with the correlation analysis, while AD appeared high-focus only 33.63% of the time. We speculate that the correlation may be significant due to the higher frequency of AD in high-focus, but counting the total frequency revealed a larger base of AD, so we excluded the behaviour AD, while TH and FI in immersive virtual reality may be more conducive to learners’ attention.
5.1.3System setup and faculty supervision could further follow behavioral patterns
Using highly focused behaviour as a constraint for sequential pattern mining, we found that the high-performing group had a timely testing to validate their ideas after thinking or finding compared to the low-performing group, and some studies have also shown that in a game-based learning environment, learners using appropriate hypothesis validation strategies will accomplish more learning objectives while having better learning outcomes [50]. Students in the low performing group chose to repeat the action after thinking and looking, which may indicate that most of the thinking and finding by low performing students were ineffective actions as they were in a state of repetitive thinking and finding, thus causing the task progress to drag or stall. This is most likely due to the fact that virtual learning environments or exploratory learning styles are relatively new to them, so it is difficult to achieve a state of deep learning.
Learning environments should provide metacognitive support for learners to acquire and understand the knowledge required in the domain, thus helping learners to develop goal-setting, planning and problem-solving skills [51, 52]. In addition, we found that monitoring was present in the previous action of both highly focused behaviours (thinking and finding) in the highly focused group, which strongly suggests that the presence of the action of monitoring is highly likely to trigger an effective high-concentration action, and that by performing the action of regulatory moderation, learners critically reflect on their progress during learning while adopting appropriate strategies for continued advancement [53].
Comparing the sequence of high-focused behaviors between the high and low performing groups, both showed adjustment behaviors, while the adjustment in the high-performing group appeared after reflection and the adjustment in the low-performing group triggered a finding activity, suggesting that students in the high-performing group were used to adjust their problem-solving strategies after reflection. Kiili also noted in her study that reflection can help learners continuously adjust their strategies in educational games to promote effective learning [54].
The study also found that the emergence of exploratory behavior in the high-performing group was appropriately timed, with students engaging in timely exploratory activities (MO-TH-EX) immediately after the emergence of monitoring behaviors that triggered reflection. This may be explained by the fact that high-performing students have their own problem-solving strategies and come to a new environment where learners explore the environment by accessing various tools, and the exploration phase is the first stage of problem solving, a finding that has been confirmed by studies [55, 56]. Therefore, if learners perform adjustment or exploration actions after thinking activities, they are likely to be in an effective high concentration state and have a strong correlation with learning outcomes.
5.2Practical implications
This study uses EEG physiological brainwave data combined with behavioural sequences from a large number of videos recorded during learning to explore sequences of highly focused behaviour across different levels of performance in an immersive virtual reality environment. The innovative method of identifying highly focused behaviour and the large amount of video encoded data help to analyse learners’ concentration states in immersive virtual reality environments, identify learners’ highly focused behaviour and explore differences in patterns of highly focused behaviour across performance. These analyses can provide a reliable basis and important reference for enhancing the effectiveness of immersive virtual technology in education, the systematic development of such educational games and learners’ self-regulation processes.
Firstly, as learner attention plays a very important role in immersive virtual reality environments, the design of virtual reality-based teaching and learning environments should improve learner attention by breaking down multiple target tasks and reminders to enhance learning outcomes.
Secondly, for the identification of highly focused behaviour, this study proposes a two-layer research method based on EEG brainwave data combined with video behavioural sequencing. This method achieves a direct link between attention and behavioural performance, explores learners’ behavioural performance at highly focused points in the learning process, and promotes the richness of attention-based learning process research. There are studies that have addressed sequential patterns of learning behaviour in immersive virtual reality environments [13, 57], however, such studies rarely address learner attention data and behavioural patterns of highly focused behaviour, and this study combines attention and behavioural data for further analysis, providing innovative reference value for future related research.
Finally, in terms of system setup, in order to facilitate the effectiveness of immersive virtual reality in future teaching and learning, this study recommends that 1) consideration be given to providing learners with adequate metacognitive support prior to formal learning to help them use problem-solving strategies in that environment. For example, before entering the formal environment for the first time, a training session could be set up to provide learners with a similar learning environment to familiarise them with the environment and learning styles while developing their metacognitive strategies; 2) the environment should have sessions to remind learners to self-monitor and reflect, which will help them to monitor or adjust their learning progress or stage results and enable them to adopt strategies to correct them in time 3) the teacher plays the role of a facilitator in this environment, intervening in a timely manner when learners are at a thinking standstill or repeating an action to guide them to be in an effective high concentration action.
However, this work has limitations; first, this study lacks consideration of ethical and moral issues in virtual reality environments, which may be influenced by mental, social, and learning habits, thus affecting the experimental results; second, related to the time and effort of the study, only a small number of subject experimenters could be selected, resulting in experimental results prone to generalization and generalization; third, this study does not address the specific content of virtual reality learning environment. The above-mentioned limitations can be used as a development direction for future research, which can take into account the influence of issues such as learners’ emotional development, learning habits, social behavior, game duration and game adaptability.
6Conclusion
In this study, we explored sequences of highly focused behaviours at different levels of performance in an immersive virtual reality environment using EEG physiological brainwave data combined with behavioural sequences from a large number of videos recorded during the learning process. Unlike previous studies, we focus on the IVR learning environment and highlight the impact of learners’ attention and behaviour on learning outcomes from the perspective of learners’ attention and behaviour, exploring the differences in behavioural performance across attention and thus analysing the differences in high-focus behavioural performance of learners at different performance levels. Thus, the innovative method of identifying high attentional behaviours and the way in which patterns of high attentional behaviours between different performances are uncovered are the contributions of this study.
Learners, educators and designers can benefit from this study. Firstly, the study analyses the differences in high-focus behaviour patterns across performance levels, and these results can provide theoretical and practical support for learner self-monitoring and educator process guidance during the learning process; secondly, this study analyses the differences in learners’ attention and behaviour from the perspective of scenario setting, and therefore the results of the study can be useful for future development and design of IVR educational games have greater application value; third, this study proposes a method for identifying and mining high concentration behaviours in IVR environments, and the results will help future scholars in their further exploration on this basis.
Acknowledgments
The work in this paper was financially supported by the project: Sichuan Institute of Arts and Sciences Research Start-up Fund (2022QD063), and Educational Reform Project of Sichuan Institute of Arts and Sciences (2020JZ039), Sichuan Revolutionary Old Area Development Research Center Project (SLQ2017B-18), Innovation and Entrepreneurship Training Program for Students of Sichuan Institute of Arts and Sciences(202310644023).
References
[1] | Birenboim A. , Dijst M. , Ettema D. et al., The utilization of immersive virtual environments for the investigation of environmental preferences[J], Landscape and Urban Planning 189: ((2019) ), 129–138. |
[2] | Cheng K.-H. and Tsai C.-C. , A case study of immersive virtual field trips in an elementary classroom: Students’ learning experience and teacher-student interaction behaviors[J], Computers & Education 140: ((2019) ), 103600. |
[3] | Radianti J. , Majchrzak T.A. , Fromm J. et al., A systematic review of immersive virtual reality applications for higher education: Design elements, lessons learned, and research agenda[J], Computers & Education 147: ((2020) ), 103778. |
[4] | Christopoulos A. , Conrad M. and Shukla M. , Increasing student engagement through virtual interactions: How?[J], Virtual Reality 22: (4) ((2018) ), 353–369. |
[5] | Wilson K. and Korn J.H. , Attention during lectures: Beyond ten minutes, Teaching of Psychology 34: (2) ((2007) ), 85–89. |
[6] | Risko E.F. , Buchanan D. , Medimorec S. et al., Everyday attention: Mind wandering and computer use during lectures[J], Computers & Education 68: ((2013) ), 275–283. |
[7] | Dixon P. and Bortolussi M. , Construction, integration, and mind wandering in reading[J], Experimental Psychology/Revue canadienne de psychologie expérimentale, US: Educational Publishing Foundation 67: (1) ((2013) ), 1–10. |
[8] | Smallwood J. , McSpadden M. and Schooler J.W. , When attention matters: The curious incident of the wandering mind, Memory & Cognition 36: (6) ((2008) ), 1144–1150. |
[9] | Smithson E.F. , Phillips R. , Harvey D.W. and Morrall M.C. , The use of stimulant medication to improve neurocognitive and learning outcomes in children diagnosed with brain tumours: A systematic review[J], European Journal of Cancer 49: (14) ((2013) ), 3029–3040. |
[10] | Lau K.W. and Lee P.Y. , The use of virtual reality for creating unusual environmental stimulation to motivate students to explore creative ideas[J], Interactive Learning Environments, Routledge 23: (1) ((2015) ), 3–18. |
[11] | Alhalabi W. , Virtual reality systems enhance students’ achievements in engineering education[J], Behaviour & Information Technology, Taylor & Francis 35: (11) ((2016) ), 919–925. |
[12] | Cheng K.-H. and Tsai C.-C. , Children and parents’ reading of an augmented reality picture book: Analyses of behavioral patterns and cognitive attainment[J], Computers & Education 72: ((2014) ), 302–312. |
[13] | Hou H.-T. , Integrating cluster and sequential analysis to explore learners’ flow and behavioral patterns in a simulation game with situated-learning context for science courses: A video-based process exploration[J], Computers in Human Behavior 48: ((2015) ), 424–435. |
[14] | Parsons T.D. , Gaggioli A. and Riva G. , Virtual Reality for Research in Social Neuroscience[J], Brain Sciences, Multidisciplinary Digital Publishing Institute 7: (4) ((2017) ), 42. |
[15] | Abdullah M.E. , Masek A. , Shamshuddin M.K. et al., A Comparative Study on the Effect of Virtual Field Trips (VFTs) Through Video Aided Learning (VAL) and Traditional Learning Approaches on Students Knowledge Acquisition[J], Advanced Science Letters 22: (12) ((2016) ), 4036–4039. |
[16] | Kobayashi K.D. , Using Flipped Classroom and Virtual Field Trips to Engage Students[J], HortTechnology, American Society for Horticultural Science 27: (4) ((2017) ), 458–460. |
[17] | Chen C.-M. , Wang J.-Y. and Yu C.-M. , Assessing the attention levels of students by using a novel attention aware system based on brainwave signals[J], British Journal of Educational Technology 48: (2) ((2017) ), 348–369. |
[18] | Ko L.-W. , Komarov O. , Hairston W.D. et al., Sustained Attention in Real Classroom Settings: An EEG Study[J], Frontiers in Human Neuroscience (2017) , 11. |
[19] | Al-Nafjan A. and Aldayel M. , Predict Students’ Attention in Online Learning Using EEG Data[J], Sustainability, Multidisciplinary Digital Publishing Institute 14: (11) ((2022) ), 6553. |
[20] | Delvigne V. , Wannous H. , Vandeborre J.-P. et al., Attention Estimation in Virtual Reality with EEG based Image Regression[A], 2020 IEEE International Conference on Artificial Intelligence and Virtual Reality (AIVR)[C], (2020) :10–16. |
[21] | Blascovich J. , Loomis J. , Beall A.C. , 等. TARGET ARTICLE: Immersive Virtual Environment Technology as a Methodological Tool for Social Psychology[J], Psychological Inquiry, Routledge 13: (2) ((2002) ), 103–124. |
[22] | Slater M. , Immersion and the illusion of presence in virtual reality[J], British Journal of Psychology (London, England: 19853), 109: (3) ((2018) ), 431–433. |
[23] | Li M. and Madina Z. , Analysis of English Education Classroom Student Interaction and Performance Based on Virtual Reality[J], Security and Communication Networks, Hindawi 2022: ((2022) ), e9042553. |
[24] | Hasenbein L. , Stark P. , Trautwein U. et al., Learning with simulated virtual classmates: Effects of social-related configurations on students’ visual attention and learning experiences in an immersive virtual reality classroom[J], Computers in Human Behavior 133: ((2022) ), 107282. |
[25] | Cheng K.-H. and Tsai C.-C. , A case study of immersive virtual field trips in an elementary classroom: Students’ learning experience and teacher-student interaction behaviors[J], Computers & Education 140: ((2019) ), 103600. |
[26] | Hu-Au E. and Okita S. , Exploring Differences in Student Learning and Behavior Between Real-life and Virtual Reality Chemistry Laboratories[J], Journal of Science Education and Technology 30: (6) ((2021) ), 862–876. |
[27] | Shin D.-H. , The role of affordance in the experience of virtual reality learning: Technological and affective affordances in virtual reality[J], Telematics and Informatics 34: (8) ((2017) ), 1826–1836. |
[28] | Yang G. , Chen Y.-T. , Zheng X.-L. et al., From experiencing to expressing: A virtual reality approach to facilitating pupils’ descriptive paper writing performance and learning behavior engagement[J], British Journal of Educational Technology 52: (2) ((2021) ), 807–823. |
[29] | Ruipérez-Valiente J.A. , Muñoz-Merino P.J. , Leony D. , et al., ALAS-KA: A learning analytics extension for better understanding the learning process in the Khan Academy platform[J], Computers in Human Behavior 47: ((2015) ), 139–148. |
[30] | Uden L. , Liberona D. , Sanchez G. et al., Learning Technology for Education Challenges[C], Cham: Springer International Publishing, (2019) :428–438. |
[31] | Tong Y. and Zhan Z. , An evaluation model based on procedural behaviors for predicting MOOC learning performance: students’ online learning behavior analytics and algorithms construction[J], Interactive Technology and Smart Education, (2023) , ahead-of-print(ahead-of-print). |
[32] | Yan N. and Au O.T.-S. , Online learning behavior analysis based on machine learning[J], Asian Association of Open Universities Journal, Emerald Publishing Limited 14: (2) ((2019) ), 97–106. |
[33] | Marion S.D. , Touchette P.E. and Sandman C.A. , Sequential analysis reveals a unique structure for self-injurious behavior, American Journal on Mental Retardation 108: (5) ((2003) ), 301–313. |
[34] | Hou H.T. , Chang K.E. and Sung Y.T. , A longitudinal analysis of the behavioral patterns in teachers using blogs for knowledge interactions, British Journal of Educational Technology 42: (2) ((2011) ), 34–36. |
[35] | Agrawal R. and Srikant R. , Mining sequential patterns[A], Proceedings of the Eleventh International Conference on Data Engineering[C], (1995) :3–14. |
[36] | Pei J. , Han J. , Mortazavi-Asl B. et al., Mining sequential patterns by pattern-growth: the PrefixSpan approach[J], IEEE Transactions on Knowledge and Data Engineering 16: (11) ((2004) ), 1424–1440. |
[37] | Papachristos N.M. , Vrellis I. and Mikropoulos T.A. , A Comparison between Oculus Rift and a Low-Cost Smartphone VR Headset: Immersive User Experience and Learning[A], 2017 IEEE 17th International Conference on Advanced Learning Technologies (ICALT)[C], (2017) :477–481. |
[38] | Moro C. , Štromberga Z. and Stirling A. , Virtualisation devices for student learning: Comon between desktop-based (Oculus Rift) and mobile-based (Gear VR) virtual reality in medical and health science education[J], Australasian Journal of Educational Technology 33: (6) ((2017) )–paris. |
[39] | Sezer A. , İnel Y. Seçkin A.Ç. , et al., An Investigation of University Students’ Attention Levels in Real Classroom Settings with NeuroSky’s MindWave Mobile (EEG) Device[A], 2015, (2015) . |
[40] | Sałabun Wojciech , Processing and spectral analysis of the raw EEG signal from the MindWave, Przeglad Elektrotechniczny 90: (2) ((2014) ), 169–174. |
[41] | MindWave User Guide, ((2009) ) 02.04.2014 tarihinde, http://developer.neurosky.com/docs/lib/exe/fetch.php?media=mindwave_user_guide_en.pdf adresinden erişilmiştir. |
[42] | Zhang T. , Fruchter R. and Frank M. , Are They Paying Attention? A Model-Based Method to Identify Individuals’ Mental States[J], Computer 50: (3) ((2017) ), 40–49. |
[43] | Chen C.-M. and Wu C.-H. , Effects of different video lecture types on sustained attention, emotion, cognitive load, and learning performance[J], Computers & Education 80: ((2015) ), 108–121. |
[44] | Chang J.-J. , Lin W.-S. and Chen H.-R. , How attention level and cognitive style affect learning in a MOOC environment? Based on the perspective of brainwave analysis[J], Computers in Human Behavior 100: ((2019) ), 209–217. |
[45] | Natural-Scene Perception Requires Attention – Michael A. Cohen, George A. Alvarez, Ken Nakayama, 2011[EB/OL]. 2021/-05-28. https://journals.sagepub.com/doi/abs/10.1177/0956797611419168 |
[46] | Wolfe J.M. , Horowitz T.S. and Michod K.O. , Is visual attention required for robust picture memory?[J], Vision Research 47: (7) ((2007) ), 955–964. |
[47] | Naveh-Benjamin M. , Craik F.I.M. , Perretta J.G. et al., The Effects of Divided Attention on Encoding and Retrieval Processes: The Resiliency of Retrieval Processes[J], The Quarterly Journal of Experimental Psychology Section A, SAGE Publications 53: (3) ((2000) ), 609–625. |
[48] | An Analysis of William James’s The Principles of Psychology | Taylor & Francis Group[EB/OL]. 2021/-05-31. https://www.taylorfrancis.com/books/mono/10.4324/9781912282494/analysis-william-james-principles-psychology-macat-team. |
[49] | Berlyne D.E. , Attention, perception and behavior theory[J], Psychological Review, US: American Psychological Association 58: (2) ((1951) ), 137–146. |
[50] | Spires H.A. , Rowe J.P. , Mott B.W. et al., Problem Solving and Game-Based Learning: Effects of Middle Grade Students’ Hypothesis Testing Strategies on Learning Outcomes[J], Journal of Educational Computing Research, SAGE Publications Inc 44: (4) ((2011) ), 453–472. |
[51] | Tan J. and Tan G.B. , (jason. Feedback for Metacognitive Support in Learning by Teaching Environments, Feedback for Metacognitive Support in Learning by Teaching Environments, The 28 th Annual Meeting of the Cognitive Science Society[M], (2006) . |
[52] | Zimmerman B.J. , A social cognitive view of self-regulated academic learning[J], Journal of Educational Psychology, US: American Psychological Association 81: (3) ((1989) ), 329–339. |
[53] | Tan J. and Biswas G. , Simulation-Based Game Learning Environments: Building and Sustaining a Fish Tank[M], Proceedings – DIGITEL 2007: First IEEE International Workshop on Digital Game and Intelligent Toy Enhanced Learning, ((2007) ), 80. |
[54] | Kiili K. , Foundation for problem-based gaming[J], British Journal of Educational Technology 38: (3) ((2007) ), 394–404. |
[55] | Liu M. , Horton L.R. , Corliss S.B. et al., Students’ Problem Solving as Mediated by Their Cognitive Tool Use: A Study of Tool Use Patterns[J], Journal of Educational Computing Research, SAGE Publications Inc 40: (1) ((2009) ), 111–139. |
[56] | Liu M. and Bera S. , An analysis of cognitive tool use patterns in a hypermedia learning environment[J], Educational Technology Research and Development 53: (1) ((2005) ), 5–21. |
[57] | Liu C.-C. , Cheng Y.-B. and Huang C.-W. , The effect of simulation games on the learning of computational problem solving[J], Computers & Education 57: (3) ((2011) ), 1907–1918. |