Quality evaluation of online courses during COVID-19 pandemic based on integrated FCE-AHP method
Abstract
After sudden outbreak of COVID-19 pandemic, the university campuses were closed and millions of university teachers and students had to shift teaching and learning activities from the classrooms to online courses in China. The COVID-19 pandemic undoubtedly brought significant negative effects to university education activities. How does COVID-19 influenced teaching quality and the degree of influences have been studied by many researches. However, the online course quality which is influences by COVID-19 pandemic was commonly evaluated qualitatively rather than quantitatively. In order to obtain quantitative evaluation results of online course quality during the pandemic period, the integrated FCE-AHP evaluation was applied. Based on real case of online courses, the influence factors of online course quality were divided into four first-level indicators and further subdivided into 14 second level indicators. The weight vectors of evaluation indicators were determined based on experts’ comments from the Teaching Affairs Committee and the fuzzy evaluation memberships were calculated based on questionnaire results of 2021 students. The evaluation results revealed that the integral performance of online courses is acceptable and the performances of students and hardware are relative weaker. Finally, some improvement measures were conducted to deal with difficulties encountered in online courses during COVID-19 pandemic period.
1Introduction
Since the beginning of 2020, the outbreak of the COVID-19 resulted in closure of university campuses for a whole semester in China. Therefore, the conventional teaching and studying activities had to be paused and transferred to online courses [1]. Over 40 million university students and 1.67 million university teachers had to stay at home and participate in online classes [2]. The significant changes on teaching and studying pattern definitely weakened the effectiveness of teaching and learning practices for both university teachers and students [3].
Outbreak of the COVID-19 pandemic impacted university teaching and studying works from multiple aspects and many researchers analyzed the main influencing factors of COVID-19 to the daily teaching and studying activities. By proposing an innovative evaluation index system, Chen et al analyzed the impacts of COVID-19 on teaching activities and revealed users’ requirements of online teaching platforms [3]. Rodriguez-Segura et al summarized the factors that influence student satisfaction in the teaching strategies during COVID-19 pandemic period and the online teaching platforms [4]. Arshad Khan et al valuated students’ perception towards e-learning based on Confirmatory Factor Analysis (CFA) technique [5]. Cassibba et al identified challenges and difficulties based on questionnaire results, as well as quantitative and qualitative analyses were conducted [6]. Moreover, based on the analyzing results of online teaching performance during the COVID-19 pandemic period, some modification and refining measures were proposed by some scholars. Sá and Serpa suggested improvement of digital sustainable development in higher education based on content analysis [7]. Dresser et al proposed improvements of strategic plans of pharmacy education based on searching results of 142 program websites [7]. Portela proposed improved TechTeach which consists of Blended-Learning and Project Based-Learning during the COVID-19 pandemic outbreak [8]. Milovanović et al overcame the difficulties on online learning of architecture students during COVID-19 pandemic outbreak by developing online workshop [9]. Aiming at improving the teaching and studying experiences for teachers and students, many researches proposed the solutions of online education activities during COVID-19 pandemic outbreak. While the quantitative studies of impact of COVID-19 on different aspects of universities education are barely reported.
The online course quality during the COVID-19 was affected on many aspects. Understanding which aspect is the most heavily affected by COVID-19 plays an important role on optimizing teaching strategy and enhancing studying effectiveness during the outbreak of COVID-19. The main purposes of online course quality evaluation are: (1) providing accurate feedbacks for teaching performances; (2) understanding the main influence factors of course quality; and (3) obtaining the references of course improvements. Many researches on the online teaching of universities during COVID-19 pandemic outbreak had been conducted and elaborated. Moreover, massive data had been collected from questionnaires. However, the multi-layer influence factors of online course quality and qualitative comments from questionnaires make the quantitative evaluation results barely obtained. Therefore, the demands of reality practices call for an appropriate evaluation method which is able transfer complex qualitative into quantitative results.
In order to accurately evaluate the impact of COVID-19 on online courses and make improvement measures more scientifically. This paper summarized the main factors of online course quality during the COVID-19 pandemic period. Based on questionnaires from 2021 students and comments from Teaching Affairs Committee, the influence degree of each factor, as well as evaluation results of online course quality were quantitatively assessed quantitatively by applying integrated Fuzzy Comprehensive Evaluation-Analytic Hierarchy Process (FCE-AHP) method. Finally, some improvement measures were proposed and implemented according to the evaluation results.
2Integrated FCE-AHP method
In recent years, popular evaluation methods include AHP (Analytic Hierarchy Process) method, FAHP (Fuzzy Analytic Hierarchy Process) method, FCE (Fuzzy Comprehensive Evaluation) method, grey relational analysis method, comprehensive evaluation based on neural network and etc. The AHP method is able to obtain solutions with high reliability and small errors. As for grey relational analysis method, data need not be normalized, and calculation is simple, but it is difficult to define curve similarity of time variables. Comprehensive evaluation based on neural network has the network with adaptive ability and fault tolerance. While the accuracy of the evaluation results is low and huge amount of training samples are required [10–19]. In this paper, the integrated FCE-AHP method is used. Fuzzy Comprehensive Evaluation (FCE) is a method of converting qualitative evaluation into quantitative evaluation based on the membership degree theory of fuzzy mathematics [20]. It had been widely applied to solve problems which are fuzzy and difficult to quantify [21]. Moreover, it is suitable for solving various non-deterministic problems. For complex problems, FCE method is difficult to directly give the weight of each evaluation indicator [22]. Therefore, the AHP method is used to calculate the weight for FCE method.
In this paper, the integrated FCE-AHP method is applied to evaluate the teaching quality and find the major influence factors of teaching activities during COVID-19 pandemic outbreak period. The AHP was used to obtain the evaluation index weight and the fuzzy comprehensive evaluation method was applied to rate the relative “priority” of the criteria. The evaluation procedure of integrated FCE-AHP method is shown in Fig. 1.
Fig. 1
Evaluation procedure of integrated FCE-AHP method.
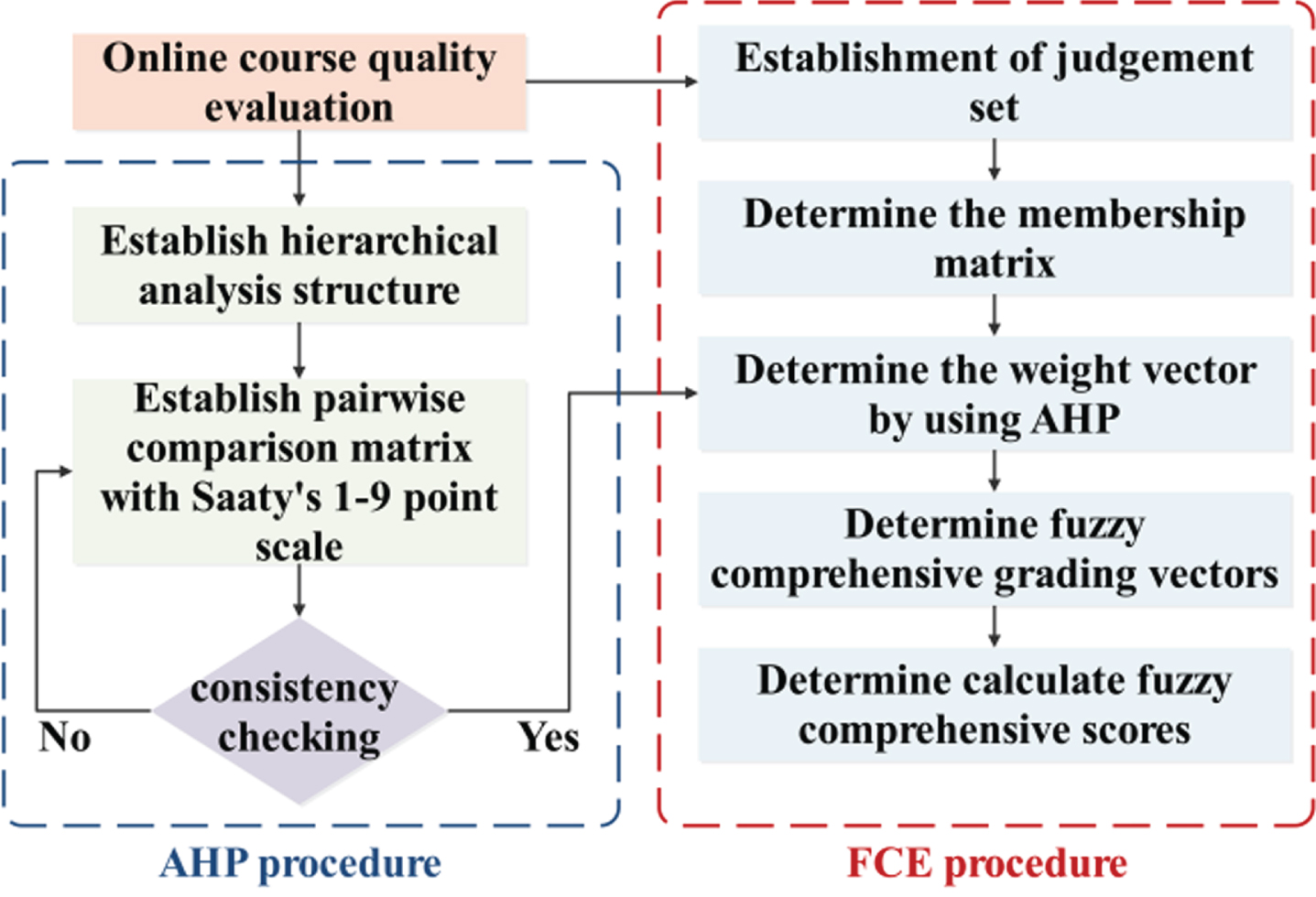
2.1Fuzzy comprehensive evaluation method
The FCE method is conducted based on the fuzzy set theory which was firstly proposed by Zadeh [23, 24]. For complex objects which are subjected to multi-factors, quantitative evaluation can be obtained by applying membership functions of FCE method. The implementation steps of FCE method are shown below:
2.1.1Establish the evaluation indicator set U and judgment set V
The quality of online course during the COVID-19 pandemic outbreak period is evaluated from different aspects. Each aspect can be designated as an evaluation indicator. The evaluation indicator set is expressed by Equation (1).
(1)
Where Ui(i=1, 2, ... , n) represents evaluation indicators, n is an integer.
The judgment set is represented as V, and shown in Equation (2):
(2)
Where Vj(i=1, 2, ... , n) represents evaluation judgments, m is an integer.
2.1.2Establish the membership matrix R
The membership matrix represents the degree of membership of an indicator to a judgment [25]. The membership degree of every single element in the alternative set V can be determined by rij and the membership matrix R is shown in Equation (3).
(3)
Where m and n represent the numbers of corresponding second-level indicators and judgment criteria, respectively, rij is the membership of the ith indicator Ui and the jth judgment Vj.
2.1.3Establish the weight vector W
The weight vector W represents the importance degree of the evaluation indicators. The weight vector W consists of wi is describe in Equation (4). In this paper, the weight vector W is calculated by AHP method which will be elaborated later.
(4)
2.1.4Determine the fuzzy comprehensive grading vector B
The grading vector elaborates the eventual comprehensive result of the online courses quality evaluation based on fuzzy arithmetic [26]. The grading vector can be calculated as Equation (5).
(5)
2.1.5Defuzzification and quantitative evaluation
Based on five levels of evaluation set, each level of online course quality is assigned a score. Suppose the online course quality set H = {excellent, good, medium, weak, poor} = {100,80,60,40,20}. Fuzzy comprehensive score of the teaching quality can be obtained as follow:
(6)
Grading the fuzzy comprehensive scores into five levels, as shown in Table 1.
Table 1
Grades of the fuzzy comprehensive scores
Grade | Excellent | Good | Medium | Weak | Poor |
Score | 90–100 | 80–89 | 60–79 | 40–59 | <40 |
2.2The AHP method
In integrated FCE-AHP evaluation approach, the AHP algorithm is used to calculate weight vector. The AHP allows users to calculate the relative weight of multiple criteria rather than given criteria intuitively. In case quantitative ratings are not available, decision makers or assessors can still recognize whether one criterion is more important than another. The steps of AHP are shown as follow:
2.2.1The hierarchical analysis structure
In interpreted FCE-AHP evaluation processes, the evaluation indicator set of FCE method is taken as the hierarchical analysis structure.
2.2.2Establishing pairwise comparison matrix
After the hierarchical analysis structure is constructed, the pairwise comparison matrix can be established as shown below:
(7)
Where Wn×n indicates pairwise comparison matrix and aij represents quantified judgment for a pair of indicators within the same level. The value of is expressed by Saaty’s 1-9 point scale which is shown in Table 2 [27, 28].
Table 2
Saaty’s 1-9 point scale of pairwise comparison matrix
Importance | Meaning |
Scale Value | |
1 | Both elements are of equal importance |
3 | One element is slightly more important than the other |
5 | One element is more important than the other |
7 | One element is extremely important than the other |
9 | One element is absolutely important than the other |
2,4,6,8 | Represents the middle value of the above judgment |
2.2.3Consistency check
The consistency check refers to the allowable range of inconsistency for the pairwise comparison matrix. The consistency ratio (CR) value is supposed to be not larger than 0.1 to claim that the pairwise comparison matrix is consistent and the CR value greater than 0.1 is not acceptable [29–32].
The consistency ratio (CR) can be calculated by using Equation (8):
(8)
Where, RI is the random index which is used for random consistency. The RI value depends on the size of the matrix and is presented in Table 3 [33].
Table 3
Random Consistency Index (RI)
n | 1 | 2 | 3 | 4 | 5 | 6 | 7 | 8 | 9 | 10 |
RI | 0 | 0 | 0.58 | 0.9 | 1.12 | 1.24 | 1.32 | 1.41 | 1.45 | 1.49 |
Consistency Index (CI) can be calculated as fellow:
(9)
Where, n is the number of rows in the pairwise comparison matrix and λmax represents the largest eigenvalue of the comparison matrix.
Calculate the maximum eigenvalue:
(10)
(11)
The initial weight coefficient can be calculated by using:
(12)
Normalize the pairwise matrix by using Equation 13:
(13)
Where, wi is the eigenvector.
3Case study
3.1Case description
The teaching quality of university courses are affected by many elements (e.g. teachers’ performance, teaching methods, learning attitudes of students, etc.). During the COVID-19 epidemic period, the online courses were conducted. This is the first time that online teaching has been conducted nationwide and worldwide. 40 million university students and 1.67 million university teachers had to stay at home and participate in online courses throughout the semester (from Feb 2020 to July 2020) [2]. The influences and results of this huge and wide range of changes in teaching forms are not clear.
The School of Civil Engineering and Architecture, Anhui University of Science and Technology has 138 teachers and over 2800 students. During the COVID-19 epidemic period, the university campus was closed and the conventional offline teaching activities in the first semester of year 2020 was canceled. All teachers and students had to made switches from classroom teaching to online teaching. In order to understand the performance of online courses and find the major factors influencing the online course quality during the COVID-19 epidemic period, students were asked to fill in a questionnaire and 2021 feedbacks were received. Based on questionnaire results, the FCE-AHP method is used to evaluate the teaching quality of online courses during the COVID-19 epidemic period.
3.2Establish indicator set
The teaching quality evaluation is consisted of four first level indicators, which are: teachers’ performance, students’ performance, teaching activity implementation, as well as hardware conditions. Moreover, four first level indicators are further subdivided into 14 second level indicators. The details of the indicator set are shown in Fig. 2 and elaborated as follows.
Fig. 2
Evaluation indicator system for online course quality during the COVID-19 epidemic period.
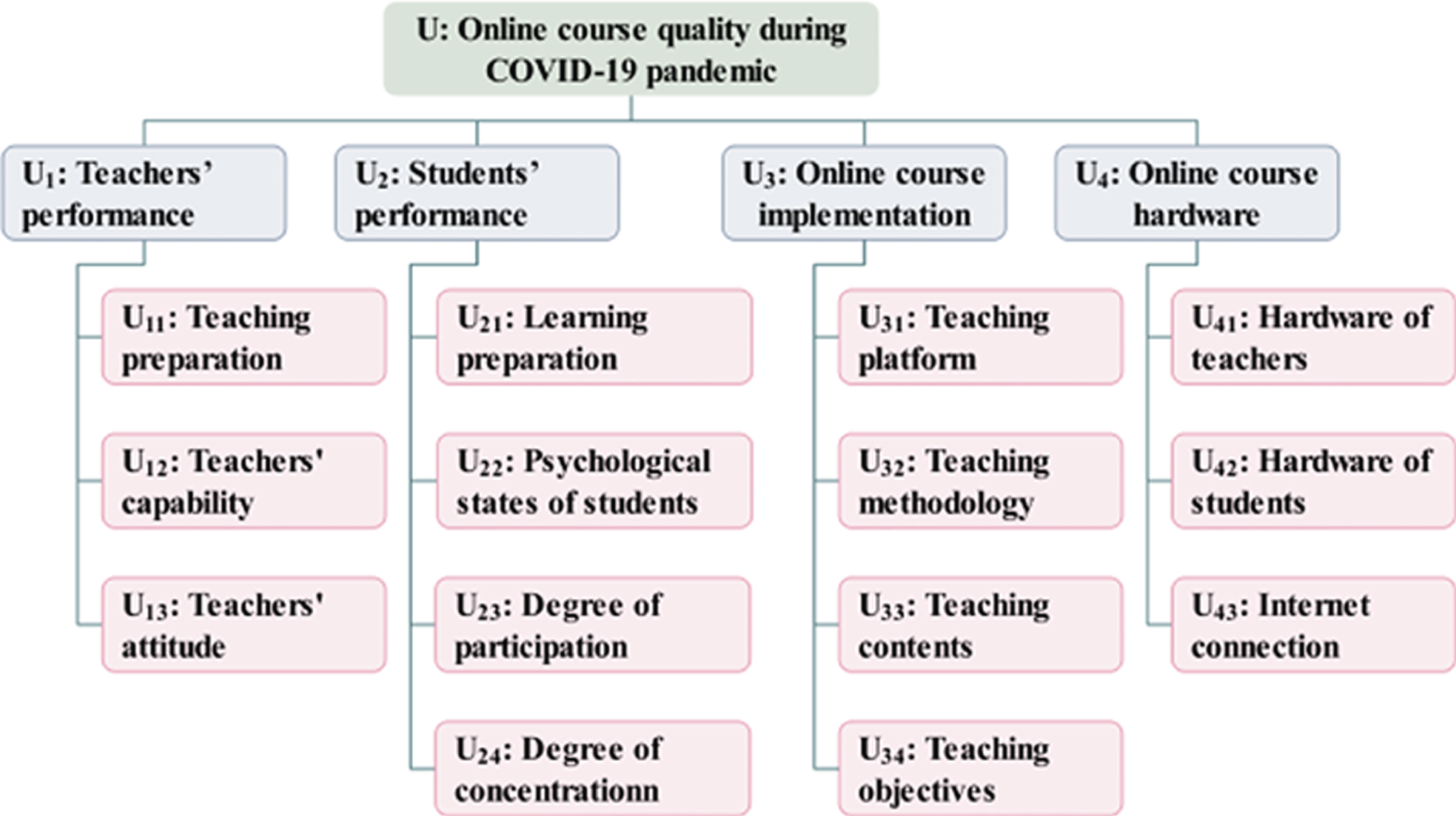
3.2.1Teachers’ performance
(1) Teaching preparation. Preparation and planning are a critical component of effective teaching. Teachers should prepare to teach a course by determining its learning objectives, developing a syllabus, and making lesson plans.
(2) Teachers’ capability. For teachers, teaching a course requires professional knowledge, and the ability to anticipate and answer questions. Lack of teaching capability could significantly results in bad teaching quality for both online and offline teaching activities.
(3) Teachers’ attitude. Attitudes mean the individuals’ prevailing tendency to respond favorably or unfavorably to an object [34]. Attitudes may be positive (favorable) or negative (unfavorable). Teachers’ negative attitudes could result in poor performance of teaching activities.
3.2.2Students’ performance
The students’ performances also heavily affect the qualities of the online teaching activities. In order to obtain good learning outcomes, the students are supposed to dedicate themselves to studies. The students’ performance is subdivided into four second level indicators.
(1) Learning preparation. Good preparation prior to lectures leads to better student performance [35]. Students are often asked to prepare for their next class by reading textbooks or previewing materials. By conducting preview, students can get an overview of textbook content, thereby enhancing better understanding for the next lecture.
(2) Psychological states of students. It was found that in the first two weeks after the COVID-19 pandemic outbreak, students suffered higher stress, anxiety, and depression [36]. These passive emotions could leads to negative impact on study.
(3) Degree of participation. In order to consolidate knowledge learnt from the online courses, students are encouraged to participate in the discussions and in-class exercises.
(4) Degree of concentration. As for students, studying at home may encounter problems of absent-mind due to lack of self-regulation. During the online teaching activities, the teachers can barely get students’ responses and attract students’ attention. Therefore, the concentration of students plays an important role in online studying activities [37].
3.2.3Online course implementation
The online course implementation is primarily teacher-dominated, but the cooperation of students is indispensable. This first-level indicator is subdivided into four second level indicators.
(1) Teaching platform. Online teaching platforms are supposed to be able to assist teachers manage their lectures and courses, evaluation students performances and responses. In addition, the online teaching platform should offer students more opportunities to obtain knowledge and information.
(2) Teaching methodology. A teaching strategy is the method that teachers use to convey information and knowledge to students. Teachers require the implementation of effective teaching strategies in order to meet students’ needs.
(3) Teaching contents. Teaching content is a hugely important part of the online teaching activities. It refers to the choice, organization and sequencing of topics.
(4) Teaching objectives. Course design starts from deciding learning objectives. Clear, student-centered and measurable teaching objectives are able to effectively guide the design and implementation of online teaching activities.
3.2.4Online course hardware
The online courses are fundamentally supported by various hardware which can be categorized into three aspects:
(1) Hardware of teachers. For teachers who are conducting online courses, the capabilities of hardware (e.g. computers, laptops, microphones, pen tablet and etc.) heavily effect on the quality and efficiency of online courses.
(2) Hardware of students. The equipment needed by students is similar to that of teachers, while the performance requirements are lower. As for students, computers or laptops are the ideal choices for online courses, and pads or cellphones are also acceptable. Lack of digital resources will definitely results in inability of students to participate in online courses at home.
(3) Internet connection. Bad connection of the networks could results in delay or interruption of image and voice transmission. After the COVID-19 pandemic outbreak, millions of teachers and students access the internet simultaneously in China. It undoubtedly increases the burden of the network. Therefore, the quality of internet connection is also important for both teachers and students.
3.3Determination of the weight vector W
The weight vectors for every evaluation indicator are calculated by AHP method which has been introduced in section 2. The members of the Teaching affair committee which includes twenty experienced university teachers and five outstanding students were invited to vote to determine the weight vectors of evaluation indicators. The information of the Teaching affair committee is illustrated in Table 4 and the AHP calculation results which include pairwise comparison, weights and consistency check are shown in Table 5 to Table 9.
Table 4
Information of the teaching affair committee
Classifications | Number | Percentage (%) | |
Gender | Male | 14 | 56% |
Female | 11 | 44% | |
Position | Professor | 7 | 28% |
Associate Professor | 9 | 36% | |
Lecturer | 4 | 16% | |
Student | 5 | 20% |
Table 5
AHP calculation results of first-level indicators
U-Ui | U1 | U2 | U3 | U4 | Weight |
U1 | 1 | 2 | 1/3 | 1/4 | 0.1358 |
U2 | 1/2 | 1 | 1/2 | 1/3 | 0.1142 |
U3 | 3 | 2 | 1 | 1/2 | 0.2796 |
U4 | 4 | 3 | 2 | 1 | 0.4704 |
λmax=4.1532 CI = 0.0511 RI = 0.90 CR = 0.0568<1. Satisfied consistency standard.
Table 6
AHP calculation results of teachers’ performance (U1)
U1-U1j | U11 | U12 | U13 | Weight |
U11 | 1 | 1/3 | 1/2 | 0.1634 |
U12 | 3 | 1 | 2 | 0.5395 |
U13 | 2 | 1/2 | 1 | 0.2970 |
λmax=3.0092 CI = 0.0046 RI = 0.58 CR = 0.0079<1. Satisfied consistency standard.
Table 7
AHP calculation results of students’ performance (U2)
U2-U2j | U21 | U22 | U23 | U24 | Weight |
U21 | 1 | 1/2 | 2 | 3 | 0.3325 |
U22 | 2 | 1 | 3 | 1/2 | 0.3325 |
U23 | 1/2 | 1/3 | 1 | 3 | 0.2124 |
U24 | 1/3 | 1/2 | 1/3 | 1 | 0.1226 |
λmax=4.2146 CI = 0.0515 RI = 0.90 CR = 0.0795<1. Satisfied consistency standard.
Table 8
AHP calculation results of online course implementation (U3)
U3-U3j | U31 | U32 | U33 | U34 | Weight |
U31 | 1 | 1/3 | 1/5 | 1/3 | 0.0797 |
U32 | 3 | 1 | 1/3 | 2 | 0.2454 |
U33 | 5 | 3 | 1 | 2 | 0.4829 |
U34 | 3 | 1/2 | 1/2 | 1 | 0.1920 |
λmax=4.1308 CI = 0.04361 RI = 0.90 CR = 0.0484<1. Satisfied consistency standard.
Table 9
AHP calculation results of online course hardware (U4)
U4-U4j | U41 | U42 | U43 | Weight |
U41 | 1 | 1/3 | 2 | 0.2493 |
U42 | 3 | 1 | 3 | 0.5936 |
U43 | 1/2 | 1/3 | 1 | 0.1571 |
λmax=3.0536 CI = 0.0268 RI = 0.58 CR = 0.0462<1. Satisfied consistency standard.
3.4Establish evaluation set
Evaluation set is used to divide the single factors into grade. There are five levels of online course quality (Excellent, good, medium, weak, and poor). The evaluation set is shown in Equation 14.
(14)
3.5Results of questionnaires and membership matrixes
The memberships of indicators were calculated based on questionnaires results. Students were asked to fill in a questionnaire and 2021 feedbacks were received. The students’ information is shown in Table 10. 5-point Likert scale is designed for questionnaires. The performances of each indicator are divided into five levels: excellent, good, medium, weak and poor. The questionnaire results are shown in Table 11.
Table 10
Students’ information
Classifications | Number | Percentage (%) | |
Gender | Male | 1156 | 57.20% |
Female | 865 | 42.80% | |
Major | Civil engineering | 1008 | 49.88% |
Engineering management | 426 | 21.08% | |
Architectural environment | 342 | 16.92% | |
Landscape architecture | 152 | 7.52% | |
Architecture | 93 | 4.60% | |
Grade | Year 1 | 423 | 20.93% |
Year 2 | 645 | 31.91% | |
Year 3 | 503 | 24.89% | |
Year 4 | 450 | 22.27% |
Table 11
Results of questionnaires
Second -level indicator | Evaluation numbers (degree of membership) | ||||
Excellent | Good | Medium | Weak | Poor | |
U11 | 766 (0.3795) | 959 (0.4745) | 286 (0.1415) | 6 (0.0030) | 3 (0.0015) |
U12 | 698 (0.3454) | 995 (0.4923) | 314 (0.1554) | 8 (0.0040) | 6 (0.0030) |
U13 | 791 (0.3914) | 940 (0.4651) | 280 (0.1385) | 6 (0.0030) | 4 (0.0020) |
U21 | 741 (0.3667) | 599 (0.2946) | 349 (0.1727) | 216 (0.1069) | 116 (0.0574) |
U22 | 921 (0.4557) | 534 (0.2642) | 373 (0.1846) | 102 (0.0505) | 91 (0.0450) |
U23 | 585 (0.2895) | 723 (0.3577) | 517 (0.2558) | 127 (0.0628) | 69 (0.0341) |
U24 | 501 (0.2479) | 723 (0.3577) | 617 (0.3053) | 109 (0.0539) | 71 (0.0351) |
U31 | 555 (0.2746) | 481 (0.2380) | 609 (0.3013) | 262 (0.1296) | 114 (0.0564) |
U32 | 757 (0.3746) | 696 (0.3444) | 544 (0.2692) | 14 (0.0069) | 10 (0.0049) |
U33 | 786 (0.3889) | 701 (0.3469) | 525 (0.2598) | 4 (0.0020) | 5 (0.0025) |
U34 | 525 (0.2598) | 789 (0.3904) | 673 (0.3330) | 22 (0.0109) | 12 (0.0059) |
U41 | 411 (0.2034) | 729 (0.3607) | 842 (0.4166) | 25 (0.0124) | 14 (0.0069) |
U42 | 628 (0.3107) | 837 (0.4142) | 516 (0.2553) | 29 (0.0143) | 11 (0.0054) |
U43 | 731 (0.3617) | 914 (0.4523) | 358 (0.1771) | 11 (0.0054) | 7 (0.0035) |
The fuzzy evaluation membership matrix Ri are calculated based on 2021 questionnaires which were answered by students. The normalized fuzzy evaluation membership matrixes of the four first-level indicators are shown as follows:
(15)
(16)
(17)
(18)
3.6Calculate fuzzy comprehensive grading vectors
Based on Equation (3), the four fuzzy comprehensive grading vectors for four first level indicators can be calculated by multiplying weight matrix and fuzzy evaluation membership matrix.
(19)
In the same manner, the fuzzy comprehensive evaluation matrixes for other first level indicators can be calculated as follows:
(20)
(21)
(22)
After finishing the calculation of the fuzzy comprehensive evaluation matrixes for the first level indicators, the next step of fuzzy comprehensive evaluation processes is calculating fuzzy comprehensive evaluation matrixes for the second level indicators. According to previous calculation results, the fuzzy comprehensive grading vectors B of teaching quality evaluation can be calculated as shown below:
(23)
3.7Defuzzification and calculate fuzzy comprehensive scores
The calculated fuzzy comprehensive grading vectors B cannot represent the online course quality directly. In order to obtain the quantitative description of the online course, as well as the influence degrees of different factors. The defuzzification is conducted by calculating the fuzzy comprehensive scores. By using Equation (6), the fuzzy comprehensive scores are shown as follows:
(24)
(25)
(26)
(27)
(28)
4Results of integrated FCE-AHP evaluation and improvement measures
4.1Evaluation results
By using integrated FCE-AHP method, the integral fuzzy comprehensive score of online course quality and scores of each evaluation indicator had been obtained. According to grade-score relationship which is shown in Table 1, the fuzzy comprehensive score of online course quality during COVID-19 pandemic period is 80.06, which score level is “good”. The fuzzy comprehensive scores for four first-level indicators (teachers’ performance, students’ performance, online course implementation and online course hardware) are: 84.04, 77.57, 80.36 and 79.35 respectively. The corresponding score levels are: good, medium, good and medium.
The evaluation results suggested that, during the COVID-19 pandemic period, the online course quality of School of Civil Engineering and Architecture, Anhui University of science and Technology is acceptable, but there is still room for improvements. The performance of teachers and online course implementation are good while the score levels of students’ and hardware are merely medium.
4.2Problem identification and Modification measures
During the COVID-19 pandemic period, the online teaching became the only method of the teaching and studying for teachers and students. There are no previous experiences of long-term and large-scale online teaching that can be taken as references. Some problems were encountered and corresponding improvement measures were conducted to promote online course quality during the COVID-19 pandemic period. The problems encountered in online course during the COVID-19 pandemic period, as well as the solutions are shown as follow.
4.2.1Psychological problems of students during the COVID-19 pandemic period
The COVID-19 pandemic caused stresses that may cause psychological symptoms challenges for students, especially for students who have pre-existing psychological problems. Many causes could results in psychological problems of students, such as: the fear of COVID-19 pandemic, rapid increasing of inflected cases, the inconvenience caused by COVID-19 epidemic, being isolated from other students, the difficulties encountered in learning activities, and etc. The psychological problems of students led to distraction or even absence from the classes. Therefore, the psychological health of students plays an important role in online courses during the COVID-19 pandemic period.
Two improvement measures had been conducted to relieve the psychological problems of students, which are shown as follow:
(1) Conduct COVID-19 epidemic prevention seminars. The fear of COVID-19 is a main cause of psychological problems of students [38]. Facing a sudden outbreak of COVID-19, many students have no idea about how to protect themselves from the COVID-19. Fear of the unknown epidemic resulted in panic spreading among students. Therefore, online epidemic prevention seminars were conducted to popularize knowledge of epidemic prevention and thereby relieving the fear of students to the epidemic.
(2) Establish psychological counselling hotline. A 24/7 direct access to certified psychological counselors via phone, Email, or Wechat was established. Professional psychological counselors evaluate the psychological states of students and make further psychological counselling plan if the student is suffering serious psychological problems. While the students who have mild psychological problems were handed over to trained volunteers who were recruited from teachers and students.
4.2.2Lack of face-to-face communications
The communication between human beings is carried out through language, body movements and facial expression. Lack of face-to-face communications significantly decreased the information exchange between teachers and students. This drawback significantly decreases the teaching and learning effectiveness. In online courses, teachers cannot get feedbacks from students’ reactions, therefore, the confusion and distraction of students cannot be founded in good time and teaching strategies cannot be modified according to students’ responses. Aiming to resolve problems of lack of face-to-face communications, some improvement measures were implemented.
(1) Question-guided teaching strategy. Teachers use questions to ensure that students are attentive and engaged, and to assess students’ understanding. Moreover, teachers can guide students on their learning process by asking a set of well-designed questions and the learning efficiency can be assessed from the answers of students.
(2) Choose suitable online course platform. Live chat software cannot be used as online course platform. An appropriate online course platform is supposed to be able to implement most interaction functions: input and output voice and video from both teachers’ and students’ sides, play ppt slides, leave comments, ask questions, make announcement, blackboard writing, exercises and quizzes, unloading and downloading studying materials and etc. Ketangpai, which is an online-class management platform were recommended to teachers and students [39]. It integrated multiple functions of daily teaching and learning activities together and can be operated on various electronic devices such as: smartphones, computers or pads.
(3) Add tutorials to answering questions. Additional tutorials were added after every two or three lectures. After learning classes and finishing exercises or homeworks, new questions derived from deep thinking may be asked by students. As for students, tutorials are ideal opportunities for consolidating knowledge and inspiring further thinking.
4.2.3Unsatisfied hardware for online courses
Due to the sudden outbreak of COVID-19 pandemic, teachers and students had to participate online course by using normal hardware that they can get at home. While, according to practical experiences of online teaching and learning practices, normal microphones or earphones are not capable of input and output voice clearly. The normal microphones which were used by teachers always output noise to students’ sides. Moreover, normal headsets of students further amplified noise to an unacceptable level and bad internet connection resulted in the problems of delay and interruption of online courses. The corresponding improvements measures were proposed as follow.
(1) Upgrade the microphone for teachers. Low quality microphone used by teachers Prevented students from hearing the teachers’ voices. Therefore, high quality microphones which have noise reduction function were equipped for teachers. In order to ensure good reading of ppt slides, students were encouraged to use good headset and large screen devices such as computer or laptop. The smartphones are not recommended to be used for online learning purposes.
(2) Solutions for bad internet connection. A few students who live in remote areas cannot get good internet services at home. The university leased mobile internet devices and sent them to students who cannot get suitable internet services by express. On the other hand, conducting online courses by millions of teachers and students in China at the same time could result in internet congestions. In case of low internet speed cause by possible internet congestions, teachers are required to save playbacks of online courses so that students can download playbacks during their casual times.
5Conclusions
After sudden outbreak of COVID-19 pandemic, unprepared shift on teaching and learning patterns were encountered by teachers and students. The online course quality during COVID-19 pandemic period and influence degrees of each influence factors are hardly obtained quantitatively. This paper identified influence factors of online course quality during COVID-19 pandemic. The influence factors were divided into four first-level indicators and further subdivided into 14 second level indicators. The integrated FCE-AHP evaluation method was applied based on the practices of online teaching and learning activities implemented by School of Civil Engineering and Architecture, Anhui University of Science and Technology. In AHP assessment operations, the weight vectors of evaluation indicators were determined based on experts’ comments from the Teaching Affairs Committee. Fuzzy evaluation memberships were calculated based on questionnaire results of 2021 students. By conducting integrated FCE-AHP evaluation, the qualitative evaluation results were transferred to quantitative scores. The fuzzy comprehensive scores of the overall performance, teachers’ performance, Students’ performance, online course implementation and online course hardware are 80.06, 84.04, 77.57, 80.36 and 79.35, respectively. The evaluation results revealed that the integral performance of online courses is acceptable. Moreover, the COVID-19 affects the teaching quality on every aspects and the performances of students and hardware are relative weaker compared with other aspects due to their lower fuzzy comprehensive scores. Finally, some improvement measures were conducted to deal with difficulties encountered in online courses during COVID-19 pandemic period.
Acknowledgment
This research was funded by the National Natural Science Foundation of China (Grant No. 51804006), Fund of State Key Laboratory of Mining Response and Disaster Prevention and Control in Deep Coal Mines (Grant No. SKLMRDPC20ZZ04), and Teaching Reform Research Fund of Anhui University of Science and Technology (Grant No. 13200237). The authors gratefully acknowledge financial support of the above-mentioned agencies.
References
[1] | Students’ Perception towards E-Learning during COVID-19 Pandemic in India: An Empirical Study, Sustainability 13: ((2020) ), 57. |
[2] | Bhargava A. and Pathy M. , Attitude of Student Teachers Towards Teaching Profession, Turkish Online Journal of Distance Education 15: ((2014) ). |
[3] | Kim E. , Kim J. and Han S. , Understanding Student Acceptance of Online Learning Systems in Higher Education: Application of Social Psychology Theories with Consideration of User Innovativeness, Sustainability 13: ((2021) ), 896. |
[4] | Chen T. , Peng L. , Jing B. , Wu C. , Yang J. and Cong G. , The Impact of the COVID-19 Pandemic on User Experience with Online Education Platforms in China, Sustainability 12: ((2020) ), 7329. |
[5] | Han J.L. , Micro-lecture Teaching for Improving the Learning Effect of Non-English Majors at North China Electric Power University, English Language Teaching 12: ((2019) ), 209. |
[6] | Jiang R. , Knowledge, attitudes and mental health of university students during the COVID-19 pandemic in China, Children and Youth Services Review 119: ((2020) ), 105494. |
[7] | Millet I. and Saaty T.L. , On the relativity of relative measures-accommodating both rank preservation and rank reversals in the AHP, European Journal of Operational Research 121: ((2000) ), 205–212. |
[8] | Milovanović A. , Kostić M. , Zorić A. , Djordjevic A. , Pešić M. , Bugarski J. , Todorović D. , Sokolovic N. and Josifovski A. , Transferring COVID-19 Challenges into Learning Potentials: Online Workshops in Architectural Education, Sustainability 12: ((2020) ), 7024. |
[9] | Portela F. , TechTeach-An Innovative Method to Increase the Students Engagement at Classrooms, Information 11: ((2020) ), 483. |
[10] | Prokhorov A.O. , Self-Regulation Of Psychological States In Educational Activity Of Students, 2020. |
[11] | Zhang H. , He X. and Mitri H. , Fuzzy comprehensive evaluation of virtual reality mine safety training system, Safety Science 120: ((2019) ), 341–351. |
[12] | Lin M. , Xu W. , Lin Z. and Chen R. , Determine OWA Operator Weights Using Kernel Density Estimation Multi-attribute group decision-making under probabilistic uncertain linguistic environment, Economic Research-Ekonomska Istrazivanja 33: ((2020) ), 1441–1464. |
[13] | Lin M. , Huang C. , Xu Z. and Chen R. , Evaluating IoT platforms using integrated probabilistic linguistic MCDM method, IEEE Internet of Things Journal 7: ((2020) ), 11195–11208. |
[14] | Lin M. , Wang H. and Xu Z. , TODIM-based multi-criteria decision-making method with hesitant fuzzy linguistic term sets, Artificial Intelligence Review 53: ((2020) ), 3647–3671. |
[15] | Lin M. , Chen Z. , Xu Z. , Gou X. and Herrera F. , Score function based on concentration degree for probabilistic linguistic term sets: An application to TOPSIS and VIKOR, Information Sciences 551: ((2020) ), 270–290. |
[16] | Lin M. , Huang C. , Chen R. , Fujita H. and Wang X. , Directional correlation coefficient measures for Pythagorean fuzzy sets: their applications to medical diagnosis and cluster analysis, Complex & Intelligent Systems 7: ((2021) ), 1025–1043. |
[17] | Liu X. , Wang Z. , Zhang S. and Liu J. , Probabilistic hesitant fuzzy multiple attribute decision-making based on regret theory for the evaluation of venture capital projects, Economic Research-Ekonomska Istraživanja 33: ((2020) ), 672–697. |
[18] | Gou X. , Xu Z. and Herrera F. , Consensus Model Handling Minority Opinions and Noncooperative Behaviors in Large-Scale Group Decision-Making Under Double Hierarchy Linguistic Preference Relations, IEEE Transactions on Cybernetics 99: ((2020) ), 1–14. |
[19] | Gou X. , Xu Z. and Herrera F. , Probabilistic double hierarchy linguistic term set and its use in designing an improved VIKOR method: The application in smart healthcare, Journal of the Operational Research Society (2020), 1–20. |
[20] | Rodriguez-Segura L. , Zamora Antuñano M.A. , Rodriguez J. , Paredes-García W. , Altamirano-Corro J. and Cruz M. , Teaching Challenges in COVID-19 Scenery: Teams Platform-Based Student Satisfaction Approach, Sustainability 12: ((2020) ), 7514. |
[21] | Rosalinda C. , Ferrarello D. , Musso P. and Pennisi M. , Teaching Mathematics at Distance: A Challenge for Universities, Education Sciences 11: ((2020) ), 1–20. |
[22] | Sá M. and Serpa S. , The COVID-19 Pandemic as an Opportunity to Foster the Sustainable Development of Teaching in Higher Education, Sustainability (Switzerland) 12: ((2020) ), 1–16. |
[23] | Saaty T.L. , Homogeneity and clustering in AHP ensures the validity of the scale, European Journal of Operational Research 72: ((1994) ), 598–601. |
[24] | Saaty T.L. , Time dependent decision-making; dynamic priorities in the AHP/ANP: Generalizing from points to functions and from real to complex variables, Mathematical and Computer Modelling 46: ((2007) ), 860–891. |
[25] | Saaty T.L. and Shang J.S. , An innovative orders-of-magnitude approach to AHP-based mutli-criteria decision making: Prioritizing divergent intangible humane acts, European Journal of Operational Research 214: ((2011) ), 703–715. |
[26] | Shimada A. , Okubo F. , Yin C. and Ogata H. , Automatic Summarization of Lecture Slides for Enhanced Student Preview-Technical Report and User Study, IEEE Transactions on Learning Technologies 11: ((2017) ), 165–178. |
[27] | Wang C. , Pan R. , Wan X. , Tan Y. , Xu L. , Ho C.S. and Ho R.C. , Immediate Psychological Responses and Associated Factors during the Initial Stage of the Coronavirus Disease (COVID-19) Epidemic among the General Population in China, International Journal of Environmental Research and Public Health 17: ((2020) ), 1–25. |
[28] | Wunong Z. , Wang Y. , Yang L. and Wang C. , Suspending Classes Without Stopping Learning: China’s Education Emergency Management Policy in the COVID-19 Outbreak, Journal of Risk and Financial Management 13: ((2020) ), 55. |
[29] | Yang X. , Li D. , Liu X. and Tan J. , Learner behaviors in synchronous online prosthodontic education during the 2020 COVID-19 pandemic, The Journal of Prosthetic Dentistry (2020). |
[30] | Zhu B. and Xu Z. , Consistency Measures for Hesitant Fuzzy Linguistic Preference Relations, IEEE Transactions on Fuzzy Systems 22: ((2014) ), 35–45. |
[31] | Gou X. , Liao H. , Xu Z. , Rui M. and Herrera F. , Group Decision Making with Double Hierarchy Hesitant Fuzzy Linguistic Preference Relations: Consistency based Measures, Index and Repairing Algorithms and Decision Model, Information Sciences 489: ((2019) ), 93–112. |
[32] | Gou X. , Xu Z. and Herrera F. , Consensus Reaching Process for Large-scale Group Decision Making with Double Hierarchy Hesitant Fuzzy Linguistic Preference Relations, Knowledge-Based Systems 157: ((2018) ), 20–33. |
[33] | Yu Y. , Yu Y. and Li B. , Effects of mindfulness and meaning in life on psychological distress in Chinese university students during the COVID-19 epidemic: A chained mediation model, Asian Journal of Psychiatry 53: ((2020) ), 102211. |
[34] | Zadeh L.A. , A fuzzy-algorithmic approach to the definition of complex or imprecise concepts, International Journal of Man-Machine Studies 8: ((1976) ), 249–291. |
[35] | Zadeh L.A. , Fuzzy logic-a personal perspective, Fuzzy Sets and Systems 281: ((2015) ), 4–20. |
[36] | Zhang H. , He X. and Mitri H. , Fuzzy comprehensive evaluation of virtual reality mine safety training system, Safety Science 120: ((2019) ), 341–351. |
[37] | Zhang P. and Feng G. , Application of fuzzy comprehensive evaluation to evaluate the effect of water flooding development, Journal of Petroleum Exploration and Production Technology 8: ((2018) ), 1–9. |
[38] | Zhang Y. , Wang R. , Huang P. , Wang X. and Wang S. , Risk evaluation of large-scale seawater desalination projects based on an integrated fuzzy comprehensive evaluation and analytic hierarchy process method, Desalination 478: ((2020) ), 114286. |
[39] | Zhou R. and Chan A. , Using a fuzzy comprehensive evaluation method to determine product usability: A proposed theoretical framework, Work 56: ((2017) ), 9–19. |