Development of sports industry under the influence of COVID-19 epidemic situation based on big data
Abstract
Based on big data, this paper studies the influence of new type of filling pneumonia on the development of sports industry. When selecting the typical economic indicators that reflect the development trend of sports industry, it is found that the data is huge according to the big industrial data, but the information that can be reflected is poor and complex. Therefore, it is necessary to process these big economic data in order to obtain the impact of new coronary pneumonia on the development of sports industry. This paper studies the feature selection algorithm of big data samples, so as to select typical economic indicators from many economic indicators of sports industry to reflect the development trend of sports industry. A deep learning algorithm based on feature selection of big data is proposed. Firstly, a feature selection framework for big data is constructed, and then data fusion and deep learning are carried out. Experiments show that the algorithm can solve the contradiction between large data and poor information. This method has a certain forward-looking, and has a certain reference value for the information discrimination of the development trend of sports industry.
1Introduction
The new corona virus pneumonia epidemic is a major public health emergency with the fastest spread in the world, the widest infection range, and the most difficult prevention and control. The epidemic situation will have a greater impact on the economic operation situation, industrial structure, industrial development mode, consumption mode, etc. As a sports industry that mainly relies on people to gather and consume and participate offline, it is also one of the more affected industries. The development of the sports industry must not only face this severe crisis, but also seek opportunities and turn them into opportunities. The sports industry should accurately predict the future development trend of national fitness and sports consumption, and seize favorable factors such as policy support, technological innovation, and compensatory consumption after the epidemic to achieve high-quality development.
Statistics on sports industry jointly released by the State Administration of sports and the National Bureau of statistics show that by the end of 2018, the total scale and added value of China’s sports industry have increased significantly [1]. The industrial added value accounted for 1.1% of GDP in that year, of which the total scale reached 2657.9 billion yuan, an increase of 20.9% over 2017; the industrial added value reached 1007.8 billion yuan, an increase of 29.0% over 2017 [2]. Although these data show that China’s sports trade industry is prosperous and active, sports service industry is expanding and upgrading, and the total scale and added value of sports industry have reached a new level. However, from the perspective of the whole sports industry, China’s sports industry is still emerging, still in the initial stage of development. Most sports enterprises belong to new and small and medium-sized micro enterprises, with weak financing ability, relatively tight capital chain and low ability to resist risks. In this situation, from the beginning of 2020, the epidemic broke out in Wuhan and spread rapidly to the whole country [3]. The country then took unprecedented prevention and control measures and launched a war against the epidemic. The epidemic inevitably causes short-term pressure and negative impact on sports industry, including sports competition and performance industry, fitness and leisure industry, sports tourism industry, ice and snow industry, sports goods manufacturing and sales industry and many other industries. The whole sports industry is in crisis.
2Influence of epidemic situation on Sports Industry
2.1Influence of the epidemic situation on sports competition performance industry
Sports competition and performance industry is an important part of sports industry and an important engine to promote the development of sports industry and build a healthy China. 2020 is supposed to be a big sports year. The Beijing Winter Olympic Games will enter the countdown to the second anniversary. The Tokyo Olympic Games will be held soon. Many international and domestic events have been prepared, just to start. However, the sudden epidemic has made all kinds of events fall into crisis, including Diving World Cup series, Asian Indoor Track and Field Championships, as well as qualification preliminary of some items of Tokyo Olympic Games, the first test of Beijing Winter Olympic Games, CSL, CBA and other major events forced to be held in other places, cancelled or postponed. In addition, many road running events have also been suspended or cancelled, which is expected to affect more than 100 million people. According to the 2018 China Marathon big data analysis report released by China Track and Field Association, there will be 1581 marathons and related sports events held in China in 2018, with an annual total consumption of 17.8 billion yuan, an annual industrial output of 74.6 billion yuan, and a total consumption of 28.8 billion yuan. According to the current epidemic situation, in 2020, the marathon The economic value of Marathon events will be greatly reduced.
2.2Influence of the epidemic on the fitness and leisure industry
The development of fitness and leisure industry is an inevitable requirement for the deep integration of national fitness and national health, and an important part of building a “healthy China". With the increasing diversified sports demand of the people, the sports consumption mode has gradually changed from physical consumption to participatory consumption, and the development of fitness and leisure industry has ushered in significant development opportunities. In October 2016, the general office of the State Council issued the guiding opinions on accelerating the development of the fitness and leisure industry, which pointed out that by 2025, the total scale of the fitness and leisure industry should reach 3 trillion yuan, basically forming a development pattern of fitness and leisure industry with complete categories, complete functions and reasonable layout. According to the annual performance law of the fitness and leisure industry, February and March are the peak business seasons. However, with the arrival of the epidemic situation, the fitness and leisure clubs with public fitness and outdoor sports as the main body are forced to close down, and their business income is greatly reduced. Some clubs have to face the problems of higher door rent, lower customer stickiness and professional brain drain, which are basically in The edge of collapse.
2.3Influence of the epidemic situation on sports tourism
Sports tourism is a comprehensive industry, with natural vulnerability and sensitivity, but also a strong dependence on the natural environment, social environment, vulnerable to infectious diseases, earthquakes and other emergencies. For example, during the SARS epidemic in 2003, people’s travel decreased significantly, the passenger flow decreased, the tourism industry was hit, and the income growth rate of the tourism industry decreased by 28%. Until the middle of August, the inbound passenger flow gradually returned to normal. Although the death rate of this epidemic is far lower than that of SARS, but the confirmed cases are far higher than that of SARS. In addition, many cities and roads are closed. The government’s prevention and control measures for the epidemic have been upgraded in an all-round way. The tourist attractions have been temporarily closed. It has become the social consensus during the epidemic prevention period that “no gathering and less going out". This has resulted in a significant reduction in the number of sports tourists, and the sports tourism industry has almost stagnated [4–6]. In order to contain the spread of the epidemic, on January 24, 2020, the Ministry of culture and tourism issued the tourism “shock” measures, which brought a sudden end to the youth Winter Camp, sports tourism boutique lines and other projects, causing serious losses to the sports tourism industry [7]. If the epidemic can be controlled in time by the end of May, it is expected to gradually improve in the third quarter, but it will take a long time to recover to the level before the outbreak.
2.4Influence of the epidemic on the manufacturing and sales of sports goods
China is a big country in sports goods manufacturing. In 2018, the sports manufacturing industry accounted for 33.7% of the added value of sports industry. In the production and sales of sports goods, the impact is also obvious. Under the epidemic situation, the sporting goods manufacturing industry is faced with problems such as limited logistics, delayed factory resumption, traffic control and so on, which has certain impact on production and transportation. It is expected that as the epidemic situation dissipates, the original production level will be restored soon. But for the sales of sports goods, the epidemic will undoubtedly bring a huge impact on the traditional offline sales, and the demand for sports consumption will be temporarily restrained because people’s participation in related sports activities is greatly reduced. For example, due to the impact of the epidemic, domestic brand Li Ning Sports goods company suspended all stores in the high epidemic area, and stores in other areas also chose to close or adjust their business hours. Anta sporting goods company announced that as of February 14, 2020, more than 60% of its stores in China are still closed, and it is expected to return to normal level in the second half of the year. According to reports, affected by the epidemic, the sporting goods industry as a whole is at a low ebb [8–10]. On the last two weeks of trading in January 2020, Li Ning, Anta, Tebu and other sporting goods stocks weakened, with a cumulative market value of 30 billion yuan evaporated, of which Anta alone lost more than 20 billion yuan [11].
3Feature selection method of typical economic big data based on deep learning algorithm
3.1Feature selection process
In machine learning algorithm, feature selection is to preprocess data. Preprocessing is to get the low dimensional representation of data from the high-dimensional data set, and to select the best feature subset that can represent the original data from the big data. Feature selection methods include filtering, embedding and encapsulation. The target object selected by the filtering method is the statistical characteristics of the data, and the features are selected by estimating the corresponding values of each feature. The premise of the embedding method is to know the model of the feature space, and then find the optimal subspace which can improve the system performance from the feature space. Then by analyzing whether there are known data in the sample, it can be divided into unsupervised learning and supervised learning. The encapsulation method uses the feature subspace to classify, and judges the features by analyzing the accuracy of classification. Because the selected feature subspace objects are different, the samples need to be trained many times. The general process of feature selection is shown in Fig. 1.
Fig. 1
General feature selection.

The essence of feature selection is the process of solving linear or nonlinear combinatorial optimization, also known as attribute reduction, which belongs to NP problem. Evaluation function and search method are the core of feature selection. As the main method to solve combinatorial optimization, search has heuristic search strategy, complete search strategy and random search strategy. As the evaluation function that affects the result of feature selection, it is the standard to measure the quality of feature set. And the evaluation function uses the criteria of error measure, consistency measure, distance measure and dependency measure as the result of feature selection.
3.2Shallow learning and deep learning
The process of extracting useful information from feature data through a series of calculations is called feature extraction, which goes through the process from artificial design to shallow learning and deep learning. Artificial design is used for feature extraction. The bottom features of the image are observed and analyzed, such as color features, texture features and local features, and then the effective information which is easy to be extracted is designed. The number of layers of shallow learning model is less, and the distinguish-ability of data is emphasized, mainly including Gaussian mixture model, sparse coding and support vector machine. Deep learning has two or more layers than shallow learning. In order to find the distribution characteristics and potential attributes of data, the combination of low-level features is transformed into high-level abstract features. Its core is to transform data from concrete to abstract, from low level to high level through nonlinear transformation, mainly including self coding and conventional neural network, restricted Boltzmann machine. The shallow learning model and deep learning model are shown in Fig. 2.
Fig. 2
Shallow learning and deep learning.
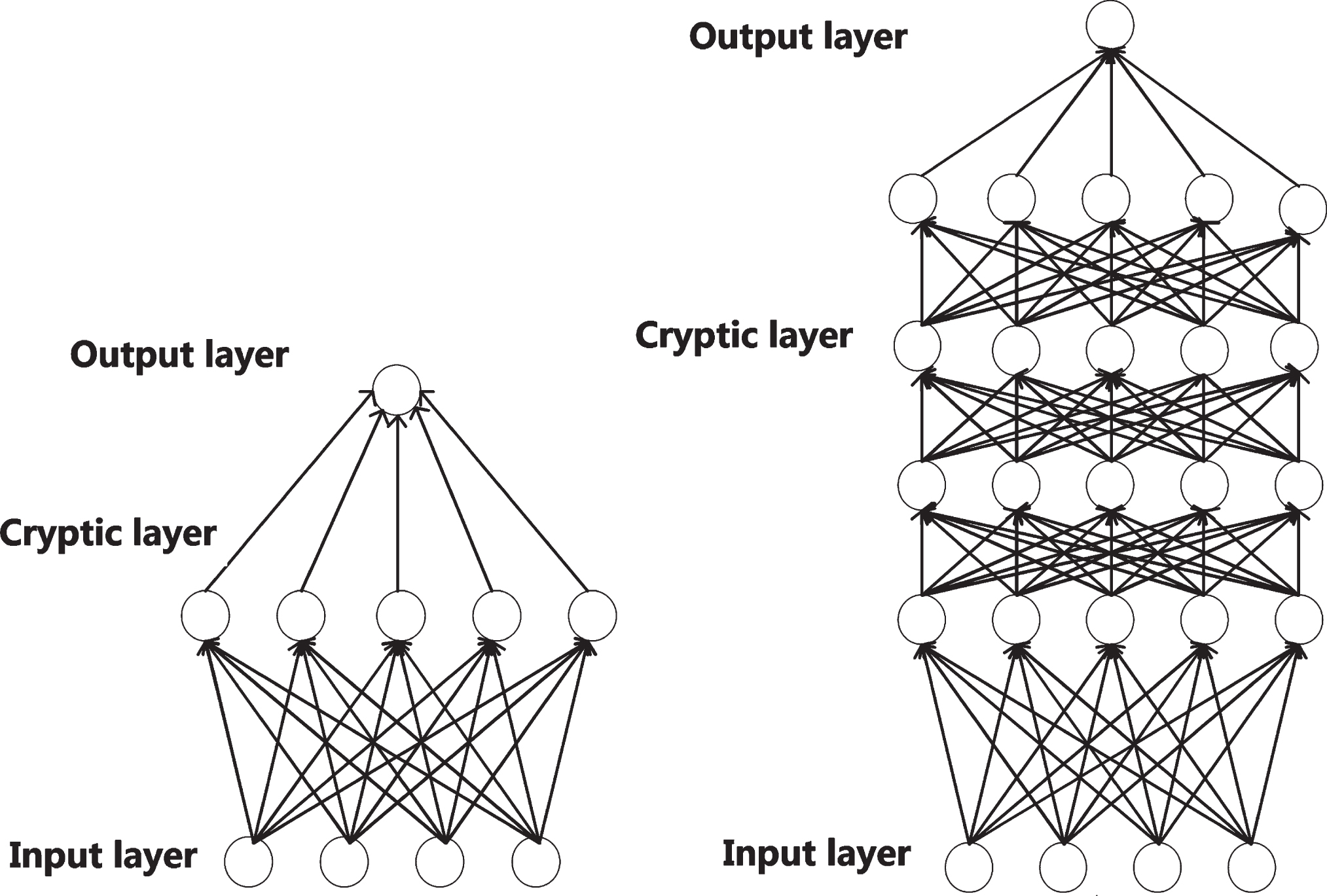
3.3Deep learning model
Deep learning uses hierarchical structure to build multiple hidden layers to process complex high-dimensional data [12]. The key technologies of deep learning are:
– Processing a large number of sample data and training complex hidden layer models put forward higher requirements for the hardware performance of the computer, and efficient hardware platform and parallel processing methods are essential.
– Deep learning relies on a large number of samples Line training, the size of the training set determines the applicability of the model, that is, generalization, so big data can avoid over fitting phenomenon and poor adaptability of generalization.
– Deep learning model has complex structure and training difficulty. In order to improve the learning effect, new optimization techniques are adopted, such as modifying linear unit to avoid over fitting, modifying nonlinear activation function to reduce computational complexity. In order to prevent the local minimum circulation, the unsupervised pre training should be done.
The typical deep learning models are depth confidence network, trestle automatic coder and multilayer convolutional neural network. A multilayer neural network is obtained by stacking several restricted Boltzmann machine models. In the pre training stage, the deep confidence network model can be trained unsupervised, without a lot of label data, which greatly improves the adaptability of the model. In the pre training process, by selecting the parameters, the convergence speed can be accelerated and the computational complexity can be reduced. The unsupervised training improves the effectiveness of the training parameters and avoids the calculation results from entering the local optimum rather than the global optimum. The deep self coding network is composed of the automatic encoder module, which has the input layer, the hidden layer and the output layer. The automatic encoder module is shown in Fig. 3.
Fig. 3
Automatic encoder module.
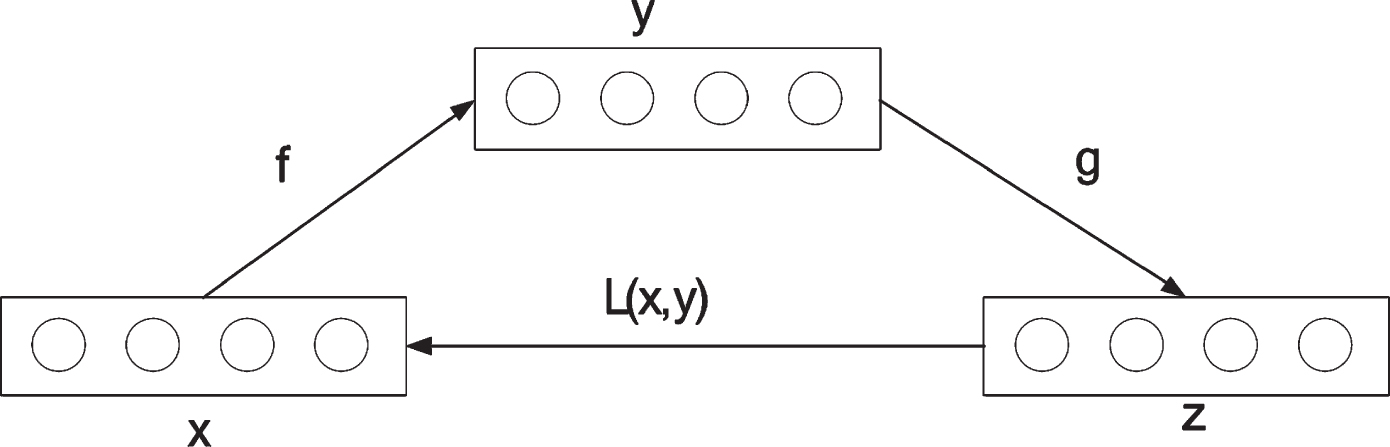
In the figure, f is the encoding function; X is the input layer data; y is the output layer data; G is the decoding function and l is the loss function. The mathematical relationship between them can be expressed as follows:
(1)
(2)
In formula (1) and (2), h is the hidden feature, SF is the nonlinear activation function; SG is the commonly used activation function; Z is the actual output after the transformation of the hidden feature; w(1), w(2) is the weight matrix; B(1), B(2) is the error vector.
The multi-layer convolution neural network model is composed of multiple convolution neural networks, which are composed of input layer, convolution layer, excitation layer, pooling layer and connection layer. The specific structure is shown in Fig. 4. Where C1, C2 is the convolution layer and S1, S2 are the pooling layer.
Fig. 4
Multilayer convolution neural network.
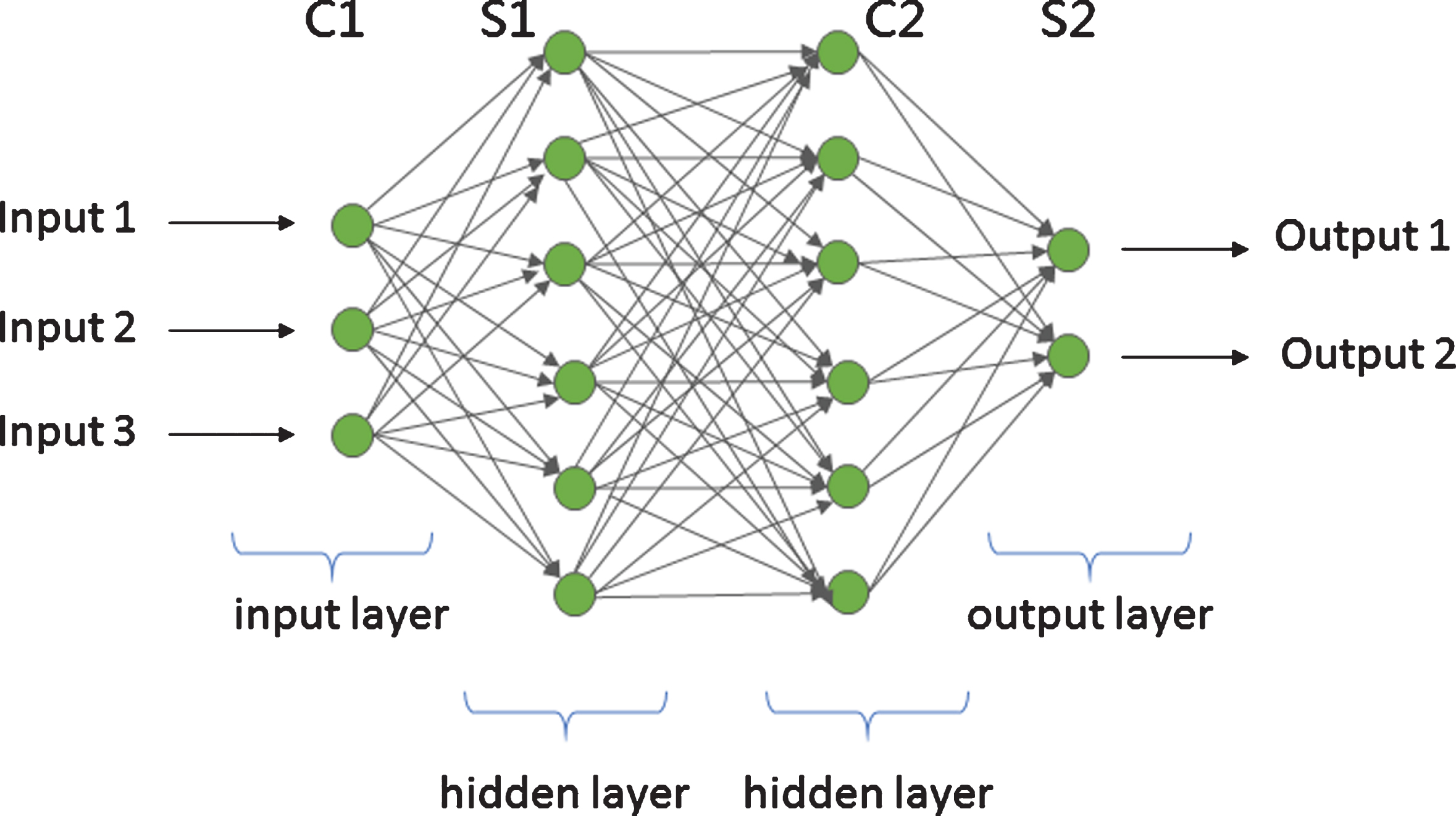
3.4Deep learning model based on big data
With the exponential growth of data scale and the improvement of people’s requirements for algorithm accuracy, deep learning involves a large number of parameters. Because of the complexity of deep learning model and the long training time, the internal iterative operation can not improve the speed of data calculation by parallel operation. When the training data can’t meet the requirements, the generalization ability of deep network is poor and over fitting is easy to occur. Therefore, in order to get the deep learning model under the feature selection of big data, the algorithm first improves the hardware platform, uses the multi-core GPU (graphics processor) to process the multi-layer convolutional neural network under the large-scale data, and then selects the appropriate filter. The size of the filter determines the number of linear equations. If a 16 * 16 = 256 size filter is used, the data with a data amount of 256 can be processed in parallel at the same time. On the other hand, the weight of the filter is affected by the gradient descent algorithm, and the reverse connection of the convolution layer cannot be obtained smoothly. Therefore, the influence of reverse error on the convolution should be considered. The reverse error is calculated as:
(3)
In formula (3),
Since the number of connections when calculating the reverse error is fixed, the number of connections from the upper layer (L + 1 layer) to the lower layer (L layer) has been determined. Therefore, the L + 1 layer is connected to the filter weight factor wi, and the L layer is pulled in the reverse direction, thereby obtaining back propagation error. The back propagation error calculation model is shown in Fig. 5.
Fig. 5
Back propagation error calculation model.
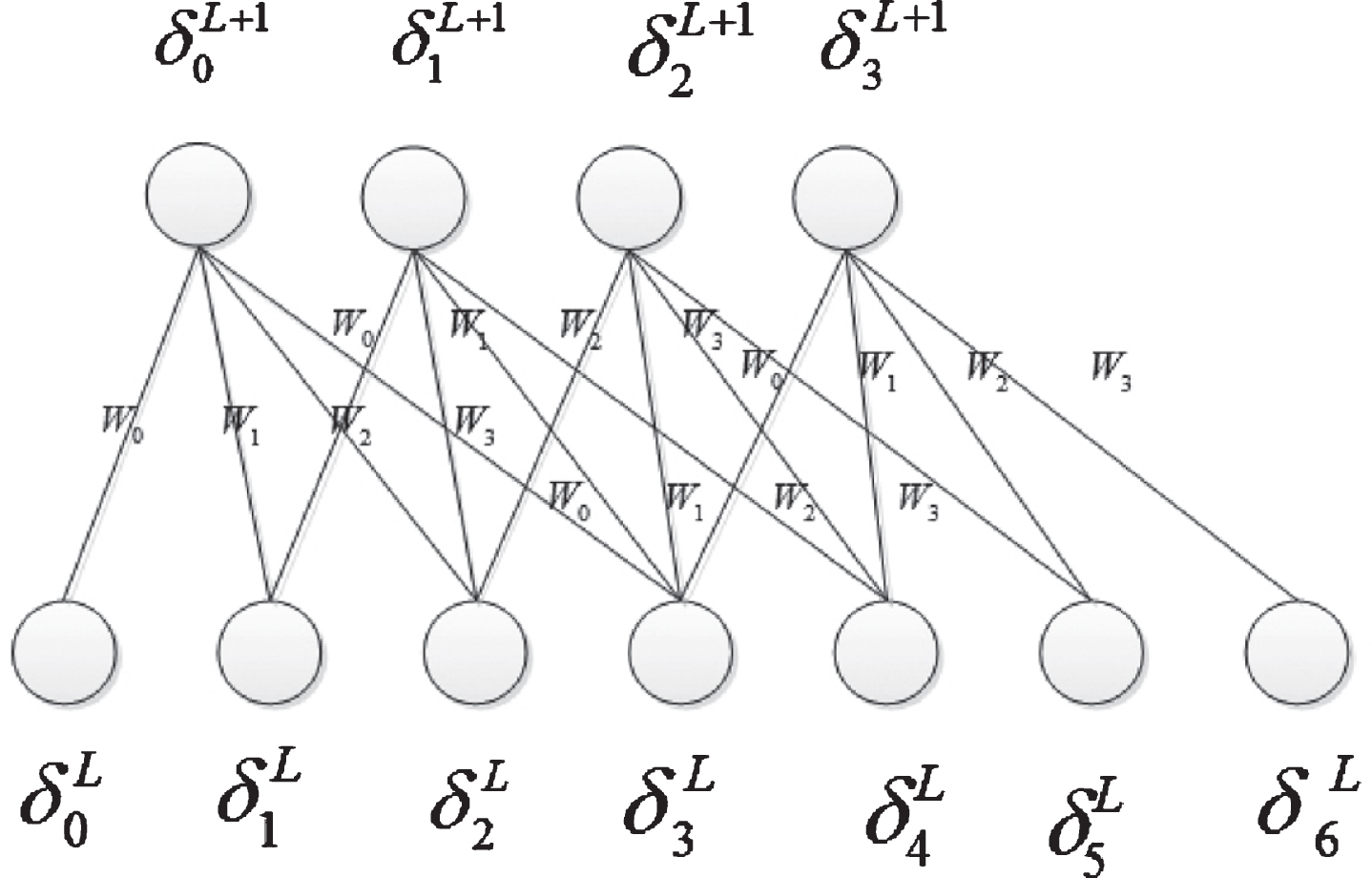
4Experiment result and analysis
4.1Classification experiment of typical economic data of different models
For the feature selection of large data samples, higher requirements are put forward for the hardware of the computer. Efficient hardware platform and parallel processing method are necessary for multi-layer deep learning. In the experiment, the hardware platform is built firstly, and the required server uses 8 cores and 16 threads. The graphics processor (GPU) adopts Tesla V100; CPU adopts Core i7 processor with main frequency of 3.8 GHz and memory of Kingston 16GB. The simulation environment is Matlab 7.0. In order to reflect the adaptability of the algorithm, the big data sample database uses two sample databases: standard economic parameter database and Yale industrial economic database. The standard economic parameter database consists of 40 categories, each of which has 16 economic indicators, which are distinguished by data weight, and the scale change is less than 0%. Yale industrial economy database contains 15 categories, each of which has 20 economic indicators, which are distinguished by the correlation degree of economic impact.
In order to verify the effectiveness of the algorithm, the algorithm in this paper is compared with the depth confidence network and the multi-layer trestle automatic coder in the economic index database. In order to verify the adaptability of the algorithm, it is necessary to mix the two economic index databases and then compare their classification accuracy, which is shown in Table 1. Through the analysis of experimental data, we can see that the deep learning algorithm based on big data feature selection has certain advantages in the classification effect of two databases, but the classification accuracy of the three models in the mixed database has a certain degree of decline, but the accuracy of this algorithm is still better than other algorithms, so it has certain adaptability.
Table 1
The styles defined in the IOSPressDoubleColumnJournal.dot file
Model | Database | Classification accuracy | Database | Classification accuracy | Database | Classification accuracy |
Deep confidence network | ORL | 89.56% | Yale | 90.14% | Hybrid database | 81.23% |
Multilayer trestle automatic coder | ORL | 85.23% | Yale | 86.31% | Hybrid database | 78.51% |
The model proposed in this paper | ORL | 94.15% | Yale | 94.23% | Hybrid database | 88.36% |
4.2Result analysis
Under the epidemic situation, the public is more and more aware of the importance of strengthening body and improving immunity, and people’s demand for strengthening body is stronger. The epidemic involves a large number of people, many patients in addition to improving their awareness of prevention, more importantly, they are aware of the importance of active health and active fitness. Without national health, there will be no well-off society in an all-round way, and national fitness is an important way to achieve national health. Many studies have shown that physical fitness is closely related to immunity. Regular physical exercise can improve the function of respiratory system and immune system. After all the major epidemics, the public’s awareness of health and sports participation will be improved. For example, after the “SARS” in 2003, there was a nationwide fitness boom, with all kinds of fitness venues full, badminton, basketball, fitness equipment and other sales increased significantly, and even some sports monopoly stores were out of stock. According to the routine needs of annual fitness, after the general Spring Festival, fitness participants have a higher demand for weight loss plasticity and sports fitness, while the outbreak of the epidemic forced most people to stay at home, “home” fitness is greatly affected, and participation in outdoor physical exercise and sports tourism has become a desire. After the epidemic, the concept of fitness of the public will also change from passive to active. Sports consumption scenarios will be more diverse. Sports demands such as physical exercise, physical fitness, physical pleasure and so on will be more vigorous, and the level of sports consumption will also be improved. Many industries such as fitness and leisure, sports goods manufacturing, sports venue service, sports tourism and so on will be favored. Sports enterprises should see the development direction of sports industry in the future, survive the “cold winter” of epidemic situation, grasp the opportunity of health industry development, expand and strengthen the main industry, and launch cross-border integration of sports and other industries.
The dividend of sports industry policy has been fully released, and the trend of online and offline integration of sports line is obvious. The relevant person in charge of the State Administration of market supervision pointed out: “all localities should formulate relief policies, and provide rent relief for individual businesses in combination with the actual situation, especially for individual businesses who rent administrative institutions and state-owned enterprise premises, encourage all localities to provide rent relief in combination with the actual situation. For renting other business houses or stalls, local governments shall, in combination with the actual situation, issue relevant preferential and incentive policies to encourage the owners to reduce the rent for the tenants “. The State General Administration of sports also held a meeting, which pointed out that we should do a good job in epidemic prevention and control as a whole, implement the requirements of orderly resumption of work and production, implement various subsidy policies and support policies, and help and promote sports enterprises to overcome difficulties. Local governments have also introduced policies to support the development of sports enterprises.
Under the epidemic situation, sports enterprises should not only enjoy the bonus of sports industry support policies, but also grasp the business opportunities, find the hot spots of sports consumption through online services, and implement the “self rescue” measures. For example, online sports platforms such as keep, super gorilla, yitrillion Wade, Lechi and other well-known fitness clubs have launched online live courses; Li Ning, Anta and other sports goods companies have promoted online sales through multiple emerging channels such as micro mall, pinduoduo, friend circle, vipshop and so on. After the epidemic, the policy dividend of sports industry will be fully released, and sports enterprises will further improve their online office or business capacity. The integration of offline and online has become an inevitable development trend.
The supply mode of sports industry has changed from extensive type to fine type. Under the epidemic situation, people “live” at home, big data, cloud computing, AI, Internet of things, VR, 5 g and other new generation information technologies are used in e-commerce, which makes the supply mode of sports industry change, from factor driven to innovation driven, from extensive development to fine development The sports industrial structure will be further optimized. In the short term, sports consumption demand is forced to shift from offline to online. The rapid development of online sales models, such as live fitness, online training, mobile games, and online sales of sporting goods, has given birth to the “house economy” and met the personalized sports consumption demand.
It can be seen that in the sports industry segmentation market, if we can find the market demand and give full play to our own advantages, we can turn crisis into opportunity. “Opinions on promoting national fitness and sports consumption to promote high-quality development of sports industry” proposed by the Sate Council clearly points out that new information technologies such as cloud computing and Internet of things should be used to promote the integrated application of event information release, sports venue activity booking, business service statistics, etc., to build e-commerce platform to provide sports consumption services, and to accelerate the promotion of big data, Internet, artificial intelligence and Sports real economy is deeply integrated. After the epidemic, with the implementation of innovation driven strategy in sports industry, the innovation driven development mode with new technology and new system as the core has become the focus. The essence of China’s shift to high-quality development in the new era requires that the development goal of sports industry must be changed from “high speed” to “high quality". In the future, more and more sports enterprises will use new technology, new business forms and new models to form new driving force for the development of sports industry.
4.3Strategies for the development of sports industry after the epidemic situation
In the short term, the epidemic has caused heavy damage to sports consumption, but the impact is temporary and phased, which will not change the long-term goal of China’s sports industry development, nor hinder the pace of promoting sports industry to become a pillar industry of the national economy. The development of sports consumption has an important value in the aspects of deepening the reform of sports field, stimulating the potential consumption demand of residents and improving the quality and efficiency of sports industry. Its internal logic is reflected in the transformation of effective demand for consumption, the promotion of the level of demand of a wide range of people, the development of potential consumption population, the improvement of consumption rationality expectation, and the expansion of consumption content space. The epidemic forced some sports enterprises to work and operate from offline to online, and also gave birth to the combination of online and offline sports consumption mode, promoting the development of digital and intelligent sports industry.
After the epidemic, local governments at all levels should actively introduce sports consumption policies, continue to implement national fitness action, carry out pilot projects to promote sports consumption, and constantly optimize the sports consumption environment. Through this epidemic, people fully realize the role of scientific and technological innovation. The information technology as the leading force of scientific and technological innovation has played an important role.
5Conclusion
Epidemic situation is not only a test of the management system and ability of sports industry, but also a test of the management level and response ability of sports enterprises. On the one hand, the huge amount of data brings dimension disaster and new challenge to computer hardware. On the other hand, big data provides a large number of training samples, which can solve the problem that the generalization ability of deep network is poor and it is easy to over fit. Therefore, the deep learning model based on the feature selection of big data has a certain practical value. Through experiments, it is found that the accuracy and adaptability of the deep computing model in economic data classification have been further improved. Through the accurate classification of economic data, the development trend of sports industry can be further studied, which has a certain reference value for the study of industrial economic development.
Acknowledgments
This paper is supported by Major Projects of Henan Higher Education Teaching Reform Research and Practice Project. (No. 2019SJGLX005).
References
[1] | Prasertpong R. and Siripitukdet M. , Generalizations of rough sets induced by binary relations approach in semigroups, Journal of Intelligent and Fuzzy Systems 36: (1) ((2019) ), 1–14. |
[2] | Philip Chen C.L. and Zhang C.Y. , Data-intensive applications, challenges, techniques and technologies: A survey on Big Data, Information Sciences 275: (11) ((2014) ), 314–347. |
[3] | Jagadish H.V. , Gehrke J. , Labrinidis A. , et al., Big Data and Its Technical Challenges, Communications of the Acm 57: (7) ((2014) ), 86, 88–94. |
[4] | Zaharia M. , Xin R.S. , Wendell P. , et al., Apache Spark: A Unified Engine for Big Data Processing, Communications of the Acm 59: (11) ((2016) ), 56–65. |
[5] | Kim G.-H. , Trimi S. and Chung J.-H. , Big-Data Applications in the Government Sector, Communications of the Acm 57: (3) ((2014) ), 78–85. |
[6] | Pijanowski B.C. , Tayyebi A. , Doucette J. , et al., A big data urban growth simulation at a national scale: Configuring the GIS and neural network based Land Transformation Model to run in a High Performance Computing (HPC) environment, Environmental Modelling & Software 51: ((2014) ), 250–268. |
[7] | Reed D.A. and Dongarra J. , Exascale Computing and Big Data, Communications of the ACM 58: (7) ((2015) ), 56–68. |
[8] | Yasrebi M. , Rafe V. , Parvin H. , et al., An efficient approach to state space management in model checking of complex software systems using machine learning techniques, Journal of Intelligent and Fuzzy Systems 38: (5) ((2019) ), 1–13. |
[9] | Bu Y. , Borkar V. , Xu G. , et al., A bloat-aware design for big data applications, ACM SIGPLAN Notices 48: (11) ((2013) ), 119–130. |
[10] | Kim G.H. , Trimi S. and Chung J.H. , Big Data Applications in the Government Sector: A Comparative Analysis among Leading Countries, Communications of the ACM 57: (3) ((2014) ), 78–85. |
[11] | Weichselbraun A. , Gindl S. and Scharl A. , Enriching semantic knowledge bases for opinion mining in big data applications, Knowledge-Based Systems 69: (oct.) ((2014) ), 78–85. |
[12] | Baccarelli E. , Cordeschi N. , Mei A. , et al., Energy-efficient Dynamic Traffic Offloading and Reconfiguration of Networked Datacenters for Big Data Stream Mobile Computing: Review, Challenges, and a Case Study. IEEE Network 30: (2) ((2016) ), 54–61. |