Building information modeling and affective occupancy evaluation: A scoping review
Abstract
Building Information Modeling (BIM) is a powerful process for creating and managing data throughout the life cycle of a building. Traditionally, measuring the well-being of building occupants has been addressed solely through objective physical variables such as temperature or relative air humidity. However, recent studies indicate that the built environment influences subjective aspects of human well-being. This article presents a scoping review to find information related to the use of BIM in the assessment of the mental and emotional state of inhabitants. A scoping review has been undertaken following the PRISMA-ScR guidelines by searching in Scopus, ACM, IEEE Xplore and PsycINFO databases. Fourteen articles meeting the inclusion criteria were found after the screening process, all of them published in the last decade, twelve in the last five years. Two ways of using BIM have been identified in relation to the subject matter of this review: (i) for visualization and monitoring of occupant well-being and (ii) for showing building design alternatives to future occupants. The included papers show that BIM has potential for assessing the mental and emotional state of building occupants. However, the results of these studies are still limited and much research in this area remains pending.
1.Introduction
With the recent development of information technologies, architects and engineers now have many options for understanding how people are feeling all the time. Biosensors, wireless devices and artificial intelligence make it possible to monitor people in real time, providing a valuable stream of information for developing products adapted to the needs of each individual [3]. Consequently, the design of the built environment is being pushed towards a new paradigm where concepts such as ambient intelligence (AmI) and user-centered design are highly present [18]. Under the AmI paradigm, people are surrounded by technology that allows the environment to respond and adapt to human presence [11]. However, the adaptability of a building in use is very limited as it is hardly possible to dynamically change building features like ceiling height and window size. Therefore, adapting a building to the needs of people must begin at the design stage; the feelings and behaviors of potential occupants of a building should guide designers in creating environments that maximize their well-being.
The characteristics of the built environment influence people’s behavior, health, productivity, and well-being [19]. An inadequate design of a building is likely to generate discomfort and dissatisfaction in residents. In this sense, post-occupancy evaluation (POE) is useful in detecting design errors [21]. POE has been defined as “an appraisal of the degree to which a designed setting satisfies and supports explicit and implicitly human needs and values of those for whom a building is designed” [13]. Such evaluation can be done by measuring physical variables such as light intensity, temperature, and sound intensity. In addition, the evaluation can be done by measuring subjective variables such as the degree of satisfaction or productivity of occupants [24]. The main inconvenience of POE is that it is performed when the building is already in use. Therefore, potential solutions to design errors will generally entail significant costs and inconveniences for the owners. It is also possible to conduct a pre-occupancy evaluation (PrOE) [22]. This basically consists of assessing the performance of the building prior to its construction, at an early stage of its design when changes to the project do not yet represent a substantial cost increase. PrOE solves the main drawback of a POE, but does not necessarily displace it. Both assessments complement each other. Indeed, the performance of a building changes over time and the best way to obtain information on a building in use and determine whether design changes are necessary is by means of POE (see Fig. 1).
Fig. 1.
Occupancy evaluation feedback loop. (a) POE. (b) PrOE.
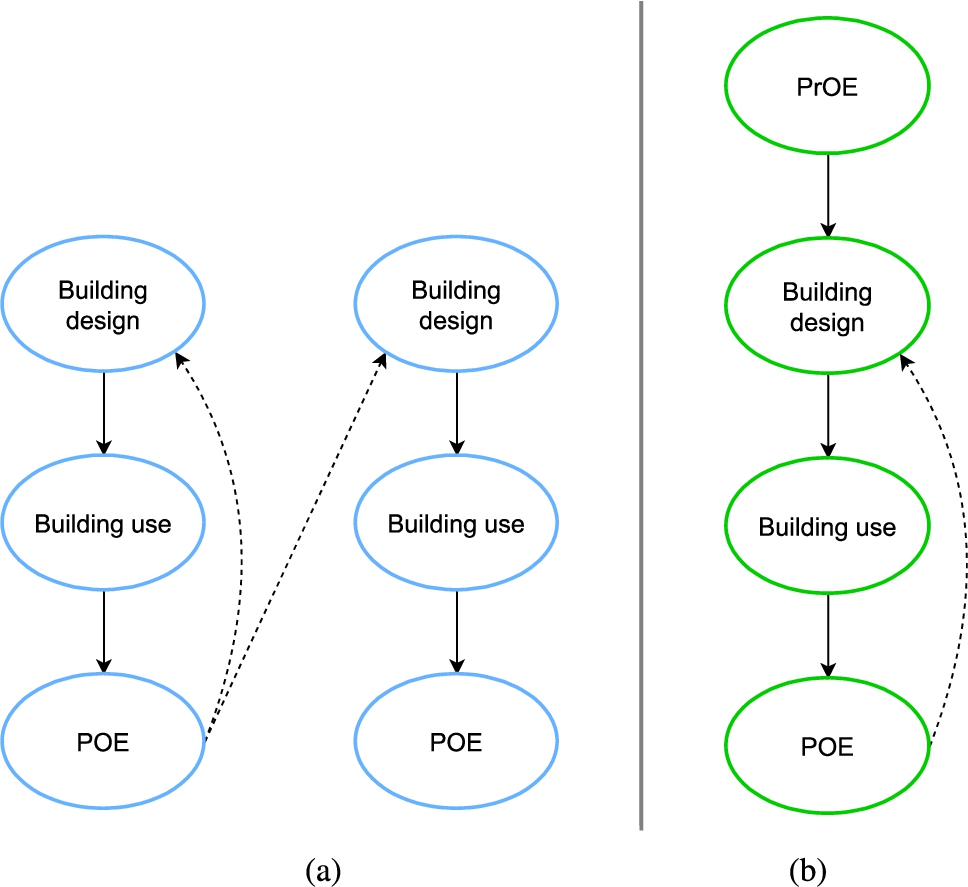
In a PrOE, instead of measuring the physical variables of the built environment in situ, a simulation is performed from a 3D model of the building using appropriate software [45]. Software simulations are used to determine, for example, what insulation material is most suitable to keep room temperatures in a range that is comfortable for people, what volume of outside air should be blown in to maintain air quality, or how many light fixtures should be installed to provide adequate light intensity for the activity in the building via augmented reality (AR) and virtual reality (VR). In this way, both design errors can be detected, and the degree of user welfare can be measured under different design alternatives [37].
Building Information Modeling (BIM) provides a very useful framework for both the POE and the PrOE. BIM may be defined as “a collaborative way for multidisciplinary information storing, sharing, exchanging, and managing throughout the entire building project life-cycle including planning, design, construction, operation, maintenance, and demolition phase” [40]. Virtually any building-related task is manageable with the aid of a BIM model, and occupancy evaluation is not an exception. A BIM model can be used in a POE to visualize and analyze the physical variables of the environment or the welfare levels of a building’s inhabitants; and it can also be used in a PrOE by exporting it to simulation software to analyze the behavior of physical variables or to a rendering software to show a photo-realistic representation of the building to potential customers. In addition, AmI-related technologies are increasingly present in the building sector, revolutionizing the way buildings interact with their occupants and improving their overall functionality and efficiency. Smart homes are a prominent application of AmI-related technologies, where intelligent systems combine to automate household tasks and improve the overall well-being of their occupants [25]. However, home automation, like any other design feature, can enhance the user experience, but can also have the opposite effect if not designed correctly. Buildings are becoming increasingly smarter, sometimes even becoming too smart when controlling variables that do not need any control [39]. Occupancy assessment is useful to determine the limits, as it allows to determine which variables or elements of a building contribute to the well-being of its inhabitants. It is equally essential to study how the design features themselves influence the affective state of the occupants of the building (see Fig. 2).
Fig. 2.
A few variables that may be used to measure the well-being of building occupants.
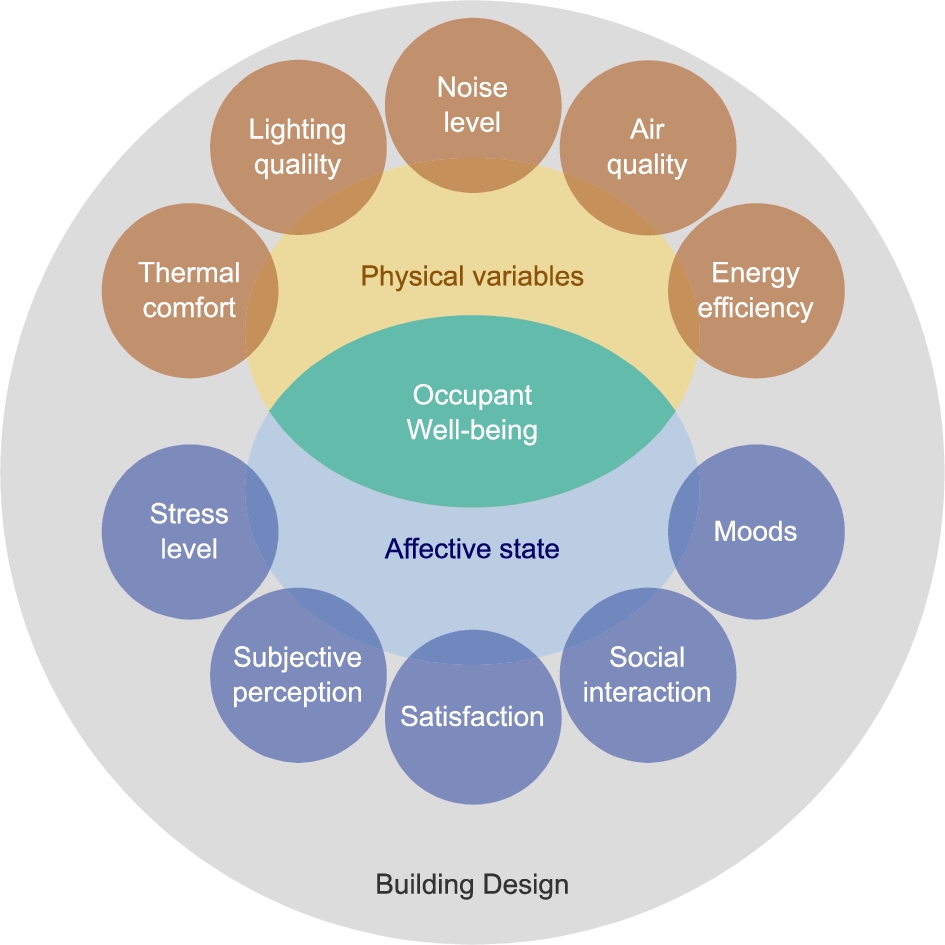
It is not clear what is available in the literature on the use of BIM to perform an occupancy assessment with the objective of determining the variables or elements of a building’s design that influence the mental and emotional state of its occupants. For this reason, a scoping review has been conducted in this paper with the objective of providing an overview of the literature on this topic, as well as to identify knowledge gaps and guide future research. This review aims to respond to the following research questions:
– What is the current state of research on the application of BIM as a framework for assessing how the design of buildings affects the mental and emotional state of their occupants?
– What scenarios, technologies, methods and techniques have been employed so far and what environmental and user variables have been measured in the studies?
2.Materials and methods
The reporting of this scoping review was prepared in accordance with the Preferred Reporting Items for Systematic Reviews and Meta-analysis Protocols Extension for Scoping Reviews (PRISMA-ScR) [42] guidelines. All articles written in English up to December 2022 addressing the use of BIM to perform an occupancy assessment with the objective of determining the building design variables that influence the mental and emotional state were considered eligible for inclusion. Multiple sources of information were selected to develop a search strategy as thorough as possible. Five databases were searched to identify all potentially relevant documents on the topic of this review. The databases consulted were Scopus, ACM Digital Library, IEEE Xplore, PubMed, and PsycINFO.
The search was performed on title, abstract and keywords. Two groups of terms were searched by combining them with Boolean operators: (“building information modeling” OR“building information modelling” OR“industry foundation classes” OR“revit” OR“archicad”) AND (“occupant satisfaction” OR “occupancy evaluation” OR“emotion” OR“emotional” OR“feeling” OR“sentiment” OR“sensation” OR“comfort” OR“affective” OR“well-being” OR“wellbeing” OR“wellness” OR“neurology” OR“neuroscience”)
The first group includes terms related to BIM, and the second group includes terms related to emotion and occupancy evaluation. As BIM-related terms, “industry foundation classes” was chosen because it is a popular open-source BIM standard, and “revit” and “archicad” were selected because they are the most widely used BIM programs. As for the terms in the second group, in addition to the synonyms of emotions and occupancy evaluation, the terms “comfort”, “well-being”, “wellbeing” and “wellness” were added because they define the feeling desired by the users of almost any building. The terms “neurology” and “neuroscience” were also included in this group.
All results were grouped in a bibliographic reference manager after the search and duplicates were eliminated. Identical references were automatically removed, and then similar references were manually examined to remove or merge duplicates. The title, abstract and keywords of all remaining articles were then examined and classified into three categories: Included, Excluded and Unsure. Articles labeled as Unsure were the ones for which, after reading the title, abstract, and keywords, it was not possible to determine whether they fitted the topic of the present review. Therefore, the full text of the articles labeled as Unsure was read to determine whether they should be included in the review.
After selecting the articles included in the review, the research team created a data sheet and exported the general data for each article (author, date of publication, title, keywords, type of document) from the bibliographic reference manager. Next, the following items were included in the data sheet: scenario; scenario type; display technology; evaluation method; environmental variables; metrics, sample size; relevant results; additional comments.
3.Results
After merging the results from the five databases (Scopus (n = 341); ACM (n = 13); IEEE Xplore (n = 17); PubMed (n = 5); PsycINFO (n = 2)), removing or merging duplicates, 349 articles were identified (see Fig. 3). Then, the records were screened by reviewing the title, abstract, and keywords. As a result, twenty-eight records were eliminated with missing full text, as well as 125 papers that were not related to the subject of the review and 169 papers that only evaluated physical variables of the built environment. This left us with twenty-seven articles that were screened in a second round of full-text review. Thirteen articles were excluded in this second round. Finally, fourteen articles were included in the review.
Fig. 3.
Review flow diagram.
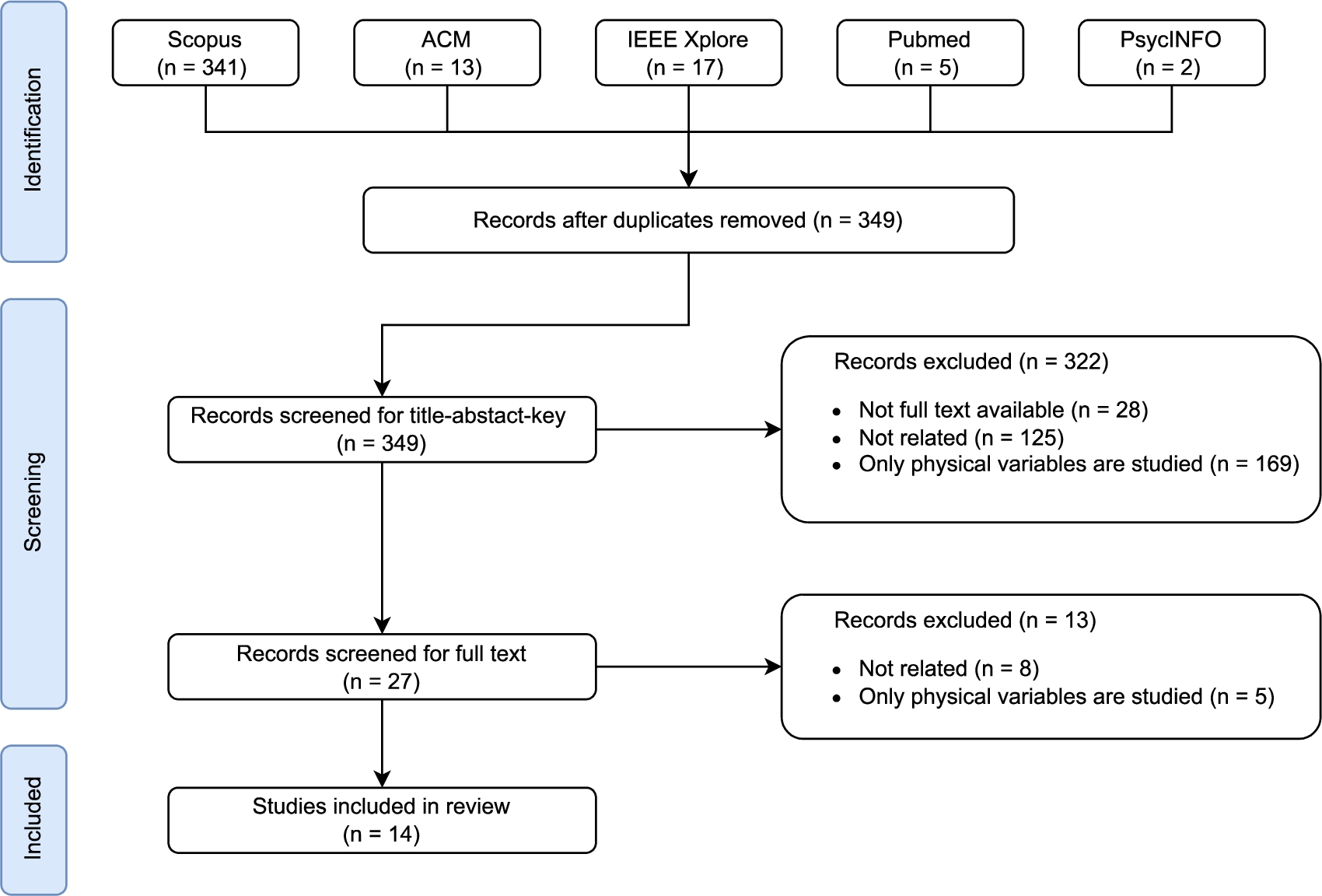
Of the articles included in the review, six are conference papers [7,16,17,32,34,48], seven are journal articles [1,5,9,36,41,44,47] and one is a book chapter [4]. The articles included in the review were published between the years 2012 and 2022. The most used terms in the keywords provided by the authors are BIM (building information modeling, building information modeling or BIM) and virtual reality, with six and five entries, respectively. Several selected articles, in addition to studying subjective or affective variables, also analyzed the evolution of different physical variables such as temperature and relative humidity and quantified the comfort and well-being of people as a function of these variables’ values. However, only data related to design variables that can influence the mental state of people and their subjective well-being were collected in this review. Table 1 presents the most relevant data, plotted by evidence source.
Table 1
Summary of individual sources of evidence
Ref | Scenario | Type | Evaluation method | Environmental variables | Metrics | Sample |
[32] | House | Virtual | Design | Geometry, materials, elements, properties and textures | NA | NA |
[7] | Train station | Virtual | Questionnaire and user location | Whole environment | Task performance and environment perception | 62 |
[5] | Office | Real | Questionnaire | Building design, building services and visual comfort | User satisfaction | 15 |
[44] | Office | Both | Questionnaire | Window aspect ratio, windows coating and lighting color temperature | Thermal sensation, sense of presence and user satisfaction | 50, 100 |
[1] | University | Real | Questionnaire | Cleanliness, space flexibility, accessibility and ergonomic furniture | User satisfaction | NR |
[47] | University | Real | Facial expression recognition | Whole environment | Emotional state | NA |
[41] | Office | Real | Questionnaire | Building design, building services and visual comfort | User satisfaction | 300 |
[4] | Dressing room | Real | Questionnaire | Lightning color and lightning position | User satisfaction | 80 |
[9] | Office | Virtual | Questionnaire and task performance | Ceiling height and type | Spatial perception, emotions, sense of presence and task metrics | 43 |
[17] | Sports facility | Virtual | Encephalography, questionnaire, eye tracking and user location | Whole environment | Excitement, Interest and Engagement | 8, 40 |
[34] | University | Virtual | Questionnaire | Whole environment | User satisfaction | NA |
[16] | Auditorium | Both | Questionnaire and eye tracking | Whole environment | Space perception and estimation, environment perception | 60 |
[48] | Office | Virtual | Task performance | Wall color | Task metrics | 43 |
[36] | Office | Virtual | Questionnaire | Whole environment | User satisfaction | NR |
Abbreviations: NA not applicable, NR not reported.
The ultimate purpose of all the articles reviewed is to enhance the well-being of the individuals using the assessed buildings. However, additional objectives are proposed in each article. Table 2 summarizes the key objectives of the articles selected in this review.
Table 2
Main objectives of the reviewed papers
Ref | Objective |
[32] | Creation of VR environments linked to BIM models, allowing non-technical users to make changes to the BIM model of a building from a virtual environment based on their preferences. |
[7] | Evaluation and comparison of different design options for a train station using BIM and VR. |
[5] | Development of a BIM-integrated post-occupancy evaluation system that collects the occupant feedback along with contextual information to enable spatio-temporal queries and visualization of collected feedback. |
[44] | Use of BIM and VR as a PrOE tool for measuring subjective occupant thermal response in office environments. |
[1] | Calculation of occupant comfort of an existing building using a probabilistic model that combines feedback from building users with spatial information. And the integration of comfort into a BIM model for visualization and to optimize decisions based on economic and environmental objectives. |
[47] | Use of BIM for monitoring and representation of environmental and emotion detection data obtained through wireless sensor networks based on the Internet of Things (IoT). |
[41] | Development of an occupant satisfaction measurement model to monitor the perceived performance of office buildings. And integration of satisfaction data with BIM to show post-occupancy performance. |
[4] | Identification of the most suitable type of lighting for the dressing rooms. And use of BIM software to visualize and compare the differences between different scenarios. |
[9] | Integration of BIM and VR for the evaluation of spatial perceptions of ceiling height and type in immersive virtual environments. |
[17] | Realization of PrOE using BIM and VR with the objective of measuring the emotional response of users from self-reported, neurophysiological and spatial data. |
[16] | Assessment of user experience in virtual reality environments created from BIM models. |
[48] | Evaluation of the potential of applying immersive virtual environments as PrOE tool to study the interior colors in office environments. |
[34] | Development of a BIM-based framework for the realization of PrOE in virtual environments. |
[36] | Development of a method for performing PrOE in BIM-based virtual environments that simulate human activities. |
3.1.Scenarios
Almost half of the articles [5,9,36,41,44,48] included in this review evaluated an office. The main objective of these articles, like the rest of the articles reviewed, was to increase the well-being of the building occupants. However, a particularity of these six articles is that increasing well-being was not only seen as an ultimate goal in itself, but also as a means of increasing worker productivity. And three of the papers reviewed evaluated a university building [1,34,47]. One major distinguishing feature of this type of building is the presence of numerous large rooms where various activities are held. Consequently, a large amount of information must be managed in their evaluation. On the other hand, the fitting rooms of three well-known clothing stores were studied [4]. Also, other types of buildings were evaluated, such as: a new section of a sports facility in Denmark [17], a medium-sized auditorium at the School of Architecture of the Royal Danish Academy of Fine Arts [16], a train station in Germany [7] or a home [32].
In terms of scenario type, six records relied solely on virtual scenarios created with BIM software as a PrOE tool [7,9,17,32,34,36,48]. Real scenarios alone were used in five records to conduct POE [1,4,5,41,47]. BIM models were primarily used for visualization and monitoring of users’ well-being in these articles. And, both types of scenarios were used in two articles to compare user behavior and response to check the validity of virtual scenarios as an occupancy assessment tool [16,44]. The technology for displaying the virtual models to the users was not reported in two papers [32,36], while it was head-mounted display (HMD) in the rest of the documents.
3.2.Evaluation methods
Questionnaires are the most commonly used method to assess well-being in both POE and PrOE [1,4,5,7,9,10,16,17,34,41,44]. In the works in which the authors have made the complete questionnaire available to the public, two types of questions have been identified: (i) asking the user to rate his or her sensations with respect to the environment, and (ii) asking the user to associate design elements and variables with adjectives.
As a complement to the questionnaires, eye-tracking was performed to determine the elements of the environment on which the user’s attention was focused [16,17]. In addition, the user’s location in the virtual environment and encephalography have been recorded [17]. On the other hand, reading comprehension tasks have been used together with questionnaires to assess the users [9]. Recently, participants were asked to move to different locations in the virtual environment and, based on their location, their way-finding behavior was assessed [7]. Assuming that people’s well-being is directly related to their productivity, it is considered that measuring their performance can be used to quantify well-being. Along these lines, proofreading tests have been used to evaluate participants in a study [48].
Another article took a different approach [32]. Instead of showing users an environment to assess their well-being in it, the authors asked them to create an environment they felt comfortable with. It has also been proposed to videotape participants and process the video using cloud-based facial expression recognition services [47].
3.3.Environmental variables
Six of the studies did not attempt to measure the influence of a specific variable on people’s well-being, but rather assessed the entire environment. The main environmental variables analyzed in each study are summarized in Table 1. Three groups of variables that are not detailed in Table 1 were examined in a couple of papers [5,41]. These variables included in each group are the following:
– Building design: Amount of space; Layout; Interior design; Furniture; Exterior design; Vibration conditions.
– Building services: Personal control; Usability of control devices; Facility management; Maintenance.
– Visual comfort: Day-lighting; Artificial lighting; Glare; Reflection; Visual privacy; View from window.
3.4.Metrics
The selected studies proposed different ways of quantifying people’s well-being. The most widely used with seven papers consists of making participants evaluate their degree of satisfaction or comfort with the environment and its variables [1,4,5,34,36,41,44], typically using a 5-point Likert scale.
In addition to the degree of satisfaction, the participant was asked to report his/her thermal sensation [44]. The emotional state of the participants was also measured in two approaches [9,47]. Two studies even assessed spatial perception. In one paper, participants were asked to rate the space shown to them with two semantic scales: (narrow-spacious) and (closed-open) [9]. On the other hand, locations and gaze fixation times were measured [16]. Participants were asked to describe the environment with adjectives, and three questions were asked to estimate the height, width, and depth of the room.
Participants were asked to rate their sense of presence in virtual environments in two studies [9,44]. Participants rated their sense of presence on a scale of −2 to 2 in the first study. This sensation was measured more comprehensively in the second paper using a questionnaire of 13 items divided into three groups, namely spatial presence, involvement, and sense of realism.
From electroencephalography signals, three metrics were obtained to assess the participants’ arousal, interest, and engagement [17]. Finally, the number of errors and time spent were the metrics used to evaluate task performance in two approaches [9,48].
3.5.Real vs virtual environment
Two papers compared a real environment with a virtual one [16,44]. A 360° image of a real environment was compared with a spherical image of a virtual environment mimicking the real one [44]. In both cases, the images were viewed using an HMD. According to the responses collected, 76% of the participants reported a preference for the image of the real environment over the virtual one. However, the same percentage rated positively the perceived sense of presence in the virtual environment. In the other article [16], participants viewed the real environment without any device, whereas the virtual environment was shown using an HMD. When comparing the eye-tracking data, relatively low differences in gaze fixation times and user behavior were found for the two environments. One potential cause may be the lack of peripheral vision when using the HMD.
3.6.Sample size
Overall, a small sample size was used in all experiments. Only two articles involved 100 or more participants [41,44]. Some of the reviewed articles did not incorporate an evaluation or the authors did not provide information on the sample size used to assess the proposed method. As for the rest of the articles, two of them divided the experiment into two parts [17,44]. In the first part, a small group of volunteers was used to test the method to detect possible failures, and in the second part, the models were evaluated with a larger group.
3.7.Findings
This subsection summarizes the most relevant findings from the reviewed articles. All the articles study a specific application case in the evaluation of a building or part of a building. Not all the methods presented in the articles were evaluated and some only presented a framework. Many of the results of the methods that were tested were evaluations of a specific environment where the influence of specific variables was not studied. Consequently, the results of these articles are not comparable or cannot be extrapolated. For this reason, only a few articles present remarkable findings (see Table 3).
Table 3
Most outstanding findings of the reviewed papers
Ref | Findings |
[44] | Thermal sensation varies across scenes in VR under same boundaries. |
[1] | BIM as a visualization tool makes it possible to detect the causes of occupant discomfort. |
[4] | Customers prefer headlights to overhead lights in dressing rooms. |
[9] | No significant differences in task performance are found when changing the type of ceiling. |
[16] | No major differences in eye-tracking data are found between a real environment and a virtual replica. |
[48] | Red color can improve productivity. |
4.Discussion
The adaptation of the built environment to human necessities involves measuring the sensations and feelings experienced when facing the different aspects of a building’s architecture. Clearly, the well-being of people is influenced by the physical variables of the environment. A person’s well-being is reduced in hot or cold weather, or by poor air quality. Most articles using BIM as an occupancy assessment tool consider that the comfort or well-being of building inhabitants depends on the physical variables of the environment like temperature or relative humidity.
However, several architectural elements of the building act as stimuli able to trigger an affective response. The geometry of the façade [29], the exposure to green walls [46] and lighting characteristics such as intensity and color temperature [35] are examples of environmental variables that influence people’s emotional state. In fact,“architectural design features involving light, color, complexity, viewing nature, olfaction, audition, and some forms of geometry, have been demonstrated to influence human behavior, health, happiness, and physiological function in myriad ways” [38]. Even the application of philosophical theories as Fengshui may induce positive emotions in building occupants [23]. Few articles, however, evaluate buildings from an affective point of view, focusing on the impact that the perception of the built environment has on people’s subjective well-being.
There are fourteen articles in this scoping review that made use of BIM for the assessment of the mental or emotional state of building occupants indexed up to September 2022. These articles reveal that there are two broad areas for this purpose. BIM can be used as a framework for monitoring and visualizing the degree of subjective well-being of the users of an existing building. Alternatively, it can be employed to perform a PrOE of a new building or also of an existing building where renovations will be undertaken. Within this second area, the most common way of using BIM is to show one or more versions of the 3D model of the building to the study participants. However, it is also possible to use a reverse approach. Instead of the designers (architects or engineers) setting all the design parameters, the control of these variables could be left to the potential occupants of the building, allowing them to create a design with which they are comfortable. In this way, the optimal building design features are determined for each individual user without requiring the designers to create numerous alternatives.
BIM has been used to evaluate offices, university buildings, train stations, homes, dressing rooms, auditoriums, and sports facilities. However, almost all the articles reviewed emphasize that BIM can evaluate practically any type of conceivable building. In addition, all the experiments performed were intended to evaluate particular application cases and were conducted with a relatively low sample size. Therefore, the results are not generalizable. Future work would recommend larger studies investigating the impact of specific design features in multiple scenarios.
Typically, architects and engineers do not ask future owners for feedback on their creations during the design phase, which may result in misdesigns [28]. VR allows designers to convey their ideas and get quick input from users. Therefore, VR is a feasible and effective tool for conducting PrOE. Immersive devices are especially attractive, as the sense of presence is increased and spatial cognition is more accurate [43]. The only virtual environment display technology reported in the studies reviewed is HMD. Although this technology offers a fairly immersive experience, it suffers from some limitations. The viewing angle is smaller than the human eye and it is not possible to use emotion recognition techniques such as facial expression recognition when wearing this device on the head. Therefore, other immersive VR technologies should be explored [15]. Besides HMD, one other immersive VR setup widely used in the construction industry is the Cave Automatic Virtual Environment (CAVE). Such system allows combining real and virtual elements as well as bringing multiple users into the same virtual environment [33].
In all cases involving virtual environments, the scenarios were shown to only one person at a time even though the buildings are shared-use spaces. Only one article included virtual people in the simulation [36]. However, testing shared-use spaces with only one person at a time is a limitation compared to real environments. Consideration should be given to introducing multiple users at the same time into the virtual environment through avatars. The building visualization system evolves towards the concept of metaverse by integrating avatars. Now, interaction between different people is made possible within the virtual environment [20].
Questionnaires are the most used method to assess the well-being of subjects. Nonetheless, other methods have also been applied like the performance of tasks or devices such as the encephalogram and eye-tracking have been deployed. However, there are other neurophysiological devices and techniques that might be useful for this purpose [8], e.g., electrodermal activity (EDA), heart rate variability (HRV) and functional near-infrared spectroscopy (fNIRS). The information reported by such devices alone is not very useful. However, it can be helpful in combination with spatial, temporal and environmental data to know how a person feels at each instant in front of any building element. In addition, their combined data set can be used to analyze the relationships between any variable and the behaviors/mental states of subjects in the built environment [14]. In an occupancy assessment, the representation of biosensor data in space makes it possible to visualize what users feel in each area of the building, and where peaks of each emotion occur [6]. When there are a large number of variables and samples it is difficult to interpret the raw data. This is where machine learning algorithms become valuable, as they convert the raw data into easily interpretable features such as discrete emotions, the level of enjoyment, the level of arousal [26], and the level of comfort [31].
An important aspect that is sometimes not sufficiently considered is the dynamic nature of the built environment. Occupancy evaluation is typically used to determine the performance of a building at a given point in time. However, lifestyles, activities, and social forms change over the course of a person’s lifetime, which can result in a building design that was originally suitable no longer being appropriate. The behavior of the built environment should not be seen as something rigid to which people must adapt; current advances in information and communication technologies make it possible to create intelligent and resilient environments that meet people’s needs at all times by adapting to changes in the needs or habits of their occupants [30]. In this sense, smart homes are presented as a powerful solution for improving people’s well-being, providing both tangible and intangible benefits that go beyond conventional notions of comfort and convenience. For example, this technology can be used to enhance social interaction and improve interpersonal relationships, ultimately contributing to an increased sense of relational well-being [12]. Using sensors and data-driven technology, smart homes detect human activity and modify some aspects of the environment accordingly. However, these devices do not eliminate the need for PrOE, but rather increase it, since not only the environment itself must be assessed, but also the compatibility of the technology used with people and building structures [27].
The concepts of occupancy evaluation and smart home have many links and should complement each other. A smart home collects thousands of data about its environment and user behavior, which are sometimes underutilized because there are many architectural variables that cannot be dynamically changed. However, this data can be of great value in conducting an occupancy assessment to improve the design of the building. With this information, it is possible to perform an occupancy evaluation not only at a specific point in time, but also on an ongoing basis, which allows you to determine when the building is no longer meeting the needs of its occupants. Typically, information from a POE is not transferable from one building to another because it is highly dependent on the people and the activities they perform. But as smart homes become more widespread, the situation may change. When people living in a smart home move to a new smart home, the information collected in the old home could be transferred to the new one. By combining this information with user guidance, the new home can be personalized to meet the user’s needs from day one [2].
5.Conclusion
This scoping review has revealed a growing interest in the use of BIM as an occupancy assessment framework for determining the variables or elements of a building’s design that influence the mental and emotional state of its owners. However, it also reflects a lack of evidence on the topic addressed. Most of the works found in the initial search treated well-being in an occupancy evaluation as something objective and quantifiable based on the values of certain physical variables of the environment, leaving aside the affective aspects.
After the screening process, only fourteen articles were found that met the inclusion criteria. These articles used a small number of technologies, scenarios and evaluation methods. Despite these limitations, the selected articles show the potential of BIM as a tool for occupancy evaluation of both existing buildings and new construction or renovation projects. However, there is a need for more ambitious studies with more methods, variables and techniques evaluated.
In our opinion, this scoping review has captured the state of research on the topic raised. This article may provide insights for researchers and professionals interested in deepening the use of BIM in the assessment of subjective aspects of human well-being.
Acknowledgement
Grants PID2020-115220RB-C21 and EQC2019-006063-P funded by MCIN/AEI/10.13039/501100011033 and by “ERDF A way to make Europe”. Grant BES-2021-097834 funded by MCIN/AEI/10.13039/501100011033 and by “ESF Investing in your future”. Grant 2023-PRED-21291 funded by Universidad de Castilla-La Mancha and by “ESF Investing in your future”. Grant 2022-GRIN-34436 funded by Universidad de Castilla-La Mancha and by “ERDF A way of making Europe”. This research was also partially funded by CIBERSAM, Instituto de Salud Carlos III, Ministerio de Ciencia e Innovación and co-funded by “ERDF A way to make Europe”.
Conflict of interest
None to report.
References
[1] | H. Alavi, N. Forcada, R. Bortolini and D.J. Edwards, Enhancing occupants’ comfort through BIM-based probabilistic approach, Autom Constr 123: ((2021) ), 103528. doi:10.1016/j.autcon.2020.103528. |
[2] | S.M.M. Ali, J.C. Augusto, D. Windridge and E. Ward, A user-guided personalization methodology to facilitate new smart home occupancy, Universal Access in the Information Society ((2022) ). doi:10.1007/s10209-022-00883-x. |
[3] | E. Allameh, M. Heidari and B. De Vries, Living preference modeling of smart homes for different target groups, J Ambient Intell Smart Environ 10: (2) ((2018) ), 103–125. doi:10.3233/AIS-180484. |
[4] | R.M. Alsaleh, H.M. Yaghmour, S.A. Khorshed, E. Mushtaha and A. El Amaireh, The effect of shop lighting on customer behavior (dressing room), Adv Sci Technol Innov Syst (2020), 215–225. doi:10.1007/978-3-030-32922-8_21. |
[5] | D. Artan, E. Ergen, B. Kula and G. Guven, Rateworkspace: BIM integrated post-occupancy evaluation system for office buildings, J Inf Technol Constr 27: ((2022) ), 441–485. doi:10.36680/j.itcon.2022.022. |
[6] | P.A. Bacevice and A. Ducao, Use of biometric data and EEG to assess architectural quality of two office spaces: A pilot experiment, Intell Build Int 14: (4) ((2021) ), 433–454. doi:10.1080/17508975.2021.1921683. |
[7] | G. Bailey, O. Kammler, S. Schneider, E. Fuchkina and R. Weiser, User-centred performances in train station design an IVE-based user study of four design options, in: Proceedings 13th International Space Syntax Symposium, SSS 2022, R.E. de Koning and A. van Nes, eds, Western Norway University of Applied Sciences (HVL), (2022) . ISBN 9788293677673. |
[8] | Y. Borgianni and L. Maccioni, Review of the use of neurophysiological and biometric measures in experimental design research, Artif Intell Eng Des Anal Manuf 34: (2) ((2020) ), 248–285. doi:10.1017/S0890060420000062. |
[9] | S.H. Cha, C. Koo, T.W. Kim and T. Hong, Spatial perception of ceiling height and type variation in immersive virtual environments, Build Environ 163: ((2019) ), 106285. doi:10.1016/j.buildenv.2019.106285. |
[10] | L. Chen and J. Wang, Application research of virtual reality technology in green building design, in: Proceedings of the 2020 5th International Conference on Smart Grid and Electrical Automation, Institute of Electrical and Electronics Engineers, (2020) , pp. 249–253. doi:10.1109/ICSGEA51094.2020.00060. |
[11] | D.J. Cook, J.C. Augusto and V.R. Jakkula, Ambient intelligence: Technologies, applications, and opportunities, Pervasive Mob Comput 5: (4) ((2009) ), 277–298. doi:10.1016/j.pmcj.2009.04.001. |
[12] | R. Creaney, L. Reid and M. Currie, The contribution of healthcare smart homes to older peoples’ wellbeing: A new conceptual framework, Wellbeing, Space and Society 2: ((2021) ), 100031. doi:10.1016/j.wss.2021.100031. |
[13] | A. Friedmann, C. Zimring and E.H. Zube, Environmental Design Evaluation, Springer, (1978) . |
[14] | N. Gao, M. Marschall, J. Burry, S. Watkins and F.D. Salim, Understanding occupants’ behaviour, engagement, emotion, and comfort indoors with heterogeneous sensors and wearables, Sci Data 9(1) (2022). doi:10.1038/s41597-022-01347-w. |
[15] | P. Havig, J. McIntire and E. Geiselman, Virtual reality in a cave: Limitations and the need for HMDs? in: SPIE Proceedings, P.L. Marasco and P.R. Havig, eds, SPIE, (2011) , p. 804107. doi:10.1117/12.883855. |
[16] | A. Hermund, L.S. Klint and T.S. Bundgaard, BIM with VR for architectural simulations building information models in virtual reality as an architectural and urban design tool, in: Proceedings of the Annual International Conference on Architecture and Civil Engineering, A. M.S.T., ed., Global Science and Technology Forum, (2018) , pp. 378–385. doi:10.5176/2301-394X_ACE18.152. |
[17] | A. Hermund, L.S. Klint and T.S. Bundgaard, Neurological feedback from perception of architecture in virtual reality: A methodology study, in: Proceedings of the International Conference of Architectural Science Association, A. Agrawal and R. Gupta, eds, Architectural Science Association, (2019) , pp. 235–244. |
[18] | A. Heydarian, E. Pantazis, A. Wang, D. Gerber and B. Becerik-Gerber, Towards user centered building design: Identifying end-user lighting preferences via immersive virtual environments, Autom Constr 81: ((2017) ), 56–66. doi:10.1016/j.autcon.2017.05.003. |
[19] | J.L. Higuera-Trujillo, C.L. Millán, A.M. i Aviñó and J.-C. Rojas, Multisensory stress reduction: A neuro-architecture study of paediatric waiting rooms, Build Res Inf 48: (3) ((2019) ), 269–285. doi:10.1080/09613218.2019.1612228. |
[20] | H. Huang, X. Zeng, L. Zhao, C. Qiu, H. Wu and L. Fan, Fusion of building information modeling and blockchain for metaverse: A survey, IEEE Open J Comput Soc 3: ((2022) ), 195–207. doi:10.1109/OJCS.2022.3206494. |
[21] | X. Huang, G. Chen, C. Zhao, Y. Peng and W. Guo, Post occupancy evaluation of indoor environmental quality of sports buildings at hot and humid climate from the perspective of exercisers, Build Environ 226: ((2022) ), 109760. doi:10.1016/j.buildenv.2022.109760. |
[22] | N. Ildiri, H. Bazille, Y. Lou, K. Hinkelman, W.A. Gray and W. Zuo, Impact of WELL certification on occupant satisfaction and perceived health, well-being, and productivity: A multi-office pre- versus post-occupancy evaluation, Build Environ 224: ((2022) ), 109539. doi:10.1016/j.buildenv.2022.109539. |
[23] | Z. Jin and Y.-K. Juan, Is Fengshui a science or superstition? A new approach combining the physiological and psychological measurement of indoor environments, Build Environ 201: ((2021) ), 107992. doi:10.1016/j.buildenv.2021.107992. |
[24] | P. Li, T.M. Froese and G. Brager, Post-occupancy evaluation: State-of-the-art analysis and state-of-the-practice review, Build Environ 133: ((2018) ), 187–202. doi:10.1016/j.buildenv.2018.02.024. |
[25] | G. Lobaccaro, S. Carlucci and E. Löfström, A review of systems and technologies for smart homes and smart grids, Energies 9: (5) ((2016) ), 348. doi:10.3390/en9050348. |
[26] | E. Lozano-Monasor, M.T. López, F. Vigo-Bustos and A. Fernández-Caballero, Facial expression recognition in ageing adults: From lab to ambient assisted living, J Ambient Intell Humaniz Comput 8: (4) ((2017) ), 567–578. doi:10.1007/s12652-017-0464-x. |
[27] | C. Ma, O. Guerra-Santin and M. Mohammadi, Smart home modification design strategies for ageing in place: A systematic review, Journal of Housing and the Built Environment 37: (2) ((2021) ), 625–651. doi:10.1007/s10901-021-09888-z. |
[28] | S. Maslova and G. Burgess, Delivering human-centred housing: Understanding the role of post-occupancy evaluation and customer feedback in traditional and innovative social housebuilding in England, Constr Manag Econ (2022), 1–16. doi:10.1080/01446193.2022.2111694. |
[29] | L. Pastore and M. Andersen, The influence of façade and space design on building occupants’ indoor experience, J Build Eng 46: ((2022) ), 103663. doi:10.1016/j.jobe.2021.103663. |
[30] | M. Pillan and S. Colombo, Will smart homes improve our lives? A design perspective towards effective wellbeing at home, The Design Journal 20: (sup1) ((2017) ), S2580–S2591. doi:10.1080/14606925.2017.1352769. |
[31] | W.J.G. Romero-Rodriguez, R. Baltazar, J.M. Carpio Valadez, H. Puga, V. Zamudio, J.F. Mosiño and M.A. Sotelo-Figueroa, A novel model for optimization of intelligent multi-user visual comfort system based on soft-computing algorithms, J Ambient Intell Smart Environ 13: (2) ((2021) ), 95–116. doi:10.3233/AIS-210594. |
[32] | V. Rostamiasl and A. Jrade, Integrating building information modeling and virtual reality to facilitate the implementation of universal design for facilities at the conceptual stage, Lect Notes Civ Eng 251: ((2023) ), 651–662. doi:10.1007/978-981-19-1029-6_49. |
[33] | B. Schiavi, V. Havard, K. Beddiar and D. Baudry, BIM data flow architecture with AR/VR technologies: Use cases in architecture, engineering and construction, Autom Constr 134: ((2022) ), 104054. doi:10.1016/j.autcon.2021.104054. |
[34] | S. Schneider, S. Kuliga, R. Weiser, O. Kammler and E. Fuchkina, VREVAL – a BIM-based framework for user-centered evaluation of complex buildings in virtual environments, in: Proceedings of the International Conference on Education and Research in Computer Aided Architectural Design in Europe, B.S. Kepczynska-Walczak A., ed., Education and Research in Computer Aided Architectural Design in Europe, Vol. 2: , (2018) , pp. 833–842. |
[35] | J. Seo, A. Choi and M. Sung, Recommendation of indoor luminous environment for occupants using big data analysis based on machine learning, Build Environ 198: ((2021) ), 107835. doi:10.1016/j.buildenv.2021.107835. |
[36] | W. Shen, Q. Shen and Z. Xiaoling, A user pre-occupancy evaluation method for facilitating the designer-client communication, Facilities 30: (7) ((2012) ), 302–323. doi:10.1108/02632771211220103. |
[37] | S. Shin, S. Jeong, J. Lee, S.W. Hong and S. Jung, Pre-occupancy evaluation based on user behavior prediction in 3D virtual simulation, Autom Constr 74: ((2017) ), 55–65. doi:10.1016/j.autcon.2016.11.005. |
[38] | P. St-Jean, O.G. Clark and M. Jemtrud, A review of the effects of architectural stimuli on human psychology and physiology, Build Environ 219: ((2022) ), 109182. doi:10.1016/j.buildenv.2022.109182. |
[39] | N. Streitz, Beyond ‘smart-only’ cities: Redefining the ‘smart-everything’ paradigm, J Ambient Intell Humaniz Comput 10: (2) ((2018) ), 791–812. doi:10.1007/s12652-018-0824-1. |
[40] | L. Tang, C. Chen, S. Tang, Z. Wu and P. Trofimova, Building information modeling and building performance optimization, in: Encyclopedia of Sustainable Technologies, Elsevier, (2017) , pp. 311–320. doi:10.1016/B978-0-12-409548-9.10200-3. |
[41] | I. Tekce, E. Ergen and D. Artan, Structural equation model of occupant satisfaction for evaluating the performance of office buildings, Arab J Sci Eng 45: (10) ((2020) ), 8759–8784. doi:10.1007/s13369-020-04804-z. |
[42] | A.C. Tricco, E. Lillie, W. Zarin, K.K. O’Brien, H. Colquhoun, D. Levac, D. Moher, M.D.J. Peters, T. Horsley, L. Weeks, S. Hempel, E.A. Akl, C. Chang, J. McGowan, L. Stewart, L. Hartling, A. Aldcroft, M.G. Wilson, C. Garritty, S. Lewin, C.M. Godfrey, M.T. Macdonald, E.V. Langlois, K. Soares-Weiser, J. Moriarty, T. Clifford, Ö. Tunçalp and S.E. Straus, PRISMA extension for scoping reviews (PRISMA-ScR): Checklist and explanation, Annals of Internal Medicine 169: (7) ((2018) ), 467–473. doi:10.7326/M18-0850. |
[43] | K.C. Tseng and D.T.N. Giau, A feasibility study of using virtual reality as a pre-occupancy evaluation tool for the elderly, Autom Constr 134: ((2022) ), 104037. doi:10.1016/j.autcon.2021.104037. |
[44] | F. Vittori, I. Pigliautile and A.L. Pisello, Subjective thermal response driving indoor comfort perception: A novel experimental analysis coupling building information modelling and virtual reality, J Build Eng 41: ((2021) ), 102368. doi:10.1016/j.jobe.2021.102368. |
[45] | T. Wei and Y. Chen, Green building design based on BIM and value engineering, J Ambient Intell Humaniz Comput 11: (9) ((2019) ), 3699–3706. doi:10.1007/s12652-019-01556-z. |
[46] | S. Yeom, H. Kim, T. Hong, C. Ji and D.-E. Lee, Emotional impact, task performance and task load of green walls exposure in a virtual environment, Indoor Air 32(1) (2021). doi:10.1111/ina.12936. |
[47] | A. Zaballos, A. Briones, A. Massa, P. Centelles and V. Caballero, A smart campus’ digital twin for sustainable comfort monitoring, Sustainability 12: (21) ((2020) ), 1–33. doi:10.3390/su12219196. |
[48] | S. Zhang, J. Kim, S.-Y. Shih, C. Koo and S.H. Cha, Immersive virtual environment (IVE) as a potential tool for interior colour study in office environments, in: Proceedings of the 34th International Symposium on Automation and Robotics in Construction, International Association for Automation and Robotics in Construction, (2017) , pp. 396–400. |